- 1Department of Tropical Plant and Soil Sciences, University of Hawai'i at Mānoa, Honolulu, HI, United States
- 2Department of Natural Resources and Environmental Management, University of Hawai'i at Mānoa, Honolulu, HI, United States
- 3School of Natural Sciences, University of California, Merced, Merced, CA, United States
Our current understanding suggests that nutrient management strategies applied to agricultural soils over multiple years are required to cause major and stable shifts in soil microbial communities. However, some studies suggest that agricultural soils can benefit even from sporadic, single additions of organic matter. Here we investigate how single additions of high-quality organic matter can cause significant shifts in microbial soil communities over multiple cropping cycles. We grew radishes in a tropical Oxisol soil for six crop cycles after a single application of a high-nitrogen compost or urea. At planting and before biomass harvest, we sampled soils influenced by the radish rhizosphere and sequenced bacterial and archaeal 16S and fungal ITS rDNA marker genes. We measured microbial richness and diversity, community composition and structure, and constructed correlation networks to predict cross-domain microbial interactions. We found that a single application of compost, compared to urea or control, resulted in a persistent improved plant biomass response and led to sustained changes in the soil microbial community throughout the duration of the 227-day study. Compost altered the structure of both the fungal and prokaryotic microbial communities, introduced new microorganisms that persisted in the resident soil system, and altered soil microbial correlation network structure and hub taxa. In contrast, fertilization with urea did not significantly alter the structure of soil microbial communities compared to the control but reduced network complexity and altered hub taxa. This study highlights the significant impacts that high-quality organic matter fertilization can exert on agricultural soil microbiomes and adds to the growing body of knowledge on using organic fertilizers as a way to steer the soil microbiome toward a healthier soil.
Introduction
Soil microbes are fundamental drivers of soil nutrient dynamics, and our ability to understand and predict microbial composition and function in agricultural soils is essential for the maintenance of healthy and sustainable soil ecosystems (1). Soil health, defined as the ability of agricultural soils to continue to provide ecosystem services while optimizing agricultural yields, consists of soil physical, chemical, and biological components (2). Some common indicators for soil health include soil physical and chemical characteristics like pH, aggregate stability, water infiltration, bulk density, soil organic matter content, and biological indicators like microbial biomass and activity, microbial diversity, and carbon and nitrogen cycling potentials (2, 3). Indicators like microbial diversity and community structure are linked in part to properties like soil pH (4) and soil organic matter (5–7) highlighting the importance in managing these soil qualities when considering the health of the soil microbiome.
Nitrogen management in agricultural systems has a strong impact on soil quality and the soil microbiome (8–11). Urea fertilizer, otherwise known as carbamide CO(NH2)2, is the most common synthetic nitrogen source applied to agricultural soils to meet crop N requirements (12). Urea is a simple compound, composed of 46% N, that hydrolyzes rapidly by soil urease enzyme into carbonic acid and ammonia (13, 14). As a result of these fast reactions, the promotion of plant growth and soil microbial community shift is most pronounced immediately after application (15). The impact of urea on the soil microbiome, both direct and indirect, however, is less predictable. Some studies showed that repeated application of urea may decrease soil health, in part due to an increase in ureolytic prokaryotes whose presence decreases plant nitrogen use efficiency by promoting N losses through gaseous forms (i.e., ammonia volatilization following urea hydrolysis, denitrification following ammonia oxidation), as well as a general decrease in microbial diversity that provide other ecosystem services (16, 17). However, other studies suggest that the application of urea fertilizers can increase plant growth and the resulting abundance of root exudates can in-turn stimulate microbial activity and increase nitrogen cycling (9, 16).
In contrast, complex nutrient sources such as composts are also known to boost agricultural productivity directly by increasing soil plant-available nitrogen (18–20). However, compost also has indirect benefits to agricultural productivity primarily associated with its more complex organic compounds, including enhanced nitrogen use efficiency via the re-coupling of carbon and nitrogen cycles (21), increased microbial abundance and activity (18, 22), improved nutrient cycling and disease suppression (23, 24), better nutrient retention (25), and greater overall soil quality (26). Therefore, maintaining soil organic matter via fertilization is a means to both increase soil fertility and diversify the soil microbiome that can help to maintain that fertility. However, trends in how the soil microbiome shifts in response to compost fertilization can vary depending on soil properties and type of compost (27, 28). However, it is unclear how the interconnected communities of dominant soil microorganisms (such as fungi and bacteria) respond to different quality of fertilizers.
Among the statistical tools available that allow us to connect the complex interactions in the soil environment, network analyses offer a promising and unique opportunity to predict interactions among microbial individuals. Using co-occurrence patterns, researchers have studied how microbial networks differ between ecosystems or habitat niches (29, 30), evaluated network dynamics in response to fertilization (31) or drought (32), and gained insight into organisms associated with specific ecosystem functions (33). “Hub” species, that is, taxa that are highly connected in an ecosystem network by centrality measurements like degree and closeness centrality (34) may provide insights into complex microbiomes such as those in soils. Most previous soil microbial network studies have used single gene amplicon analyses to gain important insight on prokaryotic and eukaryotic network interactions. Current inference techniques exist that allow for analyses among multiple marker genes, giving insight into associations across biological domains and elucidate, for instance, the importance of fungi in stabilizing bacterial network connections in the human lung microbiome (35), or across soil profiles (36) and rhizospheres after wetups (37). Cross-domain network analyses applied to the soil microbiome offer unique opportunities to hypothesize interactions among communities of fungi, bacteria, and archaea, and how these interactions may ultimately lead to biological insights relevant to soil health such as the movement and cycling of nutrients in soil environment.
Despite the active research in this area, making the connection between nitrogen fertilizer sources to changes in the microbiome is still not trivial. This study aims to fill knowledge gaps associated with how soil microbial communities respond to simple and complex organic nitrogen fertilizers. We employed high-throughput sequencing techniques as well as recently developed cross-domain co-occurrence networks to measure community responses to a single fertilization event of urea and a high N compost across six planting cycles in a nutrient-poor tropical Oxisol soil. We hypothesize that complex nitrogen fertilizers such as compost, when applied to soils, have a detectable impact on plant growth, soil microbial diversity, community structure, network topography, and change network hub taxa as compared to urea, a simple, synthetic nitrogen fertilizer.
Materials and Methods
Experimental Design
Plants were grown in a repeated measures design in a climate-controlled greenhouse with mean daytime temperature of 28°C and night time of 22°C at the University of Hawai'i at Mānoa. The Lahaina series (Very-fine, kaolinitic, isohyperthermic Rhodic Eutrustox, pH 7.9) was used as the growth medium, chosen for its naturally low fertility but agricultural importance in Hawai'i. This Oxisol is comparable to other Oxisols in tropical regions of the world (38), although the alkalinity, prior use for sugarcane production, and anecdotal evidence suggests it had been previously limed. The soil was collected from the 0 to 30 cm depth from an uncultivated field directly adjacent to an organically managed agricultural system in Waialua, HI (21.555 °N, −158.117 °W). This location had a homogenous vegetation cover of Guinea grass (Megathyrsus maximus) that helped to limit the amount of variation in the microbial community due to plant diversity (39, 40). Collected soil was air-dried, homogenized, and sieved to 2 mm. Plastic pots (7.6 L) with drainage holes, covered with a fiberglass screen mesh, were filled with 1 kg of acid-washed sand, followed by 3.5 kg of the homogenized soil mixed with each fertilizer treatment (urea, compost, and a no fertilizer control) at an application rate of 100 kg/ha plant-available nitrogen, which was determined as the sum of inorganic N content and estimated mineralizable N. We used a 3% total N EcoSan compost (3–3.7–1.8, N-P-K), a product of aerobic, thermophilic composting process from human feces and sugarcane bagasse (41, 42). An estimated N mineralization rate of 7% was used for compost based on a recent review of N mineralization in composted biosolids (43). As such, we added 215.4 g of compost (dry weight) to each pot (equivalent to 47.6 metric tons/ha). We used urea (46-0-0, N-P-K) as a contrasting synthetic fertilizer. All N in urea was considered plant-available. Fertilizers were applied only once, immediately prior to the first planting. Each treatment was replicated three times (n = 3) for a total of nine pots (N = 9). Pots were arranged in a randomized control block design to account for possible environmental heterogeneity in the greenhouse. Because the soil had been dried, pots were watered to field capacity and allowed to equilibrate for 2 days prior to planting. Nine organic radish seeds (Raphanus raphanistrum subsp. sativus ‘Cherry Belle', Burpee) were planted in each pot and grown for seven days; they were then thinned to three plants per pot. We chose radishes because of their fast growth from sowing to harvesting that allows for multiple growing cycles within a short period of time. Plants were hand-irrigated with deionized water (100–300 ml/day depending on the weather and stage of growth) to maintain approximate field capacity. Each cycle of radishes was grown for 36 days from seed to maturity, at which point they were harvested. The plants were uprooted, washed with deionized water, and divided at the crown for below and aboveground biomass. All three plants per pot were summed for a total biomass per replicate. Aboveground (shoots) and belowground (taproots) samples were put into paper bags and dried in an oven at 65°C for 2 and 7 days, respectively, for dry weight measurements. Large radish roots were sliced in half to facilitate the drying process. At the end of each harvest, pots were watered and allowed to equilibrate for 2 days prior to the sowing of the next cycle. These plantings and harvesting cycles were repeated for a total of 6 crop cycles, or 227 days, beginning on August 22, 2017.
Soil Sampling
Soil cores were collected immediately after treatment application (C0) and immediately prior to harvest on the 36th day for each of the six crop cycles (C1–C6). From each pot, three cores (1 cm diameter x 10 cm deep, total 63 samples) were taken about 2 cm from each radish taproot. After collection, these cores were composited and homogenized in a clean plastic bag. A subsample was put into a 10 mL transfer tube and stored in a−20°C freezer for microbial analysis. The remaining samples were air-dried and analyzed for pH using a slurry method with a 2 to 1 ratio of deionized water:soil. Soil samples collected after the sixth crop cycle (C6) were analyzed for total carbon, total nitrogen, and exchangeable cations (Table 1). Soil carbon and nitrogen concentrations were analyzed by combustion on an elemental analyzer (Costech 4100 Elemental Analyzer) at the University of Hawai‘i at Hilo Analytical Laboratory. Exchangeable calcium, magnesium, potassium, and sodium were extracted from soils using the ammonium acetate method buffered at pH 7.0 (44) and analyzed on a Thermo iCAP DUO 7400 ICP-OES. Cation exchange capacity was calculated as the sum of base cations.

Table 1. Chemical properties of soils fertilized with urea or compost, compared to an unfertilized control (n = 3).
Amplicon Library Preparation
DNA was extracted from 0.25 g of frozen soil using the DNeasy PowerSoil kit (QIAGEN, Germany) following the manufacturer's standard protocol. Fungal and bacterial mock communities served as positive controls (45). Primer design and sample barcoding followed a two-step amplification, dual barcoding system using a combination of a P5/P7 Illumina Adapter, 8 bp barcodes attached to each adapter, and partial P5/P7 overhangs (Supplementary Figure 1). For bacteria and archaea, the 16S rRNA gene (V4 region) was targeted using updated Earth Microbiome Project primer pairs 515F (46) and 806RB (47). For fungi, the ITS1 region was targeted using primer pairs ITS1F (48) and ITS2 (49). High-fidelity, hot-start polymerase mastermixes were used for the PCR reaction with iProof (Bio-Rad Laboratories, USA) for 16S and Phusion (ThermoFisher Scientific Inc., USA) for ITS. Internal testing showed that these polymerases amplified each respective gene more efficiently. In the first PCR, the targeted loci (16S, ITS) were amplified under the following thermocycling conditions using 3-5 ng of DNA: For 16S, polymerase activation at 98°C for 30 s, followed by 20 cycles of 98°C for 10 s, 55°C for 15 s, 72°C for 10 s, and a final extension at 72°C for 7 min; and for ITS, polymerase activation at 98°C for 30 s, followed by 20 cycles of 98°C for 10 s, 53°C for 15 s, 72°C for 10 s, and a final extension at 72°C for 7 min. PCR products were assessed using gel electrophoresis and successfully amplified samples were cleaned using AMPure SPRI beads (Beckman Coulter, USA). In the second PCR step, 1 μl of the cleaned products was used as templates and amplified using a second set of primers that included P7/P5 overhangs, barcodes, and Illumina adapters (Supplementary Figure 1) under the following thermocycling conditions: polymerase activation at 98°C for 30 s, followed by 14 cycles of 98°C for 10 s, 52°C for 15 s, 72°C for 10 s, and final extension at 72°C for 7 min. PCR products were assessed using gel electrophoresis, and cleaned using SPRI beads as above. Barcoded amplicon libraries were quantified using a Qubit 3 Fluorometer (ThermoFisher Scientific Inc., USA). Each sample for a gene library (16S, ITS) was combined at equimolar concentration, including negative controls according to Nguyen et al. (45). Gene libraries were then combined at a ratio of 3:7 (by mass) ITS to 16S, spiked with 11.75% PhiX, and sequenced together on a single lane of Illumina (MiSeq) PE250 at the University of California, Davis Genome Center. Sequence data was deposited in the Sequence Read Archive under BioProject #PRJNA551045.
Bioinformatics
Sequence data processing and quality control were performed using the QIIME2 v2018.11 workflow and available plugins (50). Raw sequences were demultiplexed and primers and adapters removed. For 16S sequences, ends of sequences with base quality of q < 25 were truncated, and reads were paired and denoised using the DADA2 plugin (51). DADA2 Amplicon Sequence Variants (ASVs) were used for further analyses. For ITS sequences, conservative regions (18S and 5.8S) that flanked the ITS1 gene were removed using ITSXpress (52), followed by pairing and denoising as described above. This step was essential to accurately classify ITS sequences as verified using our mock community data. Furthermore, DADA2 ASVs overinflated fungal mock community richness (multiple OTUs per species) so it was necessary to further cluster these OTUs into 97% similarity OTUs using open reference clustering via the VSEARCH plugin (53). This clustering was essential to accurately recover the expected mock community diversity in the dataset (45). Hereafter both 16S DADA2 ASVs and ITS 97% clustered OTUs will be referred to simply as OTUs. The Naïve Bayes Classifier was used to classify OTUs using the Greengenes Database specifically trimmed for the primer pairs 515F-806R (gg-13-8-99-515-806-nb, (54) for prokaryotic 16S, and the UNITE database version 7.2 (55) for fungal ITS. For 16S, sequences classified as “unassigned”, “mitochondria”, or “chloroplast” were removed. For ITS, only sequences that aligned with at least 70% of reference sequences were kept. Internal validation showed that sequences that do not currently match to 70% of the UNITE v7.2 database are typically non-fungal. Sequences labeled as “unassigned”, “rhizaria, “Protista”, and “Metazoa” were removed. Negative PCR controls showed a maximum occurrence of 3 OTUs for fungi and 6 OTUs for bacteria and archaea. As a quality control measure, these maximum occurrence numbers from the negative control were then used as minimum occurrence thresholds for the rest of the data. As a result, only OTUs that had more than 7 sequences were kept for the 16S dataset and only OTUs that had more than 4 sequences were kept for ITS. The 16S dataset was rarefied to 2831 sequences, and the ITS dataset was rarefied to 514 sequences. Sampling saturation was variable for datasets (Supplementary Figure 2). Trophic guild data for fungi was identified using FUNGuild (56).
Statistical Analyses
Statistical analyses were conducted in R version 3.5.1 (57) and QIIME2 version 2018.11 (50). A repeated measures approach was used to compare changes in above and below plant biomass as well as microbial community richness and diversity as response variables to the different fertilizer treatments and control. A generalized least squares (GLS) model was chosen due to its flexibility when dealing with observations across time (58), which is common for repeated measures experiments with autocorrelated residuals (59, 60). The GLS model yi= X iβ+εi, εi~(Vi), was created using the “lme” function in the package nlme (58), where yi denotes the outcome variable for the i-th group (our response), Xi is the design matrix for the fixed effects β (Treatment + Time; Treatment & Time interactions) and Vi, the variance-covariance matrix for the error terms. Vi was specified to CorAR1, which confined errors within each individual replicate (pot). For the biomass data, a box-cox transformation was performed to meet the assumption of normality and equal variance. An Analysis of Variance (ANOVA) was performed using the “anova” function in base R. Pairwise comparisons of least-squares means were calculated for sample groupings by both treatment and time. These were calculated using functions “lsmeans” and “cld” via the lsmeans and emmeans (61, 62) packages. Least-squared mean comparisons were averaged over the levels of treatment using the Satterthwaite method (63) at a confidence level of 0.95. For the biomass data only, the “glht” function in the multcomp package was used (64). Pairwise comparisons were corrected for multiple comparisons with the Tukey p-adjustment.
Sequence reads obtained through high-throughput sequencing are compositional (65). Hence, in our community composition analysis, we followed protocol as suggested for compositional datasets. The first steps of data wrangling were conducted in R using the vegan package (66). First, unrarefied abundance matrices were normalized using the function “decostand” followed by the Hellinger square root method (67). We found this was sufficient in balancing our data. Next, Euclidean distances were created using the function “vegdist” on the transformed data matrices. We chose Euclidean distances as our metric for dissimilarity because of their appropriateness in Eigenvector-type analyses such as Principal Components Analysis (68–70), which we used for visualization. Community composition was compared using the function “adonis2”, which conducted permutational analysis of variances (PERMANOVAs) on the Euclidean distances as grouped by treatment. These PERMANOVAs were performed at the first crop cycle (1) and then included subsequent crop cycle accumulations (crop cycles 1–2, 1–3, 1–4, 1–5, and 1–6). This allowed us to compare how samples grouped in “species-space” at different points throughout the study. Comparisons were conducted using both an un-nested approach that looked at interactive effects and a nested approach that limited errors within “strata” of time within each crop cycle. Pairwise comparisons of treatment groupings were performed using the Wilks statistic with FDR corrections for multiple testing (59) via the function “pairwise.perm.manova” from the package RVAideMemoire version 0.9–72 (71). Results were visualized using ggplot2 (72) and sjPlot (73).
To determine how different fertilizer types affected the abundance of any specific OTU, we conducted an Analysis of Composition of Microbiomes [ANCOM, (74)], using the “Composition” plug-in for the QIIME2 platform. ANCOM is a robust analysis tool that uses compositional constraints to reduce false discoveries and can improve identification of differentially abundant microbes in complex datasets (75). Differences in relative abundances across treatments were compared at each crop cycle for both fungi and prokaryotes. To differentiate changes that could be confounded by time, relative abundances of OTUs were also compared across crop cycles. This occurred for crop cycles (1–6) for fungi, and because of computational power constraints even at the supercomputer level, we minimized analysis of prokaryotes at intervals of crop cycles (1–4 and 4–6). In addition, we performed a similar test, “log2FoldChange”, for changes in the microbiome using the package DESeq2 (76) to detect significant changes in OTU relative abundance between urea and compost treatments for cycle 1 and cycle 6.
Network Construction
All microbial association networks were created using the R package SPIECEASI (77). The SPIECEASI method was chosen because it was developed for compositional data analysis and assumes taxon-taxon associations scale linearly with the number of measured taxa. It also features greater precision when compared to other methods such as CoNet, gCoda, SparCC, and Spearman network inferences (34). Best-practices in microbial association network construction require large sample sizes to increase the precision of networks (34, 77). Although the sample size in our study was small (n = 3 for each treatment), we found that time across the 6 cropping cycles, although significant, had a much smaller effect in shaping microbial community structure when compared to the effect of the fertilization treatments. The effect of time across cropping cycles had an R2 value ranging from 0.022 to 0.067 for fungi and 0.024 to 0.062 for bacteria & archaea, as compared to the effect of fertilizer type with R2 ranging from 0.298 to 0.387 for fungi and 0.041 to 0.117 for bacteria & archaea (Table 2). Because time across cropping cycles explained a much smaller portion of the variation compared to treatment, we combined samples from all crop cycles (C1–C6) for each fertilizer treatment, which allowed for network inference with a pseudo n = 18 (3 pots x 6 cycles). Using these combined data, we created prokaryote networks for bacteria & archaea alone, fungi alone, and a combined network of bacteria, archaea, and fungi. We recognize that the prokaryotic network is technically a cross-domain network, but for ease of discussion, will refer to the combined network as the cross-domain network henceforth.
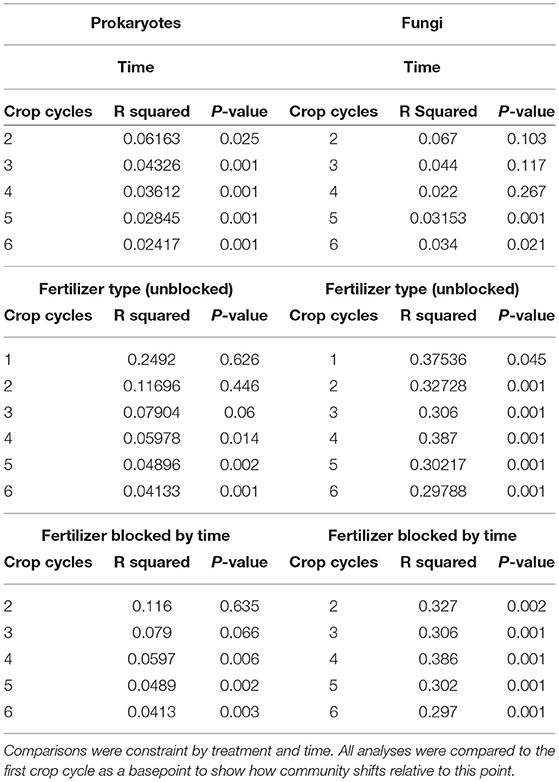
Table 2. PERMANOVA comparison of community differences across cropping cycles for prokaryotes and fungi.
To reduce the likelihood of false positive associations within networks, OTU counts were first filtered for minimum occurrence across ~33% of samples using the R package Phyloseq (78). OTUs with 0 abundance were filtered out of any individual sample, but their sums across the whole dataset were kept so as not to change the overall sample counts. SPIECEASI networks were then assembled via the “spiec.easi” function using the Meinshausen and Bühlmann or “MB” method (79) with a pulsar parameter threshold of 0.05 and lambda minimum ratio of 1e−2 following basic user guidelines (77, 80). The function “multi.spiec.easi” was used for creating cross-amplicon networks under the same parameters. Resulting networks were visualized in Cytoscape V-3.7.1 (81) and network topological properties were assessed using NetworkAnalyzer (82). Subnetworks were created by selecting individual hub OTUs from the CytoScape interface, selecting nearest-neighbors of those OTUs, and then creating a new network from the combined selections.
Network hub taxa were selected based on node degree (the number of edges linked to that node) and closeness centrality (how close a node is to all other nodes) as measurements of overall network connectivity using NetworkAnalyzer in Cytoscape. Currently, there is no consensus for what determines statistically significant network hub taxa, although previous studies determined good candidates by selecting those with higher node degree, betweenness centrality, or closeness centrality based on normal distribution fit with p < 0.1 and correlation cut-offs (83, 84). The SPIECEASI method of network inference is a conservative method that although results in fewer connections than other inference tools, can help to reduce false positives (77, 80). Therefore, we took a simpler approach for determination of hub taxa that did not dismiss non-outliers by choosing the top 5 OTUs that contained the highest degree and closeness centrality for each treatment. We limited our network hub taxa analysis to only the cross-domain networks as these were the most comprehensive network inferences.
Results
Plant Growth, Soil Chemistry, and Microbial Community Response to Fertilization
At the end of the experiment, fertilization treatments did not significantly affect soil pH, which averaged 7.8 across all treatments (Table 1). There was a significant treatment effect on soil total N (p = 0.0002) and a marginally significant effect on soil carbon (p = 0.0696). Compost increased concentrations of soil nitrogen and carbon relative to both urea and control. There was no significant treatment effect on cation exchange capacity by the end of the sixth crop cycle. Calcium was the dominant cation across all treatments. Compost significantly increased concentrations of potassium (p = 0.0031), magnesium (p = 0.0069), and sodium (p = 0.0005).
Plant growth responded differently to treatments in the six consecutive cropping cycles following a single initial application of urea or compost (Figure 1). When compared to the control, compost fertilization significantly increased both aboveground and belowground plant biomass for all crop cycles (p < 0.01). Urea fertilization, in contrast, only significantly increased above and belowground plant biomass in the first crop cycle (p < 0.001). When the treatments were compared to each other, compost had greater biomass relative to the urea treatment for crop cycles 2–6 (p < 0.001).
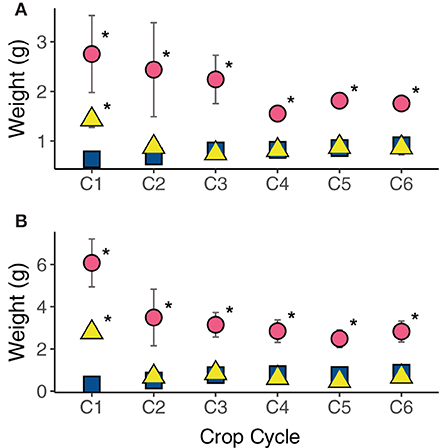
Figure 1. Plant growth response (A) aboveground and (B) belowground to compost (circles) and urea (triangles) fertilization as compared to unfertilized controls (squares) over 6 crop cycles. Asterisks (*) denote significant treatment differences within each cropping cycles compared to the control. Error bars indicates standard error.
Fertilizing soils with either compost or urea affected microbial richness and diversity differently (Figure 2). Fertilizer type did not affect prokaryote richness (p = 0.850), Shannon's Index (p = 0.984) nor Faith's Phylogenetic Diversity (p = 0.635). These metrics remained stable across the six cropping cycles (p > 0.4). These patterns were somewhat different for fungi where fertilization did not affect overall richness (p = 0.326) and Shannon's Index (p = 0.628) when compared to unfertilized soils, but over the six cropping cycles richness (p = 0.001) and diversity (p = 0.004) significantly decreased. Fungal richness and diversity were highest immediately following fertilization of field-collected soil prior to first sowing of seeds, then continued on a decreasing trend until the sixth cycle. These effects were observed for both urea and compost fertilizer sources.
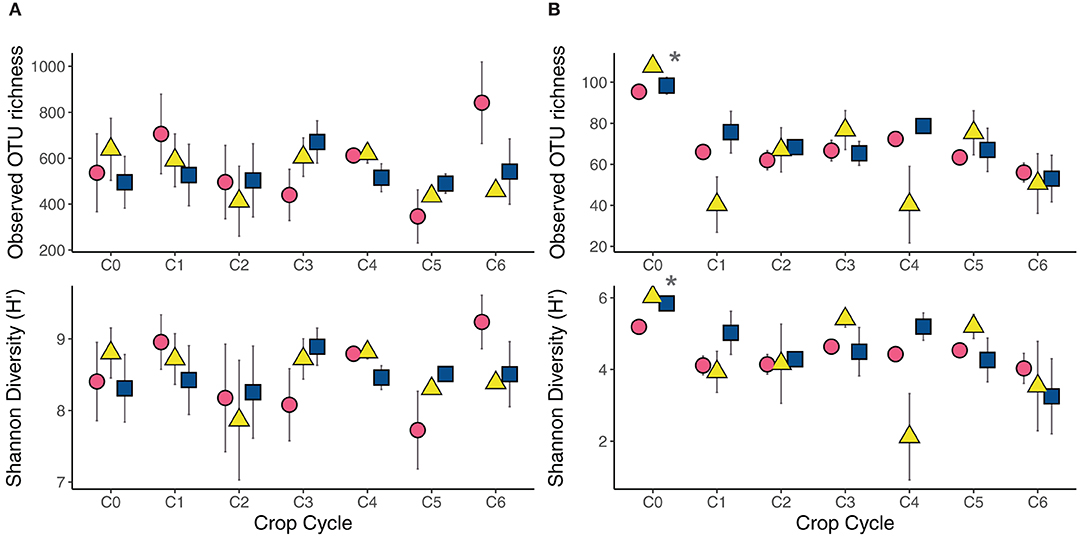
Figure 2. Observed OTUs and Shannon's Diversity index measuring the community responses of (A) prokaryotes and (B) fungi to fertilizer treatments over six crop cycles, including soils prior to planting (C0). Circles indicate compost treatment, triangles indicate urea treatment, and squares indicate controls. Asterisks (*) denote significant treatment differences across all crop cycles. Error bars indicates standard error.
We compared changes in microbial communities of different fertilizers across the six cropping cycles relative to the first cycle and found that fertilizer type significantly changed soil microbial community composition, but these effects were latent in prokaryotic communities (Figure 3, Tables 2, 3). Fungal communities in compost-fetilized soil differed significantly from unfertilized controls (p < 0.013) and urea (p < 0.013), but did not differ between urea-fertilized and unfertilized soils (p > 0.327) (Table 3). The variation in the data explained (R2) across the six cycles ranged from 0.297 to 0.386. Prokaryotic communities did not respond significantly to fertilization until the third crop cycle (p = 0.025) with increasing statistical significance until the sixth cycle. Similar to the fungal communities, prokaryotic communities from compost-fertilized soils differed from unfertilized control (p < 0.048) and urea (p < 0.036), while communities in control and urea-fertilized soils were not statistically different (p > 0.27). The variation in the data explained (R2) across the six cycles ranged from 0.249 at the first crop cycle, and consistently decreasing to 0.041 in cycle 6.
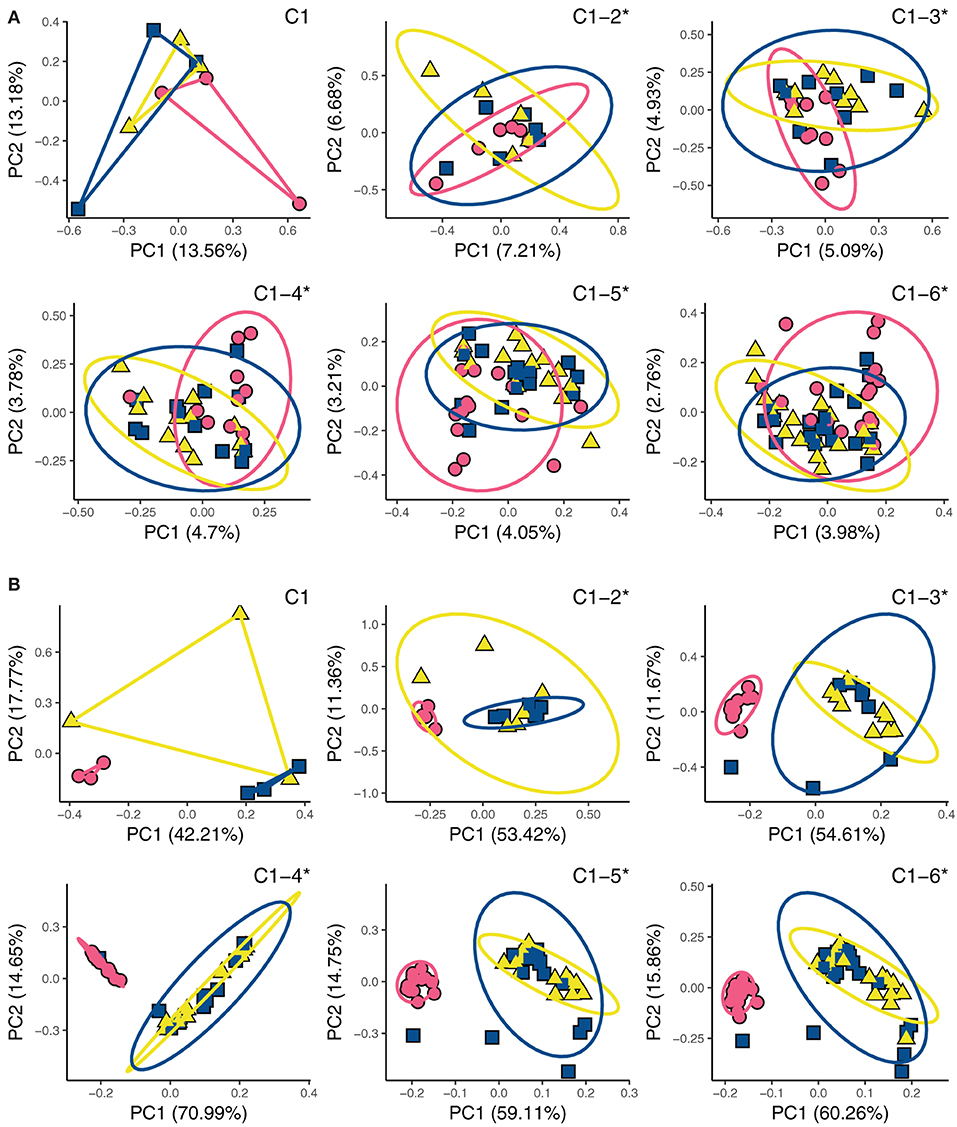
Figure 3. Principal component analysis (PCA) ordination showing (A) prokaryotic and (B) fungal community similarity. Each panel shows an accumulation of samples, starting with crop cycle 1 (C1) and ending at cycle 6 (C1-6). Asterisks (*) denote PERMANOVA significant treatment differences across crop cycles.
Fertilizer type significantly changed the relative abundance of certain individual fungal OTUs based on ANCOM analysis, but trends were more prominent for fungi than for prokaryotes across the 6 cycles (Supplementary Table 1). Two fungal OTUs, Thermomyces lanuginosus and a Myceliophthora sp. were more abundant in compost-fertilized soils. Thermomyces lanuginosus was more abundant for crop cycles 1 (w-score = 142), and crop cycle 6 (w = 146), while Myceliophthora sp. was more abundant in the second (w = 283), third (w = 245), fourth (w = 227), and fifth (w = 205) crop cycles. A Pezizaceae sp. was more abundant in the control and urea treated soils, but only at crop cycle 4. Only one bacterial OTU, an unidentified Acidomicrobiales, was more abundant in the control treatment at the first crop cycle (w = 3952). No differences in the relative abundance of prokaryotic OTUs were detected across all crop cycles.
Fold change analysis of OTU relative abundance in urea vs. compost treatments showed that prokaryotic taxa responded more strongly in crop cycle 1, whereas fungi responded more strongly in crop cycle 6. We detected 214 OTUs within 42 taxa (mostly at the genus level but some could be not assigned) that responded either strongly to urea or compost fertilization (Figure 4). Of these, OTUs of Actinomadura, Catellatospora, Iamia, Phytohabitans, Parasegitibacter, unidentified Chloroflexi, Nitrospira, Gemmata, Planctomyces, Arthrospira, Dechloromonas, Devosia, Luteibacter, Massilia, Tepidimonas, Opitutus, and Prosthecobacter significantly increased in abundance in urea-fertilized soils. In contrast, Kibdelosporangium, Mycobacterium, Rubrobacter, Streptomyces, Flavisolibacter, an unidentified Nitrospiraceae, unidentified Pirellulaceae, Geobacter, Hyphomicrobium, and Reyranella significantly increased in abundance in compost-fertilized soils. Other genera contain OTUs that show different preferences for the different types of fertilizers. No differences in the relative abundance of prokaryotic OTUs were detected at crop cycle 6. We found only one OTU of fungi, Thermomyces lanuginosus, that significantly favored compost at crop cycle 1, but six OTUs at crop cycle 6. These were Acremonium dichromosporum, T. lanuginosus, a Myceliophthora sp., and three unidentified Ascomycota in the orders Sordariales and Eurotiales. General visual comparisons of all OTUs aggregated at the phylum level across all 6 cycles can be found in Supplementary Figures 3, 4.
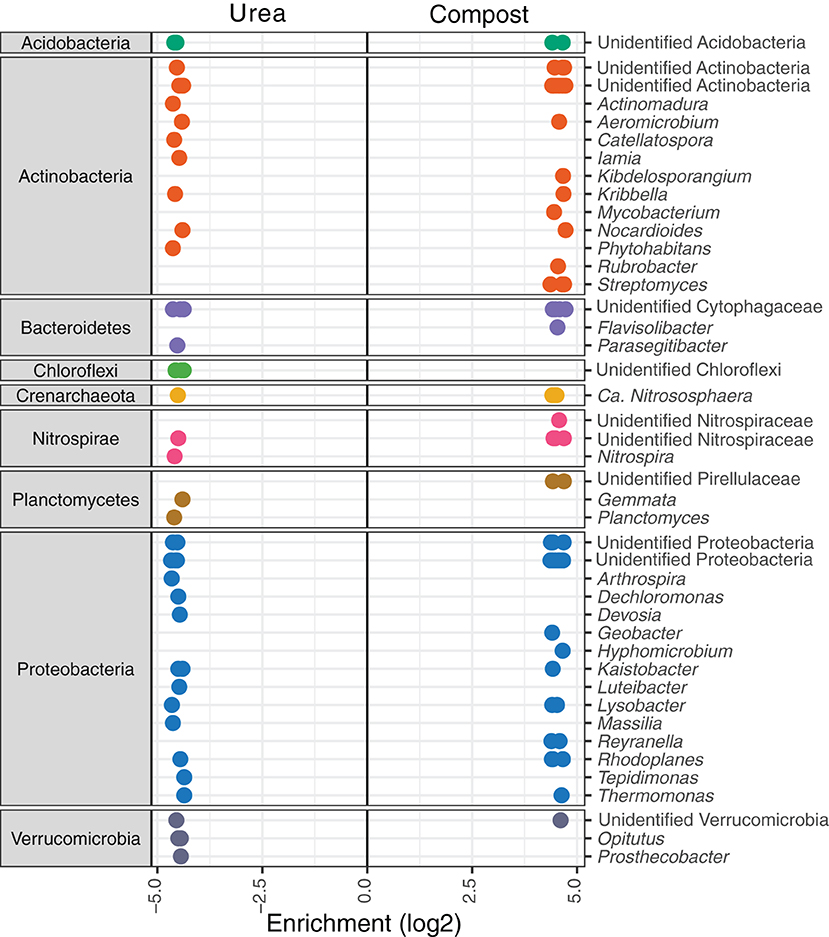
Figure 4. Significant increases in relative abundance of bacterial OTUs in urea and compost-fertilized soils at crop cycle 1. OTUs are grouped by Phylum and identified to genus when possible. Each genus may contain multiple OTUs, represented by each dot on the line. When a genus cannot be identified although they were classified as different genera based on sequence homology, the identity of the most positively identified taxonomic level is provided (e.g., Actinobacteria). Positive log2 fold changes indicate preferences toward compost and negative log2 fold changes indicate preferences toward urea.
Soil Network Complexity
Soils treated with fertilizers altered network topography for the individual prokaryotic network, the fungal network, and the cross-domain network (Figure 5). Network complexity, as measured by the number of nodes and edges, increased when compost was added to the soil, and decreased when urea was added, relative to the control. For the prokaryotic network, the control contained 134 nodes and 150 edges, the compost-fertilized network was higher with 141 nodes and 217 edges, and the urea-fertilized network was fewer with 117 nodes and 154 edges. We observed a similar trend for the fungal networks. The control network had 14 nodes and seven edges, the compost-fertilized network was higher with 44 nodes and 31 edges. The urea-fertilized network, however, had 18 edges and 11 nodes, both of which were higher than the control. The cross-domain networks followed this trend as well where the control network had 229 nodes and 372 edges, the compost-fertilized network had the highest number of 248 nodes and 408 edges, and the urea network had the lowest number of 207 nodes and 307 edges.
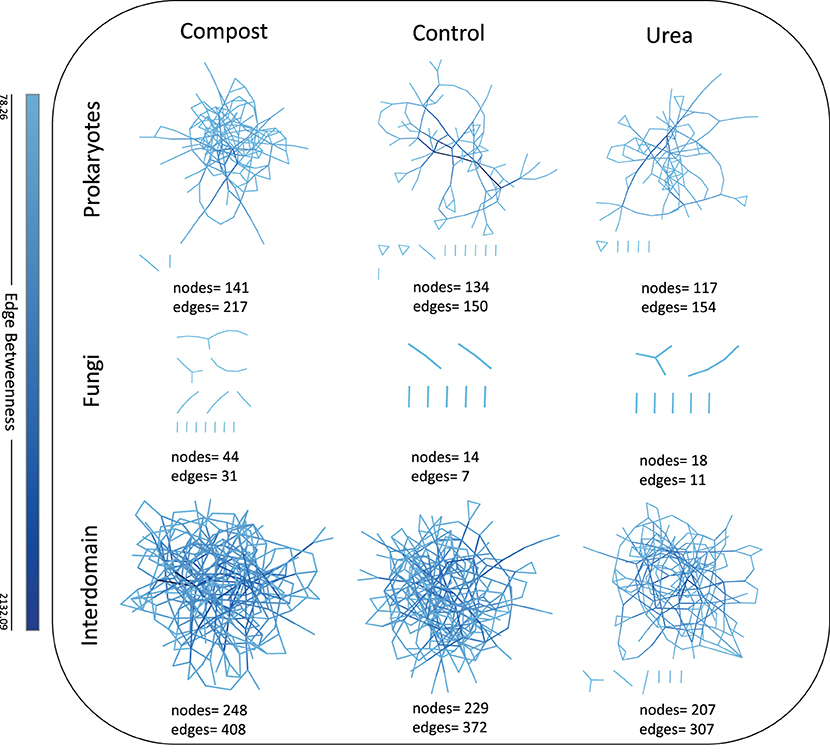
Figure 5. Visual and quantitative measures of association networks for prokaryotes, fungi, and cross-domain across compost, urea, and control. Complexity of networks are represented here by nodes and edges, which may be inferred as predicted interaction, either positive or negative, and are colored by a measurement of edge betweenness, a component of network centrality (85).
Within the networks, fertilizer type had a strong effect on network hub taxa. Not only did fertilizer type affect which OTUs became hub taxa, but it affected how they were connected in the overall networks (Figure 6). The compost-fertilized network contained three bacterial (a Nostocaceae sp., Steroidobacter sp., Bradyrhizobiaceae sp.) and two fungal (Lasidioploidia lignicola, and Tetracladium furcatum) hub taxa. The unfertilized control network contained three bacterial (a Nostocaceae sp., Rhizobiales sp., Micrococales sp.) and two fungal (Hypocreales sp., and Aspergillus purpureus) hub taxa. The urea-fertilized network contained one bacterial taxon (a Solirubrobacteriales sp.) and four fungal (Ascomycota sp., Aspergillus sp., Trechispora sp., and an unidentified fungus) hub taxa. Only one OTU, a member of the Nostocaceae, was present as a hub taxon in both the unfertilized soil and compost-fertilized network, but not the urea-fertilized network. Each hub taxon in the compost-fertilized network was directly connected to other hub taxa, and indirectly connected to 2 or more other hub taxa. In stark contrast, the hub taxa in the unfertilized network shared only indirect connections to other hub taxa, and averaged less than two connections to other hub taxa. Hub taxa in the urea-fertilized network similarly had fewer direct and indirect connections, including one hub taxa that was not connected to any other hub taxa by nearest neighbors.
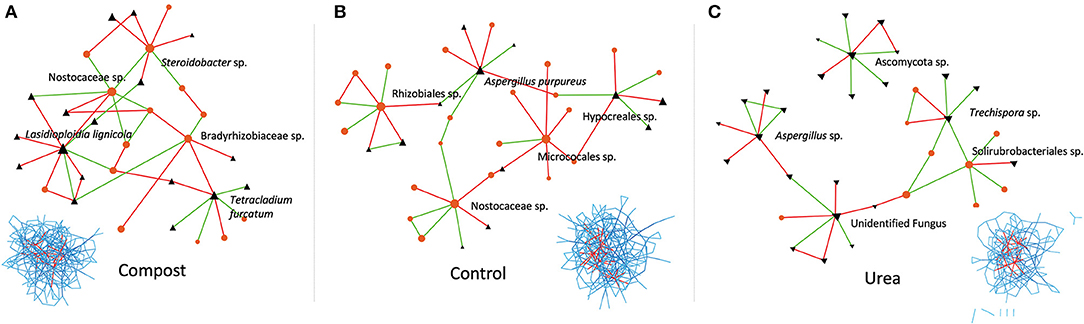
Figure 6. Cross-domain subnetworks showing hub taxa and their interactions across (A) compost, (B) control, and (C) Urea. Bacterial nodes are represented by orange circles, and fungal nodes are represented by black triangles. Inset shows the location of the subnetworks (red) within the full cross-domain network (blue). Bacterial nodes are indicated by orange circles and fungal nodes are indicated by black triangles. Edges between nodes represented a positive association (green) or negative association (red).
A comparison of OTUs found in the ANCOM analysis with those from the hub taxa analysis showed that the taxon Thermomyces lanuginosus (significantly more abundant in compost-fertilized soil) was directly connected to a hub taxon, a Steroidobacter sp. (Supplementary Figure 5). The Myceliophthora sp. was found in a separate node cluster and was neither a hub taxon nor connected to one (data not shown). No other taxa from the ANCOM analysis were found to interact closely with network hub taxa of any treatment group. The same T. lanuginosus and Myceliophthora sp. were also detected in the fold change analysis, favoring compost-fertilized soil.
Discussion
In this study, we provided direct evidence that a single application of a synthetic N source or a more complex N compost prior to planting can have different effects on plant growth, soil microbial composition and network complexity, but not microbial richness and diversity. High N composts can be an effective plant fertilizer in tropical soils (86, 87) especially in the nutrient-poor Oxisols used in this experiment. Compost imparted greater residual effects on plant growth than urea throughout the experiment. These trends were expected since urea is rapidly hydrolyzed by soil bacteria and fungi to provide a readily available N source (i.e., ) at the beginning of the first growth cycle (88, 89). This can be taken up by plant roots, consumed by microorganism, lost through ammonia volatilization (17) or be converted by ammonia oxidizing bacteria or archaea to nitrate (16). The nitrate is either taken up by plant roots, immobilized by microorganisms, denitrified, or leached out of the soil system with percolating water (90). The labile N pool in compost is subjected to the same fates, but compost also contains N sources associated with more complex organic compounds that mineralize N more slowly through microbial-mediated transformations (27, 91, 92). In this sense, high N organic compost not only provides a rapidly available nutrient pool upon application but also provides a slowly available pool of organic nitrogen, including the many additional benefits of substrates rich in organic matter that continues to support plant growth in subsequent cycles.
The relatively low to no plant response to urea fertilization contradicts expectations given that urea is also known to be an effective seasonal N fertilizer in agricultural systems in the tropics and elsewhere (93). Considering that urea is rapidly hydrolyzed, we would not expect residual effects of urea on crop growth or soil total nitrogen across multiple subsequent crop cycles, and this is reflected in our results. However, we would expect that there would be less of a difference in plant biomass between the two fertilizer types since they were applied at an equivalent available N basis. The observed significant differences between plant biomass may be explained through volatilization of a substantial portion of urea-N that reduces plant nitrogen use efficiency and thus plant growth (17). An alternative explanation is that plant growth was limited by deficiencies in other essential nutrients, such as phosphorus or potassium, especially considering that Oxisols tend to have low capacity to retain and supply nutrients (94). Compost, on the other hand, supplies the plants with more than just N (e.g., P, K, etc…), thus potentially alleviating multiple macro- and micronutrient deficiencies. While the compost treatment did not alter cation exchange capacity of the soil, as base saturation was dominated by calcium, it did increase concentrations of potassium, magnesium, and sodium.
Although a single application of different fertilizer types can have significant effects on plant growth, it did not affect the overall microbial richness and diversity. This finding was unexpected in the soils fertilized with compost given that composted materials often contain a diversity of microorganisms (95) thereby having the potential to increase a soil's richness and diversity. However, Pérez-Piqueres et al. (27) showed that community responses may be dependent upon the nature of the compost in combination with soil types. In addition, changes in physicochemical properties favoring microbial proliferation might be a more important factor for the soil community response to compost fertilization than the compost-borne organisms themselves (96). Urea fertilization also did not decrease microbial richness and diversity. These results suggest that a longer time period of urea fertilizer applications is perhaps necessary to observe the effects of decreased bacterial diversity (18, 97). Repeated planting through time did not change prokaryotic diversity but did have an effect on fungi. These measures were highest in the field-collected soil before active radish growth, suggesting a possible selection process in the rhizosphere as an effect of the active radish growth (98), in part through the production of carbon and energy rich compounds, and bioactive phytochemicals (99).
This study provides evidence that the type of soil fertilizer can affect microbial community composition differently. The concept that organic fertilizers can have a strong impact on soil microbial communities is reflected in previous literature investigating microbial community shifts under long term organic vs. conventional management systems (27, 96). In the present study, even single applications of compost caused detectable continued community shifts relative to the first sampling point for the duration of this 227-day study. Conversely, the microbial community did not significantly shift in response to a single application of urea fertilizer, although we did detect significant shifts in certain genera. Previous research suggests that negative impacts on the community can occur due to the repeated use of synthetic N fertilization over long periods of time (18, 97, 100); however, short-term applications, like in this study, may not be enough to inhibit resident microbial composition (18). This work reflects our current understanding of how disturbance events (such as those caused by fertilization, especially compost), can significantly shift microbial communities, and that the legacy effect left by roots grown in the previous cycle is not likely the strongest driver of soil microbial communities (101). The contrast in microbial community shifts between compost and urea highlights the need to better understand the mechanisms of how each of these fertilizers may affect bacteria and fungi differently. This is especially important if we were to more effectively apply as a means to reduce plant pathogens or improving soil health and fertility in the tropics (102, 103).
To gain a more nuanced understanding of how different members of the microbial community respond to the urea or compost treatment as opposed to whole community analyses discussed above, we applied ANCOM and DESeq analyses that identify significantly associated with experimental variables. Of the genera that favored urea-fertilized soils, increases in the genus Nitrospira, and OTUs of the phylum Chloroflexi are consistent with the increases in ammonium nitrogen found in the urea-fertilized soils. Nitrospira contribute to nitrogen cycling by oxidizing nitrite to nitrate, and certain members of the group can completely denitrify ammonia to nitrate (104). Chloroflexi are often found associated with nitrogen rich environments like treatment plants that are designed to remove nitrogen (105). The absence of Streptomyces in urea-fertilized soils but its significantly higher abundance in compost-fertilized soil suggests a preference for this genus for organic matter rather than simply nitrogen alone. Apart from these specific examples, most of the genera showing significant increases have members that prefer either of these substrates (Figure 4). Further work using more sensitive methods will be required to make stronger inferences into the preference of each of these taxa and how they contribute to nitrogen cycling in soil.
We found several fungal OTUs to be significantly associated with the compost treatment. Of these, T. lanuginosus and Myceliopthora sp. are thermophilic, compost-dwelling saprotrophic fungi (106). They are likely inhabitants of the compost and introduced into the study soil system via the compost fertilizer. It is possible that these organisms could be contributing to an improved role in decomposition and nutrient cycling in the soil system, or that they are displacing native soil microbes with the same ecological niche. Thermomyces lanuginosus has mostly been observed as a saprotroph, although it has been recorded as a potential opportunistic human pathogen (107), and although pathogenicity of this organism was not confirmed in this study, it is important to consider the health and ecosystem risk of organisms introduced to soil via compost fertilizer (108–110). The mechanisms for how individual OTUs, such as the two found here, might successfully establish themselves in a soil system was not part of this study [although see Gravuer and Scow (28) for interesting insights]. However, mechanisms behind rhizosphere competency are currently of great scientific and economic interest because of the growing popularity of microbes as biofertilizers. Identifying organisms that are rhizosphere competent and what factors might influence rhizosphere competency are key to developing more effective agricultural products (111).
The choice of fertilizer influenced network complexity (and thus network stability and robustness) in both individual and cross-domain networks. Complex organic fertilizers such as compost resulted in higher network complexity, similar to Schmid et al. (31) who found that long-term amendments of manure or straw to agricultural soils increased bacterial network complexity. Other studies investigating single-domain networks have shown very clear differences in network topology when comparing stark habitat differences like the rhizosphere vs. bulk soil (29) or when comparing different microbiome reactions to stressful conditions like extreme drought (32). Here we showed that networks were more complex in compost-fertilized soils as compared to the control or urea (Figure 5). The less complex networks in urea relative to the control suggests that simple sources of fertilizers may not be able to support robust networks across multiple planting cycles. Indeed, the amount of total C & N at cycle 6 (Table 1) remained generally higher in compost, lending further support to the idea that more complex fertilizer sources that slowly release nutrients may be able to support stronger networks of microbes over time. Cross-domain networks provide a more comprehensive understanding of the complexity of soil communities than individual-domain networks. Using cross-domain network interactions such as those between prokaryotes and fungi can provide a greater understanding of beneficial, antagonistic, and associative interactions of the microbes in the soil system (35, 37, 84). This study revealed greater connectivity in cross-domain networks compared to individual-domain networks, and from these network associations, we can create and test hypotheses about how these organisms might interact. For example, the OTU Nostocaceae sp. was less connected in the urea network compared to the compost where it acted as a hub taxon (Figure 6A). It is known that members of the bacterial family Nostocaceae photosynthesize, and in some cases can fix nitrogen (112). As a possible N contributor in this soil system, Nostocaceae could be important in helping to provide a source of plant N in an agricultural system. Future research is needed to investigate whether urea negatively affects potentially beneficial hub taxa in soil. Similarly, the OTU Nostocaceae sp. has a negative association with another hub taxon, Lasiodiplodia lignicola, in the compost network (Figure 6A). Many Lasiodiplodia are recognized plant and animal pathogens (113). This interaction between a possibly beneficial microbe acting antagonistically toward a potential plant pathogen could be further explored. Whether these hub taxa behave as keystone species, whose removal could cause the collapse of an ecosystem, or that they are lever species, which can steer ecosystems toward specific community types (114), by directly inhibiting or facilitating the growth of other microbes and thus affecting overall the interconnectedness of communities (115) pose an interesting set of questions to be tested in soil community ecology.
Beyond generating hypotheses for the interactions among different members of a microbiome, cross-domain networks provide a different dimension within which to quantitatively measure the microbiome interactions. For instance, Shi et al. (29) showed that rhizosphere microbiome network complexity increased with time relative to the bulk soil that had relatively consistent and weak network complexity. In the present study, network complexity can be used as a tool to quantitatively measure a shift in microbiome in response to a soil fertilizer. This has relevance in many areas of microbial community ecology since it is still not yet trivial to quantitatively compare shifts in microbial community composition across different studies. Under the framework of network complexity, such community shifts may be comparable across studies to provide a quantitative measure that can translate to ecosystem function. Development of such methods would be a leap forward in microbial ecology.
Conclusion
In this study, we provided multiple lines of evidence obtained through multiple types of analyses that partially supported the hypothesis that complex N fertilizers when applied to soils have a detectable impact on plant growth, soil microbial diversity, community structure, network topography, and change network hub taxa as compared to urea, a simpler N fertilizer. Unlike urea, a single application of compost, a complex N-fertilizer, increased plant growth throughout the duration of the experiment, selected for a subset of fungal OTUs, and shifted overall microbial community structure. Fertilization with compost also altered overall microbial network topography by increasing network and hub taxa connectivity, especially across domains. Network analysis can play an important role in detecting cross-domain interactions that might be important drivers of microbial interactions in the soil, and network complexity could be developed into a tool that allows the research community to quantitatively compare microbial shifts across studies. Although our study was limited by the number of soils tested, the type of fertilizers used, source inoculum identity in the compost, and under controlled conditions, we showed that even a single amendment of a complex N-fertilizer source such as compost can have prolonged impact on soil nitrogen concentration, plant growth, as well as soil microbial community assembly under low-input settings. Future experiments using a broader set of soils and wider range of fertilizers under field setting will provide stronger support for the concepts highlighted here.
Data Availability Statement
The datasets presented in this study can be found in online repositories. The names of the repository/repositories and accession number(s) can be found at: https://www.ncbi.nlm.nih.gov/, PRJNA551045; https://github.com/nnguyenlab/N-fertilizer-microbes, GitHub.
Author Contributions
SH, RR, and NN conceived the research and setup the experiment. SH performed the experimental work and drafted the manuscript. SH and TM analyzed the data. NN, RR, and TM edited and revised the manuscript. All authors contributed to the article and approved the submitted version.
Funding
This work was supported by the USDA National Institute of Food and Agriculture, Hatch project 8042H, managed by the College of Tropical Agriculture and Human Resources, the Foundational and Applied Science Program, grant no. 2020-67020-31173/project accession no. 1022247, from the U.S. Department of Agriculture, National Institute of Food and Agriculture, and in part by the U.S. Department of Energy Office of Science Office of Biological and Environmental Research Genomic Science program under award DE-SC0020163, subawarded to NN at UH Manoa.
Conflict of Interest
The authors declare that the research was conducted in the absence of any commercial or financial relationships that could be construed as a potential conflict of interest.
Publisher's Note
All claims expressed in this article are solely those of the authors and do not necessarily represent those of their affiliated organizations, or those of the publisher, the editors and the reviewers. Any product that may be evaluated in this article, or claim that may be made by its manufacturer, is not guaranteed or endorsed by the publisher.
Acknowledgments
We thank Michael Kantar for invaluable help with statistical analyses, Laura Tipton for assistance setting up the cross-kingdom network analyses, Ricky Lewis for DESeq analysis pipeline, Kate Porterfield for her initial work in preparing and designing the chambered pot system, as well as Naomi Jun, Jonathan Fisk, and Ishwora Dhungana for their assistance with some of the greenhouse work. We thank the three reviewers for providing detailed constructive comments that helped to improve the manuscript.
Supplementary Material
The Supplementary Material for this article can be found online at: https://www.frontiersin.org/articles/10.3389/fsoil.2022.749212/full#supplementary-material
References
1. Bharti V, Dotaniya M, Shukla S, Yadav V. Managing soil fertility through microbes: prospects, challenges and future strategies BT-agro-environmental sustainability. In: Agro-Environmental Sustainability. Cham: Springer (2017), p. 81–111.
2. Kibblewhite MG, Ritz K, Swift MJ. Soil health in agricultural systems. Philos Trans R Soc Lond B Biol Sci. (2008) 363:685–701. doi: 10.1098/rstb.2007.2178
3. Allen DE, Singh BP, Dalal RC. Soil Health Indicators Under Climate Change: A Review of Current Knowledge. In: Singh BP. Cowie AL, Chan CC, Singh BP, Cowie AL, Chan YK, editors. Soil Health and Climate Change Soil Biology. Berlin, Heidelberg. (2011) p. 25–45. doi: 10.1007/978-3-642-20256-8_2
4. Fierer N, Jackson RB. The diversity biogeography of soil bacterial communities. Proc Natl Acad Sci U S A. (2006)103:626. doi: 10.1073/pnas.0507535103
5. Li J, Li Y, Xiang-dong Y, Jian-jun Z, Zhi-an L, Bing-qiang Z. Microbial community structure and functional metabolic diversity are associated with organic carbon availability in an agricultural soil. J Integr Agric. (2015) 14:2500–11. doi: 10.1016/S2095-3119(15)61229-1
6. Fierer N. Embracing the unknown: disentangling the complexities of the soil microbiome. Nat Rev Microbiol. (2017) 15:579–90. doi: 10.1038/nrmicro.2017.87
7. Nakatsu CH, Carmosini N, Baldwin B, Beasley F, Kourtev P, Konopka A. Soil microbial community responses to additions of organic carbon substrates and heavy metals (Pb and Cr). Appl Environ Microbiol. (2005) 71:7679–89. doi: 10.1128/AEM.71.12.7679-7689.2005
8. Stark CH, Condron LM, O'Callaghan M, Stewart A, Di HJ. Differences in soil enzyme activities, microbial community structure and short-term nitrogen mineralisation resulting from farm management history and organic matter amendments. Soil Biol Biochem. (2008) 40:1352–63. doi: 10.1016/j.soilbio.2007.09.025
9. Geisseler D, Scow KM. Long-term effects of mineral fertilizers on soil microorganisms – a review. Soil Biol Biochem. (2014) 75:54–63. doi: 10.1016/j.soilbio.2014.03.023
10. Chaudhary DR, Gautam RK, Ghosh A, Chikara J, Jha B. Effect of nitrogen management on soil microbial community and enzymatic activities in Jatropha curcas L. Plantation. CLEAN - Soil, Air, Water. (2015) 43:1058–65. doi: 10.1002/clen.201400357
11. Bünemann EK, Bongiorno G, Bai Z, Creamer RE, De Deyn G, de Goede R, et al. Soil quality – a critical review. Soil Biol Biochem. (2018) 120:105–25. doi: 10.1016/j.soilbio.2018.01.030
12. Heffer P, Prud'homme M. Fertilizer outlook 2015–2019. In: 83rd IFA annual conference. Istanbul Turkey. (2015) p. 25–27.
13. Chin W, Kroontje W. Urea Hydrolysis Subsequent Loss of Ammonia. Soil Sci Soc Am J. (1963) 27:316–8. doi: 10.2136/sssaj1963.03615995002700030030x
14. Sigurdarson JJ, Svane S, Karring H. The molecular processes of urea hydrolysis in relation to ammonia emissions from agriculture. Rev Environ Sci Biotechnol. (2018) 17:241–58. doi: 10.1007/s11157-018-9466-1
15. Witte C-P. Urea metabolism in plants. Plant Sci. (2011) 180:431–8. doi: 10.1016/j.plantsci.2010.11.010
16. Zhu S, Vivanco JM, Manter DK. Nitrogen fertilizer rate affects root exudation, the rhizosphere microbiome and nitrogen-use-efficiency of maize. Appl Soil Ecol. (2016) 107:324–33. doi: 10.1016/j.apsoil.2016.07.009
17. Sun R, Li W, Hu C, Liu B. Long-term urea fertilization alters the composition and increases the abundance of soil ureolytic bacterial communities in an upland soil. FEMS Microbiol Ecol. (2019) 95:fiz044. doi: 10.1093/femsec/fiz044
18. Fauci MF, Dick RP. Soil microbial dynamics: short- long-term effects of inorganic organic nitrogen. Soil Sci Soc Am J. (1994) 58:801–6. doi: 10.2136/sssaj1994.03615995005800030023x
19. Edmeades DC. The long-term effects of manures and fertilisers on soil productivity and quality: a review. Nutr Cycl Agroecosystems. (2003) 66:165–80. doi: 10.1023/A:1023999816690
20. Gong W, Yan X, Wang J, Hu T, Gong Y. Long-term applications of chemical and organic fertilizers on plant-available nitrogen pools and nitrogen management index. Biol Fertil Soils. (2011) 47:767. doi: 10.1007/s00374-011-0585-x
21. Gardner JB, Drinkwater LE. The fate of nitrogen in grain cropping systems: a meta-analysis of 15N field experiments. Ecol Appl. (2009) 19:2167–84. doi: 10.1890/08-1122.1
22. Birkhofer K, Bezemer TM, Bloem J, Bonkowski M, Christensen S, Dubois D, et al. Long-term organic farming fosters below and aboveground biota: Implications for soil quality, biological control and productivity. Soil Biol Biochem. (2008) 40:2297–308. doi: 10.1016/j.soilbio.2008.05.007
23. van Bruggen AHC, Semenov AM. In search of biological indicators for soil health and disease suppression. Appl Soil Ecol. (2000) 15:13–24. doi: 10.1016/S0929-1393(00)00068-8
24. Mazzola M. Assessment management of soil microbial community structure for disease suppression. Annu Rev Phytopathol. (2004) 42:35–59. doi: 10.1146/annurev.phyto.42.040803.140408
25. Steiner C, Glaser B, Teixeira WG, Lehmann J, Blum WEH, Zech W. Nitrogen retention and plant uptake on a highly weathered central Amazonian Ferralsol amended with compost and charcoal. J Plant Nutr Soil Sci. (2008) 171:893–9. doi: 10.1002/jpln.200625199
26. Rivero C, Chirenje T, Ma LQ, Martinez G. Influence of compost on soil organic matter quality under tropical conditions. Geoderma. (2004) 123:355–61. doi: 10.1016/j.geoderma.2004.03.002
27. Pérez-Piqueres A, Edel-Hermann V, Alabouvette C, Steinberg C. Response of soil microbial communities to compost amendments. Soil Biol Biochem. (2006) 38:460–70. doi: 10.1016/j.soilbio.2005.05.025
28. Gravuer K, Scow KM. Invader-resident relatedness and soil management history shape patterns of invasion of compost microbial populations into agricultural soils. Appl Soil Ecol. (2021) 158:103795. doi: 10.1016/j.apsoil.2020.103795
29. Shi S, Nuccio EE, Shi ZJ, He Z, Zhou J, Firestone MK. The interconnected rhizosphere: High network complexity dominates rhizosphere assemblages. Ecol Lett. (2016) 19:926–36. doi: 10.1111/ele.12630
30. Wei Z, Hu X, Li X, Zhang Y, Jiang L, Li J, et al. The rhizospheric microbial community structure and diversity of deciduous and evergreen forests in Taihu Lake area, China. PLoS ONE. (2017) 12:e0174411. doi: 10.1371/journal.pone.0174411
31. Schmid CAO, Schröder P, Armbruster M, Schloter M. Organic amendments in a long-term field trial—consequences for the bulk soil bacterial community as revealed by network analysis. Microb Ecol. (2018) 76:226–39. doi: 10.1007/s00248-017-1110-z
32. de Vries FT, Griffiths RI, Bailey M, Craig H, Girlanda M, Gweon HS, et al. Soil bacterial networks are less stable under drought than fungal networks. Nat Commun. (2018) 9:3033. doi: 10.1038/s41467-018-05516-7
33. Wang H, Wei Z, Mei L, Gu J, Yin S, Faust K, et al. Combined use of network inference tools identifies ecologically meaningful bacterial associations in a paddy soil. Soil Biol Biochem. (2017) 105:227–35. doi: 10.1016/j.soilbio.2016.11.029
34. Röttjers L, Faust K. From hairballs to hypotheses–biological insights from microbial networks. FEMS Microbiol Rev. (2018) 42:761–80. doi: 10.1093/femsre/fuy030
35. Tipton L, Müller CL, Kurtz ZD, Huang L, Kleerup E, Morris A, et al. Fungi stabilize connectivity in the lung and skin microbial ecosystems. Microbiome. (2018) 6:12. doi: 10.1186/s40168-017-0393-0
36. Mundra S, Kjønaas OJ, Morgado LN, Krabberød AK, Ransedokken Y, Kauserud H. Soil depth matters: Shift in composition and inter-kingdom co-occurrence patterns of microorganisms in forest soils. FEMS Microbiol Ecol. (2021) 97:fiab022. doi: 10.1093/femsec/fiab022
37. Yuan MM, Kakouridis A, Starr E, Nguyen N, Shi S, Zhou J, et al. Fungal-bacterial cooccurrence patterns differ between arbuscular mycorrhizal fungi and nonmycorrhizal fungi across soil niches. MBio. (2021) 12:1–19. doi: 10.1128/mBio.03509-20
39. Grayston SJ, Wang S, Campbell CD, Edwards AC. Selective influence of plant species on microbial diversity in the rhizosphere. Soil Biol Biochem. (1998) 30:369–78. doi: 10.1016/S0038-0717(97)00124-7
40. Berg G, Smalla K. Plant species and soil type cooperatively shape the structure and function of microbial communities in the rhizosphere. FEMS Microbiol Ecol. (2009) 68:1–13. doi: 10.1111/j.1574-6941.2009.00654.x
41. Ryals R, McNicol G, Porder S, Kramer S. Greenhouse gas fluxes from human waste management pathways in Haiti. J Clean Prod. (2019) 226:106–13. doi: 10.1016/j.jclepro.2019.04.079
42. McNicol G, Jeliazovski J, François JJ, Kramer S, Ryals R. Climate change mitigation potential in sanitation via off-site composting of human waste. Nat Clim Chang. (2020) 10:545–9. doi: 10.1038/s41558-020-0782-4
43. Rigby H, Clarke BO, Pritchard DL, Meehan B, Beshah F, Smith SR, et al. A critical review of nitrogen mineralization in biosolids-amended soil, the associated fertilizer value for crop production and potential for emissions to the environment. Sci Total Environ. (2016) 541:1310–38. doi: 10.1016/j.scitotenv.2015.08.089
44. Lavkulich L. Exchangeable cations and total exchange capacity by the ammonium acetate method at pH 7.0. Canadian Society of Soil Science, Ottawa, Ontario, Canada (1981).
45. Nguyen NH, Smith D, Peay KG, Kennedy PG. Parsing ecological signal from noise in next generation amplicon sequencing. New Phytol. (2015) 205:1389–93. doi: 10.1111/nph.12923
46. Parada AE, Needham DM, Fuhrman JA. Every base matters: assessing small subunit rRNA primers for marine microbiomes with mock communities, time series and global field samples. Environ Microbiol. (2016) 18:1403–14. doi: 10.1111/1462-2920.13023
47. Apprill A, McNally S, Parsons R, Weber L. Minor revision to V4 region SSU rRNA 806R gene primer greatly increases detection of SAR11 bacterioplankton. Aquat Microb Ecol. (2015) 75:129–37. doi: 10.3354/ame01753
48. White T, Bruns TD, Lee S, Taylor J. Amplification direct sequencing of fungal ribosomal RNA genes for phylogenetics. In: Innis MA, editor. PCR protocols: a guide to methods and applications. San Diego. (1990) p 315–322. doi: 10.1016/B978-0-12-372180-8.50042-1
49. Gardes M, Bruns TD. ITS primers with enhanced specificity for basidiomycetes – application to the identification of mycorrhizae and rusts. Mol Ecol. (1993) 2:113–8. doi: 10.1111/j.1365-294X.1993.tb00005.x
50. Bolyen E, Rideout JR, Dillon MR, Bokulich NA, Abnet CC, Al-Ghalith GA, et al. Reproducible, interactive, scalable and extensible microbiome data science using QIIME 2. Nat Biotechnol. (2019) 37:852–7. doi: 10.1038/s41587-019-0209-9
51. Callahan BJ, McMurdie PJ, Rosen MJ, Han AW, Johnson AJA, Holmes SP. DADA2: High resolution sample inference from Illumina amplicon data. Nat Methods. (2016) 13:581–3. doi: 10.1038/nmeth.3869
52. Rivers AR, Weber KC, Gardner TG, Liu S, Armstrong SD. ITSxpress: Software to rapidly trim internally transcribed spacer sequences with quality scores for marker gene analysis [version 1; peer review: 2 approved]. F1000 Res. (2018) 7:1418. doi: 10.12688/f1000research.15704.1
53. Rognes T, Flouri T, Nichols B, Quince C, Mah, é F. VSEARCH: a versatile open source tool for metagenomics. PeerJ (San Fr. CA). (2016) 4:e2584. doi: 10.7717/peerj.2584
54. DeSantis TZ, Stone CE, Murray SR, Moberg JP, Andersen GL. Rapid quantification and taxonomic classification of environmental DNA from both prokaryotic and eukaryotic origins using a microarray. FEMS Microbiol Ecol. (2005) 245:271–8. doi: 10.1016/j.femsle.2005.03.016
55. UNITE Community. UNITE QIIME release. Version 01.12.2017. (2017). Available online at: https://unite.ut.ee/repository.php
56. Nguyen NH, Song Z, Bates ST, Branco S, Tedersoo L, Menke J, et al. FUNGuild: an open annotation tool for parsing fungal community datasets by ecological guild. Fungal Ecol. (2016) 20:241–8. doi: 10.1016/j.funeco.2015.06.006
57. R Core Team. R: A Language Environment for Statistical Computing. (2018). Available online at: https://www.r-project.org/.
58. Pinheiro J, Bates D. Mixed-Effects Models in S S-PLUS. New York, NY: Springer (2000). Available online at: http://www.springer.com/us/book/9780387989570
59. Hand DJ, Taylor CC. Multivariate analysis of variance and repeated measures: a practical approach for behavioural scientists. Boca Raton, FL. (1987). doi: 10.1007/978-94-009-3143-5
60. Schober P, Vetter TR. Repeated measures designs and analysis of longitudinal data: If at first you do not succeed-try, try again. Anesth Analg. (2018) 127:569–75. doi: 10.1213/ANE.0000000000003511
61. Lenth R. lsmeans: Least-Squares Means. 2.30–0. (2018). Available online at: https://cran.r-project.org/package=lsmeans.
62. Lenth R, Singmann H, Love J, Buerkner P, Herve M. emmeans: Estimated Marginal Means, aka Least-Squares Means. 1.3.3. (2019). Available online at: https://cran.r-project.org/package=emmeans.
63. Westfall PH, Tobias RD, Wolfinger RD. Multiple comparisons and multiple tests using SAS. SAS Institute. (2011).
64. Hothorn T, Bretz F, Westfall P. Simultaneous Inference in General Parametric Models. Biometrical J. (2008) 50:346–63. doi: 10.1002/bimj.200810425
65. Gloor GB, Macklaim JM, Pawlowsky-Glahn V, Egozcue JJ. Microbiome datasets are compositional: And this is not optional. Front Microbiol. (2017) 8:1–6. doi: 10.3389/fmicb.2017.02224
66. Oksanen J, Blanchet FG, Friendly M, Kindt R, Legendre P, McGlinn D, et al. vegan: Community Ecology Package. (2017). Available online at: https://cran.r-project.org/package=vegan.
67. Legendre P, Gallagher ED. Ecologically meaningful transformations for ordination of species data. Oecologia. (2001) 129:271–80. doi: 10.1007/s004420100716
68. Anderson MJ, Willis TJ. Canonical analysis of principal coordinates: a useful method of constrained ordination for ecology. Ecol. (2003) 84:511–25. doi: 10.1890/0012-9658(2003)0840511:CAOPCA2.0.CO;2
69. Ramette A. Multivariate analyses in microbial ecology. FEMS Microbiol Ecol. (2007) 62:142–60. doi: 10.1111/j.1574-6941.2007.00375.x
70. Oksanen J. Multivariate analysis of ecological communities in R: vegan tutorial. R Packag version. (2011) 1:1–43.
71. Hervé M. RVAideMemoire: Testing Plotting Procedures for Biostatistics. 0.9–75. (2020). Available online at: https://cran.r-project.org/package=RVAideMemoire.
72. Wickham H, Chang W, Henry L, Pedersen TL, Takahashi K, Wilke C. ggplot2: Create Elegant Data Visualisations Using the Grammar of Graphics. 3.1.0. (2018). Available online at: https://cran.r-project.org/package=ggplot2.
73. Lüdecke D, Schwemmer C. sjPlot: Data Visualization for Statistics in Social Science. 2.6.2. (2018). Available online at: https://cran.r-project.org/package=sjPlot.
74. Mandal S, Van Treuren W, White RA, Eggesbø M, Knight R, Peddada SD. Analysis of composition of microbiomes: a novel method for studying microbial composition. Microb Ecol Health Dis. (2015) 26:27663. doi: 10.3402/mehd.v26.27663
75. Jiang L, Amir A, Morton JT, Heller R, Arias-Castro E, Knight R. Discrete false-discovery rate improves identification of differentially abundant microbes. mSystems. (2017) 2:e00092–17. doi: 10.1128/mSystems.00092-17
76. Love MI, Huber W, Anders S. Moderated estimation of fold change and dispersion for RNA-seq data with DESeq2. Genome Biol. (2014) 15:550. doi: 10.1186/s13059-014-0550-8
77. Kurtz ZD, Müller CL, Miraldi ER, Littman DR, Blaser MJ, Bonneau RA. Sparse and compositionally robust inference of microbial eological networks. PLOS Comput Biol. (2015) 11:e1004226. doi: 10.1371/journal.pcbi.1004226
78. McMurdie PJ, Holmes S. phyloseq: An R ackage for reproducible interactive analysis and graphics of microbiome census data. PLoS ONE. (2013) 8:e61217. doi: 10.1371/journal.pone.0061217
79. Meinshausen N, Buhlmann P. High-dimensional graphs and variable selection with the Lasso. Ann Stat. (2006) 34:1436–62. doi: 10.1214/009053606000000281
80. Kurtz Z. Sparse inversE covariance estimation for ecological association and statistical inference: zdk123/SpiecEasi. (2019). Available online at: https://github.com/zdk123/SpiecEasi.
81. Shannon P, Markiel A, Ozier O, Baliga NS, Wang JT, Ramage D, et al. Cytoscape: A software environment for integrated models of biomolecular interaction networks. Genome Res. (2003) 13:2498–504. doi: 10.1101/gr.1239303
82. Assenov Y, Ramírez F, Schelhorn S-E, Lengauer T, Albrecht M. Computing topological parameters of biological networks. Bioinformatics. (2008) 24:282–4. doi: 10.1093/bioinformatics/btm554
83. Fierer N, Leff JW, Adams BJ, Nielsen UN, Bates ST, Lauber CL, et al. Cross-biome metagenomic analyses of soil microbial communities their functional attributes. Proc Natl Acad Sci - PNAS. (2012) 109:21390–5. doi: 10.1073/pnas.1215210110
84. Yurgel SN, Douglas GM, Dusault A, Percival D, Langille MGI. Dissecting Community Structure in Wild Blueberry Root and Soil Microbiome. Front Microbiol. (2018) 9:1187. doi: 10.3389/fmicb.2018.01187
85. Yoon J, Blumer A, Lee K. An algorithm for modularity analysis of directed and weighted biological networks based on edge-betweenness centrality. Bioinformatics. (2006) 22:3106–8. doi: 10.1093/bioinformatics/btl533
86. Ouédraogo E, Mando A, Zombr é NP. Use of compost to improve soil properties and crop productivity under low input agricultural system in West Africa. Agric. Ecosyst. Environ. (2001) 84:259–266. doi: 10.1016/S0167-8809(00)00246-2
87. Soumar, é M, Tack FMG, Verloo MG. Characterisation of Malian and Belgian solid waste composts with respect to fertility and suitability for land application. Waste Manag. (2003) 23:517–522. doi: 10.1016/S0956-053X(03)00067-9
88. Littman ML. An Improved Method for Detection of Urea Hydrolysis by Fungi. J Infect Dis. (1957) 101:51–61. doi: 10.1093/infdis/101.1.51
89. Lloyd AB, Sheaffe MJ. Urease activity in soils. Plant Soil. (1973) 39:71–80. doi: 10.1007/BF00018046
90. Allison FE. The fate of nitrogen applied to soils. Advances in Agronomy (Elsevier). (1966) 18:219–58. doi: 10.1016/S0065-2113(08)60651-3
91. Tognetti C, Mazzarino MJ, Laos F. Compost of municipal organic waste: Effects of different management practices on degradability and nutrient release capacity. Soil Biol Biochem. (2008) 40:2290–6. doi: 10.1016/j.soilbio.2008.05.006
92. Duong TTT, Verma SL, Penfold C, Marschner P. Nutrient release from composts into the surrounding soil. Geoderma. (2013) 195–6:42–7. doi: 10.1016/j.geoderma.2012.11.010
93. Glibert PM, Harrison J, Heil C, Seitzinger S. Escalating worldwide use of urea: a global change contributing to coastal eutrophication. Biogeochemistry. (2006) 77:441–63. doi: 10.1007/s10533-005-3070-5
94. Subbarao GV, Sahrawat KL, Nakahara K, Ishikawa T, Kishii M, Rao IM, et al. Biological nitrification inhibition—a novel strategy to regulate nitrification in agricultural systems. Adv Agron. (2012) 114:249–302. doi: 10.1016/B978-0-12-394275-3.00001-8
95. Antunes LP, Martins LF, Pereira RV, Thomas AM, Barbosa D, Lemos LN, et al. Microbial community structure and dynamics in thermophilic composting viewed through metagenomics and metatranscriptomics. Sci Rep. (2016) 6:38915. doi: 10.1038/srep38915
96. Saison C, Degrange V, Oliver R, Millard P, Commeaux C, Montange D, et al. Alteration and resilience of the soil microbial community following compost amendment: effects of compost level and compost-borne microbial community. Environ Microbiol. (2006) 8:247–57. doi: 10.1111/j.1462-2920.2005.00892.x
97. Zhang N, Wan S, Li L, Bi J, Zhao M, Ma K. Impacts of urea N addition on soil microbial community in a semi-arid temperate steppe in northern China. Plant Soil. (2008) 311:19–28. doi: 10.1007/s11104-008-9650-0
98. Broeckling CD, Broz AK, Bergelson J, Manter DK, Vivanco JM. Root exudates regulate soil fungal community composition and diversity. Appl Environ Microbiol. (2008) 74:738–44. doi: 10.1128/AEM.02188-07
99. Ellouze W, Esmaeili Taheri A, Bainard LD, Yang C, Bazghaleh N, Navarro-Borrell A, et al. Soil fungal resources in annual cropping systems and their potential for management. Biomed Res Int. (2014) 2014:1–15. doi: 10.1155/2014/531824
100. Treseder KK. Nitrogen additions and microbial biomass: a meta-analysis of ecosystem studies. Ecol Lett. (2008) 11:1111–20. doi: 10.1111/j.1461-0248.2008.01230.x
101. Jongen R, Hannula SE, De Long JR, Heinen R, Huberty M, Steinauer K, et al. Plant community legacy effects on nutrient cycling, fungal decomposer communities and decomposition in a temperate grassland. Soil Biol Biochem. (2021) 163:108450. doi: 10.1016/j.soilbio.2021.108450
102. Abawi GS, Widmer TL. Impact of soil health management practices on soilborne pathogens, nematodes and root diseases of vegetable crops. Appl Soil Ecol. (2000) 15:37–47. doi: 10.1016/S0929-1393(00)00070-6
103. Chaparro JM, Sheflin AM, Manter DK, Vivanco JM. Manipulating the soil microbiome to increase soil health and plant fertility. Biol Fertil Soils. (2012) 48:489–99. doi: 10.1007/s00374-012-0691-4
104. Daims H, Lebedeva EV, Pjevac P, Han P, Herbold C, Albertsen M, et al. Complete nitrification by Nitrospira bacteria. Nature. (2015) 528:504–9. doi: 10.1038/nature16461
105. Speirs LBM, Rice DTF, Petrovski S, Seviour RJ. The phylogeny, biodiversity, and ecology of the chloroflexi in activated sludge. Front Microbiol. (2019) 10:2015. doi: 10.3389/fmicb.2019.02015
106. Cooney DG, Emerson R. Thermophilic Fungi: An Account of Their Biology, Activities, and Classification. University of California, Los Angeles, United States (1964).
107. Howard DH. Pathogenic Fungi in Humans and Animals. Baton Rouge: CRC Press. (2002). doi: 10.1201/9780203909102
108. Déportes I, Benoit-Guyod J-L, Zmirou D. Hazard to man and the environment posed by the use of urban waste compost: a review. Sci Total Environ. (1995) 172:197–222. doi: 10.1016/0048-9697(95)04808-1
109. Noble R. Risks and benefits of soil amendment with composts in relation to plant pathogens. Australas plant Pathol. (2011) 40:157–67. doi: 10.1007/s13313-010-0025-7
110. Komnitsas K, Zaharaki D. Assessment of human and ecosystem risk due to agricultural waste compost application on soils: a review. Environ Forensics. (2014) 15:312–28. doi: 10.1080/15275922.2014.950775
111. Nadeem SM, Naveed M, Zahir ZA, Asghar HN. Plant-microbe interactions for sustainable agriculture: fundamentals and recent advances. In: Arora NK, editor. Plant Microbe Symbiosis - Fundamentals and Advances. New Delhi: Springer India (2013). p. 51–103. doi: 10.1007/978-81-322-1287-4_2
112. Komárek J. A polyphasic approach for the taxonomy of cyanobacteria: principles and applications. Eur J Phycol. (2016) 51:346–53. doi: 10.1080/09670262.2016.1163738
113. Mandyam KG, Jumpponen A. Mutualism-parasitism paradigm synthesized from results of root-endophyte models. Front Microbiol. (2014) 5:776. doi: 10.3389/fmicb.2014.00776
114. Gibson TE, Bashan A, Cao H-T, Weiss ST, Liu Y-Y. On the origins and control of community types in the human microbiome. PLoS Comput Biol. (2016) 12:e1004688. doi: 10.1371/journal.pcbi.1004688
Keywords: agriculture, tropical, soil, microbiome, network, Oxisol, Hawaii
Citation: Heisey S, Ryals R, Maaz TM and Nguyen NH (2022) A Single Application of Compost Can Leave Lasting Impacts on Soil Microbial Community Structure and Alter Cross-Domain Interaction Networks. Front. Soil Sci. 2:749212. doi: 10.3389/fsoil.2022.749212
Received: 29 July 2021; Accepted: 07 March 2022;
Published: 05 April 2022.
Edited by:
Nigel Victor Gale, University of Toronto, CanadaReviewed by:
German Andres Estrada-Bonilla, Colombian Corporation for Agricultural Research (AGROSAVIA), ColombiaJosé A. Siles, Spanish National Research Council (CSIC), Spain
Copyright © 2022 Heisey, Ryals, Maaz and Nguyen. This is an open-access article distributed under the terms of the Creative Commons Attribution License (CC BY). The use, distribution or reproduction in other forums is permitted, provided the original author(s) and the copyright owner(s) are credited and that the original publication in this journal is cited, in accordance with accepted academic practice. No use, distribution or reproduction is permitted which does not comply with these terms.
*Correspondence: Nhu H. Nguyen, bmh1Lm5ndXllbkBoYXdhaWkuZWR1