- INTEC (Universidad Nacional del Litoral–CONICET), Predio CCT CONICET-Santa Fe, Santa Fe, Argentina
A broad spectrum of application fields, including human and animal health, food safety and environmental monitoring, require performing real time, on-field chemical determinations, a concept known as point-of-need testing (PONT). In the case of infectious diseases, it allows for early diagnosis, which usually enables better treatment options for the patient, and permits early outbreak detection and intervention with proper control measures. PONT solutions are usually developed on microfluidic chips, preferably with no extra equipment, as to lower their cost, ease their delivery and portability. However, equipment-free PONT is not always feasible, since off-chip operations (e.g., pumping or heating) might be required and provided by the so-called analyzers. A typical example is the hardware for optical reading, which is the subject of this perspective article. Firstly, the state-of-the-art is briefly analyzed considering the solutions provided by both academy and industry. Special emphasis is made on the smartphone-based approaches that use no additional hardware (add-ons) for optical reading. Smartphones are the straightforward option, since they can replace a number of operations typically done by analyzers. The issues arising from using smartphones without add-ons are classified into variability sources and technical challenges. Finally, a set of methods for dealing with these challenges is proposed and briefly discussed. Simpler systems will be more easily adopted by more users, for a broader range of application cases, and will let PONT be a more powerful tool for improving disease diagnosis and outbreak management.
1 Introduction
A broad spectrum of application fields, including human and animal health, food safety and environmental monitoring, require performing real time, on-field chemical determinations (Yetisen et al., 2013; Ozer et al., 2020; Amin et al., 2023). This detection format is known as point-of-need (PONT) or point-of-care testing (POCT). In the case of infectious diseases, POCT allows for early diagnosis, which usually enables better treatment options and permits early outbreak detection and intervention with proper control measures. Examples are Chagas Disease, where early treatments have a high rate of success and low side effects (WHO, 2023a); as well as Dengue and Zika virus infections, where early detection allows confinement of the infected to avoid propagation (WHO, 2023b; WHO, 2022). The need of PONT became evident during the SARS-CoV-2 pandemic, where early in-house detection would have allowed effective isolation of the infected individuals, without massively affecting economy. PONT solutions usually rely on microfluidic chips (frequently referred to as “cassettes”) that are consumable and usually accompanied by additional hardware, here called “analyzer.” The last is a permanent piece of equipment providing the required off-chip operations, such as pumping, heating, reading (optical, electrochemical), user interface and connectivity (see, for example, QIAGEN, 2023).
The WHO gathered the characteristics of ideal PONT solutions in the REASSURED criteria, which is an acronym for: affordable, sensitive, specific, user-friendly, rapid and robust, equipment-free, deliverable to end users, real-time connectivity and easy specimen collection (Land et al., 2019). Cassette-analyzer systems successfully address some of these aspects, such as sensitivity, specificity, user-friendliness, velocity, robustness, and connectivity. However, the extra hardware is frequently detrimental for the remaining criteria. Thus, reducing such hardware is highly desirable, being the limit case a standalone cassette without analyzer. However, this is not always possible because of operations like incubation that require of an external heat source (Dos-Reis-Delgado et al., 2023). On the other hand, optical reading methods, such as turbidimetry or colorimetry (Zhao et al., 2020), are attractive because they require no specific hardware, like potentiostats (Ainla et al., 2018) or FET biosensor-based systems (Moser et al., 2016). These optical methods are compatible with immunoassays (Gosling, 1990) and molecular reactions for the detection of nucleic acids (Botella, 2022), which are the most used assays for diagnostics. Also, these optic methods can be enhanced by smartphones that, as they are widely available (Statista, 2023), do not constitute an extra cost nor an accessibility barrier to the users.
In what follows, the state-of-the-art of the optical reading approaches for PONT is analyzed with emphasis on smartphone-based options. Then, the challenges of using smartphones without add-ons are assessed. Finally, some methods for robust smartphone-based optical reading are proposed.
2 Present optical reading approaches for PONT systems
2.1 Naked eye reading
The paradigmatic example of naked eye reading are Lateral Flow Assays (LFA), depicted in Figure 1A, which are a well-established technology for rapid and easy detection of a large variety of biomarkers (Wong and Tse, 2009). LFA technology became popular in the 80s, when pregnancy tests enabled “yes/no” answer in the privacy of user’s home. Recently, during the COVID-19 pandemic, LFAs allowed large-scale serological testing, demonstrating the effectiveness of LFAs for clinical and public health purposes (Ozer and Henry, 2021; Budd et al., 2023). PONT systems entailing the simplicity of LFAs are highly desirable. Actually, naked eye detection has been historically used in biochemical laboratories for urinalysis and hemagglutination. Nowadays, the emerging technologies for blood group testing offer more sophisticated platforms for quicker and more accurate assays (Pipatpanukul et al., 2018), as well as multifunctional microfluidic devices (Lin et al., 2020); nevertheless, the reading continues to be the direct visual inspection. Turbidimetry has also notably evolved to increase the sensibility of naked eye detection (Shin et al., 2018; Spoelstra et al., 2021). Naturally, naked eye colorimetry methods are considered less adequate than instrument-based techniques for detecting small amounts of analytes or small signal variations. Nevertheless, up-to-date ELISA can perform sensitive detection of disease biomarkers by naked eye reading (De La Rica and Stevens, 2012; Wang et al., 2022). Drawbacks of naked eye reading are that it is limited to qualitative results and subject to non-uniform interpretation of signals, which strongly depends on the user and ambient conditions. For example, it was shown that non trained users are prone to miss weak positives (Schaumburg et al., 2022). Thus, even when naked eye reading is possible, the current trend is analyzing results with a smartphone (Figure 1D).
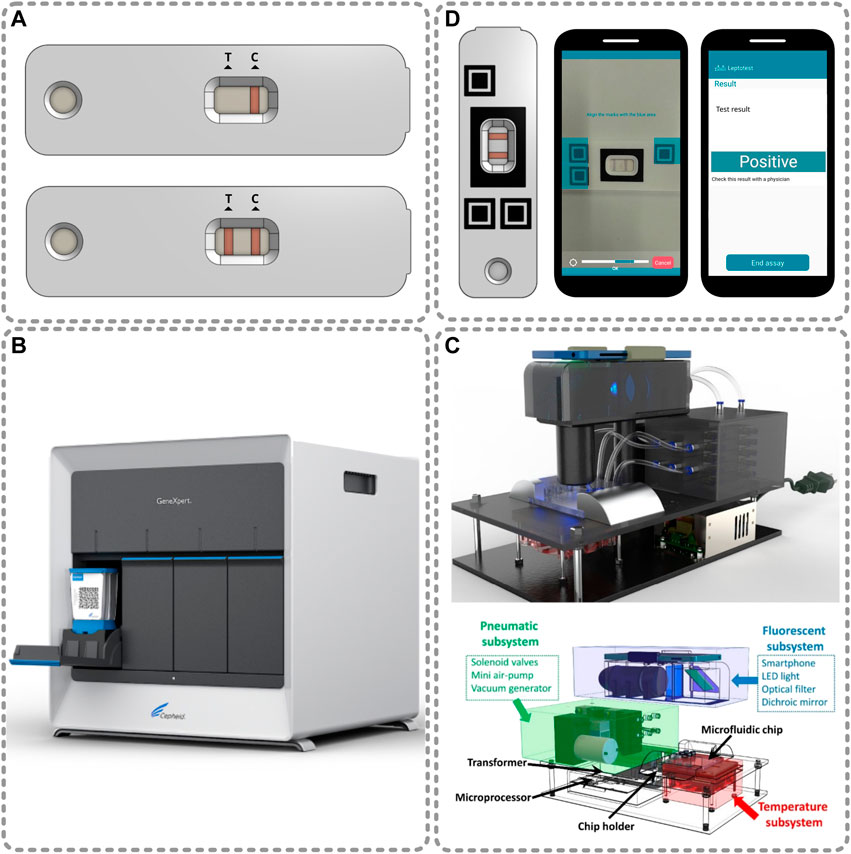
FIGURE 1. Examples and classification of the present optical reading approaches for PONT. (A) Naked eye reading: LFA can be qualitatively read by visual inspection. (B) Cassette-Analyzer systems: A typical example is the Cepheid GeneXpert. Used with permission from Cepheid (2023). (C) Smartphones with add-ons, modified from Zhu et al. (2020) and provided under a CC BY 4.0 license. (D) Smartphones without add-ons, modified from Schaumburg et al. (2022) and provided under a CC BY 4.0 license.
2.2 Cassette-analyzer system
A technical approach that solves the problems of naked eye reading consists in designing PONT systems with the format of cassette-analyzer (Figure 1B), a strategy that has found large consensus in the market. The cassette is fungible, incorporates the reagents, and has the microfluidic architecture to collect the specimen and perform the detection reactions; see, for example, Chen et al. (2010). The analyzer is a small, benchtop, frequently portable but usually not a handheld instrument, which interacts with the cassette to provide energy, control the reaction protocol, and capture the specific signal that is accurately translated into a result; see, for example, Hu et al. (2017). Analyzers can provide hardware solutions for fluid handling, thermal incubation and optical reading, such as mirrors, filters and/or specific UV-vis radiation sources for fluorescence or chemiluminescence (Chen et al., 2020). In general, cassette-analyzer systems are not thought to be used at home nor delivered to final users; however, they still fit the definition of PONT. There is a long list of commercially available cassette-analyzer systems. A couple of examples are the GeneXpert™ (Cepheid, California, United States), which is perhaps the most popular PCR-based PONT system (Figure 1B) and the ID NOW™ (Abbot, Illinois, United States), which performs isothermal amplification for the detection of infectious diseases. Although the cassette-analyzer format provides accurate results at decentralized points in a short time, the permanent equipment (and usually the fungible cassette) results too expensive for low-income countries and happens to be detrimental to deliverability to the end-user.
2.3 Smartphone-based reading with add-ons
Besides being widely available, smartphones can provide complementary features like computing power, data storage, power sourcing, sensors (e.g., accelerometers, ambient light sensors, Hall effect sensors, GPS) (Schaumburg et al., 2020). Additionally, a custom-made app can be used to improve the user experience, to provide guiding throughout the test and specific controls, and for objectively communicating the outcome of an assay (Schaumburg et al., 2022). Even more, smartphones provide connectivity for database communication and exploitation through data analytics, connecting PONT solutions to the Internet of Things (Florea and Diamond, 2022).
Regarding optical reading, most of current research relies on the photograph camera of smartphones, although there are other options available (Xiao et al., 2018). Because smartphone’s cameras are subject to many variability sources, these approaches are usually aided by additional hardware meant to guarantee repeatability of results. Thus, a black-box or a “cradle” is provided for ambient light cancellation and for fixation of the photograph geometry (i.e., the position, distances and angles between the phone and the cassette). Such a box might also include light sources, optic filters and mirrors, batteries and control electronics, among other components (Figure 1C). These approaches often employ other smartphone attributes, such us the flashlight, or computing power (Paterson et al., 2017). The hardware may also include modules to account for fluid handling or heating (Zhu et al., 2020). This approach offers similar pros and cons to the cassette-analyzer systems. The main advantage is that the overall cost decreases due to the partial hardware replacement. On the other hand, cross-contamination and hygiene issues arise with the interaction between the personal smartphone and the hardware, as well as the model compatibility problems.
2.4 Smartphone-based reading without add-ons
The fourth group of PONT systems is formed by cassettes, the optical reading of which is performed using the smartphone solely (Figure 1D). These systems may not only use the camera, but also other attributes of smartphones, such the flashlight, data processing, computing power, result reporting and connectivity, among others. This approach replaces all the hardware required for reading purposes (Schaumburg et al., 2023) and, in some special cases, all the analyzing system is the smartphone (Schaumburg et al., 2022). Advantages over the previous group (Smartphones with add-ons) are less cross-contamination, hygiene and model compatibility issues, since no contact is required between the smartphone and the cassette. Even more, this approach offers more portable, economic, deliverable and user friendly solutions than the others. The negative consequence of the hardware simplification is that the obtained images are subject to many variability sources, as discussed next.
3 Perspectives for smartphone-based reading without add-ons
3.1 Challenges for smartphone-based reading without add-ons
Success of smartphones as optical reading systems for PONT requires that the following challenges are addressed correctly.
Geometric variability, i.e., the relative position between the plane of the camera CMOS sensor and the plane of the cassette, needs to be accounted for. This variability can be described in terms of the distance, rotation, and angle between these planes. In the case of smartphones without add-ons, it is specially important because there is no hardware to fix the relative positions (Karlsen and Dong, 2017).
Inter-device variability occurs because different smartphone brands and models possess different CMOS sensors that produce different digital images for a given input. In fact, noise introduces a degree of variability between different pictures from the same sensor (ONSEMI, 2023). Phone-to-phone variability is also increased by brand/model specific processing algorithms (e.g., white balance) that are automatically applied to the raw images (Zhang and Batur, 2012). Also, the capture parameters or camera settings modify the digital image obtained by the CMOS sensor. The main parameters are the sensitivity (or ISO parameter), shutter speed or exposure time, aperture and resolution of the camera (Mancini and Sidoriak, 2017).
Ambient light also introduces an important challenge, since it constitutes an uncontrolled excitation source for the optical reading, which might be the only source if the flashlight is not used. Ambient light intensity varies within a wide range, starting from from 50 lx in artificially lit aisles, to 100,000 lx outdoors in a bright summer day. Light color temperature also influences digital images: it can take values from ∼2,500 K (which corresponds to a shade of orange) for incandescent bulbs, to ∼6,000 K (i.e., white) for daylight. Even more, ambient light cannot guarantee uniform spatial distribution. Uneven light distribution might happen because of shadows or uneven sources. Shadows are caused by any object interposed between the light source and the cassette. Given the short distances often involved, this object is usually the smartphone itself. The concept of uneven sources refers to the case where multiple light sources are spatially distributed in the room used for imaging, and none of them is strongly dominant (Karlsen and Dong, 2017). Another often regarded effect is the inter-operator variability. However, it might be considered as a combination of the factors named above.
A different set of challenges for smartphone-based reading without add-ons is not related to variability sources, but to technical aspects. Perhaps, the most relevant issue is the quantification of analytes, required in assays where a “yes/no” result is not enough. Another technical challenge is related to assays where a single read-out at the end of the assay is not sufficient, but a real-time monitoring involving multiple readings is required. This is necessary, e.g., in assays based on qPCR or qLAMP (Gong et al., 2021).
3.2 Addressing challenges: proposed solutions
Potential solutions for most of the aforementioned challenges have already been proposed, some of them with success and others still under study. These solutions can be classified into pre and post-processing measures. The former refers to actions taken before shooting, tending to achieve standardization of the image and assuring the quality of the information required next. The last refers to software processing, for image and data manipulation. Although here we focus on the first group, please note that both solution types are complementary. In what follows, we do not present a complete list of the available options, but a subset of the solutions deemed best for the purpose. For example, geometric variability has been successfully addressed with on-chip alignment marks (Figure 2). These marks: 1) provide an easy-to-find reference, 2) can help guide the operator using complementary marks in the smartphone screen and 3) allow capture only if the relative positions are within an acceptable range (Schaumburg et al., 2022; Schaumburg et al., 2023). Additionally, marks can be used for perspective correction through software processing (Karlsen and Dong, 2017).
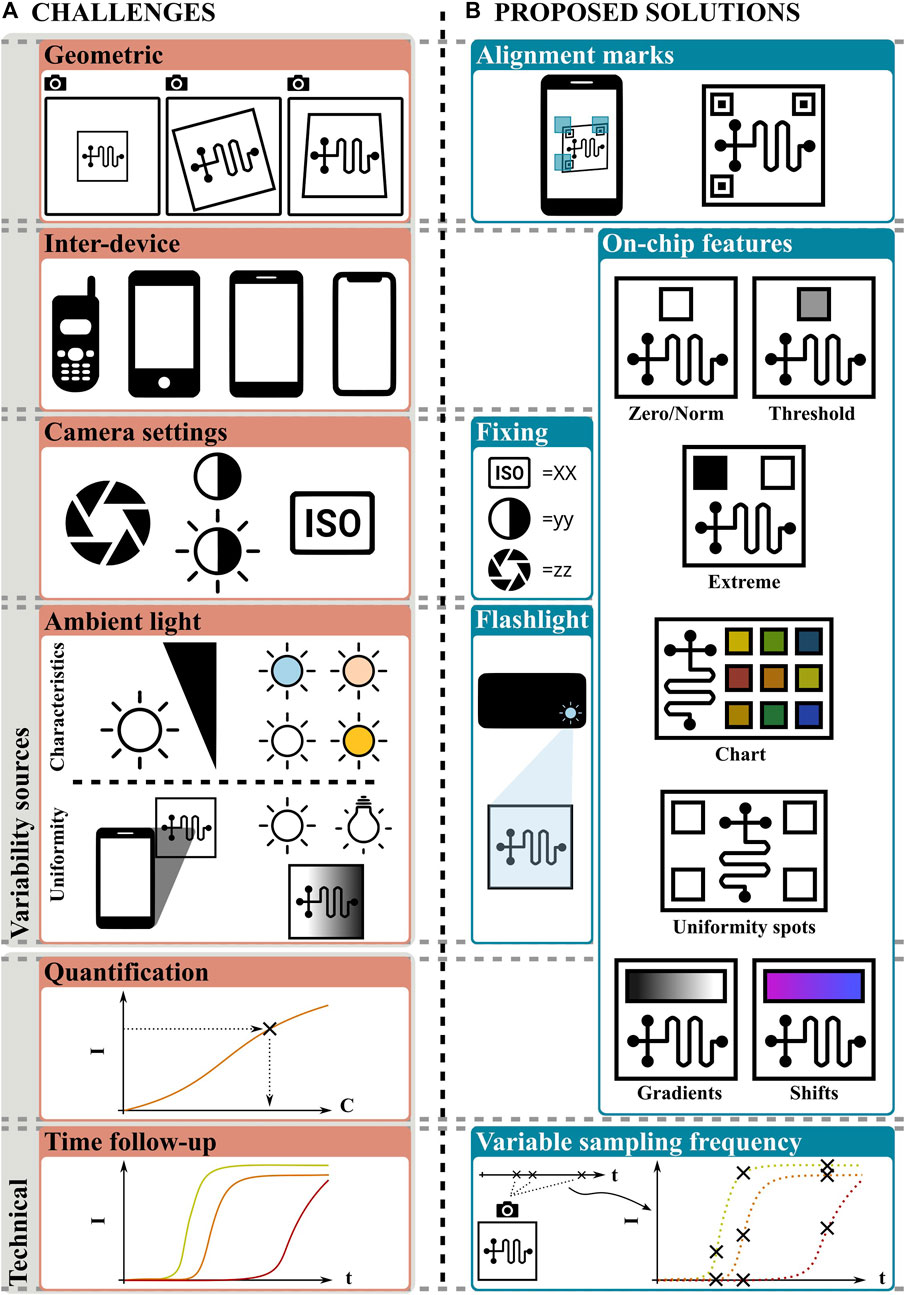
FIGURE 2. (A) Current challenges and (B) proposed solutions for reading methods based on smartphones without add-ons. Explanations are given in the text.
Capture parameters can be fixed to decrease the uncertainty produced by the camera settings (Cheng et al., 2017); nevertheless this solution is not suitable for other variability sources. Also, the smartphone flashlight has been used to standardize the lighting conditions (Kong et al., 2019). This solution is attractive because serves to achieve intensity, color and space uniformity, although it is limited to situations where the flashlight is the dominant source (ambient light intensity less than ∼1,000 lx) and entails a new problem: in mate surfaces, such as paper-based cassettes, the angle of incidence of light affects the perceived intensity and color (Karlsen and Dong, 2017); in reflective surfaces, usually found on cassettes made out of glass, polymers or in free liquids, it can produce glow, usually detrimental to image processing.
On-chip calibration features (Figure 2) are perhaps the most interesting approach. These features provide color/intensity references for the unknown signal, as they are both known and subject to the same variability sources (the features are in the same image than the unknown). Because the color/intensity variability can simultaneously be related to the brand/model, capture settings, ambient light conditions and/or the analyte concentration, proper on-chip calibration features can be used to address all of these challenges. Such features can be printed, as in pH strips, or be part of a reaction, as the test line of LFA. Some of these calibration features will be briefly described next. First, the color/intensity of a feature can be used for normalization of the unknown as in absorbance measurements (see, for example, Sun et al., 2020). Similarly, it can be used as the zero value to perform a zero-correction. This concept can be extended by including both expected extreme values (Escobedo et al., 2023) or a color chart with multiple values (Balbach et al., 2021) as illustrated in Figure 2. For yes/no results, a feature with the threshold value separating the positive and negative values can be included for direct comparison. Also, on-chip features can be used to assess non-uniform illumination: a large uniform area or multiple identical spots can be placed near the unknown, then a metric (e.g., standard deviation) can be used as a measure of uniformity (Schaumburg et al., 2023).
For quantitative and semi-quantitative analysis, the features presented above must be complemented with a calibration curve, and/or a mathematical law for interpolation. The inherent errors of the method can be enlarged if space uniformity is not guaranteed. Interestingly, an alternative way was recently presented (Schaumburg et al., 2022), where the full expected color span was provided on-chip in the form of a gradient. Thus, continuous determinations can be achieved by searching the matching value of the unknown in the gradient, in which the position represents, for example, a concentration. This concept can be easily adapted to any color-shift. Regarding time-dependent results, perhaps the most promising approach is based on sampling at variable frequencies, where the user must take a number of pictures during an established period (Figure 2, right-bottom corner). Although this task might be helped by timers and alarms from an app, the sampling frequency cannot be expected to be constant, as in laboratory equipment. This approach requires a well-defined mathematical model fed by the data obtained from images. Note that video-based approaches are an option for sampling at a constant rate, although they demand resources proportionally to the length of the recording and anchor the smartphone during the procedure, which can take, e.g., 30 min or more for a LAMP procedure.
4 Discussion
In this article we promote that using smartphones with minimal (preferably none) additional hardware is the most attractive approach for PONT. Extra hardware increases costs and hinders deliverability, constituting a barrier between the user/patient and the test. Both aspects, together with the “equipment-free” criterion, are part of REASSURED diagnostics. Even more, smartphones without add-ons guarantee contact-less interaction between the phone and the testing device, reducing cross-contamination and hygiene issues. The approach is not brand/model specific and does not anchor a personal phone during the lapse of the assay that can take several tenths of minutes. Using no add-ons for reading purposes poses the set of challenges described in previous sections. Most of these issues can be dealt with using image pre and post-processing. The former involves image standardization by on-chip alignment marks and features. Regarding the last, powerful and promising algorithms are available, like computer vision and machine learning, which have already been successfully used to address complex medical tasks (Elyan et al., 2022), and have the potential to reinforce and complement the solutions here proposed. Although complex algorithms may demand too much resources for a smartphone, the problem can be circumvented by using a remote server for heavy processing and using the smartphone just for imaging, connectivity, and reporting.
This article was focused on avoiding extra optical hardware, nevertheless, it should be noted that add-ons might still be needed for heating or fluid handling, according to the chemical reactions involved. In this regard, it is desirable the implementation of sensing mechanisms with minimum steps, ideally one-pot reactions without heating. Examples are RPA amplifications (Crannell et al., 2014) and some immunoassays (Budd et al., 2023). Simpler systems will be more easily adopted by more users, on a broader range of application cases, and will let PONT be a more powerful tool for improving detection, treatment and outbreak management for One Health.
Data availability statement
The original contributions presented in the study are included in the article/supplementary material, further inquiries can be directed to the corresponding authors.
Author contributions
FS: Conceptualization, Investigation, Methodology, Visualization, Writing–original draft. CB: Conceptualization, Investigation, Supervision, Writing–review and editing.
Funding
The authors declare financial support was received for the research, authorship, and/or publication of this article. Founding was provided by the Consejo Nacional de Investigaciones Científicas y Técnicas (CONICET), through the Grant PIP 2021–2023 GI 11220200101392CO.
Acknowledgments
The authors acknowledge the Consejo Nacional de Investigaciones Científicas y Técnicas (CONICET).
Conflict of interest
The authors declare that the research was conducted in the absence of any commercial or financial relationships that could be construed as a potential conflict of interest.
Publisher’s note
All claims expressed in this article are solely those of the authors and do not necessarily represent those of their affiliated organizations, or those of the publisher, the editors and the reviewers. Any product that may be evaluated in this article, or claim that may be made by its manufacturer, is not guaranteed or endorsed by the publisher.
References
Ainla, A., Mousavi, M. P., Tsaloglou, M.-N., Redston, J., Bell, J. G., Fernandez-Abedul, M. T., et al. (2018). Open-source potentiostat for wireless electrochemical detection with smartphones. Anal. Chem. 90, 6240–6246. doi:10.1021/acs.analchem.8b00850
Amin, N., Almasi, A., Ozer, T., Henry, C. S., Hosseinzadeh, L., and Keshavarzi, Z. (2023). Recent advances of optical biosensors in veterinary medicine: moving towards the point of care applications. Curr. Top. Med. Chem. 23, 2242–2265. doi:10.2174/1568026623666230718163613
Balbach, S., Jiang, N., Moreddu, R., Dong, X., Kurz, W., Wang, C., et al. (2021). Smartphone-based colorimetric detection system for portable health tracking. Anal. Methods 13, 4361–4369. doi:10.1039/d1ay01209f
Botella, J. R. (2022). Point-of-care dna amplification for disease diagnosis and management. Annu. Rev. phytopathology 60, 1–20. doi:10.1146/annurev-phyto-021621-115027
Budd, J., Miller, B. S., Weckman, N. E., Cherkaoui, D., Huang, D., Decruz, A. T., et al. (2023). Lateral flow test engineering and lessons learned from covid-19. Nat. Rev. Bioeng. 1, 13–31. doi:10.1038/s44222-022-00007-3
CC BY 4.0 (2023). CC BY 4.0 DEED. Available at: https://creativecommons.org/licenses/by/4.0/.
Cepheid (2023). Cepheid A better way. Available at: https://www.cepheid.com/content/dam/www-cepheid-com/images/site-images/home/Home%20Systems%20Image.png.
Chen, D., Mauk, M., Qiu, X., Liu, C., Kim, J., Ramprasad, S., et al. (2010). An integrated, self-contained microfluidic cassette for isolation, amplification, and detection of nucleic acids. Biomed. microdevices 12, 705–719. doi:10.1007/s10544-010-9423-4
Chen, W., Shao, F., and Xianyu, Y. (2020). Microfluidics-implemented biochemical assays: from the perspective of readout. Small 16, 1903388. doi:10.1002/smll.201903388
Cheng, N., Song, Y., Zeinhom, M. M., Chang, Y.-C., Sheng, L., Li, H., et al. (2017). Nanozyme-mediated dual immunoassay integrated with smartphone for use in simultaneous detection of pathogens. ACS Appl. Mater. interfaces 9, 40671–40680. doi:10.1021/acsami.7b12734
Crannell, Z. A., Rohrman, B., and Richards-Kortum, R. (2014). Equipment-free incubation of recombinase polymerase amplification reactions using body heat. PloS one 9, e112146. doi:10.1371/journal.pone.0112146
De La Rica, R., and Stevens, M. M. (2012). Plasmonic elisa for the ultrasensitive detection of disease biomarkers with the naked eye. Nat. Nanotechnol. 7, 821–824. doi:10.1038/nnano.2012.186
Dos-Reis-Delgado, A. A., Carmona-Dominguez, A., Sosa-Avalos, G., Jimenez-Saaib, I. H., Villegas-Cantu, K. E., Gallo-Villanueva, R. C., et al. (2023). Recent advances and challenges in temperature monitoring and control in microfluidic devices. Electrophoresis 44, 268–297. doi:10.1002/elps.202200162
Elyan, E., Vuttipittayamongkol, P., Johnston, P., Martin, K., McPherson, K., Jayne, C., et al. (2022). Computer vision and machine learning for medical image analysis: recent advances, challenges, and way forward. Artif. Intell. Surg. 2. doi:10.20517/ais.2021.15
Escobedo, P., Ramos-Lorente, C. E., Ejaz, A., Erenas, M. M., Martínez-Olmos, A., Carvajal, M. A., et al. (2023). Qrsens: dual-purpose quick response code with built-in colorimetric sensors. Sensors Actuators B Chem. 376, 133001. doi:10.1016/j.snb.2022.133001
Florea, L., and Diamond, D. (2022). Sensors and “the internet of biochemical things”. Front. Sensors 3, 1010212. doi:10.3389/fsens.2022.1010212
Gong, H.-y., Li, Q.-y., Zhang, H., Ye, L., Shi, L., and Feng, Y.-h. (2021). Development and comparison of qpcr and qlamp for rapid detection of the decapod iridescent virus 1 (div1). J. Invertebr. Pathology 182, 107567. doi:10.1016/j.jip.2021.107567
Gosling, J. P. (1990). A decade of development in immunoassay methodology. Clin. Chem. 36, 1408–1427. doi:10.1093/clinchem/36.8.1408
Hu, B., Li, J., Mou, L., Liu, Y., Deng, J., Qian, W., et al. (2017). An automated and portable microfluidic chemiluminescence immunoassay for quantitative detection of biomarkers. Lab a Chip 17, 2225–2234. doi:10.1039/C7LC00249A
Karlsen, H., and Dong, T. (2017). Smartphone-based rapid screening of urinary biomarkers. IEEE Trans. Biomed. circuits Syst. 11, 455–463. doi:10.1109/TBCAS.2016.2633508
Kong, T., You, J. B., Zhang, B., Nguyen, B., Tarlan, F., Jarvi, K., et al. (2019). Accessory-free quantitative smartphone imaging of colorimetric paper-based assays. Lab a Chip 19, 1991–1999. doi:10.1039/c9lc00165d
Land, K. J., Boeras, D. I., Chen, X.-S., Ramsay, A. R., and Peeling, R. W. (2019). Reassured diagnostics to inform disease control strategies, strengthen health systems and improve patient outcomes. Nat. Microbiol. 4, 46–54. doi:10.1038/s41564-018-0295-3
Lin, J.-H., Tsai, T.-T., Zeng, Q., Chang, C.-Y., Guo, J.-Y., Lin, C.-J., et al. (2020). A multifunctional microfluidic device for blood typing and primary screening of blood diseases. ACS sensors 5, 3082–3090. doi:10.1021/acssensors.0c00969
Mancini, K., and Sidoriak, J. (2017). “Basic photography principles,” in Fundamentals of forensic photography (England, UK: Routledge), 23–38.
Moser, N., Lande, T. S., Toumazou, C., and Georgiou, P. (2016). Isfets in cmos and emergent trends in instrumentation: a review. IEEE Sensors J. 16, 6496–6514. doi:10.1109/jsen.2016.2585920
Ozer, T., and Henry, C. S. (2021). Paper-based analytical devices for virus detection: recent strategies for current and future pandemics. TrAC Trends Anal. Chem. 144, 116424. doi:10.1016/j.trac.2021.116424
Ozer, T., McMahon, C., and Henry, C. S. (2020). Advances in paper-based analytical devices. Annu. Rev. Anal. Chem. 13, 85–109. doi:10.1146/annurev-anchem-061318-114845
Paterson, A. S., Raja, B., Mandadi, V., Townsend, B., Lee, M., Buell, A., et al. (2017). A low-cost smartphone-based platform for highly sensitive point-of-care testing with persistent luminescent phosphors. Lab a Chip 17, 1051–1059. doi:10.1039/c6lc01167e
Pipatpanukul, C., Kataphiniharn, C., Wangkam, T., Sutapun, B., Kitpoka, P., Kunakorn, M., et al. (2018). Polymethyl methacrylate (pmma) point of care for abo-rh (d) blood typing. Sensors Actuators B Chem. 273, 703–709. doi:10.1016/j.snb.2018.06.074
QIAGEN (2023). Qiagen. Available at: https://www.qiagen.com/us/product-categories/diagnostics-and-clinical-research/infectious-disease/qiastat-dx-syndromic-testing.
Schaumburg, F., Kler, P. A., Carrell, C. S., Berli, C. L., and Henry, C. S. (2020). Usb powered microfluidic paper-based analytical devices. Electrophoresis 41, 562–569. doi:10.1002/elps.201900273
Schaumburg, F., Pujato, N., Peverengo, L. M., Marcipar, I. S., and Berli, C. L. A. (2023). Coupling elisa to smartphones for poct of chronic and congenital chagas disease. Talanta 256, 124246. doi:10.1016/j.talanta.2022.124246
Schaumburg, F., Vidocevich, J. P., Gerlero, G. S., Pujato, N., Macagno, J., Kler, P. A., et al. (2022). A free customizable tool for easy integration of microfluidics and smartphones. Sci. Rep. 12, 8969. doi:10.1038/s41598-022-13099-z
Shin, C., Lee, H. N., Ryu, J. S., and Chung, H. J. (2018). Rapid naked-eye detection of gram-positive bacteria by vancomycin-based nano-aggregation. RSC Adv. 8, 25094–25103. doi:10.1039/c8ra03540g
Spoelstra, W. K., Jacques, J. M., Gonzalez-Linares, R., Nobrega, F. L., Haagsma, A. C., Dogterom, M., et al. (2021). Crispr-based dna and rna detection with liquid-liquid phase separation. Biophysical J. 120, 1198–1209. doi:10.1016/j.bpj.2021.02.013
Statista (2023). Statista. Available at: https://www.statista.com/statistics/245501/multiple-mobile-device-ownership-worldwide/.
Sun, F., Ganguli, A., Nguyen, J., Brisbin, R., Shanmugam, K., Hirschberg, D. L., et al. (2020). Smartphone-based multiplex 30-minute nucleic acid test of live virus from nasal swab extract. Lab a Chip 20, 1621–1627. doi:10.1039/d0lc00304b
Wang, M., Lin, Y., Lu, J., Sun, Z., Deng, Y., Wang, L., et al. (2022). Visual naked-eye detection of sars-cov-2 rna based on covalent organic framework capsules. Chem. Eng. J. 429, 132332. doi:10.1016/j.cej.2021.132332
WHO (2022). Zika virus. Available at: https://www.who.int/news-room/fact-sheets/detail/zika-virus.
WHO (2023a). Chagas disease (American trypanosomiasis). Available at: https://www.who.int/health-topics/chagas-disease.
WHO (2023b). Dengue and severe dengue. Available at: https://www.who.int/news-room/fact-sheets/detail/dengue-and-severe-dengue.
Xiao, W., Huang, C., Xu, F., Yan, J., Bian, H., Fu, Q., et al. (2018). A simple and compact smartphone-based device for the quantitative readout of colloidal gold lateral flow immunoassay strips. Sensors Actuators B Chem. 266, 63–70. doi:10.1016/j.snb.2018.03.110
Yetisen, A. K., Akram, M. S., and Lowe, C. R. (2013). Paper-based microfluidic point-of-care diagnostic devices. Lab a Chip 13, 2210–2251. doi:10.1039/c3lc50169h
Zhang, B., and Batur, A. U. (2012). “A real-time auto white balance algorithm for mobile phone cameras,” in 2012 IEEE International Conference on Consumer Electronics (ICCE) (IEEE), 1–4, Las Vegas, NV, USA, January 6-8, 2023.
Zhao, V. X. T., Wong, T. I., Zheng, X. T., Tan, Y. N., and Zhou, X. (2020). Colorimetric biosensors for point-of-care virus detections. Mater. Sci. energy Technol. 3, 237–249. doi:10.1016/j.mset.2019.10.002
Keywords: point-of-need testing (PONT), point-of-care testing (POCT), optical reading, colorimetry, turbidimetry, smartphones, internet of things (IoT), microfluidics
Citation: Schaumburg F and Berli CLA (2023) Challenges and proposed solutions for optical reading on point-of-need testing systems. Front. Sens. 4:1327240. doi: 10.3389/fsens.2023.1327240
Received: 24 October 2023; Accepted: 22 November 2023;
Published: 15 December 2023.
Edited by:
Naresh Kumar Mani, Manipal Academy of Higher Education, IndiaReviewed by:
Tugba Ozer, Yıldız Technical University, TürkiyeCopyright © 2023 Schaumburg and Berli. This is an open-access article distributed under the terms of the Creative Commons Attribution License (CC BY). The use, distribution or reproduction in other forums is permitted, provided the original author(s) and the copyright owner(s) are credited and that the original publication in this journal is cited, in accordance with accepted academic practice. No use, distribution or reproduction is permitted which does not comply with these terms.
*Correspondence: Federico Schaumburg, ZnNjaGF1bWJ1cmdAaW50ZWMudW5sLmVkdS5hcg==; Claudio L. A. Berli, Y2JlcmxpQHNhbnRhZmUtY29uaWNldC5nb3YuYXI=