- 1Institute of Special Education, University of Fribourg, Fribourg, Switzerland
- 2Swiss Center for Affective Sciences, University of Geneva, Geneva, Switzerland
- 3Faculty of Psychology, Unidistance Suisse, Brig, Switzerland
- 4Faculty of Psychology and Educational Sciences, University of Geneva, Geneva, Switzerland
- 5Institut de Recherche en Informatique et Systèmes Aléatoires, Lannion, France
Social emotion regulation, which can be understood as the intentional efforts by one person to regulate emotions of another person, is something we encounter and benefit from every day, and becomes especially important when a person is unable to handle an emotion or an emotional event by themselves. A paradigm that examines whether someone can perceive and benefit from regulatory efforts by another person, represented here by a virtual agent, would be highly relevant for experimental studies investigating social emotion regulation, as well as for interventions in the clinical and sub-clinical context. Virtual reality (VR) provides perhaps the ideal opportunity to test social interactions and difficulties with them, as it counters typical methodological problems of behavioral experiments, such as the trade-off between ecological validity and experimental control, as well as the difficulty of replicating social situations. The goal of the present methods paper is twofold: to provide a detailed description of the development of a novel paradigm consisting of two scenarios in VR designed to test the efficacy of social emotion regulation, and to present the anticipated results for the target populations of typically developing and autistic youth. Participants are presented with a virtual school environment and take part in two activities with a class of students and a teacher, all of whom are virtual agents. In both scenarios, participants experience a potentially stressful situation and are subsequently offered emotional support by a friendly student. Throughout the experiment, self-reports in the form of virtual smiley scales and psychophysiological measurements are collected as markers of the participants’ emotional states. Pilot results will be discussed in line with anticipated outcomes, to indicate that the experiment will be able to show the efficacy of social support by a virtual agent and provide insight into social emotion regulation for different populations. The school environment and the character of the friendly student also have the potential to be adapted for follow-up experiments on additional aspects of social emotion regulation for a variety of contexts.
Introduction
Emotion regulation, the process of modifying how one experiences and expresses one’s emotions, is an integral part of a person’s life and highly relevant for their wellbeing (Gross 1998; Gross 2015). Social, or interpersonal, emotion regulation can be understood as the intentional efforts by one person, the regulator, to regulate the emotions of another person, the regulatee (or target) (Reeck et al., 2016; Nozaki and Mikolajczak 2020). It can also be one person’s efforts to regulate their own emotions by receiving and implementing input from another person - this in turn is called intrinsic, as opposed to the extrinsic part played by the regulator (Zaki and Williams 2013). This paper presents a method to study social emotion regulation, from the perspective of the regulatee, by simulating situations of social support in virtual reality, and explores why and how this method could inform research on populations who experience difficulties regulating their emotions in general, and youth with and without autism in particular.
Social emotion regulation is an important aspect in the field of emotion regulation that has grown increasingly in the last decade, with over 350 results on Google Scholar including ‘social emotion regulation’ since 2011, compared to 34 from the decade before. Similarly, for ‘interpersonal emotion regulation’ results grew from 134 to over 2′800 between the same time periods. Social emotion regulation is essential in a variety of contexts, particularly when someone is not able to appropriately deal with their emotions by themselves. Such inability may be caused by age, when a child is still developing their emotional competences (Martin and Ochsner 2016), context, when one’s abilities are temporally impaired (Marroquín 2011), or the magnitude of the precipitating event, when a person is overwhelmed, but it appears to be particularly prevalent for individuals with neurodevelopmental disorders, since they consistently report emotion regulation difficulties (Cai et al., 2018).
People with neurodevelopmental disorders, specifically those on the autism spectrum, typically present with a variety of difficulties in emotion regulation at all stages of the regulatory process. They tend to have trouble with recognizing and understanding emotions and might thus not be able to set appropriate or relevant regulation goals (Mazefsky and White 2014). They are also often less flexible in their choice of regulatory strategy due to a reduced variety of options and the preference for a few, familiar ones, and also tend to have more difficulty implementing them (Samson et al., 2012; Samson et al., 2015a; Samson et al., 2015b; Cai et al., 2018). Difficulties in satisfactorily regulating one’s own emotions can affect one’s social life, but there are additional challenges when it comes to the regulation of another person’s emotions: Failure to correctly identify another person’s emotional state and their need or wish for regulation, the choice of a regulatory strategy not suitable for the other person’s disposition, and the inappropriate implementation of an otherwise sensible strategy, can all be detrimental to the interaction with said person. At the same time, the probability of such failure is highly dependent on one’s social understanding and skills (Nozaki and Mikolajczak 2020) - skills that individuals on the autism spectrum often struggle with (Williams White et al., 2007).
From the perspective of the regulatee, potential difficulties also concern one’s self-regulation abilities. As formulated by (Zaki and Williams 2013), the intrinsic part of the interpersonal regulation process, i.e., the perception and reception of the process by the regulatee, is dependent on one’s ability to label appropriately how one is feeling and to recognize intended safety signaling by others. But whether one can benefit from another person’s attempt is also dependent on one’s social motivation, i.e., the preference of the individual to orient to the social world, seek social interactions and work to maintain social bonds (Chevallier et al., 2013). It is not only: Do I understand regulation attempts by others? But also: Do I seek them out and/or appreciate them? This is particularly important, since research suggests reduced social motivation as a main characteristic in autism (Chevallier et al., 2013; Treichel et al., 2021). When taken into consideration with the many significant points raised above, the study of social emotion regulation becomes particularly important for people with emotion regulation difficulties and/or social interaction in general and for individuals on the autism spectrum in particular, in order to gain a more profound understanding of the processes involved in order to provide targeted support and interventions in the clinical and non-clinical context.
The study of social situations and interactions faces the perennial methodological problems of getting the balance right between ecological validity and experimental control, and the difficulty of replicating situations including the verbal and nonverbal behavior of a person (Blascovich et al., 2002; Meuleman and Rudrauf 2018). These problems can undoubtably help explain why the experimental research on social emotion regulation is relatively restricted, in spite of its importance. Many papers deal with its theoretical implications and connections with other constructs, be it a collection of regulation strategies (Niven et al., 2009), an integration with the concept of empathy (Zaki, 2020), or the role of maternal co-regulation for the development of socio-emotional competences (Silkenbeumer et al., 2016). Others with a more empirical approach rely on recall and self-assessment about social emotion regulation skills, strategies and goals (Williams White et al., 2007; Coats et al., 2008; Hofmann et al., 2016; Liddell and Williams 2019; Chan and Rawana 2021). There are notable experimental paradigms used in related fields, such as the manipulation of physical closeness by having mother-daughter dyads either hold hands or not, and examining its impact on so-called ‘load sharing’, the distribution of the load of emotional distress among relationship partners (Lougheed et al., 2016), and also non-experimental studies like the exploratory observation of co-regulation tactics employed by mothers and their children with autism (Gulsrud et al., 2010). Still, re-enactment or simulation of the actual, relevant situation for the experimental assessment of social emotion regulation is as rare as it is difficult. One way to bypass these problems was chosen by Hallam and colleagues in an fMRI study, who showed participants pre-recorded videos of other people supposedly watching the same emotional video clip as they were at the same time and asked them to help the others regulate their reactions (Hallam et al., 2014).
Virtual Reality thus enriches this area of research by allowing us to take a look at social interaction in a maximally controlled environment, using virtual agents as either the intended regulator or regulatee for the participant. By developing relevant environments and scenarios, we now have the ability to untangle social interaction in concrete situations which a person can experience more acutely, even possibly enhancing relevant stimuli, while at the same time benefiting from a unique level of standardization and control. This is done with the expectation that virtual social cues can elicit affective experiences and reactions equivalent to, or at least very similar to, those of the real world (for a review of relevant studies, see Bombari et al., 2015).
The two virtual scenarios created in this current project focus on social emotional support. Social support can be defined as an individual’s or a social network’s “provision of psychological and material resources intended to benefit an individual’s ability to cope with stress” (Cohen, 2004, 676; Nozaki and Mikolajczak, 2020). It can thus only be considered a strategy of social emotion regulation, when defined as intentional, not contextual, and specifically focused on emotion. We operationalize the term here as an offer of a supportive regulatory attempt towards a person experiencing emotional distress and focus on the receiving end, the regulatee.
The backdrop is a novel school environment with the participant being introduced as a new student and experiencing their first day at school. By making the participant the regulatee and a virtual agent the regulator, we aim to assess the impact of emotional support by another, rather unfamiliar person on one’s emotion regulation. This will be assessed in the two different situations and with two different populations here, namely school-aged children and adolescents who are either typically developing or who are on the autism spectrum and who have no intellectual disability. Each scenario provides a situation to which people respond negatively: the first concerns an ambiguous or confusing situation of being scolded by a classroom teacher. An agent, a fellow student, who has previously been established as friendly towards the participant, then gives a positive appraisal of the situation. The idea is that the participant can adopt this for their own cognitive reappraisal, the reinterpretation of emotional events, which has been shown to be a potentially highly apt self-regulation strategy (Gross, 2002). The second scenario concerns being excluded from a group in the context of a ball game, an adaptation of the Cyberball paradigm (Williams and Jarvis, 2006). After experiencing exclusion, once again the fellow student offers support in the form of a positive appraisal of the situation, but this time it is juxtaposed with two other forms of social support a person could benefit from: distraction by the virtual agent away from the exclusion situation, a strategy aimed at emotional disengagement (Sheppes et al., 2011), and social buffering, an effect capable of ameliorating stress responses through the sheer presence of another person. Social buffering occurs in many situations although not always necessarily intentionally (Zaki and Williams, 2013; Bratec et al., 2020), and can be seen as a baseline to every condition including the agent.
These regulatory strategies and/or processes are situated at different stages of the social emotion regulation process, are employed to differentiate potential layers of a discovered effect, and provide evidence of the strategies to be focused upon in the future, since they might differ in efficacy. This is addressed in the Cyberball conditions and not in the randomized Classroom conditions, due to practical assessment restrictions concerning the large number of subgroups (particularly when recruiting clinical populations) that would be necessary to vary three forms of support over three conditions. In terms of autistic individuals, there is reason to believe that distraction as an attentional deployment strategy might be an effective strategy, while cognitive reappraisal might not be (Samson et al., 2012). Social buffering is believed to be reliant on at least a normatively well-established relationship with a person (Bratec et al., 2020) that this experiment might not be able to create.
The goal of these scenarios is thus to present the participant with relatable virtual situations that trigger negative emotional responses and subsequently offer emotional support through an agent. To achieve this, a number of areas have to be covered: First, the main target groups are school-aged children and adolescents with and without developmental disorders, with these being at critical stages in their life concerning their emotional and social development (Samson et al., 2015a; Volkaert et al., 2020). The scenarios therefore need to be developed with this age group in mind. Second, the context and environment should be engaging and interesting to the participants, while still being standardized enough for experimental conditions. Thirdly, the relevant virtual characters need to be able to form a relationship with them, since social support is partly dependent on the relationship between the regulator and the regulatee (Reeck et al., 2016; Lindsey, 2020). And lastly, the emotional reactions need to be measured reliably in a way that is accessible to children. The explanations of how we worked towards this goal will be supported by results of a pilot study on a typically developing adult population and by feedback from youth with and without developmental disorders.
This article presents a method suitable to study social emotion regulation in virtual reality. Using said method, our ongoing study of our target group of children and adolescents serves as an evaluation of the virtual environment for the purpose of assessing the efficacy of virtual social support, and as a step towards a wider approach of studying social emotion regulation in VR.
Gaining insight into this part of the process of social emotion regulation will ultimately help uncover the kind of support that can and should be provided to young individuals struggling with emotion regulation. This includes finding out the type of regulatory strategy to focus on and the direction of a training, either towards understanding the intent and content of the regulatory attempt, or towards a more habitual call for support from others.
Materials and Equipment
Hardware and Software Needed for the Experiment
The virtual world was designed and programmed in Unity3D 2018.2.21f1 and C#, with the visual editing, modeling, animation, sound production and other aspects developed using a list of other software (see Supplementary Material). The Unity Asset Store provided two sets of VR avatars that were purchased to create the virtual human characters populating the school grounds, Toon people for the teacher (JBGarraza, 2021b) and Toon kids (JBGarraza, 2021a) for the students. Other Unity assets were obtained for free (see Supplementary Material) or developed in-house. Character voices were recorded on a Zoom H5 portable recorder for both a German and a French version.
The experiment is run on Microsoft® Windows 10 in Steam® VR 1.13.10 using the Unity3D game engine and hardware of the HTC VIVE© line, consisting of a head-mounted display with built-in microphone, one wireless hand-held controller and two base stations. Depending on the location of the session, in lab or off-site, the set is either from the VIVE© Pro series with built-in audio straps and base stations and controllers 2.0, or from the VIVE© series with additional headphones (Beyerdynamic Custom Street) and base stations and controllers 1.0. The sessions are recorded as in-game screen capture and externally as camcorder footage from a corner of the room, using a Canon Legria HF R806. The participant spends the experiment sitting in a revolving chair with the possibilities to turn around in it freely and use the controller for any other movement and interaction.
Emotion Self-Reports
As the main measure of emotional experience, two 4-point smiley scales were created to pop up one after the other as rows of floating boxes in the virtual environment and to be grabbed by the VR controller whenever a self-report reply is required (see Figure 1 for a picture of the negative scale). The scale always appears in front of the participant. One scale is of negative valence, one of positive, with accompanying voice messages by a robot named Marvin (see Figure 1), stating respectively “please state how negatively you are feeling right now” and “please state how positively you are feeling right now”. The scales consist of the categories “Not at all”, “A little”, “Medium” and “Very” and are read aloud by Marvin each time the controller touches the respective smiley. For the benefit of the reader, the English translation of the original language versions is used here (see Supplementary Material, Section 2, for the other languages). The respective smiley is chosen after being grabbed by the participant for 3 s and there is no time limit for the response.
Psychophysiological Measurement
For the relevant physiological signals, Biopac® Bionomadix 2-CH Wireless hardware is used, with the recording software Acqknowledge 5.0.2. The four domains collected are pulse (PPG), skin conductance (EDA, exosomatic with direct current), respiration (RSP) and heart rate (three-lead ECG), thus requiring the two joint transmitters for PPG-EDA and RSP-ECG (BN-PPGED-T and BN-ECG2-T respectively). For EDA, one-way adhesive gel electrodes (EL507) are attached to the middle and fourth finger of the non-dominant hand, while one-way adhesive cloth snap electrodes (EL513) are also used for the ECG. Said hand is then rested on the armrest of a revolving chair the participant is asked to sit in, to reduce artifacts in the recording. The recording includes trigger points at important events throughout the experiment that are being fed into the recording from the SteamVR log through a Neurospec trigger box converting from a USB serial device to a parallel port. To ensure an accurate interpretation of the collected data, a baseline period of 3 min is collected early in the experiment. Post-processing scripts were written using Python 3.0 and the integrated development environment Spyder. Kubios HRV 2.2 is used for manual artifact correction and calculation of heart-rate values.
Post-Experiment Questionnaires
A VR questionnaire (VRQ) administered post-experiment consists of demographic information, an item on whether the participant has already experienced immersive VR to check for potential effects of experience, and several open or mixed questions concerning their experience of the VR experiment, designed to help improve the environment in the future: They are asked how they found the VR experience in general, whether they noticed anything about the experiment, how they experienced the steering/control inside the environment (with a 5-point difficulty scale and free lines to comment), whether they would change anything about the experiment, and whether they found the virtual characters likeable. In addition, as a manipulation check for the social relevance of the presented virtual agents, participants are questioned about the two most notable students of the school environment, specifically, the friendly student offering social support in both scenarios, and the “troublemaker”, the student disrupting the class in the Classroom scenario. Their perceived relationship with the two characters is evaluated with two 5-point scales on valence and closeness of the relationship each, and participants are given the opportunity to comment verbally to add to their responses on the scales, and about whether they felt that the two virtual agents had any impact on their own behavior, again with two 5-point scales to measure the extent and valence of that impact plus a further opportunity to comment verbally. The questionnaire ends with a manipulation check on the exclusion and social support of the Cyberball scenario: participants can check the respective boxes whether and in which games they felt included or excluded, and are asked what they think about the friendly student’s arrival after the third ballgame, and whether they felt comforted by him (see procedure in 3.1.3 below). In case the participant has trouble understanding the scales and questions, the questionnaire is completed with the help of the experimenter.
Methods
Overview of the Experimental Procedure
The experiment was performed in accordance with the Declaration of Helsinki and approved by the local ethics committee. When participants arrive and have given their informed consent, or if their parents have given theirs when the potential participant is under 18 years old, the participants are instructed to sit on a revolving chair in the center of the experiment room, calibrated as the starting position for the VR equipment. They are free to turn and move their arms in every direction to orientate themselves and take in the visual experience of the virtual world.
All four points of psychophysiological measurements (PPG, EDA, RSP, ECG) are attached on the body and the non-dominant hand, with the experimenter showing the resulting signals on screen and describing their general function. Then the HMD and handheld controller are explained and the experimenter outlines the order of events that is to come: First a tutorial is given of how to use the controller and interact with the environment, secondly there is a break of 3 minutes to create a baseline for the physiological measurements, thirdly there is a situation in the classroom with the new class, and lastly, there are ball games in the school’s gymnasium. The participants are instructed that they can stop the experiment at any point if they feel dizzy or have other problems and that the experimenter will always be near to help. After this explanation and when they have no more questions, the participant puts on the HMD, receives the controller for their dominant hand, and is ready to start the experiment. The camcorder recording the session is started.
The VR experiment itself consists of the forementioned introduction to the novel virtual environment, including the tutorial of about 2.5–4 min, and a 3-min baseline period for the psychophysiological measurements, a first “Classroom” scenario of about 8.5–10 min, then a “Cyberball” scenario of about 7.5–8.5 min, followed by a reward sequence at the end. In total, completion of the VR experience should take 25–30 min—depending on the participant’s reaction times and how much they choose to explore the environment. When it is finished, the experimenter helps the participant remove the HMD and the different points of physiological measurement. Once the participant has completed the post-VR questionnaire, and when they have no further questions or comments, they are remunerated for their time, thanked, and free to leave.
General Aspects of the Environment
The developed virtual environment consists of the school grounds which are accessible from a front gate on a suburban looking street. The front gate functions as the starting point of the participant’s experience. Implementations in the virtual world to make it more immersive include explorable features of the grounds like green and planted areas with grass and leaves moving in the wind, picnic and play areas on the playground, additional classrooms (empty of people), a hot-air balloon (relevant for the ending of the experiment), a pond, a fenced-off forest at the back, and a rotating windmill and mountain scenery visible in the distance. In addition, sound is spatialized, characters move their lips in synchrony with voice recordings, and there are environmental sounds like birds chirping noisily outside (and quieter inside) and the students’ excited cries while playing ball in the gymnasium. To add to the playing experience during the Cyberball games, every throw from the participant results in a celebratory sound, while there is no score being kept to avoid inducing competitiveness.
Several times throughout the experiment, after a virtual character has announced a next step or task, glowing circles and lines on the ground are incorporated as a visual guide to where or in which direction to teleport. Following the instructions and teleporting to the appropriate place acts as a trigger for the next step of the experiment to begin. This means, while participants can often teleport around freely and discover the whole map, the experimental paradigm does not continue unless they follow the lines or enter the circles. Indeed, participants are eventually encouraged by the experimenter to resume their places and tasks when they have decided to roam the school grounds instead. Other characters of the virtual world only interact with the participants when the latter are following the instructions and partaking in the scenarios, keeping the experience as standardized as possible without constricting wishes to explore.
The Introduction in Virtual Reality
The participant starts off at the front entrance of the school grounds and is greeted by Marvin, the school’s janitor robot. Marvin welcomes the participant at their new school and demonstrates how the controller can be used to grab virtual objects with a button activated by the index finger (holding the object until the button is released) and teleport to a spot of one’s choice within a certain distance using the directional pad activated by the thumb (Figure 2). The emotion smiley scales are explained and presented in-game for the first time and, after a successful response on both, a star appears that can be collected (Figure 3). Marvin states that he would be delighted if the participant could collect stars that they might encounter during their day, since he has lost his and is looking for them. In fact, a star appears each time the participant has completed an important step in the two scenarios. When practicing the teleportation method, the participant is asked to teleport into a white circle marked on the ground in front of an easel carrying a map of the world (visible in Figure 2). The participant then has to wait for 3 minutes looking at the world map, before Marvin continues the experiment by inviting the participant to go to their class. A blue line on the ground shows them where to teleport, step by step, but they are also free to explore the school grounds before finally arriving in front of their class for the experiment to continue.
The Classroom Scenario
Once the participant arrives in front of the classroom in a marked blue square, they are invited in by the female teacher. When inside, the participant is asked to introduce themselves to the other students. The introduction task ends either when the experimenter presses a button, or automatically after 10 s. After introducing themselves to the class, participants can collect a star appearing on top of the teacher’s desk as a reward. The teacher then asks the participant to sit down next to student Pete, a place marked by a white circle, and Pete introduces himself and also asks the participant to sit down next to him. Pete is the friendly student who will provide support throughout the paradigm (see Figure 4). He is, like the other students, a more cartoon-like than real-looking human character, since this has not only been shown to be more readily accepted by people, but also to be perceived as more friendly (McDonnell et al., 2012; Ring et al., 2014). Since studies evaluating the difference a virtual agent’s gender may make on their effect on participants have as yet not been conclusive (Krämer et al., 2016; Shang et a., 2019), and to keep the design simple, it was decided on the toss of a coin that the virtual agent would be designed as a boy (see Section 4.1 for a discussion of this point). He is the first and only student of the class who directly talks directly to the new arrival, is seated closer to him than anyone else, and is the only one who introduces himself, thus establishing an at least normatively special and positive (or at the very least, neutral) connection to the participant. The teacher then explains they will be watching a movie (A short introduction of the Hubble Telescope in the respective language). The participant is asked how they feel at this stage and the smiley scales are presented. When successfully answered, the scales disappear and the movie starts. During the playing of the movie, the participant cannot teleport away from the seat they are in (setup seen in Figure 5).
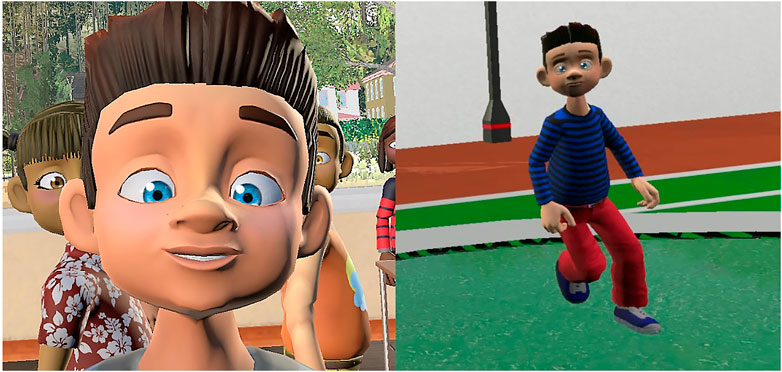
FIGURE 4. Pete, virtual agent and supportive fellow student: face and body (assets by JBGarraza, 2021a).
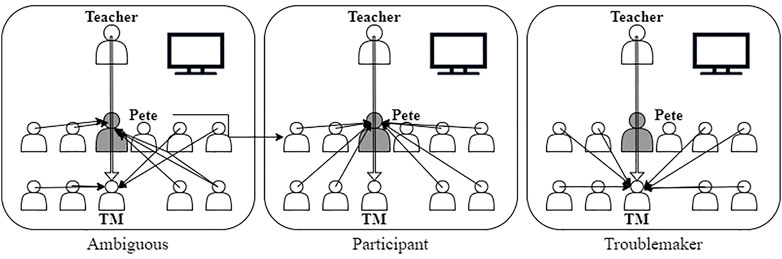
FIGURE 5. Classroom setup in the Classroom scenario, going through three possible intensity conditions of stress induction concerning the direction of the student’s gazes towards the (dark grey) participant in the center: Ambiguous, Participant, Troublemaker (TM).
The teacher interrupts the movie three times, forming three different conditions of different intensity of stress induction presented in a randomized order across participants. Each time, the participant is asked twice how they feel and the negative and positive smiley scales appear: First, the teacher scolds a student, then Pete says he is not sure about the cause of this and the participant is prompted with the scales for the first time. For the second time, the teacher resumes the scolding, next Pete offers emotional support and the participant is prompted with the scales once more. This effectively splits the situation into two halves that can be compared later in their effect on the participant, one without support and one with social support (see Figure 6, tCR1 and tCR2).
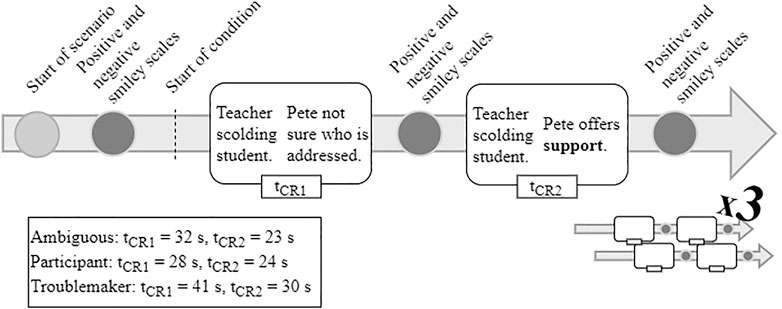
FIGURE 6. Sequence of each condition of the Classroom scenario with the three conditions in a randomized order.
In the “Ambiguous” condition, the teacher scolds someone who apparently has made noises, while looking directly at the participant. Pete explains he is not sure whether the participant is addressed. The other students are either looking at the participant (half of the class) or the student behind them, the “troublemaker” (other half of the class, see schematic depiction of the three conditions with lines of sight displayed in Figure 6). Pete proceeds to comfort the participant by saying that it was probably not them who was the target of the scolding.
In the “Participant” condition, the teacher also scolds the person who has made noises, while looking directly at the participant. Pete again says he is not sure whether the participant or the troublemaker behind them is the target. This time, the other students are all looking at the participant. Pete again comforts the participant and explains that they were probably not the actual target.
In the “Troublemaker” condition, the teacher again scolds the person who has made noises, while looking directly at the participant. Again, Pete expresses uncertainty about whether the participant is the target. This time, the other students are all looking at the troublemaker, the student behind the participant. Pete comforts the participant again, saying that they were probably not the target.
After all three conditions have appeared, the movie ends and the participant can collect a star as a reward, after which the teacher dismisses the class and the students leave the building.
The Cyberball Scenario
The participant is told by the voice of Marvin that Pete is waiting for them in the hallway. After they have joined Pete in the hallway, Pete tells them that the students are playing ball games in the gymnasium and that the participant can join each game once one of the three students playing each game leaves. The play area is indicated by a blue circle. The participant is asked to follow Pete towards the gymnasium as he continues to walk along a blue line on the ground. Once they arrive, a slingshot-like throwing device or bat appears on top of the participant’s controller (see Figure 7).
Pete explains that they can use the button activated by the index finger on their controller to throw the ball when it has been thrown at them, and leaves again. The participant then goes through three consecutive games of 60 s each, with play areas spread inside the building (also seen in Figure 7), constituting three conditions in a fixed order. The different conditions, inclusion in the game to exclusion from the game to then exclusion with the offer of emotional support, can then be compared in terms of their effect on the participant.
Each play area can house three players for a ball game, although the first one only has two players as the participant enters the room, allowing the participant to begin immediately. The second and third game can only be joined once one of the playing agents has left the relevant play area. After 50 s of each game, the participant is asked how they feel with the smiley scales. Then, 15 s after each game has finished, they are asked again. During each game and the 15 s afterwards, the participants are not allowed to leave the play area and can only teleport around inside of it. After they have picked their answer on the second smiley scale, they are free to leave the area and can join the next one once one of the other players has left. The three conditions are shown in Figure 8.
In the first (Inclusion) condition, two students play with the participant, with the participant generally receiving the ball a third of the time, i.e. at the same frequency as the other two players.
In the second (first Exclusion) condition (without social support), two students throw the ball to the participant for the first two times. After that, they exclude the participant completely for the rest of the game.
In the third (second Exclusion) condition (with social support), two students throw the ball to the participant for the first two times and afterwards exclude the participant completely for the rest of the game. After the game, Pete joins the participant standing in the gym and there are three options for the intended social support, varying randomly between participants: either Pete is only present and keeps quiet for 15 s (s), or he talks to the participant about information related to ball playing for 15 s and thus offers distraction, or he talks to the participant, offering a positive appraisal of the situation for 15 s. As a final reward, a star appears and can be collected.
The Reward Sequence
After the last star has been collected, robot Marvin’s voice invites the participant to take a hot-air balloon ride over the school grounds. Marvin, the teacher and all the students are already waiting next to the balloon. Marvin explains that he would like to reward the participant’s successful star collecting with a balloon ride together with Pete. This also serves as a positive final experience to leave participants with the best possible emotional state after having gone through the two scenarios created to induce a negative emotional reaction. After everyone has started clapping and the participant has teleported into a white circle where Pete is already standing, they are automatically transported into the balloon that begins to take off. A tune is played and a few stars dance around the basket of the hot-air balloon while it is slowly rising up. The surrounding neighborhood and landscape come into view. At this point, the experimenter helps the participant remove the HMD.
Pre-Processing
The two data sources for pre-processing and analysis are the SteamVR data and the Acqknowledge recording of psychophysiological data in reference to the relevant time markers transferred from the VR experiment. The smiley self-reports, coded as values from 0 to three for each scale, are exported from the SteamVR log in long-format single file and ready to be analyzed. The Acqknowledge recording is converted to a Matlab file and slices for the relevant time frames, or epochs, are extracted for the two domains that are in focus here: skin conductance and heart rate. For both domains, the epochs are each half of each condition in the Classroom scenario (Figure 7, ta1 and a2, tp1 and p2, tt1 and t2), each condition in-game and during recovery period respectively in the Cyberball scenario (Figure 8, tc1 and tc3) and the last minute of the baseline period at the beginning.
Individual skin conductance level (SCL, tonic EDA) minimum and mean values for these slices are exported and range-corrected using the minimum of the baseline and the formula suggested by Lykken et al. (1966):
The heart rate signal slices are loaded into Kubios and artifacts are corrected manually, before the two variables, mean heart rate (or beats per minute) and root mean square of differences (RMSSD) between interbeat intervals, are computed. The latter being a measure of heart rate variability that can be used with ultra-short time spans of 1 minute and lower (Nussinovitch et al., 2011).
Having prepared the data in this way, it is possible to analyze the different variables using repeated-measure ANOVAs and paired-sample T-tests over the different conditions.
Results
Pilot Results: Implementations and Adjustments
The presented paradigm includes two scenarios: Classroom and Cyberball. A pilot study using an earlier version of the paradigm (article submitted, Stallmann et al., 2021) with a sample of 29 typically developed adults recruited through online study advertising and university bulletin boards, showed that the experimental design (here with a focus on the Cyberball scenario) was able to elicit the desired emotional responses: participants reported more negative and less positive emotions in the exclusion conditions than in the inclusion condition, and less negative and more positive emotions after having received social support. However, the psychophysiological measures, specifically electrodermal activity and heart rate variability, while suggesting differences between conditions, were inconclusive. When asked an open question on how they liked the experiment in the earlier, less detailed version of the post-experiment questionnaire, participants reported that they experienced it as positive and interesting or exciting. The tutorial at the beginning was accepted well by the participants, there were no dropouts, and everyone managed to get through the experiment using the controller. Still, due to a few observations during this earlier pilot and other concerns regarding our intended target populations, certain aspects and details in the design have been modified and are presented below.
After taking into account the intended target population of people with developmental disorders and pre-testing of the scale with children, the positive and negative smiley scales were reduced from a 5-point to a 4-point scale, since 5-point scales seemed to overwhelm the children in the pre-test. As this scale is also part of other connected studies and integral to our emotion report, young participants are presented with the scale beforehand, either on a touch screen or with the help of cardboard boxes, in a short training session in which its logic is applied to real-life situations familiar to the participants. Another adjustment for a younger and potentially less physically mobile or cognitively impaired population was the implementation of a non-self-steering mode: if the experimenter sees the participant having acute trouble with teleportation during the tutorial, the self-steering can be modified so that the participant can confirm whether they would like to be teleported to a predetermined spot in their visual field via the grabbing button at their index finger. This continues throughout the experiment and enables the experience to be as close to the self-steering one as possible.
Other adjustments making the relevant points of comparison as standardized as possible affected the Cyberball scenario: Having the three play areas spread across the playground, as it was in the previous version, prompted some participants to leave the ongoing games when they were getting bored (for example, when they were excluded) to explore the rest of the school grounds. To possibly instill a stronger feeling of commitment and make the self-report and psychophysiological data more comparable, the setting was changed to that of a gymnasium, making it less of a free-time activity and more of the continuation of school hours. Importantly, the play areas are also enclosed by an invisible wall from the point the participant enters them to play until they have responded to the second smiley scales 15 s after the ball game has finished. This restricts movement, distraction and other confounding influences on the comparison of the conditions.
Further testing is being carried out with the current version of the presented paradigm and using the current post-experiment questionnaire. This time, 30 typically-developing youth and 30 autistic youth without intellectual disability will take part for statistical analysis of the self-report and psychophysiological data (see Section 4.2 for sample size calculation). Taking a first, descriptive look at the questionnaire data, 20 of the 23 participants tested so far (age range: 9–17 years, four of 23 on the autism spectrum), recruited through sports clubs and through associations, gave positive feedback when asked about their general experience, and there were no dropouts. When focusing specifically on the four participants on the autism spectrum, two reported finding the experiment interesting and fun, while the other two found the experiment to be made for children younger than them. Steering through the environment was found to be easy, scoring a general average of 1.4 on a difficulty scale from 1 to 5, with three of the 23 participants marking a three and everyone else answering lower. This experiment thus promises to be indeed quite accessible to a younger target group.
The participants rated their relationship to Pete, the supportive student, on average as rather close (3.9 on a scale from 1 [very distant] to 5 [very close]; SD = 1.0) and rather positive (4.3 on a scale from 1 [very negative] to 5 [very positive]; SD = 0.9), while that to the “troublemaker”, the disruptive student, was judged as rather distant (M = 2.0; SD = 1.0) and rather negative (M = 2.2; SD = 0.9). Concerning the Cyberball scenario, the majority of the participants (13 of 17 filling in the respective items of the questionnaire) felt included only in the first ball game. 11 of 17 felt excluded in both the second and third ball game, while four people only felt excluded in either the second or third. When being asked what they thought of Pete’s appearance after the game, 12 of 17 participants responded positively about it, and when specifically asked whether he had been a comfort to them, 9 of 17 agreed that indeed he had. When focusing only on the participants grouped into the two support conditions in which Pete is talking to the participant (appraisal and distraction), 8 out of 11 agreed.
While the manipulation seems to be adapted to this young population, this also shows the limits of the experimental situation created. Clearly, not everyone will react in the same manner in a social situation: four participants reported that they did not feel supported since Pete had not stayed with them during the games, but only reappeared afterwards. Furthermore, the aspect of it being virtual adds another possible filter: Two participants expected Pete to present them with the next task of the experiment instead of coming to support them, as he had already played a big part in structuring the other two scenarios, representing a potential bias because of one’s expectations about the structure of computer games. One participant stated that they did not care about Pete being there at all, since everyone was computer-generated anyway.
One open question concerns the importance of the supportive agent’s gender, as current research has not yet established the specific contexts in which either same-gender or cross-gender interactions might be more beneficial (Krämer et al., 2016). While the questionnaire data indicates that both male and female participants accepted Pete (a boy) equally, as intended, future research could include a design to evaluate the role the regulator’s gender might play in social emotion regulation, and adaptations of the paradigm for more individual and targeted intervention approaches could consider more variability in the regulator’s appearance.
Anticipated Results
When conducting future studies on the two targeted populations of typically-developing and autistic children and adolescents, we expect a pattern similar to that of a previous version with adults to emerge. Considerations of feasibility, distributional convergence of the data and of a-priori power analyses using G*Power 3.1.9.7 (Faul et al., 2007) resulted in a goal of 30 participants per group.1 Looking at the negative smiley scale, participants should report more negative emotions when being confronted with the difficult situations of both scenarios, and they should benefit from the support offered by virtual agent Pete.
Getting potentially scolded by the teacher and having the whole class look at you should create a more intensive negative reaction than the potential scolding in a situation in which everybody else is looking at a different potential perpetrator. For the Classroom scenario, we would thus find a condition effect, with reported emotions being most negative in the Participant condition, when all students are looking at the participant, and least negative in the Troublemaker condition, when all students are looking at the troublemaker. In addition, we would expect the support offered by Pete to have a positive impact (see Figure 9 for a depiction of these anticipated results). Given the differences in the reception of social cues individuals on the autism spectrum tend to show, they might be less susceptible to the awkwardness of the ambiguous situation and thus report less negative reaction to it when all students are looking at the participant in the Participant condition. On the other hand, they might also benefit less from the support offered by Pete.
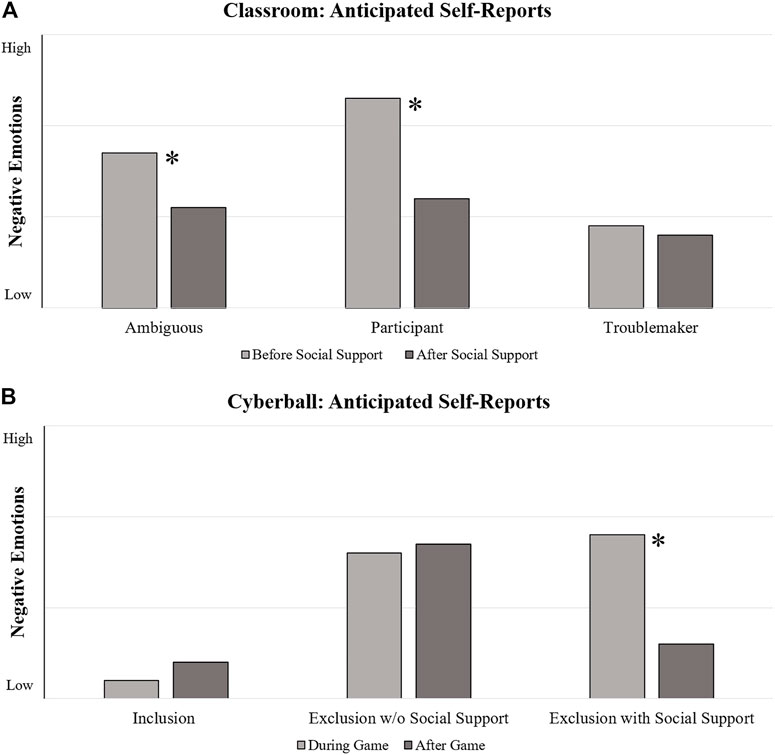
FIGURE 9. Anticipated negative self-report results for both scenarios, Classroom (A) and Cyberball (B), in the typically developing sample. Significant effects are marked with an asterisk.
For the Cyberball scenario, being excluded in the ball games should result in more negative self-reports than being included and Pete’s support should again alleviate negative reactions (compare Figure 9), but it is again possible that individuals on the autism spectrum might not benefit as much from the social support offered.
The three different types of support Pete offers towards the ending of the Cyberball scenario, presence, distraction and reappraisal, are expected to differ, although results might vary, and reappraisal might be more effective for TD youth than for autistic youth (Samson et al., 2012), while the presence condition is expected to be the least effective, given that Pete is always present.
Concerning the psychophysiological measurement, with the order of the ambiguity conditions randomized and the movement of the participant restricted and reduced to potential head turns, the Classroom scenario presents a cleaner measurement situation than the Cyberball scenario. We would expect the most stressful condition, the participant condition, to induce the highest arousal, and the least stressful condition, the troublemaker condition, to induce the lowest arousal. When separating the first half of each condition from the second half (ta1, tp1, tt1 from ta2, tp2, tt2), expectations become less clear. Receiving support from Pete in the second half might lower arousal levels compared to the first half, but any spontaneous reactions like excitement and appreciation towards Pete, would blur this result.
The Cyberball scenario with its definite order of events does not offer the ultimate context for physiological measurements, since order effects might occur and participants move relatively often but inconsistently to catch and throw balls. Taking into account findings by Iffland et al. (2014) who worked on the physiological effects of social exclusion in Cyberball, one would expect a decrease in skin conductance level and an increase in heart rate (potentially accompanied by a decrease in heart rate variability) over the course of the three conditions.
The interpretability of these physiological results is limited, given the restrictions of the paradigm and the expectation of inconsistent movement by the participants. Still, one of the advantages of this virtual experience is that it is closer to a real-life experience and people are more physically active to play ball. The results can thus offer additional data to support the findings of the self-report measurements and act as a check on whether the experiment is indeed able to elicit emotional reactions, also on a physiological basis.
Discussion
This new VR experiment was developed to assess an aspect of social emotion regulation in youth with and without neurodevelopmental disorders, namely the impact of social support on one’s self-regulation. This was achieved by creating two scenarios triggering negative emotional reactions and a virtual agent offering emotional support to help with regulation in a school environment.
Early results, including the outcome of a pilot realized with an adult sample, and insights from feedback by school-aged children and adolescents, reveal the potential of this environment for future studies. These early results were obtained after careful development of a relatively large, explorable and realistic environment, complete with a janitor robot, a class of students and a teacher, characters who can be interacted with at certain points. Participants are able to engage with the virtual world, form relationships with the relevant virtual agents and report emotional reactions through the use of smiley scales.
Results on the efficacy of virtual social support, after the induction of a negative emotional reaction, in the two samples of typically-developing and autistic youth with no intellectual disability will, firstly, inform the study on autistic traits on potential new avenues for research and training. Current research increasingly suggests reduced social motivation as a main factor in autism characteristics (Treichel et al., 2021) rather than impairment of social cognition. More detailed results about whether and how much individuals on the spectrum would accept and benefit from social regulation would inform current and future training programs on emotion regulation and social skills.
Insights on the more impactful strategies of social support, be it reappraisal, distraction or even presence only, will also help with future developments of both assessments or training. Reappraisal is the most dependent on the regulatee’s understanding of the intent and content of the regulatory attempt, while presence is the least dependent. However, the simple presence of someone else, although helpful, is likely to be the least effective support given the participant’s short acquaintance with regulator Pete. In any case, it is useful as a baseline condition for the other social support conditions. At the same time, presence would also be the least effortful strategy for the regulator, once the regulator is not computer-generated anymore.
This leads to a promising route for the future of this method: Having established the virtual environment as engaging and even enjoyable, and the virtual characters as socially relevant, the environment has great potential as a basis for other research programs on social emotion regulation and perhaps even in social interaction, more generally. Research paradigms could most obviously include role-reversals with participants being asked to offer support to virtual agents, thus evaluating the other side of the interaction, although many directions could be envisaged given the possibility to create different social and non-social scenarios, and integrating other possible regulation strategies offered.
While adaptations and further developments of this particular environment are possible and promising, the experiment itself also encourages virtual reality to be used more frequently as a general method in the study of social interactions and emotions. There are a number of applications for immersive virtual worlds to date in rehabilitation programs (Howard, 2017), vocational training (Bernardo, 2017; Burke et al., 2018), and also in a line of social-skill trainings for people on the autism spectrum (Parsons and Cobb, 2011). With virtual reality becoming more accessible for private households, training programs and serious games have the potential to reach a wider audience and expand the duration of their programs almost indefinitely.
Data Availability Statement
The raw data supporting the conclusions of this article will be made available by the authors, without undue reservation.
Ethics Statement
The studies involving human participants were reviewed and approved by Cantonal Commission for Ethics and Research, Geneva. Written informed consent to participate in this study was provided by the participants’ legal guardian/next of kin.
Author Contributions
AS, DR, LS, DD, MT, and VD contributed to theoretical conception, methodological and game design of the study. AS supervised the research, acquired funding and provided the resources. MT and VD developed the virtual environment. LS and MT conducted the assessment, curated the data and performed formal analysis. LS wrote the first draft of the manuscript. All authors contributed to manuscript revision, read, and approved the submitted version.
Funding
This project was supported by the Swiss National Science Foundation (SNSF; PP00P1_176,722 for AS), the Incentive Funding from the Swiss Center for Affective Sciences and the Research Fund of Unidistance Suisse.
Conflict of Interest
The authors declare that the research was conducted in the absence of any commercial or financial relationships that could be construed as a potential conflict of interest.
Publisher’s Note
All claims expressed in this article are solely those of the authors and do not necessarily represent those of their affiliated organizations, or those of the publisher, the editors and the reviewers. Any product that may be evaluated in this article, or claim that may be made by its manufacturer, is not guaranteed or endorsed by the publisher.
Supplementary Material
The Supplementary Material for this article can be found online at: https://www.frontiersin.org/articles/10.3389/frvir.2022.826241/full#supplementary-material
Footnotes
1In the pilot study on an adult sample, both a rmANOVA on the emotion reports during the three Cyberball conditions (ηp2 = 0.64 for the negative scales) and a 2 × 2 rmANOVA on the emotion reports of the two exclusion conditions with and without support during and after the game (ηp2 = 0.24 for timing and ηp2 = 0.28 for the interaction) produced extremely large effect sizes. Thus, a minimum sample size for the first analysis, with a more conservatively estimated, medium effect size (ηp2 = 0.06), two groups, a mean correlation of 0.38 between measurements taken from the pilot, a significance criterion of α = 0.05 and power = 0.80, would be N = 34. A minimum sample size for the second was again calculated with a medium effect size (ηp2 = 0.06), two groups, a number of four measurements, a correlation between measurements of 0.56 taken from the pilot, α = 0.05 and power = 0.80, resulting in N = 22. 60 participants will be ample for our research goal.
References
Bernardo, A. (2017). Virtual Reality and Simulation in Neurosurgical Training. World Neurosurg. 106, 1015–1029. doi:10.1016/j.wneu.2017.06.140
Blascovich, J., Jim, Jack., Loomis, J., Beall, A. C., Swinth, K. R., Hoyt, C. L., et al. (2002). TARGET ARTICLE: Immersive Virtual Environment Technology as a Methodological Tool for Social Psychology. Psychol. Inq. 13 (2), 103–124. doi:10.1207/S15327965PLI1302doi:10.1207/s15327965pli1302_01
Bombari, D., Schmid Mast, M., Canadas, E., and Bachmann, M. (2015). Studying Social Interactions through Immersive Virtual Environment Technology: Virtues, Pitfalls, and Future Challenges. Front. Psychol. 6 (June), 1–11. doi:10.3389/fpsyg.2015.00869
Braithwaite, J. J., Watson, D. G., Jones, R., and Rowe, M. (2013). A Guide for Analysing Electrodermal Activity (EDA) & Skin Conductance Responses (SCRs) for Psychological Experiments. Psychophysiology 49 (1), 1017–1034.
Burke, S. L., Bresnahan, T., Li, T., Epnere, K., Albert, R., Mary, P., et al. (2018). Using Virtual Interactive Training Agents (ViTA) with Adults with Autism and Other Developmental Disabilities. J. Autism Dev. Disord. 48 (3), 905–912. doi:10.1007/s10803-017-3374-z
Cai, R. Y., Richdale, A. L., Dissanayake, C., Trollor, J., and Uljarević, M. (2018). Emotion Regulation in Autism: Reappraisal and Suppression Interactions. Autism 23 (3), 737–749. doi:10.1177/1362361318774558
Chan, S., and Rawana, J. S. (2021). “Examining the Associations between Interpersonal Emotion Regulation and Psychosocial Adjustment in Emerging Adulthood.”. Cogn. Ther. Res. 45 (4), 652–662. doi:10.1007/s10608-020-10185-2
Chevallier, C., Kohls, G., Troiani, V., Brodkin, E. S., and Schultz, R. T. (2013). The Social Motivation Theory of Autism. Trends Cogn. Sci. 16 (4), 231–239. doi:10.1016/j.tics.2012.02.007
Coats, A. H., Blanchard-Fields, F., and Blanchard-Fields, F. (2008). Emotion Regulation in Interpersonal Problems: The Role of Cognitive-Emotional Complexity, Emotion Regulation Goals, and Expressivity. Psychol. Aging 23 (1), 39–51. doi:10.1037/0882-7974.23.1.39
Cohen, S. (2004). Social Relationships and Health. Am. Psychol. 59 (8), 676–684. doi:10.1037/0003-066x.59.8.676
Faul, F., Erdfelder, E., Lang, A.-G., and Buchner, A. (2007). G*Power 3: A Flexible Statistical Power Analysis Program for the Social, Behavioral, and Biomedical Sciences. Behav. Res. Methods 39 (2), 175–191. doi:10.3758/BF03193146
Gross, J. J. (1998). The Emerging Field of Emotion Regulation: An Integrative Review. Rev. Gen. Psychol. 2 (3), 271–299. doi:10.1037/1089-2680.2.3.271
Gross, J. J. (2002). Emotion Regulation. Affective, Cogn. Soc. Consequences. Psychophysiology. The Regulation of Emotion. 39, 281–291. doi:10.1017/S0048577201393198
Gulsrud, A. C., Jahromi, L. B., and Kasari, C. (2010). The Co-regulation of Emotions between Mothers and Their Children with Autism. J. Autism Dev. Disord. 40, 227–237. doi:10.1007/s10803-009-0861-x
Hallam, G. P., Webb, T. L., Sheeran, P., Miles, E., Niven, K., Wilkinson, I. D., et al. (2014). The Neural Correlates of Regulating Another Person’s Emotions: An Exploratory FMRI Study. Front. Hum. Neurosci. 8 (JUNE), 1–12. doi:10.3389/fnhum.2014.00376
Hofmann, S. G., CarpenterCarpenter, J. K., and Curtiss, J. (2016). Interpersonal Emotion Regulation Questionnaire (IERQ): Scale Development and Psychometric Characteristics. Cogn. Ther. Res. 40 (3), 341–356. doi:10.1007/s10608-016-9756-2
Howard, M. C. (2017). A Meta-Analysis and Systematic Literature Review of Virtual Reality Rehabilitation Programs. Comput. Hum. Behav. 70, 317–327. doi:10.1016/j.chb.2017.01.013
Iffland, B., Sansen, L. M., Catani, C., and Neuner, F. (2014). Rapid Heartbeat, but Dry Palms: Reactions of Heart Rate and Skin Conductance Levels to Social Rejection. Front. Psychol. 5 (AUG), 1–10. doi:10.3389/fpsyg.2014.00956
JBGarraza, (2021a). Toon Kids. Unity Asset Store. Available at: https://assetstore.unity.com/packages/3d/characters/humanoids/toon-kids-55945.
JBGarraza, (2021b). Toon People. Unity Asset Store. Available at: https://assetstore.unity.com/packages/3d/characters/humanoids/toon-people-111042.
Krämer, N. C., Karacora, B., Lucas, G., Dehghani, M., Rüther, G., and Gratch, J. (2016). Closing the Gender gap in STEM with Friendly Male Instructors? on the Effects of Rapport Behavior and Gender of a Virtual Agent in an Instructional Interaction. Comput. Edu. 99, 1–13. doi:10.1016/j.compedu.2016.04.002
Liddell, B. J., and Williams, E. N. (2019). Cultural Differences in Interpersonal Emotion Regulation. Front. Psychol. 10 (999). doi:10.3389/fpsyg.2019.00999
Lindsey, E. W. (2020). Relationship Context and Emotion Regulation across the Life Span. Emotion 20 (1), 59–62. doi:10.1037/emo0000666
Lougheed, J. P., Koval, P., and Hollenstein, T. (2016). Sharing the burden: The Interpersonal Regulation of Emotional Arousal in Mother−daughter Dyads. Emotion 16 (1), 83–93. doi:10.1037/emo0000105
Lykken, D. T., Rose, R., Luther, B., and Maley, M. (1966). Correcting Psychophysiological Measures for Individual Differences in Range. Psychol. Bull. 66 (6), 481–484. doi:10.1037/h0023922
Marroquín, B. (2011). Interpersonal Emotion Regulation as a Mechanism of Social Support in Depression. Clin. Psychol. Rev. 31 (8), 1276–1290. doi:10.1016/j.cpr.2011.09.005
Martin, R. E., and Ochsner, K. N. (2016). The Neuroscience of Emotion Regulation Development: Implications for Education. Curr. Opin. Behav. Sci. 10, 142–148. doi:10.1016/j.cobeha.2016.06.006
Mazefsky, C. A., and White, S. W. (2014). Emotion Regulation: Concepts & Practice in Autism Spectrum Disorder. Child Adolesc. Psychiatr. Clin. North America North America 23 (1), 1–12. doi:10.1016/j.chc.2013.07.002.Emotion
McDonnell, R., Breidty, M., and Bülthoff, H. H. (2012). Render Me Real? Investigating the Effect of Render Style on the Perception of Animated Virtual Humans. ACM Trans. Graphics 31 (4). doi:10.1145/2185520.2185587
Meuleman, E. J. H., and Rudrauf, David. (2018). Oud Worden. IEEE Trans. Affective Comput. 12 (1), 189–195. doi:10.1109/TAFFC.2018.286473010.1007/978-90-368-2064-6_13
Mulej Bratec, S., Bertram, T., Starke, G., Brandl, F., Xie, X., Sorg, C., et al. (2020). Your Presence Soothes Me: a Neural Process Model of Aversive Emotion Regulation via Social Buffering. Soc. Cogn. Affective Neurosci. 15 (5), 561–570. doi:10.1093/scan/nsaa068
Niven, K., Totterdell, P., and Holman, D. (2009). A Classification of Controlled Interpersonal Affect Regulation Strategies. Emotion 9 (4), 498–509. doi:10.1037/a0015962
Nozaki, Y., and Mikolajczak, M. (2020). Extrinsic Emotion Regulation. Emotion 20 (1), 10–15. doi:10.1037/emo0000636
Nussinovitch, U., Elishkevitz, K. P., Katz, K., Nussinovitch, M., Segev, S., Volovitz, B., et al. (2011). Reliability of Ultra-short ECG Indices for Heart Rate Variability. Ann. Noninvasive Electrocardiol. 16 (2), 117–122. doi:10.1111/j.1542-474X.2011.00417.x
Parsons, S., and Cobb, S. (2011). State-of-the-art of Virtual Reality Technologies for Children on the Autism Spectrum. Eur. J. Spec. Needs Edu. 26 (3), 355–366. doi:10.1080/08856257.2011.593831
Reeck, C., Ames, D. R., and Ochsner, K. N. (2016). The Social Regulation of Emotion: An Integrative, Cross-Disciplinary Model. Trends Cogn. Sci. 20 (1), 47–63. doi:10.1016/j.tics.2015.09.003
Ring, L., Utami, D., and Bickmore, T. 2014. The Right Agent for the Job? 374–384. doi:10.1007/978-3-319-09767-1_49
Samson, A. C., Hardan, A. Y., Podell, R. W., Phillips, J. M., and Gross, J. J. (2015a). Emotion Regulation in Children and Adolescents with Autism Spectrum Disorder. Autism Res. 8 (1), 9–18. doi:10.1002/aur.1387
Samson, A. C., Huber, O., and Gross, J. J. (2012). Emotion Regulation in Asperger's Syndrome and High-Functioning Autism. Emotion 12 (4), 659–665. doi:10.1037/a0027975
Samson, A. C., Wells, W. M., Phillips, J. M., Hardan, A. Y., and Gross, J. J. (2015b). Emotion Regulation in Autism Spectrum Disorder: Evidence from Parent Interviews and Children's Daily Diaries. J. Child. Psychol. Psychiatr. 56 (8), 903–913. doi:10.1111/jcpp.12370
Shang, X., Kallmann, M., and Arif, A. S. (2019). “Effects of Virtual Agent Gender on User Performance and Preference in a VR Training Program,” in Advances in Information and Communication. FICC 2019. Lecture Notes in Networks and Systems. Editors K. Arai, and R. Batia. 69th ed (Cham: Springer), 482–495. doi:10.1007/978-3-030-12388-8_34
Sheppes, G., Scheibe, S., Suri, G., and Gross, J. J. (2011). Emotion-Regulation Choice. Psychol. Sci. 22 (11), 1391–1396. doi:10.1177/0956797611418350
Silkenbeumer, J., Schiller, E. M., Holodynski, M., and Kärtner, J. (2016). The Role of Co-regulation for the Development of Social-Emotional Competence. J. Self-Regulation Regul. 02 (1), 16–33. doi:10.11588/josar.2016.2.34351
Stallmann, L., Michel, T., Rudrauf, D., Dukes, D., and Samson, A. C. (2021). Simulating Social Emotion Regulation in Virtual Reality: Effect of Virtual Social Support Following Ostracism in a Cyberball Game. Manuscript submitted for publication.
Treichel, N., Dukes, D., Barisnikov, K., and Samson, A. C. (2021). How Cognitive, Social, and Emotional Profiles Impact Humor Appreciation: Sense of Humor in Autism Spectrum Disorder and Williams Syndrome. HUMOR 35 (1), 113–133. doi:10.1515/humor-2021-0038
Virtanen, P., GommersOliphant, R., Oliphant, T. E., Haberland, M., Reddy, T., Cournapeau, D., et al. (2020). SciPy 1.0: Fundamental Algorithms for Scientific Computing in Python. Nat. Methods 17 (3), 261–272. doi:10.1038/s41592-019-0686-2
Volkaert, B., Wante, L., Van Beveren, M.-L., Vervoort, L., and Braet, C. (2020). Training Adaptive Emotion Regulation Skills in Early Adolescents: The Effects of Distraction, Acceptance, Cognitive Reappraisal, and Problem Solving. Cogn. Ther. Res. 44 (3), 678–696. doi:10.1007/s10608-019-10073-4
Williams, K. D., and Jarvis, B. (2006). Cyberball: A Program for Use in Research on Interpersonal Ostracism and Acceptance. Behav. Res. Methods 38 (1), 174–180. doi:10.3758/BF03192765
Williams White, S., Keonig, K., Scahill, L., and Scahill, L. (2007). Social Skills Development in Children with Autism Spectrum Disorders: A Review of the Intervention Research. J. Autism Dev. Disord. 37 (10), 1858–1868. doi:10.1007/s10803-006-0320-x
Zaki, J. (2020). Integrating Empathy and Interpersonal Emotion Regulation. Annu. Rev. Psychol. 71, 517–540. doi:10.1146/annurev-psych-010419-050830
Keywords: virtual reality, cyberball, virtual agent, social support, social exclusion, social emotion regulation
Citation: Stallmann L, Dukes D, Tran M, Durand de Gevigney V, Rudrauf D and Samson AC (2022) Socially Supported by an Embodied Agent: The Development of a Virtual-Reality Paradigm to Study Social Emotion Regulation. Front. Virtual Real. 3:826241. doi: 10.3389/frvir.2022.826241
Received: 30 November 2021; Accepted: 15 February 2022;
Published: 10 March 2022.
Edited by:
Ali Oker, Université de Reims Champagne-Ardenne, FranceReviewed by:
Thomas Schubert, University of Oslo, NorwayShuo Zhou, University of Colorado Denver, United States
Copyright © 2022 Stallmann, Dukes, Tran, Durand de Gevigney, Rudrauf and Samson. This is an open-access article distributed under the terms of the Creative Commons Attribution License (CC BY). The use, distribution or reproduction in other forums is permitted, provided the original author(s) and the copyright owner(s) are credited and that the original publication in this journal is cited, in accordance with accepted academic practice. No use, distribution or reproduction is permitted which does not comply with these terms.
*Correspondence: Andrea C. Samson, YW5kcmVhLnNhbXNvbkB1bmlmci5jaA==
†ORCID: Lina Stallmann, orcid.org/0000-0003-1549-9433; Daniel Dukes, orcid.org/0000-0001-8360-849X; Valentin Durand de Gevigney, orcid.org/0000-0002-8714-9355; David Rudrauf, orcid.org/0000-0002-9621-1800; Andrea C. Samson, orcid.org/0000-0001-6807-3132