1
Center for Sensory-Motor Interaction, Aalborg University, Aalborg, Denmark
2
Institute of Medical Psychology and Behavioral Neurobiology, Eberhard-Karls-University, Tübingen, Germany
3
Department of Psychology, Universitat of the Baleares, Palma, Spain
4
Istituto di Ricovero e Cura a Carattere Scientifico, Ospedale San Camillo, Italy
The study investigated the possibility of identifying the speed of an imagined movement from EEG recordings in amyotrophic lateral sclerosis (ALS) patients. EEG signals were acquired from four ALS patients during imagination of wrist extensions at two speeds (fast and slow), each repeated up to 100 times in random order. The movement-related cortical potentials (MRCPs) and averaged sensorimotor rhythm associated with the two tasks were obtained from the EEG recordings. Moreover, offline single-trial EEG classification was performed with discrete wavelet transform for feature extraction and support vector machine for classification. The speed of the task was encoded in the time delay of peak negativity in the MRCPs, which was shorter for faster than for slower movements. The average single-trial misclassification rate between speeds was 30.4 ± 3.5% when the best scalp location and time interval were selected for each individual. The scalp location and time interval leading to the lowest misclassification rate varied among patients. The results indicate that the imagination of movements at different speeds is a viable strategy for controlling a brain-computer interface system by ALS patients.
Many individuals suffer from motor impairment caused by traumatic brain injury, hypoxia, stroke, tumor, or neurodegenerative diseases, such as amyotrophic lateral sclerosis (ALS). In these patients, sensory, emotional, and cognitive processing often remains largely intact despite extensive degeneration of the motor system. Specifically in ALS, the disease progresses from the first symptoms of muscular or respiratory weakness to the locked-in state, where initially a minimal residual voluntary muscle activity is present and at a later stage the condition becomes a complete locked-in state with no muscular control (Kübler et al., 2007
). Paralysis greatly limits independence and communication. This situation has a significant effect on the quality of life of the patients and their families (Moss et al., 1996
).
The ultimate goal of brain-computer interface (BCI) technology for these disorders is to provide a non-muscular communication and control channel to convey messages and control the external environment for severely disabled individuals (Wolpaw et al., 2002
). The scalp-recorded EEG could be the basis for BCIs that restore and replace lost communication and control (Birbaumer et al., 1999
; Donoghue, 2002
; Kübler et al., 2001
; Wolpaw et al., 2002
). It has been shown that patients with severe motor disabilities are able to control a BCI system by means of regulation of slow cortical potential or sensorimotor rhythms (SMR) and detection of P300 (Birbaumer et al., 1999
; Kübler and Birbaumer, 2008
; Kübler et al., 2005
; Nijboer et al., 2008
; Pfurtscheller et al., 2000
; Piccione et al., 2006
; Vaughan et al., 2004
). With slow cortical potential BCI and SMR-BCI, the patients learn the regulation of the amplitude or power of the EEG in specific subbands through online feedback whereas a P300-BCI is based on external stimuli. Although the control of slow cortical potentials requires long training time, these systems have allowed severely disabled and locked-in state patients to communicate messages of considerable length (Kübler et al., 2007
; Neumann et al., 2003
). With the use of SMR-BCI, it has been shown that ALS patients achieve 75% of accuracy with less than 20 training sessions (Kübler et al., 2005
). Moreover, ALS patients can use P300-BCI systems with online accuracies of up to 79%, with stable performance over several months (Nijboer et al., 2008
). However, there is still a relatively small number of studies in which BCI systems are applied in ALS patients. In this study, we propose an alternative paradigm for BCI control and we investigate the feasibility of this control strategy.
Studies on healthy subjects have identified the movement-related parameters that can be distinguished from MRCPs on a single-trial basis (do Nascimento and Farina, 2008
; Farina et al., 2007
). Moreover, it has been reported that the speed of an imagined movement can be better classified than two different movements at the wrist joint in healthy subjects, with average offline accuracy of 79% (Gu et al., 2009
). These results are promising for the application in ALS patients. There have not been attempts in using the decoding of movement-related parameters, such as speed, in ALS patients. Therefore, in this study we explored the possibility of distinguishing a movement imagined at two speeds in ALS patients. Averaged MRCPs, event related desynchronization (ERD)/event related synchronization (ERS), and single-trial EEG traces were analyzed for imagination of wrist extensions. The aim was to assess with offline analysis if imagination of a movement at different speeds can be effectively used to control a BCI system by ALS patients.
Subjects
Four patients diagnosed with ALS, aged 40–70 years (one man and three women) participated in the study. The experimental protocol was approved by the ethics committee of the University of Tübingen, Medical Faculty. The degree of physical impairment of each patient is described in Table 1
.
Experimental Procedure
The subject seated in a wheel chair with the arms resting on arm supports. The subjects were asked to imagine one side wrist extension at two speeds (Fast and Slow). The choice of the investigated side depended on the degree of impairment of the hand/wrist. The more severely impaired side was examined and when the two sides were equally impaired the right wrist was investigated. Patient 3 was examined for both left and right wrist imagination. The fast speed corresponded to a movement executed as fast as possible whereas slow speed was associated with a movement performed in approximately 3 s.
To instruct patients on the task, the experimenter described the movements while performing them in front of the patient. In addition, the experimenter passively executed the tasks with the patient’s hand. The subjects were then asked to feel themselves performing the movements, instead of visualising movements. The two imaginary tasks were performed randomly, controlled by a computer program. At time 0 of each trial period, a recorded voice indicated “ready for fast” or “ready for slow”, which corresponded to the task to be imagined subsequently. After a random time interval in the range 2–3 s after task indication, a beep sounded (250 Hz tone presented for 10 ms), which indicated the time to start imagination. After finishing the imagination task, the patient rested for a random interval in the range 5–6 s and waited for the next task. Each patient performed up to 200 trials, with 5–10 min of break after each block of 20 trials. Each trial took an interval of time of 10–13 s. The instruction on imaginary movements and experimental procedure took approximately 20 min. Patient 3 participated in two sessions on 2 days, with imagination of the tasks with the right wrist in the first session and left wrist in the second session (Table 1
).
Signal Recordings
The EEG recordings were performed with a 40-channel digital DC EEG amplifier (Neuro Scan Labs, NuAmps). Ag/AgCl ring electrodes were mounted in a standard EEG cap (Easy Cap EC20, EASYCAP GmbH) according to the 10–20 system (Klem et al., 1999
). The EEG recordings (F3, Fz, F4, C3, Cz, C4, P3, Pz and P4) were referenced to linked Ag/AgCl electrodes at the earlobes (A1, A2) and grounded at the forehead. Three Ag/AgCl ring electrodes were used for EOG recordings, one positioned above the left eye and two on the outer canthi of the eyes. Electrode impedances were kept below 5 kΩ. The EEG/EOG signals were amplified (Neuro Scan Labs, NuAmps), low-pass filtered with cut-off frequency 200 Hz and sampled at 500 Hz using a 22-bit A/D converter. EEG/EOG signals were notch filtered at 50 Hz.
Pre-Processing
Epochs starting 3 s before the imagination onset and 4 s after were extracted by the software EEGlab (Delorme and Makeig, 2004
). Trials in which the EOG signal exceeded 75 μV were discarded visually from further analysis. Further trials contaminated by facial muscles’ movement which appeared in the EEG recording channels were rejected, with visual assessment. The baseline was corrected on each EEG channel by subtracting the mean amplitude value in the interval −2 s to −1.8 s with reference to the imagination onset (time 0). The EEG signals were low-pass filtered at 45 Hz.
Statistical Analysis on MRCPs
The peak negativity, time delay of peak negativity referenced to the imagination onset (time 0) and the rebound rate after imagination onset were identified from single trials. First, the EEG signals were smoothed by moving average over 400 time samples. Then, the peak negativity was calculated as the lowest value between −1 and 2.5 s. The time delay was computed as the time of occurrence of the peak negativity with reference to the imagination onset time. The rebound rate of the potential was calculated as the difference between the amplitude of the potential at 0.2 s after the peak negativity and the peak negativity, divided by 0.2 s. Different time intervals have been tested for calculating the rebound rate. The resultant choice of 0.2 s was empirical. Peak negativity, time delay, and rebound rate were analyzed with a two-way ANOVA with factors the channel (F3, Fz, F4, C3, Cz, C4, P3, Pz, P4) and the speed (fast and slow) for each patient. Post-hoc multiple pair-wise comparisons were performed with Tukey’s least significant difference test. Outcomes were considered significant at P < 0.05. Results are presented as mean and SD.
Offline Single-Trial Classification
The applied pattern recognition method (Farina et al., 2007
) extracted features from the discrete wavelet transform of the recordings and used a support vector machine (SVM) as classifier. Selection of the mother wavelet, optimisation of the SVM parameters, and estimation of the probability of error was performed by a 3-fold cross-validation procedure, as described previously (Farina et al., 2007
). Figure 1
shows the block diagram of the method. The following paragraphs will give a brief introduction on this pattern recognition method.
Figure 1. Block diagram of the algorithm used for single-trial classification. θ is the parameter for tuning the mother wavelet. σ and C are the parameters of the SVM classifier. Details can be found in (Farina et al., 2007
).
Feature extraction
The discrete wavelet transform (DWT) projects the signal into translated and scaled versions of a prototype function (mother wavelet). The selection of the mother wavelet provides the way for obtaining a feature space adapted to a specific classification problem. The mother wavelet can be parameterized by the Multi Resolution Analysis (MRA) framework (Farina et al., 2007
; Maitrot et al., 2005
; Mallat, 1989
). The group of mother wavelets can be generated by tuning a parameter vector θ. The DWT provides a set of detail coefficients di(j, k), where 2j is the scaling factor, k the translation parameter, and i the index identifying the mother wavelet. The marginals of the detail coefficients were the features used as inputs to the classifier:
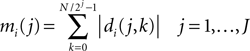

where J is the deepest level of the decomposition and N the length of the signal. The feature vector Mi contains information on the distribution of the wavelet coefficients over J bands. The analyzing wavelet was chosen on the basis of a learning phase (supervised classification), as described below. Changes of MRCPs and SMR have been reliably and consistently observed during voluntary movement and imagination of voluntary movement from the same cortical areas (Shibasaki and Hallett, 2006
). The MRCP energy is mainly concentrated at the low frequency bandwidth (up to 1 Hz), while SMR rhythms are located at higher frequency bands. Since the active frequency bands are subject dependant, the full frequency bandwidth of the signal was used in this study for classification.
Classification
A SVM classifier with Gaussian kernel of parameterized width σ was used in this study. The data were classified by building a hyperplane from a training set of signals. Given a training set (xi, yi), i = 1,…,N, where xi ∈ Rn and yi = {±1}, the standard SVM requires the solution of the following optimization problem (Cortes and Vapnik, 1995
):
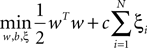
subject to 

where the function ϕ maps xi into a higher dimensional space. w is the weight vector and b is the bias of the hyperplane. A slack variable (ξi) and a penalty parameter (c) are introduced if the training data cannot be separated without error. As a consequence, training samples can be at a small distance ξi on the wrong side of the hyperplane. In practice, there is a trade-off between a low training error and a large margin. This trade-off is controlled by the penalty parameter c.
Cross-validation
A double 3-fold cross-validation was applied to test the results. The signal data set was randomly divided into three subsets of equal size. One subset was used as testing set and the remaining two subsets as training set (first cross-validation). The signals in the training set were further divided into three subsets of equal size (second cross-validation), two used for optimizing the parameters (designing wavelet parameter θ, penalty parameter c and Gaussian kernel parameter σ) and the last for estimating the probability of error of the optimized parameters (this cross-validation was performed for generalization purposes). The set of parameters (c, σ, θ) were searched by the inner cross-validation in the following ranges, chosen empirically:


The set of parameters (c, σ, θ) corresponding to the lowest probability of error estimated from the inner cross-validation was applied to the test set from which the accuracy was computed.
The single-trial classification was performed on the data epochs [0, 1]s, [0, 2]s, [0, 4]s, [1, 2]s, [2, 3]s, [−1, 0]s, and [−1, 3]s (0 corresponds to imagination onset).
MRCPs
Figure 2
shows the averaged MRCPs from all patients. In patient 1, the surface negativity started increasing around −2 s for both tasks. The rate of increase of negativity during the fast movement was faster than that during the slow movement before imagination onset. At approximately 0.6 s, the potential started to rebound for the fast movement, whereas the potential rebounded from approximately 1.3 s for the slow movement. Patients showed different characteristics of the MRCP components. However, the time delay to peak negativity was shorter for fast than that for slow speed in all patients (Figure 2
).
Figure 2. Averaged MRCPs from all patients at the channel Cz during the fast and slow speed tasks. The time samples were averaged over trials and over time. The imaginary movement onset is represented by time 0. N: number of averaged trials. TD: time delay.
Figure 3
shows the topography of averaged peak negativity, time delay, rebound rate and Mu band power from subject 3 during left wrist imagination. The Mu band power was calculated as following. The wavelet coefficients covering the frequency band 8–12 Hz during the time period [−1, 0] s were squared, averaged over time samples, and averaged over trials. Finally, the mean value was taken from the above calculation. Peak negativity distribution did not show obvious differences between fast and slow tasks (visual inspection). Visually, time delays were shorter for fast than that for slow tasks. The shortest time delay occurred at the right hemisphere for both speeds. The rebound rate of fast was stronger than that of slow tasks in the frontal and central areas. The Mu band power of fast tasks was greater than that of slow tasks before the imagination onset.
Figure 3. Topography of peak negativity, time delay, rebound rate and Mu band power from the patient 3 during left wrist imagination.
Table 2
shows the average values for peak negativity, time delay, and rebound rate associated to the two speeds for all subjects at Cz and Table 3
reports the corresponding statistical analysis. Speed had a significant effect on time delay (highlighted in Table 3
) in all patients. This analysis shows that fast speed was associated to shorter time delay than slow speed (Tables 2 and 3
).
ERD/ERS
Figure 4
shows the time-frequency maps from all patients. ERD/ERS activities could be identified from each map. Each patient displayed individual characteristics of ERD/ERS. In patient 1, ERD during the fast task had earlier onset than during the slow task in the pre-imagination phase whereas ERS during the fast task had earlier onset than during the slow task in the after-imagination phase. ERD/ERS occurred in different time windows for the two speeds in patient 1. In patient 2, ERD could be observed during the fast task in the interval [0.5, 1]s whereas during the slow task it was observed in the interval [1.2, 1.8]s. In patient 4, ERD/ERS were similar after imagination for both speeds whereas a slight difference could be observed in the pre-imagination phase.
Figure 4. Time-frequency maps from all subjects at Cz. The absolute wavelet coefficients covering the frequency band 8–12 Hz during the time period [−2, 4]s were averaged over time samples, transformed in logarithmic scale, and averaged over trials.
Single-Trial Classification
Table 4
shows the lowest classification error on single trials with selected channel and epoch. The average classification error across patients was 30.4 ± 3.5%. Patients were different in terms of best scalp location and best time interval for the classification but the best classification accuracy was similar across patients. Binomial tests were performed for each patient to verify if the classification accuracy was above chance level. For all patients, the classification of single-trials EEG traces had accuracy above chance level (P < 0.001 for all patients except patient 3, left wrist, in which case P = 0.003) for the channel and time interval reported in Table 4
.
The study has shown that the speed of an imagined task could be discriminated on a single-trial offline basis in patients with ALS. Thus, this mental task may be an alternative strategy for controlling BCI systems by these patients. Although the objective proof of BCI performance is based on feedback sessions with online classification, offline analysis is a necessary preliminary step when testing strategies for BCI control (Dornhege et al., 2007
). The single-trial classification used in this study could be easily extended to online implementation with tuned parameters of the pattern recognition algorithm performed during a calibration session. The proposed approach is based on DWT and SVM which can be efficiently implemented online.
The peak negativity, time delay of peak negativity, and rebound rate were computed from the single-trial MRCPs for statistically analyzing the effect of speed of the task imagined. The statistical analysis has shown great variability for these features among patients as well as a large trial-to-trial variability within the same patient (Tables 2 and 3
). Despite this variability, speed had a significant effect on time delay for all patients, with fast speed associated to shorter time delay than slow speed.
The average ERD/ERS showed that the time interval of ERD/ERS shifted to the right with slow speed compared with fast speed for patients 1 and 2. However, the characteristics of ERD/ERS were very different among patients. The variability may be due to the different degree of physical impairment and psychological status of each individual (Kübler and Birbaumer, 2008
; Shibasaki and Hallett, 2006
).
Despite the variability in the characteristics of MRCPs and ERD/ERS, the single-trial classification accuracy was similar among patients (range 66–75%). This may be due to the fact that the applied pattern recognition method (Farina et al., 2007
) overcomes the inter-subject variability by tuning the mother wavelet and the parameters of the classifier based on training on each individual. Furthermore, different time intervals were used for classification and the best time interval was chosen for each patient. As can be noted from Table 4
, there was a large variability in the optimal time intervals among patients. For example, in patient 1 the best classification could be obtained by analyzing the signal portion preceding the imagination onset and characterizing the preparation phase whereas in the other patients the optimal time interval included a portion of signal after the imagination onset.
The classification accuracies obtained in each patient were above chance level. The classification accuracy between tasks imagined at different speeds in this study for ALS patients (69.6 ± 3.5%) is lower than that obtained with a similar experimental paradigm in healthy subjects (79 ± 2.4%; Gu et al., 2009
). A possible explanation for lower accuracy in patients is that healthy subjects have probably a better feeling of imagined movements due to the intact motor function pathway and the continuous performance of movements. In addition, the measures on patients were in a clinical environment rather than in a research laboratory, as for healthy subjects, and thus the signal quality was in general lower for the patient recordings.
Successful feedback studies with other mental strategies in ALS patients (Kübler and Birbaumer, 2008
) were based on using slow cortical potentials, sensorimotor rhythms and P300. In such systems, learning regulation of slow cortical potentials and sensorimotor rhythms requires extensive training over weeks or months (Kübler et al., 2005
; Kübler and Birbaumer, 2008
; Neuper et al., 2003
). Moreover, although it does not require training, a P300-BCI requires the patient to control the gaze, which limits its applicability in severely disabled patients, who may not be able to fixate an object on a screen (Kübler et al., 2007
). The proposed mental strategy is easy to understand since the difference between fast and slow speed is intuitive. However, the present study is limited to offline classification and constitutes the basis for the future development of an online feedback system based on the proposed mental strategy. The classification results are indeed promising considering that the patients were investigated in only one experimental session and did not have training periods over several sessions, contrary to previous studies (Kübler et al., 2005
; Neuper et al., 2003
). Moreover, the patients did not receive any feedback on the classification of their mental commands since the classification was performed offline. It is expected that with training over several sessions and feedback, the single-trial classification accuracy will further improve since previous studies have reported improved accuracy of EEG control after several training sessions and with continuous feedback during training (Curran and Stokes, 2003
; Kübler and Birbaumer, 2008
; Kübler et al., 2005
; Piccione et al., 2006
). However, it is not possible to directly extend the current results to online classification since the effect of the feedback may also be negative for low classification accuracies.
Imagination of movements from the same joint in the proposed mental strategy may make the control more natural than associating commands to movements of different limbs (McFarland and Wolpaw, 2005
; Pfurtscheller et al., 2006
). Moreover, the identification of movement parameters is an intuitive way to expand the degrees of freedom of BCIs. It may be interesting in future studies to combine two speeds, as in this study, with different limbs’ movement to double the number of control inputs.
In conclusion, the results of this study present the evidence that single-trial EEG traces associated to a movement imagined at two speeds by patients with ALS can be differentiated with accuracy above chance level. This provides a novel mental strategy to build a communication channel for patients with ALS.
The authors declare that the research was conducted in the absence of any commercial or financial relationships that could be construed as a potential conflict of interest.
The Danish Research Agency (Project “New generation of brain-computer interface (BCI) for reestablishment of complex motor tasks”, Contract nr. 2117-05-0083); the Obel Family Foundation; Deutsche Forschungsgemeinschaft (DFG), BMBF, Bundesministerium für Bildung und Forschung, Bernstein Center, Neurocomputation (Nr 01GQ0831); Spanish Ministry of Science and European Funds (FEDER) (Nr SEJ2007-62312).
Nijboer, F., Sellers, E. W., Mellinger, J., Jordan, M. A., Matuz, T., Furdea, A., Halder, S., Mochty, U., Krusienski, D. J., Vaughan, T. M., Wolpaw, J. R., Birbaumer, N., and Kübler, A. (2008). A P300-based brain–computer interface for people with amyotrophic lateral sclerosis (ALS). Clin. Neurophysiol. 119, 1909–1916.