- 1Department of Economics, Faculty of Economics, University of Economics in Prague, Prague, Czechia
- 2Department of Social and Economic Policy, Faculty of Economics, University of Economics in Prague, Prague, Czechia
This study focuses on the housing market in Central European countries. We analyze the housing prices and their sensitiveness to selected variables, especially regarding construction activity in the V4 countries and Austria. By employing a specification of a recursive VAR model for each country, we show that the most responsive to changes in construction activity is housing market in Austria. Meanwhile housing prices in the V4 countries do not exhibit any strong association with construction activity, perhaps due to under-supply of housing in the countries. The paper provides discussion on the variables dependencies explanations.
Introduction
The housing market is a hotly debated topic in the Czech Republic and other V4 countries. Many blame the ever-increasing prices on the lack of construction projects due to bureaucratic obstacles and complicated legislature (Machova et al., 2022; Pojar et al., 2022). Other authors also blame local residents for insufficient new construction projects (Hromada and Cermakova, 2021). However, little has been mentioned in the recent literature regarding the V4 countries. The prices were rising steadily since the recovery from the great recession until the COVID pandemic lockdowns. Researchers have claimed that the region, especially the Czech Republic, has been inflating a real estate bubble that was supposed to burst anytime (Venhoda, 2022). Now, we are in a different situation. The nominal interest rates are at their maximum in more than a decade, and the countries experience almost galloping inflation. The housing markets are facing a new reality. The high inflation and high mortgage costs would likely cause a decrease in demand for housing. However, the war in Ukraine and the subsequent influx of war refugees constitute an increased demand for housing. Čermáková et al. (2022) documented a decreased number of transactions on the property market in this period, while a drop in property prices was observed only in some regions (Hromada et al., 2023).
How much do we actually know about the relationship among the main forces in the housing market? How much does increased construction activity transmit into the housing market prices and rents? What are the dynamics between the rents and housing prices? This study strives to answer these questions. In this theory, new housing, as opposed to second-hand, is the driving force of housing market supply (Conefrey and Whelan, 2013; Charles et al., 2016). Therefore, one of the key price determinants should be construction activity in the country. If the construction activity does not significantly affect the price, it might be caused by a severe deficit in the housing supply which decouples changes in construction activity from changes in the housing prices. Another fact that the literature agrees on is that the markets are fragmented and prone to market-specific effects as found by Borowiecki (2009) and Duca et al. (2011). Therefore, we check the housing market dynamics with the VAR models calibrated specifically for each country. As far as we know, there is no study that attempts to do it in the region employing this technique and approach.
We employ a recursive1 VAR model on the data provided by Eurostat. The results show the relationship and predictors for housing prices and rents in the examined countries. One of the main results shows that the Austrian housing market is the only market in the region that is really sensitive to construction activity, which suggests that this is the only market out of the five countries that may not be severely undersupplied. This study aims to fill complete the current knowledge about the Central European housing markets and bring evidence of the effect of increased construction activity.
Literature review
The real estate price literature is ample as the demographical, macroeconomic, and bureaucratic environment changes over time similar to the conditions that affect the housing market. The factors that contribute to the housing market price formation are diverse. Here, we strive to provide a brief overview of the research.
The textbook describes the housing market and its equilibrium prices as set by the interaction of supply and demand. The demand is usually a function of income, price, rents, credit availability, wealth, and demographic factors. In comparison, supply is equal to the sum of the available inventory of houses (i.e., second-hand houses on the market) and the supply of new houses. The supply of new houses is a function of price, costs, credit availability, seasonal factors, and delays in the building process (Charles et al., 2016).
Glaeser et al. (2005) explained the ever-increasing US housing prices by several changing factors. From the 1950s until approximately 1970s, the prices soared due to the improving dwelling standards. In the later decades, the authors argued that obtaining permission to build constructions became more difficult, negatively affecting the market’s supply side and increasing prices. They also argued that the local residents gaining the power to influence, delay, or block new construction projects push the prices up. Some more recent research studies reached conclusions similar to the studies by Klement et al. (2022) and Lukavec and Kadeřábková (2017).
Jud and Winkler (2002) examined the determinants of housing prices in 130 metropolitan areas in the United States. The main factors in forming the price are demographic changes, changes in real income, construction costs, and interest rates. The housing prices vary across the areas due to the location-specific fixed effects. The size of the fixed effects is positively correlated with the local council construction imposed limits and restrictions on land availability within the area.
Yiu (2021) conducted a panel regression analysis on the European Union, the United Kingdom, the United States, Canada, Australia, and New Zealand in the time range from 2017 to 2021. He found that real interest rates have a significantly negative impact on housing prices as they lower the affordability of credit for households. The panel data study of monetary policies by Cihak et al. (2008) confirmed the finding of an inverse relationship between housing prices and real interest rates. Moreover, Chen and Lin (2021) found a link between a loose monetary policy and housing price increases. Duca et al. (2011) focused on credit availability, macroprudential policy, and house price expectation in their metastudy. However, he concluded that the environment differs across regions, and therefore, it is hard to make global conclusions. The conclusion that stands out from his study is that property prices are based on adaptive expectations. Hence, the housing markets are prone to bubbles. Among the most cited demand-side determinants of housing prices are GDP per capita, real interest rate, macroprudential policy, credit availability, demography, equity prices, and disposable income. However, each of the determinants has a different magnitude as the environment is very heterogeneous, even in such small countries as Switzerland Borowiecki (2009).
Literature divides the supply side of the housing price determinants into the newly built houses and second-hand supply. Conefrey and Whelan (2013) modeled the relationship between house price changes and different supply-side determinants. They claimed that the newly built dwellings have a much more substantial effect on the housing market than the changes in the second-hand housing supply.
Cadil (2009) employed a VAR model to analyze Czech real estate data and claimed that the flat market tends to form bubbles more often than detached houses. Hlavácek and Komarek (2011) claimed that housing prices are reliable predictors of rents in the Czech Republic.
Akkoyun et al. (2013) argued that transaction numbers are not correlated with housing prices in the short term, but they are positively correlated in the long term.
Jara and Romero (2016) provided a fascinating insight. They showed that banking integration in the Eurozone increases housing price synchronicity among the countries.
The era of COVID-19 brought about increased interest in the real estate prices literature. The prices in tourist areas decreased substantially, while other specific locations appreciated due to the pandemic. The rents also stagnated or declined. The decrease in prices was only significant during the first phase of the pandemic. Once the central banks dropped the interest rates to help restart the economy, real estate prices, including housing, experienced strong growth (Čermáková and Hromada, 2022).
V4 countries as a group are not analyzed often in the economic literature although there are some remarkable contributions worth mentioning. Studies by Adair et al. (2000) and Špirková and Ivanička (2009) pointed out the importance of foreign direct investments in the real estate markets in the V4 countries mentioning higher growth of prices together with higher risk premiums.
There is relatively scarce research on the direct effect of building construction activity on property prices despite the conclusion by economists that newly built houses have a decisive impact on the housing market supply curve. We believe that this is because many construction activities are directly proportional to credit availability (or monetary policy looseness). However, we believe there might be other strong determinants that might be more difficult to capture numerically, and these are government or non-government imposed limitations on construction projects. In other words, the monetary policy is not the only determinant of construction activity; hence, it surely is useful to find its impact on housing prices and rents.
Argument
Houses are long-term assets that provide services over their lifetime. Economic subjects can buy or rent houses. Therefore, there are separate yet related markets within the housing domain. This study is focused on housing prices to purchase and rents.
As in most markets, supply and demand determine housing prices. Economic theory says income, wealth, rental prices, credit availability, and demography impact the demand function for houses to purchase. The supply side consists of the already built houses, i.e., the second-hand market and newly built houses. New house supply is a function of price, material costs, credit availability, seasonal factors, and bureaucratic and similar obstacles. The second-hand buildings act as a substitute for the new ones, and their prices are inferred from the prices of the newly built properties (Conefrey and Whelan, 2013).
Researchers show that the number of transactions is a good predictor of housing prices in some cases. Akkoyun et al. (2013) claimed that the relationship is positive, while Arslan et al. (2015) suggested that it can also be negative in certain situations.
Similarly, rents impact the price equilibrium in housing markets through their effect on the demand for housing. The rents function as a substitute for purchasing a property and yield for the investors. Therefore, the property price/rent ratio plays a vital role in the demand for housing.
We also believe that construction activity plays a significant role in the supply of the housing market. Construction cycles are crucial to the market’s supply side as the newly built housing has a decisive impact on the prices. This part of supply has the power to adjust prices and is associated with whole market movements (Conefrey and Whelan, 2013). The study strives to clarify the housing market dynamics in the region. We analyze the V4 countries to see how relatively similar economies might have different housing market determinants. As the researchers suggest, each country’s market has a specific price formation condition (Roulac, 1996 or Coskun et al., 2020).
The study aimed to shed light on whether housing prices and rents can be predicted by construction activity and the number of transactions in the market.
Analysis and data
The data we use are provided by the Eurostat. The house prices index2 expresses inflation on any dwellings, and only the transacted properties are accounted for. We use the quarterly data with the index set at 100 in 2015. The availability of the data determines its length; however, all the time series finish in the fourth quarter of 2022. The house sales index3 functions as a measure of the number of transactions. It is from the same dataset as the house price index, with the same base year.
The rent inflation is an item extracted from the HICP index4, and the base is in 2015, but the frequency reported in the dataset is monthly. Therefore, we only take the values at the end of each quarter.
The construction data index5 provides information on the value-added volume in the construction sector output for section F of NACE classes filtered for “building constructions” only. The index also has a base in 2015.
Table 1 shows the Hungarian dataset. Unfortunately, the time series are relatively short, slightly lowering the model’s general usage. The problem with the data is that the Czech Republic and Slovakia do not have sufficient length of the time series for the transaction variable; therefore, in their case, we omitted the variable from the model. Hence, we have two groups of countries with two model specifications. The first one includes Austria, Hungary, and Poland, and the second one consists of the Czech Republic and Slovakia.
Figure 1 shows the situation in Hungary, where we can observe that housing prices, transaction numbers, and construction activity follow the same path while the rents grow steadily.
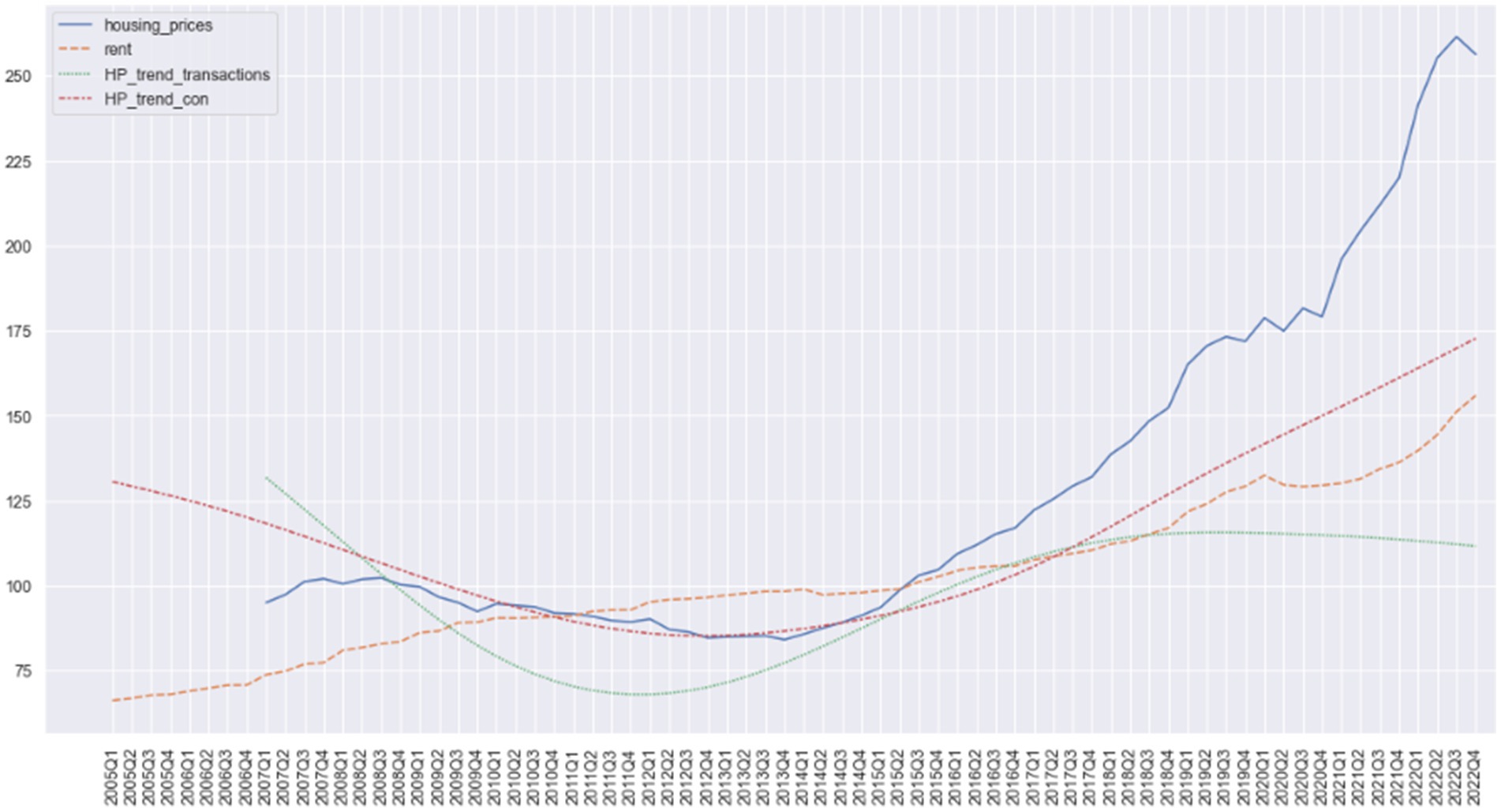
Figure 1. Hungarian time series with HP trend of transactions and constructions that otherwise would have a strong seasonality component (HP filter is only used for graph visualizations, where λ = 1,600).
Table 2 indicates that all the variables may be related, so we can proceed to investigate the relationship further.

Table 2. Granger Causality Matrix for the Hungarian time series, the matrix shows the p-values for H0: Variables in the columns (x) do not Granger cause the variables in rows (y).
We logged the variables and ran cointegration (Johansen) and stationarity tests for each country, further differencing until the series became stationary. Based on the ADF test, the Hungarian time series required two differencing to turn stationary. We selected the VAR order based on the best-fit criteria such as AIC, BIC, FPE, and HQIC.
As is visible from Table 3, we considered three lags in the Hungarian VAR model.
The estimated equations with only statistically significant on the 95% confidence level are as follows:
In the case of Hungary, we can observe slightly anti-intuitive results. First, the third lag of construction production is significantly positively associated with changes in housing prices. This might be explained by the earlier transmission of loose monetary policy toward the companies’ credit rather than the households as Hungary’s primary housing purchasers. The first lag of rents is negatively associated with housing prices, which means that the effect of competition between purchasing and renting an apartment is stronger than the investment effect. If the investment effect was stronger, the price/rent ratio should remain relatively stable; thus, the rents and house prices would be positively associated.
Interestingly, the second and third lags of construction production are positively associated with the rent change. The second lag of housing prices has also a positive impact on the change in rents.
Looking at the whole picture
We conducted the whole analysis for all the countries, and Figure 2 shows the significant (95% CI) coefficient for housing prices in all the examined countries. We can see a strong association between the first and second rent lags with housing prices in Poland. This would suggest a stronger investment effect in Poland than the competitive effect between housing prices and rents. The significant negative predictors of housing prices in Poland are the second lag of transaction and the third lag of construction although the coefficients are rather low (Gallin, 2008).
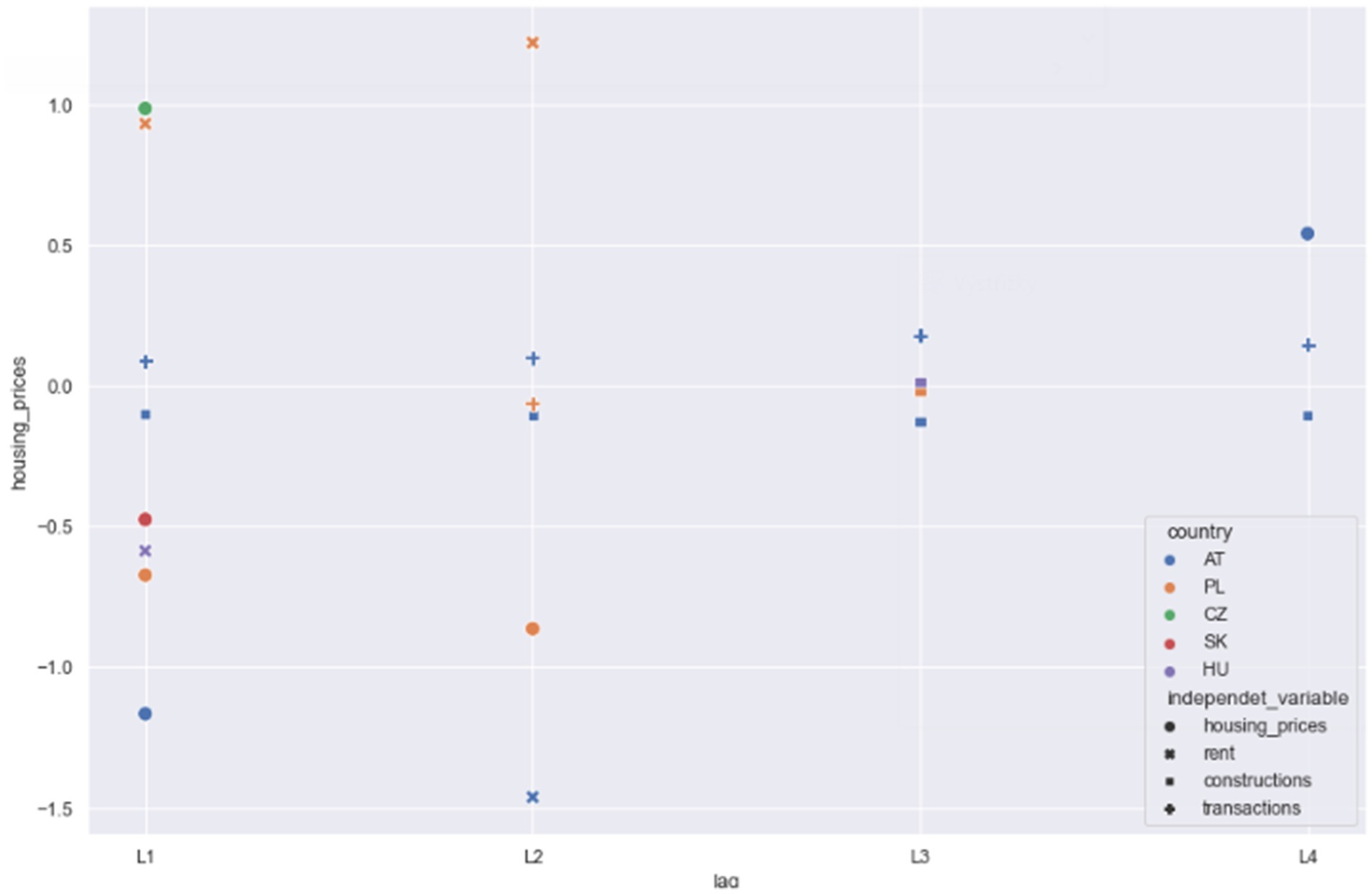
Figure 2. Significant predictors of housing prices across V4 + Austria countries. The X-axis shows lags, and the Y-axis shows coefficient values.
Positive predictors of housing prices in Austria are all the four transaction lags. This is explained as demand-driven price forming by Čermáková et al. (2022). Moreover, all four construction lags are significant and negative predictors of house prices in Austria. Construction productivity appears to be transmitted to the housing market via positive supply shocks created by construction.
The Czech Republic’s only significant predictor of housing prices is the same variable in the previous quarter. It might be a sign of very strong adaptive expectations.
The significant rent predictor in the case of Austria is the third lag of transactions. Interestingly, Hungary, Slovakia, and Poland rent first lags negatively affect the rents. It hints at relatively stable development of changes. If it grows in one period, it slows down in the next period (Figure 3).
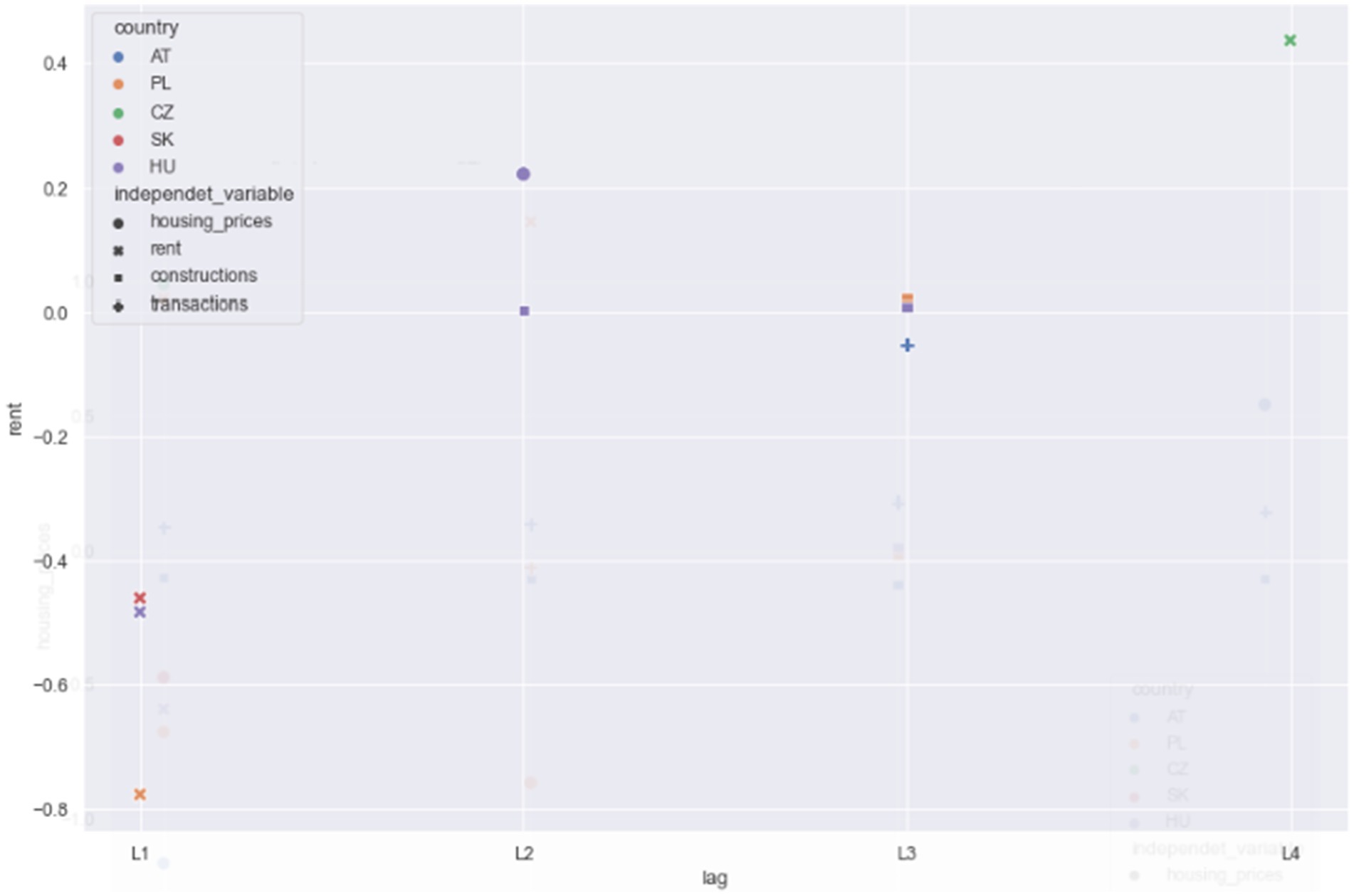
Figure 3. Significant predictors of rents across V4 + Austria countries. The X-axis shows lags, and the Y-axis shows coefficient values.
This analysis confirms the view of Conefrey and Whelan (2013) that each region’s housing market is specific and has region-specific characterization. Construction activity significantly impacts the housing market, especially in Austria, where its lags are negatively associated with housing prices. Moreover, transaction numbers contribute to the pricing formation in the housing market. The higher the number of transactions, the higher the prices in the following periods. In other countries, the results are less evident than in Austria. However, the patterns are discernible. The most significant predictor for housing prices in Poland is the development of rents. In the Czech Republic, housing prices changed in the last period. In Hungary, there are more, yet weaker predictors.
In Hungary, Poland, and Slovakia, the obvious conclusion from the rent analysis is that their changes tend to balance out, and the rents do not grow rapidly in more than one period.
If we assume that construction activity is a function of monetary policy, then our results support economists such as Kohn (2007) or Mishkin (2007) who downplay the immediate role of monetary policy in housing prices and who claim that housing prices move rather due to the market emotions and animal spirits than due to the movements in interest rates. The results are somewhat opposing to the studies by Chen and Lin (2021) and Duca et al. (2011), but this might be an overstatement as our research has limitations as well.
Conclusion
The analysis of the relationship among housing prices, rents, number of transactions, and construction activity is complicated by insufficient length of time series in some countries. With caution, we can draw some conclusions.
First, the analysis shows that the dynamic of the housing markets in Central Europe differs rapidly. The strong impact of construction activity in Austria shows that sufficient construction projects may alleviate inflation pressures in housing markets. The housing demand seems to play a substantial role in short-term price formation as the transaction numbers predict an increase in housing prices in Austria. In contrast, the most significant predictor of housing prices in the Czech Republic is the prices in the previous period. Housing prices in Poland are the most sensitive to changes in the rents. All these different results hint at the other economic structures of the Central European countries.
In the case of the Czech Republic, the data do not show that construction activity affects housing prices in the medium term. Several reasons might explain this. First, the constructions may take longer than 2 years on average, which was the maximum length that we tested the number of lags in the model. The other reason might be that the demand effect on the market is much more significant than the supply side effect.
Interestingly, the most significant predictor of the rents in three of the examined countries is the rent change in the previous period. One might explain it because we can see steadily but slowly increasing rents in all countries.
When making conclusions on the results of this study, one should be aware that construction activity determines only part of the stock of houses that are available to the housing market supply although the most important one as Conefrey and Whelan (2013) claim. However, these are the best data that are available at the moment. The second limitation also considers the data as we do not have sufficient comparable data for the number of transactions in the Czech Republic and Slovakia. Therefore, the models are not directly comparable to the other countries’ models.
The recommendations following from this analysis are that the V4 countries might find inspiration in Austrian policies regarding the housing market as it is desirable for housing market prices to respond to changes in the supply, i.e., to the construction activity. It is also likely indicative that all the V4 countries’ housing markets are likely undersupplied, and therefore, sudden changes in the construction activity do not project into market price changes.
Data availability statement
Publicly available datasets were analyzed in this study. This data can be found at: https://ec.europa.eu/eurostat/.
Author contributions
BK: Conceptualization, Funding acquisition, Methodology, Project administration, Supervision, Validation, Writing – review & editing. PŘ: Methodology, Project administration, Supervision, Validation, Writing – review & editing.
Funding
The authors declare financial support was received for the research, authorship, and/or publication of this article. This research was supported by VŠE IGS F5/4/2023.
Acknowledgments
We thank Ondrej Bednar for his valuable contributions.
Conflict of interest
The authors declare that the research was conducted in the absence of any commercial or financial relationships that could be construed as a potential conflict of interest.
Publisher’s note
All claims expressed in this article are solely those of the authors and do not necessarily represent those of their affiliated organizations, or those of the publisher, the editors and the reviewers. Any product that may be evaluated in this article, or claim that may be made by its manufacturer, is not guaranteed or endorsed by the publisher.
Footnotes
1. ^Stock, J. H. and Watson, M. W. (2001). Vector autoregressions. J. Econ. Perspect., 15, 101–115.
2. ^Code: PRC_HPI_Q.
3. ^Code: PRC_HPI_HSNQ.
4. ^Code: PRC_HICP_MIDX, item C041.
5. ^Code: EI_ISBU_Q.
References
Adair, A., McGreal, S., Smyth, A., Cooper, J., and Ryley, T. (2000). House prices and accessibility: The testing of relationships within the Belfast urban area. Housing studies 15, 699–716.
Akkoyun, H. C., Arslan, Y., and Kanik, B. (2013). Housing prices and transaction volume. J. Hous. Econ. 22, 119–134. doi: 10.1016/j.jhe.2013.02.001
Arslan, Y., and Kanık, B., and Köksal, B. (2015). Anticipated vs. Unanticipated house price movements and transaction volume. J. Hous. Econ. 28, 121–129.
Borowiecki, K. J. (2009). The determinants of house prices and construction: an empirical investigation of the Swiss housing economy. Int. Real Estate Rev. 12, 193–220. doi: 10.53383/100112
Cadil, J. (2009). Housing price bubble analysis–case of the Czech Republic. Prague Econ. Pap. 2009, 38–47.
Čermáková, K., and Hromada, E. (2022). Change in the affordability of owner-occupied housing in the context of rising energy prices. Energies 15:1281. doi: 10.3390/en15041281
Čermáková, K., Hromada, E., Henrique Filho, E. A., and Krulickỳ, T. (2022). The effects of homeownership on wealth distribution. Eur. J. Interdiscip. Stud. 14, 68–86. doi: 10.24818/ejis.2022.05
Charles, K. K., Hurst, E., and Notowidigdo, M. J. (2016). The masking of the decline in manufacturing employment by the housing bubble. J. Econ. Perspect. 30, 179–200.
Chen, S.-S., and Lin, T.-Y. (2021). Revisiting the link between house prices and monetary policy. B.E. J. Macroecon. 22, 481–515. doi: 10.1515/bejm-2021-0099
Cihak, M. M., Iossifov, M. P., and Shanghavi, A. (2008). Interest rate elasticity of residential housing prices. International Monetary Fund. Washington, DC
Conefrey, T., and Whelan, K. (2013). Supply, demand and prices in the US housing market. UCD Centre for Economic Research Working Paper Series.
Coskun, Y., Seven, U., Ertugrul, H. M., and Alp, A. (2020). Housing price dynamics and bubble risk: the case of Turkey. Housing Studies 35, 50–86.
Duca, J. V., Muellbauer, J., and Murphy, A. (2011). House prices and credit constraints: making sense of the US experience. Econ. J. 121, 533–551. doi: 10.1111/j.1468-0297.2011.02424.x
Gallin, J. (2008). The long-run relationship between house prices and rents. Real Estate Econ. 36, 635–658. doi: 10.1111/j.1540-6229.2008.00225.x
Glaeser, E. L., Gyourko, J., and Saks, R. E. (2005). Why have housing prices gone up? Am. Econ. Rev. 95, 329–333. doi: 10.1257/000282805774669961
Hlavácek, M. , and Komarek, L. (2011). Regional analysis of housing price bubbles and their determinants in the Czech Republic. Finance a Uver 61:67.
Hromada, E. , and Cermakova, K. (2021). Financial unavailability of housing in the Czech Republic and recommendations for its solution. Int. J. Eco. Sciences 2, 47–58. doi: 10.52950/Es.2021.10.2.003
Hromada, E., Heralová, R. S., Čermáková, K., and Piecha, M., and Kadeřábková, B. (2023). Impacts of crisis on the real estate market depending on the development of the region. Buildings 13:896. doi: 10.3390/buildings13040896
Jara, A., and Romero, N. (2016). International synchronicity of housing prices. Rev. de Anal. Econ. 31, 115–134. doi: 10.4067/S0718-88702016000200005
Jud, G. D., and Winkler, D. (2002). The dynamics of metropolitan housing prices. J. Real Estate Res. 23, 29–46. doi: 10.1080/10835547.2002.12091069
Klement, J., Kozák, J., Ševčík, M., and Pekárek, Š. (2022). Causes of opposition to residential development: a survey-framing experiment from the Czech Republic. Eur. Plan. Stud. 31, 306–327. doi: 10.1080/09654313.2022.2049709
Kohn, D. L. (2007). Success and failure of monetary policy since the 1950s: a speech at monetary policy over fifty years, a conference to mark the fiftieth anniversary of the deutsche Bundesbank, Frankfurt, Germany, September 21, 2007, Washington, DC. Board of Governors of the Federal Reserve System (US).
Kučerová, M. (2017). Financial and business cycles in central, Eastern and Southeastern Europe emerging markets.
Lukavec, M., and Kadeřábková, B. (2017). How much does a minute of commuting time cost? An examination of property prices in relation to distance to the city center in Prague, Czech Republic. Civ. Eng. J. 26:4. doi: 10.14311/CEJ.2017.04.0044
Machova, V., and Hromada, E., and Čermáková, K. (2022). Comparison of property price development in regions affected by mining with other regions of the CR. Acta Montanistica Slovaca 27, 491–504.
Mishkin, F. S. (2007). Housing and the monetary transmission mechanism. National Bureau of Economic Research Cambridge, MA
Pojar, J., Macek, D., Schneiderová Heralová, R., and Vitásek, S. (2022). Advances in costs optimization methods – key study of maintenance and restoration of cultural heritage. Int. J. Econ. Sciences 11, 163–178.
Roulac, S. (1996). The strategic real estate framework: processes, linkages, decisions. J. Real Estate Res. 12, 323–346.
Špirková, D. , and Ivanička, K. (2009). Dynamics of Bratislava urban and housing development. New models for innovative management and urban dynamics, 41.
Venhoda, O. (2022). Application of Dsti and Dti macroprudential policy limits to the mortgage market in the Czech Republic for the year 2022. Int. J. Econ. Sciences 1, 105–116. doi: 10.52950/Es.2022.11.1.007
Keywords: economics, housing, real estate, econometrics, Central Europe
Citation: Kadeřábková B and Řežábek P (2023) Does increased construction activity transmit into housing prices and rents? Evidence from the V4 countries and Austria. Front. Sustain. Cities. 5:1267964. doi: 10.3389/frsc.2023.1267964
Edited by:
Sławomir Kalinowski, Polish Academy of Sciences, PolandReviewed by:
Daniel Macek, Czech Technical University in Prague, CzechiaLucie Kurekova, CEVRO Institute, Czechia
Elroi Hadad, Sami Shamoon College of Engineering, Israel
Mariola Grzebyk, University of Rzeszow, Poland
Copyright © 2023 Kadeřábková and Řežábek. This is an open-access article distributed under the terms of the Creative Commons Attribution License (CC BY). The use, distribution or reproduction in other forums is permitted, provided the original author(s) and the copyright owner(s) are credited and that the original publication in this journal is cited, in accordance with accepted academic practice. No use, distribution or reproduction is permitted which does not comply with these terms.
*Correspondence: Božena Kadeřábková, b.kaderabkova@centrum.cz