- Department of Earth Sciences, University of Memphis, Memphis, TN, United States
Urban studies related to previous pandemics and impacts on cities focused on vulnerable categories including poor and marginalized groups. We continue this tradition and analyze unemployment outcomes in a context of a multi-dimensional social disadvantage that is unfolding during the ongoing public health crisis. For this, we first propose an approach to identify communities by social disadvantage status captured by several key metrics. Second, we apply this methodology in the study of the effect of social disadvantage on unemployment during the COVID-19 and measure the COVID-19-related economic impact using the most recent data on unemployment. The study focuses upon vulnerable communities in in the southeastern US (Tennessee) with a concentration of high social vulnerability and rural communities. While all communities initially experienced the impact that was both sudden and severe, communities that had lower social disadvantage pre-COVID were much more likely to start resuming economic activities earlier than communities that were already vulnerable pre-COVID due to high social disadvantage with further implications upon community well-being. The impact of social disadvantage grew stronger post-COVID compared with the pre-pandemic period. In addition, we investigate worker characteristics associated with adverse labor market outcomes during the later stage of the current economic recession. We show that some socio-demographic groups have a systematically higher likelihood of being unemployed. Compared with the earlier stages, racial membership, poverty and loss of employment go hand in hand, while ethnic membership (Hispanics) and younger male workers are not associated with higher unemployment. Overall, the study contributes to a growing contemporaneous research on the consequences of the COVID-19 recession. Motivated by the lack of the research on the spatial aspect of the COVID-19-caused economic recession and its economic impacts upon the vulnerable communities during the later stages, we further contribute to the research gap.
Introduction
The earlier view of the global epidemic representing a systematic disadvantage that may equally affect economic activity of any individual, with any socioeconomic status or from any geographic location, was rejected while finding that certain factors may either increase or decrease people's vulnerability to the financial stress related to COVID-19 (Qian and Fan, 2020). In China's context, these factors include education, family income, membership in Communist Party, and state-sector employment. Besides health, other important side effects of COVID-19 impacting well-being include forced unemployment, loss of income and social isolation. The on-going coronavirus (COVID-19) pandemic has affected unemployment rates for every state, industry, and major demographic group in the United States (Falk et al., 2020), as well as globally (Radulescu et al., 2021). While vulnerable workers are both unemployed and underemployed during the COVID-19 era (Svicher and Di Fabio, 2021), many disadvantaged communities are disproportionally impacted so. Particularly disadvantaged were already low-wage industry segment workers and those working in retail sectors of the economy as they had a much greater chance of being laid off and a smaller likelihood to coming back to work (Bartik et al., 2020).
Studies have documented devastating impact of unemployment on whole communities (International Labor Organization, 2020), as strong as that on mental health of individuals (Paul and Moser, 2009; Wanberg, 2012). A major research question is to what extent does this unemployment crisis vary between communities? Exploring the role of social disadvantage during this crisis should yield research findings that can inform meaningful policy guidance at individual and systemic levels aiming to support and sustain both affected people and entire areas such as direct cash assistance of those identified communities.
To answer the above question and to study the effect of social disadvantage, we need to identify communities with high social disadvantage using consistent criteria. Accordingly, we developed an approach to identify communities with high social disadvantage captured by several key metrics (described below in Identification of Socially Disadvantaged Areas section). We applied it in this study of unemployment in Tennessee as a test case. TN is part of the southeastern United States which is both rural and socially vulnerable (Drakes et al., 2021).
The literature recognizes the complex interrelationship between employment, overall health, and well-being with unemployment impacting all-cause mortality. Regarding the employment impacts, the COVID-19 caused a drop in consumer demand across all industrial sectors resulting in economic recession and massive unemployment where not only hourly workers but salaried professionals lost their jobs (Petterson et al., 2020).
Some communities can absorb the impact of economic downturns due to more favorable economic and social factors protecting residents from adversity, yet other communities are witnessing the effect of rising unemployment in the time of COVID-19. Further, the link was found between incidence of the virus in the preceding month and employment. One recent study used a county-level analysis finding that counties with higher COVID-19 incidence suffered the greatest declines in jobs, however, various sectors responded differently to the effects of the virus incidence with largest employment declines observed in leisure and hospitality and other services (such as nail salons, beauty salons, barber shops), thus, control of virus transmission is important to avoid further local employment declines (Dalton, 2020).
Disadvantaged low-income workers are more likely to be concentrated in industries with lower average hourly wages such as leisure and hospitality and other service industries which do not allow remote working (Antipova, 2020a), however, telework-friendly industries such as finance and insurance have little to no changes in unemployment. Since the economic effects of COVID-19 in the United States might be felt for years to come (Weinstock, 2020), while the official US unemployment rate is estimated to increase during the near months (Forsythe et al., 2020), identifying communities where disadvantage exists is of upmost importance as it may mitigate the COVID-19-related adverse economic outcomes.
We use the official unemployment rate released monthly by the U.S. Bureau of Labor Statistics (BLS), also known as the U3 series. First, we review the recent studies on the topic, second, identify vulnerable communities (also termed here “social disadvantaged”), and third, statistically analyze the geographic distribution of unemployment rates across communities by their social disadvantage status.
Recent studies on the impact of COVID-19 on the labor market note that the most vulnerable segments of the active population including lower wage workers, those working in poor working conditions, women and young workers may be impacted the greatest (Radulescu et al., 2021). In this paper, we attempt to specifically consider how communities that were already socially disadvantaged before the onset of the pandemic, were affected by the COVID-19-caused economic downturn both during the early and later stages of the recession. Specifically, we study the economic impact of the outbreak on vulnerable communities using the official unemployment rates.
Geography plays a big role in preventing the spread of the COVID-19 due to the strong spatial variation of social injustice, infrastructure, and neighborhood cohesion contributing to incidence of COVID-19 (Vaz, 2021). Socio-economic characteristics are geographical in nature due to proximate things being more related while farther away things are more dissimilar (Tobler, 1970). We focus, first, on the delineation of areas with high social disadvantage whose residents already have an increased vulnerability to poor health due to greater psychosocial stress such as discrimination, unhealthy behaviors, and poorer health status (Hajat et al., 2015) which combined may lead to even greater COVID-19-related economic disparities. For this, we identify areas with high spatial concentration of social risk factors that indicate social disadvantage. Second, we compare unemployment rates between areas of high and low social disadvantage using Tennessee as the study area. Then, the study investigates what demographic factors contribute to adverse labor market conditions during the later stage of the current economic recession and place higher unemployment outcomes in a context of the public health crisis. Motivated by the lack of the research on the spatial aspect of the COVID-19-caused economic recession and its economic impacts upon the vulnerable communities, thus, we contribute to the research gap.
The paper is organized as follows. The first section, the Introduction, introduces the topic and explains the significance of the study, and reviews the recent studies on the employment impacts of the COVID-19-caused current recession, discusses the drivers of unemployment, and presents the study area. Materials and Methods, provides details on material and methods used in the study including an approach used to identify communities by social disadvantage status captured by several key metrics (see Identification of Socially Disadvantaged Areas section), Results and Discussion sections discuss the results and offers a discussion, respectively.
Literature Review
The COVID-19 pandemic caused economic damage affecting almost every state (and the District of Columbia) and every industry in the United States.
A recent report analyzed the national- and state-level patterns of unemployment rates using the Bureau of Labor Statistics (BLS) data finding factors impacting unemployment rates which include racial minority, industry sector type, full- and part-time work status, worker education level, age, and gender (Falk et al., 2020). Age, racial minority, and worker education level, age, industry sector type are more persisting than others. The younger, less educated, minority workers tend to have a limited access to credit and the informal resources (Bartik et al., 2020). At the same time, some racial groups disproportionally work jobs that cannot be done from home leading to higher risks of exposure to the COVID-19. Socially disadvantaged groups are more likely to have worse health outcomes compared to those experienced among more affluent populations groups (Antipova, 2020b). Minorities and lower-income groups often live in multiunit structures and in multigenerational households and have fewer options for protecting both their health and economic well-being (Gould and Wilson, 2020). Overall, socioeconomic attributes strongly impact life outcomes including jobs and health even in good times and under normal circumstances.
Regarding demographic characteristics of the unemployed, some demographic groups have systematically higher unemployment outcomes during the current recession than others. During the early stage, an analysis of the worker characteristics most associated with job loss found that workers of both older (over 65) and younger (16–25 years old) age, with no high school degree, who are Black, Asian, and Hispanic, and women were more likely to exit work in April 2020, while 26–37 year-old, college graduates, Whites, male, and married workers were less so (Bartik et al., 2020). Similar patterns in the distribution of job losses held on as the same attributes as those observed during the earlier periods of the pandemic persisted in May and June 2020 (Bartik et al., 2020).
A recent report analyzed the national- and state-level patterns of unemployment rate using the BLS data (Falk et al., 2020). Factors impacting national unemployment rates include racial minorities, economy sector type, full- and part-time status, worker education level, age, and gender. Within these groups, the major demographic groups that are affected more by the COVID-19 pandemic include young workers, women, low-educated workers, part-time workers, and racial minorities as reflected in a relatively higher unemployment rate among these groups both in April 2020 and October 2020. Among the listed categories, according to the BLS, teenaged workers (16–19 years), and racial and ethnic minorities have the highest unemployment rate (BLS, at www.bls.gov/cps/effects-of-the-coronavirus-covid-19-pandemic.htm). Even prior to the current health crisis, youth were already vulnerable within the workforce; young people across the globe are feeling particularly painful impacts from the recent massive job losses and increasing work uncertainty (Blustein et al., 2020). Besides younger age, another important unemployment factor earlier in the recession was female as women experienced a higher rate of unemployment than men in April 2020 because of their employment concentration in heavily affected sectors including restaurants, and to fulfill increased childcare responsibilities as many schools and kindergartens closed down essentially preventing female workers from being able to work (Alon et al., 2020). While the gender effect seems to be short-term as the gap between men and women has narrowed down by October 2020, younger age is a more lasting factor as teenagers are still experiencing a higher rate of unemployment compared to older workers. These conditions have improved, as teenaged men have the slightly higher unemployment rate than the rate for teenaged women (15.6 and 12.3%, respectively) in October 2020.
Even under normal circumstances, less educated workers have higher unemployment rates. During the COVID-19-caused contraction, education seems both an important and a longer-lasting factor with better educated workers having the lowest unemployment, with the gap persisting in October 2020 among all education groups. During both the peak of unemployment rate and later, joblessness rates for workers with less than a high school diploma were higher compared with workers from other education groups (21.2% in April and 9.8% in October, respectively). Workers with at least a Bachelor's degree were the least impacted, both as their unemployment rate peaked at 8.4% in April and dropped back to 4.2% in October 2020 (Falk et al., 2020).
At the start of the recession, Black, Asian, and White workers suffered sharp employment losses. However, Hispanics and Blacks experienced higher peak unemployment rates in April (18.9 and 16.7%), while non-Hispanic and White workers experienced 13.6 and 14.2% unemployment rates, respectively. Hispanics also experienced a steeper increase of 13.7% from February when their unemployment rate was just 5.2%. Racial and ethnic minorities continued having high unemployment rates in October 2020 with Black workers' unemployment of 10.8% compared with 6% and 7.6% among White and Asian workers, respectively (Falk et al., 2020). Interestingly, a peak unemployment rate occurred at different times for White, Black, and Asian workers, with White workers experiencing an earlier peak unemployment rate in April (at 14.2%), and it peaked for Black and Asian workers later on in May (at 14.5 and 16.7%, respectively). In October 2020, the gaps have narrowed down as an unemployment rate among Hispanic workers was at 8.5%, compared to 6.2% for non-Hispanics.
Low-wage service workers had experienced especially high decreases in employment compared with workers in other industries, both as the recession started and later. Within the affected industries, some workers had a higher chance to lose their jobs than others: for example, in services industries, low-wage workers suffered disproportionately large unemployment (Bartik et al., 2020; Cortes and Forsythe, 2020).
Changes in unemployment over short term may affect future employment patterns as economic activities continue to resume however with the virus is still present in the United States (Dalton, 2020). Driven by the identified disparities in job outcomes among major demographic groups occurring soon after the recession began, we investigate worker characteristics associated with adverse labor market outcomes during the later stage of the current economic recession and analyze unemployment outcomes in a context of the social disadvantage that continues to play out during the ongoing public health crisis.
This study analyzes unemployment across counties in Tennessee which is part of the US southeastern area with clusters of high social vulnerability and rural areas (Drakes et al., 2021), and which is among the U.S. states with the highest rates of cases per capita (as of this writing, Tennessee is the 6th state using this metric as of mid-June 2021), further adding to vulnerability. Specifically, we analyze unemployment outcomes in a context of a multi-dimensional social disadvantage that continues to play out during the ongoing public health crisis. Study identifies communities by social disadvantage status captured by several key metrics and examines the geographic distribution of labor underutilization across communities by social disadvantage status.
Materials and Methods
Study Area
The study analyzes unemployment rates across counties in Tennessee (Figure 1). Tennessee is a part of the southeastern United States where concentration of both rural and socially vulnerable areas has been found (Drakes et al., 2021). Thus, the study area represents a natural test case to study the impacts of social disadvantage. It concentrates areas which are more likely to rely on federal assistance as such communities are slower to recover from adversity compared with communities with better resources and which can recover more readily. As of this writing, Tennessee is among the US states with the highest rate of COVID-19 cases (the 6th highest, with 127,934 total cases per 1 million people on July 16, 2021) (Worldometers, 2021), while <38.3% of population is fully vaccinated (compared with almost 49% nationwide as of July 16, 2021), further adding to vulnerability. Over the year, from November 2019 to November 2020, the state had a statistically significant employment change: from 3,143,200 to 3,030,400 jobs (a decline of 112,800 jobs, or over-the-year change of −3.6%) ( BLS, 2020). The state's poverty of 12.1% which represents 813,000 people, is higher than the nationwide rate of 11.5% for 2017–2019 (Census Bureau, 2020). The earlier estimates of poverty by the U.S. Census Bureau which used the Current Population Survey, and 2017–2019 Annual Social and Economic Supplements, reported 847,000 individuals, or 12.7 % of people were in poverty in Tennessee using 3-year average over 2016–2018 compared with the 12.3% nationwide (U.S. Census Bureau, 2019. The Supplemental Poverty Measure: 2018). Shown in Figure 1, the unemployment rates in the state of Tennessee at 7.4%, seasonally adjusted, as of October 2020 is higher than the national average of 6.9% which measures all industries and all occupations for population 16 years and over (Bureau of Labor Statistics, LAUS). The peak of unemployment in the state was in April 2020 when it reached 15.5% (compared with 3.3% in November 2019) (Figure 1).
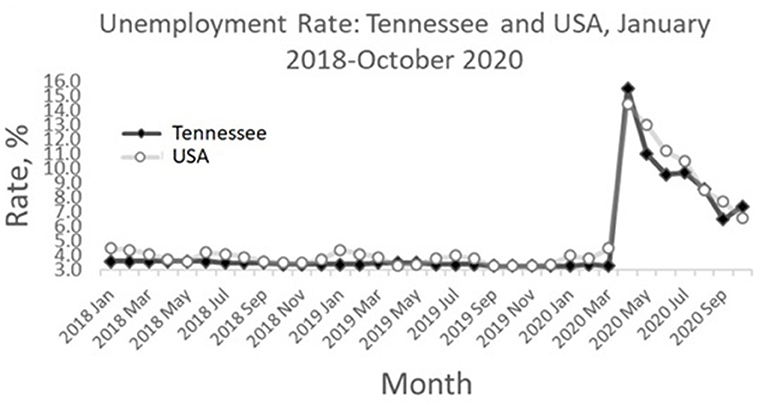
Figure 1. Unemployment rate for workers 16 years and over, January 2018–October 2020, % (seasonally adjusted headline number published each month).
Data
We relied on two primary sources to explore the impact of the COVID-19-caused economic recession upon the labor market. First, we used the labor force data measured by unemployment rates by county as defined in the Background information section, not seasonally adjusted, from the Bureau of Labor Statistics (BLS), Local Area Unemployment Statistics (LAUS) program, which is the primary source of the official unemployment rate which we used to track monthly unemployment changes at a county level in Tennessee (retrieved from https://www.bls.gov/web/metro/laucntycur14.txt). While relatively limited by the scale of the analysis (the county level is the finest available scale), it nevertheless allows to study the effect of social disadvantage on unemployment across communities during various stages of recession, on a month-by-month basis. Additionally, as stated above, whole communities have been documented to suffer the pernicious impact of unemployment (International Labor Organization, 2020), further justifying the choice of the scale of the analysis in this research.
We begin with the across-county comparison of economic collapse in a context of the social disadvantage that continues to play out during the current public health crisis. For this, we identified communities by their social disadvantage status using a composite variable created as the summed z-scores of the initial variables selected to represent social disadvantage. Since the main focus of the study is the pandemic impact on unemployment in vulnerable communities, we divide counties into two groups by the cut-off value of the composite variable. Recent studies report poor and working class communities are disproportionately impacted by the crisis due to the inequality (Blustein et al., 2020). Accordingly, next, to understand the impact of social disadvantage and to investigate how much the employment collapse varies between communities with high and low social disadvantage, we answer the following questions: (1) are unemployment rates different between communities with high and low social disadvantage during pre-COVID-19 (that is, during the period of August 2019–March 2020); (2) are unemployment rates different between the communities during COVID-19-caused economic recession (that is, during the period April 2020–April 2021); and (3) did the impact of disadvantage change during COVID-19 compared with the pre-COVID-19 period? We tested the hypothesis of no difference in unemployment rates between the two types of social disadvantage against the alternative that these communities have different rates. Next, because the COVID-19 pandemic has aggravated existing inequities in the labor market where millions of the US workers had precarious jobs even before the health crisis (Blustein et al., 2020), we further hypothesized that in more disadvantaged communities, the impact of social disadvantage on unemployment may be felt stronger during COVID-19 than pre-COVID reflected in even worse labor market outcomes. We describe in detail the methods applied below.
Identification of Socially Disadvantaged Areas
To understand the effect that social disadvantage has on unemployment, we first, identified areas that were already vulnerable before the beginning of the COVID-19 pandemic and designate these as “socially disadvantaged areas” using an approach based on a set of consistent criteria. Second, we compared unemployment rates between disadvantaged areas and communities with low social disadvantage. We describe below the rationale for delineation of the communities and summarize socio-economic and demographic characteristics of these and other communities regarding race, ethnicity, poverty, and health status as well analyze unemployment.
Some socio-economic and demographic conditions consistently and significantly impact some communities more often than others, particularly racial and ethnic minority members, lower-income people, and residents of rural areas. The conditions include a consistent lack of economic opportunity, environmental factors, transportation and housing, as well as systemic issues such as fragmented health care system (within which some individuals do not get health care in a timely fashion), racism and structural disparities in education, income, wealth (Petterson et al., 2020). Many of these already existing disparities unfold during COVID-19 as black communities are disproportionately affected by unemployment, financial stress, and higher death rates due to the COVID-19 virus (Thebault et al., 2020).
To capture social disadvantage, we will consider a number of the key metrics as outlined in (Bullard, 2000). Research identifies characteristics associated with disadvantage in studies on various aspects of health and environmental justice and standardly includes poverty as a measure of economic well-being, occupations below management and professional levels, low rent, and a high concentration of black residents caused by residential segregation and discriminatory housing practices, and poor air quality (Bullard, 2000, p. 6). To represent advantaged and disadvantaged communities in the United States at a national county level, Miranda et al. (2011) suggested using the following key metrics of environmental justice: race, ethnicity, age, and poverty rates. Neighborhood disadvantage includes poor housing and overcrowding, as well as stress from poverty, violence with tenants in poverty having little control over these factors (Malhotra et al., 2014). The literature identifies minorities, Hispanics, crowded households, dense areas, obesity, poverty as important factors contributing to social disparity. When it is difficult to choose a primary variable from several outcomes, then the composite of these variables can be created (Freemantle et al., 2003). Additionally, it is challenging to isolate the separate effects of the listed and other factors such as unemployment, minority and ethnicity, and population density. Accordingly, we created a composite variable to logically represent a multi-dimensional social disadvantage by “critically analyzing the theoretically intended meaning of a concept” (Song et al., 2013). Farther, an evaluation of a phenomenon by single variables results in methodological problems, thus, studies rely on a development of composite indices consisting of more than one variable (Salaffi et al., 2000).
We claim that places where certain socio-economic and racial attributes, existing obesity, and workforce hierarchies co-locate spatially, are experiencing additionally economic stresses exactly as the COVID-19 pandemic is playing out that may eventually contribute to a greater vulnerability to COVID-19 infection and mortality. A recent study identified young families with children, low income population, and social assistance recipients as demographics vulnerable to COVID and areas with higher shares of low-income families and higher crime as social injustice factors similarly related to COVID-19 transmission (Vaz, 2021). Accordingly, in creating a composite variable to stand in for social disadvantage, the following variables were used: % African American, % Hispanic, % below 100% federal poverty level, % estimated obese adults reporting to be obese with the BMI 30 or greater, % unemployment, % less than high school graduates.
Because the original variables have different variances, standardization is needed to avoid a disproportionate impact upon the association between the composite social disadvantage score and variables of interest caused by any one original variable with a large variance. Accordingly, we applied the z-score transformation by averaging the original variables and computing z-scores with a mean of 0 and values ranging from negative to positive numbers, and next summing up z scores of the original variables (Salaffi et al., 2000; Song et al., 2013). By converting the original variables to z-scores, the distribution of the raw scores is preserved and the contributions of the original variables are equal.
Based on the distribution of values of the composite variable, the cut-off value was established for social disadvantage to be assigned to areal units within the study area as areas with high or low social disadvantage, as explained below. After this, we answered a question whether these different communities are statistically different regarding all key variables used in creating a composite variable for identification of communities with social disadvantage. We statistically analyzed both community types and compared two community types for statistical difference of those variables.
The composite variable ranged between −8.27 to 10.02 (mean =0.002, St.Dev. =3.39). Since a criterion measure is absent (Tubach et al., 2005a,b), to determine an optimal threshold of a composite index, cut-offs are defined by different techniques (Dougados et al., 2012). Researchers rely either on expert consensus (that is, the threshold is investigator-defined), or statistically-based methods (Beaton, 2003; Crosby et al., 2003). To identify a cut-off point, we visually evaluated our empirical data distribution and observed where it starts to fall off more quickly in the frequency distribution using a constructed histogram of the composite variable (Figure 2). We also reviewed the literature on the topic where the past studies proposed to use the value of the final score at the 75th percentile corresponding to the cut-off point (Tubach et al., 2005a,b). Using the frequency distribution on the histogram, we empirically observed that frequencies declined relatively steeply after the value of 1.53, while, the value corresponding to the 75th percentile for the range of the composite variable occurred at 1.45 which is close to the cut-off point determined empirically above and easier to derive. Thus, we applied the 75th percentile technique and used the score value of 1.45 (corresponding to the 75th percentile) as a cutoff value for defining disadvantaged communities: those with a score >1.45 have been assigned as communities with high social disadvantage (Nhigh =24) while those below as communities with low social disadvantage (Nlow =71), respectively. As explained above, to validate the approach taken to identify disadvantaged communities as well as the selected cut-off value at the 75th percentile in choosing the point to designate disadvantaged communities, we conducted an independent two-sample difference of means T-test of the difference in the 2019 Census variables between disadvantaged and less disadvantaged communities that we used in creating a composite variable to stand in for social disadvantage using 5% as the established level of statistical significance (the sample data met the requirements of parametric testing such normality in the distribution and sample size).
Table 1 presents the results as means, t-statistics and p-values. It also reports the descriptive statistics across counties in TN. It includes the summaries of demographic characteristics for workers of communities with high and low social disadvantage allowing to compare the variables of interest between these communities and the results of the T-test. The following variables are reported: percent African American, percent Hispanic, median income, percent of people over 25 years who are less than high school graduates, estimated percent of obese adults, percent households below 100% of poverty, and percent of multi-generation households. The two-sample difference of means T-test found significant difference in all selected variables except the share of Hispanic residents: the disadvantaged communities have over three times as many African-American residents as less-disadvantaged communities (15 versus 4.8%), 4% more residents who are less than high school graduates (19 versus 15%), their median income is over $2,000 less ($22,000 versus $24,000), a higher share of residents below poverty 100% (21.3 versus 16.8%), more households that are multi-generation, and 2% more obese adults (35.4 versus 33.7%). Our approach allows to locate communities where disadvantage existed pre-COVID-19 that continued to unfold after the pandemic began reflected in worse outcomes further impacting well-being of these communities and the residents (described below). For visualization, we mapped the spatial distribution of counties with high and low social disadvantage within our study area (Figure 3): the counties surrounding cities (shown with a red star symbol on the figure) are urban and the remaining are rural.
Statistical Analyses
After we identified disadvantaged communities, where social vulnerability existed before the COVID-19, to understand the impact of disadvantage, we analyzed the variable of interest (unemployment rates) for both types of communities before and after the pandemic-caused economic recession for the period of August 2019–April 2021. In this section, we describe the hypotheses tested and the analyses undertaken to understand the distribution of the job losses across counties.
Hypothesis 1: Over an entire study period (August 2019–April 2021), for all counties combined, regardless
of social disadvantage status, and broken down by disadvantage, unemployment rates did not vary significantly by month.
Alternative hypothesis: Over an entire study period (August 2019–April 2021), for all counties combined, regardless of social disadvantage status, and broken down by disadvantage, unemployment rates varied significantly by month.
Hypothesis 2: Counties with different social disadvantage status had similar unemployment rates during all stages of the recession.
Alternative hypothesis: Counties with different social disadvantage status had significantly different unemployment rates during all stages of the recession and unemployment rates significantly varied by month.
For Hypothesis 1, we tested if unemployment rates varied by month starting with August 2019 data through April 2021 (the latest month for which data is available at BLS). The 21-month-worth unemployment data for all TN counties combined approximates normal distribution. We conducted a one-way ANOVA for unemployment rates (a dependent variable) for all counties combined for all 21 months, regardless of their social disadvantage status, and broken down by disadvantage. The model is estimated on data from August 2019 through April 2021. The results are shown in Figure 4. The analysis performed employed the SAS statistical software package (SAS, 2010).
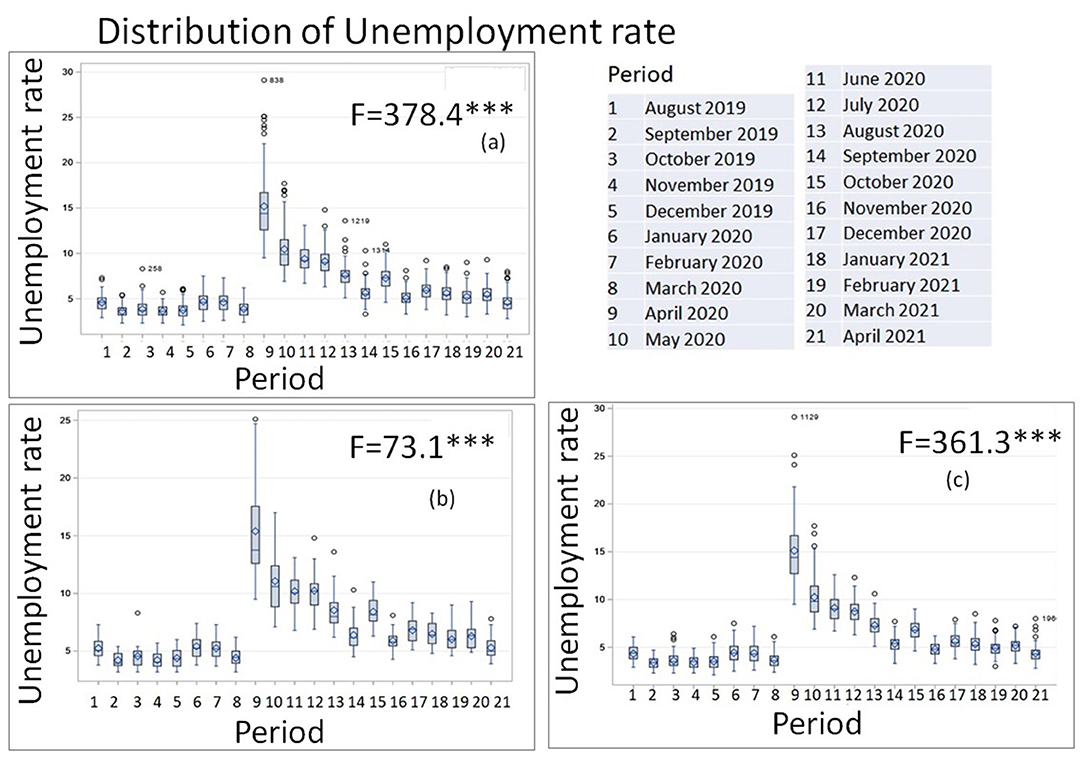
Figure 4. One-Way ANOVA of unemployed rate for: (A) all counties combined for 15 months (August 2019–April 2021), regardless of social disadvantage status; and by social disadvantage status, (B) for counties with high social disadvantage; (C) for counties with low social disadvantage.
For Hypothesis 2 to determine whether unemployment rates differed between areas with the different social disadvantage status (here, high and low social disadvantage), we used a parametric t-test (unemployment data come from a distribution close to normal distribution, see Appendix A, Figure A1 presenting a histogram of unemployment rates in communities with high and low disadvantage for August 2019–July 2020). The 2-sided T-test was applied to the more and less disadvantaged areas.
In a regression model, we also explored worker characteristics that are most associated with unemployment during the late stage of economic recession (unemployment rate is estimated using September 2020 unemployment rate data). A cross-sectional analysis is insufficient to show the causal relationship between variables of interest (here, social vulnerability index and unemployment). However, cross-sectional design allows studying the association of the outcome and the exposure in the population (Setia, 2016). Accordingly, a cross-sectional analysis for September 2020 was implemented to assess the role of social disadvantage in unemployment. More specifically, we studied the association between the exposure to social disadvantage measured by social vulnerability index and unemployment both before and during the COVID-19-caused economic recession.
Urbanity is often understood in terms of density (Storper and Scott, 2016). Thus, urban elements in this study were captured by population density. This variable was used to distinguish between urban and rural areas (counties) in the regression model. Recent research found no correlation between COVID-19-related hospitalization and mortality and the population density or urbanity of the municipality, even controlling for age and public health factors (Boterman, 2020). Thus, COVID-19 variables including mortality and morbidity have not been directly included in the regression. In order to be able to meaningfully compare the role of social disadvantage on unemployment between the pre-COVID-19 period and during the pandemic, a consistent model framework was applied using social vulnerability index as a dependent variable and the same independent regressors for both periods. We estimate multiple regression models that include a range of worker characteristics as predictors.
Often, in spatial data, observed variation in the dependent variable may be attributed to spatial dependence, that is, sample data collected for nearby features in space tend to exhibit similar values of the dependent variable (LeSage, 2008). We tested for spatial dependence (spatial autocorrelation) based on county locations and attribute values using global Moran's I test statistic. Spatial autocorrelation, or spatial association, is one of two classes of spatial effects. It follows directly from the First Law of Geography (Tobler, 1979) resulting in spatial clusters and thus, violating the statistical assumption of independence of observations (Anselin, 1992). Ignoring spatial dependence may result in the invalid results of data analyses (Anselin and Griffith, 1988). Accordingly, we measured spatial autocorrelation in our dependent variable (here, unemployment rate) using inverse distance. When spatial relationships have been conceptualized with inverse distance, proximate neighboring areas (here, counties) have a greater influence on the computations for a variable of interest (here, unemployment) than far away counties. There is no spatial dependence in the attribute (Moran's Index = 0.047, p value = 0.48) allowing for a regression modeling.
Our first regression model (Regression 1), in rows 1–2 of Table 4, includes a multi-dimensional composite variable capturing exposure to social disadvantage as a single predictor of unemployment, while the next regression model (Regression 2) included the following predictors: percent of African-American, percent of Hispanics, percent of population below federal poverty level 100%, percent of multigenerational households, population density per square mile, percent of male population over 16, and percent of male population between 16 and 19 years of age. We did not include women into our unemployment model since the significant gender effect observed in April 2020 has much decreased during the subsequent stages. We conducted all statistical analyses in SAS statistical software, and carried out visualization using ArcMap 10.8 version.
Results
In this section we explore the results of the analyses of the distribution of the job losses across counties in Tennessee by month and community type.
Figure 4 presents the results of a one-way ANOVA for unemployment rates for all counties combined for all 21 months, regardless of their social disadvantage status (Figure 4A), and by social disadvantage status, for counties with high disadvantage (Figure 4B), and low disadvantage (Figure 4C). As expected, it shows that starting April 2020 unemployment statistically differed from that during August 2019–March 2020 at the conventional P=0.05 level. Figure 4 provides the detailed results and illustrates the distribution of mean unemployment rates by month across all counties combined within the study area, August 2019–April 2021 based on the one-way ANOVA results. According to the test results, for all counties, average monthly unemployment rates are not significantly different from August 2019 through March 2020 after which the rate steeply increased (N= 95, F= 378.35, Pr > F < 0.0001). In April 2020–April 2021 unemployment rates for all counties combined were significantly different compared with previous months (August 2019–March 2020).
To satisfy another objective and see if social disadvantage affects unemployment, we compared unemployment rate for counties broken down by social disadvantage status by month. Using a consistent framework, we identified communities with high social disadvantage by applying consistent criteria (N= 24) out of total of 95 counties in Tennessee. Then, we analyzed monthly unemployment data separately for communities with high and low social disadvantage. We tested a hypothesis whether unemployment rates at the more and less disadvantaged areas were different using a two-sample t-test (reported in Table 2). Figure 5 presents the results of the two-sample t-test visually and shows the distribution of unemployment rates between areas of high and low disadvantage starting in April 2020 through March 2021. The unexpected finding from the analysis is that two-sided p-values are not significant at the =0.05 level for communities with the different social disadvantage status in April 2020 and May 2020 indicating no difference in unemployment rate during this period. As the pandemic started, high unemployment struck both advantaged and disadvantaged communities resulting in similarly high rates. This finding is supported by Chetty et al. (2020) who analyze the initial impacts of COVID-19 on economic activity reporting unemployment rates surging also in affluent areas with relatively low unemployment rates in prior downturns. Some state-level response policies introduced to contain the infection spread, may contribute to job losses, including restaurant and bar limitations and non-essential business closures which together explain 12.4% of unemployment insurance claims (Kong and Prinz, 2020). However, as recession progressed, for June 2020–March 2021 the results suggest that there is a statistically significant difference between the underlying distributions of the unemployment rates of communities with high social disadvantage and the unemployment rates of communities with low social disadvantage (p < 0.0001) letting us to reject the null hypothesis of similar unemployment between these communities during the later stages of the recession.
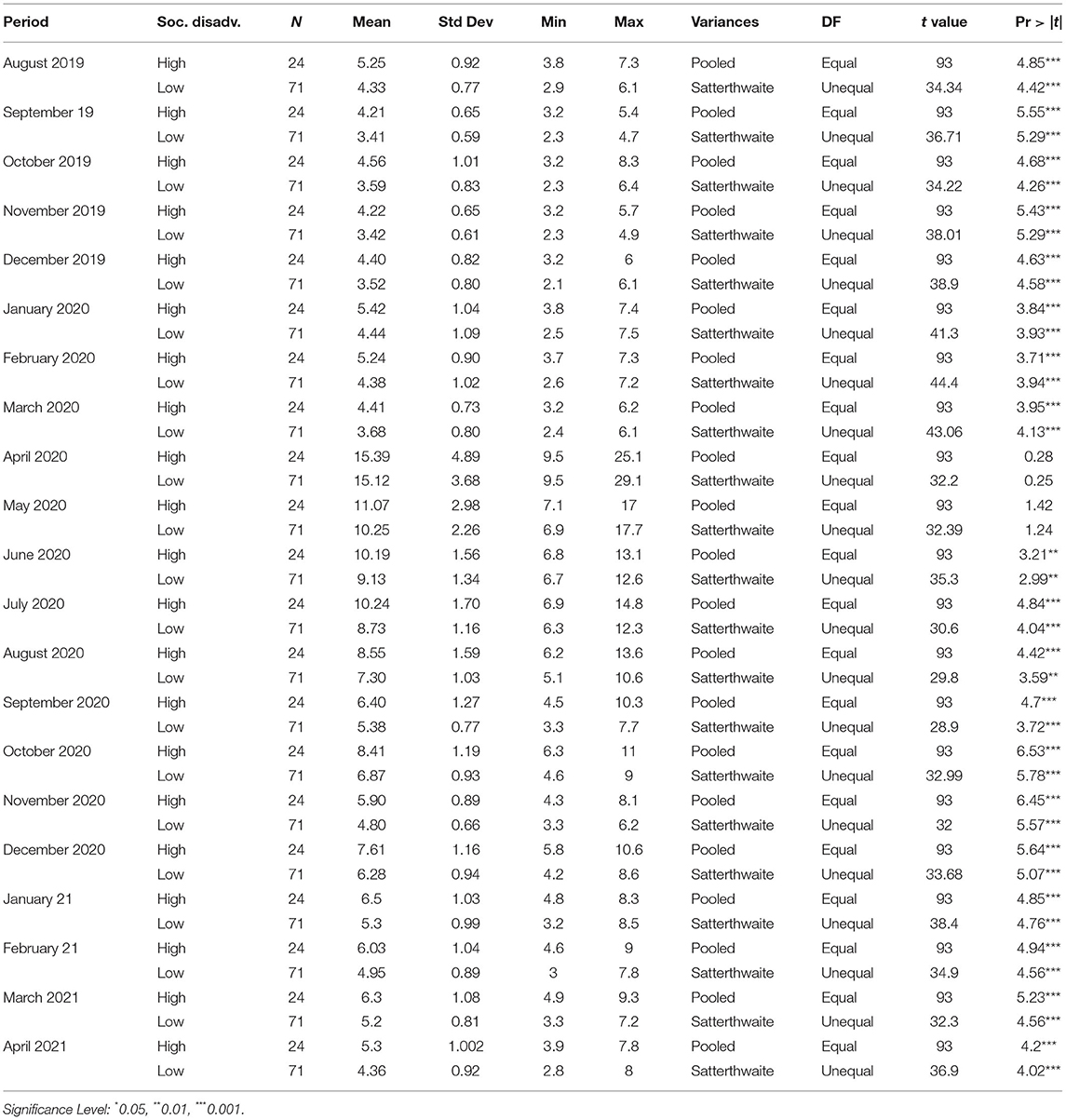
Table 2. T-test results of unemployment rates between communities with high and low disadvantage, August 2019–April 2021.
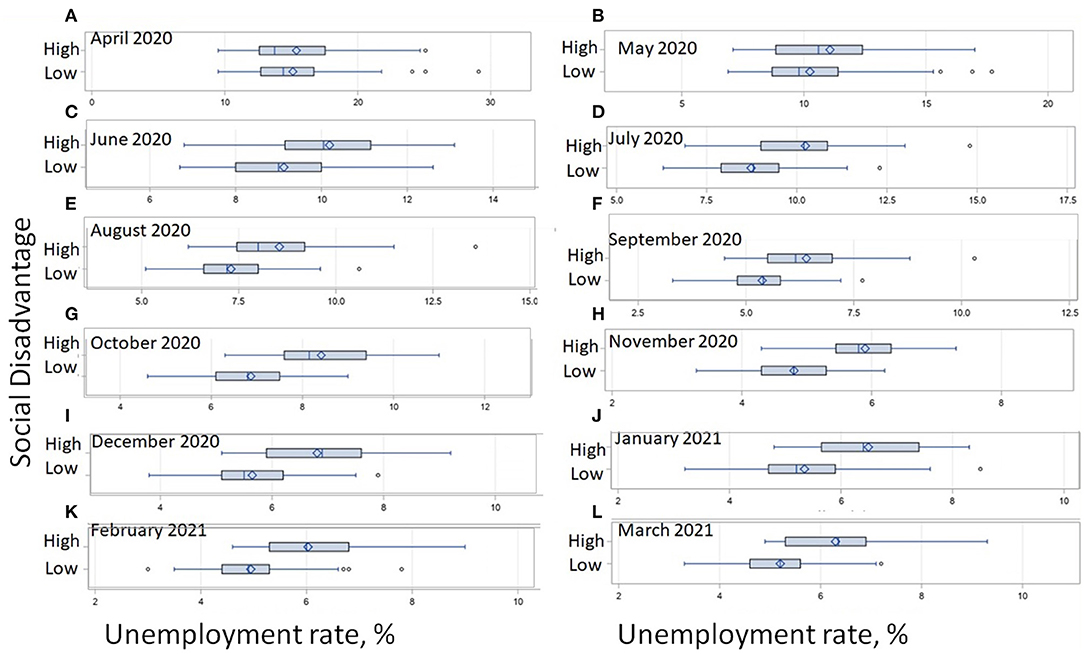
Figure 5. Distribution of unemployment rates for areas with high and low social disadvantage for the period of April 2020-March 2021: (A) April 2020; (B) May 2020; (C) June 2020; (D) July 2020; (E) August 2020; (F) September 2020; (G) October 2020; (H) November 2020; (I) December 2020; (J) January 2021; (K) February 2021; (L) March 2021.
Overall results show that unemployment peak occurred in April 2020 where both types of communities had similarly high rates, however while economic activities resumed in most counties, it did not happen in counties with high disadvantage in Tennessee which continued suffering from significantly higher joblessness well into the later stages. The unemployment accumulates as recession progresses into Summer and becomes significantly different between the two types of communities by social disadvantage with partial economic recovery. Areas with high social disadvantage, already vulnerable are additionally burdened with a higher unemployment and inability to resume economic activity. We conclude that the true average of unemployment for the various exposure to social disadvantage is different and is greater for socially higher disadvantaged areas than for low disadvantaged areas.
Figure 6 reports the effects from a regression model for the impact of social disadvantage on the unemployment rates using September 2020 data with unemployment rates increasing the higher the disadvantage. We did not use October 2020 data due to it being preliminary, and thus, higher than that for the preceding month (seen in Figure 2). Starting with the estimates for the relationship between disadvantage and unemployment in Figure 6, we see that for the composite score with a value of around −8 (negative 8), which corresponds to the least disadvantaged areas, the predicted unemployment level was at about 4%, while the areas with the score of about 10 had an estimated unemployment of about 8% in September. Conceptually similar to a recent finding regarding struggling businesses (Bartik et al., 2020), communities that had lower social disadvantage pre-COVID are much more likely to resume economic activity faster and add jobs than communities that were already vulnerable due to high social disadvantage.
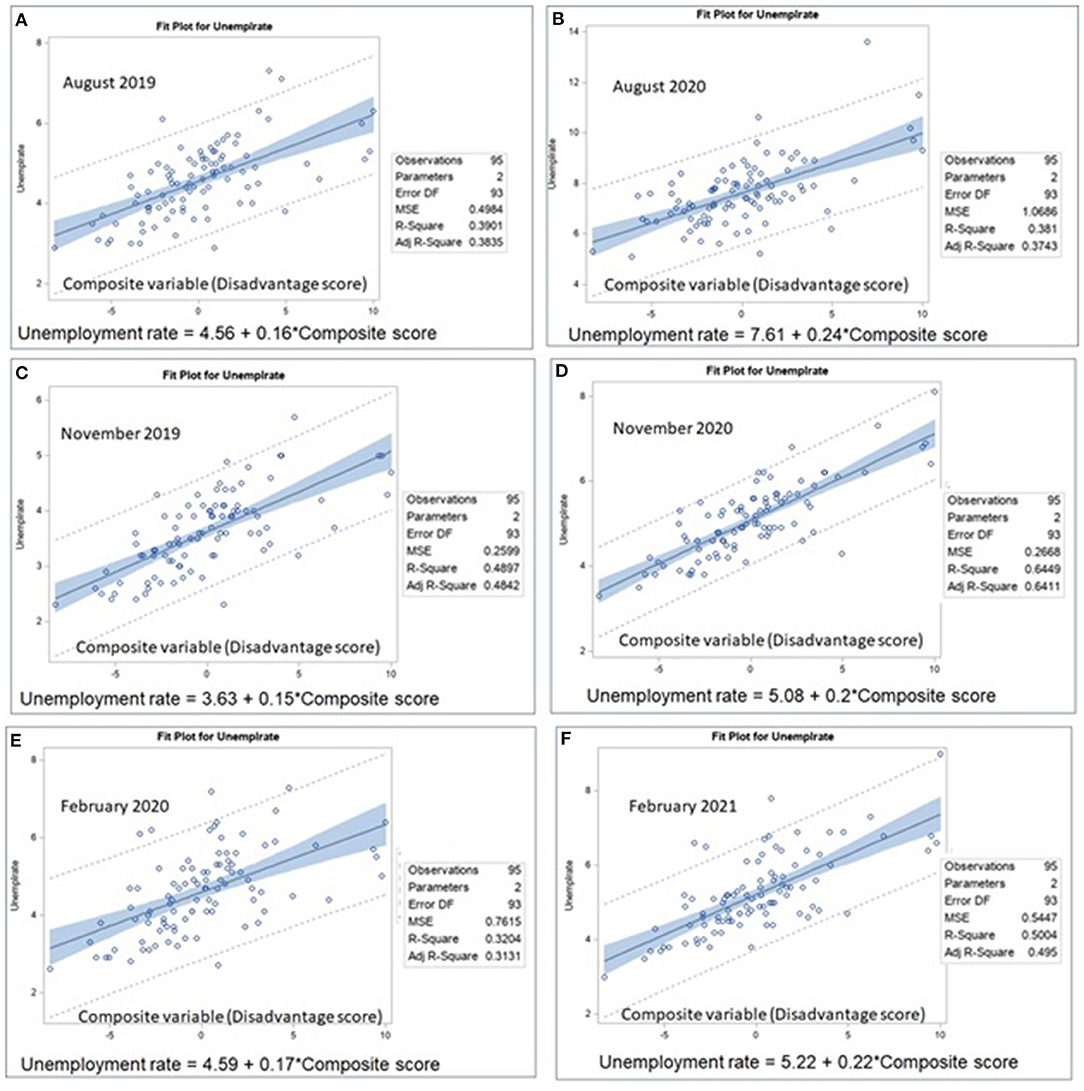
Figure 6. Line fit plots for an unemployment and social disadvantage for TN counties for before- and post-COVID-19: (A) August 2019; (B) August 2020; (C) November 2019; (D) November 2020; (E) February 2020; (F) February 2021.
Table 3 reports the results of the regression analysis (correlation between independent variables can be found in Table A1 in Appendix A). Social disadvantage affects unemployment, with the higher values of a composite variable capturing several dimensions of social vulnerability associated with greater unemployment rates. Racial membership and poverty are both positively and highly statistically significantly associated with joblessness. A similar finding was on job loss and poverty disproportionately experienced by Black and Latino workers and their families amidst the COVID-19 crisis (Parolin and Curran, 2020). Poverty and loss of employment go hand in hand: the finding on the association between households below poverty line and unemployment was in line with Chetty et al. (2020) who report that workers who were in poverty had much higher drops in employment rates compared with more earning workers: the bottom quartile wage earners had their employment dropped by 37% in April 2020, versus 14% decrease for workers in the top quartile of the pre-COVID wage distribution (Chetty et al., 2020). Similarly, the greater the number of multi-generation households, the higher unemployment rate. As explained above, the variable of population density was used to distinguish between urban and rural counties in the regression. As expected, there is a significant positive association between concentration of people and joblessness. The US urban economies are primarily services-oriented. Unemployment results from an imbalance between the supply of labor (population size) and the demand for it. Since the demand for non-essential services has steeply decreased after the COVID-19 started, areas with substantial populations had very steep increases in unemployment simply due to the sheer size of population whose demand for non-essential services dropped and workforce employed in the non-essential services sector. Urban areas are expected to be disproportionately impacted by COVID-19 due to high population densities (Boterman, 2020). However, although the statistical association has been identified, we did not find big differences between urban and rural counties, most likely due to the county scale used for the analysis. The variation in population densities within the state of Tennessee might just be too small to expect large differences (Boterman, 2020). Areas with high population density, urban areas in the context of TN such as Shelby county with Memphis, also concentrate a substantial percent of disadvantages workers and contribute to joblessness level. In November 2020, the most recent data on unemployment rate in Shelby county is at 7.3% (compared with November 2019 at 3.7%) while the state's level is 5.3% (TN Department of Labor Workforce Development, 2020).
Cross-sectional studies may also be used to calculate the odds ratios (ORs) (Setia, 2016). For example, to understand the association between exposure to social disadvantage and outcomes of COVID-19-caused unemployment, we will be able to create a 2 × 2 table for the above-mentioned cross-sectional study (Table 4).
In our study, ethnic membership (Hispanics) is not associated with higher unemployment, although this category was associated with a greater likelihood of losing a job during the earlier stages of the current recession. In agreement with other research studying the impact of COVID-19 on gender equality in the United States where male workers may easier adjust to the changes in employment caused by the pandemic while women may find it harder and at a greater risk of losing their jobs (Alon et al., 2020), in our study, male workers are negatively related to unemployment (though, not statistically significant). While in previous economic downturns which impacted more heavily manufacturing and construction which account for 24% of men's overall employment and only 8% of women's overall employment (Coskun and Dalgic, 2020), thus, affecting the employment of male workers rather than female workers due to a greater concentration of men in those industries, Another factor which featured earlier in the recession as contributing to unemployment is younger age, as teenaged workers experienced a higher rate of unemployment than other age groups in April 2020. However, our model finds younger male worker is negatively associated with unemployment rate.
Discussion
Overall, the study contributes to a growing contemporaneous research on the consequences of the COVID-19 recession. We focus on the economic disparities of the unemployment brought about by the pandemic. More specifically, this study provides a novel contribution to the body of work documenting the differing COVID-19-related economic impacts in high and low socially disadvantaged communities with social disadvantage unfolding during the pandemic and across demographic groups in a state with a high rate of the virus per capita as the ongoing economic recession has progressed.
A range of monthly cyclical indicators including employment estimates produced by the Bureau of Labor Statistics (BLS) is used in determining peaks and troughs in a business cycle, with a recession occurring during the interval between the ups and the downs of the economy when economic activity is diminishing (NBER, 2020). In this study, we measured labor market outcomes by unemployment rate and calculated it as a percentage of unemployed workers in the total labor force. We combined official unemployment county-level data with the traditional government survey data to measure the COVID-19-related economic impact using the most recent data.
The premise of the study was that areas with high social disadvantage might fare worse in terms of unemployment which is an indicator of social disadvantage. Documented by recent research, unemployment has pernicious effects on many domains at the individual and community levels including the psychological, economic, and social well-being effects (Blustein, 2019). Compared with communities with low social disadvantage, we did not find evidence of the difference during the early COVID-19 period. Even when the pandemic struck, during the initial stages, the impact was so sudden and severe, that both types of communities had similarly high unemployment levels. No difference in unemployment was observed over April through May 2020 between both types of communities. However, we did find a significant variation in unemployment during the subsequent stages of the recession. Once economic activities began to resume, though on a limited basis across counties, it happened on a much smaller scale in communities with high social disadvantage. In communities with a low social disadvantage, joblessness is significantly lower than in vulnerable communities.
There was a statistically significant difference in jobless workers of socially disadvantaged communities starting in June and continuing through October 2020. A recent study had a similar finding concerning those firms that struggled before the onset of the COVID as their odds of closing down and of remaining shut down have much increased during the COVID crisis (referred to as the recession-based “cleansing effect”), likely due to insufficient cash and inability to withstand the shock, or less business productivity in the economy (Bartik et al., 2020).
We show that some socio-demographic groups have a systematically higher likelihood of being unemployed as the economic recession continues. Communities with a greater share of African Americans are more likely to have greater unemployment rates. Prior studies tested validity of “the last hired the first fired” as blacks are claimed to be the last hired during economic prosperity however the first fired in recessions, finding substantial evidence that as the business cycle becomes weaker blacks are the first laid off (Couch and Fairley, 2005). Additionally, we find that poverty, multi-generation households, higher population density are associated with unemployment during the later stages of the ongoing pandemic-caused recession (using unemployment data for September 2020). This finding confirms similar results studying the impacts of worker demographic factors upon unemployment during the early pandemic period. During the COVID, the monthly poverty rates grew compared with the pre-COVID period (at 15% in February 2020 to 16.7% September 2020) (Parolin et al., 2020). Even given restoration of the Coronavirus Aid, Relief, and Economic Security (CARES) Act unemployment provisions, poverty remains high and Black and Hispanic persons and children are acutely affected, poverty rates are estimated for the US population at 15.1–17.5% in January 2021 (Parolin and Curran, 2020).
A different gender impact emerged in this economic downturn that has not been observed previously due to different industries being impacted. While manufacturing and construction were more heavily impacted during previous economic downturns so that men lost more jobs, the COVID-19 impacted the different types of occupations such as education and health services disproportionately held by women (accounting for 21% of women's overall employment and only 6% of men's overall employment) (Coskun and Dalgic, 2020). Additionally, large-scale closures of daycares and schools have taken place during the pandemic, forcing parents to care for and educate their children at home, thus, representing substantial childcare challenges, especially for single parents, with women providing most childcare needs (Doepke and Kindermann, 2019). Female workers' ability to continue working might be reduced as not all female workers with children are able to combine work with childcare responsibilities due to their occupation. On a positive side, benefits from a pandemic recession are predicted to accrue to women with major childcare responsibilities who may use a greater work flexibility during a pandemic recession and gender inequality including the gender wage gap despite initial widening during the pandemic and as it will recede, is expected to reduce over the longer term (Alon et al., 2020).
Past research indicated that compared with prime age white males, other demographic groups including blacks, women, and youths react to poor labor markets by exiting the labor force (Smith et al., 1974), a “discouraged workers” phenomenon where many unemployed workers stop actively searching for work that has been observed during the COVID-19-caused severe recession (Coibion et al., 2020).
Some state-level response policies introduced to reduce the movement and social interactions during the COVID-19 and to contain the infection spread, may increase job losses due to reduced economic activity. Response policies that directly and indirectly impact economic activity include restaurant and bar limitations, stay-at-home and shelter-in-place orders, large-gathering bans, school closures, emergency declarations, and non-essential (such as hair salons, museums, movie theaters, indoor recreational centers) business closures. A recent study on the labor-market effects of several of these policies determined that restaurant and bar limitations and non-essential business closures together explained 12.4% of unemployment insurance claims filed short-term (Kong and Prinz, 2020). Similarly, Bartik et al. (2020) find only a modest impact of government policies collectively referred to as shut-down and re-open orders promulgated by states upon economic activity and state labor market outcomes during the crisis, while public health fears, economic concerns, and the calendar time effects provide a greater impact both upon product demand and labor supply, especially in the “in-person” industrial sectors. However, since the policies are imposed at the state level, we could not analyze the effect of response policies.
Infection rate itself is another contributing factor. Dalton (2020) found a stronger negative COVID-19 impact on employment in local labor markets that had a higher infection incidence in April 2020. Analyzing April 2019 (as a control), February 2020, March 2020, and April 2020 showed that a county's decrease in 1% of COVID-19 cases per 100 leads to a 4.3% increase in overall employment at a facility located within the county. The variation in employment over several months across geographic areas with a higher COVID-19 rate is not explained by similar local characteristics in geographic areas such as population density, industry mix within sectors, etc.
We followed contemporary COVID-related research using county-level scale for the analysis. Dalton (2020) used counties to represent communities in the study of local labor market impacts of COVID-19 finding the link between incidence of the virus in the preceding month and employment at county level. Another recent study assessed the association between density and urbanity and COVID-19 health measures (cases, the hospitalization and the reported deaths) with the Poisson regression models at the municipal level in the Netherlands (Boterman, 2020). Similarly, in this study, the spatial scale of input data is at county level, also due to a reason that this is the finest disaggregated spatial units at which the unemployment data is available presently. Thus, differences within counties cannot be captured. The inability to measure the intra-county variability using census data at census tract level as a robustness check of the chosen unit of analysis represents a limitation of the study.
Contemporary research calls for the use of rigorous quantitative methods to identify distinct groups of unemployed individuals such as cluster analysis assessing their external circumstances including financial condition, family composition, and living conditions to understand the nature of unemployment during this health crisis period so that interventions at individual and systemic scales could be created and assessed involving the identified affected individuals and communities with a high share of such individuals (Blustein et al., 2020). This research helps toward this new understanding. Building on the research findings, meaningful interventions may be created that include systemic county-level interventions based on analyses of the communities with high shares of minorities, low income, low education, multi-generation households, and obese adults.
The following caveats concern the validity of the assessment results. As mentioned, this being a cross-sectional study, it may have all the limitations of a cross-sectional study. Thus, the generalizability from these data may be limited. Also, the county scale may mask the intra-county variations in the phenomenon investigated.
Among the study's contributions to existing knowledge is the development of an approach to identify communities with high social disadvantage captured by several metrics, and integration in a single composite index of multi-dimensional social disadvantage. Identifying communities where disadvantage exists is of upmost importance as it may mitigate the COVID-19-related adverse economic outcomes.
We show that in communities that were already vulnerable due to high social disadvantage it is harder to resume economic activity and add jobs. With the high levels of unemployment as those observed since June 2020 through October 2020 and which are significantly greater in communities with high social disadvantage, we see little evidence of economic recovery, where “many firms remain closed and many workers have not returned” (Bartik et al., 2020, p. 17), while the laid-off workers may find it much harder re-entering the labor market, a situation exacerbated by a second wave of business closings.
Overall, the post-pandemic economic recovery is expected to be delayed affecting vulnerable communities. Further, assuming the pandemic lingers, while new remote working arrangements may permanently shift many jobs worked from home, many disadvantaged workers are unable to telework or stay home. Yet other potential pandemic consequences include spatial distributions of jobs, labor supply, and worker spending, affecting the future of cities (Barrero et al., 2020), and therefore, having potentially profound effects on vulnerable communities which already experienced greater employment exodus from their residential areas (Antipova, 2020a). Future months will confirm whether concerns about the transmission of infectious diseases result in the expected business, jobs, workers and capital big-scale reallocations away from dense cities with further implications for disadvantaged communities.
Data Availability Statement
Publicly available datasets were analyzed in this study. This data can be found here: https://www.bls.gov/web/metro/laucntycur14.txt and https://censusreporter.org/.
Ethics Statement
Ethical review and approval was not required for the study on human participants in accordance with the local legislation and institutional requirements. Written informed consent for participation was not required for this study in accordance with the national legislation and the institutional requirements.
Author Contributions
AA was responsible for research, methodology design, writing, and data analysis. EM was responsible for research and editing. All authors contributed to the article and approved the submitted version.
Funding
This work was supported by the UTHSC/UofM SARS-CoV-2/COVID-19 Research CORNET (Collaboration Research Network) Award.
Conflict of Interest
The authors declare that the research was conducted in the absence of any commercial or financial relationships that could be construed as a potential conflict of interest.
Publisher's Note
All claims expressed in this article are solely those of the authors and do not necessarily represent those of their affiliated organizations, or those of the publisher, the editors and the reviewers. Any product that may be evaluated in this article, or claim that may be made by its manufacturer, is not guaranteed or endorsed by the publisher.
Acknowledgments
The authors are thankful to an undergraduate student Bethany Brashiers who provided help with the research.
References
Alon, T., Doepke, M., Olmstead-Rumsey, J., and Tertilt, M. (2020). This time it's different: the role of women's employment in a pandemic recession. CRC TR 224 Discussion Paper Series CRC TR 224_2020_198, University of Bonn and University of Mannheim, Germany. doi: 10.3386/w27660
Anselin, L. (1992). Spatial Data Analysis with GIS: an Introduction to Application in the Social Sciences. Technical Report 92-10. Available online at: http://ncgia.ucsb.edu/technical-reports/PDF/92-10.pdf (accessed July 29, 2021).
Anselin, L., and Griffith, D. A. (1988). Do spatial effects really matter in regression analysis? Pap. Reg. Sci. Assoc. 65, 11–34. doi: 10.1111/j.1435-5597.1988.tb01155.x
Antipova, A. (2020a). Analysis of commuting distances of low-income workers in Memphis Metropolitan Area, TN. Sustainability 12:1209. doi: 10.3390/su12031209
Antipova, A. (2020b). “Analysis of exposure to ambient air pollution: Case study of the link between environmental exposure and children's school performance in Memphis, TN,” in Spatiotemporal Analysis of Air Pollution and Its Application in Public Health (Amsterdam: Elsevier), 217–275. doi: 10.1016/B978-0-12-815822-7.00011-X
Barrero, J. M., Bloom, N., and Davis, S. J. (2020). COVID-19 also a reallocation shock. Working paper NO. 2020-59. Available online at: https://bfi.uchicago.edu/wp-content/uploads/BFI_WP_202059.pdf. doi: 10.3386/w27137
Bartik, A. W., Bertrand, M., Lin, F., Rothstein, J., and Unrath, M. (2020). Measuring the labor market at the onset of the COVID-19 crisis. NBER Working Paper No. 27613, July 2020. JEL No. E24,E32,J2,J63. doi: 10.3386/w27613
Beaton, D. E. (2003). Simple as possible? Or too simple? Possible limits to the universality of the one half standard deviation. Med Care 41, 593–596. doi: 10.1097/01.MLR.0000064706.35861.B4
Blustein, D. L. (2019). The Importance of Work in an Age of Uncertainty: the Eroding Work Experience in America. Oxford University Press: New York, NY. doi: 10.1093/oso/9780190213701.001.0001
Blustein, D. L., Duffy, R., Ferreira, J. A., Cohen-Scali, V., Cinamon, R. G., and Allan, B. A. (2020). Unemployment in the time of COVID-19: a research agenda. J. Voc. Behav. 119:103436. doi: 10.1016/j.jvb.2020.103436
Boterman, W. R. (2020). Urban-rural polarisation in times of the corona outbreak? The early demographic and geographic patterns of the SARS-CoV-2 epidemic in the Netherlands. Tijds. Voor Econ. Soc. Geog. 111, 513–529. doi: 10.1111/tesg.12437
Bullard, R. (2000). Dumping in Dixie: Race, Class, and Environmental Quality, Third Edition. Boulder, CO: Westview Press.
Bureau of Labor Statistics (BLS) (2020). Economic News Release. State Employment and Unemployment—November 2020. USDL-20-2267. Available online at: https://www.bls.gov/news.release/laus.nr0.htm (accessed December 18, 2020).
Census Bureau (2019). The Supplemental Poverty Measure: 2018. Current Population Report. Available online at: https://www.census.gov/content/dam/Census/library/publications/2019/demo/p60-268.pdf (accessed June 6, 2021).
Census Bureau (2020). The Supplemental Poverty Measure: 2019. Current Population Report. Available online at: https://www.census.gov/content/dam/Census/library/publications/2020/demo/p60-272.pdf (accessed June 6, 2021).
Chetty, R., Friedman, J. N., Hendren, N., and Stepner, M. (2020). The Economic Impacts of COVID-19: Evidence From a New Public Database Built Using Private Sector Data. National Bureau of Economic Research Working Paper Series. Working Paper No. 27431. Available online at: https://www.nber.org/system/files/working_papers/w27431/w27431.pdf (accessed July 29, 2021). doi: 10.3386/w27431
Coibion, O., Gorodnichenko, Y., and Weber, M. (2020). Labor markets during the COVID-19 crisis: a preliminary view. NBER Working Paper No. 27017. doi: 10.3386/w27017 (accessed April 2020)
Cortes, G. M., and Forsythe, E. (2020). The heterogeneous labor market impacts of the Covid-19 pandemic. Upjohn Institute Working Paper, May 2020. doi: 10.17848/wp20-327
Coskun, S., and Dalgic, H. (2020). The emergence of procyclical fertility: the role of gender differences in employment risk. CRC TR 224 Discussion Paper Series No. 142.
Couch, K. A., and Fairley, R. (2005). Last Hired, First Fired? Black–White Unemployment and the Business Cycle. Economics Working Papers: 200550. Available online at: https://opencommons.uconn.edu/econ_wpapers/200550 (accessed July 29, 2021).
Crosby, R. D., Kolotkin, R. L., and Williams, G. R. (2003). Defining clinically meaningful change in health-related quality of life. J. Clin. Epidemiol. 56, 395–407. doi: 10.1016/S0895-4356(03)00044-1
Dalton, M. (2020). Labor Market Effects of Local Spread of COVID-19. U.S. Bureau of Labor Statistics. Working Paper 524. Available online at: https://www.bls.gov/osmr/research-papers/2020/pdf/ec200060.pdf (accesed June 2020)
Doepke, M., and Kindermann, F. (2019). Bargaining over babies: theory, evidence, and policy implications. Am. Econ. Rev. 109, 3264–3306. doi: 10.1257/aer.20160328
Dougados, M., Brault, Y., Logeart, I., van der Heijde, D., Gossec, L., and Kvien, T. (2012). Defining cut-off values for disease activity states and improvement scores for patient-reported outcomes: the example of the Rheumatoid Arthritis Impact of Disease (RAID). Arthritis Res. Therapy 14, R129. doi: 10.1186/ar3859
Drakes, O., Tate, E., Rainey, J., and Brody, S. (2021). Social vulnerability and short-term disaster assistance in the United States. Int. J. Disaster Risk Reduc. 53:102010. doi: 10.1016/j.ijdrr.2020.102010
Falk, G., Carter, J. A., Nicchitta, I. A., Nyhof, E. C., and Romero, P. D. (2020). Unemployment Rates During the COVID-19 Pandemic: In Brief. Prepared by the Congressional Research Service (CRS). CRS Report R46554. Available online at: https://fas.org/sgp/crs/misc/R46554.pdf (accessed November 2020)
Forsythe, E., Kahn, L. B., Lange, F., and Wiczer, D. (2020). Labor demand in the time of COVID-19: evidence from vacancy postings and UI claims. J. Public Econ. 189:104238. doi: 10.1016/j.jpubeco.2020.104238
Freemantle, N., Calvert, M., Wood, J., Eastaugh, J., and Griffin, C. (2003). Composite outcomes in randomized trials: greater precision but with greater uncertainty? JAMA 289, 2554–2559. Available online at: https://www.ctsi.ucla.edu/education/files/view/training/docs/2003-currier.pdf (accessed July 29, 2021). doi: 10.1001/jama.289.19.2554
Gould, E., and Wilson, V. (2020). Black Workers Face Two of the Most Lethal Preexisting Conditions for Coronavirus-Racism and Economic Inequality. Economic Policy Institute: Washington, DC. Available online at: https://www.epi.org/publication/black-workers-covid/ (accessed July 29, 2021).
Hajat, A., Hsia, C., and O'Neill, M. S. (2015). Socioeconomic disparities and air pollution exposure: a global review. Curr. Environ. Health Rep. 2, 440–450. doi: 10.1007/s40572-015-0069-5
International Labor Organization (2020). Young workers will be hit hard by COVID-19's economic fallout. Available online at: https://iloblog.org/2020/04/15/young-workers-will-be-hit-hard-by-covid-19s-economic-fallout/ (accessed July 29, 2021).
Kong, E., and Prinz, D. (2020). Disentangling policy effects using proxy data: Which shutdown policies affected unemployment during the COVID-19 pandemic? J. Public Econ. 189:104257. doi: 10.1016/j.jpubeco.2020.104257
LeSage, J. P. (2008). An introduction to spatial econometrics. Rev. Econ. Ind. 123, 19–44. doi: 10.4000/rei.3887
Malhotra, K., Baltrus, P., Zhang, S., Mcroy, L., Immergluck, L. C., and Rust, G. (2014). Geographic and racial variation in asthma prevalence and emergency department use among medicaid-enrolled children in 14 southern states. J. Asthma 51, 913–921. doi: 10.3109/02770903.2014.930479
Miranda, M. L., Edwards, S. E., Keating, M. H., and Paul, C. J. (2011). Making the environmental justice grade: the relative burden of air pollution exposure in the United States. Int. J. Environ. Res. Public Health 8, 1755–1771. doi: 10.3390/ijerph8061755
Parolin, Z., and Curran, M. A. (2020). Monthly poverty to spike after expiration of the CARES act unemployment benefits. Poverty and Social Policy Fact Sheet. Center on Poverty and Social Policy, Columbia University. Available online at: https://www.povertycenter.columbia.edu/publication/2020/forecasting-poverty/expiring-cares-act-jan-poverty-rise (accessed July 29, 2021).
Parolin, Z., Curran, M. A., Matsudaira, J., Waldfogel, J., and Wimer, C. (2020). Monthly poverty rates in the United States during the COVID-19 pandemic. Poverty and Social Policy Discussion Paper. New York, NY: Center on Poverty and Social Policy. Available online at: https://www.povertycenter.columbia.edu/news-internal/2020/covid-projecting-monthly-poverty (accessed July 29, 2021).
Paul, K. I., and Moser, K. (2009). Unemployment impairs mental health: meta-analyses. J. Voc.Behav. 74, 264–282. doi: 10.1016/j.jvb.2009.01.001
Petterson, S., Westfall, J. M., and Miller, B. F. (2020). Projected Deaths of Despair During the Coronavirus Recession. Well Being Trust, 04-20. WellBeingTrust.org. Available online at: https://wellbeingtrust.org/wp-content/uploads/2020/05/WBT_Deaths-of-Despair_COVID-19-FINAL-FINAL.pdf (accessed July 29, 2021).
Qian, Y., and Fan, W. (2020). Who loses income during the COVID-19 outbreak? Evidence from China. Res. Soc. Stratif. Mobil. 68:100522. doi: 10.1016/j.rssm.2020.100522
Radulescu, C. V., Ladaru, G. R., Burlacu, S., Constantin, F., Ioanăș, C., and Petre, I. L. (2021). Impact of the COVID-19 pandemic on the romanian labor market. Sustainability 13:271 doi: 10.3390/su13010271
Salaffi, F., Peroni, M., and Ferraccioli, G. F. (2000). Discriminating ability of composite indices for measuring disease activity in rheumatoid arthritis: a comparison of the Chronic Arthritis Systemic Index, Disease Activity Score and Thompson's articular index. Rheumatology 39, 90–96. doi: 10.1093/rheumatology/39.1.90
Setia, M. S. (2016). Methodology series module 3: cross-sectional studies. Indian J. Dermatol. 61, 261–264. doi: 10.4103/0019-5154.182410
Smith, R. E., Vanski, J. E., and Holt, C. C. (1974). Recession and the employment of demographic groups. Brook. Papers Econ. Activity 3, 737–760. doi: 10.2307/2534252
Song, M. K., Lin, F. C., Ward, S. E., and Fine, J. P. (2013). Composite variables: When and how. Nurs. Res. 62, 45–49. doi: 10.1097/NNR.0b013e3182741948
Storper, M., and Scott, A. J. (2016). Current debates in urban theory: a critical assessment. Urban Stud. 53, 1114–1136. doi: 10.1177/0042098016634002
Svicher, A., and Di Fabio, A. (2021). Job crafting: a challenge to promote decent work for vulnerable workers. Front. Psychol. 12:681022. doi: 10.3389/fpsyg.2021.681022
Thebault, R., Tran, A. B., and Williams, V. (2020). African Americans are at Higher Risk of Death from Coronavirus—The Washington Post. The Washington Post. Available online at: https://www.washingtonpost.com/nation/2020/04/07/coronavirus-is-infecting-killing-black-americans-an-alarmingly-high-rate-post-analysis-shows/?arc404=true (accessed April, 07, 2020).
TN Department of Labor Workforce Development (2020). County Based Unemployment Rate for November 2020. Available online at: https://www.tn.gov/workforce/tennessee-economic-data-/labor-force-statistics/unemployment-rates.html (accessed July 29, 2021).
Tobler, W. (1970). A computer movie simulating urban growth in the Detroit region. Econ. Geogr. 46 (Supplement), 234–240. doi: 10.2307/143141
Tobler, W. (1979). “Cellular geography,” in Philosophy in Geography, eds S. Gale and G. Olsson (Dordrecht: Reidel), 379–386. doi: 10.1007/978-94-009-9394-5_18
Tubach, F., Ravaud, P., Baron, G., Falissard, B., Logeart, I., Bellamy, N., et al. (2005a). Evaluation of clinically relevant changes in patient reported outcomes in knee and hip osteoarthritis: the minimal clinically important improvement. Ann. Rheum. Dis. 64, 29–33. doi: 10.1136/ard.2004.022905
Tubach, F., Ravaud, P., Baron, G., Falissard, B., Logeart, I., Bellamy, N., et al. (2005b). Evaluation of clinically relevant states in patient reported outcomes in knee and hip osteoarthritis: the patient acceptable symptom state. Ann. Rheum. Dis. 64, 34–37. doi: 10.1136/ard.2004.023028
Vaz, E. (2021). COVID-19 in Toronto: a spatial exploratory analysis. Sustainability 13:498. doi: 10.3390/su13020498
Wanberg, C. R. (2012). The individual experience of unemployment. Annu. Rev. Psychol. 63:369–396. doi: 10.1146/annurev-psych-120710-100500
Weinstock, L. R. (2020). COVID-19: How Quickly Will Unemployment Recover? Prepared by the Congressional Research Service (CRS). CRS Report IN11460. Available online at: https://crsreports.congress.gov/product/pdf/IN/IN11460 (accessed July 29, 2021).
Worldometers (2021). COVID-19 Coronavirus Pandemic. Available online at: https://www.worldometers.info/coronavirus/country/us/
Appendix A
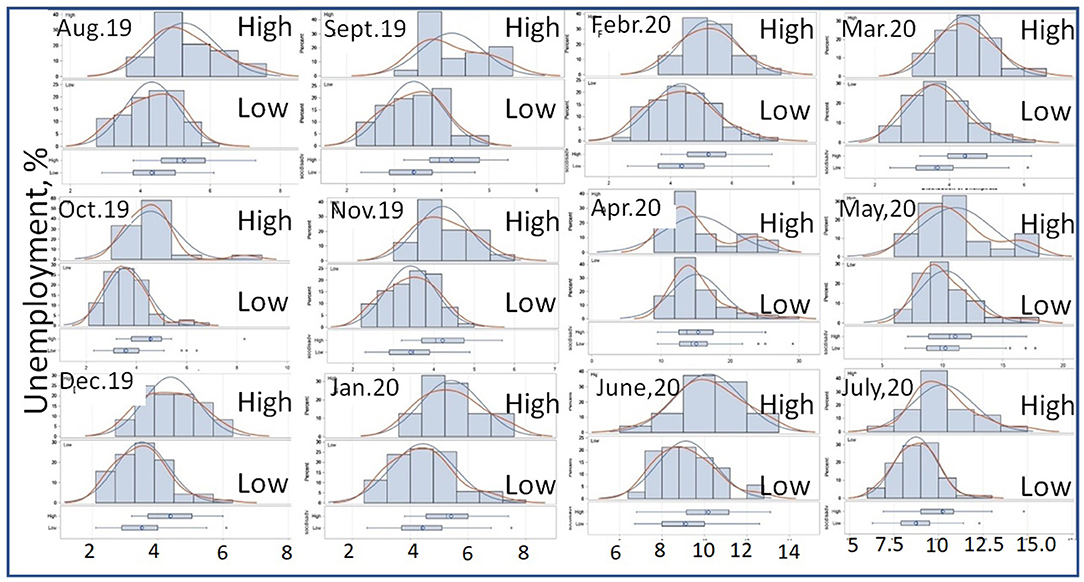
Figure A1. Histogram of unemployment rates in communities with high and low disadvantage August 2019–July 2020.
Keywords: unemployment, social disadvantage, social disadvantage index, COVID-19, Tennessee
Citation: Antipova A and Momeni E (2021) Unemployment in Socially Disadvantaged Communities in Tennessee, US, During the COVID-19. Front. Sustain. Cities 3:726489. doi: 10.3389/frsc.2021.726489
Received: 17 June 2021; Accepted: 22 July 2021;
Published: 16 August 2021.
Edited by:
Tiit Tammaru, University of Tartu, EstoniaCopyright © 2021 Antipova and Momeni. This is an open-access article distributed under the terms of the Creative Commons Attribution License (CC BY). The use, distribution or reproduction in other forums is permitted, provided the original author(s) and the copyright owner(s) are credited and that the original publication in this journal is cited, in accordance with accepted academic practice. No use, distribution or reproduction is permitted which does not comply with these terms.
*Correspondence: Anzhelika Antipova, YW50aXBvdmFAbWVtcGhpcy5lZHU=