- 1Department of Computer and Information Science and Engineering, University of Florida, Gainesville, FL, United States
- 2Department of Pediatrics, University of Florida, Gainesville, FL, United States
This initial exploratory study’s primary focus is to investigate the effectiveness of a virtual patient training platform to present a health condition with a range of symptoms and severity levels. The secondary goal is to examine visualization’s role in better demonstrating variances of symptoms and severity levels to improve learning outcomes. We designed and developed a training platform with a four-year-old pediatric virtual patient named JAYLA to teach medical learners the spectrum of symptoms and severity levels of Autism Spectrum Disorder in young children. JAYLA presents three sets of verbal and nonverbal behaviors associated with age-appropriate, mild autism, and severe autism. To better distinguish the severity levels, we designed an innovative interface called the spectrum-view, displaying all three simulated severity levels side-by-side and within the eye span. We compared its effectiveness with a traditional single-view interface, displaying only one severity level at a time. We performed a user study with thirty-four pediatric trainees to evaluate JAYLA’s effectiveness. Results suggest that training with JAYLA improved the trainees’ performance in careful observation and accurate classification of real children’s behaviors in video vignettes. However, we did not find any significant difference between the two interface conditions. The findings demonstrate the applicability of the JAYLA platform to enhance professional training for early detection of autism in young children, which is essential to improve the quality of life for affected individuals, their families, and society.
1 Introduction
Virtual patients represent a compelling application of embodied conversational agents. These agents are computer-generated characters that demonstrate human-like behaviors and properties in conversations, including engagement with and response to verbal and nonverbal communications (Cassell et al., 2000). Virtual patients are widely used for training in the medical field (Talbot et al., 2019). A research study shows that applying virtual patients for testing clinical examination interview skills is valid (Johnsen et al., 2007). This study reports a significant correlation between students' performance when interviewing a virtual patient and students' performance when interviewing a standardized patient, trained actors who pretend to have a particular health condition (Lopreiato, 2016). But, using young children as standardized patients is challenging; often, they cannot behave reliably or consistently, and hiring them is not universally accepted for ethical reasons (Tsai, 2004). Additionally, certain common examinations and procedures are not ethical to perform on a child for training purposes (e.g. a genital examination) (Khoo et al., 2017). Pediatric virtual patients may offer a safe, effective, and ethical means to train healthcare professionals.
Many clinical conditions have a spectrum of symptoms ranging from mild to severe, including Autism Spectrum Disorder (ASD). ASD is a neurodevelopmental disorder that is characterized by impairments in social and communication skills as well as stereotypical behaviors (Association, 2013). ASD cannot be cured, but early diagnosis and interventions are crucial to reducing the symptoms and improving the quality of life for children with ASD and their families (Buescher et al., 2014). In January 2020, the American Academy of Pediatrics called for building increased capacity to care for children and youth with ASD, including a more comprehensive focus on educating pediatric trainees about screening, diagnosis, and management (Hyman et al., 2020). Data indicates that 33–50% of children with ASD are not diagnosed until after the age of six (Sheldrick et al., 2017). Delayed diagnoses is associated with disparities in the training of healthcare professionals, low perceived self-efficacy and failure to correctly screen for ASD (Mussap et al., 2020). To best serve their communities and increase the proportion of children who are correctly diagnosed with ASD, enhanced training methods are needed. The next generation of pediatric professionals needs access to novel teaching tools that will bridge disparities in training, increase self-efficacy and reduce diagnostic error, a noble goal to which we hope to contribute.
Virtual patients have the power to simulate disorders with a spectrum of symptoms in a controlled learning environment (Kotranza et al., 2010). Unlike a real medical setting, controlled learning environments are free of distractions and have defined educational objectives (Issenberg et al., 2005), providing the potential to reduce cognitive load and increase learning outcomes (Choi et al., 2014). When used to simulate a spectrum of symptoms, virtual patients enable learners to compare and contrast across severity levels (Kotranza et al., 2010). Virtual patient simulations can be deployed to different platforms with varying levels of immersion ranging from desktop virtual environments to head-mounted displays, but 2D platforms are accessible to a broader audience. A review of literature related to best practices in user interface design for comparative practice provided evidence that juxtaposition design, placing elements close together in space and within eye span, can improve comparison tasks (Gleicher et al., 2011). Thus, it would follow that virtual patient training using an interface constructed based on juxtaposition design principles, referred to as the “spectrum-view” in this study, may better present disorders with a range of symptoms, enhancing learning outcomes compared to a “single-view” interface.
This study introduces JAYLA (Junior Agent to typifY Levels of Autism), a pediatric virtual patient platform. The JAYLA platform is a desktop-based application that provides a technical framework for presenting health conditions with a spectrum of symptoms and severity levels. JAYLA can portray the verbal and nonverbal behaviors of a four-year-old African American girl in the context of a bubble-blowing activity with an examiner (See Figure 1). JAYLA is designed with three sets of verbal and nonverbal behaviors (age-appropriate, mild autism, severe autism) to demonstrate differences among them.
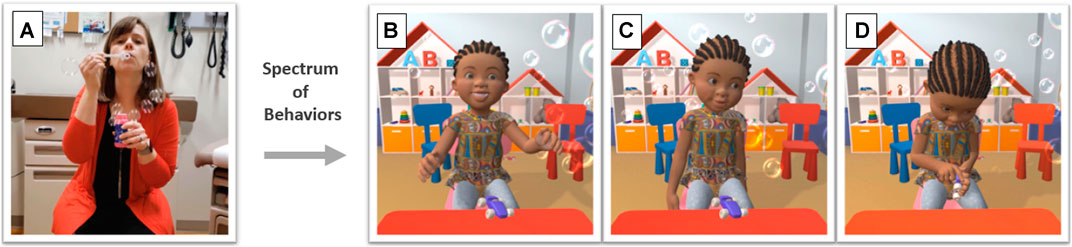
FIGURE 1. Spectrum of simulated behaviors of JAYLA toward the examiner’s action. (A): The examiner is blowing bubbles, (B): JAYLA shows age-appropriate behaviors, (C): JAYLA shows mild autism behaviors, (D): JAYLA shows severe autism behaviors.
We conducted a user study with pediatric trainees to examine the effectiveness of the JAYLA platform and its two interfaces. Trainees were randomly assigned to the single-view or the spectrum-view interface (See Figure 2). In each condition, JAYLA portrayed three different behaviors: age-appropriate, mild autism, and severe autism. Trainees in the single-view interface observed one version of JAYLA at a time on the screen. However, trainees in the spectrum-view interface could compare and contrast all three versions of JAYLA at once on the screen. To measure the learning outcomes, trainees were asked to assess the behaviors of real children in three short video vignettes using a validated screening tool in a pre- and post-test. Then, trainees’ ratings were compared to experts’ ratings. In this paper, we address the following research questions:
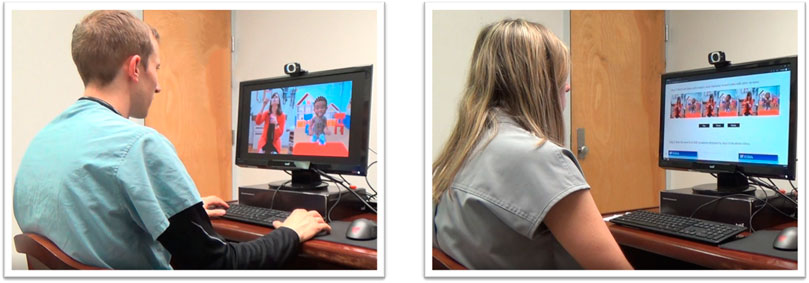
FIGURE 2. Trainees using the two interfaces: single-view interface (left), spectrum-view interface (right).
RQ1: After training with the JAYLA platform, would trainees be able to better identify and classify real children’s behaviors in video vignettes using a validated screening tool and their ratings more closely match experts’ ratings in the post-test stage?
RQ2: Would the choice of interface (single-view vs. spectrum-view) affect the trainees’ ability to identify and classify real children’s behaviors in video vignettes using a validated screening tool and their ratings more closely match experts’ ratings in the post-test stage?
The main contributions of this initial exploratory study are:
1) Introducing a new effective training platform using a pediatric virtual patient to teach various symptoms and severity levels of autism in young children to healthcare professionals. The effectiveness of the JAYLA platform offers promising new directions, paving the way for developing more interactive versions of the JAYLA simulation and studying how its flexibility impacts learning outcomes. In this initial study, trainees learned deficits in social reciprocity are among the main characteristics of ASD. They also learned the variety of symptoms and severity levels of ASD and the process of identifying them.
2) Evaluating the simulated emotions and behaviors of JAYLA (age-appropriate, mild, and severe) and the overall simulation experience. We learned that the JAYLA’s simulated emotions were expressive, her behaviors were appropriate, and the overall simulation experience was perceived as helpful for ASD training.
3) Exploring the impact of visualization for presenting a health condition with a spectrum of symptoms and severity levels. While our analysis did not show a statistically significant difference between the two interfaces, the qualitative analysis of open feedback results suggests that the spectrum-view could have some advantages over the single-view interface. This initial exploratory study provided insights regarding the effects of visualization, hopefully encouraging further research for more conclusive results.
2 Background
2.1 Training With Video Vignettes Vs. Virtual Character Simulations
Videos vignettes of real pediatric patients have been used in residency training programs to demonstrate the difference between typical and atypical play in children (Major, 2015). Although these videos are beneficial in teaching specific concepts, this approach involves some challenges, such as invasion of patients’ privacy or inability to control differences in individual characteristics or environment settings. Another approach is producing training videos by recruiting experienced clinicians, actors, or standardized patients to play in particular training scenarios (Major, 2015; Verkuyl et al., 2016). Some drawbacks are associated with this approach, such as being resource-intensive, costly, and lacking flexibility in modifying contents after production. There is an opportunity to use virtual characters to achieve similar effectiveness in training at a lower cost.
Research in both behavioral and neuroimaging fields suggests that virtual characters are perceived comparably to real human beings. For example, there is empirical evidence that users’ perception ratings of nonverbal behaviors performed by human beings in videos do not significantly differ from the same behaviors performed by virtual characters, indicating overall correspondence between video recordings and animations (Bente et al., 2001). Additionally, research shows that virtual emotional expressions are recognized as effective as natural emotional expressions for displaying emotions (Dyck et al., 2008). Similar results are shown in functional neuroimaging research, which suggests that emotional expressions of virtual faces can elicit the same brain responses evoked by real human faces (Bahrani et al., 2019). One advantage of virtual characters over videotaped human beings is virtual characters are capable of demonstrating realistic behaviors, but they can also be systemically manipulated and fully controlled (Vogeley and Bente, 2010). Hence, virtual characters can provide a good balance between ecological validity and experimental control. Another advantage is the independence of the animation and appearance of the virtual characters, making it easy to scale up the simulation with various virtual characters of different ages, sex, race, and ethnicity with the same animation (Bente and Krämer, 2011). Also, virtual character simulations have the potential to become interactive which can further enhance the effectiveness of training.
2.2 Pediatric Training Using Simulations
In healthcare, simulation-based training includes any educational activity that leverages simulation to replicate clinical scenarios (Ziv et al., 2005). Simulation-based training provides a safe space for participants to learn from their mistakes without adverse consequences (Ziv et al., 2005). Virtual patients are computer simulations, a promising technological innovation, that can provide: 1) uniform and reliable training, 2) replication of a wide range of patients’ behaviors (Talbot et al., 2019), and 3) simulation of nearly any demographic group, including young children. Yet, research related to pediatric virtual patients lags behind the body of work associated with adult virtual patients (Dukes et al., 2016).
Initial research on pediatric virtual patients showed even low-fidelity characters can provide a valuable learning experience as long as the simulation scenarios cover important clinical topics (Bloodworth et al., 2010; Pence et al., 2013). Emerging research provides evidence that a pediatric virtual patient platform that incorporates medical experts during the design and evaluation phases of development considerably reduces the scenario creation time. For example, authors in (Dukes et al., 2016) developed a platform that could generate pediatric virtual patients with different appearances and scenarios, and reduced scenario creation time from approximately nine months to approximately 30 min. However, their platform was limited in its ability to generate diverse nonverbal symptoms.
2.3 Virtual Character Applications in Autism
It has been evident that individuals with autism are attracted to technologies and like to spend plenty of time watching television, using computers and other mobile devices (Mineo et al., 2009). This makes computers a good platform for delivering social and communicational skill training to individuals with ASD and resulted in numerous ASD-specific computer-assisted learning applications, including virtual reality training applications (Parsons and Mitchell, 2002; Putnam and Chong, 2008). Virtual reality simulations that leverage reinforcement learning and multimodal interactions have shown promising results in improving the communication and social skills of people with ASD across the spectrum and in different age groups. A number of meta-studies provided a review of existing work on computer-based and virtual reality-based interventions for autism (Bellani et al., 2011; Ramdoss et al., 2012; Georgescu et al., 2014). A variety of ASD related deficits have been targeted for these interventions including: social skills (Hopkins et al., 2011), social norm understanding (Mitchell et al., 2007), communication and language development (Tartaro and Cassell, 2008; Bernardini et al., 2014), joint attention (Cheng and Huang, 2012), emotions recognition (Didehbani et al., 2016), and empathy (Cheng et al., 2010). In virtual reality interventions, virtual characters are used in a wide range of applications such as role-playing or computer-aided diagnosis. In role-playing applications, virtual characters can play the role of social interaction partners, coaches, or tutors. For example, virtual characters acting in the roll of peers are shown to be more effective than typically developing peers in engaging children with ASD in storytelling and contingent discourse (Tartaro and Cassell, 2008). In job training, virtual agents as interviewers are shown to be effective in providing individuals with ASD the opportunity to practice repeatedly and help them overcome their anxiety and become better-prepared (Burke et al., 2018; Smith et al., 2014). A recent study has used the nonverbal interaction between virtual characters and individuals with ASD as an objective measure for computer-assisted diagnosis assessment of ASD (Roth et al., 2020). However, very few studies attempted to use virtual characters to simulate ASD related behaviors that can be used for training doctors, special education teachers, and caregivers.
2.4 Simulating Autistic Behaviors Using Agents
Research shows there is inadequate training for key individuals, such as healthcare professionals and teachers, surrounding the spectrum of symptoms indicative of ASD (Golnik et al., 2009; Busby et al., 2012). This has motivated researchers to develop training simulations that can educate professionals about various symptoms and severity levels of ASD and fill this gap.
In the education field, TLE TeachLivE™ (Bousfield, 2017), a mixed-reality classroom with student avatars who can respond in real-time, was used to simulate the behaviors of students with ASD. They investigated the difference between novice and expert teachers’ pedagogy skills toward a nineteen-year-old male student avatar with ASD in an inclusive secondary classroom. An interactor in real-time controlled the student avatars’ verbal and nonverbal behaviors. An interactor is a person “trained in acting, improvisation, and human psychology” (Bousfield, 2017). The interactor’s role is vital for the immersive and individualized learning experience, but it is resource-intensive and requires training to ensure consistency and fidelity of the simulation. In addition, the avatar with ASD is modeled based on an individual with specific symptoms of ASD. Thus it is not representative of the spectrum of behaviors associated with ASD.
In a similar context, authors in (Best, 2019) developed training for pre-service teachers to teach how to identify and adequately respond to bullying situations involving elementary age students with ASD. This study used the TeachLivE™ simulator to record scenarios in which a virtual student with ASD is bullied by typically developing virtual students. The characteristics of the virtual student with ASD were designed based on the DSM-5 (Association, 2013) and reviewed by an expert. Similar to (Bousfield, 2017), this study used only one virtual student with ASD who displayed specific characteristics that are not representative of all individuals with ASD.
In robotics, researchers developed an interactive training tool using a NAO humanoid robot to simulate four different severity levels of autism in children (Baraka et al., 2017). The robot’s behaviors were developed as a response to three predefined stimuli used in ASD diagnosis, including response to name, shared attention, and asking for a snack preference. This interactive humanoid robot can be used to train ASD therapists and educate people about different forms of ASD. However, NAO humanoid robots cannot display facial expressions and eye contact, which are important for identifying ASD. Also, robots are less accessible compared to web-based virtual characters.
Overall, the prior works simulating autistic behaviors, despite their limitations, offer a promising opportunity for training and educating different groups of people about the characteristics and severity levels of autism. To our knowledge, JAYLA is the first pediatric virtual patient platform that is designed to show a spectrum of verbal and nonverbal symptoms and severity levels of ASD.
2.5 ASD Assessment
ASD is a highly prevalent, lifelong neurodevelopmental disorder primarily characterized by mild to severe deficits in: 1) reciprocal social interactions, 2) communication, and 3) restricted and repetitive patterns of behaviors (Association, 2013). We chose ASD for two reasons: 1) high prevalence, currently estimated at one in fifty-four children (Baio et al., 2018), and 2) substantial impact of early diagnosis and intervention on children’s development (Buescher et al., 2014).
To evaluate the effectiveness of training, we needed videos of real children across the spectrum of ASD for pre- and post-testing. We were looking for structured videos where the participants followed the same set of activities to demonstrate social reciprocity. Social reciprocity is defined as a back-and-forth flow of social interaction (Association, 2013). This led us to the Multimodal Dyadic Behavior (MMDB) dataset. The MMDB dataset contains multimodal recordings of 160, three to 4 min sessions of semi-structured play interactions between trained adult examiners and children between the ages of fifteen and thirty months old following Rapid-ABC protocol (Rehg et al., 2013). Rapid-Attention Back and Forth, Communication (Rapid-ABC) is a 4 min validated autism screener, with five activities: 1) saying hello, 2) playing ball, 3) turning pages of a book, 4) pretending a book is a hat on your head, and 5) tickling (Ousley et al., 2013). These activities are designed to elicit social-communication behaviors such as affect, eye contact, joint attention behaviors (e.g. mutual focus of two individuals on the same thing), and social reciprocity (Ousley et al., 2013). The presence or absence of each behavior, along with an ease-of-engagement score which was calculated as part of the original study, helped us pick appropriate videos (Ousley et al., 2013). In this study, we focused on training for social reciprocity and used the playing ball activity videos.
To assess the behaviors of JAYLA and children in the videos in a standardized way, we used the Childhood Autism Rating Scale, Second Edition (CARS-2) (Schopler et al., 2010). The CARS-2 is a validated rating scale which is based upon direct observation and provides assessment opportunities on the range of autism symptoms and the spectrum of severity. For this study, we utilized the portion of the CARS-2 focused on social reciprocity.
3 System Design
Different components related to the design and development of the JAYLA platform include: 1) scenario, 2) JAYLA development, 3) interfaces development, and 4) scaffolding strategies.
3.1 Scenario
One of the main characteristics of ASD is deficits in social reciprocity. Individuals with ASD have difficulties with social reciprocity, including troubles with back-and-forth conversation, reduced sharing of interests, and failure to initiate or respond to social interactions (Association, 2013). There is no deterministic medical test (such as a blood test) for diagnosing ASD, and healthcare professionals must rely on their clinical judgment based on interviews with parents and observing the child’s behaviors to diagnose ASD (On Children with Disabilities, 2001). Healthcare professionals usually engage a child in social games, such as a bubble-blowing activity, to carefully observe and assess the child’s behaviors and level of engagement (On Children with Disabilities, 2001). Based on prior research and to investigate JAYLA’s effectiveness in a case study, we implemented a bubble-blowing scenario. This activity shows back-and-forth interactions between JAYLA and the examiner to demonstrate different social reciprocity responses characteristic of typically developing (age-appropriate) children and those with mild and severe ASD.
3.2 JAYLA Development
The work described here reflects our efforts in conceptualizing JAYLA as a flexible platform to investigate pediatric virtual patients to simulate ASD behavioral symptoms across different severity levels. JAYLA’s behaviors were developed through an iterative design process in collaboration with a pediatric physician as a subject matter expert and the text-book definitions of ASD severity levels. To evaluate the validity of training with JAYLA, we focused on the bubble-blowing activity, a standard clinical examination to assess social reciprocity in young children (On Children with Disabilities, 2001).
Research shows that African American children and girls are under-diagnosed with ASD (Baio et al., 2018). Healthcare simulators, writ large, also suffer from a lack of diversity. This motivated us to choose an African American girl 3D model for JAYLA. The 3D model was purchased from the DAZ3D studio and was rigged and animated using the mixamo website. The animations were modified using MAYA and Unity Mecanim system, which can support complex interactions between animations. To ensure high-levels of realism, JAYLA’s voice was recorded by a young girl.
The JAYLA platform used a modular architecture and broke down the process of creating multimodal behaviors in small and meaningful parts called action units, allowing reusability and sharing across different scenarios. For example, non-verbal action units of JAYLA in the bubble-blowing activity (e.g., fidgeting, hand flapping, and body rocking) are not context-specific. They can be reused for additional activities that demonstrate other characteristics of ASD. To modularize JAYLA’s behaviors, we created different pools of action units for each version of JAYLA, including: audio tracks, facial expressions, and animation clips. Each reaction of JAYLA is created by synchronization and blending multiple action units selected from different pools. This forms a state machine with each state containing a mix of an audio track, eye-contact, facial expression, and animation. The transitions between states can be triggered based on input signals (e.g., voice commands, appropriate buttons, or the Unity3D script). The eye-contact behaviors are controlled from the unity3D script and simulated by adding varying degrees of random looking behavior. The age-appropriate JAYLA keeps the longest eye-contact by looking at the center of the virtual camera. The mild JAYLA shows less consistent eye-contact by looking away, and severe JAYLA mostly avoids eye contact by looking at the toy or around the room. Also, different dummy 3D targets were placed around the virtual room to help to simulate the eye gaze when JAYLA is looking at the bubbles. Table 1 summarizes these behaviors.
In this initial study, we aimed to create a standardized experience and simplify the evaluation of JAYLA’s simulated behaviors, demonstrating different severity levels of ASD. We decided to use an entirely controlled version of JAYLA that is synchronized with a 1 min video of an examiner, which also makes it consistent with the length of the video vignettes of the real children. For this purpose, we recorded a 1 min video of a physician acting as an examiner trying to engage a child in a bubble-blowing activity and controlled the examiner’s actions. Then, JAYLA’s verbal and nonverbal behaviors were synchronized with the examiner’s actions to create three side-by-side videos of JAYLA and the examiner.
3.3 Interfaces Development
As stated previously, juxtaposition design has been shown to be effective for comparison tasks (Gleicher et al., 2011). To investigate the effects of visualization on learning outcome, we developed two interfaces: 1) the single-view interface, and 2) the spectrum-view interface (see Figure 2).
The single-view interface displays one of the three side-by-side videos of JAYLA and the examiner at a time on the screen and in random order. After each video, trainees were asked to assess JAYLA’s behaviors. The spectrum-view interface displays all three side-by-side videos of JAYLA and the examiner in random order within the eye span of the participants and in one screen. This allows participants to compare and contrast JAYLA’s behaviors between different versions. Participants could either watch videos individually or watch all three videos at the same time. To avoid talk overs while playing all three videos at once, trainees were able to mute/unmute the videos. Trainees were asked to assess each video only after they finished watching all three videos.
3.4 Scaffolding Strategies
Scaffolding can reduce the learner’s cognitive load, i.e. amount of working memory resources, and therefore improve learning outcomes (Van de Pol et al., 2010). The JAYLA platform incorporates various scaffolding strategies, including: 1) instructing, 2) modeling, 3) questioning, and 4) feedback (Van de Pol et al., 2010) that are discussed next.
Instructions are an integral part of the learning process (Van de Pol et al., 2010). Prior research shows that the instructor’s credibility plays a key role in students’ learning (Martin and Myers, 2018). Therefore, providing instructions by a credible instructor could be a factor for enhanced learning outcomes. The JAYLA platform has some instructional videos regarding the definition of social reciprocity and its identifying factors. The instructional videos are made by recording our pediatric physician collaborator, who is also a clinician educator for pediatric trainees, explaining the concepts.
Modeling is widely studied and shown to be effective in boosting learning (Van de Pol et al., 2010). Modeling is a demonstration of a particular skill and has two types: behavioral (mimicking the behaviors) and cognitive (mimicking the thought process) (Dennen, 2004). The JAYLA platform incorporates both types of modeling. JAYLA utilizes behavioral modeling to demonstrate the interaction between an examiner and three simulated versions of JAYLA. In addition, JAYLA leverages cognitive modeling by explaining the rationale behind the correct choice using feedback videos discussed below.
Questioning requires trainees to provide an active linguistic or cognitive answer to questions (Van de Pol et al., 2010). The JAYLA platform incorporates questioning by asking participants to assess the behaviors of real children in videos using the CARS-2 screening tool.
Feedback provides the opportunity for trainees to learn from their mistakes and correct errors before applying their new skills in a real medical setting (Ziv et al., 2005). The JAYLA platform provides instant feedback to trainees. After submitting the answers to the CARS-2 assessment questions, trainees receive their results and watch a feedback video that summarizes details of the ASD symptoms portrayed by JAYLA for each severity level. Feedback videos also help with cognitive modeling by explaining the relevant considerations and rationale for the correct assessment. The trainees are then expected to use similar strategies for identifying ASD in the post-test videos of real children.
4 Experiment
A user study with pediatric trainees was conducted to evaluate the learning outcomes of training with the JAYLA platform and test the two designed interfaces. Evaluation of the learning outcomes was done based on a pre- and post-test.
4.1 Participants
Thirty four pediatric trainees (24 females, 10 males) were recruited and randomly assigned into the two conditions. Due to incomplete surveys, four trainees were excluded. 16 trainees (nine females, seven males) used the single-view interface, and fourteen trainees (12 females, two males) used the spectrum-view interface. Pediatric trainees were recruited from different professional programs at various stages of the learning continuum, including: 21 pediatric residents, five medical students on their required pediatric rotation, three physician assistants, and one nurse practitioner studying to be a Doctor of Nursing Practice. Each study session took approximately 40 min, and trainees were compensated with a $20 Amazon gift card.
4.2 Study Design and Procedure
The Institutional Review Board approved the study design, and all trainees provided consent prior to the start of the study. All of the sessions were video and audio recorded to have a log of events. Prior to beginning, trainees were instructed about the different stages of the study. The study is organized into five stages (See Figure 3). Note that the main difference between the two conditions is in stage three, autism training with JAYLA, which has two different interfaces: a single-view interface and a spectrum-view interface. This study is computer-based, and trainees used mouse and keyboards to complete the surveys.
Stage 1: A pre-survey with demographic questions.
Stage 2 & 4: In both the pre- and post-test stages, trainees were asked to assess the behaviors of three real children in short (
Stage 3: Trainees first watched an instructional video of a board-certified general pediatrician (“DH”), with more than ten years of clinical practice experience in academic settings, explaining the concept of social reciprocity and providing instructions on how to identify deficits in social reciprocity by paying attention to language, eye contact, facial expression, stereotypical movements such as hand flapping, and the child’s engagement throughout the activity. Trainees were then randomly assigned to either the single-view or the spectrum-view interface. Similar to the pre- and post-tests, after each JAYLA video, trainees were asked to assess JAYLA’s behaviors using the CARS-2. However, unlike the pre- and post-tests, trainees were provided with two types of immediate feedback: one correct answers along with their scores, two feedback videos explaining the reasoning for the right answers.
Stage 5: A post-survey with two open-ended questions.
4.3 Measures
We used the four questions related to social reciprocity from the CARS-2 questionnaire as a measure for evaluating the video vignettes of real children and videos of JAYLA. During the study, trainees completed nine CARS-2 questionnaires, three in each pre-test, training, and post-test stage. The CARS-2 questions are in a multiple-choice format with four answers for different severity levels ranging from age-appropriate to severe autism. For this study, we implemented three severity levels. So, we removed one of the choices related to moderate autism. The three remaining choices correspond to: 1) one-Age-appropriate, 2) two-Mild autism, and 3) three-Severe autism. Additionally, trainees completed a post-survey with eight, seven-point Likert-scale questions from -3: Strongly Disagree to +3: Strongly Agree (Table 2) and two open-ended questions providing general feedback on the study.
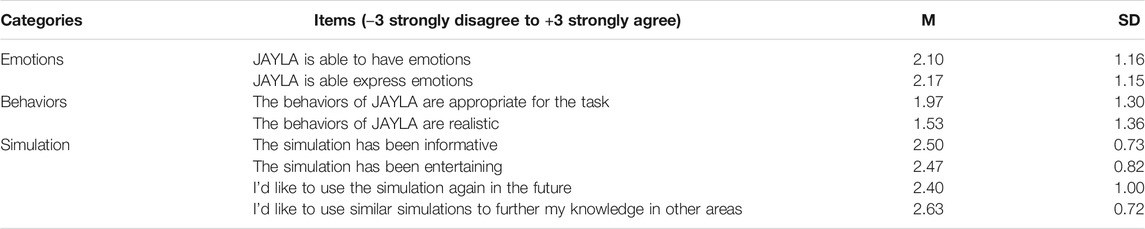
TABLE 2. Summary of the trainees’ ratings on emotions and behaviors of JAYLA and the simulation experience.
The last four questions in (Table 2) are adapted from the standard System Usability Scale (Bangor et al., 2008) and were modified based on feedback from medical professionals that were consulted for this study, with a focus on shortening it to reduce the amount of time required from the participants.
5 Results
This section describes the results of the quantitative analysis of the CARS-2 learning measure from the pre- and post-test stages. We then report the main themes that emerged through a qualitative analysis of the two open-ended questions from the post-survey.
5.1 CARS-2 Learning Measure
Trainees’ learning was assessed in a pre- and post-test format. Before and after training with JAYLA, the trainee’s ability to correctly identify three different behavior categories (age-appropriate, mild autism, and severe autism) in three different video vignettes of real children was assessed. Trainees’ answers to the four CARS-2 questions after each video vignette was used as a learning measure.
Learning data was analyzed using a two-factor split-plot (one within-subjects factor and one between-subjects factor) ANOVA. CARS-2 questions served as within-subject variables while the interface served as a between-subject factor. The data were normally distributed, and there were no outliers. There was homogeneity of variances
5.2 Simulation Ratings
To assess the simulation quality and its relevance for ASD training, the trainees were asked to respond to eight Likert-scale type questions in the post-survey regarding all three versions of JAYLA and the overall simulation experience. This was done at the end of the training experience after participants were exposed to both real children’s video vignettes and JAYLA’s simulated videos. The trainees’ attitudes toward simulated emotions and behaviors of JAYLA and the overall simulation experience were positive, they considered JAYLA’s emotions expressive and JAYLA’s behaviors appropriate for the task. The trainees found the simulation informative, entertaining, and they wish to use the same or similar simulations in the future. Table 2 summarizes the mean and standard deviation of ratings for all trainees.
5.3 Qualitative Results
To better understand the effectiveness of the JAYLA platform on trainees learning and gain insight on trainees’ perceptions toward the training, we conducted a qualitative analysis on the trainees’ answers to the two open-ended questions from the post-survey:
1. What did you learn about ASD and its variety of symptoms?
2. Please specify your additional comments or feedback.
Thematic analysis with an open coding method was used to derive common themes. We grouped the major themes into three categories: 1) Learning Areas, 2) Interface Feedback, and 3) Other Feedback.
5.3.1 Learning Areas
Deficits in social reciprocity. (16 of 30) trainees stated that the simulation experience provided new learning related to deficits in social reciprocity, one of the behavioral characteristics associated with ASD, e.g. “I hadn’t considered that an inability to stay attuned to social interaction could be a sign of ASD.” or “social interaction is the main defect.”
Variety of symptoms and severity levels of ASD. (17 of 30) trainees stated that the simulation reinforced or provided new learning related to the range of variety of symptoms associated with ASD, “I learned that ASD is, as the name implies, a spectrum of symptoms. There can be different severity levels.” or “There are various degrees, all containing the same basic principles that are hallmarks of Autism but just to lesser degrees in milder forms and there are tell-tale signs that we as examiners can look for in every child, even with minimal interaction.”
Process of identifying ASD symptoms. (17 of 30) trainees described specific symptoms of ASD and where to look to find them, e.g., “To evaluate, look for eye contact, shared emotion, ability to maintain attention to the activity, and for repetitive movements” or “Symptoms include lack of eye contact, less social reciprocity, stereotypical movement, decreased ability to express emotions and less verbal communication.”
The JAYLA platform was informative and useful. (9 of 30) trainees reported that the simulation was informative and furthered their knowledge, e.g., “Interesting use of JAYLA to show the spectrum of autism. The videos of the children, JAYLA, and the feedback videos were useful in further my understanding of what characteristics to look for along the spectrum of autism.” or “I like JAYLA. I like the simulation as it allows me to watch eye contact, hands, and conversation. It makes you more aware of what to look for in real patients.”
5.3.2 Interface Feedback
Single-view interface may be confusing. (4 of 16) trainees in the single-view interface mentioned it was hard for them to rate JAYLA’s behavior since they don’t have a baseline for what a virtual toddler is capable of doing, e.g., “Also, some exposure to what an age-appropriate JAYLA is like and is capable of as a simulation would have been helpful to keep in the back of your mind and compare to each simulation. Prior to seeing the age-appropriate JAYLA, I was unsure what things the simulation was capable of, so I did not have a framework to work with what abnormal for the simulation would have been.” or “examples for a computerized child beforehand to understand the spectrum of emotions the computerized child can have give us an idea of how realistic to expect the child to be because that may affect our responses.”
Spectrum-view interface may be appealing. (2 of 14) trainees in the spectrum-view condition provided positive feedback about the spectrum-view format, e.g., “The three-column format is unique” and “I liked the format.”
5.3.3 Other Feedback
Mild autism is subtle. (12 of 30) trainees stated that mild autism is more difficult to identify compared to age-appropriate behaviors or severe autism, e.g., “Sometimes, I felt that the mild symptoms were harder to pick up on, whether in the videos of real children or the simulation.” or “it was easier to identify the children with severe autism and the children without autism. I learned more about the different presenting variations of mild autism and feel I am better equipped to identify patients with mild and severe autism”. This finding is aligned with prior ASD research that indicates ASD symptoms may stay unrecognized for young children with mild ASD, which can lead to delayed diagnosis (Faras et al., 2010).
6 Discussion
This study’s primary purpose was to investigate the effectiveness of the JAYLA platform as a training tool for identifying the spectrum of symptoms and severity levels of ASD in young children. We also explored two interfaces for presenting the spectrum of the disorder. A user study was conducted to examine two research hypotheses:
H1 (Improved Learning): Trainees’ assessments of real children video vignettes using the CARS-2 screening tool will more closely match experts’ ratings in the post-test stage.
H2 (Interface Comparison): Trainees who used the spectrum-view interface will better assess real children video vignettes using the CARS-2 screening tool and more closely match experts’ ratings in the post-test stage compared to the single-view interface trainees.
6.1 Improved Learning (H1)
We accept the primary hypothesis based on the statistically significant difference between the CARS-2 scores in pre-test and post-test with a large effect size. The JAYLA platform is shown to be effective in significantly improving the trainees’ performance in identifying and classifying real children’s behaviors in the post-test videos using the CARS-2 screening tool. After training with JAYLA, trainees’ assessments of the real children from the MMDB videos more closely matched the experts’ ratings.
The results of the qualitative analysis of learner comments support the quantitative findings regarding our main hypothesis. Trainees highlighted different learning areas when responding to the open-ended questions. This provides evidence that the trainees demonstrated an understanding of the main objectives of the training, which are critical for identifying ASD in young children. Table 4 summarizes the main qualitative themes and the number of trainees who mentioned them.
The effectiveness of the JAYLA platform may be associated with several scaffolding strategies that are incorporated into the simulation, including: 1) instructing, 2) modeling, 3) questioning, and 4) feedback. Prior research shows that these scaffolding strategies are effective in reducing cognitive load and increasing learning outcomes (Van de Pol et al., 2010). We are unable to discern which strategy is the most effective component without further examination. However, trainees wrote positive comments about some of these strategies, which provide some evidence of efficacy. For example, one trainee highlighted the value of JAYLA in teaching signs of ASD, “I like Jayla. I like the simulation as it allows me to watch eye contact, hands, and conversation. It makes you more aware of what to look for in real patients.” Another trainee referred to the instructor’s name while answering a question. It suggests that the information that was given by the instructor was a learning source, “As mentioned by Dr. DH, if you smile at someone you expect them to smile back at you”. Additionally, a trainee highlighted the benefit of a combination of different components: “The videos of the children, Jayla and the feedback videos were useful in further my understanding of what characteristics to look for along the spectrum of autism.”
6.2 Interface Comparison (H2)
Our statistical analysis does not demonstrate a statistically significant difference between the two interfaces. This could be associated with the small sample size. Based on the qualitative results, (4 of 16) trainees who experienced the single-view interface mentioned that it was difficult for them to recognize the severity level of JAYLA’s behaviors before seeing the age-appropriate video of JAYLA. Trainees asserted that they had no prior knowledge of what a simulated virtual toddler is capable of doing. However, the trainees who experienced the spectrum-view interface did not describe this issue, and (2 of 14) trainees mentioned that they liked the interface format.
Trainees who experienced the spectrum-view interface had the advantage of comparing and contrasting all three versions of JAYLA, yet this did not lead to a different outcome. To gain insights, we further studied the prior work on the effects of spectrum-view interface design on potential outcomes. We identified two potential factors: 1) JAYLA video size, and 2) The split-attention effect.
JAYLA video size. In the spectrum-view interface, the same display was split among three side by side videos of JAYLA and the examiner, resulting in videos of relatively small size and low resolution. Prior studies show improved comparison task performance with increased display size and resolution (Ni et al., 2006), as well as affecting the appeal and accuracy of retention (Hou et al., 2012). We plan to incorporate larger display sizes with a juxtaposition design to explore the impact in the future.
Split-attention effect. Trainees’ attention was divided between the three views of JAYLA, which could result in a split-attention effect. The split-attention effect is a type of cognitive load that occurs when a modality, such as video, is used to deliver different types of information within the same screen (Castiello and Umiltà, 1990). This requires learners to split their attention between sources of information. While spectrum-view visualization can enhance learning by providing the ability to compare and contrast, the split-attention effect can be an unintended consequence. Therefore, whether the spectrum-view interface design, based on juxtaposition principles, can improve the JAYLA platform needs further investigation.
6.3 Limitations
There are some limitations to this study. First, recruiting medical trainees (medical students and residents) was challenging, leading to a limited sample size. We recruited thirty-four participants from the target user group, but this number was further reduced after excluding four participants because of incomplete surveys. Second, due to time constraints for pediatric residents and the time frame of recruitment, it was necessary to recruit from a diverse population of healthcare trainees. In the future, and informed by the lessons learned, we plan to extend our data with more samples from a broader range of backgrounds. Third, our study did not have a control group who received traditional training (e.g., through a PowerPoint presentation) to have a baseline for comparison. Although having a controlled group could give higher validity to our findings, two main reasons prevented us: 1) lack of a standard ASD training tool to use as a baseline, and 2) a limited number of participants, which led us to explore the most exciting research questions, e.g., comparing the effectiveness of spectrum-view interface with a more commonly used single-view interface. Future studies could explore the effectiveness of JAYLA compared to other available training tools such as text books or PowerPoint.
7 Conclusion and Future Work
This initial exploratory study presents our approach to applying a virtual pediatric patient for presenting a health condition with a spectrum of symptoms and severity levels. The JAYLA platform was designed to teach the variety of symptoms and severity levels of ASD to pediatric trainees. The design is based on various scaffolding strategies with JAYLA as its main component. JAYLA simulates three sets of behaviors that contrast mild and severe autism as well as age-appropriate behaviors in a controlled manner. To investigate the effect of visualization on learning outcomes, two different interfaces, single-view and spectrum-view, were implemented. The spectrum-view interface was designed based on juxtaposition principles to enhance the comparison task. A user study with thirty pediatric trainees was conducted, and the quantitative and qualitative data were analyzed. The results show that the JAYLA platform is effective in teaching important characteristics of ASD and improving trainees’ ability to assess the video vignettes of real children accurately, but we did not find any significant difference between the two interfaces.
As JAYLA’s modular architecture can support interactivity, we plan to utilize more benefits of this virtual patient platform by enabling behavior transitions through trainee voice commands, a feature under development at the moment that would allow trainees to explore the spectrum interactively at any time or place. We also aim to expand the simulations to include moderate autism (closer to severe) in addition to mild autism (closer to age-appropriate) and other important characteristics of ASD, such as joint attention impairment. The JAYLA platform can also be used to train various groups of individuals, such as pre-school teachers and parents. We would like to explore the spectrum presentation further and improve on the spectrum-view interface limitations.
Overall, the JAYLA platform opens the path to a number of exciting training applications, including other disorders with a spectrum of symptoms and severity levels. We hope that JAYLA inspires more research in pediatric virtual patients for training pediatric specialists in a controlled manner in the spectrum of impairment, which can help distinguish the symptoms of each severity level, and consequently lead to a more accurate diagnosis.
Data Availability Statement
The raw data supporting the conclusion of this article will be made available by the authors, without undue reservation.
Ethics Statement
The studies involving human participants were reviewed and approved by the Institutional Review Board (IRB02) of the University of Florida. The participants provided their written informed consent to participate in this study.
Author Contributions
FT, DH, EB, and BL conceived and planned the experiments. FT implemented the application, conducted the user study, analyzed data, and took the lead in writing the manuscript. DH and EB recruited participants for the user study and offered domain expertise throughout. BL provided expert guidance and supervised the research. JG provided critical feedback and general guidance. All authors contributed to the writing and evaluation of the manuscript.
Conflict of Interest
The authors declare that the research was conducted in the absence of any commercial or financial relationships that could be construed as a potential conflict of interest.
Supplementary Material
The Supplementary Material for this article can be found online at: https://www.frontiersin.org/articles/10.3389/frvir.2021.660690/full#supplementary-material
References
Association, A. P. (2013). Diagnostic and Statistical Manual of Mental Disorders (DSM-5®). American Psychiatric Pub.
Bahrani, A. A., Al-Janabi, O. M., Abner, E. L., Bardach, S. H., Kryscio, R. J., Wilcock, D. M., et al. (2019). Post-acquisition Processing Confounds in Brain Volumetric Quantification of white Matter Hyperintensities. J. Neurosci. Methods 327, 108391. doi:10.1016/j.jneumeth.2019.108391
Baio, J., Wiggins, L., Christensen, D. L., Maenner, M. J., Daniels, J., Warren, Z., et al. (2018). Prevalence of Autism Spectrum Disorder Among Children Aged 8 years–Autism and Developmental Disabilities Monitoring Network, 11 Sites, united states, 2014. MMWR Surveill. Summ. 67. 1279. doi:10.15585/mmwr.ss6706a1
Bangor, A., Kortum, P. T., and Miller, J. T. (2008). An empirical evaluation of the system usability scale. Intl. J. Human Comput. Interact. 24. 574-594.
Baraka, K., Melo, F. S., and Veloso, M. (2017). “Autistic Robots’ for Embodied Emulation of Behaviors Typically Seen in Children with Different Autism Severities,” in International Conference on Social Robotics. Springer, 105–114.
Bellani, M., Fornasari, L., Chittaro, L., and Brambilla, P. (2011). Virtual Reality in Autism: State of the Art. Epidemiol. Psychiatr. Sci. 20, 235–238. doi:10.1017/s2045796011000448
Bente, G., and Krämer, N. C. (2011). Virtual Gestures: Embodiment and Nonverbal Behavior in Computer-Mediated Communication. Face-to-face Commun. over Internet, 176–209. doi:10.1017/cbo9780511977589.010 Issues, research, challenges.
Bente, G., Petersen, A., Krämer, N. C., and De Ruiter, J. P. (2001). Transcript-based Computer Animation of Movement: Evaluating a New Tool for Nonverbal Behavior Research. Behav. Res. Methods Instr. Comput. 33, 303–310. doi:10.3758/bf03195383
Bernardini, S., Porayska-Pomsta, K., and Smith, T. J. (2014). Echoes: An Intelligent Serious Game for Fostering Social Communication in Children with Autism. Inf. Sci. 264, 41–60. doi:10.1016/j.ins.2013.10.027
Best, J. (2019). Utilizing Asynchronous Online Modules to Educate Preservice Teachers to Address Bullying for Elementary Students with Asd.
Bloodworth, T., Cairco, L., McClendon, J., Hodges, L. F., Babu, S., Meehan, N. K., et al. (2010). Initial Evaluation of a Virtual Pediatric Patient System
Bousfield, T. (2017). An Examination of Novice and Expert Teachers’ Pedagogy in a Mixed-Reality Simulated Inclusive Secondary Classroom Including a Student Avatar with Autism Spectrum Disorders.
Buescher, A. V. S., Cidav, Z., Knapp, M., and Mandell, D. S. (2014). Costs of Autism Spectrum Disorders in the united kingdom and the united states. JAMA Pediatr. 168, 721–728. doi:10.1001/jamapediatrics.2014.210
Busby, R., Ingram, R., Bowron, R., Oliver, J., and Lyons, B. (2012). Teaching Elementary Children with Autism: Addressing Teacher Challenges and Preparation Needs. Rural educator 33, 27–35.
Burke, S. L., Bresnahan, T., Li, T., Epnere, K., Rizzo, A., Partin, M., et al. (2018). Using virtual interactive training agents (vita) with adults with autism and other developmental disabilities. J. Autism Dev. Disorders 48, 905–912.
Cassell, J., Sullivan, J., Churchill, E., and Prevost, S. (2000). Embodied Conversational Agents. MIT press.
Castiello, U., and Umiltà, C. (1990). Size of the Attentional Focus and Efficiency of Processing. Acta psychologica 73, 195–209. doi:10.1016/0001-6918(90)90022-8
Cheng, Y., Chiang, H.-C., Ye, J., and Cheng, L.-h. (2010). Enhancing Empathy Instruction Using a Collaborative Virtual Learning Environment for Children with Autistic Spectrum Conditions. Comput. Edu. 55, 1449–1458. "doi:10.1016/j.compedu.2010.06.008
Cheng, Y., and Huang, R. (2012). Using Virtual Reality Environment to Improve Joint Attention Associated with Pervasive Developmental Disorder. Res. Dev. Disabil. 33, 2141–2152. doi:10.1016/j.ridd.2012.05.023
Choi, H.-H., van Merriënboer, J. J. G., and Paas, F. (2014). Effects of the Physical Environment on Cognitive Load and Learning: towards a New Model of Cognitive Load. Educ. Psychol. Rev. 26, 225–244. doi:10.1007/s10648-014-9262-6
Dennen, V. P. (2004). Cognitive Apprenticeship in Educational Practice: Research on Scaffolding, Modeling, Mentoring, and Coaching as Instructional Strategies. Handbook of research on educational communications and technology.
Didehbani, N., Allen, T., Kandalaft, M., Krawczyk, D., and Chapman, S. (2016). Virtual Reality Social Cognition Training for Children with High Functioning Autism. Comput. Hum. Behav. 62, 703–711. doi:10.1016/j.chb.2016.04.033
Dukes, L. C., Meehan, N., and Hodges, L. F. (2016). “Usability Evaluation of a Pediatric Virtual Patient Creation Tool,” in 2016 IEEE ICHI, 118–128.
Dyck, M., Winbeck, M., Leiberg, S., Chen, Y., Gur, R. C., and Mathiak, K. (2008). Recognition Profile of Emotions in Natural and Virtual Faces. PloS one 3, e3628. doi:10.1371/journal.pone.0003628
Faras, H., Al Ateeqi, N., and Tidmarsh, L. (2010). Autism Spectrum Disorders. Ann. Saudi Med. 30, 295–300. doi:10.4103/0256-4947.65261
Georgescu, A. L., Kuzmanovic, B., Roth, D., Bente, G., and Vogeley, K. (2014). The Use of Virtual Characters to Assess and Train Non-verbal Communication in High-Functioning Autism. Front. Hum. Neurosci. 8, 807. doi:10.3389/fnhum.2014.00807
Gleicher, M., Albers, D., Walker, R., Jusufi, I., Hansen, C. D., and Roberts, J. C. (2011). Visual Comparison for Information Visualization. Inf. Visualization 10, 289–309. doi:10.1177/1473871611416549
Golnik, A., Ireland, M., and Borowsky, I. W. (2009). Medical Homes for Children with Autism: a Physician Survey. Pediatrics 123, 966–971. doi:10.1542/peds.2008-1321
Hopkins, I. M., Gower, M. W., Perez, T. A., Smith, D. S., Amthor, F. R., Casey Wimsatt, F., et al. (2011). Avatar Assistant: Improving Social Skills in Students with an Asd through a Computer-Based Intervention. J. Autism Dev. Disord. 41, 1543–1555. doi:10.1007/s10803-011-1179-z
Hou, J., Nam, Y., Peng, W., and Lee, K. M. (2012). Effects of Screen Size, Viewing Angle, and Players' Immersion Tendencies on Game Experience. Comput. Hum. Behav. 28, 617–623. doi:10.1016/j.chb.2011.11.007
Hyman, S. L., Levy, S. E., and Myers, S. M.Council on Children with Disabilities, Section on Developmental and Behavioral Pediatrics (2020). Identification, Evaluation, and Management of Children with Asd. Pediatrics 145. doi:10.1542/peds.2019-3447
Issenberg, B., Mcgaghie, W. C., Petrusa, E. R., Lee Gordon, D., and Scalese, R. J. (2005). Features and Uses of High-Fidelity Medical Simulations that lead to Effective Learning: a Beme Systematic Review. Med. Teach. 27. 10-28. doi:10.1080/01421590500046924
Johnsen, K., Raij, A., Stevens, A., Lind, D. S., and Lok, B. (2007). “The Validity of a Virtual Human Experience for Interpersonal Skills Education,” in Proceedings Of the SIGCHI Conference on Human Factors in Computing Systems.
Khoo, E. J., Schremmer, R. D., Diekema, D. S., and Lantos, J. D. (2017). Ethical Concerns when Minors Act as Standardized Patients. Pediatrics 139, e20162795. doi:10.1542/peds.2016-2795
Kotranza, A., Cendan, J., Johnsen, K., and Lok, B. (2010). Simulation of a Virtual Patient with Cranial Nerve Injury Augments Physician-Learner Concern for Patient Safety. Bio-Algorithms and Med-Systems 6, 25–34.
Lopreiato, J. O. (2016). Healthcare Simulation Dictionary. Agency for Healthcare Research and Quality).
Major, N. E. (2015). Autism Education in Residency Training Programs. AMA J. Ethics 17, 318–322. doi:10.1001/journalofethics.2015.17.4.medu1-1504
Mineo, B. A., Ziegler, W., Gill, S., and Salkin, D. (2009). Engagement with Electronic Screen media Among Students with Autism Spectrum Disorders. J. Autism Dev. Disord. 39, 172–187. doi:10.1007/s10803-008-0616-0
Mitchell, P., Parsons, S., and Leonard, A. (2007). Using Virtual Environments for Teaching Social Understanding to 6 Adolescents with Autistic Spectrum Disorders. J. Autism Dev. Disord. 37, 589–600. doi:10.1007/s10803-006-0189-8
Mussap, M., Siracusano, M., Noto, A., Fattuoni, C., Riccioni, A., Rajula, H. S. R., et al. (2020). The Urine Metabolome of Young Autistic Children Correlates with Their Clinical Profile Severity. Metabolites 10, 476. doi:10.3390/metabo10110476
Ni, T., Bowman, D. A., and Chen, J. (2006). Increased Display Size and Resolution Improve Task Performance in Information-Rich Virtual Environments. Graphics Interf., 2006. 139–146. doi:10.1145/1143079.1143102
On Children with Disabilities (2001). The Pediatrician's Role in the Diagnosis and Management of Autistic Spectrum Disorder in Children. Pediatrics 107, 1221–1226. doi:10.1542/peds.107.5.1221
Ousley, O. Y., Arriaga, R. I., Morrier, M. J., Mathys, J. B., Allen, M. D., and Abowd, G. D. (2013). Beyond Parental Report: Findings from the Rapid-Abc, a New 4-minute Interactive Autism. Georgia Inst: Center for Behavior Imaging.Technol
Parsons, S., and Mitchell, P. (2002). The Potential of Virtual Reality in Social Skills Training for People with Autistic Spectrum Disorders. J. Intellect. Disabil. Res. 46, 430–443. doi:10.1046/j.1365-2788.2002.00425.x
Pence, T. B., Dukes, L. C., Hodges, L. F., Meehan, N. K., and Johnson, A. (2013). “The Effects of Interaction and Visual Fidelity on Learning Outcomes for a Virtual Pediatric Patient System,” in 2013 IEEE ICHI, 209–218.
Putnam, C., and Chong, L. (2008). “Software and Technologies Designed for People with Autism: what Do Users Want?,” in Proceedings of the 10th International ACM SIGACCESS Conference on Computers and Accessibility, 3–10.
Ramdoss, S., Machalicek, W., Rispoli, M., Mulloy, A., Lang, R., and O’Reilly, M. (2012). Computer-based Interventions to Improve Social and Emotional Skills in Individuals with Autism Spectrum Disorders: A Systematic Review. Dev. Neurorehabil. 15, 119–135. doi:10.3109/17518423.2011.651655
Rehg, J., Abowd, G., Rozga, A., Romero, M., Clements, M., Sclaroff, S., et al. (2013). “Decoding Children’s Social Behavior,” in 2013 IEEE CVPR.
Roth, D., Jording, M., Schmee, T., Kullmann, P., Navab, N., and Vogeley, K. (2020). (AIVR) (IEEE), 115–122.”Towards Computer Aided Diagnosis of Autism Spectrum Disorder Using Virtual Environments,” in 2020 IEEE International Conference on Artificial Intelligence and Virtual Reality
Smith, M. J., Ginger, E. J., Wright, K., Wright, M. A., Taylor, J. L., Humm, L. B., et al. (2014). Virtual reality job interview training in adults with autism spectrum disorder. J. Autism Dev. Disorders 44, 2450–2463.
Schopler, E., Van Bourgondien, M. E., Wellman, G. J., and Love, S. R. (2010). Cars-2: Childhood Autism Rating Scale. second edition. [Dataset].
Sheldrick, R. C., Maye, M. P., and Carter, A. S. (2017). Age at First Identification of Autism Spectrum Disorder: an Analysis of Two Us Surveys. J. Am. Acad. Child Adolesc. Psychiatry 56, 313–320. doi:10.1016/j.jaac.2017.01.012
Talbot, T., and Rizzo, A. S. (2019). “Virtual Human Standardized Patients for Clinical Training,” in Virtual Reality for Psychological and Neurocognitive Interventions (Springer), 387–405. doi:10.1007/978-1-4939-9482-3_17
Tartaro, A., and Cassell, J. (2008). Playing with Virtual Peers. Bootstrapping Contingent Discourse in Children with Autism.
Tsai, T. C. (2004). Using Children as Standardised Patients for Assessing Clinical Competence in Paediatrics. Arch. Dis. Child. 89, 1117–1120. doi:10.1136/adc.2003.037325
Van de Pol, J., Volman, M., and Beishuizen, J. (2010). Scaffolding in Teacher-Student Interaction: A Decade of Research. Educ. Psychol. Rev. 22, 271–296. doi:10.1007/s10648-010-9127-6
Verkuyl, M., Atack, L., Mastrilli, P., and Romaniuk, D. (2016). Virtual Gaming to Develop Students' Pediatric Nursing Skills: A Usability Test. Nurse Edu. Today 46, 81–85. doi:10.1016/j.nedt.2016.08.024
Vogeley, K., and Bente, G. (2010). “artificial Humans”: Psychology and Neuroscience Perspectives on Embodiment and Nonverbal Communication. Neural Networks 23, 1077–1090. doi:10.1016/j.neunet.2010.06.003
Keywords: virtual patient, pediatric virtual patient, training, simulation, autism spectrum disorder
Citation: Tavassoli F, Howell DM, Black EW, Lok B and Gilbert JE (2021) JAYLA (Junior Agent to typifY Levels of Autism): A Virtual Training Platform to Teach Severity Levels of Autism. Front. Virtual Real. 2:660690. doi: 10.3389/frvir.2021.660690
Received: 29 January 2021; Accepted: 29 June 2021;
Published: 21 July 2021.
Edited by:
Liwei Chan, National Chiao Tung University, TaiwanReviewed by:
Daniel Roth, University of Erlangen Nuremberg, GermanyArindam Dey, The University of Queensland, Australia
Copyright © 2021 Tavassoli, Howell, Black, Lok and Gilbert. This is an open-access article distributed under the terms of the Creative Commons Attribution License (CC BY). The use, distribution or reproduction in other forums is permitted, provided the original author(s) and the copyright owner(s) are credited and that the original publication in this journal is cited, in accordance with accepted academic practice. No use, distribution or reproduction is permitted which does not comply with these terms.
*Correspondence: Fatemeh Tavassoli, ZnRhdmFzc29saUB1ZmwuZWR1