- 1Department of Electrical, Electronics and Computer Engineering, University of Catania, Catania, Italy
- 2Department of Physics and Astronomy, University of Catania, and Sezione Istituto Nazionale di Fisica Nucleare (INFN) of Catania, Catania, Italy
In response to the Positive Energy District transition, this paper proposes an energy tool for the modeling of energy sharing configurations among buildings equipped with energy production systems and distributive storages. The model is targeted for urban planners and energy policymakers and gives insights into the role of buildings in fostering the achievement of net-zero energy balances in districts when virtual or physical peer-to-peer configurations are established in the area. A real urban district is considered as a case study and the energy performances are measured against properly defined Key Performance Indicators. Results confirm the strategic role played by energy sharing among buildings in achieving self-sufficient and carbon-neutral areas. In particular, the insertion of storages allows not only for higher self-sufficiency of the area (by facilitating the coupling of production and demand) but also for higher distribution rates among buildings. However, photovoltaic insertion and storages should be appropriately balanced since it has been observed that at increasing the number of production and storage systems, the distribution is reduced in favor of autonomy, thus limiting the usefulness of an interconnected local distribution grid.
Introduction
Built-up areas are responsible for a high level of energy consumption and consequent carbon emissions (United Nations Framework Convention on Climate Change, 2015). The integration of renewable energy production systems at the urban scale has proven to be a significant solution to target the EU's strategic objectives in terms of both energy efficiency and emissions reduction. In this sense, urban communities play a pivotal role in fostering the transition toward sustainability and climate neutrality. Beyond the widely acknowledged advantages of energy efficiency and carbon-neutrality, renewable sources have changed the way urban areas have been so far conceived. Indeed, the insertion of renewables implied, de facto, the rising of decentralized distribution configurations among buildings, able now to produce, self-consume, and distribute energy within their neighborhood (Parag and Sovacool, 2016). This emergent feature of the building sector paves the way for inclusive urbanization, in which the citizen's role of shaping sustainable and autonomous districts can no longer be neglected (Fichera et al., 2016a). Recently, the Strategic Energy Technology (SET) Plan, Action 3.2, stressed the need for planning the diffusion of Positive Energy Districts (PEDs), i.e., urban agglomerates with annual net-zero energy imports and net-zero emissions (European Commission, 2018). Among the peculiar characteristics of PEDs, “interaction and integration between buildings,” as well as “an integrative approach including technology, spatial, […] perspectives” are considered as fundamental pillars for strategic energy planning (European Commission, 2018). Therefore, the modeling of energy sharing among buildings, including physical or virtual distribution, peer-to-peer topologies, and infrastructure, is crucial to achieving a fruitful transition toward PEDs. Under these premises, any long-term urban energy strategy should build upon integrated energy models coupling local production, local distribution among buildings and local distributive energy storage as key interlinked characteristics for the achievement of sustainable urban energetic configurations.
Up to now, many studies and regulations have proposed energy modeling tools and energy efficiency measures targeted to the built environment and focusing on the building scale (Directive 2010/31/EU, 2010; Directive 2012/27/EU, 2012). Magrini et al. (2020) discussed the most recent European directives concerning a building chosen as a reference case for energy-independent urban areas. On the premises that newly built edifices should be nearly-zero energy buildings (Cellura et al., 2017) and that energy planning decisions should refer to the district level, Sougkakis et al. (2020) compared energy performances of new and retrofitted buildings of a Greek neighborhood highlighting the best combination in terms of installed technologies and financial incentives. Keiner et al. (2019) investigated several energy scenarios for the optimal covering of electrical, thermal, and mobility demands for the residential sector. Other studies enlarged the building-based perspective to comprehend residential blocks of up to 25 buildings. Among these, Dorneanu et al. (2019) proposed a mixed-integer linear programming model to optimize the design and operation of energy distribution systems integrated with heating/cooling networks and connected to the main grid. Soutullo et al. (2020) mapped the existing European living laboratories and pursued a statistical analysis to develop guidelines orienting the evaluation of suitable and energy-efficient urban configurations. An interesting application of energy flexibility at the building scale is represented by the review of Al Dakheel et al. (2020), who set guidelines for the implementation of retrofit measures in existing buildings.
Among the unquestionable merits of these studies, they contributed to the increased awareness of the need to develop energy actions focused on the specific features and characteristics of buildings, as well as on the use of renewable-based technologies for the net-zero target (Cellura et al., 2011). Nevertheless, despite highly detailed, some of these tools cannot be easily scaled to the district level due to the computational intensity that would inevitably derive from extending these features to a significant number of households. This is even more relevant if the chance for buildings to share the own produced energy is added to the analysis; in these cases the possible energy interactions that should be considered for proper modeling would exponentially increase at increasing the number of buildings. Therefore, the traditional building-based perspective needs to be enlarged to reach the district level (Fichera et al., 2016b; Eicker, 2019; Bossi et al., 2020). In this direction, Ali et al. (2018) proposed a GIS-based tool to inform urban planners for the definition of energy strategies aiming at reducing both energy consumption and emissions. For the optimal design of energy systems, Jing et al. (2019) proposed a hierarchical methodology constituted of an optimization model and a clustering technique.
As said, the diffusion of renewable sources within urban areas enhanced the autonomy of the built-up areas. However, to improve the competitiveness and the advantages deriving from self-produced energy, the mismatch between production and consumption needs to be solved. A solution in this sense consists of coupling energy storage to district energy systems (Thomsen and Overbye, 2016). Near-optimal and real-time operation of buildings with installed renewable systems and batteries can be achieved by implementing a non-linear programming model to obtain positive energy balances (Cortès et al., 2020), by optimal pricing strategies for economic viability in electricity markets (Lin and Wu, 2017), or by considering the optimal positing of storage within the grid (Korjani et al., 2018). On this topic, Sameti and Haghighat (2018) proposed a mixed-integer linear programming model for the optimal design of distributed thermal and electrical energy storage with an application for a district of seven residential buildings.
Overall, the aforementioned literature recognizes the important impact of storage in urban areas. Nonetheless, the insertion of renewable energy production systems coupled to storage still needs to be deepened from the distribution perspective. Indeed, the distributive behavior of storages is usually considered at the sole micro-grid level (Liu et al., 2019) and the debate on the role that buildings can play in energy sharing is still open to discussion.
To this aim, this work aims at contributing to the existing state-of-art by measuring to what extent energy distribution among buildings can represent a key characteristic in shaping positive energy districts. In this direction, an integrated tool is here proposed to model spatial, energetic, and technological features of future PEDs with particular attention to sharing configurations arising from energy distribution among buildings. The developed methodology is intended for urban planners and decision-makers, being it able to offer a comprehensive evaluation of urban districts in which energy sharing mechanisms are planned to be introduced. Going into the details, simulations run under the methodological framework of the agent-based theory, successfully implemented in literature for modeling interactions among parts, at both the building (Sun et al., 2018) and district-scale (Fichera et al., 2018). The output of the energy simulations is discussed against Key Performance Indicators (KPIs), useful metrics if aiming at evaluating the energy performances of buildings (Angelakoglou et al., 2020) and PEDs (Clemente et al., 2019). In particular, in this study, KPIs are formulated about the impact of local energy sharing on the achievement of a positive energy balance for the area.
That said, the novelties of this paper can be summarized as:
- Assessment of local energy sharing as a key feature for PEDs development;
- Coupling of local production and local distributive energy storage at the district level;
- Definition of an agent-based model able to highlight the high granularity of energy flows among interacting buildings;
- Definition of focused KPIs measuring to what extent local distribution may affect the energy performances of PEDs and formulated to be replicable for comparison among other studies.
The following of this paper is organized as follows: section “Methodology” presents the proposed methodological approach, section “District Modeling” describes the district chosen as a case study and section “Discussion” discusses the results. Conclusions on the main implications deriving from the output of the proposed methodology are reported in section “Conclusions”.
Methodology
The proposed methodology aims at supporting urban planners and energy policymakers toward the definition of proper action plans targeted for PEDs with enabled energy sharing among buildings equipped with local energy production systems and energy storage. The modeling procedure relies on the following main pillars:
- On-site energy production from renewables;
- Energy storages to account for the mismatch between production and consumption;
- Sharing distance among connected buildings within the spatial boundary.
The adopted methodological path is reported in Figure 1, where three main divisions can be identified: the input data section, the simulation toolbox, and the output section.
Input Data
The input section collects data focusing on the spatial, energetic, and technological aspects.
The first module implements the modeling boundaries of the chosen urban district, featured within the GIS environment. Geo-referenced building-specific information such as location, construction data, rooftop area, orientation, and shading factors, is determined. In addition, the maximum distance along which buildings share energy has to be decided to balance energy flows exchanged within the districts and between each building and the main grid. It is worth noting that the required specifications for spatial input data may vary for the chosen technology. In this paper, an application with PV panels coupled to EESs is studied, being PV a leading technology due to its scalability, fast installation, and low maintenance and due to the presence of incentives that favor its diffusion in buildings (Lovati et al., 2020). To the scope, specifications on rooftops' area and typology (span/flat roof), inclination, orientation, and shading are included as input data for the simulation tool.
Case-by-case, urban planners may decide to include other relevant aspects, for example, surface and occupied volume, number of inhabitants, buildings' age, wall stratigraphy, and building function, depending on the simulation needs.
The second step consists of the definition of electrical and thermal consumption profiles for each building, taking also into account the information deriving from the spatial module. Electrical and thermal profiles are available on a daily basis for each household. Then, hourly profiles have been calculated matching the available data with the typical electrical consumption per person derived from the Italian Regulatory Authority for Energy (2020), and electrical profiles from the study of Capozzoli et al. (2016), considered as general and replicable for the Mediterranean basin. In this paper, the sole electrical profile is included in the simulation, being electrical energy suitable for the bidirectional peer-to-peer mechanism.
The third module gives detail on both the installed energy production technology and the energy storage systems. In this study, PV panels are coupled with electrical energy storage (EES) to account for the fluctuations between production and demand. EESs operate in a distribution mode, i.e., stored electricity can be distributed to neighboring buildings. Input data for this section are power sizes, determined by matching data from the spatial module (rooftop available area, typology, orientation, inclination, and so on) and data from the energy module (electrical and, if modeled, thermal loads). Electrical production profiles at varying the installed PV sizes are calculated for the site solar irradiance. Data on specific PV power output, direction normal irradiation, global and diffuse horizontal irradiations, and optimum tilt of the modules and hourly profiles for each month are extracted from the Global Solar Atlas (2021) for the geographical coordinates of the area deriving from the GIS-based information included in the spatial module. Data on EESs are included in terms of storage technology, storage capacity, inverter power and size, state of charge, and depth of discharge. As suggested by Huld (2011), good results on the overall performances between PV panels and EES can be achieved by assuming a conversion ratio of 65% to include any system losses (cables, DC/AC converter, charging and discharging, shadows, and dust on the modules).
Model
The simulation tool is developed within the theoretical framework of agent-based models, which have proven to be particularly suitable to solve problems characterized by a significant amount of interactions among the constituting parts (Khan et al., 2019), as in the case of districts with enabled energy distribution among buildings (Fichera et al., 2020). Agents represent interacting buildings aiming at both fulfilling their demands and achieving a positive energy balance at the district level. Interactions among buildings depend on a spatial-energetic constraint built upon their relative geographical location and their energy balance. Going into the detail of the spatial issue, the stakeholder (urban planner, policy-maker) has to define the geographical distance d along which energy distribution is enabled. All feasible connections respecting the selected spatial distance are collected under the spatial matrix M:
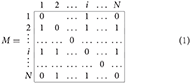
The matrix reports which buildings from the set i = 1, 2, …, i, …, N are connected on the ground of the chosen distance. Data are reported in a binary form, being aij the x connection between building i with spatial position (xi, yi) and building j with spatial position (xj, yj) in the space of matrix M:
It is worth noting that the diagonal of the matrix contains 0, having connections i−i no sense from the distribution perspective. The matrix represents the mathematical representation of the initial energy distribution scheme, from which energy balances will be calculated.
Referring to the energy aspect, energy balances between production and demand and balances at the EESs are calculated as:
The energy balance at each time t for each building i can be either positive or negative. A positive balance indicates that the production has covered the demand of the building and that residual energy can be either stored or shared among connected buildings. In this model, distribution has priority over storage and, in addition, the stored energy can be distributed to connected buildings if necessary. A negative balance indicates that the building's demand still needs to be covered: to this aim, energy may be supplied from other buildings with positive production balance or those with positive storage balance or, as the last option, from the main grid.
Electrical energy in EESs is stored at time t−1 and is distributed at time t. The balance at the EESs is obtained by considering the residual amount of produced energy after the distribution within the district. The stored electrical energy needs to respect a maximum and minimum storage level, due to the physical and technological characteristics of the chosen battery. Therefore, the following constraint:
Therefore, considering the introduced spatial matrix and the energy balances, distribution occurs if buildings are connected and respecting the energy flow direction from buildings with a positive balance to buildings with a negative balance. In addition, the energy sharing among buildings respects a priority criterion for which the produced (and stored) energy satisfies the energy need of the building owning the equipment, then the eventual excess is distributed to other requiring buildings and, if further energy is available it can be again stored (if possible) and finally released to the main grid. According to this criterion, buildings cannot receive energy if their energy balance is already positive to avoid utilitarian and profit-oriented behaviors at the expense of the district's needs.
Key Performance Indicators
Properly defined Key Performance Indicators describing energy distribution among buildings are derived from the output data section.
The performances of the modeled district are measured against three indicators. The first evaluates to what extent buildings share energy along the (physical or virtual) enabled connections built in the matrix M. In particular, it compares the number of connections used for the distribution in a 24 h horizon for the number of connections established in the matrix M:
The total useful connections Spconn in Equation (6) gives important insights on the spatial distance considered for the energy sharing balance among buildings since it calculates the percentage of connections effectively used for the exchange with respect to the initial allowed configuration. This indicator varies within the interval [0, 1], being Spconn = 0 indicative of a disconnected district and Spconn = 1 suggesting that all feasible connections (depending on the distance chosen by the energy planner) are effectively exploited.
The amount of energy distributed within the district as part of the production is measured referring to the KPI reported in the following:
The distributed energy Endistrict returns the rate of energy production shared among buildings and net of the rate used for self-consumption. The lower limit of this indicator, the distributed energy Endistrict = 0, indicates the case for which buildings do not exchange energy, either because they are not connected or because the produced energy has been used to cover the energy demand of installers.
Hourly energy balances at the district level are finally displayed to verify if the area is eligible for the transition toward the PED configuration.
District Modeling
The modeled district is located in Catania, Sicily, and counts 108 buildings, represented in Figure 2. Buildings are heterogeneous in terms of use, including residential households, a few single-houses, offices, and commercial edifices, distributed as in Figure 3. For each building, the roof surface and typology, volume, number of floors, surface exposition are known and used for the simulation. The overall available surface for PV installation is around 11,095 m2. On average, residential buildings have 3–4 floors. For residential buildings, the number of inhabitants has also been evaluated to eventually calibrate the hourly electrical consumption.
Detailed information about the electrical consumption profile of each building has been made available thanks to a dedicated mapping campaign. The electrical production profile of the installed PV panels is calculated by coupling the available data on rooftop areas and typologies for each building (spatial data from module 1) to the direct normal irradiation per square meter extracted from Global Solar Atlas (2021). The hourly electrical profile of the area for a typical summer day and the direct normal irradiation are reported in Figure 4.
Concerning the storage technology, lithium-ion batteries have been selected in this study for their diffusion at the building scale (Speidel and Braeunl, 2016). Technological and operational characteristics of batteries have been derived from the current literature (Shen et al., 2017; Hoang and Lee, 2018) as summarized in Table 1.
The storage capacity measures the amount of electricity that can be stored in the battery. State of Charge (SoC) and Depth of Discharge (DoD) are two operational parameters affecting the functioning of the battery. In particular, SoC measures the amount of electricity stored in the battery with respect to its capacity. DoD measures the usable storage capacity, i.e., rate of usable energy at a given time t with respect to the total capacity of the battery. Both are given as percentages, being defined as ratios between capacities.
Two modeling scenarios are discussed, each of them characterized by different percentages of PV diffusion in the territory. In detail, scenarios differ concerning the percentage of rooftops' area covered by PV panels. It is worth pointing that PV panels are randomly inserted in buildings and that the insertion respects the spatial constraints derived from the spatial input data section. Each scenario is then modeled with and without EESs to assess if EESs have a beneficial impact on both the distribution and the positive energy balance at the district level. A summary of the two scenarios, labeled as #Sc1 and #Sc2 and distinguished for the presence or not of EES, is reported in Table 2.
Finally, simulations run in NetLogo (Wilensky, 1999) for a 24 h cycle and respecting an energy priority rule regulating the energy distribution mechanisms within the district. Assuming that the spatial-energy criterion is satisfied, buildings are allowed to share the produced energy if the following conditions take place:
- Energy flows can be shared following the direction from a building with positive energy balance to a building with negative energy balance;
- Energy production is first used to satisfy the own energy needs;
- Exceeding production is firstly distributed and then stored;
- Eventual exceeding production (that can not be further stored or distributed) is sent to the main grid and, conversely, buildings with residual demand are supplied from the main grid.
Discussion
Results are discussed against the KPIs introduced in subsection Key Performance Indicators. As previously introduced, the achievement of a positive energy balance within a district cannot neglect the evaluation of the energy sharing impact. This aspect can be measured from different perspectives and under several scenarios, In this study, the district energy performances and the sharing impact are analyzed at varying the installed energy production on the territory and including or not EESs, as summarized in Table 2.
The values of the useful connections exploited among buildings, Spconn, are reported in Table 3 at varying the distance at which distribution may occur and for the identified scenarios.
As a remark, the useful connections Spconn indicator evaluates the rate of connections used for energy sharing with respect to the number of initial connections established on the ground of the allowed distance d. The first initial consideration arising from the graph regards the impact of distributive storages in terms of exchanged energy flows. In particular, when EES is not included in the simulation, the rate of connected buildings decreases and, consequently, energy distribution decreases as well. This is more evident at increasing the distance of connection, being higher distances bearer of higher sharing connections (virtual or physical, as specified). Precisely, this gap increases correspondently for each partial increase in the spatial distance among buildings. Otherwise, a general improvement regarding the sharing performances of the district can be noticed if EESs are coupled to PV panels, independently of the size of installed PV systems. This can be explained by the fact that even if energy production increases, the energy storage capacity of batteries remains unchanged, thus yielding no significant differences between the two scenarios. In addition, for buildings equipped with PV and EES systems, the impact of the distance is less significant, i.e., available energy connections have a good exploitation rate even among buildings in close spatial proximity. Overall, distances around 100 m permit to have a more connected district, and energy sharing among buildings is significant, with percentages around 80% of total and feasible connections crossed by energy flows during the day.
Moving forward, the distributed energy Endistrict indicator is now analyzed. Results are reported in Figure 5 at varying the distance of connection among buildings and for the two scenarios introduced in Table 2.
Again, the inclusion of EESs meaningfully affects the amount of energy distributed among buildings, yielding a higher share of energy distributed within the district. This consideration is valid independently from the modeled distance and scenario. Thus, the impact of EESs to reach any self-sufficient and autonomous targets for urban areas is significant not only from the spatial connection perspective (as reported in Table 3) but also considering the effective amount of energy production shared among buildings. As can be observed from Figure 5, indeed, an increase of circa 10–15% of total distributed energy is achieved within the territory, corresponding to higher participation of buildings in the energy sharing mechanisms and improving the energy efficiency of the area.
Surprisingly, the rate of energy shared among buildings is higher for #Sc1, i.e., for the scenario characterized by a lower energy production capacity. This can be explained by considering that, consistently with the results reported in Table 3 for fixed energy production, #Sc1 ensures for a more connected district, being the majority of established connections among building effectively crossed by energy flows; therefore, a higher amount of energy is distributed within the district.
Finally, the hourly electricity balances at the district level are shown in Figures 6, 7 for both scenarios. Considering both Figures, it emerges how the chosen area can be effectively candidates for planning the transition toward the PED configuration. A significant excess of electricity production can be recorded both in #Sc1 and #Sc2, despite the self-consumption and the distribution among buildings. This electricity excess can be exploited to balance nightly consumption and, generally, to fulfill any electrical demand not covered from the PV production. In addition, being the excess particularly significant, strategic planning can include the insertion of electric vehicles to the electricity needs of the area (Inturri et al., 2017).
Going into the detail of both Figures 6, 7, the significant impact of distributed storages within the area can be again recognized. As can be seen, EESs are beneficial to increase both the rate of self-sufficiency and energy sharing among buildings by passing the main grid and thus contributing toward the hourly net-zero balance, as recommended by the Directive (European Commission, 2018).
The decrease of the electricity supply from the main grid is much more evident in #Sc2 due to the higher diffusion of PV panels (and, therefore, higher production). Coherently, being higher the electricity production capacity of the area, the electricity excess is more significant than in #Sc1. In this scenario, the role of distributive EESs is less evident being more buildings able to reach self-sufficiency without requesting electricity from either the neighborhood or the main grid. Finally, it is worth pointing that, despite the insertion of PVs and EESs, a residual amount of electricity is still requested to the main grid: this is due to the fact that both Figures record the electricity balances of the area separately, and that amount of electricity from the main grid is going to balance the buildings' electricity demands not covered by PV production or by district sharing.
Conclusions
In this study, a model for the carbon-neutral and sustainable energy transition toward PEDs is proposed and targeted for the definition of strategic urban planning. Taking inspiration from the existing literature on the field of energy modeling and bearing in mind the guidelines of the SET-Plan Action 3.2, the proposed methodology includes the energy sharing among buildings and the presence of local storage as characterizing features of urban energy modeling. Spatial, energy, and technological input data are used to simulate energy exchange among buildings. Results are discussed against properly defined KPIs highlighting the spatial connection and energy distribution rates within the areas, as well as by measuring the hourly energy balance in the area. The developed simulation tool can be used for any district, being each step of the proposed methodology replicable at varying the input data.
The model has been tested in a district in South Italy and simulating the insertion of PV panels and Lithium-Ion batteries (the most diffused and scalable technological choice at the building level). Two main energy production scenarios are then simulated to account for different rates of PV penetration in the area and including or not electrical storage systems.
In particular, if considering the topological configuration of the distribution among buildings, it is worth noting that the insertion of EESs coupled to PV systems can be considered as beneficial to stress the decentralization of energy distribution and, consequently, the autonomy of the area. Into the details, allowed distance around 100 m can achieve a widely connected district. If evaluating the electricity flows exchanged into the area, again, EESs can guarantee higher rates of energy distribution among virtual or physically connected buildings, with an impact of circa 10–15% compared to the sole production scenario (without EESs). In addition, posing attention to the PED balance of the area, it can be effectively argued that there is the chance for the neighborhood to be designed as an eligible candidate for PED transition, being exceeding production sensitively higher than the overall demand. Careful scenarios' analyses can be dedicated to evaluating how to exploit the electricity excess. Strategic solutions in this sense could be directed to promote sustainable mobility for the considered area or to match nightly demand with diurnal production from PV systems.
From a broader perspective, results confirm the strategic role of connected and sharing buildings toward the net-zero or positive energy district configuration. In this direction, any urban action plan focusing on the definition of strategic measures favoring the insertion of renewable-based production systems coupled to energy storages should take the impact of energy distribution among buildings as crucial to increasing energy efficiency, carbon neutrality, and competitiveness of the area.
Further research should be focused on assessing the economic and environmental impact of buildings' sharing decisions on the area, as well as on the definition of the optimal energy production systems position for fostering energy exchanges.
Data Availability Statement
The original contributions presented in the study are included in the article/supplementary material, further inquiries can be directed to the corresponding author/s.
Author Contributions
All authors listed have made a substantial, direct and intellectual contribution to the work, and approved it for publication.
Funding
This research has been funded by the European Union and the Italian Ministry of Education, University and Research, under the project AIM—Attrazione e Mobilità Internazionale, in attuazione dell'Azione I.2 Mobilità dei Ricercatori dell'Asse I del PON R&I 2014–2020—Linea di Intervento 1, AIM1889410.
Conflict of Interest
The authors declare that the research was conducted in the absence of any commercial or financial relationships that could be construed as a potential conflict of interest.
References
Directive 2010/31/EU of the European Parliament and of the Council on the Energy Performance of Building (Recast). (2010).
Directive 2012/27/EU of the European Parliament and of the Council of 25 October 2012 on Energy Efficiency Amending Directives 2009/125/EC and 2010/30/EU and Repealing Directives 2004/8/EC and 2006/32/EC. (2012).
Al Dakheel, J., Del Pero, C., Aste, N., and Leonforte, N. (2020). Samrt buildings features and key performance indicators: a review. Sustain. Cities Soc. 61:102328. doi: 10.1016/j.scs.2020.102328
Ali, U., Shamsi, M. H., Hoare, C., and ODonnell, J. (2018). “GIS-based residential building energy modeling at district scale,” in Proceedings of BSO 2018: 4th Building Simulation and Optimization Conference (Cambridge).
Angelakoglou, K., Kourtzanidis, K., Giourka, P., Apostolopoulos, V., Nikolopoulos, N., and Kantorovich, J. (2020). From a comprehensive pool to a project-specific list of key performance indicators for monitoring the positive energy transition of smart cities - an experience-based approach. Smart Cities 3, 705–735. doi: 10.3390/smartcities3030036
Bossi, S., Gollner, C., and Theierling, S. (2020). Towards 100 positive energy districts in Europe: preliminary data analysis of 61 European cases. Energies 13:6083. doi: 10.3390/en13226083
Capozzoli, A., Corgnati, S. P., Di Nicoli, M. V., Fabi, V., Piscitelli, M. S., and Spigliantini, G. (2016). Report RdS/PAR2016/008, Attività di monitoraggio energetico e ambientale, diagnostica ed energy engagement degli utenti degli use case (in Italian). Italian National Agency for New Technologies, Energy and Sustainable Economic Development (ENEA).
Cellura, M., Campanella, L., Ciulla, G., Guarino, F., Brano, V., Cesarini, D., et al. (2011). “A net zero energy building in Italy: design studies to reach the net zero energy target,” in Proceedings of Building Simulation 2011: 12th Conference of International Building Performance Simulation Association (Sydney, NSW).
Cellura, M., Ciulla, G., Guarino, F., and Longo, S. (2017). Redesign of a rural building in a heriatge site in Italy: towards the net zero energy target. Buildings 7:68. doi: 10.3390/buildings7030068
Clemente, C., Civiero, P., and Cellurale, M. (2019). Solutions and services for smart sustainable districts: innovative key performance indicators to support transition. Int. J. Sustain. Energy Plan. Manag. 24, 95–106. doi: 10.5278/ijsepm.3350
Cortès, P., Auladell-Leòn, P., Munuzuri, J., and Onieva, L. (2020). Neat-optimal operation of the distributed energy resources in a smart microgrid district. J. Clean. Product. 252:119772. doi: 10.1016/j.jclepro.2019.119772
Dorneanu, B., Sidnell, T., Clarke, F., Mechleri, E., and Arellano-Garcia, H. (2019). A mixed-integer linear programming model for the optimal operation and design of residential neighbourhoods. IFAC Papers Online Conf. Paper Arch. 52, 934–939. doi: 10.1016/j.ifacol.2019.06.182
Eicker, U. (ed). (2019). “Introduction: the challenges of the urban energy transition,” in Urban Energy Systems for Low-Carbon Cities (Elsevier Inc.), 1–15. doi: 10.1016/B978-0-12-811553-4.09993-5
European Commission (2018). European Commission, SET-Plan ACTION 3.2 Implementation Plan. Europe to Become a Global Role Model in Integrated, Innovative Solutions for the Planning, Deployment, and Replication of Positive Energy Districts.
Fichera, A., Frasca, M., Palermo, V., and Volpe, R. (2016a). Application of the complex network theory in urban environments. A case study in Catania. Energy Proced. 101, 345–351. doi: 10.1016/j.egypro.2016.11.044
Fichera, A., Inturri, G., La Greca, P., and Palermo, V. (2016b). A model for mapping the energy consumption of buildings, transport and outdoor lighting of neighbourhoods. Cities 55, 49–60. doi: 10.1016/j.cities.2016.03.011
Fichera, A., Pluchino, A., and Volpe, R. (2018). A multi-layer agent-based model for the analysis of energy distribution networks in urban areas. Phys. A 508, 710–725. doi: 10.1016/j.physa.2018.05.124
Fichera, A., Pluchino, A., and Volpe, R. (2020). Modelling energy distribution in residential areas: a case study including energy storage systems in Catania, Southern Italy. Energies 13:3715. doi: 10.3390/en13143715
Global Solar Atlas (2021). Data obtained from the “Global Solar Atlas 2.0, a Free, Web-Based Application is Developed and Operated by the Company Solargis s.r.o. on behalf of the World Bank Group, utilizing Solargis data, with Funding Provided by the Energy Sector.
Hoang, K., and Lee, H. (2018). Accurate power sharing with balanced battery state of charge in distributed DC microgrid. IEEE Trans. Industr. Electron. 66, 1883–1893. doi: 10.1109/TIE.2018.2838107
Huld, T. (2011). “Estimating solar radiation and photovoltaic system performance, the PVGIS Approach,” in AFRETEP 1ST Regional Workshop (Kampala).
Inturri, G., Ignaccolo, M., Le Pira, M., Caprì, S., and Giuffrida, N. (2017). Influence of accessibility, land use and transport policies on the transport energy dependence of a city. Transport. Res. Proced. 25, 3273–3285. doi: 10.1016/j.trpro.2017.05.165
Italian Regulatory Authority for Energy Networks, and Environment. (2020). Delibera 602/2020/R/eel. ARERA.
Jing, R., Wang, M., Zhang, Z., Wang, X., Li, N., Shah, N., et al. (2019). Distributed or centralized? Designing district-level urban energy systems by a hierarchical approach considering demand uncertainties. Appl. Energy 252:113424. doi: 10.1016/j.apenergy.2019.113424
Keiner, D., Breyer, C., and Sterner, M. (2019). Cost and self-consumption optimised residential PV prosumer systems in Germany covering residential electricity, heat and mobility demand. Int. J. Sustain. Energy Plan. Manag. 21:35–58. doi: 10.5278/ijsepm.2019.21.4
Khan, M. W., Wang, J., Ma, M., Xiong, L., Pengham, L., and Wu, F. (2019). Optimal energy management and control aspects of distributed microgrid using multi-agent systems. Sustain. Cities Soc. 44, 855–870. doi: 10.1016/j.scs.2018.11.009
Korjani, S., Facchini, A., Mureddu, M., Caldarelli, G., and Damiano, A. (2018). Optimal positioning of storage systems in microgrids based on complex networks centrality measures. Sci. Rep. 8:16658. doi: 10.1038/s41598-018-35128-6
Lin, B., and Wu, W. (2017). Economic viability of battery energy storage and grid strategy: a special case of China electricity market. Energy 124, 423–434. doi: 10.1016/j.energy.2017.02.086
Liu, X., Yan, Z., and Wu, J. (2019). Optimal coordinated operation of a multi-energy community considering interactions between energy storage and conversion devices. Appl. Energy 248, 256–273. doi: 10.1016/j.apenergy.2019.04.106
Lovati, M., Zhang, X., Huang, P., Olsmats, C., and Maturi, L. (2020). Optimal simulation of three peer to peer (P2P) business models for individual PV prosumers in a local electricity market using agent-based modelling. Buildings 10:138. doi: 10.3390/buildings10080138
Magrini, A., Lentini, G., Cuman, S., Bodrato, A., and Marenco, L. (2020). From nearly zero energy buildings (NZEB) to positive energy buildings (PEB): The next challenge - The most recent European trends with some notes on the energy analysis of a forerunner PEB example. Develop. Built Environ. 3:100019. doi: 10.1016/j.dibe.2020.100019
Parag, Y., and Sovacool, B. K. (2016). Electricity market design for the prosumer era. Nat. Energy Perspect. 1:16032. doi: 10.1038/nenergy.2016.32
Sameti, M., and Haghighat, F. (2018). Integration of distributed energy storage into net-zero energy district systems: optimum design and operation. Energy 153, 575-591. doi: 10.1016/j.energy.2018.04.064
Shen, X., Shahidehpour, M., Han, Y., Zhu, S., and Zheng, J. (2017). Expansion planning of active distribution networks with centralzied and distributed energy storage systems. IEEE Trans. Sustain. Energy 8, 126–134. doi: 10.1109/TSTE.2016.2586027
Sougkakis, V., Lymperopoulos, K., Nikolopoulos, N., Margaritis, N., Giourka, P., and Angelakoglou, K. (2020). An investigation on the feasibility of near-zero and positive energy communities in the greek context. Smart Cities 3, 362–384. doi: 10.3390/smartcities3020019
Soutullo, S., Aelenei, L., Nielsen, P., Ferre, J., and Goncalves, H. (2020). Testing platforms as drivers for positive-energy living laboratories. Energies 13:5621. doi: 10.3390/en13215621
Speidel, S., and Braeunl, T. (2016.). Leaving the grid - the effect of combining home energy storage with renewable energy generation. Renew. Sustain. Energy Rev. 60, 1212–1224. doi: 10.1016/j.rser.2015.12.325
Sun, Y., Silva, E., Tian, W., Choudhary, R., and Leng, H. (2018). An integrated spatial analysis computer environment for urban-building energy in cities. Sustainability 10:4235. doi: 10.3390/su10114235
Thomsen, P., and Overbye, P. (2016). “Energy storage for district energy systems,” in Advanced District Heating and Cooling (DHC) Systems, ed R. Wiltshire (Ramboll Group, Elsevier Ltd.), 145–166. doi: 10.1016/B978-1-78242-374-4.00007-0
United Nations Framework Convention on Climate Change (2015). UNFCCC, 2015: Adoption of the Paris Agreement FCCC/CP/2015/L.9/Rev.1. United Nations Framework Convention on Climate Change.
Wilensky, U. (1999). NetLogo: Center for Connected Learning and COmputer-Based Modeling. Evanston, IL: Northwestern University. Available online at: http://ccl.northwestern.edu/netlogo
Keywords: positive energy districts, energy modeling, buildings, storage, renewable energy, agent-based models, energy sharing, energy distribution
Citation: Fichera A, Pluchino A and Volpe R (2021) Local Production and Storage in Positive Energy Districts: The Energy Sharing Perspective. Front. Sustain. Cities 3:690927. doi: 10.3389/frsc.2021.690927
Received: 04 April 2021; Accepted: 02 June 2021;
Published: 01 July 2021.
Edited by:
Francesco Guarino, University of Palermo, ItalyReviewed by:
Giovanni Tumminia, Istituto di Tecnologie Avanzate per l'Energia “Nicola Giordano” (ITAE), ItalyElisabetta Palumbo, RWTH Aachen University, Germany
Copyright © 2021 Fichera, Pluchino and Volpe. This is an open-access article distributed under the terms of the Creative Commons Attribution License (CC BY). The use, distribution or reproduction in other forums is permitted, provided the original author(s) and the copyright owner(s) are credited and that the original publication in this journal is cited, in accordance with accepted academic practice. No use, distribution or reproduction is permitted which does not comply with these terms.
*Correspondence: Rosaria Volpe, cm9zYXJpYS52b2xwZUB1bmljdC5pdA==
†These authors have contributed equally to this work