- 1Department of Design and Environmental Analysis, Cornell University, Ithaca, NY, United States
- 2Department of Communication, Cornell University, Ithaca, NY, United States
What are strategies for the design of immersive virtual environments (IVEs) to understand environments’ influence on behaviors? To answer this question, we conducted a systematic review to assess peer-reviewed publications and conference proceedings on experimental and proof-of-concept studies that described the design, manipulation, and setup of the IVEs to examine behaviors influenced by the environment. Eighteen articles met the inclusion criteria. Our review identified key categories and proposed strategies in the following areas for consideration when deciding on the level of detail that should be included when prototyping IVEs for human behavior research: 1) the appropriate level of detail (primarily visual) in the environment: important commonly found environmental accessories, realistic textures, computational costs associated with increased details, and minimizing unnecessary details, 2) context: contextual element, cues, and animation social interactions, 3) social cues: including computer-controlled agent-avatars when necessary and animating social interactions, 4) self-avatars, navigation concerns, and changes in participants’ head directions, and 5) nonvisual sensory information: haptic feedback, audio, and olfactory cues.
Introduction
The Influence of Surrounding Environments on Behavior: Research Limitations
Our surrounding physical environment can influence behavior (Waterlander et al., 2015) as it “affords” (per Gibson, 1979) the activities of the broader social, political, and cultural world. By understanding how our surrounding environment affects occupants, researchers can identify evidence-based design approaches such as developing standardized evaluation toolkits (Joseph et al., 2014; Rollings and Wells, 2018), identifying design moderators (Rollings and Evans, 2019), and ultimately informing policy, including guidelines governing how facilities are built, renovated, and maintained (Sachs, 2018). By understanding how environments affect behaviors on a microbehavioral (i.e., unconscious) level, researchers can identify appropriate interventions (e.g., providing more sidewalks to encourage physical activity) and thereby inform the development of more effective informational and environmental interventions to improve desirable behavior (Marcum et al., 2018).
However, experimentally examining the influence of our surrounding environment on behavior is challenging. Real-life environmental manipulations may be costly and even politically challenging to implement (Schwebel et al., 2008). On the other hand, behaviors induced in conventional lab-based environments may not be generalizable to real-life environments (Ledoux et al., 2013). The influence of the surrounding environment on behaviors might be better understood (Ledoux et al., 2013) if researchers could immerse participants in complex physical and social environments that are ecologically valid while being highly controlled (Veling et al., 2016). Because of this, simulations are sometimes used to explore the relationship between environment and behavior (Marans and Stokols, 2013). Potential simulations can include mockups, sketches, photographs, models, and immersive virtual environments (IVEs). While CAVE automatic virtual environments (CAVEs, Cruz-Neira et al., 1993) and head-mounted displays (HMDs) have both been used to simulate such environments, the recent increase in the availability of consumer HMDs means that many more researchers can now use IVEs to answer questions about the effects of surrounding environments on behaviors. In this review, we reviewed and synthesized peer-reviewed research that used IVEs presented in HMDs for research on behavior influenced by our surrounding environment, with the aim of showcasing the solutions found by previous researchers. As virtual reality (VR) and IVEs will be frequently mentioned in this review, it is important to distinguish “VR” as the technology used to create “IVEs.”
Immersive Virtual Environment Tools for Human Behavior Research: Making the Case
Past research suggests that VR is a useful research tool to simulate real-life environmental features, as it allows researchers to immerse participants in hypothetical contexts and study their responses to controlled environmental manipulations otherwise difficult to examine in real-life environments (Parsons et al., 2007; Schwebel et al., 2008; Poelman et al., 2017; Ahn, 2018). Considerable work has demonstrated VR’s ability to elicit behavioral responses to virtual environments, even when the participant is well aware that the environment is not “real” as in demonstrations of the classic “pit demo” (Meehan et al., 2003).
In 2002, Blascovich and colleagues foresaw the advantages of VR as a tool for research in the social sciences. Although Blascovich’s original article discussed the use of VR as a tool for social psychology specifically, the advantages he describes for balancing experimental control and mundane realism and improving replicability and representative sampling have made it a tool of interest for researchers in several social science fields. VR has a high degree of realism: users tend to react to scenarios as if they were occurring in the real world. VR allows for a high degree of experimental control. Environments, events, and even virtual people can be programmed to appear to every user in the same way. Thus, VR has already been used extensively for diagnosis (Parsons et al., 2007), clinical education (Lok et al., 2006; Atesok et al., 2016), and clinical and experimental interventions (Difede and Hoffman, 2002; Wiederhold and Wiederhold, 2010; Wiederhold, 2017).
VR provides critical benefits over other methods available for behavior research (Schwebel et al., 2008). These advantages are particularly applicable when considering the influence of environments on behavior. VR has the potential to examine how people behave in real-life situations, without exposing participants to the risk and inconsistency of real-world environments (Blascovich et al., 2002). Participants can safely experience immersion in the virtual environment when the real environment is hazardous (Viswanathan and Choudhury, 2011), permitting researchers to ethically examine potentially dangerous behaviors (Schwebel et al., 2012). Additionally, it is relatively easy to manipulate environmental factors such as noise and crowding in virtual environments (Neo et al., 2019).
The Design of VR Environments for Behavior Studies: Research Gap
A prototype “is an artifact that approximates a feature (or multiple features) of a product, service, or system” (Otto and Wood, 2001; Camburn et al., 2017, p. 1) and “a virtual prototype is one which is developed (and tested) on a computational platform” (Camburn et al., 2017, p. 17). VR, especially its prototyping functions (i.e., the test-refinement-completion of designs using digital mockups, Ulrich and Eppinger, 2012), has been increasingly applied to behavior research. In this review, we examine VR’s potential to address environmental effects on behavior. In these cases, IVEs should be designed such that interactions between the individual and the virtual environment are as analogous as possible to interactions that would take place if the individual were in the actual environment, with the ultimate goal of developing a more robust way of examining the impact of the surrounding environment on behavior.
VR is generally considered to be a high-presence medium. Presence refers to the sense of “being there” in the VR environment (Heeter, 1992; Slater et al., 2009). While presence and immersion are terms sometimes used interchangeably, researchers have distinguished between the subjective psychological sense of presence and immersion, which can be considered a quality of the technology (Slater, 2018). A virtual reality setup that provides highly detailed visual content, spatialized sound, and haptic feedback (e.g., through vibrating controllers) would be considered more immersive than a scene rendered on a desktop monitor. Greater immersion is generally considered to increase presence (Cummings and Bailenson, 2016). Because consumer HMDs have reduced cost and expense while retaining a high sense of presence; it is plausible for many more researchers to use VR for prototyping applications; thus, we focus our recommendations on this larger pool of potential researchers.
While other considerable valuable works have used CAVE or desktop-based virtual environments to examine behavior, we have limited our analysis in this review to studies that use HMDs, to study behavior as it relates to the environment. The relatively lower cost and portability of new consumer HMDs mean that researchers who have not previously engaged with virtual reality now have the opportunity to use these systems for their research. This review aims to provide a summary of design considerations pulled from existing research in virtual reality that might prove useful to potential researchers who are not experienced in this area.
The qualities of HMDs provide special opportunities and constraints. HMDs combine portability with the ability to block out the surrounding environment, making them good for “in-the-wild” studies (Oh et al., 2016). The greater presence HMDs can provide is particularly important to these behavioral studies but comes with tradeoffs. Users do not see their real bodies, so researchers must decide whether or not to include avatars. HMDs allow users to experience spaces that may be larger than the physical space that they are actually in, meaning that users’ abilities to navigate must be programmed and controlled. Such environments allow for the ready tracking of behavioral data (Yaremych and Persky, 2019) and interaction with objects, but all of these interactions must be designed. In this review, we highlight the solutions and tradeoffs that previous researchers have made in this context.
Best Practices for Successful IVE-Based Experimental Studies
Heydarian and Becerik-Gerber (2017) describe “four phases of IVE-based experimental studies” and discuss best practices for consideration in different phases of experimental studies (Figure 1, see Heydarian and Becerik-Gerber, 2017 for in-depth discussion).
In this review, we focus on the “development of experimental procedure” phase, described by Heydarian and Becerik-Gerber as Phase 2. This includes the design and setup of the IVEs, especially considerations involving the level of detail required (i.e., factor(s) recognizable by participants; Heydarian and Becerik-Gerber, 2017). This may differ between studies and can include visual appearance, behavioral realism, and virtual human behavior. To meet the study objectives, a sense of presence is key, allowing study participants to feel “there” and thus behave as if they were in the actual environment.
However, information on the design process in Phase II can be hard to find. Researchers typically describe the “final environment” they have designed in publications, but justifications for the many design decisions they have made in the development of the virtual environment are less common, probably at least in part due to publication length limitations. However, this information is extremely valuable. The following review expands on the work by Heydarian and Becerik-Gerber (2017) by reviewing and synthesizing strategies from 18 studies using IVEs for behavior research. In addition, we have created a wiki (https://osf.io/gyadu/) to collect citations for other papers that use IVEs for this purpose so that this database can be updated. We hope this synthesis and this wiki will be an additional resource for researchers new to this space to build on the knowledge of previous researchers to make informed choices when they are designing such IVEs.
Methods
Inclusion Criteria
Inclusion criteria were as follows: (a) the study examined nonclinical populations, (b) the study used IVEs presented via HMDs to examine behaviors influenced by the environment, (c) users experienced a virtual environment that plausibly represented an actual environment, and (d) the study provided sufficient details on how it designed and set up the IVE.
Review Procedure and Data Extraction
After consultation with a research librarian, we applied the following keywords (Table 1) and MeSH search terms: virtual reality, behavior, prototype, and design (Table 2). Terms were combined with the Boolean operators “and” and “or” to identify relevant studies. We did not conduct searches separately by specific behaviors, e.g., “grocery shopping” or environments, e.g., “grocery store,” but narrowed down the results from an initial search focusing on VR. Using PubMed, Web of Science, Scopus, and Google Scholar databases, we conducted a systematic search to identify English-language, peer-reviewed publications, and conference proceedings on experimental and proof-of-concept studies that described the design, manipulation, and setup of the IVEs to examine behaviors influenced by the environment. The search targeted articles were published before May 15, 2020 (i.e., no lower bound cutoff date). Reviewer 1 assessed retrieved texts to determine if they met the inclusion criteria. If a study was deemed potentially eligible, the full article was retrieved for further assessment and inclusion. A second reviewer screened all included articles. The selection was finalized after discussion and consensus between reviewers. We identified additional studies by searches of the references provided in the included publications (Greenhalgh and Peacock, 2005). Once the finalized list of papers was determined, these data were extracted: first author, year, behavior, environment, and strategies in designing IVEs for behavior research.
Data Synthesis and Analysis
Due to the heterogeneity of research in the field, quantitative synthesis was not planned. To conduct a narrative synthesis, two reviewers independently categorized studies based on strategies in designing IVEs for behavior research. Based on past use of VR in behavior research, our research question was as follows: What are strategies that researchers identified as effective in designing IVEs for behavior research?
Results
We screened 203 citations and reviewed 61 full texts, of which 18 met the inclusion criteria (Figures 2 and 3). All the studies we found were in the range of 2015–2020. Exclusion criteria were as follows: (a) the study examined clinical populations (57 citations), (b) the study did not use IVE presented via HMDs to examine behaviors influenced by the environment (32), (c) the study did not use VR to create a virtual environment (28), and (d) the study did not provide sufficient details on how it designed and set up the IVE (50). Case studies that did not describe behavioral outcomes were thus also excluded (Lovreglio et al., 2018). As some studies were rejected for more than one reason, the sum of studies is greater than 142. For brevity, the types of behaviors and environments are summarized in Table 3.
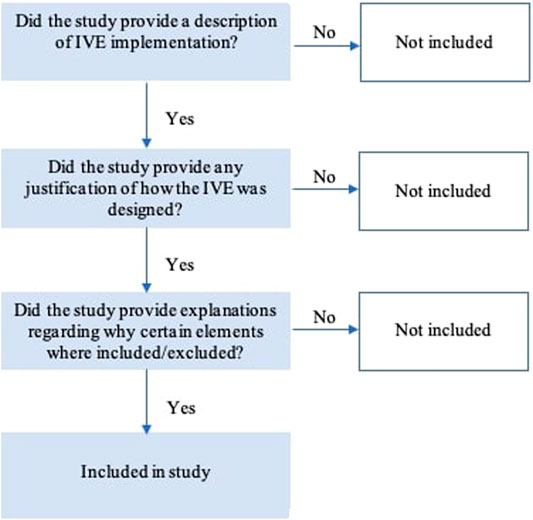
FIGURE 3. A yes/no flowchart to decide if a study provided sufficient details on how it designed and set up the IVE.
Discussion
Building from recent research using IVEs as a prototyping tool to examine the relationship between the environment and human behaviors, this review provides strategies drawn from previous IVEs designed to understand environments’ influence on behaviors. This discussion is grounded in our analysis of what design researchers have reported as effective in previous experiments and on the outcomes in the reviewed studies. Five key categories emerged from this analysis: (1) the appropriate level of detail (primarily visual) in the environment, (2) context, (3) social cues, (4) participant tracking and rendering, and (5) nonvisual sensory information (Figure 4. A high-resolution version of this figure is available as Supplementary Figure S1).

FIGURE 4. Flow diagrams summarizing the strategies for the design of IVEs to understand environments’ influence on behaviors.
In this review, many recommendations (e.g., including dynamic representation of the participant’s body) are dependent on the research goal and tradeoffs (e.g., additional cost, development time, and technical capabilities). Hence, before any meaningful discussion of what researchers have reported as effective in designing IVEs for environment-behavior research, all strategies and decisions should be evaluated in the context of two design principles: (1) the research goal and (2) potential tradeoffs to design choices, such as development cost and scalability. Additionally, the recommendations featured in this review were specific examples used by researchers in past studies. It is important to note that there are various ways to approach these considerations that may or may not have been discussed in past studies.
Level of Detail in the Environment
Important Commonly Found Environmental Accessories
Researchers proposed that IVEs should incorporate typical elements (features such as furniture or features) of actual environments. In a study examining gambling behavior, Dickinson et al. (2020) included items such as paper slips, pens, and stools in the betting shop (Figure 5).
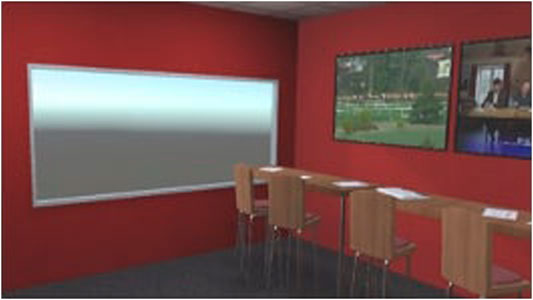
FIGURE 5. Dickinson et al. (2020) included items such as paper slips, pens, and stools in the betting shop.
Realistic Textures
Realistic textures can be created by taking photos of the actual product before attaching it to the virtual objects. This is particularly important if participants are expected to move around the environment and pick up objects to examine them (Morrongiello et al., 2015; Lombart et al., 2019; Siegrist et al., 2019). For example, in a study examining purchase behavior, Lombart et al. (2019) used real product textures with high-resolution pictures from real products (Figure 6).
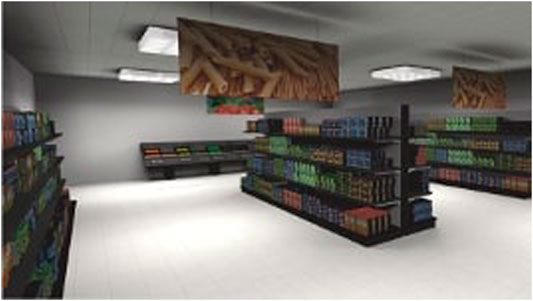
FIGURE 6. Lombart et al. (2019) used real product textures with high-resolution pictures from real products.
Computational Costs Associated With Increased Details
Realistic rendering can increase an individual’s sense of presence in the virtual environment. According to Slater et al. (2009), visual realism has two components: geometric realism (i.e., virtual and real objects look alike) and illumination realism (i.e., the fidelity of the lighting model). However, building complex IVEs can be time-consuming, requires heavy computational algorithms, and may even decrease frame rate when participants view the IVE (Sobhani et al., 2017; Lin et al., 2020).
Researchers must then decide which extraneous features in the surrounding environment are not key to the research question and can be removed while maintaining ecological validity. For example, in examining distracted pedestrian crossing behavior, Sobhani et al. (2017) excluded other pedestrians and cyclists, focusing on vehicles and the participant (Figure 7). Before finalizing the research design and hypotheses, researchers should always consider the complexity of their desired IVE since the impact of a “suboptimal” IVE (e.g., an IVE that lacks critical details of the surrounding environment) could have significant effects on participants’ behaviors (Lin et al., 2020).
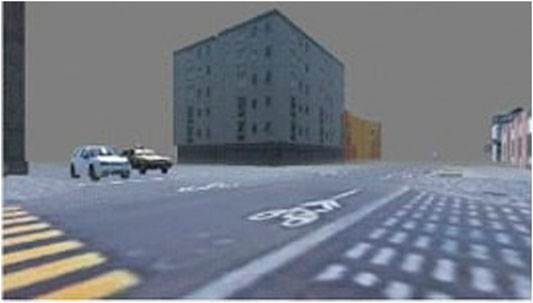
FIGURE 7. Sobhani et al. (2017) excluded other pedestrians and cyclists, focusing on vehicles and the participant.
Minimizing Unnecessary Detail
IVEs may be perceived as more immersive than lab conditions (Dickinson et al., 2020). However, high levels of visual realism (i.e., consistency between one’s virtual vs. real-world experience Witmer and Singer, 1998) might increase expectations for other aspects (e.g., nonvisual and tactile) of the simulation to be equally realistic (Dickinson et al., 2020). This raises a critical consideration in designing IVEs for research: while realistic IVEs generally increase the ecological validity of research, some kinds of realism (e.g., adding avatars) inherently increase the complexity and confounding variables associated with experimental environments. Here, we define a confounding variable as an “extraneous variable whose presence affects the variables being studied so results do not reflect the actual relationship between the variables under study” (Pourhoseingholi et al., 2012, p. 79).
Too much detail can reintroduce some challenges associated with research in actual environments (Dickinson et al., 2020). Before designing an IVE, researchers can use input from past research, end-users, and subject experts to identify possible confounds and evaluate the risks and benefits of including a given feature into the IVE (Persky et al., 2018). For example, in a study examining navigation behaviors in powered wheelchair driving simulators, Alshaer et al. (2017) did not tell participants about the passability of the doorframes or gaps to preserve realism and did not include furnishings or decorations to avoid distractions and to remove cues to size and distance provided by familiar objects (Figure 8).
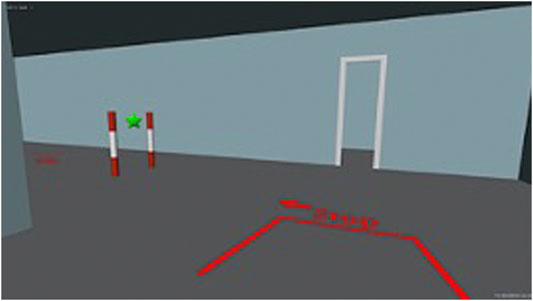
FIGURE 8. Alshaer et al. (2017) did not tell participants about the passability of the doorframes or gaps to preserve realism. Furnishings or decorations were excluded to avoid distractions.
Context
Before creating IVEs to examine behaviors, researchers need to identify what contextual cues are necessary and evaluate whether VR can incorporate all the required elements. Social elements involve perhaps the most complicated tradeoffs, discussed in the following section.
Contextual Elements
In IVEs, participants give special attention to objects most relevant to looking behaviors, such as windows (Lee et al., 2019). Participants may also engage in more exploratory behaviors (e.g., looking intensely at objects irrelevant to the research questions), behaviors that may not be as salient in actual environments (Lee et al., 2019). For example, in Lee et al. (2019), participants spent more time looking at “see-through” surfaces such as windows (Figure 9). Perhaps, participants learned that available behaviors in IVEs were mostly “looking around” and focused their attention on windows, instead of objects with functionality (e.g., portability) in the real but not virtual world (Lee et al., 2019). Participants’ attention to display surfaces or windows highlights the notion of multiple embeddedness during interactions in virtual environments (Lee et al., 2019). A person’s experience in an IVE is still embedded in the surrounding environment (Lee et al., 2019). For example, when participants allocate visual attention to window surfaces displaying extra information regarding the exterior, they may be creating a mental model of the IVE’s location and themselves in the virtual space (Lee et al., 2019). Researchers should consider whether such features (e.g., windows) should be included or excluded as participants may devote unnecessary attention to “checking them out.” For example, including a “skybox” and trees as a surrounding exterior makes users think that they are inside a building with windows (i.e., virtual realism) (Morrongiello et al., 2015; Nordbo et al., 2015).
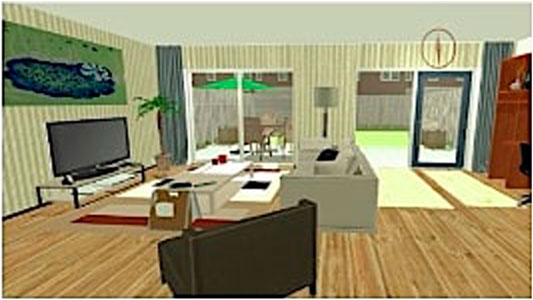
FIGURE 9. In Lee et al. (2019), participants spent more time looking at “see-through” surfaces such as windows.
Cues
Stimulatory and instructional cues can provide relevant information and navigation within IVEs. In a study examining energy consumption behavior, Saeidi et al. (2019) used stimulatory cues related to the spatial and temporal configuration of the IVE to simulate relevant information about the IVE, such as the sense of time, weather, and crowding. Specifically, Saeidi et al. (2019) used lighting and moving traffic to evoke a sense of time. However, researchers should consider using such cues in moderation. For example, in a study examining evacuation behavior by Tucker et al. (2018), smoke was incorporated only to enhance participants’ anxiety and perceived hazard and not to the extent where the simulating impacts of smoke hinder visibility (Tucker et al., 2018) (Figure 10).
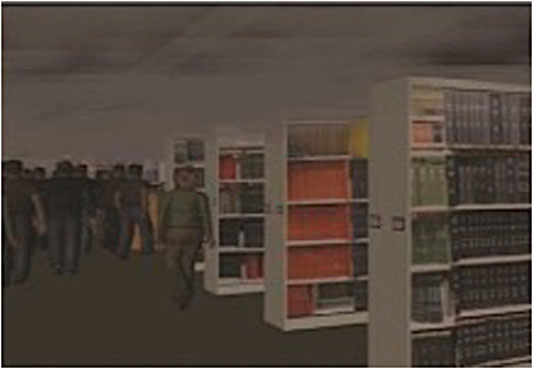
FIGURE 10. Tucker et al. (2018) incorporated smoke only to enhance participants’ anxiety and perceived hazard and not to the extent where the simulating impacts of smoke hinder visibility.
Saeidi et al. (2019) also used instructional cues to help participants navigate within the IVE and distinguish and interact with operable virtual objects. For example, as participants hover the controller over operable objects, they would start blinking, signaling activation (Saeidi et al., 2019) (Figure 11).
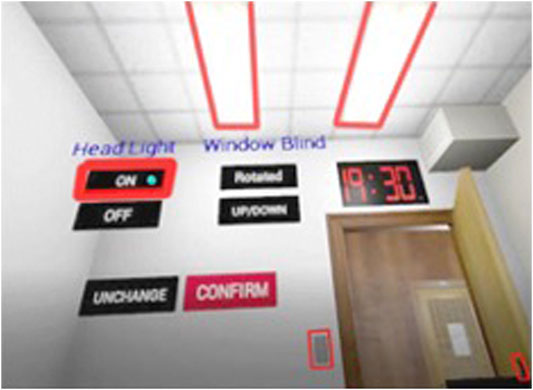
FIGURE 11. Saeidi et al. (2019) used instructional cues to help participants navigate within the IVE and distinguish and interact with operable virtual objects.
Animating Interactions
In an IVE, a feature (e.g., traffic and avatar) may be moving at a simulated speed. Identifying and creating the appropriate speed may be important for research examining behaviors such as road crossing and emergency evacuation. To reduce potential confounders (i.e., walking speed), in this example, the researchers kept the demonstration avatar’s walking speed constant based on the staff’s walking speed (Shi et al., 2019). If possible, researchers should use pretests to ensure that calibration accuracy between participants’ actual movements and virtual animations is acceptable to participants (Shi et al., 2019). Specifically, there should be minimal movement mismatches in the virtual environment when participants rotate their bodies as these mismatches can affect their feelings of presence (Shi et al., 2019). In a study by Lin et al. (2020) examining evacuation behaviors, the range of avatar speeds depended on their age and gender.
Social Cues
If social aspects are important, researchers need to find ways to incorporate associated cues or agents into the IVE (Neo et al., 2019).
Include Computer-Controlled Agent-Avatars When Necessary
Computer-controlled agent-avatars may enhance the realism of IVEs and facilitate the examination of behaviors such as distractions and emergency evacuation. However, avatars may also unduly distract participants from engaging in the behavior of interest. For example, in Dickinson et al. (2020), no agent-avatars were placed in the IVE, so as not to distract participants playing the electronic gaming machine.
Animating Social Interactions
In general, introducing avatars of the self or of others requires a number of decisions. If agent-avatars are introduced, they must be animated appropriately. A number of animations are available for free or for purchase (e.g., Mixamo.com) can be designed using modeling programs or can be generated from human motion (Gonzalez-Franco et al., 2020). These animations can be automatically triggered by the actions of the participant, but sometimes a “wizard-of-Oz” scenario, in which a key digital element is actually controlled by a human user (for example, see Lucas et al., 2019) may be more useful. For example, in examining fall risk, instead of relative to playing a prerecorded animation, Shi et al. (2019) used a lab staff member to control another avatar in real time as the human-controlled avatar seemed more “natural and acceptable” to the participants.
Participant Tracking and Rendering
Self-Avatars
Self-avatars can enhance a user’s sense of presence (Fox and Bailenson, 2010). People tend to experience an elevated sense of presence when there is a virtual representation of oneself in the VR environment and when other users (avatars) recognize them (Nash et al., 2000; Fox and Bailenson, 2010).
When investigating certain behaviors, such as pedestrian road crossing, it may be helpful for a participant to see a dynamic representation of their body to increase their sense of presence (Kooijman et al., 2019). Kooijman et al. (2019) propose that the representation needs to be realistic (i.e., not robot-looking) and gender-specific and provides synchronous tactile-visual feedback to evoke a full-body illusion (Kooijman et al., 2019). However, as other researchers have found, a first-person perspective alone can aid in creating a useful body-ownership illusion (Slater et al., 2010).
As suggested by Kooijman et al. (2019), motion suits are increasingly used in road safety research and may become more common for other types of VR-based research. Different headsets may have different capabilities to track and represent user behavior. Some newer HMDs, for example, the Oculus Quest, now offer the ability to track and render users’ hands without requiring them to hold hand controllers.
Navigation Concerns
Navigation via real walking can enhance one’s sense of presence in IVEs (Shi et al., 2019); however, this option is constrained by the physical space available (Iryo-Asano et al., 2018; Kooijman et al., 2019). For example, in Kooijman et al. (2019), which examined pedestrian crossing behaviors, participants were asked not to walk beyond the third “zebra stripe” due to the space constraints of the laboratory. Given this conflict, allowing participants to control their walking direction and speed can help them feel as if they are walking in VR environments (Morrongiello et al., 2015; Marcum et al., 2018).
Changes in Participants’ Head Directions
Mizuchi and Inamura (2018) evaluated human behavior difference with a restricted field of view in real and IVEs and found that large changes in the head direction and some head-mounted display properties affect spatial perception about recognition speed and manipulation skill in IVEs. A scenario in which participants must frequently and dramatically change head direction may be unfavorable for observing behaviors in IVEs. In a study by Shi et al. (2019) examining fall risk behaviors, slight movement mismatches in the IVE when participants rotate their heads can affect their sense of presence (i.e., being there in the simulated world) (Figure 12). IVEs and scenarios should be designed to avoid large changes in participants’ head direction, for example, putting participants in a narrow space (Mizuchi and Inamura, 2018).
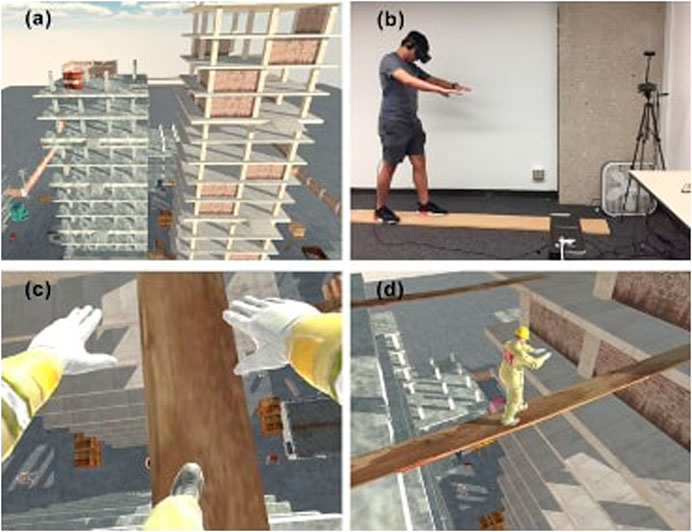
FIGURE 12. Shi et al. (2019) highlighted that slight movement mismatches in the IVE when participants rotate their heads can affect their sense of presence. Shi et al. (2019) used real planks to provide haptic feedback while participants walked on “virtual planks.”
Nonvisual Sensory Information
The realism of virtual simulations was highly rated in various studies; however, most simulations lack some characteristics of the real environment (e.g., haptic feedback, sounds, smell, and other sensory contents) (Ledoux et al., 2013). As with other elements that might increase immersion but create technical difficulties, adding additional sensory modalities requires the careful consideration of tradeoffs.
Haptic Feedback
Some VR-based studies integrate real elements besides the VR displays, for example, to provide passive haptic feedback. For example, in a study examining fall risk behaviors, Shi et al. (2019) used real planks to provide haptic feedback while participants walked on “virtual planks.” With such integration, the real and virtual element (e.g., a plank) should be carefully calibrated to enhance realism, and yet address safety issues (e.g., a participant falling off a plank) (Shi et al., 2019). To enhance setups with real and virtual elements, avatars may be included to reflect any interactions observed with the real elements, such as falling off a real plank (Shi et al., 2019).
Audio
IVEs may include relevant (i.e., typical of the actual environment) audio to isolate viewers from the real world (Dickinson et al., 2020). Researchers should first determine if the sounds can enhance the IVE’s realism or if they might confound the study (Morrongiello et al., 2015). In a study examining evacuation behaviors, Markwart et al. (2019) included sounds of a storm (i.e., rain; thunder), wind, cars driving by, splatter sounds from the water fountain, and footsteps. To make virtual experiences more realistic, certain sounds, such as avatars' voices, should be gender-specific (Markwart et al., 2019).
Olfactory Cues
None of the studies included olfactory cues in the IVE. However, some studies cited the lack of characteristics of a real food choice environment (e.g., food smell) as a key limitation (Ledoux et al., 2013; Marcum et al., 2018). For example, the lack of olfactory feedback might become a factor if participants were asked to pick up the virtual food (Ledoux et al., 2013; Marcum et al., 2018). Olfactory cues have been used in studies examining virtual food; for example, Li and Bailenson (2018) explored the role of olfactory cues of a virtual donut on satiation and eating behavior and Stelick et al. (2018) developed a proof-of-concept study to determine if the pungent flavor notes of blue cheese may be enhanced by showing participants a virtual dairy barn. In Li and Bailenson (2018), the authors attached a scented cotton bud to the front of the HMD with Velcro strips at the exact same time the participants saw and smelled a virtual donut.
Overall, more targeted haptic, auditory, and olfactory feedback will likely become possible with the rapid growth of VR technology. Nonvisual sensory information may be more important if participants engage in, for example, food selection behavior, where aesthetic and reward-oriented features may be more important (Marcum et al., 2018). Adding nonvisual sensory information will also help researchers who need to capture the potential responses of a more diverse participant pool, for example, participants with low vision (Zhao et al., 2019).
Limitations of This Review
This review summarizes published information about researchers’ design decisions when creating IVEs to test the effect of environments on behavior. However, we recognize that much valuable information remains unpublished due to page limits and other constraints of academic publishing. While we hope this paper can be useful, especially for researchers less familiar with this area, providing experienced researchers with resources to share their design experiences will greatly aid the research community. This would allow for the inclusion of projects that did not include behavioral data at the time of publication of this paper but that are likely to contain valuable information, for example, (Lovreglio et al., 2018).
Due to the heterogeneity of human behaviors, we could not design a search strategy for each behavior (e.g., human behavior in a supermarket; human behavior in pedestrian crossing). Our search returned limited results due to our strict inclusion criteria (i.e., a study must use HMDs) and the resulting small number of studies. Our decision to limit this review to studies using HMDs may have reduced the breadth and depth of our analysis, as well as the variety of environments and behaviors. Variations in research questions and perspectives also limited our results, despite our best efforts to systematically identify and categorically include relevant studies. Some studies met the inclusion criteria but provided no discussion on how to design IVE to examine behaviors. Due to a reduced pool of studies from which to draw conclusions, some environmental considerations highlighted in this review (e.g., haptic feedback; olfactory cues) depended on the intended application and purpose. By using the term “human behavior” relatively broadly as the starting point, recommendations can be generalized to a larger and more diverse audience including researchers, designers, and practitioners.
Conclusion
Our review analyzed the use of IVEs in behavioral science research and provided design considerations when prototyping IVEs for research on the interaction between the environment and human behavior. We found that rather than trying to replicate every aspect of the physical environment, researchers carefully considered the level of detail needed for each element. We also found that interdisciplinary collaboration is required to conceptualize, plan, design, and execute a VR study to examine behaviors (Metsis et al., 2019; Neo et al., 2019). We provided some sample workflows illustrated with examples from published work.
With these design considerations gleaned from experienced VR researchers in mind, other behavioral scientists may be able to better use VR to develop designs in order to examine behaviors. Ultimately, by enhancing our knowledge of the design and use of VR, researchers can better understand environmental factors that influence behavior and how to effectively alter environmental and/or policy-based interventions. However, the valuable information about the design decisions researchers makes in creating these virtual environments can be hard to find. Studies currently available only as pilots or case studies (e.g., Lovreglio et al., 2018), if used in the future to examine and capture behavioral data, provide promising opportunities to help researchers better understand the environments’ influence on behaviors.
While this review aims to provide a summary of relevant research up to the present, finding ways for more researchers to be able to easily share their hard-won knowledge is an important need for the community of current and interested researchers.
Data Availability Statement
The original contributions presented in the study are included in the article/Supplementary Material; further inquiries can be directed to the corresponding author.
Author Contributions
JN was involved in conceptualization, data curation, formal analysis, methodology, project administration, writing-original draft preparation, review, and editing. AW and MS were involved in formal analysis, methodology, writing-original draft preparation, review, and editing.
Conflict of Interest
The authors declare that the research was conducted in the absence of any commercial or financial relationships that could be construed as a potential conflict of interest.
Acknowledgments
The authors would like to acknowledge the help of Ms. Amelia Kallaher (Applied Social Science Librarian, Cornell University) for developing the search strategy.
Supplementary Material
The Supplementary Material for this article can be found online at: https://www.frontiersin.org/articles/10.3389/frvir.2021.603750/full#supplementary-material
References
Ahn, S. J. (2018). Virtual exemplars in health promotion campaigns, J. Media Psychol. Theor. Methods Appl. 30, 91–103. doi:10.1027/1864-1105/a000184
Alshaer, A., Regenbrecht, H., and O'Hare, D. (2017). Immersion factors affecting perception and behaviour in a virtual reality power wheelchair simulator. Appl. Ergon. 58, 1–12. doi:10.1016/j.apergo.2016.05.003
Atesok, K., Satava, R. M., Van Heest, A., Hogan, M. V., Pedowitz, R. A., Fu, F. H., et al. (2016). Retention of skills after simulation-based training in orthopaedic surgery. J. Am. Acad. Orthop. Surg. 24 (8), 505–514. doi:10.5435/JAAOS-D-15-00440
Camburn, B., Viswanathan, V., Linsey, J., Anderson, D., Jensen, D., Crawford, R., et al. (2017). Design prototyping methods: state of the art in strategies, techniques, and guidelines. Des. Sci. 3, E13. doi:10.1017/dsj.2017.10
Cruz-Neira, C., Sandin, D. J., and DeFanti, T. A. (1993). Surround-screen projection-based virtual reality: the design and implementation of the CAVE,” in Proceedings of the 20th annual conference on computer graphics and interactive techniques, 135–142.
Cummings, J. J., and Bailenson, J. N. (2016). How immersive is enough? A meta-analysis of the effect of immersive technology on user presence. Media Psychol. 19 (2), 272–309. doi:10.1080/15213269.2015.1015740
Dickinson, P., Gerling, K., Wilson, L., and Parke, A. (2020). Virtual reality as a platform for research in gambling behaviour. Comput. Hum. Behav. 107, 106293. doi:10.1016/j.chb.2020.106293
Difede, J., and Hoffman, H. G. (2002). Virtual reality exposure therapy for world trade center post-traumatic stress disorder: a case report. Cyberpsychol. Behav. 5 (6), 529–535. doi:10.1089/109493102321018169
Fox, J., and Bailenson, J. N. (2010). The use of doppelgängers to promote health and behavior change. Cyberther. Rehabil. 3 (2), 16–17. doi:10.1037/e530522011-003
Glotzbach, P. A., and Heft, H. (1982). Ecological and phenomenological approaches to perception. Hoboken: Nous, 16108–16121.
Gonzalez, G. M., Lehr, J., Krämer, N., and Gratch, J. (2019). “The effectiveness of social influence tactics when used by a virtual agent,”in Proceedings of the 19th ACM international conference on intelligent virtual agents, 22–29.
Gonzalez-Franco, M., Egan, Z., Won, G. M., and Wang, G. M. (2020). MoveBox: democratizing MoCap for the Microsoft Rocketbox Avatar Library (IEEE AIVR 2020). Berlin: Springer.
Greenhalgh, T., and Peacock, R. (2005). Effectiveness and efficiency of search methods in systematic reviews of complex evidence: audit of primary sources. BMJ 331 (7524), 1064–1065. doi:10.1136/bmj.38636.593461.68
Heeter, C. (1992). Being there: the subjective experience of presence. Presence Teleop. Virt. Environ. 1 (2), 262–271. doi:10.1162/pres.1992.1.2.262
Heydarian, A., and Becerik-Gerber, B. (2017). Use of immersive virtual environments for occupant behaviour monitoring and data collection. J. Build. Perform. Simul. 10 (5–6), 484–498. doi:10.1080/19401493.2016.1267801
Hurwitz, J., Loomis, J., Beall, A. C., Swinth, K. R., Hoyt, C. L., and Bailenson, J. N. (2002). Target article: immersive virtual environment technology as a methodological tool for social psychology. Psychol. Inq. 13, 103–124. doi:10.1207/s15327965pli1302_01
Iryo-Asano, M., Hasegawa, Y., and Dias, C. (2018). Applicability of virtual reality systems for evaluating pedestrians' perception and behavior. Transp. Res. Proced. 34, 67–74. doi:10.1016/j.trpro.2018.11.015
Joseph, A., Quan, X., Keller, A. B., Taylor, E., Nanda, U., and Hua, Y. (2014). Building a knowledge base for evidence-based healthcare facility design through a post-occupancy evaluation toolkit. Intell. Build. Int. 6 (3), 155–169. doi:10.1080/17508975.2014.903163
Kooijman, L., Happee, R., and de Winter, J. (2019). How do eHMIs affect pedestrians' crossing behavior? A study using a head-mounted display combined with a motion suit. Information 10 (12), 386. doi:10.3390/info10120386
Ledoux, T., Nguyen, A. S., Bakos-Block, C., and Bordnick, P. (2013). Using virtual reality to study food cravings. Appetite 71, 396–402. doi:10.1016/j.appet.2013.09.006
Lee, J., Eden, A., Ewoldsen, D. R., Beyea, D., and Lee, S. (2019). Seeing possibilities for action: orienting and exploratory behaviors in VR. Comput. Hum. Behav. 98, 158–165. doi:10.1016/j.chb.2019.03.040
Li, B. J., and Bailenson, J. N. (2018). Exploring the influence of haptic and olfactory cues of a virtual donut on satiation and eating behavior. Presence Teleop. Virt. Environ. 26 (03), 337–354. doi:10.1162/pres_a_00300
Lin, J., Cao, L., and Li, N. (2020). How the completeness of spatial knowledge influences the evacuation behavior of passengers in metro stations: a VR-based experimental study. Autom. Construct. 113, 103136. doi:10.1016/j.autcon.2020.103136
Lok, B., Ferdig, R. E., Raij, A., Johnsen, K., Dickerson, R., Coutts, J., et al. (2006). Applying virtual reality in medical communication education: current findings and potential teaching and learning benefits of immersive virtual patients. Virt. Real. 10 (3–4), 185–195. doi:10.1007/s10055-006-0037-3
Lombart, C., Millan, M., Normand, J.-M., Verhulst, A., Labbé-Pinlon, B., and Moreau, G. (2019). Consumer perceptions and purchase behavior toward imperfect fruits and vegetables in an immersive virtual reality grocery store. J. Retail. Consum. Serv. 48, 28–40. doi:10.1016/j.jretconser.2019.01.010
Lovreglio, R., Gonzalez, V., Feng, Z., Amor, R., Spearpoint, M., Thomas, J., et al. (2018). Prototyping virtual reality serious games for building earthquake preparedness: the Auckland City Hospital case study. Adv. Eng. Inform. 38, 670–682. doi:10.1016/j.aei.2018.08.018
Marcum, C. S., Goldring, M. R., McBride, C. M., and Persky, S. (2018). Modeling dynamic food choice processes to understand dietary intervention effects. Ann. Behav. Med. 52, 252–261. doi:10.1093/abm/kax041
Markwart, H., Vitera, J., Lemanski, S., Kietzmann, D., Brasch, M., and Schmidt, S. (2019). Warning messages to modify safety behavior during crisis situations: a virtual reality study. Int. J. Disaster Risk Reduct. 38, 101235. doi:10.1016/j.ijdrr.2019.101235
Meehan, M., Razzaque, S., Whitton, M. C., and Brooks, F. P. (2003). “Effect of latency on presence in stressful virtual environments,” in Proceedings of the IEEE virtual reality, 141–148.
Metsis, V., Lawrence, G., Trahan, M., Smith, K. S., Tamir, D., and Selber, K. (2019). 360 Video: a prototyping process for developing virtual reality interventions. J. Technol. Hum. Serv. 37 (1), 32–50. doi:10.1080/15228835.2019.1604291
Mizuchi, Y., and Inamura, T. (2018). “Evaluation of human behavior difference with restricted field of view in real and VR environments,” in 27th IEEE international symposium on robot and human interactive communication, RO-MAN, 196–201.
Morrongiello, B. A., Corbett, M., Switzer, J., and Hall, T. (2015). Using a virtual environment to study pedestrian behaviors: how does time pressure affect children's and adults' street crossing behaviors. J. Pediatr. Psychol. 40, 697–703. doi:10.1093/jpepsy/jsv019
Nash, E. B., Edwards, G. W., Thompson, J. A., and Barfield, W. (2000). A review of presence and performance in virtual environments. Int. J. Hum.-Comput. Interact. 12 (1), 1–41. doi:10.1207/s15327590ijhc1201_1
Neo, J. R. J., Won, A. S., and Shepley, M. M. (2019). “Virtual reality as a prototyping tool in health behavior research: Current research, limitations, and recommendations for future research,” in Paper presented at the 69th International Communication Association Conference, DC, USA.
Nordbo, K., Milne, D., Calvo, R. A., and Allman-Farinelli, M. (2015). “Virtual food court: a VR environment to assess people's food choices,” in Proceedings of the Annual Meeting of the Australian Special Interest Group for Computer Human Interaction, 2015 (New York, NY: ACM), 69–72.
Oh, S. Y., Shriram, K., Laha, B., Baughman, S., Ogle, E., and Bailenson, J. (2016). Immersion at scale: researcher's guide to ecologically valid mobile experiments. IEEE Virt. Real. 14, 249–250. doi:10.1109/vr.2016.7504747
Otto, K., and Wood, K. (2001). Product design: techniques in reverse engineering and new product design. Upper Saddle River: Prentice-Hall.
Parsons, T. D., Bowerly, T., Buckwalter, J. G., and Rizzo, A. A. (2007). A controlled clinical comparison of attention performance in children with ADHD in a virtual reality classroom compared to standard neuropsychological methods. Child. Neuropsychol. 13, 363–381. doi:10.1080/13825580600943473
Persky, S., Goldring, M. R., Turner, S. A., Cohen, R. W., and Kistler, W. D. (2018). Validity of assessing child feeding with virtual reality. Appetite 123, 201–207. doi:10.1016/j.appet.2017.12.007
Poelman, M., Kroeze, W., Waterlander, W., de Boer, M., and Steenhuis, I. (2017). Food taxes and calories purchased in the virtual supermarket: a preliminary study. BFJ 119, 2559–2570. doi:10.1108/bfj-08-2016-0386
Pourhoseingholi, M. A., Baghestani, A. R., and Vahedi, M. (2012). How to control confounding effects by statistical analysis. Gastroenterol. Gastroenterol Hepatol Bed Bench 5 (2), 79–83. doi:10.1002/0471476471.ch13
Rollings, K. A., and Evans, G. W. (2019). Design moderators of perceived residential crowding and chronic physiological stress among children. Environ. Behav. 51 (5), 590–621. doi:10.1177/0013916518824631
Rollings, K. A., and Wells, N. M. (2018). Cafeteria assessment for elementary schools (CAFES): development, reliability testing, and predictive validity analysis. BMC Public Health 18, 1154. doi:10.1186/s12889-018-6032-2
R. W. Marans, and D. Stokols (2013). Environmental simulation: research and policy issues. Berlin: Springer Science and Business Media.
Sachs, N. A. (2018). EBD at the macro Level: how research informs policy. Health Environ. Res. Des. J. 11 (4), 108–110. doi:10.1177/1937586718812120
Saeidi, S., Chokwitthaya, C., Zhua, Y., and Sun., Ming. (2019). Spatial-temporal event-driven modeling for occupant behavior studies using immersive virtual environments. Autom. Construct. 94, 381–382. doi:10.1016/j.autcon.2018.07.019
Schwebel, D. C., Gaines, J., and Severson, J. (2008). Validation of virtual reality as a tool to understand and prevent child pedestrian injury. Accid. Anal. Prev. 40, 1394–1400. doi:10.1016/j.aap.2008.03.005
Schwebel, D. C., Stavrinos, D., Byington, K. W., Davis, T., O'Neal, E. E., and de Jong, D. (2012). Distraction and pedestrian safety: how talking on the phone, texting, and listening to music impact crossing the street. Accid. Anal. Prev. 45 (2), 266–271. doi:10.1016/j.aap.2011.07.011
Shi, Y., Du, J., Ahn, C. R., and Ragan, E. (2019). Impact assessment of reinforced learning methods on construction workers' fall risk behavior using virtual reality. Autom. Construct. 104, 197–214. doi:10.1016/j.autcon.2019.04.015
Siegrist, M., Ung, C.-Y., Zank, M., Marinello, M., Kunz, A., Hartmann, C., et al. (2018). Consumers' food selection behaviors in three-dimensional (3D) virtual reality. Food Res. Int. 117, 50–59. doi:10.1016/j.foodres.2018.02.033
Slater, M. (2018). Immersion and the illusion of presence in virtual reality. Br. J. Psychol. 109 (3), 431–433. doi:10.1111/bjop.12305
Slater, M., Khanna, P., Mortensen, J., and Yu, I. (2009). Visual realism enhances realistic response in an immersive virtual environment. IEEE Comput. Graph Appl. 29, 76–84. doi:10.1109/mcg.2009.55
Slater, M., Spanlang, B., Sanchez-Vives, M. V., and Blanke, O. (2010). First person experience of body transfer in virtual reality. PloS one 5 (5), e10564. doi:10.1371/journal.pone.0010564
Sobhani, A., Farooq, B., and Zhong, Z. (2017). “Distracted pedestrians crossing behaviour: application of immersive head mounted virtual reality,” in IEEE 20th international conference intelligent transportation systems (ITSC), 1–6.
Stelick, A., Penano, A. G., Riak, A. C., and Dando, R. (2018). Dynamic context sensory testing—a proof of concept study bringing virtual reality to the sensory booth. J. Food Sci. 83, 2047–2051. doi:10.1111/1750-3841.14275
Tucker, A., Marsh, K. L., Gifford, T., Lu, X., Luh, P. B., and Astur, R. S. (2018). The effects of information and hazard on evacuee behavior in virtual reality. Fire Safe. J. 99, 1–11. doi:10.1016/j.firesaf.2018.04.011
Ulrich, K. T., and Eppinger, S. D. (2012). Product and design development. 5th ed. New York, NY: McGraw Hill Companies, Inc.
Veling, W., Counotte, J., Pot-Kolder, R., van Os, J., and van der Gaag, M. (2016). Childhood trauma, psychosis liability and social stress reactivity: a virtual reality study. Psychol. Med. 46, 3339–3348. doi:10.1017/S0033291716002208
Viswanathan, A. M., and Choudhury, B. (2011). Virtual reality anatomy: is it comparable with traditional methods in the teaching of human forearm musculoskeletal anatomy? Anat. Sci. Educ. 4 (3), 119–125. doi:10.1002/ase.214
Waterlander, W. E., Jiang, Y., Steenhuis, I. H., and Ni Mhurchu, C. (2015). Using a 3D virtual supermarket to measure food purchase behavior: a validation study. J. Med. Internet Res. 17, e107. doi:10.2196/jmir.3774
Wiederhold, B. K. (2017). What can behavioral healthcare learn from digital medicine? Cyberpsychol. Behav. Social Netw. 20 (12), 725–726. doi:10.1089/cyber.2017.29092.bkw
Wiederhold, B. K., and Wiederhold, M. D. (2010). Virtual reality treatment of posttraumatic stress disorder due to motor vehicle accident. Cyberpsychol. Behav. Soc. Netw. 13 (1), 21–27. doi:10.1089/cyber.2009.0394
Witmer, B. G., and Singer, M. J. (1998). Measuring presence in virtual environments: a presence questionnaire. Presence 7 (3), 225–240. doi:10.1162/105474698565686
Yaremych, H. E., and Persky, S. (2019). Tracing physical behavior in virtual reality: a narrative review of applications to social psychology. J. Exp. Soc. Psychol. 85, 1–8. doi:10.1016/j.jesp.2019.103845
Keywords: immersive virtual environment, human behavior, design, prototype development, environmental psychology, virtual reality
Citation: Neo JRJ, Won AS and Shepley MM (2021) Designing Immersive Virtual Environments for Human Behavior Research. Front. Virtual Real. 2:603750. doi: 10.3389/frvir.2021.603750
Received: 07 September 2020; Accepted: 13 January 2021;
Published: 04 March 2021.
Edited by:
Ronan Boulic, École Polytechnique Fédérale de Lausanne, SwitzerlandReviewed by:
Aitor Rovira, University of Oxford, United KingdomSusan Persky, National Human Genome Research Institute (NHGRI), United States
Tomas Trescak, Western Sydney University, Australia
Copyright © 2021 Neo, Won and Shepley. This is an open-access article distributed under the terms of the Creative Commons Attribution License (CC BY). The use, distribution or reproduction in other forums is permitted, provided the original author(s) and the copyright owner(s) are credited and that the original publication in this journal is cited, in accordance with accepted academic practice. No use, distribution or reproduction is permitted which does not comply with these terms.
*Correspondence: Jun Rong Jeffrey Neo, am40NThAY29ybmVsbC5lZHU=