- 1The Roslin Institute and Royal (Dick) School of Veterinary Studies, University of Edinburgh, Edinburgh, United Kingdom
- 2Agri-Food and Biosciences Institute, Belfast, United Kingdom
- 3Scotland's Rural College, Midlothian, United Kingdom
- 4Institute of Mathematical and Computer Sciences, University of São Paulo, São Paulo, Brazil
Host resistance and infectivity are genetic traits affecting infectious disease transmission. This Perspective discusses the potential exploitation of genetic variation in cattle infectivity, in addition to resistance, to reduce the risk, and prevalence of bovine tuberculosis (bTB). In bTB, variability in M. bovis shedding has been previously reported in cattle and wildlife hosts (badgers and wild boars), but the observed differences were attributed to dose and route of infection, rather than host genetics. This article addresses the extent to which cattle infectivity may play a role in bTB transmission, and discusses the feasibility, and potential benefits from incorporating infectivity into breeding programmes. The underlying hypothesis is that bTB infectivity, like resistance, is partly controlled by genetics. Identifying and reducing the number of cattle with high genetic infectivity, could reduce further a major risk factor for herds exposed to bTB. We outline evidence in support of this hypothesis and describe methodologies for detecting and estimating genetic parameters for infectivity. Using genetic-epidemiological prediction models we discuss the potential benefits of selection for reduced infectivity and increased resistance in terms of practical field measures of epidemic risk and severity. Simulations predict that adding infectivity to the breeding programme could enhance and accelerate the reduction in breakdown risk compared to selection on resistance alone. Therefore, given the recent launch of genetic evaluations for bTB resistance and the UK government's goal to eradicate bTB, it is timely to consider the potential of integrating infectivity into breeding schemes.
Introduction
Bovine tuberculosis (bTB) is a zoonotic disease, which can compromise both human health and international livestock trade. Zoonotic TB caused by Mycobacterium bovis, is responsible for an estimated 10–15% of human TB cases (1) and was estimated in 2016 as causing 12,500 deaths globally (2, 3). Addressing bTB infection in humans has been embedded within the United Nations Sustainable Development Goals 2016–2030 and World Health Organisation's (WHO) End TB Strategy framework, which employs a “One Health” approach aiming to end the global TB epidemic by 2030 (2–4).
In the UK, bTB has been the most pressing animal health problem, with financial losses amounting to over £175 m per annum (5). Tackling bTB has been a persistent challenge for the livestock industry, veterinary profession and policy-makers, and also the research community. The current national bTB eradication strategy involves the systematic testing of herds to identify and then remove infected cattle, and uses the Single Intradermal Comparative Cervical Test (SICCT), complemented by abattoir carcass inspections and, with increasing frequency, interferon-gamma testing. This surveillance regime has been successful in reducing disease spread in areas where bTB is prevalent and many EU countries and regions, including Scotland, have achieved Officially bTB Free (OTF) status (6). However, bTB persists in several regions (7) and herd incidence has increased in Wales, and also in High Risk and Edge areas in England (March 2018), despite the decrease in the overall herd incidence in England (8). Therefore, the continuing difficulties in eradicating bTB necessitate further exploration of additional disease control interventions that can complement existing strategies.
Selective breeding can complement classic disease control strategies, reducing the requirement for biosecurity measures and movement restrictions which have a major economic impact for herds undergoing a bTB breakdown (9, 10). Within the last few decades, breeding programmes (genetic and genomic selection) in livestock have achieved a remarkable improvement in production, e.g., milk yield in dairy cattle (11), and fitness traits such as fertility (12). Expanding the breeding objectives to include health and welfare traits offers new opportunities for disease control (10). The focus of genetic disease control so far has been on selection for improved resistance to becoming infected or diseased after exposure to pathogens. For example, by exploiting heritable genetic variation in disease resistance it has been possible to reduce mastitis incidence in cattle (13, 14) and mortality caused by infectious pancreatic necrosis in Atlantic salmon (15). Many studies have presented overwhelming evidence for genetic variation in resistance to bTB in cattle (16–19), which supports inclusion of bTB resistance in cattle breeding objectives in countries where bTB is prevalent. Recent efforts to combine national bTB surveillance and genetic data have enabled the publication of cattle evaluations for resistance to bTB in the UK (TB Advantage), which are currently used by farmers on a voluntary basis (20).
Veterinarians and epidemiologists have long considered reducing host infectiousness as an effective means to decrease disease transmission (21, 22). Infectiousness can be defined as the product of the contact rate between the infected individual and non-infected individuals, the propensity to transmit infection once infected (termed “infectivity” herein), and the duration of the infectious period (23, 24). For bTB, the contact rate between infected and non-infected herds is reduced by the movement restrictions imposed on herds with a bTB breakdown status. The duration of the infectious period is reduced by the test-and-cull policy which removes detectable infected animals, albeit with moderate animal-level sensitivity. In principle, infectivity can be reduced by vaccination, however, currently there are no vaccines (or subsequent tests) commercially available that allow differentiating between naturally infected and vaccinated individuals (i.e., a DIVA test) and would hence enable the safe use of vaccination for bTB control. Phenotypic variation in infectiousness is supported by numerous epidemiological studies showing that the Pareto principle commonly applies in epidemics, such that 20% of individuals are responsible for 80% of transmission events (22, 25–28). The individual differences in disease transmission are often attributed to different shedding patterns which may indicate phenotypic variation in host infectivity.
Emerging evidence suggests that infectivity can be, at least to some extent, under host genetic control (21, 29–33). Resistance and infectivity are thus two potentially distinct host genetic traits affecting disease transmission (see Table 1 for definitions and statistical and mechanistic distinctions between resistance and infectivity). Hence, if genetic variation in infectivity exists, can be estimated reliably, and has no significant impact on other desired traits, reduced infectivity could be a target for genetic improvement, in addition to disease resistance. Several authors have previously proposed (29, 34, 35) or demonstrated theoretically (36–39), that breeding livestock for both resistance and reduced infectivity can be an effective approach to reduce disease risk and prevalence.
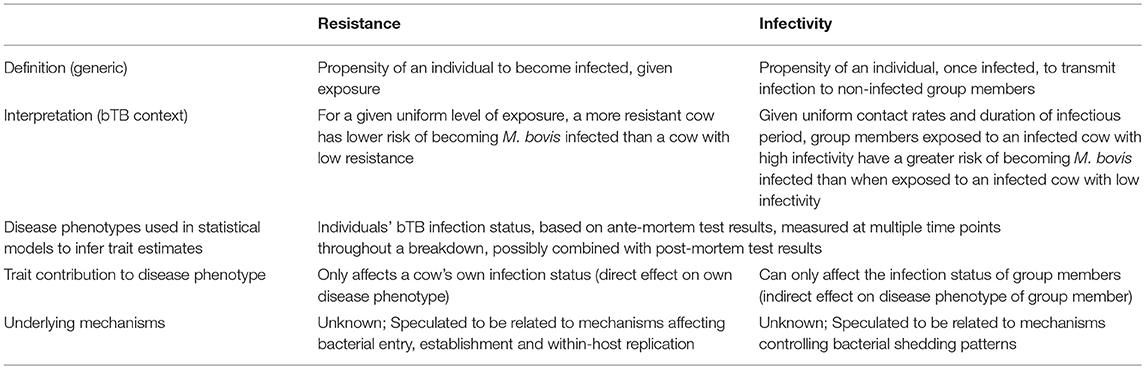
Table 1. Mechanistic and statistical distinction between resistance and infectivity in the context of bTB.
In this Perspectives article, we (a) review existing evidence that cattle may genetically differ in their bTB infectivity, (b) outline data and methodology requirements for estimating genetic infectivity for bTB, (c) discuss the benefits from considering infectivity in genetic evaluations, and (d) identify key challenges and future research opportunities for incorporating infectivity, in addition to resistance, in cattle breeding programmes aiming to reduce bTB prevalence.
Emerging Evidence That Infectivity Is Genetically Controlled
In bTB, differences in shedding patterns of M. bovis have been reported in various studies, but those have been mostly attributed to phenotypic variation rather than host genetics. For example, the number and frequency of episodes of shedding of M. bovis in cattle, were found to be dose- and infection route-dependent (40). Even amongst controlled experimentally infected calves, significant variation in shedding patterns have been described amongst individuals when presented with the same dose and infection route (41). In wild boars, the intensity and shedding of mycobacteria from the M. tuberculosis complex were found to affect the probability of new infections, while shedding intensity was shedding-route-dependant (42). In badgers, heterogeneity in shedding was found between different social groups (43) which may indicate family, and hence genetic, differences in shedding. Other studies found that the type of tuberculous lesions developed can affect the potential of infected individuals for transmitting infection (44), while evidence suggests that cattle with and without confirmed lesions may constitute, at least to some extent, genetically different subpopulations (45). Heterogeneity in lesion formation and stability of infected individuals suggests variation in mechanisms underlying infectivity rather than resistance, as less stable lesions are more prone to breaking open and thus to higher bacterial shedding. Human tuberculosis epidemiology is consistent with the existence of M. tuberculosis super-spreaders (46, 47), which may indicate the existence of individuals with high infectivity. In bTB epidemiological studies, the best model fit has been observed when accounting for M. bovis super-spreaders (48, 49), and super-spreading has been proposed for badgers and other wildlife species (7, 50, 51). However, there remains a controversy about the existence of super-spreaders in bTB (52).
In other diseases, genetic variation in infectivity was found to manifest itself in various ways, such as through genetic differences in the potential for, quantity and type of infectious material shed by infected hosts. For example, genetic variation was found in the fecal egg count of sheep artificially infected with the same gastro-intestinal parasite strain and dose (53, 54). Furthermore, in cases of hosts infected with more than one genotype of the same pathogen, host immune response can affect pathogen strain competition and diversity with subsequent effect on host infectivity (55). More direct evidence for genetic differences in host infectivity has been recently obtained from transmission experiments of viral and protozoal infections in fish (31, 33). In these studies fish were found to differ in their probability of becoming diseased depending on the family or genotype of the initially infected fish that seeded the infection.
In summary, phenotypic variation in host infectivity is a common phenomenon, and for some diseases, this was shown to encompass genetic variation. In bTB, phenotypic variation in M. bovis shedding has been demonstrated by a few studies, but the extent to which this variation is due to cattle genetics is currently unknown. It is possible that host disease resistance and infectivity share some common genetic pathways controlling pathogen replication and consequently shedding (Table 1). This raises the question as to what extent bTB infectivity and resistance are genetically correlated, and how combined resistance and infectivity can affect bTB transmission.
Data and Methodology Requirements for Estimating Genetic Effects for bTB Infectivity
Infectivity, referring to an individual's ability to transmit infection (Table 1), is difficult to measure directly from field data where transmission routes (who-infects-whom) are difficult to trace and many transmissions are not observed or detected. Infectivity phenotypes can be obtained by measuring individual shedding rates (56). Measuring shedding has only been practical in special cases, e.g., fecal egg count for nematodes, and is very challenging if carried out routinely on the scale of sample sizes needed to inform breeding programmes.
However, shedding is not the only phenotype that can be used to track infectivity. Instead, it is possible to estimate genetic variation in infectivity by monitoring the progression of infection, i.e., the infection status of individuals, in different herds over time. Recently, novel inference methods have been developed to simultaneously estimate and untangle genetic effects for resistance and infectivity from longitudinal data of individual infection status (Table 1) (34, 36, 57, 58).
Common requirements for estimating genetic variation in infectivity using these novel methods are that (i) genetically related individuals are spread over different epidemics (herds/breakdowns), (ii) individual epidemics occur in “closed” groups with minimum between-group transmission, and (iii) individual infection times differ, and are known or can be inferred. These requirements appear to be satisfied by bTB. The UK national bTB eradication scheme has generated systematic repeated records of SICCT test results for a large number of herds containing related animals. In addition, due to movement restrictions imposed on herds undergoing a breakdown, herds can be considered as closed groups during the breakdown period, and data collected can be used to infer infectivity. Although the exact time of cattle infection with M. bovis is unknown, the repeated SICCT testing during this period provides longitudinal measurements of individuals' infection status, from which infection times can be inferred using Bayesian inference and data augmentation methods (34, 58).
It remains to be tested with field bTB data, how various sources of uncertainty affect genetic infectivity estimates. For example, these methods assume knowledge of the true infection status of an individual, which raises the question whether SICCT and other monitoring records are appropriate for this purpose. Of these, SICCT is the most commonly available measurement but its relatively poor sensitivity is well documented, i.e., its ability to correctly identify infected individuals; published sensitivity estimates range from 26 to 91% (59–63). Whether the test result reflects the true infection status of an animal is under on-going investigation within the bTB research community. Nevertheless, the positive predictive value of the test, i.e., the proportion of individuals that test positively and truly have the disease, is sufficiently high that false positives are likely to be few amongst the observed reactors. The specificity of SICCT in officially tuberculosis free herds has been estimated to be 99.98% (64). Therefore, already recorded SICCT phenotypes can provide information to search for genetic effects associated with infectivity. Including information from culling associated with SICCT testing has proven adequate for obtaining sufficiently accurate estimated breeding values (EBVs) for bTB resistance in the current bTB genetic evaluations (20). Expanding these evaluations to consider both resistance and infectivity would be expected to be beneficial primarily in high bTB risk areas, where the positive predictive value of SICCT is higher due to the higher disease prevalence (65).
Expected Benefits From Implementing Infectivity as an Additional Disease Phenotype in Genetic Evaluations for bTB Control
bTB has been a seemingly intractable problem in the UK in recent decades and understanding how cattle genetics influences bTB spread is important for eradication. Under the hypothesis that some cattle infected with bTB are genetically more infectious than others, reducing the occurrence of cattle with higher genetic infectivity through selective breeding would (i) reduce bTB transmission between cattle by removing highly infectious individuals comprising a major risk factor for herds, (ii) reduce shedding of M. bovis and hence reduce a major source of infection for the environment (30) and wildlife vectors, e.g., indirectly reduce bTB spill over to badgers. Badgers are susceptible to M. bovis infection and reducing infectivity in cattle should also reduce the pathogen burden in the environment (shedding e.g., in milk, feces, air, etc.).
If we were to estimate infectivity effects, it would enable breeders and farmers to select bulls whose offspring are not only expected to be less likely to become infected (more resistant), but also less likely to transmit bTB infection, if infected. Selection on breeding values for resistance and infectivity is expected to reduce the population R0 (37, 39), i.e., the expected number of secondary cases produced by a typical infectious individual in a completely susceptible population (66), hence contributing to disease control. However, bTB transmission occurs within and across different species. Hence the overall bTB R0 is composed of R0cattle, R0badgers, R0cattle−to−badgers, and R0badgers−to−cattle (67, 68), where the cross-species relationships warrant further investigation. Reducing cattle infectivity would be expected to reduce R0cattle and R0cattle−to−badgers, as well as the infection feedback loop from R0badgers−to−cattle. A small reduction in each component may suffice to bring the overall R0 to below 1 and make the risk of new breakdowns negligible (10, 69). Investigating individual differences in infectivity might shed light on the variation observed in herd bTB prevalence and the relationship of infectivity with detectable bTB status, and why in some herds bTB persists with recurrent/chronic breakdowns, while other herds appear to be able to rapidly clear infection. Investigating variation in infectivity would also shed light on the weather bTB super-spreaders exist, as animals at the tail of the distribution would be “super spreaders” relative to all others, and what is their role in bTB spread.
A genetic-epidemiological simulation model can be used to assess the relative benefits of using a selection index that includes both resistance and infectivity compared to selecting on resistance alone. For this purpose we extended a stochastic epidemiological Susceptible-Latent-Infectious-Test sensitive model for bTB that originally assumed genetic variation only in resistance (70) with parameter values from the British genetic evaluations for bTB resistance (20) to incorporate hypothetical additional variation in infectivity (34, 38). We then used this model to simulate bTB spread in each herd and predict the impact of selection on breakdown risk, defined by the proportion of simulated bTB epidemics where infected index cases generated secondary cases. This is pertinent as field characteristics of epidemics often show curvilinear responses to control strategies. We found that when adding infectivity alongside resistance to the breeding objective, the reduction of the risk of a bTB breakdown was substantial and more pronounced in the early generations (Figure 1) (34, 38). For example, assuming 50% selection on sires, moderate heritabilities and prediction accuracies for resistance and infectivity, and zero correlation between resistance and infectivity, the relative epidemic risk at generation 5 was ~0.2 with selection for resistance alone, but < 0.1 for combined selection for both resistance and infectivity, even when external sources of infection were included (Figure 1). These simulations designed as proof-of-principle, provide a crude estimate of the predicted effects which will depend on e.g., the magnitude of the genetic variance in the objective traits and various demographic factors. However, these findings are indicative that by targeting both resistance and infectivity in combination, disease control benefits can be of larger magnitude (i.e., more effective) and more responsive (i.e., quicker to see results) (38).
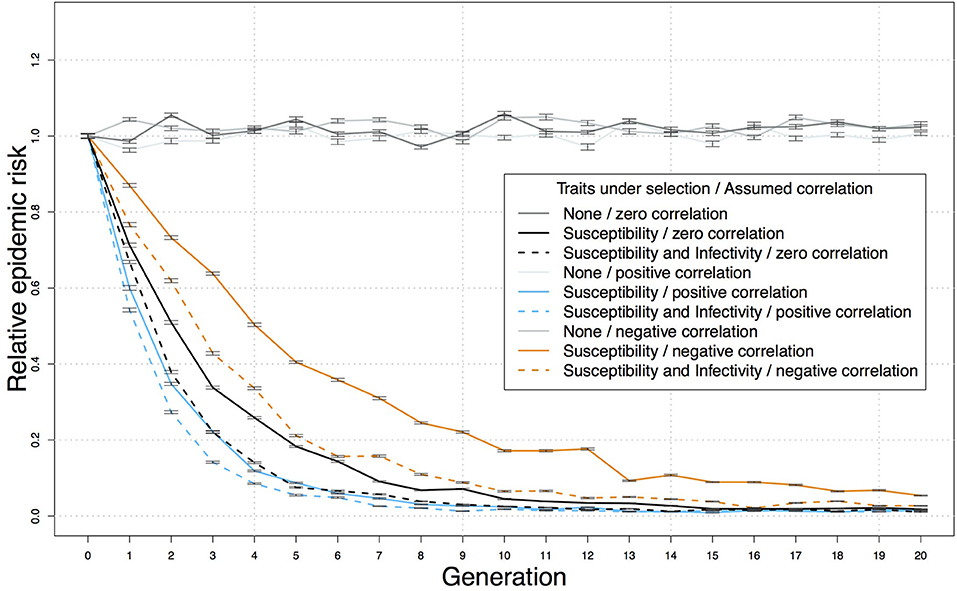
Figure 1. Reduction in the predicted relative risk of bTB breakdown in a herd, following introduction of an infected cow, over 20 generations of selection for resistance and lower infectivity, or for resistance alone. Predictions from a stochastic genetic-epidemiological simulation model incorporating genetic variation in resistance and infectivity (38), comprising populations of 10,000 half-sib individuals randomly distributed into 100 herds of the same size. Means and standard errors were obtained over 50 replicates. The parameter values were based on the British genetic evaluations for bTB resistance (20) and a previous genetic-epidemiological model (70) as follows: bTB testing intervals of 60 days, SICCT sensitivity of 60%, 50% selection on the sires, accuracy of 0.5 and latent heritability of 0.6 [corresponding to heritability for the observed indicator traits of below 0.2 (70)] for both resistance and infectivity, economic values of one for both traits, and external force of infection of 5 × 10−5 (70). Each breakdown was initiated by one infectious individual, and variance and accuracy were assumed constant over generations. The correlation between resistance and infectivity was assumed to be zero, 0.5 or −0.5.
The epidemiological benefits and additional gain expected from adding infectivity to the breeding goal depends on its genetic correlation with other traits of economic interest. A classic example of the impact of adverse correlated responses, is the reduction of cattle fertility following selection on milk yield, due to adverse genetic correlation with milk yield (12). Based on estimated genetic correlations among traits, genetic selection for enhanced bTB resistance is not expected to adversely affect other traits in the breeding goal (16, 20), and was found to be unlikely to change the probability of correctly identifying non-infected animals via the SICCT diagnostic test (71). However, genetic correlations between resistance and infectivity may affect the outcome of genetic bTB control. Indeed, based on the genetic-epidemiological bTB model described above (38) the strongest benefit of adding infectivity into the selection criterion compared to selecting on resistance alone is observed in the case of an adverse genetic correlation between the traits (Figure 1), and this is because the progress achieved by breeding schemes targeting only resistance would be delayed due to an indirect increase in infectivity. Considering breeding values for infectivity can help alleviate this delay and accelerate progress toward disease eradication (38).
Future Opportunities and Challenges
In principle, bTB surveillance schemes such as those implemented in the UK, RoI, and NZ, would permit researchers to pioneer the estimation of infectivity genetic effects without the need to collect new data. Current genetic evaluations for bTB resistance (20) use phenotype and pedigree information, with increasing amounts of genomic data. As the incidence of bTB reduces, the information obtained from pedigrees will also reduce. Therefore, genomic information becomes increasingly vital, and high-density genomic data can now be obtained cost-effectively by genotype imputation (72, 73). It has been shown that genomic prediction for bTB resistance using genomic information is feasible (18), and prediction accuracies can be improved by using larger training sets of genotyped animals and genome sequence information. This is pertinent for infectivity, as it has been shown in simulation studies that the prediction accuracy for infectivity is expected, at least initially, to be modest (34). This genomic information also allows investigation of the genetic architecture of bTB infectivity and the search for causal variants.
Challenges arising in the analysis of bTB data to uncover genetic infectivity include accounting for multiple and poorly understood transmission routes of M. bovis, and obtaining more reliable disease phenotypes. Separating the effects of the infectious dose from host response is extremely challenging in field situations where exposure may not be uniform (74). However, sophisticated Bayesian inference methods, coupled with phylodynamics and M. bovis genome sequence information, can help to infer transmission routes and obtain information on the networks of who-infects-whom (49, 75–78), which is useful for predicting infectivity (79). More reliable disease phenotypes could be obtained by quality control on individual tester performance to improve the consistency of data recording on the farms (80), and by developing improved diagnostics. Machine learning techniques (Deep Learning) hold the promise that sufficiently accurate disease phenotypes can be obtained in a cost-effective manner for large sample sizes using routinely collected mid infra-red spectral data from milk recording (Coffey M. personal communication October 2018). Together, continuous development of improved diagnostic and modeling tools provide promising prospects for genetic bTB control.
Conclusion
Host infectivity is an important trait for disease transmission and emerging evidence suggests that it may be under genetic control to some extent; however, the role of genetic infectivity of cattle in bTB spread remains to be explored. Infectivity might be difficult to capture from noisy field data; however, the UK bTB surveillance database and newly developed statistical methods provide the opportunity to estimate genetic effects for infectivity. Exploiting genetic variation in infectivity as a complementary bTB control method is a low-investment high-return approach, as it can be developed at minimal cost using data already available. Simulation studies suggest that breeding for both disease resistance and infectivity can complement and substantially enhance current disease control approaches toward bTB eradication. Using UK data to determine genetic regulation of disease transmission can create a platform for controlling bTB in other countries and for controlling other infectious diseases.
Author Contributions
ST, AA, RS, GB, JW, and AD-W conceived the perspectives. ST drafted the manuscript, designed and carried out the simulations and participated in the interpretation of findings. JW and AD-W contributed to the initial draft and simulation designs. All authors contributed to later versions of the manuscript, read and approved the final manuscript.
Conflict of Interest Statement
The authors declare that the research was conducted in the absence of any commercial or financial relationships that could be construed as a potential conflict of interest.
Acknowledgments
This work was carried out with funding from the Biotechnology and Biological Sciences Research Council Institute Strategic Programme grants BB/J004235/1 (ISP1) and BB/P013740/1 (ISP2) (OA, AD-W, GB and JW), and the European Union FP7 project FISHBOOST (KBBE - 7-613611) (ST). GB was also supported by the Rural and Environment Science and Analytical Services Division of the Scottish Government.
References
1. Michel AL, Muller B, van Helden PD. Mycobacterium bovis at the animal-human interface: a problem, or not? Vet Microbiol. (2010) 140:371–81. doi: 10.1016/j.vetmic.2009.08.029
2. World Health Organization. Roadmap for Zoonotic Tuberculosis (2017). Available online at: http://www.fao.org/3/a-i7807e.pdf
3. Olea-Popelka F, Fujiwara PI. Building a multi-institutional and interdisciplinary team to develop a zoonotic tuberculosis roadmap. Front Public Health (2018) 6:167. doi: 10.3389/fpubh.2018.00167
4. Dean AS, Forcella S, Olea-Popelka F, Idrissi AE, Glaziou P, Benyahia A, et al. A roadmap for zoonotic tuberculosis: a one health approach to ending tuberculosis. Lancet Infect Dis. (2018) 18:137–8. doi: 10.1016/S1473-3099(18)30013-6
5. Abernethy DA, Upton P, Higgins IM, McGrath G, Goodchild AV, Rolfe SJ, et al. Bovine tuberculosis trends in the UK and the Republic of Ireland, 1995–2010. Vet Rec. (2013) 172:312. doi: 10.1136/vr.100969
6. DEFRA. The Strategy for Achieving Officially Bovine Tuberculosis Free Status for England (2014). Available online at: https://assets.publishing.service.gov.uk/government/uploads/system/uploads/attachment_data/file/300447/pb14088-bovine-tb-strategy-140328.pdf
7. Allen AR, Skuce RA, Byrne AW. Bovine tuberculosis in Britain and Ireland - a perfect storm? The confluence of potential ecological and epidemiological impediments to controlling a chronic infectious disease. Front Vet Sci. (2018) 5:109. doi: 10.3389/fvets.2018.00109
8. DEFRA. Quarterly Publication of National Statistics on the Incidence and Prevalence of Tuberculosis (Tb) in Cattle in Great Britain – To End March 2018. (2018) Available online at: https://www.gov.uk/government/statistics/incidence-of-tuberculosis-tb-in-cattle-in-great-britain
9. Bishop SC, Woolliams JA. Genomics and disease resistance studies in livestock. Livest Sci. (2014) 166:190–8. doi: 10.1016/j.livsci.2014.04.034
10. Bishop S, Axford R, Nicholas F, Owen J. Breeding for Disease Resistance in Farm Animals. 3rd Ed. Wallingford: CABI Publishing (2010).
11. Weigel KA, VanRaden PM, Norman HD, Grosu H. A 100-year review: methods and impact of genetic selection in dairy cattle-from daughter-dam comparisons to deep learning algorithms. J Dairy Sci. (2017) 100:10234–50. doi: 10.3168/jds.2017-12954
12. Wall E, Brotherstone S, Woolliams JA, Banos G, Coffey MP. Genetic evaluation of fertility using direct and correlated traits. J Dairy Sci. (2003) 86:4093–102. doi: 10.3168/jds.S0022-0302(03)74023-5
13. Heringstad B, Klemetsdal G, Ruane J. Selection for mastitis resistance in dairy cattle: a review with focus on the situation in the Nordic countries. Livest Prod Sci. (2000) 64:95–106. doi: 10.1016/S0301-6226(99)00128-1
14. Martin P, Barkema HW, Brito LF, Narayana SG, Miglior F. Symposium review: novel strategies to genetically improve mastitis resistance in dairy cattle. J Dairy Sci. (2018) 101:2724–36. doi: 10.3168/jds.2017-13554
15. Houston R, Bishop SC, Woolliams J, Haley C. Marker-Assisted Selection to breed for resistance to Infectious Pancreatic Necrosis in Salmon Research Excellence Framework (REF), impact case studies (2014). Available online at: http://impact.ref.ac.uk/CaseStudies/CaseStudy.aspx?Id=23913
16. Brotherstone S, White IM, Coffey M, Downs SH, Mitchell AP, Clifton-Hadley RS, et al. Evidence of genetic resistance of cattle to infection with Mycobacterium bovis. J Dairy Sci. (2010) 93:1234–42. doi: 10.3168/jds.2009-2609
17. Bermingham ML, Bishop SC, Woolliams JA, Pong-Wong R, Allen AR, McBride SH, et al. Genome-wide association study identifies novel loci associated with resistance to bovine tuberculosis. Heredity (2014) 112:543–51. doi: 10.1038/hdy.2013.137
18. Tsairidou S, Woolliams JA, Allen AR, Skuce RA, McBride SH, Wright DM, et al. Genomic prediction for tuberculosis resistance in dairy cattle. PLoS ONE (2014) 9:e96728. doi: 10.1371/journal.pone.0096728
19. Woolliams J, Brotherstone S, Coffey M. A Preliminary Analysis of Existing Data to Provide Evidence of a Genetic Basis for Resistance of Cattle to Infection with M. bovis and for Reactivity to Currently Used Immunological Diagnostic Tests. Defra, London, UK (2008).
20. Banos G, Winters M, Mrode R, Mitchell AP, Bishop SC, Woolliams JA, et al. Genetic evaluation for bovine tuberculosis resistance in dairy cattle. J Dairy Sci. (2017) 100:1272–81. doi: 10.3168/jds.2016-11897
21. Geenen PL, Van der Meulen J, Bouma A, De Jong MCM. Estimating transmission parameters of F4+ E. coli for F4-receptor-positive and-negative piglets: one-to-one transmission experiment. Epidemiol Infect. (2004) 132:1039–48.
22. Lloyd-Smith JO, Schreiber SJ, Kopp PE, Getz WM. Superspreading and the effect of individual variation on disease emergence. Nature (2005) 438:355–9. doi: 10.1038/nature04153
23. Keeling MJ, Rohani P. Modeling Infectious Diseases in Humans and Animals. Woodstock, NJ; Oxfordshire: Princeton University Press (2008).
24. Lipschutz-Powell D, Woolliams JA, Doeschl-Wilson AB. A unifying theory for genetic epidemiological analysis of binary disease data. Genet Sel Evol. (2013) 46:15. doi: 10.1186/1297-9686-46-15
25. Woolhouse MEJ, Dye C, Etard J-F, Smith T, Charlwood JD, Garnett GP, et al. Heterogeneities in the transmission of infectious agents: implications for the design of control programs. Proc Natl Acad Sci USA. (1997) 94:338–42.
26. Jankowski MD, Williams CJ, Fair JM, Owen JC. Birds shed RNA-viruses according to the pareto principle. PLoS ONE (2013) 8:e72611. doi: 10.1371/journal.pone.0072611
27. Gopinath S, Lichtman JS, Bouley DM, Elias JE, Monack DM. Role of disease-associated tolerance in infectious superspreaders. Proc Natl Acad Sci USA. (2014) 111:15780–5. doi: 10.1073/pnas.1409968111
28. Shen Z, Ning F, Zhou W, He X, Lin C, Chin DP, et al. Superspreading SARS events, Beijing, 2003. Emerg Infect Dis. (2004) 10:256–60. doi: 10.3201/eid1002.030732
29. Raszek MM, Guan LL, Plastow GS. Use of genomic tools to improve cattle health in the context of infectious diseases. Front Genet. (2016) 7:30. doi: 10.3389/fgene.2016.00030
30. Doeschl-Wilson AB, Davidson R, Conington J, Roughsedge T, Hutchings MR, Villanueva B. Implications of host genetic variation on the risk and prevalence of infectious diseases transmitted through the environment. Genetics (2011) 188:683–93. doi: 10.1534/genetics.110.125625
31. Doeschl-Wilson A, Anacleto O, Nielsen HM, Karlsson-Drangsholt TM, Lillehammer M, Gjerde B. New opportunities for genetic disease control: beyond disease resistance. In: Proceedings of the World Congress on Genetics Applied to Livestock Production (Auckland) (2018).
32. Lyall J, Irvine RM, Sherman A, McKinley TJ, Nunez A, Purdie A, et al. Suppression of avian influenza transmission in genetically modified chickens. Science (2011) 331:223–6. doi: 10.1126/science.1198020
33. Anacleto O, Cabaleiro S, Villanueva B, Saura M, Houston RD, Woolliams JA, et al. Genetic differences in host infectivity affect disease spread and survival in epidemics. biorxiv[Preprint]. (2018). doi: 10.1101/483602
34. Anacleto O, Garcia-Cortés LA, Lipschutz-Powell D, Woolliams JA, Doeschl-Wilson AB. A novel statistical model to estimate host genetic effects affecting disease transmission. Genetics (2015) 201:871–84. doi: 10.1534/genetics.115.179853
35. Brooks-Pollock E, de Jong MCM, Keeling MJ, Klinkenberg D, Wood JLN. Eight challenges in modelling infectious livestock diseases. Epidemics (2015) 10:1–5. doi: 10.1016/j.epidem.2014.08.005
36. Biemans F, de Jong MCM, Bijma P. A model to estimate effects of SNPs on host susceptibility and infectivity for an endemic infectious disease. Genet Sel Evol. (2017) 49:53. doi: 10.1186/s12711-017-0327-0
37. Lipschutz-Powell D, Woolliams JA, Bijma P, Doeschl-Wilson AB. Indirect genetic effects and the spread of infectious disease: are we capturing the full heritable variation underlying disease prevalence? PLoS ONE (2012) 7:e39551. doi: 10.1371/journal.pone.0039551
38. Tsairidou S, Anacleto O, Raphaka K, Sanchez-Molano E, Banos G, Woolliams JA, et al. Enhancing genetic disease control by selecting for lower host infectivity. In: Proceedings of the World Congress on Genetics Applied to Livestock Production (Auckland) (2018).
39. Anche M, Jong M, Bijma P. On the definition and utilization of heritable variation among hosts in reproduction ratio R0 for infectious diseases. Heredity (2014) 113:364–74. doi: 10.1038/hdy.2014.38
40. McCorry T, Whelan AO, Welsh MD, McNair J, Walton E, Bryson DG, et al. Shedding of Mycobacterium bovis in the nasal mucus of cattle infected experimentally with tuberculosis by the intranasal and intratracheal routes. Vet Rec. (2005) 157:613–8. doi: 10.1136/vr.157.20.613
41. Kao RR, Gravenor MB, Charleston B, Hope JC, Martin M, Howard CJ. Mycobacterium bovis shedding patterns from experimentally infected calves and the effect of concurrent infection with bovine viral diarrhoea virus. J R Soc Interface (2007) 4:545–51. doi: 10.1098/rsif.2006.0190
42. Barasona JA, Torres MJ, Aznar J, Gortazar C, Vicente J. DNA detection reveals Mycobacterium tuberculosis complex shedding routes in its wildlife reservoir the Eurasian wild boar. Transbound Emerg Dis. (2017) 64:906–15. doi: 10.1111/tbed.12458
43. King HC, Murphy A, James P, Travis E, Porter D, Sawyer J, et al. Performance of a noninvasive test for detecting Mycobacterium bovis shedding in European badger (Meles meles) populations. J Clin Microbiol. (2015) 53:2316–23. doi: 10.1128/JCM.00762-15
44. Shaler CR, Horvath CN, Jeyanathan M, Xing Z. Within the enemy's camp: contribution of the granuloma to the dissemination, persistence and transmission of Mycobacterium tuberculosis. Front Immunol. (2013) 4:30. doi: 10.3389/fimmu.2013.00030
45. Wilkinson S, Bishop SC, Allen AR, McBride SH, Skuce RA, Bermingham M, et al. Fine-mapping host genetic variation underlying outcomes to Mycobacterium bovis infection in dairy cows. BMC Genomics (2017) 18:477. doi: 10.1186/s12864-017-3836-x
46. Walker TM, Ip CL, Harrell RH, Evans JT, Kapatai G, Dedicoat MJ, et al. Whole-genome sequencing to delineate Mycobacterium tuberculosis outbreaks: a retrospective observational study. Lancet Infect Dis. (2013) 13:137–46. doi: 10.1016/S1473-3099(12)70277-3
47. Ypma RJ, Altes HK, van Soolingen D, Wallinga J, van Ballegooijen WM. A sign of superspreading in tuberculosis: highly skewed distribution of genotypic cluster sizes. Epidemiology (2013) 24:395–400. doi: 10.1097/EDE.0b013e3182878e19
48. Trewby H, Wright DM, Skuce RA, McCormick C, Mallon TR, Presho EL, et al. Relative abundance of Mycobacterium bovis molecular types in cattle: a simulation study of potential epidemiological drivers. BMC Vet Res. (2017) 13:268. doi: 10.1186/s12917-017-1190-5
49. O'Hare A, Orton RJ, Bessell PR, Kao RR. Estimating epidemiological parameters for bovine tuberculosis in British cattle using a Bayesian partial-likelihood approach. Proc Biol Sci. (2014) 281:20140248. doi: 10.1098/rspb.2014.0248
50. Santos N, Almeida V, Gortázar C, Correia-Neves M. Patterns of Mycobacterium tuberculosis-complex excretion and characterization of super-shedders in naturally-infected wild boar and red deer. Vet Res. (2015) 46:129. doi: 10.1186/s13567-015-0270-4
51. Delahay RJ, Langton S, Smith GC, Clifton-Hadley RS, Cheeseman CL. The spatio-temporal distribution of Mycobacterium bovis (bovine tuberculosis) infection in a high-density badger population. J Anim Ecol. (2000) 69:428–41. doi: 10.1046/j.1365-2656.2000.00406.x
52. Bourne FJ, Donnelly CA, Cox DR, Gettinby G, McInerney JP, Morrison WI, et al. Re: TB policy and the ISG's findings. Vet Rec. (2007) 161:633–5. doi: 10.1136/vr.161.18.633-b
53. Bishop S, Jackson F, Coop R, Stear M. Genetic parameters for resistance to nematode infections in Texel lambs and their utility in breeding programmes. Anim Sci. (2004) 78:185–94. doi: 10.1017/S1357729800053972
54. Sallé G, Jacquiet P, Gruner L, Cortet J, Sauvé C, Prévot F, et al. A genome scan for QTL affecting resistance to Haemonchus contortus in sheep. J Anim Ecol. (2012) 90:4690–705. doi: 10.2527/jas.2012-5121
55. Read AF, Taylor LH. The ecology of genetically diverse infections. Science (2001) 292:1099–102. doi: 10.1126/science.1059410
56. Charpin C, Mahe S, Keranflec'h A, Belloc C, Cariolet R, Le Potier MF, et al. Infectiousness of pigs infected by the porcine reproductive and respiratory syndrome virus (PRRSV) is time-dependent. Vet Res. (2012) 43:69. doi: 10.1186/1297-9716-43-69
57. Anche MT, Bijma P, De Jong MC. Genetic analysis of infectious diseases: estimating gene effects for susceptibility and infectivity. Genet Sel Evol. (2015) 47:85. doi: 10.1186/s12711-015-0163-z
58. Pooley C, Bishop S, Marion G. Estimation of single locus effects on susceptibility, infectivity and recovery rates in an epidemic using temporal data. In Proceedings of the 10th World Congress on Genetics Applied to Livestock Production (Vancouver, BC)(2014).
59. de la Rua-Domenech R, Goodchild AT, Vordermeier HM, Hewinson RG, Christiansen KH, Clifton-Hadley RS. Ante mortem diagnosis of tuberculosis in cattle: a review of the tuberculin tests, gamma-interferon assay and other ancillary diagnostic techniques. Res Vet Sci. (2006) 81:190–210. doi: 10.1016/j.rvsc.2005.11.005
60. Clegg TA, Duignan A, Whelan C, Gormley E, Good M, Clarke J, et al. Using latent class analysis to estimate the test characteristics of the gamma-interferon test, the single intradermal comparative tuberculin test and a multiplex immunoassay under Irish conditions. Vet Microbiol. (2011) 151:68–76. doi: 10.1016/j.vetmic.2011.02.027
61. Bermingham ML, More SJ, Good M, Cromie AR, Higgins IM, Brotherstone S, et al. Genetics of tuberculosis in Irish Holstein-Friesian dairy herds. J Dairy Sci. (2009) 92:3447–56. doi: 10.3168/jds.2008-1848
62. Nunez-Garcia J, Downs SH, Parry JE, Abernethy DA, Broughan JM, Cameron AR, et al. Meta-analyses of the sensitivity and specificity of ante-mortem and post-mortem diagnostic tests for bovine tuberculosis in the UK and Ireland. Prev Vet Med. (2018) 153:94–107. doi: 10.1016/j.prevetmed.2017.02.017
63. Lahuerta-Marin A, Milne MG, McNair J, Skuce RA, McBride SH, Menzies FD, et al. Bayesian latent class estimation of sensitivity and specificity parameters of diagnostic tests for bovine tuberculosis in chronically infected herds in Northern Ireland. Vet J. (2018) 238:15–21. doi: 10.1016/j.tvjl.2018.04.019
64. Goodchild AV, Downs SH, Upton P, Wood JLN, de la Rua-Domenech R. Specificity of the comparative skin test for bovine tuberculosis in Great Britain. Vet Rec. (2015) 177:258. doi: 10.1136/vr.102961
65. Drewe JA. Bovine tuberculosis: how likely is a skin test reactor to be uninfected? Vet Rec. (2015) 177:256–7. doi: 10.1136/vr.h4760
66. Diekmann O, Heesterbeek JAP, Metz JAJ. On the definition and the computation of the basic reproduction ratio R0 in models for infectious diseases in heterogeneous populations. J Math Biol. (1990) 28:365–82. doi: 10.1007/BF00178324
67. Brooks-Pollock E, Wood JLN. Eliminating bovine tuberculosis in cattle and badgers: insight from a dynamic model. Proc Biol Sci. (2015) 282:20150374. doi: 10.1098/rspb.2015.0374
68. Heesterbeek JA, Roberts MG. The type-reproduction number T in models for infectious disease control. Math Biosci. (2007) 206:3–10. doi: 10.1016/j.mbs.2004.10.013
69. Aznar I, Frankena K, Byrne A, More S, De Jong M. Infection dynamics and effective control options of tuberculosis in cattle and badgers. In: 6th International M. bovis Conference. Cardiff (2014).
70. Raphaka K. Genetics of Bovine Tuberculosis Resistance in Dairy Cattle. Ph.D. Thesis, The University of Edinburgh (2018).
71. Tsairidou S, Brotherstone S, Coffey M, Bishop SC, Woolliams JA. Quantitative genetic analysis of the bTB diagnostic single intradermal comparative cervical test (SICCT). Genet Sel Evol. (2016) 48:90. doi: 10.1186/s12711-016-0264-3
72. Burdick JT, Chen W-M, Abecasis GR, Cheung VG. In silico method for inferring genotypes in pedigrees. Nat Genet. (2006) 38:1002–4. doi: 10.1038/ng1863
73. Calus MP, Bouwman AC, Hickey JM, Veerkamp RF, Mulder HA. Evaluation of measures of correctness of genotype imputation in the context of genomic prediction: a review of livestock applications. Animal (2014) 8:1743–53. doi: 10.1017/S1751731114001803
74. Bishop SC, Doeschl-Wilson AB, Woolliams JA. Uses and implications of field disease data for livestock genomic and genetics studies. Front Genet. (2012) 3:114. doi: 10.3389/fgene.2012.00114
75. Brooks-Pollock E, Roberts GO, Keeling MJ. A dynamic model of bovine tuberculosis spread and control in Great Britain. Nature (2014) 511:228–31. doi: 10.1038/nature13529
76. Green DM, Kiss IZ, Mitchell AP, Kao RR. Estimates for local and movement-based transmission of bovine tuberculosis in British cattle. Proc Biol Sci. (2008) 275:1001–5. doi: 10.1098/rspb.2007.1601
77. Kao RR, Haydon DT, Lycett SJ, Murcia PR. Supersize me: how whole-genome sequencing and big data are transforming epidemiology. Trends Microbiol. (2014) 22:282–91. doi: 10.1016/j.tim.2014.02.011
78. Lam TT-Y, Wang J, Shen Y, Zhou B, Duan L, Cheung C-L, et al. The genesis and source of the H7N9 influenza viruses causing human infections in China. Nature (2013) 502:241–4. doi: 10.1038/nature12515
79. Lipschutz-Powell D, Woolliams JA, Bijma P, Pong-Wong R, Bermingham ML, Doeschl-Wilson AB. Bias, accuracy, and impact of indirect genetic effects in infectious diseases. Front Genet. (2012) 3:215. doi: 10.3389/fgene.2012.00215
Keywords: disease resistance, disease control, animal breeding, infectivity, bovine Tuberculosis
Citation: Tsairidou S, Allen A, Banos G, Coffey M, Anacleto O, Byrne AW, Skuce RA, Glass EJ, Woolliams JA and Doeschl-Wilson AB (2018) Can We Breed Cattle for Lower Bovine TB Infectivity? Front. Vet. Sci. 5:310. doi: 10.3389/fvets.2018.00310
Received: 22 August 2018; Accepted: 22 November 2018;
Published: 07 December 2018.
Edited by:
Flavie Vial, Animal and Plant Health Agency, United KingdomReviewed by:
Douwe Bakker, Universidad Complutense de Madrid, SpainHeinzpeter Schwermer, Federal Food Safety and Veterinary Office, Switzerland
Copyright © 2018 Tsairidou, Allen, Banos, Coffey, Anacleto, Byrne, Skuce, Glass, Woolliams and Doeschl-Wilson. This is an open-access article distributed under the terms of the Creative Commons Attribution License (CC BY). The use, distribution or reproduction in other forums is permitted, provided the original author(s) and the copyright owner(s) are credited and that the original publication in this journal is cited, in accordance with accepted academic practice. No use, distribution or reproduction is permitted which does not comply with these terms.
*Correspondence: Smaragda Tsairidou, c21hcmFnZGEudHNhaXJpZG91QHJvc2xpbi5lZC5hYy51aw==