Artificial intelligence unveils key interactions between soil properties and climate factors on Boletus edulis and B. reticulatus mycelium in chestnut orchards of different ages
- 1Agrobiotech for Health, Plant Biology and Soil Science Department, Biology Faculty, University of Vigo, Vigo, Spain
- 2Pharmacology, Pharmacy, and Pharmaceutical Technology Department, Santiago de Compostela University, Santiago de Compostela, Spain
The main objective of this study was to determine the possible interaction of two important abiotic factors (soil and climate) on the mycelial concentration and frequency of the ectomycorrhizal fungi Boletus edulis and B. reticulatus, using traditional statistics and artificial neural network tools. The frequency and concentration of Boletus mycelium were determined over three months (September, October, and November), and two years (2018 and 2020), in three hybrid chestnuts (Castanea × coudercii) orchards of 40-, 10-, and 3- years-old, using real-time qPCR. Statistical analysis revealed a significant effect of the year on B. edulis mycelium concentration and of the sampling plot (different tree ages) on B. reticulatus frequency. The combination of artificial intelligence networks (ANN) with fuzzy logic, named neurofuzzy logic (NF), allowed the construction of two robust models. In the first, using year, month, and sampling plot as inputs, NF identified hidden interactions between year and month on B. edulis mycelium concentration and between sampling plot and sampling month on B. reticulatus mycelium frequency, thus improving the information obtained from the statistical analysis. In the second model, those three factors were disaggregated into 44 inputs, including 20 soil properties and 24 climatic factors, being NF able to select only 8 as critical factors to explain the variability found in both ectomycorrhizal Boletus species regarding mycelial frequency and concentration. Specifically, NF selected two chemical soil properties (cation exchange capacity and total carbon) and three physical properties (macroaggregates, total porosity, and soil moisture at field capacity), as well as their interactions with three climatic elements (cumulative difference between precipitation and potential evapotranspiration (P-PET-1-2) and water deficit (WD-1-2) in the previous two months and excess water (WE-1) in the month prior to sampling. These results provide a much deeper understanding and new insights into the ecology and the role of abiotic factors which explain the different mycelial development patterns of ectomycorrhizal fungi such as B. edulis and B. reticulatus in chestnut agroecosystems.
Introduction
Mycelium of ectomycorrhizal fungi creates in the soil an extensive and dynamic mycelial network which plays a crucial role not only for the nutrient uptake by the host plants, but also promotes the mobilization of soil nutrients (1), enhances the soil C cycle (2, 3), drives the assemblages of microfauna and microbiota communities (2, 4), and contributes to the formation of soil structure through a variety of biochemical and biophysical mechanisms (5). For tracking fungal species along the whole biological cycle, detection, identification, and quantification of extraradical mycelium in the soil represent valuable information to complete the scenario obtained from sporocarp and ectomycorrhizal root tip samplings. However, the distribution and density of the extraradical mycelium were poorly understood until appropriate methods and modern data analysis tools for its study were developed (6). Real-time PCR (qPCR), for instance, provides a precise species-specific measure of mycelial biomass, in comparison to other quantification techniques such as total hyphal length, biochemical markers, or species-specific primers (7).
Biological processes are generally governed by non-deterministic rules, being so difficult to understand and explain by simple statistical analysis, particularly when the experimental design is poor or imbalanced, the databases are complex, vague, noisy, incomplete, or diverse types of data (continuous, discrete, binomial) are used (8, 9). Artificial intelligence tools have demonstrated advantages over statistical analysis in understanding and deciphering hidden patterns and interactions among factors in the complex multifactorial process (10, 11), as is the case of mycelia development. In addition, modern computer-based tools such as artificial neural networks (ANNs) algorithms have been designed to generate mathematical models to learn autonomously (with little human intervention), to detect the effect of several factors, to predict and optimize complex biological processes (12), which could be applied to the study of the mycelial development of ectomycorrhizal fungi.
The Boletus edulis complex (B. edulis Bull.: Fr. sensu stricto, B. aereus Bull.: Fr., B. pinophilus Pilat et Dermek, and B. reticulatus Schaeff.) represents a group of highly valuable edible mushrooms with a broad geographic distribution across Eurasia and North America, also been introduced into several Southern Hemisphere countries including South Africa and New Zealand (13). The identification of the fruiting bodies of these four species traditionally has been difficult because it is based exclusively on a few highly variable morphological characters. Recent studies showed that these four species can be successfully discriminated by an extensive analysis of the internal transcribed spacer of the nuclear rDNA region (14). Many plants are suitable hosts for Boletus spp., belonging to the family Fagaceae (Castanea, Castanopsis, Fagus, Lithocarpus, Quercus), Betulaceae (Carpinus, Corylus, Betula, Ostrya, Populus), Malvaceae (Tilia), Cistaceae (Cistus), Salicaceae (Salix), Ericaceae (Arctostaphylos), and Pinaceae (Abies, Keteleeria, Picea, Pinus, Tsuga) (15, 16). In Spain, the chestnut, Castanea sativa (Mill. Fagaceae), occupies 272,400 ha, being the dominant species in 154,500 ha (17). Galicia (NW Spain) is the main producing area in the country of this plant species, and both forest stands and cultivated chestnut orchards cover a total surface area of 49,308 ha (18). In this region, Rigueiro (19) reported that 100,000–800,000 kg of B. edulis and allied species are marketed and exported annually from Lugo Province (Galicia, Spain).
Despite its ecological and economic importance, the autecology of the B. edulis complex is still poorly understood, and field studies have been carried out only in Italy (20–24) and Spain (4, 25–32).
The climatic niche and the soil characteristics of the association B. edulis-Cistus ladanifer have been investigated, creating a model that could predict territories climatically and lithologically suitable in peninsular Spain (26). Soil parameters involved in significant interactions with B. edulis in Pinus pinaster habitat have been also investigated by Martinez-Peña et al. (28). Peintner et al. (23), studying the soil fungal communities in a Castanea sativa forest, showed that the overlap between above and belowground fungal communities was very low. In their study, Boletus mycelium was rare and scattered, whereas their sporocarps were abundant. This finding was corroborated by De la Varga et al. (27), who indicated that Boletus sporocarps production was correlated neither with the concentration of soil mycelium nor with the presence or abundance of ectomycorrhizas. This high degree of spatial heterogeneity of the mycelium in the soil could depend on the fact that Boletus mycorrhiza is characterized by a long-distance exploration type, having their mycelia concentrated as rhizomorphs (33). Parladé et al. (31) showed as timber harvesting, by either clearcutting or partial cutting, produces a dramatic and rapid decrease of B. edulis extraradical mycelium in the soil. Also, thinning and litter removal affected B. edulis sporocarp production, highlighting as this species does not need a dense canopy but an open and sunny wood habitat to maintain or increase its productivity (22).
Abiotic factors, such as climate elements (light, temperature, humidity, precipitation) and soil properties (nutrient availability or soil structure), exert a decisive influence on mycelium and sporocarp formation (34–36). Moreover, Martinez-Peña et al. (28) showed that rainfall and temperature are the most critical climate factors for B. edulis sporocarps development and used such parameters to develop a predicting model of mushroom production. Similarly, De la Varga et al. (29) concluded that B. edulis mycelium is strongly dependent on the climate, being positively correlated with precipitation, and negatively correlated with the mean temperature of the previous month. Also, soil structure affects fungal development mainly through water availability, organic matter distribution, or bulk density (5).
In the present study, the frequency and concentration of B. edulis and B. reticulatus mycelium were determined over three months (September, October, and November), and two years (2018 and 2020), in three hybrids experimental orchards of Castanea × coudercii orchards of 40-, 10-, and 3-years-old. The existence of a significant impact of these three factors on Boletus mycelium was investigated. We hypothesized a higher concentration of mycelium in mature (40-years-old) orchards in comparison with younger orchards (10- and 3-years-old) because B. edulis is considered a late-stage fungus that produces sporocarps in mature stands and should require an important level of carbon supply (28, 30). A second objective was to assess the existence of significant interactions between chemical and physical soil properties and climate elements in chestnut orchards and B. edulis and B. reticulatus mycelium concentration and frequency, identifying what parameters support mycelium development, using artificial intelligence tools. This study represents the first report on the impact of soil properties and climate factors on two members of the Boletus edulis complex in the Castanea agroecosystem. To the best of our knowledge, this is the first time that artificial intelligence tools have been used to unveil the effect of an abiotic factor on soil mycelium development.
Materials and methods
Study site and experimental orchards
The study site was located in the province of Pontevedra, Galicia, NW Spain. It is characterized by a humid Atlantic climate with a mild mean annual temperature (14.8°C) and low thermal amplitude (11°C). Annual precipitation is high (1613 mm), with significant seasonal variability, being the autumn period as the rainiest season and the months of July and August the lowest precipitation months (44 and 56 mm, respectively), although no dry month is observed throughout the year (www.aemet.es). The soils of the area are mainly Leptosols, Umbrisols, Cambisols, and Regosols (37, 38).
The experimental orchards are located at Bora (Pontevedra), at the Biotechnology Centre of Hifas Foresta company, located at 140 m.a.s.l. (42° 25′ 56.5″ N–8° 34′ 41″ W). In 2018, we used three orchards of chestnut hybrid Castanea × coudercii (C. sativa x C. crenata), sorted among three age classes (two repetitions each), designed as 40-years-old (A40), 10-years-old, (A10), and 3-years-old (A3). In 2020, the study was replicated in the same experimental orchards with chestnut trees of different ages (named B40, B10, and B3, respectively) as indicated in Santolamazza-Carbone et al. (4). The experimental orchards (approximately 300 m2 each) were in the same area (from 200 to 800 m apart from each other) and shared identical climate conditions and geological material (granitic rock). They are surrounded by pastures, fruit orchards, mature chestnuts, and oak trees (Quercus robur). From 2018 to 2020, in the 40-years old orchards, 134 sporocarps belonging to Boletus edulis complex have been observed (S. Santolamazza-Carbone personal observation).
Soil characterization and climate data
Samplings to assess the soil properties of each experimental orchard were carried out in October 2018 and in September 2020. Three soil samples were taken per orchard in the first horizon with a soil corer (5 x 5 cm) for bulk density determination, and another three soil samples at 20 cm deep for the other analyses for a total of 36 samples (3 experimental orchards x 2 replicates x 3 soil samples x 1 sampling month x 2 years).
Soil physical properties such as particle size distribution (sand, silt, and clay %), aggregate size distribution (dry mean weight diameter and aggregates), bulk density (BD; kg m-3), total porosity (Pt; %), and soil moisture at field capacity (FC; % v/v) as well as soil chemical properties, such as pH, total carbon (C; %), total nitrogen (N; %), C/N ratio, available phosphorus (P; mg kg-1), Ca2+, Mg2+, K+, Na+, Al3+ exchange cations, and cation exchange capacity (CEC) expressed in cmol(+) kg-1, and base saturation (V; %) were determined. Most of the soil properties were analyzed according to international standards (39). Others were determined as follows: total C and N with an elemental analyzer. Available P was determined by the Olsen method (40) and aggregate size distribution was determined following the procedure described by Kemper and Rosenau (41) by mechanically sieving the smaller-than-10 mm fractions through sieves of 5, 2, 1, 0.25, and 0.05 mm mesh size. Aggregate size distribution was expressed as the dry mean weight diameter (MWD, mm), % macro- (>0.25 mm), and % micro-aggregates (<0.25 mm) fractions.
The climatic parameters monitored in the two years of study and during the period of 25 years (1985-2020) were the following: mean monthly temperature (Tm,°C), mean monthly maximum temperature (Tmax,°C), mean monthly minimum temperature (Tmin,°C), mean air relative humidity (H, %), monthly precipitation (P, mm). The monthly water balance was characterized by monthly potential evapotranspiration (PET, mm), the monthly difference between precipitation and potential evapotranspiration (P-PET, mm), monthly water deficit (WD, mm), and monthly water excess (WE, mm). The empirical method of Thornthwaite (42) was applied to calculate the monthly water balance parameters: P-PET, WD, and WE, considering a soil maximum water retention of 100 mm.
Meteorological data were obtained from the Pontevedra-Mourente station (42° 26’ 18’’ N - 8° 36’ 57’’ W), about 3 km from the sampling sites (AEMET, Government of Spain, www.aemet.es).
Molecular detection and quantification of B. edulis and B. reticulatus mycelium
To assess mycelium concentration and frequency soil samples were taken in mid-September, mid-October, and mid-November of 2018 and 2020. Three soil samples were extracted per orchard (each year and month), for molecular detection and quantification of Boletus mycelium constituting another 108 samples (3 experimental orchards x 2 replicates x 3 soil samples x 3 sampling months x 2 years). Samples were taken next to the angles and in the center of the square orchard with a maximum distance of 30 cm apart from the tree trunk, according to De la Varga et al. (27) procedure. Soil samples were individually introduced in marked plastic bags and transported to the laboratory, where they were stored at 4°C until processed.
All soil samples from the 2018 (N = 54) and 2020 (N = 54) surveys were individually processed. Soil DNA extractions were carried out with the DNeasy® PowerSoil® Kit (QIAGEN Group), from 0.25 g of soil per sample according to the manufacturer’s instruction. The extracted DNA was stored at −20°C until used.
The concentration (ng mycelium/kg soil) and frequency (calculated as the number of samples that resulted positive for mycelium presence/total samples per plot and sampling month in each year), of extraradical soil mycelium of the 108 soil samples were both assessed by RT-PCR (qPCR).
The DNA samples from 2018 and 2020 (total N = 108) were shipped to the AllGenetics laboratories (AllGenetics & Biology SL, www.allgenetics.eu). Boletus edulis mycelia identification and quantification were performed using a quantitative PCR (qPCR) assay, targeting the ITS genomic region using the primers FWD-Bedu (CTGTCGCCGGCAACGT) and RVS-Bedu (TGCACAGGTGGATAAGGAAACTAG), and TaqMan® probe STQBedu (6FAM-CCCTTTCTCTTTCGTGGAACCTCCCC-BHQ1) designed by De la Varga et al. (27). The dye 6-carboxy-fluorescein (6-FAM) and the Black Hole Quencher (BHQ1) were attached to the primers’ 5’ and 3’ ends, respectively. The qPCRs were carried out in a final volume of 20 μL, containing 10 μL of NZY qPCR Probe Master Mix ROX plus (NZYTech), 0.25 μM of the probe, 0.9 μM of the amplification primers, 2 μL of template DNA, and ultrapure water up to 20 μL. The reaction mixture was incubated as follows: an initial incubation at 95°C for 10 min, followed by 40 cycles of denaturation at 95°C for 15 s, annealing at 55°C for 1 min, extension at 60°C for 1 min; and a final extension step at 60°C for 30 s. Negative qPCR controls that contained no DNA were included to check for cross-contamination. A total of 9 plates were analyzed in this study, with the qPCR reactions performed in triplicate on each sample and control. The standard curve for quantification of B. edulis mycelium in the soil, established by using sporocarp DNA, fulfilled the requirements for real-time PCR in terms of efficiency (R2 = 0.99; efficiency = 96.93%).
Identification and quantification of B. reticulatus were obtained using a primer pair for targeting the ITS genomic region, designed for the first time for this study by using Geneious 10.2.3 (Biomatters Ltd). The primer pair designed for ITS amplification were as follows: Bret_ITS2_F: 5’ GGTGAATCGCTTCCAATTCC 3’ and Bret_ITS2_R: 5’ GTCTCTCGAAGGTCAAAGGT 3’. PCRs were carried out in a final volume of 12.5 μL, containing 1.25 μL of 1:10 diluted template DNA, 0.9 μM of primers, 6.25 μL of Supreme NZYTaq 2x Green Master Mix (NZYTech), and ultrapure water up to 12.5 μL. The reaction mixture was incubated at an initial denaturation of 95°C for 10 min, followed by 40 cycles of 95°C for 15 s, and 64°C for 1 min. This PCR product was purified and used to verify the amplification and to generate the standard curve in the qPCR experiment. The standard curve, established by using sporocarp DNA, had a satisfactory efficiency (R2 = 0.98; efficiency = 99.54%).
Statistical analysis
The impact of the sampling year (2018 and 2020), the sampling month (September, October, November), and the three chestnut orchards of different ages (40-, 10-, and 3-years-old), and the interaction between them were assessed by using factorial ANOVA, firstly on soil physical and chemical properties (assessed from samplings in October 2018 and September 2020) and later on B. edulis and B. reticulatus mycelium concentration and frequency. Pairwise comparisons were tested using Tukey´s Studentized Range (HSD) post hoc test, both at α = 0.05 significance level, using the open software STATISTICA v.12 (43).
Before the statistical analyses, variables were checked for normality by the Levene test and Bartlett’s test for homogeneity of variances. Mycelium concentration data were previously subjected to logarithm transformation (log x+1) to assume equal variance conditions.
Artificial neural networks models
A unique database (Table S1) including a total of 47 inputs or factors and 4 outputs or parameters was analyzed using FormRules® v4.03 (44). Two models were built: the first includes as inputs the 3 factors studied (year, month, and plot) and as outputs the concentration and frequency of mycelium of B. edulis and B. reticulatus. In the second model, two of those inputs (year and month), were better characterized by including the next 24 climatic elements (as inputs): monthly Tm, Tmax, Tmin, H, P, P-PET, WD, and WE. For all temperatures and humidity parameters, the mean of the sampling month (Tm and Hm), the mean of the previous month (Tm-1 and Hm-1), and the mean of two previous months (Tm-1-2 and Hm-1-2) were calculated. For precipitation and water balance parameters the value of the sampling month (P and W), the value of the previous month (P-1 and W-1), and the accumulated value of two previous months (P-1-2 and W-1-2) were also calculated (Table S1). The third input (plot) was disaggregated into 20 soil physical and chemical properties that best described the characteristics of each experimental orchard (Table S1).
FormRules combines the strength of artificial neural networks with those of fuzzy logic and has been designed to model and decipher cause-and-effect relations between the factors to the parameters, particularly efficient when complex multifactorial biological processes are studied (12). All modeling procedures have been described in detail previously (45, 46). In short, this software permits the selection of the ASMOD (adaptive-spline-modelling-of-data) algorithm to maximize model accuracy while minimizing the number of significant factors and model complexity (47). ASMOD split the model into smaller submodels, starting with a set of the simplest ones, for each parameter (48). Model quality was assessed by the coefficient of determination (Train Set R2) expressed in percentage:
yi represents the experimental values, yi’ represents the predicted values by the model, and yi’’ represents the mean of the dependent variable. The Train Set R2 coefficient describes how much of the variance of the parameters (dependent variables) are considered during model building: the higher the R2 value, the more the model has captured the variation in the training data. Thus, train Set R2 percentages between 70 and 99 are indicative of good to excellent model performance, below those rates (50-70%) model loss accuracy and models may not be reliable, finally, if high values ≥ 99% indicated over-fitting, meaning the model present poor predictability, in this case, the model should be readjusted (10, 49). The model quality was also determined by the ANOVA parameters (f-ratio): if the model F- ratio is higher than the training data f-critical there are no statistical differences between experimental and predicted values, thus the model presents high predictability and accuracy (50).
Five fitness criteria can be selected: two focus on the validation such as Cross-Validation (CV) and Leave One Out Cross-Validation (LOOCV) and three focus on statistical significance such as Bayesian Information Criterion (BIC), Minimum Description Length (MDL), and Structural Risk Minimization (SRM). Details about the convenience of using one or the other can be found elsewhere (51–53). In this study, SRM fitness criterium was selected showing a high accuracy and predictability models. The training parameters selected to build the neurofuzzy logic models 1 and 2 are shown in Table S2.
Although this software built a predictive black box model, advantageously delivers a set of IF (condition)–THEN (observed behavior) rules per submodel with their corresponding membership degree, ranging from 0 to 1 (54 and references therein). These rules use linguistic tags with a certain range level from Low to High, facilitating the interpretation of the predicted results by the model (12).
Results
Soil characterization
The results of soil physical and chemical properties of the experimental orchards and the existence of significant differences between them are summarized in Table S3. All the soils were characterized by presenting a sandy-loam texture, and a clear predominance of the macro-aggregates (63.8-81.0%) in comparison with micro-aggregates (19.0-36.2%). The scarce presence of clays makes the aggregation of the soils very weak, being reflected in the low values of MWD (0.9-1.8 mm). The bulk density (BD) was low (950-1194 kg m-3), which translates into a high porosity (Pt) (53.5-64.1%) and low-mid soil moisture (FC) (20.2-34.1% w/w). In addition, these soils are strongly acid (pH 4.7-5.0), with high C contents (3.2-6.9%), a very weak CEC (2.8-4.9 cmol(+) kg-1), and very low assimilable P (4.6-14.5 mg kg-1) values (Table S3).
The ANOVA showed significant differences (p<0.05) in the soil properties depending on the year and sampling plot factors however no interaction between year and plot (P = 0.065) was found. Regarding physical properties, no significant differences in the size particle distribution (sand, silt, and clay) have been found (Table S3). However, a higher percentage (p<0.05) of soil macro-aggregates and MWD have been detected in younger chestnut orchards (10- and 3-years-old plots) compared to mature chestnuts (40-years-old plots) in both years, whereas micro-aggregates were significantly more abundant in 40-years-old orchards. In addition, BD and Pt were significantly different only when comparing the 3-year-old orchards in 2018 with the 40-year-old orchards in 2020. Finally, FC was significantly higher in 10-year-old plots in both years (Table S3).
Regarding the chemical properties, pH and assimilable P content did not change across the sampling plots. On the other hand, C and N contents were significantly higher in 40- and 10-years-old orchards in 2020 compared to 40-old orchards in 2018, whereas the C/N ratio in 40-years-old orchards in 2018 was the highest value obtained in both years in all the orchards. Finally, significant differences in CEC and V% were also observed in the soils of the 3-year-old orchards compared to 40-year-old orchards, with higher values in the young plots.
Climate conditions characterization
In 2018 a mild mean temperature (14.9°C) and high precipitation (1755 mm), like the mean climate of the area were measured (Table S4). However, mean maximum and minimum temperatures during the 2018 summer (Jul-Sept) period were of the order of 2-3°C higher than those of the 25-year average climate, and precipitation decreased from 51.4 mm in this period compared to 195 mm in the same period of the 25-year average (Table S4). Consequently, three consecutive dry months (Pm ≤ 2 Tm) can be observed in the climograph (Figure S1B). Even more, a negative water balance that extended from July to the end of September in 2018 with a cumulative P-PET value of -288.5 mm and WD of 214.4 mm were observed (Table S4).
During 2020, the mean annual temperature was slightly higher than in 2018 (15.4°C) and annual precipitation was also high (1668 mm). However, it stands out, only the month of July without precipitation (Table S4), being the warmest month of the year (Tm 22.7°C, Tmax 29.3°C), resulting in the only dry month of this year (Figure S1C). Precipitation in August and September was much higher (149.9 mm) than in 2018 (16.6 mm), while November was much drier (106.0 mm) than in 2018 (310.5 mm) (Table S4 and Figure S1). The water deficit started earlier, in June (1.2 mm), but the recovery of the soil water reserve was faster and earlier than in 2018 (Table S4), thanks to rainfall from August (92.1 mm) onwards (Figure S1C).
Mycelium concentration and frequency
Both mycelium concentration, expressed as log (x+1), and mycelium frequency of B. edulis increased significantly (p<0.05) in 2020 in comparison with 2018 (Figures 1A, D), but no significant effect (p>0.05) of sampling month (Figure 1B) and plot (Figures 1C, E), nor the interactions among all the factors were found. The mycelium frequency of B. reticulatus was significantly higher in the 40-year-old orchard (Figure 1F), whereas mycelium concentration did not change depending on the years, months, and plot (Figures 1A–C).
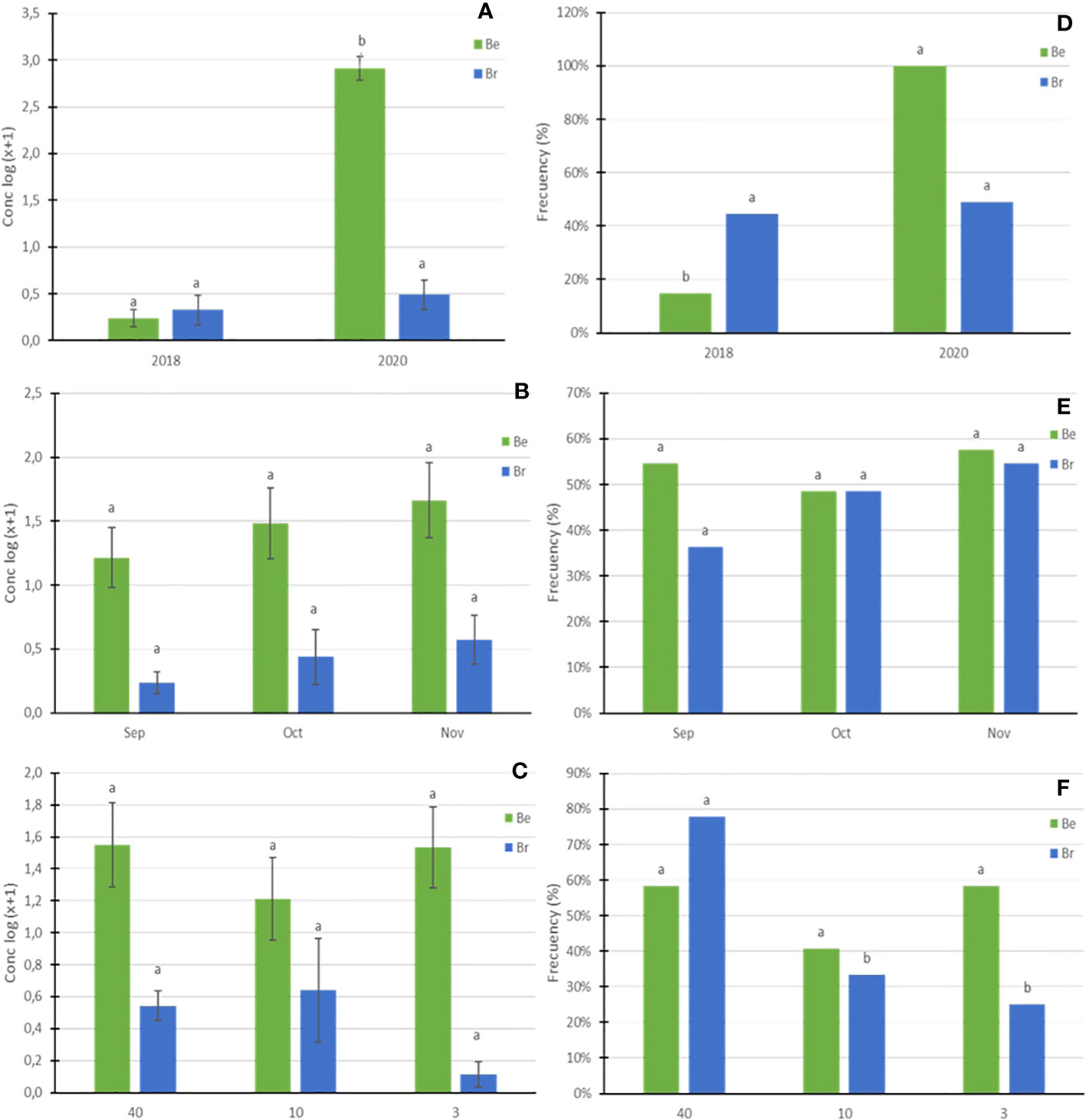
Figure 1 Logarithmic transformation (Log x+1) of the mean of mycelial concentration (A–C) and frequency (D–F) of B. edulis (Be) and B. reticulatus (Br) as a function of the three abiotic factors studied: year (A, D), month (B, E) and plot (C, F). Bars show standard deviation. Different lowercase letters represent significant differences between the groups (α = 0.05).
Neurofuzzy models for mycelium concentration and frequency
The first neurofuzzy logic model using a database (Table S1) which includes three factors (year, month, and plot) as inputs, and the four parameters (concentration and frequency of B. edulis and B. reticulatus) was built.
The ASMOD algorithm was able to divide the models into 1 or 2 submodels for each of the four parameters, selecting in each case the simplest submodels, which were those that maximized accuracy and minimized the number of significant factors (up to four inputs per submodel; see Table S2), reducing the complexity of the model (Table 1). Specifically, neurofuzzy logic was able to build two robust submodels that predicted successfully (percentage Train Set R2 > 70%) and accurately (F ratio > f critical) with no significant differences between the predicted and the experimental values, the concentrations and frequency of B. edulis. The interaction of year and month was the most significant critical factor for the mycelium concentration of B. edulis, while only the year was significant for frequency (Table 1A).
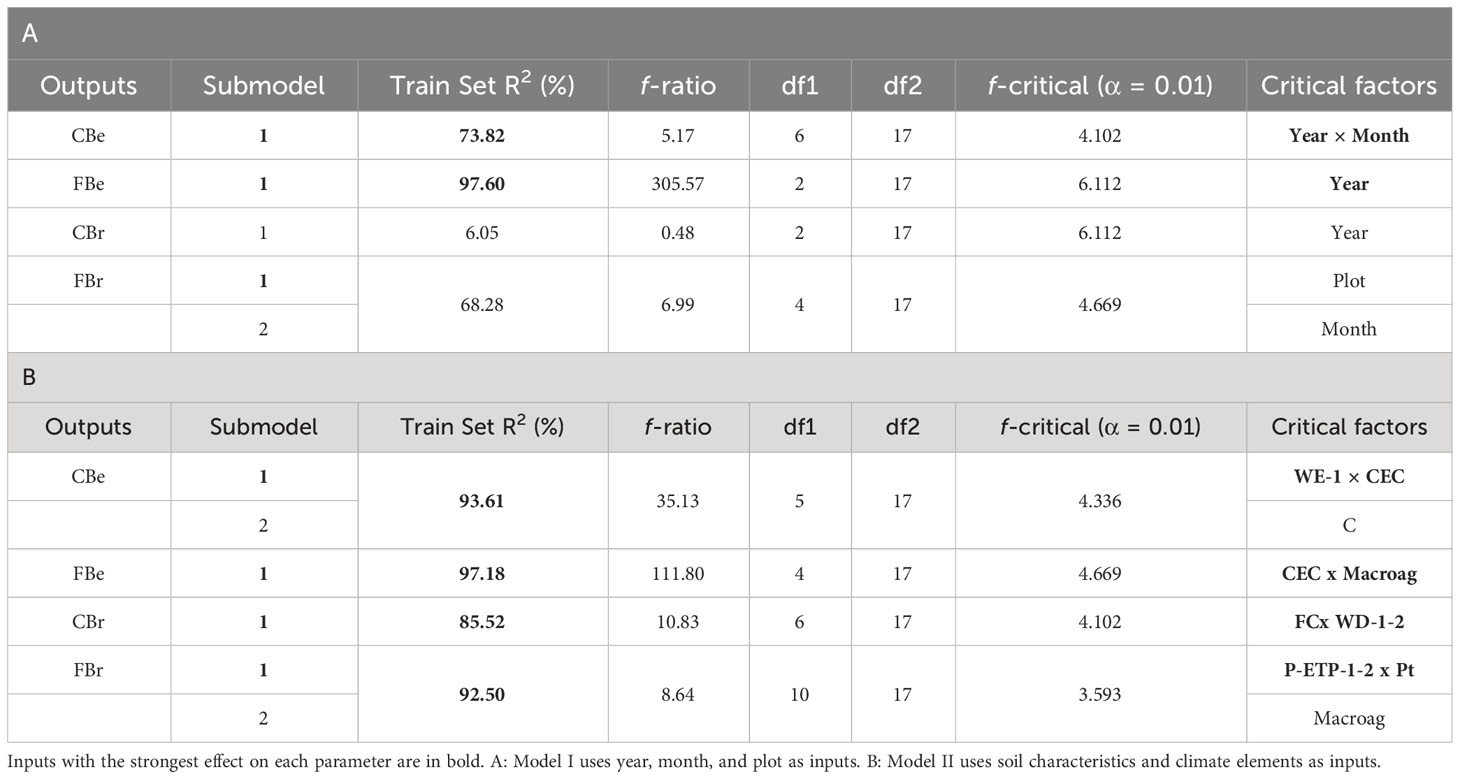
Table 1 Neurofuzzy logic model quality parameters Train Set R2 and ANOVA (f-ratio, degrees of freedom and F -critical) and inputs causing a significant effect on each output: concentration (C) and frequency (F) of B. edulis (Be) and B. reticulatus (Br).
Contrarily, the submodels built for B. reticulatus showed extremely low predictability for mycelium concentration (Train Set R2 = 6.05%) and no accuracy (F ratio< f critical). On the other hand, the submodel showed a bit lower predictability (68.28%) but a good accuracy (F ratio > f critical) for the mycelium frequency (Table 1A), being the plot the most significant factor. Despite this result suggests an important contribution of the three factors studied on this specie, it should be not taking into account due to model low robustness, predictability, and accuracy.
The rules delivered by neurofuzzy logic submodels facilitate the interpretation of the results predicted by the model (Table 2). The rules describe how the critical factors affect each output. Thus, the highest concentration of B. edulis mycelium was obtained in November 2020 and the lowest in September 2018 (rules 6 and 1, respectively; Table 2). The membership (MD) value (0.61) means that the concentration of the mycelium achieved under the climatic and soil conditions of November 2020 falls more into the High (0.61) than in the Low (1.00-0.61 = 0.39) range of concentrations obtained in this study data. However, if the climatic conditions were those found in September 2018 (rule 1; Table 2), the concentration of mycelium predicted will be always included in the low (MD 1.00) range of concentrations determined. Almost the same results were obtained for the rest of the months of 2018 (rules 2-3; Table 2) since low concentration was predicted by the model with an MD of 0.99. This interaction between year and month suggests that climate factors, rather than soil properties, were the cause of this effect.
The frequency of B. edulis mycelium (rules 7-8; Table 2) also indicated that the factor year was essential, being higher in 2020, and predicted that under those climatic and soil conditions will be always high (MD 1.00).
Regarding B. reticulatus, the models built for mycelium frequency may be no reliable (predictability a bit lower than 70%, Table 1A), but accurate, suggesting that if experimental orchards were constituted by 40-years-old trees (rule 13; Table 2), and if the climatic conditions were those found in November (rule 16; Table 2), these conditions facilitate the frequency of this mycelium with a membership of 1.00 and 0.65, respectively. In this case, no interaction between both factors was detected, meaning their effect was independent of each other.
All those results suggest that some climatic and soil characteristics conditions together with the age of the trees (plot factor), may favor the mycelium concentration and frequency, however, no information was obtained regarding what climatic element and soil properties were responsible for those effects. For this reason, to better characterize the factors “year” and “month” those factors were substituted by adding 24 climatic elements, whereas for the factor “plot” it was by 20 soil physical and chemical properties (Table S1).
Again, the ASMOD algorithm was able to divide the model built by neurofuzzy logic into only 1 or 2 submodels for each of the four parameters, to select the simplest ones and to minimize the number of significant factors, reducing the complexity of the model, but maximizing its accuracy (Table 1B). With this new database, neurofuzzy logic successfully predicted all parameters (Train Set R2 > 70%) with precision and accuracy (f critical> F ratio) for B. edulis and B. reticulatus (Table 1B).
Two submodels were delivered for the concentration of B. edulis: submodel 1 pointed out that the interaction between one climatic parameter related to water balance (WE-1) and one soil chemical characteristic (CEC) play the most key role in the mycelium concentration, followed by (submodel 2) another soil chemical characteristic (C%) (Table 1B). For the mycelium frequency of B. edulis, the most crucial factors were the interaction between soil CEC and one physical factor (Macroag).
For B. reticulatus, only one submodel was delivered by neurofuzzy logic, pointing out that the interaction between one soil physical character (FC) and one climate factor (WD-1-2) was essential to increase the mycelium concentration. On the other hand, for B. reticulatus mycelium frequency two submodels were released: the first highlighted the interaction between one climate element (P-PET-1-2) and soil porosity (Pt), whereas the second showed the impact of the soil macro-aggregates (Table 1B).
The rules from the neurofuzzy logic model also facilitate the interpretation of the predicted results (Table 3).
The highest concentration of B. edulis mycelium was obtained when a high amount of water excess one month before the sampling (WE-1) interacts with low CEC in the soil (rule 2; Table 3), as can be observed in Figure 2A. The membership (MD) value (1.00) means that the concentration achieved under those climatic and soil conditions always will fall into the highest values obtained in this study. Secondly, the submodel 2 (Table 1B) pinpointed that high concentrations of carbon in the soil (C) also favor the amount of mycelium concentration found (rule 6; Table 3).
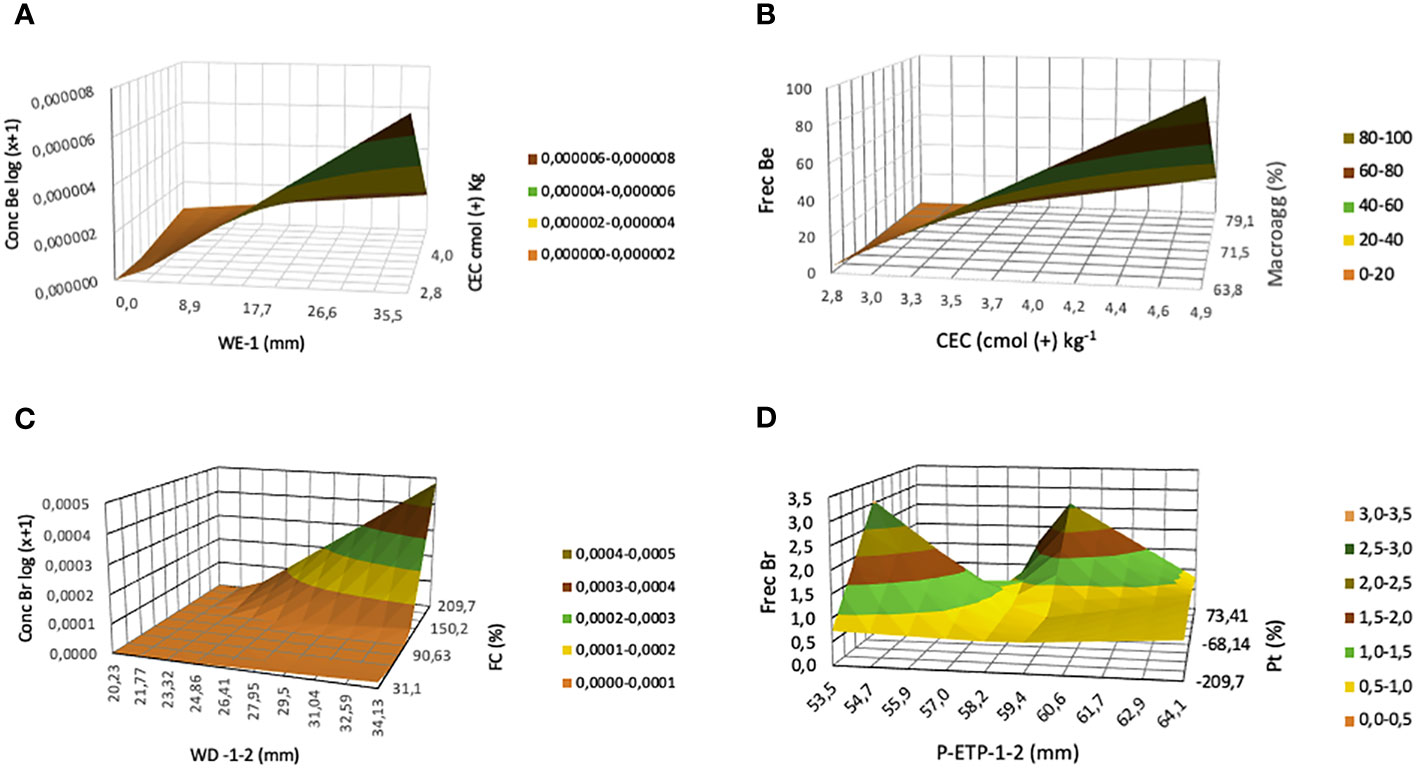
Figure 2 Tridimensional plot predicted by the neurofuzzy logic model for each parameter: mycelial concentration (A, C) and frequency (B, D) of B. edulis (Be) and reticulatus (Br) respectively, as a function of key soil properties and climate elements.
The interaction between high water deficit two months before the sampling (WD-1-2) and high field capacity (FC) caused the strongest effect on the mycelium concentration of B. reticulatus (rule 16; Table 3 and Figure 2C).
As described previously (Table 1B), neurofuzzy logic was able to build highly predictive and accurate models for the frequency of both Boletus species, in Model II in contrast to Model I. The rules derived for B. edulis frequency indicated that if the amount of cation exchange capacity (CEC) was high (rules 9-10; Table 3), independently of the macro-aggregates values, then the frequency was always high, as clearly can be visualized in Figure 1B.
Finally, the neurofuzzy logic rules revealed that most of the combinations of soil porosity (Pt) and accumulation of evapotranspiration during the two months previous to the sampling (P-PET-1-2), caused a positive effect on the frequency of B. reticulatus (rules 17-25; Table 3), although the strongest negative effect was obtained with low porosity and High P-PET-1-2 (rule 18; Table 3), as can be observed in Figure 2D. Finally, if soil presents a high percentage of macro-aggregates then a low frequency of mycelium was achieved (rule 27; Table 3).
Discussion
Currently, the knowledge on the factors related to mycelium development of ectomycorrhizal fungi highlights the importance of both biotic and abiotic factors, such as soil microbiota (4, 32), climate elements (29, 31), seasons (29, 31, 55), and forest management (30, 31). This investigation area, however, is much less prominent in comparison with the study of the factors promoting sporocarp fruiting of ectomycorrhizal macrofungi (21, 26, 28, 34–36, 56, 57 58, 59), probably because wild edible fungi have a great social, economic, and ecological value, being not only a source of food, income, and jobs but also an important reason to maintain forest health (35).
It has been also established that soil and climate parameters only do not fully explain fungal dynamics being silvicultural management (31), stand structure, and local site characteristics (28, 60), stand basal area (28), and plant age class (61) of great importance. However, despite these important advances, at present, the specific factors responsible for B. edulis complex mycelial development and sporocarp yield are still unclear.
Regarding the soil characteristics that may favor the presence and development of B. edulis mycelium, in 1998 Hall et al. reported that this species can be found in a wide range of habitats and edaphic conditions. More recently, Alonso Ponce et al. (26) provided models to describe the realized niche of B. edulis in Cistus ladanifer habitat, assessing that the appropriated soil pattern had low pH, sandy loam texture, high C/N content, low nitrogen, and poor P, K, Mg, and Ca content. Also, Martínez-Peña et al. (28) through the study of B. edulis yield in Pinus sylvestris stands, confirmed the importance of high C/N content, low mineral nutrient, and low pH, and highlighted the positive correlation with sand percentage and water retention capacity. Similar conclusions were shown by Pereira et al. (62), who reported that high contents of organic matter and medium-low concentrations of macro and micronutrients were key factors for Boletus sp. development.
Our results of soil characterization of chestnut orchards generally agree with these works and confirm, therefore, that the optimal edaphic environment for the development of Boletus species has a sandy loam texture, a clear predominance of macro-aggregates, low mean diameter aggregates, and low bulk density, which caused high porosity and low-mid field capacity. Furthermore, the experimental soils were strongly acids (pH 4.7-5.0), with high carbon content, weak CEC, and low content in essential cations (Ca2+, Mg 2+, K+, Na +, and Al 3+) and P available. The soil P content did not change significantly between the sampling orchards and neither statistical tests nor artificial intelligence did find a significant impact on boletes mycelium, thus, in the present study the influence of this important nutrient on mycelium development was not detected.
Significant differences among the experimental orchards, regarding soil properties have been found. This result was not surprising because it is well-known that plant age may influence plant-soil interactions through significant changes in soil microbiota, which in turn shapes soil performance (63–65). The younger plots (3-year-old) presented higher bulk density, macro-aggregates, mean diameter aggregates, cation exchange capacity, and base saturation, in comparison with the 40-years-old plots, which, in contrast, have a higher content of C, N, and C/N. This could be also due to the different management of the experimental chestnut orchards. Mature orchards were conserved to obtain new plant material for grafting, consequently, the accumulation and decomposition of leaf litter increased soil mineral nutrients such as C, N, and C/N ratio (66, 67). On the other hand, 3-years-old orchards were destinated for commercialization and have been irrigated and fertilized with macronutrients. This different management also promoted higher soil bacterial and fungal diversity in younger orchards compared to the mature (40-years-old) ones (4).
B. edulis and B. reticulatus are highly valued ectomycorrhizal fungi with a wide distribution in the world, particularly in acidic soils in the warmer parts of temperate zones (68). The study area (Galicia, NW Spain) is characterized by acidic soils (pH 5.0) and a warm climate, with abundant rainfall (around 1,700 mm) and mild temperatures (around 15°C) over the year, being adequate for the development of these two boletes. For B. edulis, both precipitation and temperature have been positively correlated with sporocarp development (28). On the other hand, a positive correlation of B. edulis mycelia with precipitation, but a negative correlation with the mean temperature of the previous month have been also reported (29). In a more recent study, in which B. edulis mycelium biomass was determined, no correlation with mean monthly temperature was found, although a negative correlation with mean monthly precipitation was detected (31). In agreement with this last study, we did not find any impact of the air temperature on B. edulis and B. reticulatus mycelium development, whereas climate factors relative to precipitations such as field capacity (FC), the difference between precipitation and potential evapotranspiration (P-PET), water deficit (WD) and water excess (WE), had a significant effect. In the present study, in 2020 summer precipitations (from July to September) were much higher than in 2018 (195 mm and 51 mm, respectively), triggering a significant increase in soil water availability (FC), which had a positive effect on B. reticulatus mycelium concentration. However, for B. edulis this abiotic factor did not have a significant impact, suggesting for the first time different climatic needs for the mycelium development of these fungal species.
Boletus edulis is considered a late-stage ectomycorrhizal fungus requiring elevated levels of carbon and fruiting in mature stands (28, 30). Considering that mature agroforestry habitats commonly have a higher soil organic C due to the abundance of organic matter input in the form of litterfall and fine roots from trees (69), it was expected a greater mycelium development in mature orchards. Accordingly with this hypothesis, we found that B. edulis mycelium concentration was positively affected by high C% in the soil, however, data analyses showed that not only B. edulis mycelium concentration equally spread irrespective of plant age, in agreement with previous research (4), but also B. reticulatus mycelium concentration followed the same pattern. Nonetheless, the highest frequency of B. reticulatus mycelium was found in 40-years-old orchards, suggesting that the presence of this specie was favored by some factors specific to this plant age, possibly related to a different soil microbiota assemblage in this age class in comparison with younger orchards (4).
By using the ANOVA, no significant differences were found between the sampling months, neither for mycelium concentrations nor for the frequency of both boletes. However, neurofuzzy logic has autonomous learning capabilities, being able to simultaneously identify nonlinear and hidden relationships between the studied factors (70), facilitating decision-making by helping researchers to understand the cause-effect relationships between those factors (11, 12, 71). Two neurofuzzy models were built to decipher the role of soil properties and local climate elements on the frequency and concentration of B. edulis and B. reticulatus mycelium. In the first, year, month, and plot were used as inputs, and in the second, soil and climate characteristics were disaggregated into 20 soil physical and chemical properties and 24 climate elements, simultaneously modeling 44 inputs and 4 outputs.
Advantageously, neurofuzzy unveils some interactions not detected by statistics. Firstly, the variability detected for B. edulis mycelium concentration was caused by the interaction of year and month, being the highest concentration found in November 2020. This output was also corroborated by the ANOVA, which detected the highest mycelium concentration in 2020. Moreover, neurofuzzy predicted low B. reticulatus mycelium concentration in both years, suggesting that despite both fungal species being exposed to the same climate conditions during the autumn of both years in the same chestnut orchards, they have different requirements for mycelium development. Also, neurofuzzy was able to unveil that the highest B. reticulatus mycelium frequency was caused by the interaction between the soil conditions of mature (40-years-old) orchards combined with the climate factors found in November. These new results suggested hidden relationships between soil characteristics and climate elements.
Soil requirements of B. edulis complex extra-radical mycelium and sporocarps development are still poorly understood. Martinez-Peña et al. (28) assessed that in general soil characteristics were not significant predictors of the annual yield of ectomycorrhizal sporocarps such as Lactarius and B. edulis in Pinus sylvestris stands. However, these authors highlighted the existence of simple negative relationships between silt content and soil pH with B. edulis yield, whereas sand content and water field capacity were positively correlated with the development of the sporocarps.
In the present study, artificial intelligence found that the existence of a high CEC, and the predominance of macro-aggregates, both characters typical of fertilized 3-years-old orchards, determined a high mycelium frequency of B. edulis. On the other hand, low CEC associated with high water excess one month before the sampling date (WE-1) triggered a high mycelium concentration, whereas the opposite was found if WE-1 was low. The response of B. edulis mycelium to soil CEC is difficult to interpret because it has been shown that the mycelium frequency and concentration of this species did not depend on the plot.
Seasonal fluctuations of Boletus mycelia concentration during the fructification period, from October to December, can be due to the change in the allocation of resources in the mycelium to produce sporocarps (29). We detected a significant increase in B. edulis mycelium concentration in 2020, which could be explained by the increase in monthly water excess found in November of both 2018 and 2020. Rainfalls in August and September 2020 were higher (149.9 mm) than in 2018 (16.6 mm), although November 2020 was much drier (106.0 mm) than in 2018 (310.5 mm). In addition, the recovery of the soil water reserve in 2020 was faster and earlier than in 2018, thanks to rainfall from August onwards. However, it has been also shown that severe precipitations provoke a lack of oxygen caused by temporary flooding which could severely affect the mycorrhizal formation and mycelium development of B. edulis (31). These results suggest that for B. edulis mycelium development soil water availability represents a critical factor, which agrees with the finding of Parladé et al. (31).
This is the first time that the ecology of B. reticulatus regarding the interaction with soil parameters and climate factors has been investigated. In agreement with our hypothesis, the mycelium frequency of B. reticulatus did increase in 40-year-old orchards, although mycelium concentration was not affected by year, month, or sampling plot. It is known that ectomycorrhizal fungi preferred soil with high percentages of organic matter, low bulk density, and high porosity because it is crucial for the increased water-holding capacity and nutrient availability (72). The C, N, and C/N ratio were significantly higher in 40-year-old orchards, accompanied by a lower bulk density, a better equilibrium between macro- and micro-aggregates, and a higher water retention capacity, which may explain the significant increase of B. reticulatus mycelium frequency in these plots. The interaction between a high water deficit one month before the sampling (WD-1-2) and a high soil moisture (FC) induced a high mycelium concentration, suggesting that under dry weather and water stress conditions, B. reticulatus mycelium can develop effectively only if the soil has high water retention capacity. Other soil characteristics such as a high soil porosity combined with a high P-PET two months before the sampling, positively affected mycelium frequency. Soil porosity seems to be an important factor because low values of this parameter triggered a negative effect on mycelium frequency. The study of the impact of soil physical conditions on fungal colonization is important to understand how the fungi explore the pore volume within soil being clustering, connectivity, and tortuosity of the pore space of great importance (73). An increase in bulk density and a reduction in aggregate size increased the fraction of micropores in the air-filled pore volume, thus resulting in smaller, more slowly expanding fungal colonies and also reducing soil volume from which a nutrient source can be colonized (73).
Finally, while the ability to explain complex interactions may be limited, the advantages of artificial neural networks compared to traditional statistical analysis in uncovering concealed patterns and interactions within complex biological processes (10, 11), have once again been demonstrated. Here, the application of neural networks has successfully unveiled the crucial soil properties and climate factors influencing soil mycelia development, never described previously.
Conclusions
Climate-soil interactions, through the water balance and water availability in the soil, together with certain soil chemical (C % and CEC) and physical (macro-aggregates, porosity) properties, have been the main determinants of the mycelial frequency and concentration of two species of the Boletus edulis complex.
Thanks to the use of artificial intelligence, this study allows for the first time to appreciate the different abiotic requirements of B. edulis and B. reticulatus under the same soil conditions and climate parameters. Both species have found more favorable conditions in 2020 and in November, probably because of the higher precipitations that characterized this year at the end of the summer. However, they completely differed regarding soil requirements, being B. reticulatus mycelium more frequently found in mature 40-years-old orchards, and more dependent on high soil water availability and soil porosity than B. edulis. This last species was equally spread in all the orchards, especially in November, and was significantly affected by soil CEC, C content, and macro-aggregates.
Data availability statement
The original contributions presented in the study are included in the article/Supplementary Material. Further inquiries can be directed to the corresponding authors.
Author contributions
Conceptualization, SS-C and PG. Methodology, SS-C, LI-B, ER, MB. Data curation and software: ML, MB and PG. Writing—original draft preparation, SS-C, ER, MB. Writing-review and editing, all; supervision, SS-C and PG. Funding acquisition, MB and PG. All authors contributed to the article and approved the submitted version.
Funding
This research was funded by the Spanish Ministry of Science, Innovation, and Universities (grant number RTI2018-095568-B-I00), by the Spanish Research Agency (grant EQC2019-006178-P; MCIN/AEI/10.13039/501100011033) through the “ERDF A way of making Europe” program, by the Xunta de Galicia (Regional Government) (grants numbers (030_IN848D_2020_1120193 and FEADER 2021/076A) and through the R&D contracts (CO-0014-21 and CO-0065-21) between company Hifas Foresta and the University of Vigo.
Acknowledgments
The authors acknowledge the Spanish Ministry of Science, Innovation, and Universities (grant number RTI2018-095568-B-I00) for the Postdoctoral Contract to Serena Santolamazza-Carbone.
Conflict of interest
The authors declare that the research was conducted in the absence of any commercial or financial relationships that could be construed as a potential conflict of interest.
The authors declare that this study received funding from Hifas Foresta. The funder's involvement in the study was limited to allowing soil sample collection in their chestnut orchards.
The author PPG declared that they were an editorial board member of Frontiers, at the time of submission. This had no impact on the peer review process and the final decision.
Publisher’s note
All claims expressed in this article are solely those of the authors and do not necessarily represent those of their affiliated organizations, or those of the publisher, the editors and the reviewers. Any product that may be evaluated in this article, or claim that may be made by its manufacturer, is not guaranteed or endorsed by the publisher.
Supplementary material
The Supplementary Material for this article can be found online at: https://www.frontiersin.org/articles/10.3389/fsoil.2023.1159793/full#supplementary-material
References
1. Rillig MC, Mummey DL. Mycorrhizas and soil structure. New Phytol (2006) 171:41–53. doi: 10.1111/j.1469-8137.2006.01750.x
2. Courty PE, Buée M, Gamby Diedhiou A, Frey-Klett P, Le Tacon F, Rineau F, et al. The role of ectomycorrhizal communities in forest ecosystem processes: new perspectives and emerging concepts. Soil Biol Biochem (2010) 42(5):679–98. doi: 10.1016/J.SOILBIO.2009.12.006
3. Ekblad A, Wallander H, Godbold DL, Cruz C, Johnson D, Baldrian P, et al. The production and turnover of extramatrical mycelium of ectomycorrhizal fungi in forest soils: role in carbon cycling. Plant Soil (2013) 366:1–27. doi: 10.1007/s11104-013-1630-3
4. Santolamazza-Carbone S, Iglesias-Bernabé L, Sinde-Stompel E, Gallego PP. Soil microbiota impact on Boletus edulis mycelium in chestnut orchards of different ages. Appl Soil Ecol (2023) 185:104790. doi: 10.1016/j.apsoil.2022.104790
5. Ritz K, Young IM. Interactions between soil structure and fungi. Mycologist (2004) 18(2):52–9. doi: 10.1017/S0269-915X(04)00201-0
6. Anderson IC, Cairney JW. Diversity and ecology of soil fungal communities: increased understanding through the application of molecular techniques. Environ Microbiol (2004) 6:769–79. doi: 10.1111/j.1462-2920.2004.00675
7. Landeweert R, Veenman C, Kuyper TW, Fritze H, Wernars K, Smit E. Quantification of ectomycorrhizal mycelium in soil by real-time PCR compared to conventional quantification techniques. FEMS Microbiol Ecol (2003) 45:283–92. doi: 10.1016/S0168-6496(03)00163-6
8. Coruzzi GM, Burga AR, Katari MS, Gutiérrez RA. Systems biology: principles and applications in plant research. In: Coruzzi GM, Gutiérrez RA, editors. Plant Systems Biology, vol. 35 . Wiley-Blackwell, UK: Blackwell Publishing Ltd (2009). p. 3–40.
9. Gago J, Martínez-Núñez L, Landín M, Gallego PP. Artificial neural networks as an alternative to the traditional statistical methodology in plant research. J Plant Physiol (2010) 167:23–7. doi: 10.1016/j.jplph.2009.07.007
10. Landin M, Rowe RC, York P. Advantages of neurofuzzy logic against conventional experimental design and statistical analysis in studying and developing direct compression formulations. Eur J Pharm Sci (2009) 38:325–31. doi: 10.1016/j.ejps.2009.08.004
11. Gago J, Martínez-Núñez L, Landín M, Gallego PP. Strengths of artificial neural networks in modelling complex plant processes. Plant Signal Behav (2010) 5(6):1–3. doi: 10.4161/psb.5.6.11702
12. Gallego PP, Gago J, Landin M. Artificial neural networks technology to model and predict plant biology process. In: Suzuki K, editor. Artificial Neural Networks - Methodological Advances and Biomedical Applications. Rijeka, Croatia: IntechOpen (2011), ISBN: ISBN 978-953-307-243-2. p. 197–216.
13. Sitta N, Davoli P. Edible ectomycorrhizal mushrooms: international markets and regulations. In: Zambonelli A, Bonito GM, editors. Edible Ectomycorrhizal Mushrooms: Current Knowledge and Future Prospects, vol. 34. Berlin, Germany: Soil Biology Springer (2012). p. 355–80.
14. Leonardi M, Paolocci F, Rubini A, Simonini G, Pacioni G. Assessment of inter- and intraspecific variability in the main species of Boletus edulis complex by ITS analysis. FEMS Microbiol Let (2005) 243(2):411–6. doi: 10.1016/j.femsle.2005.01.003
15. Hall IR, Lyon AJE, Wang Y, Sinclair L. Ectomycorrhizal fungi with edible fruiting bodies. 2 Boletus edulis Econ Bot (1998) 52(1):44–56. doi: 10.1007/BF02861294
16. Águeda B, Parladé J, Fernández-Toirán LM, Cisneros O, de Miguel AM, Modrego MP, et al. Mycorrhizal synthesis between Boletus edulis species complex and rockroses (Cistus sp.). Mycorrhiza (2008) 18:443–9. doi: 10.1007/s00572-008-0192-3
17. MAPAMA. Cuarto Inventario Forestal Nacional. Área de Inventario y Estadísticas Forestales, Dirección General de Desarrollo Rural y Política Forestal, Ministerio de Agricultura y Pesca, Alimentación y Medio Ambiente. Madrid, Spain (2013).
18. Xunta de Galicia. O Monte Galego en cifras. Consellería de Medio Ambiente. Dirección Xeral de Montes e Medio Ambiente Natural. Santiago de Compostela, Spain (2001).
19. Rigueiro A. Produccións Complementarias de Bosque. In: Xornadas da Sustentabilidade dos Territorios Agroforestais da Iberia Húmida Diante do Fenómeno do Despoboamento. Allariz, Orense, Galicia, Spain (2000).
20. Meotto F, Pellegrino S, Bounous G. Evolution of Amanita caesarea (Scop.: Fr.) Pers. and Boletus edulis Bull.: Fr. synthetic ectomycorrhizas on European chestnut (Castanea sativa Mill.) seedlings under field conditions. Acta Hortic (1999) 494:201–4. doi: 10.17660/ActaHortic.1999.494.30
21. Laganà A, Salerni E, Barluzzi C, Perini C, De Dominicis V. Macrofungi as long-term indicators of forest health and management in central Italy. Cryptogamie Mycol (2002) 23:39–50.
22. Salerni E, Perini C. Experimental study for increasing productivity of Boletus edulis s.l. in Italy. For Ecol Manage (2004) 201:161–70. doi: 10.1016/j.foreco.2004.06.027
23. Peintner U, Iotti M, Klotz P, Bonuso E, Zambonelli A. Soil fungal communities in a Castanea sativa (chestnut) forest producing large quantities of Boletus edulis sensu lato (porcini): where is the mycelium of porcini? Environ Microbiol (2007) 9:880–9. doi: 10.1111/j.1462-2920.2006.01208.x
24. Ambrosio E, Zotti M. Mycobiota of three Boletus edulis (and allied species) productive sites. Sydowia (2015) 67:197–216.
25. Oria-de-Rueda JA, Olaizola J, Martín-Pinto P. Bolete productivity of cistaceous scrublands in northwestern Spain. Econ Bot (2008) 62:323–30. doi: 10.1007/s12231-008-9031-x
26. Alonso Ponce R, Águeda B, Ágreda T, Modrego MP, Aldea J, Fernández-Toirán LM, et al. Rockroses and Boletus edulis ectomycorrhizal association: realized niche and climatic suitability in Spain. Fungal Ecol (2011) 4(3):224–32. doi: 10.1016/j.funeco.2010.10.002
27. De la Varga H, Águeda B, Martínez-Peña F, Parladé J, Pera J. Quantification of extraradical soil mycelium and ectomycorrhizas of Boletus edulis in a Scots pine forest with variable sporocarp productivity. Mycorrhiza (2012) 22(1):59–68. doi: 10.1007/s00572-011-0382-2
28. Martínez-Penãa F, De-Miguel S, Pukkala T, Bonet JA, Ortega-Martínez P, Aldea J, et al. Yield models for ectomycorrhizal mushrooms in Pinus sylvestris forests with special focus on Boletus edulis and Lactarius group delicious. For Ecol Manage (2012a) 282:63–9. doi: 10.1016/j.foreco.2012.06.034
29. De la Varga H, Águeda B, Ágreda T, Martínez-Peña F, Parladé J, Pera J. Seasonal dynamics of Boletus edulis and Lactarius deliciosus extraradical mycelium in pine forests of central Spain. Mycorrhiza (2013) 23(5):391–402. doi: 10.1007/s00572-013-0481-3
30. Mediavilla O, Hernández-Rodríguez M, Olaizola J, Santos-del-Blanco L, Oria-de-Rueda JA, Martín-Pinto P. Insights into the dynamics of Boletus edulis mycelium and fruiting after fire prevention management. For Ecol Manage (2017) 404:108–14. doi: 10.1016/j.foreco.2017.08.031
31. Parladé J, Martínez-Peña F, Pera J. Effects of forest management and climatic variables on the mycelium dynamics and sporocarp production of the ectomycorrhizal fungus Boletus edulis. For Ecol Manage (2017) 390:73–9. doi: 10.1016/j.foreco.2017.01.025
32. Mediavilla O, Geml J, Olaizola J, Oria-de-Rueda JA, Baldrian P, Martín-Pinto P. Effect of forest fire prevention treatments on bacterial communities associated with productive Boletus edulis sites. Microb Biotechnol (2019) 12(6):1188–98. doi: 10.1111/1751-7915.13395
33. Agerer R. Exploration types of ectomycorrhizae. A proposal to classify ectomycorrhizal mycelial systems according to their patterns of differentiation and putative ecological importance. Mycorrhiza (2001) 11:107–14. doi: 10.1007/s005720100108
34. Erland S, Taylor AFS. Diversity of ectomycorrhizal communities in relation to the abiotic environment. In: van der Heijden MGA, Sanders IR, editors. Mycorrhizal ecology. Berlin, Heidelberg: Springer (2002). p. 163–200.
35. Barroetaveña C, La Manna L, Alonso MV. Variables affecting Suillus luteus fructification in ponderosa pine plantations of Patagonia (Argentina). For Ecol Manage (2008) 256(11):1868–74. doi: 10.1016/j.foreco.2008.07.029
36. Murat C, Mello A, Abba S, Vizzini A, Bonfante P. Edible mycorrhizal fungi: identification, life cycle, and morphogenesis. Mycorrhiza. In: Varma A, editor. State of the Art, Genetics and Molecular Biology, Eco-Function, Biotechnology, Eco-Physiology, Structure and Systematics, 3rd ed. Berlin: Springer (2008), ISBNISBN: 9783540788249. p. 707–32.
37. IUSS Working Group WRB. Base referencial mundial del recurso suelo 2014, Actualización 2015. In: Sistema internacional de clasificación de suelos para la nomenclatura de suelos y la creación de leyendas de mapas de suelos. Informes sobre recursos mundiales de suelos, vol. 106. Roma: FAO (2015).
38. Carballas T, Rodríguez-Rastrero M, Artieda O, Gumuzzio J, Díaz-Raviña M, Martín A. Soils of the temperate humid zones. In: Gallardo JF, editor. The soils of Spain. Berlín, Germany: Springer (2016). p. 49–144.
39. USDA. Soil survey laboratory methods manual. In: Burt R, editor. Soil Survey Investigation Report No. 42. USDA. USA: Scientific Publishers (2012).
40. Olsen SR, Dean LA. Phosphorus. In: Black CA, editor. Methods of Soil Analysis, part 2. Chemical and Microbiological Properties. Madison, WI: American Society of Agronomy (1965). p. 1035–49.
41. Kemper WD, Rosenau RC. Aggregate stability and size distribution. In: Klute A, editor. Methods of Soil Analysis Madison, Wisconsin, USA: SSSA Books Series. (1986). doi: 10.2136/sssabookser5.1.2ed.c17
42. Thornthwaite CW. An approach toward a rational classification of climate. Geogr Rev (1948) 38(1):55–94. doi: 10.2307/210739
45. Nezami-Alanagh E, Garoosi GA, Landín M, Gallego PP. Combining DOE with neurofuzzy logic for healthy mineral nutrition of pistachio rootstocks in vitro culture. Front Plant Sci (2018) 9:1474. doi: 10.3389/fpls.2018.01474
46. Hameg R, Arteta TA, Landin M, Gallego PP, Barreal ME. Modeling and Optimizing culture medium mineral composition for in vitro propagation of Actinidia arguta. Front Plant Sci (2020) 11:554905. doi: 10.3389/fpls.2020.554905
47. Kavli T, Weyer E T, Weyer E. ASMOD (adaptative spline modeling of observation data)—some theoretical and experimental results. IEE Colloq (Digest) (1994) 136:1–7.
48. Shao Q, Rowe RC, York P. Comparison of neurofuzzy logic and neural networks in modelling experimental data of an immediate release tablet formulation. Eur J Pharm Sci (2006) 28:394–404. doi: 10.1016/j.ejps.2006.04.007
49. Colbourn E, Rowe R. Neural computing and pharmaceutical formulation. In: Swarbrick J, Boylan JC (eds) Encyclopedia of pharmaceutical technology, 3rd edn. New York: Marcel Dekker. (2005).
50. Landin M, Rowe RC. Artificial neural networks technology to model, Understand, and optimize drug formulations. In: Aguilar JE, editor. Formulation Tools for Pharmaceutical Development. Cambridge, UK: Elsevier (2013), ISBN: ISBN 978-1-907568-99-2. p. 7–37.
51. Arteta TA, Hameg R, Landin M, Gallego PP, Barreal ME. Artificial neural networks elucidated the essential role of mineral nutrients versus vitamins and plant growth regulators in achieving healthy micropropagated plants. Plants (2022) 11:1284. doi: 10.3390/plants11101284
52. García-Pérez P, Lozano-Milo E, Zhang L, Miras-Moreno B, Landin M, Lucini L, et al. Neurofuzzy logic predicts a finetuning metabolic reprogramming on elicited Bryophyllum PCSCs guided by salicylic acid. Front Plant Sci (2022) 13:991557. doi: 10.3389/fpls.2022.991557
53. Vapnik VN. The Nature of Statistical Learning Theory, 2nd ed.; Statistics for Engineering and Information Science. New York, NY, USA: Springer (2010).
54. Nezami-Alanagh E, Garoosi GA, Haddad R, Maleki S, Landin M, Gallego PP. Design of tissue culture media for efficient Prunus rootstock micropropagation using artificial intelligence models. Plant Cell Tissue Org Cult (2014) 117:349–59. doi: 10.1007/s11240-014-0444-1
55. Queralt M, Parladé J, Pera J, De Miguel AM. Seasonal dynamics of extraradical mycelium and mycorrhizas in a black truffle (Tuber melanosporum) plantation. Mycorrhiza (2017) 27:565–76. doi: 10.1007/s00572-017-0780-1
56. Vogt KA, Bloomfield J, Ammirati J, Ammirati SR, Carroll GC, Wicklow DT. Sporocarp production by Basidiomycetes, with emphasis on forest ecosystems. In: Carroll G, Wicklow D, editors. The Fungal Community: its organization and role in the ecosystem. New York: Marcel Dekker Inc. (1992). p. 563e581.
57. Hernández-Rodríguez M, de-Miguel S, Pukkala T, Oria-de-Rueda JA, Martín-Pinto P. Climate-sensitive models for mushroom yields and diversity in Cistus ladanifer scrublands. Agric For Meteorol (2015) 213:173–82. doi: 10.1016/j.agrformet.2015.07.001
58. de Aragón JM, Bonet JA, Fischer CR, Colinas C. Productivity of ectomycorrhizal and selected edible saprotrophic fungi in pine forests of the pre-Pyrenees mountains, Spain: predictive equations for forest management of mycological resources. Forest Ecol Manage (2007) 252(1-3):239–256.
59. Olano JM, Martínez-Rodrigo R, Altelarrea JM, Agreda T, Fernández-Toirán M, Garcia-Cervigón Al, et al. Primary productivity and climate control mushroom yields in Mediterranean pine forests. Agric For Meteorol (2020) 288:108015. doi: 10.1016/j.agrformet.2020.108015
60. Taye ZM, Martinez-Peńa F, Bonet JA, de Aragón JM, de-Migue S. Meteorological conditions and site characteristics driving edible mushroom production in Pinus pinaster forests of Central Spain. Fungal Ecol (2016) 23:30–41. doi: 10.1016/j.funeco.2016.05.008
61. Martínez-Peña F, Ágreda T, Águeda B, Ortega-Martínez P, Fernández-Toirán LM. Edible sporocarp production by age class in a Scots pine stand in northern Spain. Mycorrhiza (2012b) 22:167–74. doi: 10.1007/s00572-011-0389-8
62. Pereira G, Sepúlveda J, Novoa P, Pozo A, Atala C. Characterization of a relict native forest with annual fructification of the edible fungi Boletus loyo Phil. ex Speg. Quebracho (2016) 24):18–25.
63. Wu Z, Haack SE, Lin W, Li B, Wu L, Fang C, et al. Soil microbial community structure and metabolic activity of Pinus elliottii plantations across different stand ages in a subtropical area. PLoS One (2015) 10(8):e0135354. doi: 10.1371/journal.pone.0135354
64. Gao P, Zheng X, Wang L, Liu B, Zhang S. Changes in the soil bacterial community in a chronosequence of temperate walnut-based intercropping systems. Forests (2019) 10(4):299. doi: 10.3390/f10040299
65. Qu Z, Liu B, Ma Y, Sun H. Differences in bacterial community structure and potential functions among Eucalyptus plantations with different ages and species of trees. Appl Soil Ecol (2020) 149:103515. doi: 10.1016/j.apsoil.2020.103515
66. Liu J, Yang Z, Dang P, Zhu H, Gao Y, Ha VN, et al. Response of soil microbial community dynamics to Robinia pseudoacacia L. afforestation in the loess plateau: a chronosequence approach. Plant Soil (2018) 423(1):327–38. doi: 10.1007/s11104-017-3516-2
67. Mushinski RM, Phillips RP, Payne ZC, Abney RB, Jo I, Fei S, et al. Microbial mechanisms and ecosystem flux estimation for aerobic NOy emissions from deciduous forest soils. Proc Natl Acad Sci USA (2019) 116(6):2138–45. doi: 10.1073/pnas.1814632116
68. Mello A. State of the art of the research on Boletus edulis. In: Zambonelli A, Bonito G, editors. Edible Ectomycorrhizal Mushrooms. Soil Biology, vol. 34. Berlin, Heidelberg: Springer (2012). doi: 10.1007/978-3-642-33823-6_5
69. Mosquera-Losada MR, Freese D, Rigueiro-Rodríguez A. Carbon sequestration in European agroforestry systems. In: Kumar B, Nair P, editors. Carbon Sequestration Potential of Agroforestry Systems. Advances in Agroforestry, vol. 8. Dordrecht: Springer (2011). doi: 10.1007/978-94-007-1630-8_3
70. Nezami-Alanagh E, Garoosi GA, Maleki S, Landín M, Gallego PP. Predicting optimal in vitro culture medium for Pistacia vera micropropagation using neural networks models. Plant Cell, Tissue and Organ Culture (PCTOC), (2017) 129, 19–33. doi: 10.1007/s11240-016-1152-9
71. Gago J, Landín M, Gallego PP. A neurofuzzy logic approach for modeling plant processes: a practical case of in vitro direct rooting and acclimatization of Vitis vinifera. Plant Sci (2010) 179:241–9. doi: 10.1016/j.plantsci.2010.05.009
72. Bergemann SE, Largent DL. The site specific variables that correlate with the distribution of the Pacific Golden Chanterelle, Cantharellus formosus. For Ecol Manag (2000) 130:99–107. doi: 10.1016/S0378-1127(99)00177-2
Keywords: abiotic parameters, artificial intelligence, ectomycorrhiza, extra-radical mycelium, hybrid chestnuts, plant age, soil structure, water balance
Citation: Santolamazza-Carbone S, Iglesias-Bernabé L, Landin M, Rueda EB, Barreal ME and Gallego PP (2023) Artificial intelligence unveils key interactions between soil properties and climate factors on Boletus edulis and B. reticulatus mycelium in chestnut orchards of different ages. Front. Soil Sci. 3:1159793. doi: 10.3389/fsoil.2023.1159793
Received: 07 February 2023; Accepted: 20 September 2023;
Published: 06 October 2023.
Edited by:
Davey Jones, Bangor University, United KingdomReviewed by:
Hafiz Umair Masood Awan, University of Helsinki, FinlandXavier Parlade, Institute of Agrifood Research and Technology (IRTA), Spain
Copyright © 2023 Santolamazza-Carbone, Iglesias-Bernabé, Landin, Rueda, Barreal and Gallego. This is an open-access article distributed under the terms of the Creative Commons Attribution License (CC BY). The use, distribution or reproduction in other forums is permitted, provided the original author(s) and the copyright owner(s) are credited and that the original publication in this journal is cited, in accordance with accepted academic practice. No use, distribution or reproduction is permitted which does not comply with these terms.
*Correspondence: Serena Santolamazza-Carbone, anaphes@gmail.com; Pedro Pablo Gallego, pgallego@uvigo.es