Sensitive Measures of Soil Health Reveal Carbon Stability Across a Management Intensity and Plant Biodiversity Gradient
- 1School of Environment and Natural Resources, The Ohio State University, Wooster, OH, United States
- 2W.K. Kellogg Biological Station, Michigan State University, Hickory Corners, MI, United States
- 3Department of Plant, Soil, and Microbial Sciences, East Lansing, MI, United States
Soil carbon (C) is a major driver of soil health, yet little is known regarding how sensitive measures of soil C shift temporally within a single growing season in response to short-term weather perturbations. Our study aimed to i) Examine how long-term management impacts soil C cycling and stability across a management intensity and plant biodiversity gradient and ii) Assess how sensitive soil health indicators change temporally over the course of a single growing season in response to recent weather patterns. Here we quantify a variety of sensitive soil C measures at four time points across the 2021 growing season at the W.K. Kellogg Biological Station’s Long Term Ecological Research Trial (LTER) located in southwest Michigan, USA. The eight systems sampled included four annual soybean (Glycine max) systems that ranged in management intensity (conventional, no-till, reduced input, and biologically-based), two perennial biofuel cropping systems (switchgrass (Panicum virgatum) and hybrid poplars (Populus nigra x P.maximowiczii)), and two unmanaged systems (early successional system and a mown but never tilled grassland). We found that unmanaged systems with increased perenniality enhanced mineralizable C (Min C) and permanganate oxidizable C (POXC) values. Additionally, all soil health indicators were found to be sensitive to changes in short-term weather perturbations over the course of the growing season. The implications of this study are threefold. First, this study assess indicators of labile and stable C pools over the course of the growing season and reflects the stability of soil C in different systems. Second, POXC, Min C, and ß-glucosidase (GLU) activity are sensitive soil health indicators that fluctuate temporally, which means that these soil health indicators could help elucidate the impact that weather patterns have on soil C dynamics. Lastly, for effective monitoring of soil C, sampling time and frequency should be considered for a comprehensive understanding of soil C cycling within a system.
Introduction
A primary motivation for enhanced soil carbon (C) is improved soil health, which is intimately connected with short-term outcomes like increased crop productivity and long-term outcomes such as soil fertility and C sequestration (1, 2). Soil health is the ability of the soil to provide essential services such as plant and animal health, water and air quality, and plant productivity (3). Soil C is the most important underlying property of soil health because it drives biological, chemical, and physical processes in the soil. For instance, greater soil C pools are associated with a greater abundance of bacteria and fungi (4–6). Soil C is also associated with aggregate stability and improved soil structure, which allows soils to retain more nutrients (7). Soil C accumulation is also vital for climate mitigation (8, 9). In order to reach short and long-term soil health outcomes, assessing the trajectory of soil C in a given system is essential.
Soil C is a large and dynamic pool with several moving components. To better understand the mechanisms that drive soil C decay and accumulation, it’s imperative to separate out pools that are functionally different from one another. The soil health framework is a conceptual model that is based on the collection of an array of biological, chemical, and physical soil health indicators that are then used to inform management decisions (10). The soil health framework provides several soil health indicators that reflect functionally different pools of C. For instance, mineralizable C (Min C) strongly reflects a more labile or active pool of C and is critical for nutrient mineralization and crop productivity (1, 11, 12). Additionally, ß-glucosidase (GLU) enzymes breaks down cellulose into glucose which can then be utilized by the microbial community (13). Therefore, GLU activity can indicate the rate of decay of organic material and the available C for microbial use. In contrast, permanganate oxidizable C (POXC) reflects a more processed pool of C and is more closely correlated with the total soil organic C pool (12, 14). Several studies have demonstrated that Min C and POXC reflect different pools of C (11, 15, 16). For instance, (17) reports that Min C is able to detect larger differences between perennial polycultures and annual row crops relative to POXC. This demonstrates that Min C may serve as an indicator of a more labile pool of C that represents C mineralization processes, whereas POXC may reflect a slower pool of C that takes a longer period of time to respond to management and reflects C stabilization processes. Residuals from a linear regression model of Min C and POXC can be used to visualize if a system trends more so towards C stabilization processes (POXC) or C mineralization processes (Min C) (11, 18).
Soil health indictors that reflect functionally different pools have been measured across a wide range of managed and unmanaged systems (15, 19, 20), yet we have a limited understanding of how Min C, POXC, and GLU respond to short-term changes in temperature and precipitation at the field scale. Previous laboratory-based experiments have widely demonstrated that labile and protected pools of C respond differently to changes in moisture and temperature (21–23). For instance, Cates etal. (23) found that low molecular weight C storage was impacted by temperature and moisture, while more protected pools of C were solely altered by moisture. Similarly, Benbi and Khosa (21) found that labile C pools were more sensitive to temperature relative to mineral associated organic matter. Currently, little is known regarding how these soil C pools function in relation to precipitation and temperature within a field setting, as most experiments have been conducted within a laboratory setting. Exploring how POXC, Min C, and GLU respond to seasonal temperature and precipitation changes, could provide further insight into their functionality and ability to reflect soil C stability.
Seasonal trends in POXC, Min C, and GLU have been reported in row-crop agriculture and generally demonstrate that these indicators are heavily influenced by plant growth and nutrient demand (1). For instance, Martin etal. (24) demonstrated that fine root production and decay in corn-based systems influence labile pools of C and N more so than processed pools of C. However, soil C cycling under contrasting management juxtaposed with intense climatic conditions is largely unknown at the field scale. Numerous studies have demonstrated that increases in soil organic matter contribute to yield stability in drought conditions (25–28). Additionally, Acosta-Martínez etal. (29) reported increased enzyme activity in agricultural soil when under drought conditions. However, many of the studies that report impacts of drought on soil C have been conducted in laboratory conditions or simulated within the field. Thus, more information is needed regarding how drought may naturally impact soil C pools and which mechanisms and kinetics lead to this stability in soil C over short and long-term time frames. Can soil health indicators help to uncover within season soil C dynamics that contribute to ecosystem resilience and long-term soil C accumulation?
Here we explore soil C dynamics over the course of a single growing season in systems that have undergone long-term management. The aim of this study is to i) Examine how long-term management impacts soil C cycling and stability across a management intensity and plant biodiversity gradient and ii) Assess how sensitive soil health indicators of soil C change temporally over the course of a single growing season in response to recent weather patterns. We hypothesize that systems with high diversity and reduced management intensity will have greater soil C stability over the course of a single growing season and exhibit greater C accumulation relative to systems dominated by annual row crops. Second, we hypothesize that soil health indicators reflecting labile C pools (i.e. Min C and GLU) will be more responsive to within season weather patterns relative to POXC, which will remain more stable throughout the growing season because it reflects a more processed pool of C.
Methods
Site Description
This study was conducted at the W.K. Kellogg Biological Station’s (KBS) Long-term Ecological Research site (LTER), located at 85° 24’W, 42° 24’ N. The LTER lies on the Kalamzoo and Oshtemo soil series. Both soil types are a mixed mesic Typic Hapludalf. The main difference between the two soil series is the thickness of the B/Bt horizon. Daily precipitation and temperature values were obtained from the KBS National Weather Service Station, located at 85° 23’W, 42° 24’ N at an elevation of 277.4 meters. The average annual temperature for months January-December is 9.74°C and the average annual precipitation for months January-December is 1005 mm a year (30).
Experimental Design
The KBS LTER Main Cropping System Experiment (MCSE) consists of four annual cropping systems, two perennial systems, and two unmanaged systems. The four annual cropping systems were established in 1989 and range in management, including a conventional, no-till, reduced-input, and biologically based system. The two perennial systems are switchgrass (Pancium virgatum) and poplar (Populus nigra x P.maximowiczii) which were established and sampled in 1989. The two unmanaged communities are an early successional community, which was abandoned from row-crop agriculture in 1989 and a mown grassland (never tilled) which was established on a cleared woodlot in 1959. The four annual cropping systems, two perennial systems, and the early successional community comprise the LTER Main Cropping System Experiment (MCSE). Systems are situated in a randomized complete block design, with 1 ha (90 x 110 m) plots that are each replicated in six blocks. The mown grassland is located 200 m to the south of the LTER main site with 15 x 30 m plots, replicated four times.
Management varied within each system and detailed information on timing can be found in Figure 1. The four annual systems move through a corn (Zea mays)-soybean (Glycine max)-wheat (Triticum aestivum) rotation. In 2021, the systems were in the soybean phase of the rotation and were planted with Roundup Ready soybeans at 67 kg ha-1. The conventional system was chisel plowed and received standard chemical inputs. The no-till system received the same standard chemical inputs as the conventional system but has been under permanent no-till conditions since trial establishment. In 2021, conventional systems were fertilized with 228 kg ha-1 of 28% UAN, 4 kg ha-1 of ammonium sulfate, 135 kg ha-1 of 0-46-0 phosphorus, and 168 kg ha-1 of 0-0-60 potassium. No-till systems were fertilized with 3.5 kg ha-1 of ammonium sulfate, 112.5 kg ha-1 of 0-46-0 phosphorus, and 168 kg ha-1 of potassium. Conventional system had 3.51 kg ha-1 of Warrant and 4.5 kg ha-1 of Roundup Power Max (48% glyphosate) applied. No-till systems had 7.5 kg ha-1 of Roundup Power Max (48% glyphosate), 4.68 kg ha-1 of 2,4-D Enlist One, and 1.17 kg ha-1 of Fierce MTZ applied. The reduced input systems are managed to reduce synthetic chemical inputs through the use of a cover crop and nitrogen fertilizer reductions. Specifically, the reduced input system receives 33% of the nitrogen fertilizer and chemical inputs that the conventional system and no-till systems receive. In 2021, the reduced in-put system was fertilized with 4 kg ha-1 of ammonium sulfate, 113 kg ha-1 of 0-46-0 phosphorus, and 147 kg ha-1 of 0-0-60 potassium. The reduced input system also had 2.25 kg ha-1 of Roundup Power Max (48% glyphosate). Additionally, the reduced input system is chisel plowed and has a winter cover crop of red clover (Trifolium pratense) or annual ryegrass (Lolium multiflorum) that is plowed under prior to planting during corn and soy years. The crop rotation cycle in the reduced input system is a corn-ryegrass-soybean-winter wheat-red clover rotation. The biologically based system is managed without the application of synthetic chemical inputs, this system is chisel plowed, under mechanical weed control, and has a corn-ryegrass-soybean-winter wheat-red clover rotation. Additionally, there is no manure or compost applied. For conventional, no-till, reduced input, and biologically based systems the average 2021 soybean yield was 4226, 4180, 4094, and 2005 kg ha-1.
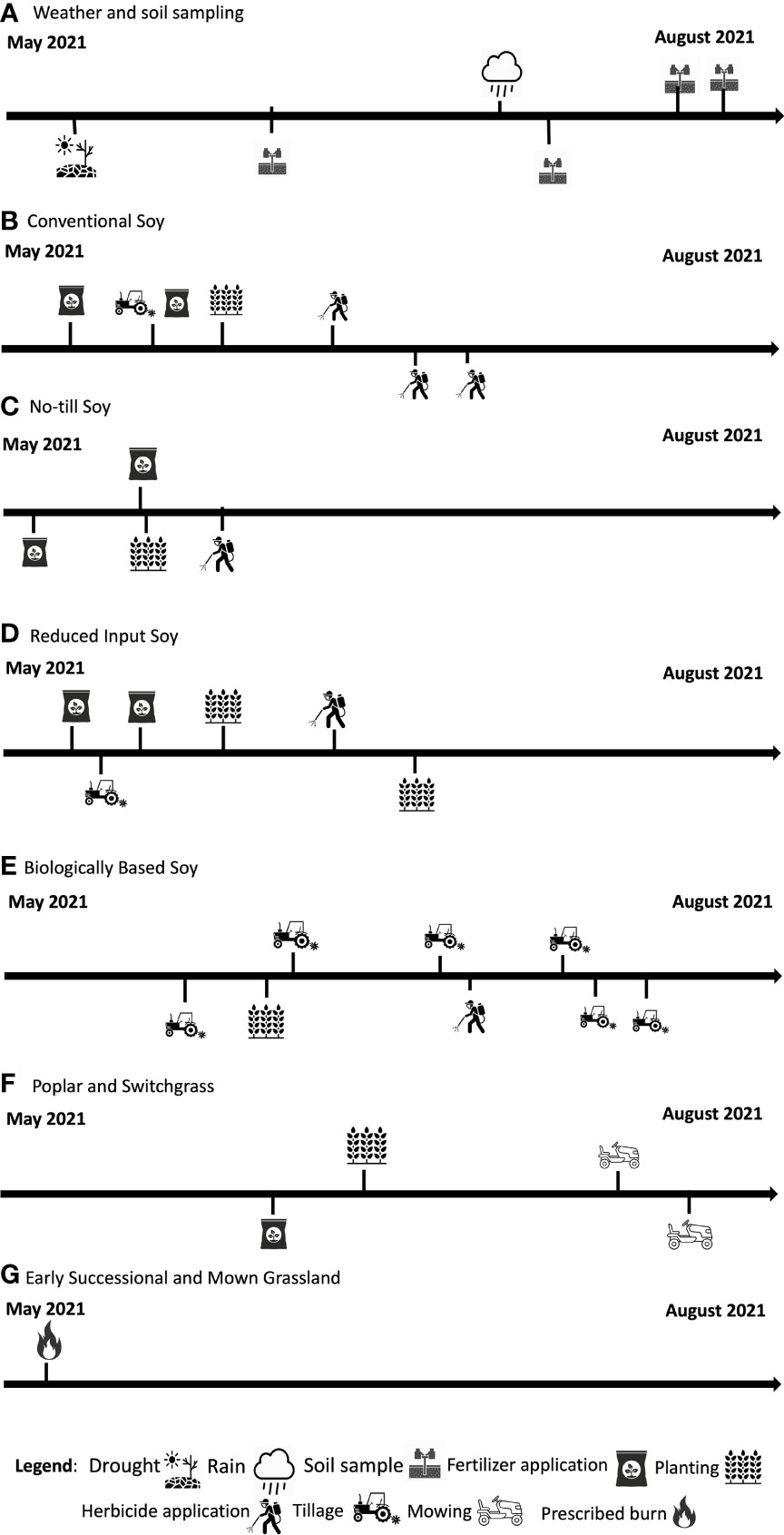
Figure 1 Management events over the course of the 2021 growing season across eight systems at the KBS LTER.
The switchgrass system is in a 5-year rotation with winter wheat as a 1-year break crop. This perennial system was planted with alfalfa from 1989-2019 and has since been moved to a switchgrass perennial system starting in 2019. Switchgrass was planted at 10 kg ha-1. In 2021, switchgrass was in its third year. Switchgrass was fertilized with 4 kg ha-1 28% UAN. The average aboveground biomass was 7367 kg ha-1 in 2021. The poplar system was established in 1989 and is planted with hybrid poplar trees that are harvested on a 10-year harvest cycle. The poplar system was last harvested in 2018, and a new crop was planted in 2019. Poplar yields in were 46543 kg ha-1in 2018.
The early successional community was historically tilled and abandoned in 1988, this system is unmanaged however the system is burned every spring to control for woody species. The mown grassland is predominantly unmanaged except for annual fall mowing to control for woody species.
Field Sampling
Soil sampling occurred four times over the course of the growing season within each system (Figure 1). Sampling time is defined as the point of the growing season in which samples are collected (i.e., May, June July, or August). System is defined as the management system that samples were taken from (i.e., Conventional soy, no-till soy, reduced input soy, biologically based soy, poplar, switchgrass, early successional, or mid-successional). During each sampling, soil cores were taken using a 1.9 cm diameter push probe down to a 10 cm depth. Soil cores were taken from five randomly chosen sampling stations within each system plot. Five soil cores were taken at each station. Ultimately, 25 cores were taken per each 1 ha plot and composited. Soils were then immediately processed for gravimetric soil moisture content (Table S1; 31).
Soil Health Indicators
Permanganate oxidizable C reflects a more processed C pool and was analyzed using methods adapted by Weil etal. (32) and Culman etal. (14). Soils were dried at 40°C, ground, and sieved to 2 mm prior to analysis. Potassium permanganate (0.02 M) (KMnO4) was added to soil (2.5 g), shaken (2 min), and then settled (10 min). Supernatant was removed and diluted to a 99:1 deionized water to supernatant ratio. Absorbance was measured in 96-well plate reader spectrophotometer at 550 nm.
Mineralizable C represents the soil C pool that is available to the microbial community within the soil. Mineralizable C was measured using methods adapted from Franzluebbers etal. (33) and Hurisso etal. (34). Prior to analysis soil was dried at 40°C, ground, and sieved to 2 mm. Soil (10 g) was rewetted to 50% water-holding capacity with deionized water and incubated (25°C) for 24 hrs. To measure the concentration of carbon dioxide headspace air (1 mL) was injected into a LI-820 infrared gas analyzer (LI-COR, Biosciences, Lincoln, NE).
The enzyme activity, ß-glucosidase (GLU), was determined using the fluorescence microplate enzyme assay (35). Soil was prepared by blending 2.75 g of soil and 91 mL of 50 mM acetate buffer. Soil was then poured into a glass bowl and placed on a stir plate where the soil was mixed on low. Standards of 0, 0.0005, 0.001, 0.002, 0.005, 0.01, and 0.02 µmols were prepared for each sample by combining 4-methylumbelliferone (MUB) (200 µL) and prepared soil (800 µL) in a well of 96-well plate. Soil (800 µL) and 4-MUB- ß-D-glucopyranoside substrate (200 µL) was combined in a separate 96-well plate. Standard and GLU enzyme assay plates were sealed, inverted ten times, and incubated at 35°C for 1.5 hrs. Plates were centrifuged for 2 min at 1500 rpm and 250 µL of solution from each well was pipetted into a black 96-well plate. Sodium hydroxide (0.5 M NaOH) was then added to each sample. Fluorescence was measured on an H1 synergy Biotek microplate reader at an excitation wavelength of 365 and emission wavelength of 450.
Statistical Analysis
An analysis of variance (ANOVA) was performed using the lme function from the {lem4} package (36) in R version 4.1.0, where system, sampling time, and the interaction between time and system were fixed effects and replicate was a random effect. Sampling time point was treated as a repeated measure to control for the variability of measurement within each timepoint. Normality of the data was determined via studentized residual plots with {MASS} in R (37). Mineralizable C was log transformed to obtain greater normality of the data. Tukey’s pairwise comparison means separation was obtained through the emmeans function using the {multcomp} package in R (38). Significant differences were determined at α=0.05.
To assess soil C stability in this study, we used a linear regression model adapted from Hurisso etal. (11). This model utilized Min C as a predictor variable and POXC as a response variable. Residuals were then extracted from the model output. Positive residuals depict systems trending towards POXC or C stabilization, whereas negative residuals indicate systems trending towards Min C or mineralization processes.
Multiple linear regressions were performed between independent variables of average temperature and precipitation and dependent variables of POXC, Min C, and GLU. For all models, systems were combined to solely test for effects of weather. For each sampling timepoint the average monthly precipitation and temperature was used as the independent variable. Multiple linear regressions were executed using the lm function in R (Supplemental Materials). Graphing was conducted using {ggplot2} in R (39).
Results
Weather
Daily average temperature and daily precipitation measurement readings were obtained from the KBS LTER weather station located at 42.408537, -85.373637. The 2021 cumulative precipitation and the 32-year average was calculated for, March 1-August 30, to encapsulate the sampling time points and key months during the growing season. Cumulative growing degree days (GDD) were calculated from the average daily temperature using a base of 10°C (40). The growing season in 2021 was characterized as abnormal compared to the 32-year average. From April through the end of June, cumulative precipitation was 57% lower in 2021 than the 32-year average (Figure 2A). Large rain events in July caused precipitation to be 8% greater from July to August in 2021 compared to the 32-year average (Figure 2A). Additionally, throughout the entire growing season average temperatures were 22% higher than the 32-year average, which resulted in a greater number of GDD over the course of the growing season (Figure 2B).
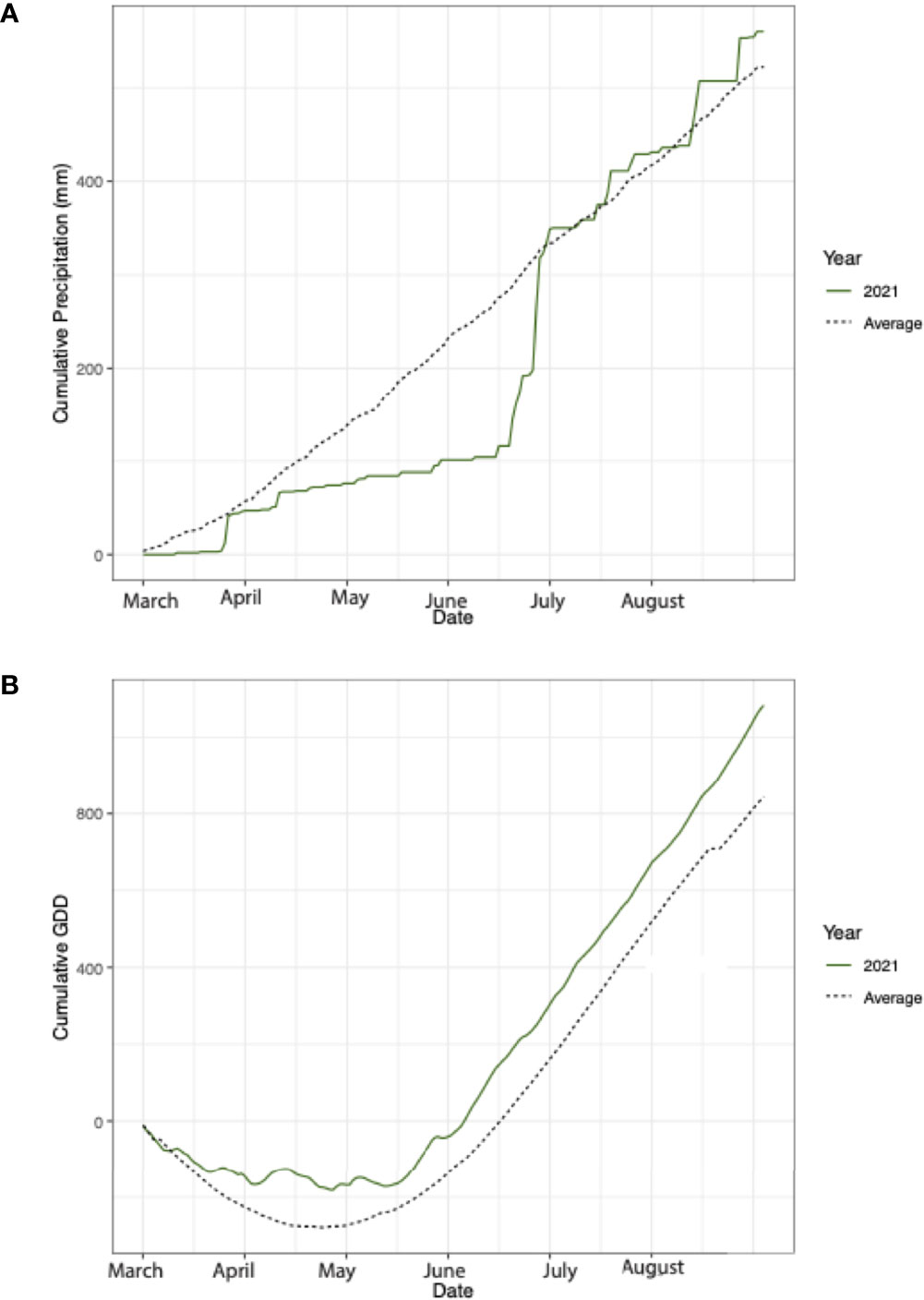
Figure 2 (A) Cumulative precipitation from March-August in 2021 (dashed) and the 32-year average (green). (B) Cumulative growing degree days from March-August in 2021 (dashed) and the 32-year average (green).
Mineralizable Carbon
System significantly affected Min C (p<0.05; Table 1), where differences in management intensity and perenniality had a strong influence. When averaged over the growing season Min C was 68% greater in unmanaged systems that consisted of a mown grassland and early successional community, compared to all other systems (Table S2; Figure 3). Additionally, perennial systems had 41% greater mineralizable C than conventional and no-till systems (Table S2; Figure 3). Biologically-based and reduced input soy systems were found to have comparable levels of Min C to those of perennial systems (Table S2; Figure 3).

Table 1 F-statistics and levels of significance of system, time, and the system x time interaction for mineralizable carbon (mg kg-1), ß-Glucosidase (umol g-1 h-1), permanganate oxidizable carbon (POXC), and residuals. ****p<0.00001 ***p<0.0001 **p<0.001 *p<0.05.
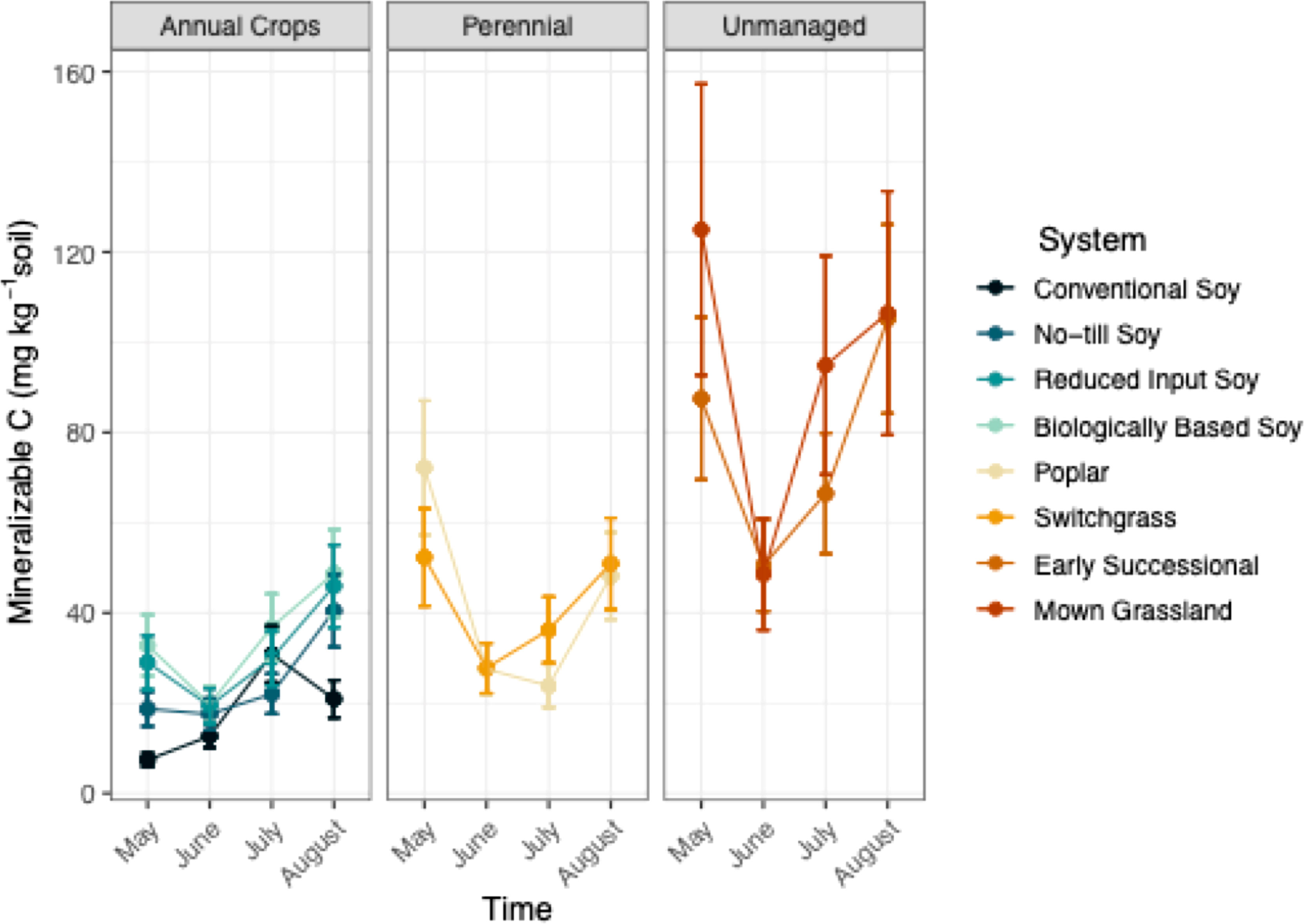
Figure 3 Mineralizable carbon (mg kg-1) from May-August within eight systems faceted by system type (annual crop, perennial, and unmanaged). Color represents a different system. Standard error bars represent one standard error of the mean (n=6).
Mineralizable C significantly changed over the course of a single growing season (p<0.05; Table 1). Overall, unmanaged and perennial systems appeared to experience more drastic shifts in Min C levels compared to annual systems (Figure 3). From May to June, Min C decreased two-fold, within the perennial and unmanaged systems (Figure 3). Likewise, Min C decreased in most annual systems between May and June but to a lesser extent (Figure3). After the sharp decline in Min C that occurred in June, measurements proceeded to increase in July and August within all systems except the conventional system (Figure 3).
β-Glucosidase
Sampling time point but not system was found to have a significant effect on GLU activity (p<0.05; Table 1; Table S2). Generally, GLU activity decreased two-fold over the growing season in unmanaged systems, whereas GLU activity in perennial and annual systems still decreased but to a lesser extent (Figure 4).
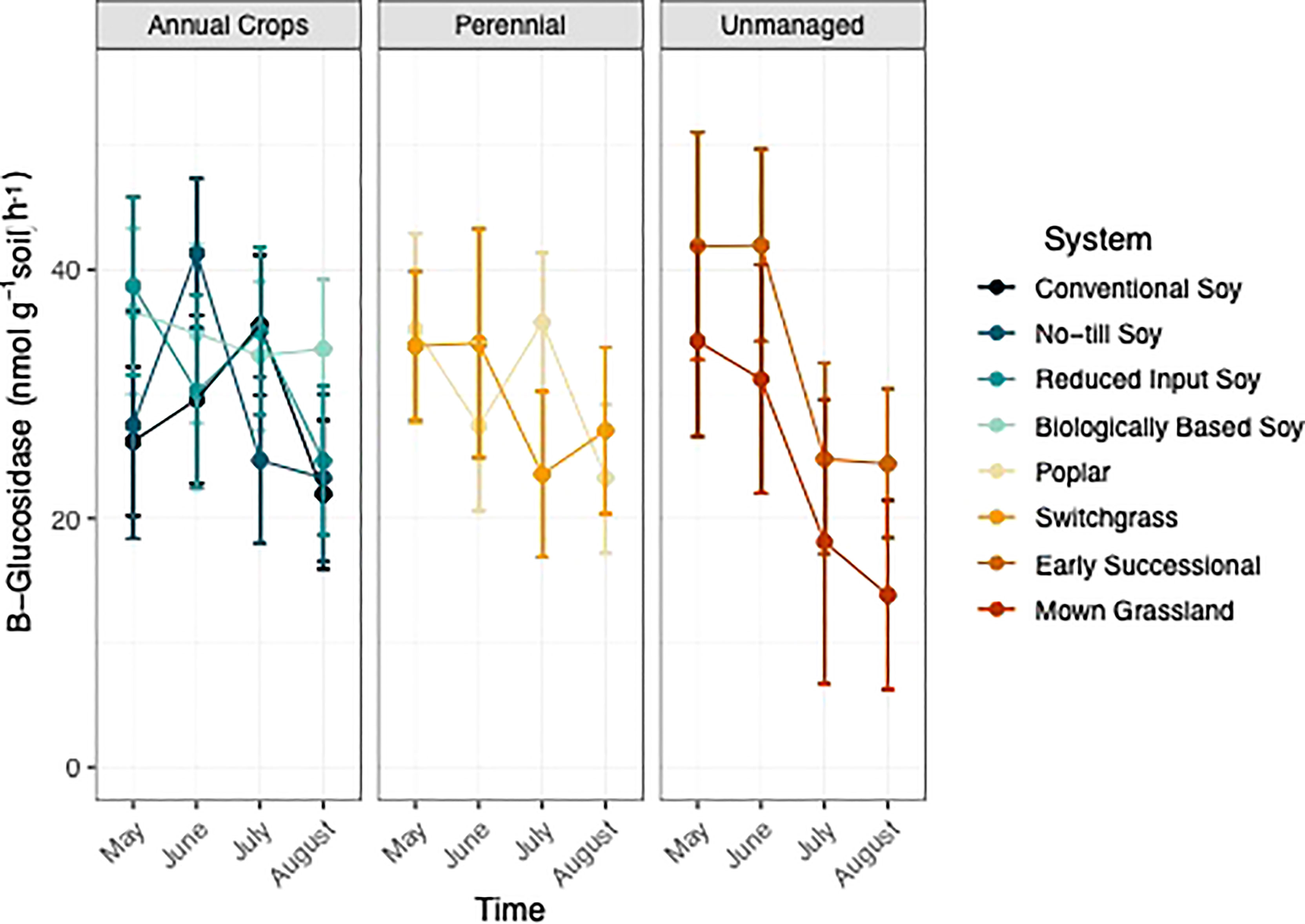
Figure 4 ß-glucosidase (nmol g-1 soil h-1) from May-August within eight systems faceted by system type (annual crop, perennial, and unmanaged). Color represents a different system. Standard error bars represent one standard error of the mean (n=6).
Permanganate Oxidizable Carbon
System had a significant effect on POXC (p<0.05; Table 1). When averaged across timepoint the unmanaged systems had 37% greater POXC than compared to all other systems, when averaged (Table S2). When averaged across timepoint, both perennial systems had 31% greater POXC compared to no-till and conventional systems, when averaged (Table S2). The reduced input and biologically based systems had similar levels of POXC compared to the perennial systems but significantly greater POXC levels relative to the conventional system.
Time had a significant effect on POXC (p<0.05; Table 1), with POXC levels fluctuating over the course of a single growing season (Figure 5). Permanganate oxidizable C decreased by two-fold by July within unmanaged, perennial, and reduced input systems (Figure 5). In addition, POXC levels also decreased over the growing season in biologically, no-till, and conventional systems but to a lesser degree (Figure 5).
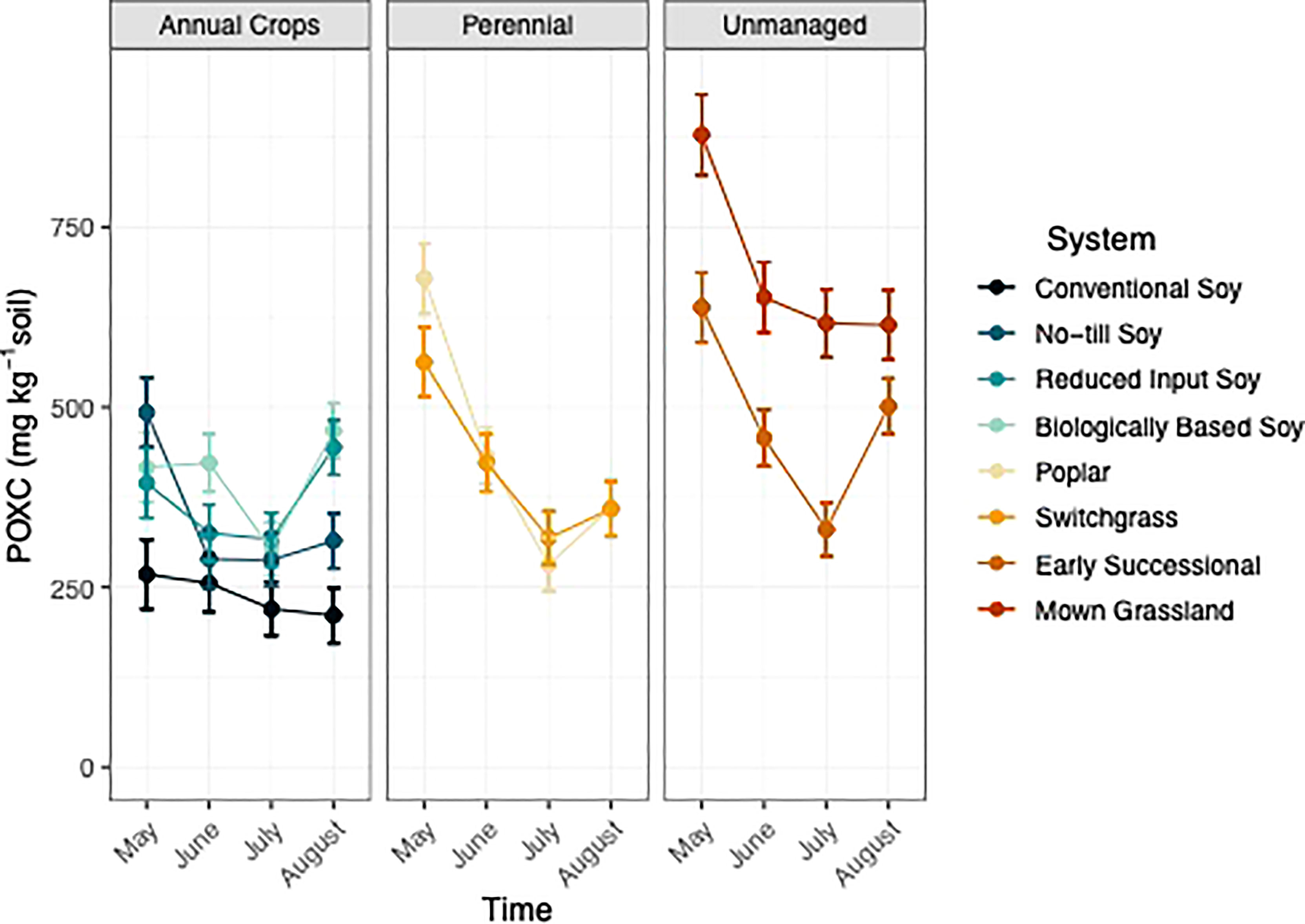
Figure 5 Permanganate oxidizable carbon mg kg-1) from May-August within eight systems faceted by system type (annual crop, perennial, and unmanaged). Color represents a different system. Standard error bars represent one standard error of the mean (n=6).
Residuals
In general, systems with greater perenniality and reduced management intensity were found to trend towards C storage, whereas the annual dominated systems trended towards C mineralization (Figure 6). When averaged over the growing season mown grasslands, switchgrass, poplar, and biologically based systems had positive residuals that indicated C storage (Table S2). In contrast, the conventional, no-till, early successional, and reduced input systems had negative residuals indicating that these systems trended towards C mineralization. However, averages over the growing season may have been biased given the broad range of fluctuation of the residuals within a single growing season.
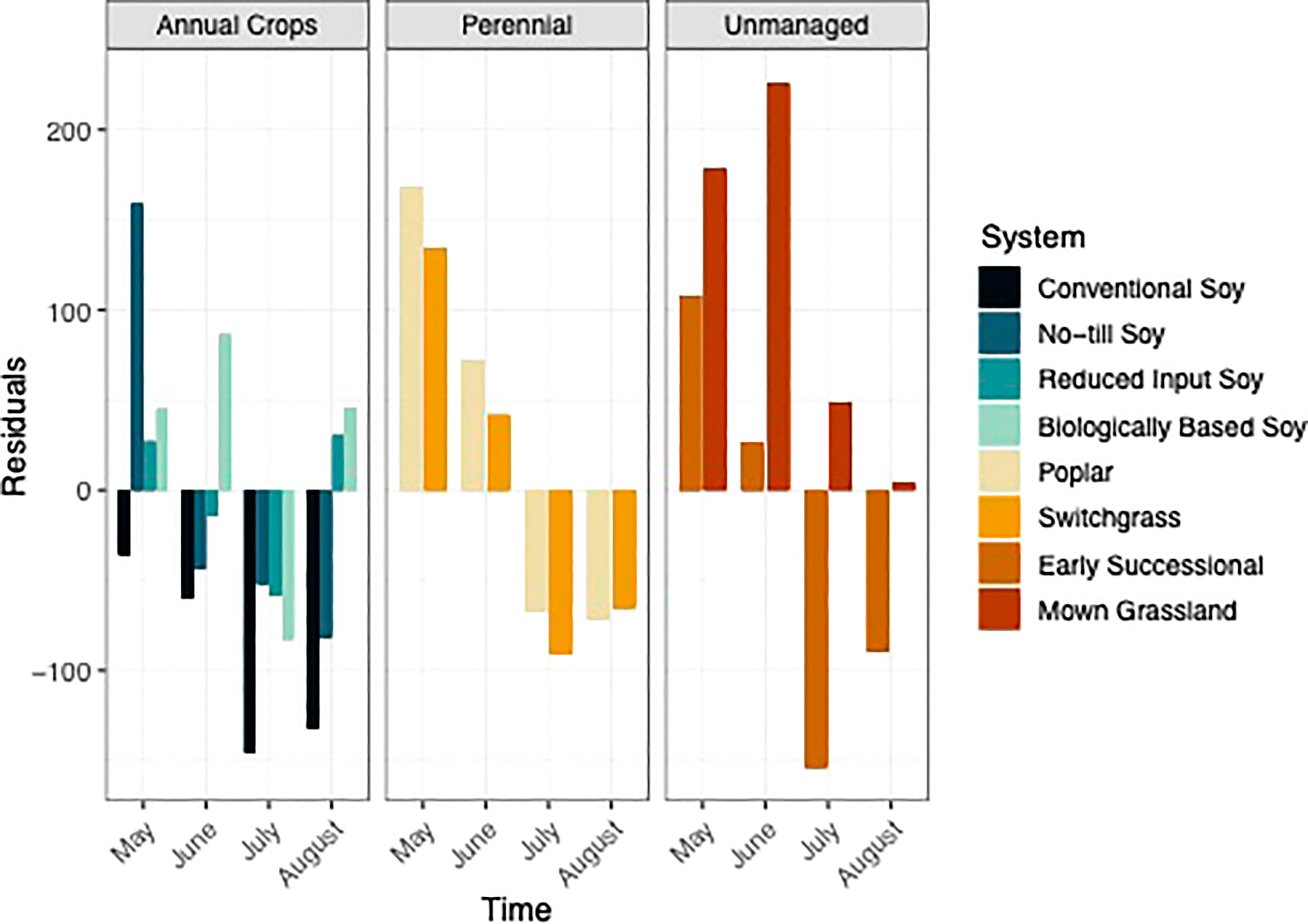
Figure 6 Visualization of residuals from May-August within eight systems faceted by system type (annual crop, perennial, and unmanaged). Color represents a different system.
Additionally, the extent to which systems mineralized versus stabilized C, shifted over the course of the growing season. All unmanaged and perennial systems were found to trend towards C stabilization in May and June (Figure 6). In the second half of the growing season however, both perennial systems as well as the early successional system trended towards C mineralization (Figure 6). The mown grassland system was the only system that trended towards C stabilization over the entire growing season (Figure 6). The annual cropping systems all trended towards C stabilization in May, except for the conventional system (Figure 6). However, over the rest of the growing season, most annual systems trended towards C mineralization (Fig 6).
Effect of Climate on Carbon Cycling
A series of multiple linear regressions were conducted to investigate the effect that precipitation and temperature had on the various soil health indicators. Through taking the monthly averages of precipitation and temperature that corresponded to each sampling period we were able to account for changes in precipitation and temperature over the growing season. The Min C model found that precipitation had a significant effect on Min C, while temperature did not. In general, we found that increased precipitation decreased Min C (ß= -0.12; Table 2). The GLU model indicated that both temperature and precipitation had a significant effect on GLU activity (Table 2). Specifically, increased temperature was found to decrease GLU activity (ß= -1.39) and increased precipitation was found to increase GLU activity (ß=0.27). Moreover, temperature appeared to have a greater effect on GLU activity. The POXC model found that both temperature (ß=0.27) and precipitation (ß=-22.17) had a significant effect on POXC, where precipitation had a greater effect on POXC relative to temperature (Table 2).

Table 2 Multiple linear regression models between independent variables (Mineralizable C, ß-Glucosidase, POXC) and dependent variables (temperature and precipitation). For this specific analysis, temperature and precipitation effects were performed on all eight systems combined.
Discussion
Reduced Management Intensity Fosters C Stabilization Across Systems
Our results indicate that systems with greater perenniality and diversity can enhance C cycling and C stability. Overall, unmanaged systems had consistently greater Min C and POXC, which supported our first hypothesis that systems under reduced management intensity would have greater C stability and cycling. Most noteworthy, is that the monoculture perennial systems had similar Min C and POXC values to those of the annual row crop systems. These results are like others who have reported that it is plant perennial diversity that may cause greater accumulation of labile and stabile C pools rather than just perenniality (41–43). It is also important to note that the aboveground biomass of all annual and perennial switchgrass systems were harvested, this removal of biomass may have also contributed to reduced soil C pools in these systems as less organic matter was available for assimilation into C pools. The addition of nitrogen (N) fertilizer to the soil within conventional and switchgrass systems may have also contributed to reduced soil C pools due to potentially increasing soil organic mineralization (44, 45). However, it is more likely that the contribution of belowground biomass from extensive and diverse root systems had the largest effect on enhancing soil C accumulation across these systems (46–51). For instance, Rasse etal. (47) reports that the mean residence time of C derived from roots is 2.4 times greater than C derived from shoots, due to increased recalcitrance and the protection of C by root hairs and mycorrhizae in aggregates. Diverse perennial systems have also been found to have increased aggregate stability due to lack of mechanical disturbance (52). Enhanced aggregate stability can lead to greater macroaggregates and physically protect processed pools of soil C, which can result in increased C stabilization and accumulation (53, 54).
Differences in root production between varying systems may have had a significant impact on soil C pools. For example, switchgrass systems have been found to increase soil C stocks when compared to soybean systems because of their deep root systems that have higher C/N ratios (55). Soybean systems have shallow roots with low C/N ratios and low biomass, which adds less organic matter to soil systems (56). Additionally, the low C/N ratios may cause increased C mineralization rather than C stabilization (55). Moreover, within early successional and mid-successional systems root biomass comprises a majority of plant standing biomass (57), where the decomposition of root biomass has been shown to increased microbial activity and the assimilation of organic matter into soil C pools (58). Moreover, plant diversity can also accelerate root production due to plant complementarity from legumes (59). To our knowledge, root production has never been measured within this experiment. That said, multiple studies have demonstrated that perennial cropping systems produce significantly more root biomass compared to annuals crops (60, 61) and this often leads to greater C pools within perennial systems (12, 43, 62, 63).
Soil C trends across the management intensity gradient were assessed through the calculation of residuals, where positive trends indicate C stabilization and negative trends indicate C mineralization (11, 18). The conventional system had the largest negative residuals across all systems, which indicates that these systems trend towards C mineralization. Mown grassland systems had the greatest positive residuals when compared to all other systems. Surprisingly, early successional communities substantially differed from mown grasslands as early successional systems trended towards C mineralization. Our results indicate that early successional systems may foster a greater labile C pool, but more processed C pools may be suppressed. Likewise, Sprunger and Robertson (43) also indicate that early successional systems have similar slow C pools to those of annual systems. Moreover, the mown grassland has never been disturbed and fosters a diverse perennial community whereas the early successional system is dominated by annual plant species, which likely reduces fine root productivity and ultimately C accumulation (59). Additionally the early successional system was managed intensely until 1989. Ward etal. (64) reported that legacy effects of previous management intensity can have a substantial effect on soil C inventories. Our results indicate that both plant community composition as well as past management disturbance likely influence long-term C stabilization.
Our results also indicate that long-term management rather than within season management perturbations influenced labile and more processed C pools, given that soil C indicators were not altered after planting and tillage events took place. For instance, all three soil C indicators within the biologically based system remained relatively static over the course of the growing season and did not decrease, even following frequent mechanical disturbance (Figure 1). These results are consistent with others that have found larger C pools in the biologically based systems relative to the conventional system (62, 65, 66). The greater C levels despite frequent disturbance may be due to continuous C inputs from the cover crops included in the rotation (62). Like our study, Culman etal. (1) reports that long-term rotational diversity and cover cropping rather than within season management perturbations had a substantial effect on soil C pools over the course of a growing season. It was also surprising that GLU was not responsive to system. The lack of differences between GLU in system varying in management intensity may have been caused by the effect of weather perturbation from drought confounding system effects (67). Additionally, GLU activity is highly variable because it reflects the microbial community decomposition of cellulose in a specific moment in time. This variability brings into question whether GLU activity is reflecting C decay based on long-term management or on the rapid changes of the microbial community that are consistently taking place within all systems.
Short-Term Weather Alters Soil C Pools and Stability Over a Single Growing Season
The sensitive soil health indicators measured in this study were clearly influenced by weather patterns over the course of the growing season. Our results indicate that measures of labile and more processed C pools were significantly impacted by both precipitation and temperature partially disproving our hypothesis that only Min C would demonstrate temporal variability. Our results indicated that measures of the labile C pool were sensitive to precipitation but not temperature, whereas measures of C decay and processed pools of C were impacted by both precipitation and temperature. Given that moisture and a minimum temperature is essential for aggregate formation, it is not surprising that both precipitation and temperature would affect processed C pools, as increased aggregation can lead to the protection of processed C pools (23, 68–70). Precipitation has been found to alter labile C pools, reduced precipitation shrinks soil water films and increases the soils ionic strength to C, which results in a decrease of labile C (71, 72). Temperature may have affected POXC and GLU as increased temperature has been found to enhance microbial activity and the decomposition of complex C (73, 74). These results differ from others who report that labile C pools are impacted by temperature and moisture, and stable C pools are affected by only moisture (21, 23, 75). Our results may differ from others simply because precipitation swings during the 2021 growing season may have overwhelmed temperature effects (23, 75). That being said, within season fluctuations in soil C pools may have also been impacted by short-term management perturbations and not just weather events. Our findings demonstrate that more research is needed to understand how labile and stable C pools function in response to temperature, precipitation, and short-term management perturbations at the field scale.
Seasonal temperature and precipitation changes were also found to alter soil C stability. Drought periods within a growing season were found to substantially decrease both labile and more processed C pools. However, re-wetting periods after drought were found to increase Min C pools, while POXC remained reduced. Thus, it was not surprising that systems trended towards mineralization processes at the end of the growing season. These results are like others that have found that wetting events that follow droughts result in rapid respiration due to the rewetting of destabilized C from drought events (76–78). These rewetting events then transport soluble C via soil water, which changes the bioavailability of the C and potential for decomposition (71, 79). Increased temperatures may have also played a role in prompting mineralization processes within most systems, as warmer temperatures have been reported to increase the microbial respiration costs associated with maintaining enzyme production and biomass (80). In addition to changes in weather, plant nutrient demand (81) as well as increased root turnover (59) may have caused increases in the labile C pool at the end of the growing season.
The extent to which soil C cycling fluctuated over the course of a growing season in response to weather events differed across the eight systems. While all systems were impacted by weather perturbations, unmanaged systems always maintained greater POXC and Min C levels over the course of the growing season than compared to perennial and annual systems. Moreover, the mown grassland system always trended towards C stabilization, even when experiencing large precipitation swings. These results indicate that increased plant diversity and reduced disturbance are key in enhancing mechanisms that foster soil C stability, including belowground C transfer between functionally different plants and aggregation (82, 83). Such rhizosphere interactions are critical to stabilizing C and could explain why more diverse systems are resilient to weather perturbations. Surprisingly, perennial monoculture systems had similar Min C and POXC values as annual systems over the growing season and trended towards C mineralization. This finding is like Syswerda etal. (62) and indicates that although perennial systems may have greater C input through root biomass (46), most of this C is in a labile form that is susceptible to loss (61). Changes in weather over the growing season appeared to have a minimal effect on soil C pools in the conventional system. One may speculate that because the conventional system is consistently losing soil C over time (Cordova et al., under review), weather disturbances have minimal effects on soil C pools within a single growing season.
Given that soil health indicators were only measured over the course of a single growing season our study is limited in the extent to which we can infer how soil health indicators may shift in the absence of roots and dormant perennial roots. The measurement of soil health indicators between growing seasons is important as the presence of cover crop or dormant perennial roots can stimulate the microbial community and enhance nutrient cycling (43). Additionally, the crop that is currently growing in rotation may have influenced the soil health indicators, more studies that measure soil C indicators over the course of an entire crop rotation could be useful to comprehensively understand how soil C pools are shifting. Conclusions on the extent in which weather can alter soil C indicators are limited in this study, given that only one long-term field site in southwest Michigan was used. A study that uses multiple field sites in regions that vary distinctly in weather shifts could be compared to determine assess weather pattern effects on soil C more concretely. In addition, our model was limited in its ability to account for the effect of management in combination with weather on soil C measures within a single growing season.
Implications for Future Soil Health Sampling
The soil health community has made significant advancements in recent years by identifying indicators that are sensitive to recent changes in management (84). These sensitive indicators are critical for informing agronomic performance and assessing C sequestration potential (1, 18). Furthermore, as C markets continue to expand, it will be critical to find indicators that accurately reflect C stabilization within agroecosystems (85). This study demonstrates that soil health indicators are not only sensitive to long-term management but clearly respond to weather perturbations as well. This sensitivity to extended periods of drought and large precipitation events has practical implications for soil health sampling and interpretation. While it has been noted that soil biological health indicators are susceptible to temporal variation over a single growing season (86, 87), the conversation is rarely extended to how this variability might impact soil health testing, interpretation, and recommendations to farmers. Based on our results, we recommend that soil health testing should occur at the same time every year. Moreover, given that weather patterns can strongly dictate these sensitive measures of soil C, it may be beneficial to increase the frequency of soil sampling and take multiple samples within a growing season to comprehensively understand how within season perturbations may be impacting C stabilization, nutrient availability, and overall soil health.
Conclusion
Our study found that diverse perennial systems under long-term management can enhance both labile and more processed C pools. Moreover, systems with greater perennial diversity were able to consistently trend towards C stabilization over the course of a single growing season. In addition, POXC and Min C were both found to fluctuate over the course of the growing season and were affected by major precipitation events and temperature. Our study indicates that 1) long-term management can substantially affect C cycling and stability 2) Increased perenniality combined with greater plant diversity can foster soil C pools that are resilient to extreme variability in temperature and precipitation and 3) Soil health testing should take place at the same time every year given that sensitive indicators can heavily vary over the course of a growing season. Data Availability Statement
The raw data supporting the conclusions of this article will be made available by the authors, without undue reservation.
Author Contributions
CS and TM both worked on conceptualizing study, both worked on data analysis, and both split writing duties evenly. All authors contributed to the article and approved the submitted version.
Funding
Financial support for this research was provided by the Great Lakes Bioenergy Research Center, Office of Science, Office of Biological and Environmental Research (Award DESC0018409), by the National Science Foundation Long-term Ecological Research Program (DEB 1832042) at the Kellogg Biological Station, and by Michigan State University AgBioResearch.
Conflict of Interest
The authors declare that the research was conducted in the absence of any commercial or financial relationships that could be construed as a potential conflict of interest.
Publisher’s Note
All claims expressed in this article are solely those of the authors and do not necessarily represent those of their affiliated organizations, or those of the publisher, the editors and the reviewers. Any product that may be evaluated in this article, or claim that may be made by its manufacturer, is not guaranteed or endorsed by the publisher.
Acknowledgments
We would like to thank the Kellogg Biological Station Long-term Ecological Site for their support and assistance while sampling. Additionally, we would like to thank Ainsley Lightcap, Jacob Murray, and Meredith Mann for their assistance with laboratory work.
Supplementary Material
The Supplementary Material for this article can be found online at: https://www.frontiersin.org/articles/10.3389/fsoil.2022.917885/full#supplementary-material
References
1. Culman SW, Snapp SS, Green JM, Gentry LE. Short- and Long-Term Labile Soil Carbon and Nitrogen Dynamics Reflect Management and Predict Corn Agronomic Performance. J Agron (2013) 105:493–502. doi: 10.2134/agronj2012.0382
2. Oldfield EE, Bradford MA, Wood SA. Global Meta-Analysis of the Relationship Between Soil Organic Matter and Crop Yields. SOIL (2019) 5:15–32. doi: 10.5194/soil-5-15-2019
3. Doran JW, Zeiss MR. Soil Health and Sustainability: Managing the Biotic Component of Soil Quality. Appl Soil Ecol (2000) 15:3–11. doi: 10.1016/S0929-1393(00)00067-6
4. Bastida F, Kandeler E, Moreno JL, Ros M, Garcıa C. Application of Fresh and Composted Organic Wastes Modifies Structure, Size and Activity of Soil Microbial Community Under Semiarid Climate. Appl Soil Ecol (2008) 12:318–329. doi: 10.1016/j.geoderma.2008.08.007
5. Zhang X, Li Q, Zhu A, Liang W, Zhang J, Steinberger Y. Effects of Tillage and Residue Management on Soil Nematode Communities in North China. Ecol Indic. (2012) 13:75–81. doi: 10.1016/j.ecolind.2011.05.009
6. Tiemann LK, Grandy AS, Atkinson EE, Marin-Spiotta E, McDaniel MD. Crop Rotational Diversity Enhances Belowground Communities and Functions in an Agroecosystem. Ecol. (2015) 18:761–71. doi: 10.1111/ele.12453
7. Al-Kaisi MM, Douelle A, Kwaw-Mensah D. Soil Microaggregate and Macroaggregate Decay Over Time and Soil Carbon Change as Influenced by Different Tillage Systems. J Soil Water Conserv (2014) 69:574–80. doi: 10.2489/jswc.69.6.574
8. Lal R. Soil Carbon Sequestration Impacts on Global Climate Change and Food Security. Science (2004) 304:1623–7. doi: 10.1126/science.1097396
9. Longbottom T, Wahab L, Min K, Jurusik A, Moreland K, Dolui M, et al. What’s Soil Got to Do With Climate Change? GSAT. (2022) 32:4–10. doi: 10.1130/GSATG519A.1
10. Harris RF, Karlen DL, Mulla DJ. A Conceptual Framework for Assessment and Management of Soil Quality and Health. Methods for Assessing Soil Quality (John Wiley and Sons, Ltd) (1997) 61–82. doi: 10.2136/sssaspecpub49.c4
11. Hurisso TT, Culman SW, Horwath WR, Wade J, Cass D, Beniston JW, et al. Comparison of Permanganate-Oxidizable Carbon and Mineralizable Carbon for Assessment of Organic Matter Stabilization and Mineralization. Soil Sci Soc Am J (2016) 80:1352–64. doi: 10.2136/sssaj2016.04.0106
12. Sprunger CD, Culman SW, Peralta AL, DuPont ST, Lennon JT, Snapp SS. Perennial Grain Crop Roots and Nitrogen Management Shape Soil Food Webs and Soil Carbon Dynamics. Soil Biol Biochem (2019) 137:107573. doi: 10.1016/j.soilbio.2019.107573
13. Tabatabai MA. Soil Enzymes. In: Methods of Soil Analysis. Madison, WI: John Wiley & Sons, Ltd (1994). p. 775–833.
14. Culman SW, Snapp SS, Freeman MA, Schipanski ME, Beniston J, Lal R, et al. Permanganate Oxidizable Carbon Reflects a Processed Soil Fraction That is Sensitive to Management. Soil Sci Soc Am J (2012) 76:494–504. doi: 10.2136/sssaj2011.0286
15. Awale R, Emeson MA, Machado S. Soil Organic Carbon Pools as Early Indicators for Soil Organic Matter Stock Changes Under Different Tillage Practices in Inland Pacific Northwest. Front Ecol Evol (2017) 5:96. doi: 10.3389/fevo.2017.00096
16. Fine AK, van Es HM, Schindelbeck RR. Statistics, Scoring Functions, and Regional Analysis of a Comprehensive Soil Health Database. Soil Sci Soc Am J (2017) 81:589–601. doi: 10.2136/sssaj2016.09.0286
17. Sprunger CD, Martin T, Mann M. ). Systems With Greater Perenniality and Crop Diversity Enhance Soil Biological Health. Agric Enviorn Lett (2020) 5:e20030. doi: 10.1002/ael2.20030
18. Wade J, Maltais-Landry G, Lucas DE, Bongiorno G, Bowles TM, Calderón FJ, et al. Assessing the Sensitivity and Repeatability of Permanganate Oxidizable Carbon as a Soil Health Metric: An Interlab Comparison Across Soils. Geoderma (2020) 366:114235. doi: 10.1016/j.geoderma.2020.114235
19. DuPont ST, Culman SW, Ferris H, Buckley DH, Glover JD. No-Tillage Conversion of Harvested Perennial Grassland to Annual Cropland Reduces Root Biomass, Decreases Active Carbon Stocks, and Impacts Soil Biota. Agric Ecosyst Environ (2010) 137:25–32. doi: 10.1016/j.agee.2009.12.021
20. Sprunger CD, Culman SW, Deiss L, Brock C, Jackson-Smith D. Which Management Practices Influence Soil Health in Midwest Organic Corn Systems? J Agron (2021) 113:4201–19. doi: 10.1002/agj2.20786
21. Benbi DK, Khosa MK. Effects of Temperature, Moisture, and Chemical Composition of Organic Substrates on C Mineralization in Soils. CSOSA (2014) 45:2734–53. doi: 10.1080/00103624.2014.950423
22. Karhu K, Hilasvuori E, Järvenpää M, Arppe L, Christensen BT, Fritze H, et al. Similar Temperature Sensitivity of Soil Mineral-Associated Organic Carbon Regardless of Age. Soil Biol Biochem (2019) 136:107527. doi: 10.1016/j.soilbio.2019.107527
23. Cates AM, Jilling A, Tfaily MM, Jackson RD. Temperature and Moisture Alter Organic Matter Composition Across Soil Fractions. Geoderma (2022) 409:115628. doi: 10.1016/j.geoderma.2021.115628
24. Martin T, Culman S, Sprunger CD. Quality or Quantity? Determining the Impact of Fine Root Traits on Soil Health in Row Crop Agriculture. J Soil Sci Plant Nutr (2022) 22:2322–2333. doi: 10.1007/s42729-022-00811-1
25. Gaudin ACM, Tolhurst TN, Ker AP, Janovicek K, Tortora C, Martin RC, et al. Increasing Crop Diversity Mitigates Weather Variations and Improves Yield Stability. PloS One (2015) 10:e0113261. doi: 10.1371/journal.pone.0113261
26. Bowles TM, Mooshammer M, Socolar Y, Calderón F, Cavigelli MA, Culman SW, et al. Long-Term Evidence Shows That Crop-Rotation Diversification Increases Agricultural Resilience to Adverse Growing Conditions in North America. One Earth (2020) 2:284–93. doi: 10.1016/j.oneear.2020.02.007
27. Kane DA, Bradford MA, Fuller E, Oldfield EE, Wood SA. Soil Organic Matter Protects US Maize Yields and Lowers Crop Insurance Payouts Under Drought. Environ Res Lett (2021) 16:044018. doi: 10.1088/1748-9326/abe492
28. Nouri A, Yoder DC, Raji M, Ceylan S, Jagadamma S, Lee J, et al. Conservation Agriculture Increases the Soil Resilience and Cotton Yield Stability in Climate Extremes of the Southeast US. Commun Earth Environ (2021) 2:1–12. doi: 10.1038/s43247-021-00223-6
29. Acosta-Martínez V, Cotton J, Gardner T, Moore-Kucera J, Zak J, Wester D, et al. Predominant Bacterial and Fungal Assemblages in Agricultural Soils During a Record Drought/Heat Wave and Linkages to Enzyme Activities of Biogeochemical Cycling. Appl Soil Ecol (2014) 84:69–82. doi: 10.1016/j.apsoil.2014.06.005
30. Robertson GP, Hamilton SK. Long-Term Ecological Research in Agricultural Landscapes at the Kellogg Biological Station LTER Site: Conceptual and Experimental Framework. In: Hamilton SK, Doll JE, Robertson GP, editors. The Ecology of Agricultural Landscapes: Long-Term Research on the Path to Sustainability. New York, NY: Oxford University Press (2015). p. 1–32.
31. Reynolds SG. The Gravimetric Method of Soil Moisture Determination Part I A Study of Equipment, and Methodological Problems. J Hydrol. (1970) 11:258–73. doi: 10.1016/0022-1694(70)90066-1
32. Weil RR, Islam IR, Stine MA, Gruver JB, Samson-liebig SE. Estimating Active Carbon for Soil Quality Assessment: A Simplified Method for Laboratory and Field Use. Am J Altern Agric (2003) 18:3–17. doi: 10.1079/AJAA200228
33. Franzluebbers AJ, Wright SF, Stuedemann JA. Soil Aggregation and Glomalin Under Pastures in the Southern Piedmont USA. Soil Sci Soc Am J (2000) 64:1018–26. doi: 10.2136/sssaj2000.6431018x
34. Hurisso TT, Moebius-Clune DJ, Culman SW, Moebius-Clune BN, Thies JE, van Es HM. Soil Protein as a Rapid Soil Health Indicator of Potentially Available Organic Nitrogen. Agric Environ Lett (2018) 3:180006er. doi: 10.2134/ael2018.02.0006er
35. Bell CW, Fricks BE, Rocca JD, Steinweg JM, McMahon SK, Wallenstein MD. High-Throughput Fluorometric Measurement of Potential Soil Extracellular Enzyme Activities. J Vis Exp (2013) 81:50961. doi: 10.3791/50961
36. Bates D, Mächler M, Bolker B, Walker S. Fitting Linear Mixed-Effects Models Using Lme4. J Stat Softw (2015) 67(1):1–48. doi: 10.18637/jss
38. Hothorn T, Bretz F, Westfall P. Simultaneous Inference in General Parametric Models. J Biomet. (2008) 50:356–63. doi: 10.1002/bimj.200810425
39. Wickham H. Ggplot2: Elegant Graphics for Data Analysis. New York, New York: Springer-Verlag New York (2016). Available at: https://ggplot2.tidyverse.org.
40. Baskerville GL, Emin P. Rapid Estimation of Heat Accumulation From Maximum and Minimum Temperatures. Ecol. (1969) 50:514–7. doi: 10.2307/1933912
41. Fornara DA, Tilman D. Plant Functional Composition Influences Rates of Soil Carbon and Nitrogen Accumulation. Ecology (2008) 96:314–22. doi: 10.1111/j.1365-2745.2007.01345.x
42. Steinbeiss S, Beßler H, Engels C, Temperton VM, Buchmann N, Roscher C, et al. Plant Diversity Positively Affects Short-Term Soil Carbon Storage in Experimental Grasslands. Glob Change Biol Bioenergy (2008) 14:2937–49. doi: 10.1111/j.1365-2486.2008.01697.x
43. Sprunger CD, Philip Robertson G. Early Accumulation of Active Fraction Soil Carbon in Newly Established Cellulosic Biofuel Systems. Geoderma (2018) 318:42–51. doi: 10.1016/j.geoderma.2017.11.040
44. Mulvaney RL, Khan SA, Ellsworth TR. Synthetic Nitrogen Fertilizers Deplete Soil Nitrogen: A Global Dilemma for Sustainable Cereal Production. J Environ Qual. (2009) 38:2295–314. doi: 10.2134/jeq2008.0527
45. Russell AE, Cambardella CA, Laird DA, Jaynes DB, Meek DW. Nitrogen Fertilizer Effects on Soil Carbon Balances in Midwestern US Agricultural Systems. Ecol Appl (2009) 19:1102–13. doi: 10.1890/07-1919.1
46. Zan CS, Fyles JW, Girouard P, Samson RA. Carbon Sequestration in Perennial Bioenergy, Annual Corn and Uncultivated Systems in Southern Quebec. Agric Ecosyst Enviorn. (2001) 86:135–44. doi: 10.1016/S0167-8809(00)00273-5
47. Rasse DP, Rumpel C, Dignac M-F. Is Soil Carbon Mostly Root Carbon? Mechanisms for a Specific Stabilisation. Plant Soil (2005) 269(1–2):341–56. doi: 10.1007/s11104-004-0907-y
48. Lange M, Eisenhauer N, Sierra CA, Bessler H, Engels C, Griffiths RI, et al. Plant Diversity Increases Soil Microbial Activity and Soil Carbon Storage. Nat Commun (2015) 6:1–8. doi: 10.1038/ncomms7707
49. Austin EE, Wickings K, Mcdaniel MD, Robertson GP, Grandy AS. Cover Crop Root Contributions to Soil Carbon in a No-Till Corn Bioenergy Cropping System. GCB Bioenergy (2017) 9:1–12. doi: 10.1111/gcbb.12428
50. Yang Y, Reilly EC, Jungers JM, Chen J, Smith TM. Climate Benefits of Increasing Plant Diversity in Perennial Bioenergy Crops. One Earth (2019) 1:434–45. doi: 10.1016/j.oneear.2019.11.011
51. Wang S, Sanford GR, Robertson GP, Jackson RD, Thelen KD. Perennial Bioenergy Crop Yield and Quality Response to Nitrogen Fertilization. Bioenerg. Res (2020) 13:157–66. doi: 10.1007/s12155-019-10072-z
52. Grandy AS, Robertson GP. Land-Use Intensity Effects on Soil Organic Carbon Accumulation Rates and Mechanisms. Ecosystems (2007) 10:59–74. doi: 10.1007/s10021-006-9010-y
53. Kallenbach CM, Frey SD, Grandy AS. Direct Evidence for Microbial-Derived Soil Organic Matter Formation and its Ecophysiological Controls. Nat Commun (2016) 7:13630. doi: 10.1038/ncomms13630
54. Cotrufo MF, Ranalli MG, Haddix ML, Six J, Lugato E. Soil Carbon Storage Informed by Particulate and Mineral-Associated Organic Matter. Nat Geosci (2019) 12:989–94. doi: 10.1038/s41561-019-0484-6
55. van Dam NM, Tytgat TOG, Kirkegaard JA. Root and Shoot Glucosinolates: A comparison of Their Diversity, Function and Interactions in Natural And managed Ecosystems. Phytochem Rev. (2009) 8:171–86 doi: 10.1007/s11101-008-9101-9
56. Liebig MA, Johnson HA, Hanson JD, Frank AB. Soil Carbon Under Switchgrass Stands and Cultivated Cropland. Biomass and Bioenergy (2005) 28:347–354. doi: 10.1016/j.biombioe.2004.11.004
57. Poorter H, Sack L. Pitfalls and Possibilities in the Analysis of Biomass Allocation Patterns in Plants. Frontiers in Plant Science 3. (2012). Available at: https://www.frontiersin.org/article/10.3389/fpls.2012.00259
58. Fontaine S, Mariotti A, Abbadie L. The Priming Effect of Organic Matter: A Question of Microbial Competition? Soil Biol Biochem (2003) 35:837–43. doi: 10.1016/S0038-0717(03)00123-8
59. Sprunger CD, Oates LG, Jackson RD, Robertson GP. Plant Community Composition Influences Fine Root Production and Biomass Allocation in Perennial Bioenergy Cropping Systems of the Upper Midwest, USA. Biomass Bioenergy (2017) 105:248–58. doi: 10.1016/j.biombioe.2017.07.007
60. Dietzel R, Jarchow ME, Liebman M. Above- and Belowground Growth, Biomass, and Nitrogen Use in Maize and Reconstructed Prairie Cropping Systems. Crop Sci (2015) 55(2):910–23. doi: 10.2135/cropsci2014.08.0572
61. Sprunger CD, Culman SW, Robertson GP, Snapp SS. Perennial Grain on a Midwest Alfisol Shows No Sign of Early Soil Carbon Gain. Renewable Agric Food Syst (2018) 33(4):360–72. doi: 10.1017/S1742170517000138
62. Syswerda SP, Corbin AT, Mokma DL, Kravchenko AN, Robertson GP. Agricultural Management and Soil Carbon Storage in Surface vs. Deep Layers. Soil Sci Soc Am J (2011) 75:92–101. doi: 10.2136/sssaj2009.0414
63. Audu V, Rasche F, Dimitrova Mårtensson LM, Emmerling C. Perennial Cereal Grain Cultivation: Implication on Soil Organic Matter and Related Soil Microbial Parameters. Appl Soil Ecol (2022) 174:104414. doi: 10.1016/j.apsoil.2022.104414
64. Ward SE, Smart SM, Quirk H, Tallowin JRB, Mortimer SR, Shiel RS, et al. Legacy Effects of Grassland Management on Soil Carbon to Depth. Glob Change Biol Bioenergy (2016) 22:2929–38. doi: 10.1111/gcb.13246
65. Robertson GP, Paul EA, Harwood RR. Greenhouse Gases in Intensive Agriculture: Contributions of Individual Gases to the Radiative Forcing of the Atmosphere. Science (2000) 289:1922–5. doi: 10.1126/science.289.5486.1922
66. Senthilkumar S, Basso B, Kravchenko AN, Robertson GP. Contemporary Evidence of Soil Carbon Loss in the U.S. Corn Belt. Soil Sci Soc Am J (2009) 73:2078–86. doi: 10.2136/sssaj2009.0044
67. Acosta-Martínez V, Pérez-Guzmán L, Veum KS, Nunes RM, Dick RP. Metabolic Activity-Enzymes. In: Karlen DL, Stott DE, Mikha MM, editors. Soil Health Series: Volume 2 Laboratory Methods for Soil Health Analysis. Madison, WI: John Wiley & Sons (2021). p. 194–251.
68. Horn R, Smucker A. Structure Formation and its Consequences for Gas and Water Transport in Unsaturated Arable and Forest Soils. Soil Tillage Res (2005) 82:5–14. doi: 10.1016/j.still.2005.01.002
69. McCarthy JF, Ilavsky J, Jastrow JD, Mayer LM, Perfect E, Zhuang J. Protection of Organic Carbon in Soil Microaggregates via Restructuring of Aggregate Porosity and Filling of Pores With Accumulating Organic Matter. Geochimica Cosmochimica Acta (2008) 72:4725–44. doi: 10.1016/j.gca.2008.06.015
70. Kaneda S, Ohkubo S, Wagai R, Yagasaki Y. Soil Temperature and Moisturebased Estimation of Rates of Soil Aggregate Formation by the Endogeic Earthworm Eisenia Japonica (Michaelsen, 1892). Biol Fertil Soils (2016) 52:789–797. doi: 10.1007/s00374-016-1119-3
71. Clark JM, Chapman PJ, Adamson JK, Lane SN. Influence of Drought-Induced Acidification on the Mobility of Dissolved Organic Carbon in Peat Soils. Glob Change Biol Bioenergy (2005) 11:791–809. doi: 10.1111/j.1365-2486.2005.00937.x
72. Chowdhury N, Marschner P, Burns R. Response of Microbial Activity and Community Structure to Decreasing Soil Osmotic and Matric Potential. Plant Soil (2011) 344:241–54. doi: 10.1007/s11104-011-0743-9
73. Prescott CE. Litter Decomposition: What Controls it and How can We Alter it to Sequester More Carbon in Forest Soils? Biogeochemistry (2010) 101:133–49. doi: 10.1007/s10533-010-9439-0
74. Bradford MA, Crowther TW. Carbon Use Efficiency and Storage in Terrestrial Ecosystems. New Phytol (2013) 199:7–9. doi: 10.1111/nph.12334
75. Zhang K, Dang H, Zhang Q, Cheng X. Soil Carbon Dynamics Following Land-Use Change Varied With Temperature and Precipitation Gradients: Evidence From Stable Isotopes. Glob Change Biol Bioenergy (2015) 21:2762–72. doi: 10.1111/gcb.12886
76. Göransson G, Larson M, Bendz D. Variation in Turbidity With Precipitation and Flow in a Regulated River System &Ndash; River Göta Älv, SW Sweden. Hydrol Earth Syst Sci (2013) 17:2529–42. doi: 10.5194/hess-17-2529-2013
77. Meisner A, Rousk J, Bååth E. Prolonged Drought Changes the Bacterial Growth Response to Rewetting. Soil Biol Biochem (2015) 88:314–22. doi: 10.1016/j.soilbio.2015.06.002
78. Zhao M, Guo S, Wang R. Diverse Soil Respiration Responses to Extreme Precipitation Patterns in Arid and Semiarid Ecosystems. Appl Soil Ecol (2021) 163:103928. doi: 10.1016/j.apsoil.2021.103928
79. Worrall F, Burt TP. The Effect of Severe Drought on the Dissolved Organic Carbon (DOC) Concentration and Flux From British Rivers. J Hydrol. (2008) 361:262–74. doi: 10.1016/j.jhydrol.2008.07.051
80. Tucker CL, Bell J, Pendall E, Ogle K. Does Declining Carbon-Use Efficiency Explain Thermal Acclimation of Soil Respiration With Warming? Glob Change Biol Bioenergy (2013) 19:252–63. doi: 10.1111/gcb.12036
81. Du L, Zhu Z, Qi Y, Zou D, Zhang G, Zeng X, et al. Effects of Different Stoichiometric Ratios on Mineralisation of Root Exudates and its Priming Effect in Paddy Soil. Sci Total Environ (2020) 743:140808. doi: 10.1016/j.scitotenv.2020.140808
82. Pohl M, Graf F, Buttler A, Rixen C. The Relationship Between Plant Species Richness and Soil Aggregate Stability can Depend on Disturbance. Plant Soil (2012) 355:87–102. doi: 10.1007/s11104-011-1083-5
83. Kravchenko AN, Zheng H, Kuzyakov Y, Robertson GP, Guber AK. Belowground Interplant Carbon Transfer Promotes Soil Carbon Gains in Diverse Plant Communities. Soil Biol Biochem (2021) 159:108297. doi: 10.1016/j.soilbio.2021.108297
84. Lazicki P, Mazza Rodrigues JL, Geisseler D. Sensitivity and Variability of Soil Health Indicators in a California Cropping System. Soil Sci Soc Am J (2021) 85:1827–42. doi: 10.1002/saj2.20278
85. Oldfield EE, Eagle AJ, Rubin RL, Rudek J, Sanderman J, Gordon DR. Crediting Agricultural Soil Carbon Sequestration. Science (2022) 375(6586):1222–5. doi: 10.1126/science.abl7991
86. Dick RP, Thomas DR, Halvorson JJ. Standardized Methods, Sampling and Sample Pretreatment. In: Doran JW, Jones AJ, editors. Methods for Assessing Soil Quality. Madison, WI: SSSA (1996). p. 107–22.
Keywords: agroecosystems, soil carbon, climate, POXC, mineralizable C, soil health assessment
Citation: Martin T and Sprunger CD (2022) Sensitive Measures of Soil Health Reveal Carbon Stability Across a Management Intensity and Plant Biodiversity Gradient. Front. Soil Sci. 2:917885. doi: 10.3389/fsoil.2022.917885
Received: 11 April 2022; Accepted: 22 June 2022;
Published: 14 July 2022.
Edited by:
Carolyn Olson, US Geological Survey Emerita, United StatesReviewed by:
Gina Garland, ETH Zürich, SwitzerlandEngracia Madejon, Spanish National Research Council (CSIC), Spain
Copyright © 2022 Martin and Sprunger. This is an open-access article distributed under the terms of the Creative Commons Attribution License (CC BY). The use, distribution or reproduction in other forums is permitted, provided the original author(s) and the copyright owner(s) are credited and that the original publication in this journal is cited, in accordance with accepted academic practice. No use, distribution or reproduction is permitted which does not comply with these terms.
*Correspondence: Christine D. Sprunger, sprunge5@msu.edu