- 1Faculty of Medicine, Department of Clinical Physiology, King Abdulaziz University, Jeddah, Saudi Arabia
- 2Public Health Sector, General Directorate of Health Affairs in Najran, Ministry of Health, Najran, Saudi Arabia
- 3Faculty of Medicine, Department of Pediatrics, King Abdulaziz University, Jeddah, Saudi Arabia
Attention deficit hyperactivity disorder (ADHD) is one of the most common neurodevelopmental disorders among children. Working memory deficits underlie many of the behavioural symptoms of ADHD. Alongside psychostimulant medications, strategies to improve working memory may play an important adjuvant role in the management of ADHD. In this study, we review the role of working memory deficits in ADHD, the evidence surrounding working memory training strategies in the management of the condition, and the factors affecting the success of these strategies in alleviating ADHD symptoms. More specifically, we review several non-pharmacological interventions that target working memory deficits in ADHD, with special emphasis on cognitive working memory training. We conclude that the development of evidence-based interventions such as computerised cognitive training (CCT) could provide an alternative or adjunct to the use of psychostimulants, especially in cases where side effects are a major issue.
Introduction
Attention deficit hyperactivity disorder (ADHD) is one of the most common neurodevelopmental disorders among children (Benyakorn et al., 2016). It is a chronic disorder with a complex and heterogeneous clinical presentation (Kofler et al., 2019), including age-inappropriate and impairing levels of inattention, hyperactivity, and impulsivity (Franke et al., 2018).
The worldwide prevalence of ADHD among children and adolescents is 7.2% (Wolraich et al., 2019) and among adults is between 2% and 5% (Polyzoi et al., 2018). Different etiological factors and numerous prenatal risk factors are associated with ADHD, including maternal substance use, stress during pregnancy, prematurity, low birth weight, and several complications of pregnancy, labour, delivery, and infancy (Sciberras et al., 2017).
ADHD affects children of both sexes, but males are diagnosed with ADHD more frequently than females, with a male to female ratio of 2–4:1 (Wolraich et al., 2019). These differences may be attributable to hyperactive behaviour being more apparent in males (Wolraich et al., 2019). Conversely, females are more prone to receive a diagnosis of the inattentive subtype of ADHD (Magnus et al., 2021). Although the disorder is classically thought of as a developmental disorder, most children diagnosed with ADHD will continue to experience symptoms during adolescence and into adulthood (Gallo and Posner, 2016). Persistent ADHD is associated with multiple negative consequences including academic underachievement, substance abuse, risky sexual behaviour, car accidents and injuries, unstable peer relationships (Bélanger et al., 2018), decreased community functioning, unemployment, and reduced income (Holbrook et al., 2016; Danielson et al., 2018).
Additionally, ADHD may present with other comorbid disorders including anxiety (Lopez et al., 2018), depression (Lopez et al., 2018), autism spectrum disorders (ASD), oppositional defiant disorder (ODD), and conduct disorder (Franke et al., 2018). Mortality is also high in people with ADHD (Bélanger et al., 2018).
The diagnosis of ADHD remains challenging due to a lack of symptom specificity, a broad list of differential diagnoses, and the presence of comorbidities (Bélanger et al., 2018). For these reasons, a comprehensive and detailed clinical assessment is particularly important (Bélanger et al., 2018).
According to the Diagnostic and Statistical Manual of Mental Disorders (DSM-5), there are three types of ADHD: predominantly inattentive, predominantly hyperactive/impulsive, and the combined type (Lopez et al., 2018). A similar definition was recommended by the International Classification of Diseases (ICD-10). There are differences in the age of onset and the necessary number of symptoms for diagnosis (Lopez et al., 2018). To diagnose a child with ADHD, the child must present with six out of the nine symptoms on the two sets of core domains (inattention and hyperactivity/impulsivity) as described in DSM-5. In youths and adults, only five of these symptoms are necessary for diagnosis. The symptoms must be observed for at least 6 months and cause substantial impairment in social, academic, and occupational performance in two or more different settings (e.g., home and school).
The age of onset of ADHD symptoms was raised from 7 years (DSM-4) to 12 years (DSM-5) to allow further flexibility in diagnosing older adolescents and adults (Wolraich et al., 2019). The symptoms of inattention include an inability to maintain focus on details, making imprudent mistakes, an inability to stay focused on duties, appearing to not listen when being spoken to, an inability to adhere to directions or arrange tasks, keeping away from duties that need mental exertion, losing important things, getting preoccupied with external stimuli, and being careless in everyday activities. The symptoms of hyperactivity/impulsivity include: squirming, feeling as if being continuously driven by an “inward engine,” an inability to stay seated when required, jumping on things, being noisy, exclaiming answers, blabbering, an inability to wait their turn, and a tendency to hinder or interrupt others. Because each of these symptoms has a different underlying neurological substrate, it is possible that different neurobiological mechanisms contribute to the clinical features of ADHD (Table 1).
Medications are essential for the management of patients with ADHD (Sonuga-Barke et al., 2014). Psychostimulants are considered the first-line pharmacological treatment option for ADHD (Lambez et al., 2020), with methylphenidate being the most prescribed psychostimulant. Methylphenidate can enhance cognitive function in patients with ADHD and improve cerebral cortex activity by increasing the availability of catecholamines, which play a critical role in cognitive functioning (Farr et al., 2014). However, the use of stimulants increases the risk of anorexia, weight loss, and insomnia (Briars and Todd, 2016).
Non-pharmacological interventions have also been investigated for improving cognitive function in ADHD (Sharma et al., 2015). Dietary supplementation with minerals and Omega-3 resulted in modest improvements in ADHD behavioural symptoms and emotional lability (Sharma et al., 2015). Meditation-based practices such as yoga and mindfulness are commonly practised in patients with ADHD (Sharma et al., 2015). Yoga has been found to modulate the activity of the autonomic nervous system and induce parasympathetic activity and thus improve anxiety and mood. It also reduces impulsive behaviour (Sharma et al., 2015). Mindfulness increases the density of grey matter in areas associated with memory, emotion control, and learning (Sharma et al., 2015). However, there are substantial differences between studies in the methods and the targeted ADHD subtypes and deficits, and hence these conclusions remain to be corroborated (Sharma et al., 2015).
Another promising non-pharmacological approach is neurofeedback (NFB; Ros et al., 2014). It improves self-control by using a brain-computer interface and improves behavioural symptoms of ADHD for up to 1 year after treatment (Van Doren et al., 2019). NFB training also improves visual and auditory short-term memory and auditory working memory (Nesayan et al., 2019).
Cognitive-behavioural therapy (CBT) is another non-pharmacological approach that can be implemented on an individual or group basis, or for parental education (Shabanpour et al., 2017). Previous studies have shown that CBT reduces the behavioural symptoms of ADHD (Shabanpour et al., 2017). In addition, there is evidence for other non-invasive, non-pharmacological interventions that can be offered to ADHD patients such as physical exercise, transcranial direct current stimulation (tDCS), and transcranial magnetic stimulation (TMS) to improve the behavioural and cognitive domains of ADHD (Lambez et al., 2020). However, there are relatively few studies in this field (Lambez et al., 2020).
The combination of non-pharmacological and pharmacological interventions seems promising for achieving improved cognitive function with lower medication doses, thus potentially reducing side effects. These non-pharmacological interventions target cognitive domains considered central to the cognitive deficits in ADHD, most notably working memory. Moreover, these interventions provide benefits beyond what is achievable through medication alone (Holmes et al., 2010; Catalá-López et al., 2017). Thus, training exercises targeting working memory are a promising adjunct treatment option for people with ADHD.
In this review, we provide a review of working memory and working memory training and their role in the management of ADHD. We provide an overview of the mechanisms underlying the therapeutic benefit of working memory training and identify novel directions for research to improve ADHD treatment protocols.
Working Memory
Working memory refers to the active mental workspace that can briefly hold and manipulate information (Fang et al., 2016). Working memory capacity determines the rate and level of learning and predicts performance on mental tasks such as reading comprehension, language acquisition, reasoning, and problem-solving (Fang et al., 2016; Emch et al., 2019).
Working memory is a hierarchical process that connects detailed sensory representations to specific behavioural responses. These are linked via intermediate task-relevant representations and action plans in a network of different brain areas (Christophel et al., 2017).
Working memory plays a fundamental role in cognition, allowing one to hold information “in mind.” Working memory is defined by its flexibility: people are capable of storing, at least temporarily, any information. According to Baddeley’s multi-element model of working memory, considered the predominant hypothetical model (Baddeley, 2007), the working memory system includes three anatomically and functionally discrete elements:
1. The visuospatial sketchpad, that stores visual and spatial material with limited capacity (Emch et al., 2019).
2. The phonological loop, which has two components. The first component, the phonological store, holds visually presented and auditory-verbal information that can be kept active in the second component, the articulatory loop, through subvocal rehearsal (Emch et al., 2019).
3. The central executive, which allocates the attentional resources for the organisation, deeper processing, and storage of different types of information elements and is considered the master element of working memory (Emch et al., 2019).
The episodic buffer integrates information from the phonological loop, visuospatial sketchpad, and long-term memory (Emch et al., 2019). The buffer is a passive system with limited capacity, believed to be linked to long-term memory and semantic meaning (Baddeley, 2017). Its main function is to link information across different domains to form integrated elements of visual, spatial, and verbal information that are ordered in an episodic and chronological manner.
Working memory includes multiple stages: encoding, maintenance, and retrieval, as well as some process of attention regulation that resists interruption by irrelevant information (Emch et al., 2019).
Encoding is the initial process of perceiving and learning information. Working memory stores information for immediate or long-term use (long-term memory). Encoding can be visual (converting images and visual sensory information to memory), elaborative (relating new information to previously stored knowledge), semantic (processing and encoding sensory input that has a particular meaning), acoustic (encoding auditory inputs), or other (tactile, odours, tastes; Baddeley, 2017).
Maintenance (or storage) of information is the process of placing the acquired information into memory. Memory can be stored in short-term or long-term memory, with the former being a component of working memory. Short-term memory is only used to refer to the storage of information for a short while and working memory refers to the components of memory that uses the information to manipulate this information (Baddeley, 2017).
Retrieval is the mental process of recalling information that was previously stored. There are three main types of recall: free, cued, and serial. Free recall occurs when individuals are asked to recall items previously presented on a list. Cued recall is when a person receives a list of items to remember and is then offered cues to help them recall those items during testing. Serial recall refers to recalling events or items in the order in which they occurred (Baddeley, 2017).
Neural Correlates of Working Memory
Early studies that used resting-state functional magnetic imaging (fMRI) have shown that large-scale brain regions exhibit high-amplitude fluctuations, which are enhanced during rest and reduced during cognitive tasks (Konrad and Eickhoff, 2010; Castellanos and Proal, 2012). This intrinsic functional inter-neuronal connection represents the brain’s physiological reference and the so-called default mode network (DMN; Konrad and Eickhoff, 2010; Castellanos and Proal, 2012). The DMN involves the anterior medial prefrontal cortex (amPFC), the posterior cingulate cortex (PCC), the medial temporal lobe (MTL) subsystem, and the dorsomedial PFC (dmPFC) subsystem (Castellanos and Proal, 2012).
The executive control network (ECN), also known as the frontoparietal network, includes the dorsolateral PFC (dlPFC), anterior PFC (aPFC), anterior cingulate cortex (ACC), lateral frontal pole, lateral cerebellum, anterior insula, caudate, and inferior parietal lobe (Konrad and Eickhoff, 2010; Castellanos and Proal, 2012). This circuit has been defined as the task-positive circuit as it shows more activity during tasks than during rest (Konrad and Eickhoff, 2010). It also directs decision making by incorporating exterior stimuli with the corresponding interior representations (Castellanos and Proal, 2012). The DMN and ECN are inversely correlated as the activation of the ECN is associated with lower activity of the DMN and vice versa (Konrad and Eickhoff, 2010).
The dorsal attentional network includes the intraparietal sulcus and frontal eye fields, which are essential in attention shifting and control (Castellanos and Proal, 2012). The ventral attentional network, also known as the salience network (SN), involves the fronto-insular cortex (FIC), temporoparietal junction and supramarginal gyrus (Castellanos and Proal, 2012). The visual network includes the visual cortex and lateral temporal region MT+, which is linked to DAN via the superior parietal lobule and intraparietal cortex (IPC). MT+ also is connected with frontal areas (Castellanos and Proal, 2012). The occipital cortex, which contains most of the visual cortex regions, interacts with DAN to hold attention and suppress attention to the distractor (Castellanos and Proal, 2012). The motor network involves simultaneous spontaneous low-amplitude fluctuations between the supplementary motor cortex, primary motor cortex, primary and secondary sensory cortex, putamen, cerebellum, thalamus, and ventral premotor cortex (Castellanos and Proal, 2012). These fundamental networks can be investigated in ADHD and other neurocognitive disorders (Castellanos and Proal, 2012).
Functional neuroimaging studies have demonstrated that working memory is related to the prefrontal cortex (PFC), inferior and middle temporal lobes, and zones close to the IPC (Fang et al., 2016). Similarly, these regions are linked to cognitive function, PLL, declarative memory, and episodic processing (Fang et al., 2016). Moreover, working memory task-based fMRI studies have shown that information encoding and manipulation is related to dorsolateral PFC (dlPFC). Error recognition and execution adjustment, on the other hand, are related to activity in the dorsal ACC (dACC), which is considered to be the attention organiser (Chai et al., 2018). Information selection, retrieval, and inhibition regulation are linked to neurons extending from the ventrolateral PFC (vlPFC) to the anterior insula (Fang et al., 2016). The left PFC and the right PFC might be primarily associated with verbal working memory and spatial working memory, respectively, as indicated in previous meta-analyses (Emch et al., 2019). However, there is no common agreement on the functional organisation of this brain region (Emch et al., 2019).
fMRI studies have also proposed that the articulatory loop is linked to Broca’s area, premotor cortex (BA6), supplementary motor area, and insula on the left hemisphere. The phonological store is linked to BA 40, relating to the inferior parietal lobule in the left hemisphere (Emch et al., 2019). Hence these areas are critical for all kinds of visual working memory tasks (Emch et al., 2019). The cerebellum has been suggested to be involved in subvocal rehearsal (Emch et al., 2019). Similarly, the basal ganglia (BG) are essential brain structures involved in motor control, facilitating appropriate motor behaviour and inhibiting inappropriate motor behaviour (Emch et al., 2019). The BG is also involved in working memory and language production (Emch et al., 2019). Furthermore, visual working memory is related to parts of the limbic system such as the cingulate (Emch et al., 2019). However, the contribution of the cerebellum, BG, and limbic system to working memory has long been undervalued (Emch et al., 2019).
In addition, studies that used resting-state fMRI have revealed that working memory functioning is related to resting-state neuronal activity (Fang et al., 2016). For example, Hampson et al. (2010), reported that working memory accuracy was related to the coherent neuronal interconnection between the dlPFC and medial PFC (Hampson et al., 2010; Fang et al., 2016). However, such complex cognitive functions are characterised by cooperation between multiple brain areas rather than being driven by one or two regions (Fang et al., 2016). Furthermore, the individual disparities in working memory are related to the efficient functional connection from dlPFC to Dacc and from the right dlPFC to the left FIC. The high sensitivity of left FIC to inputs from dlPFC assists in efficient manipulation of information and hence improved working memory functioning during working memory tasks (Fang et al., 2016).
Moreover, diffusion MRI in healthy individuals has also demonstrated that working memory capacity is linked to a corticocortical pathway between the frontal and parietal regions. In addition, the updating of working memory information included a subcortical neural pathway linking between frontal and parietal regions through the thalamus and BG (Ekman et al., 2016). Moreover, working memory capacity is directly correlated to the integrity of white matter in frontal and parietal regions on diffusion MRI (Ekman et al., 2016).
Cognitive Deficits in ADHD
Although many cognitive targets have been investigated in ADHD (see Table 2), this review focuses on working memory. Working memory deficits are an important potential endophenotype of ADHD (Kasper et al., 2012; Chacko et al., 2013). The earliest model of the neuropsychological correlate of ADHD was the prefrontal-striatal-cerebellar model (Castellanos and Proal, 2012).
Animal Studies
Animal models of ADHD show good predictive validity that allows the assessment and development of new therapeutic interventions. For example, they have shown polymorphism in several genes related to catecholaminergic neurotransmission. These include the dopamine transporter (DAT), dopamine D4 receptor (DRD4), and dopamine beta-hydroxylase genes. A lack of DAT results in decreased release of dopamine from the nerve terminal, which is accompanied by a fivefold increase in the concentration of extracellular dopamine. On the presynaptic side, mRNA and D1 and D2 receptor protein levels in the BG decrease. Compounds such as amphetamine, methylphenidate, and cocaine have a direct action on DATs and inhibit hyperactivity (Rahi and Kumar, 2021). While studies with transgenic mice have provided valuable information on the neurobiological factors underlying ADHD, no single gene or transgenic animal model represents the entire ADHD spectrum. Therefore, complex gene-gene as well gene-environment interactions must also be taken into account.
Human Studies
Recent studies propose that working memory deficits occur in approximately 80–85% of children with ADHD when evaluated with cognitive tasks (Coghill et al., 2014; Karalunas et al., 2017; Kofler et al., 2018a, 2020). Studies have also shown that children with ADHD have more working memory deficits than typically developing children (Kasper et al., 2012). Several studies have shown that children diagnosed with ADHD are impaired in all working memory elements, with the most significant impairment found in the central executive, resulting in an inability to focus on a task (Kofler et al., 2010).
Working memory deficits have been linked with inattention (Kofler et al., 2010), hyperactivity (Hudec et al., 2015), and impulsivity (Raiker et al., 2012). They have been associated with ADHD-related impairment in academics (Friedman et al., 2018), organisational (Kofler et al., 2018b), social (Bunford et al., 2015), and family life (Kofler et al., 2017). Longitudinal studies show that less severe working memory deficits are associated with lower symptom severity (Halperin et al., 2008; van Lieshout et al., 2016; Salari et al., 2017), and reductions in ADHD symptoms with age seem to be limited to a subset of children who show improvements in working memory over time (Karalunas et al., 2017). These studies highlight the significant influence that working memory has on the symptomatology of many children with ADHD and have motivated a recent surge in studies aiming to enhance working memory in children with ADHD (Kofler et al., 2018a).
Neural Correlates of ADHD
Functional neuroimaging studies using specific or multiple cognitive tasks in ADHD patients have found widespread multiregional dysfunctions. These include the lateral PFC and its connection to the BG, medio- and orbitofrontal regions, and the cingulate cortex. In addition, dissociation in neural connectivity in the frontoparietal, fronto-limbic and fronto-cerebellar networks have also been observed.
A meta-analysis of fMRI studies that were conducted on ADHD patients (n = 111) and controls (n = 113), revealed a reduction in neural activity in superior and middle PFC in both hemispheres, as well as the medial frontal cortex and ACC in the left hemisphere. A recent functional MRI study investigated more than 100 children and adults with ADHD using a visual-spatial working memory task. It showed two separate effects according to working memory demand: enhancement of neural activity in the inferior prefrontal cortex (IFC) under high working memory demand and a reduction in neural activity in IFC under low working memory demand (Rubia, 2018).
Working Memory Training
Working memory training aims to improve working memory through a series of tasks that help the trainee engage and practice this cognitive function. Prevalent are computerised cognitive training (CCT) interventions, which can provide training in a wide range of cognitive tasks (e.g., working memory, attention, inhibitory control) in multiple training sessions over several weeks, often in a game format design (Table 3). The duration and number of sessions differ according to each specific implemented program (Veloso et al., 2019). CCT is described as adaptive when the level of task difficulty is automatically adapted to the user’s performance, and the training sessions can be accomplished in any preferred setting (e.g., a clinic, home, or school; Sonuga-Barke et al., 2014).
Available CCT products that include working memory training are listed in Table 4 and include the Cogmed Working Memory Training (Klingberg et al., 2005; Beck et al., 2010; Green et al., 2012; Chacko et al., 2013; Egeland et al., 2013; Hovik et al., 2013; van Dongen-Boomsma et al., 2014; van der Donk et al., 2015; Bigorra et al., 2016), BrainTrain (Steiner et al., 2014), Braingame Brian (van der Oord et al., 2014), CogniPlus (Minder et al., 2018), Activate (Sinnari et al., 2019), Project: Evo (Davis et al., 2018), Attention Pay (van der Donk et al., 2015), Persian software (Azami et al., 2016), Lumosity (Azami et al., 2016), and Captain’s log (Rabiner et al., 2010). These products have similar goals but differ in the extent to which they include elements like acquiring points, providing feedback, representing skill progression, accumulating rewards, and framing the training within a narrative context (Oldrati et al., 2020).
The Cogmed Working Memory Training program (CWMT, Pearson, UK) has become the most popular and widely studied CCT program, and it has both supporters and detractors (Shinaver et al., 2014; Sonuga-Barke et al., 2014). The program consists of sessions of working memory tasks in the form of simple games on a computer or tablet. The training sessions take around 45–50 min to complete, 5 days per week, over 5 weeks, with weekly rewards. The complexity of the tasks is automatically adjusted based on the person’s performance.
Effects of Working Memory Training
In this section, we discuss the evidence for the efficacy of working memory training in the general population, which helps us understand its potential role in ADHD management. Figure 1 provides an overview of the potential mechanisms to improve working memory.
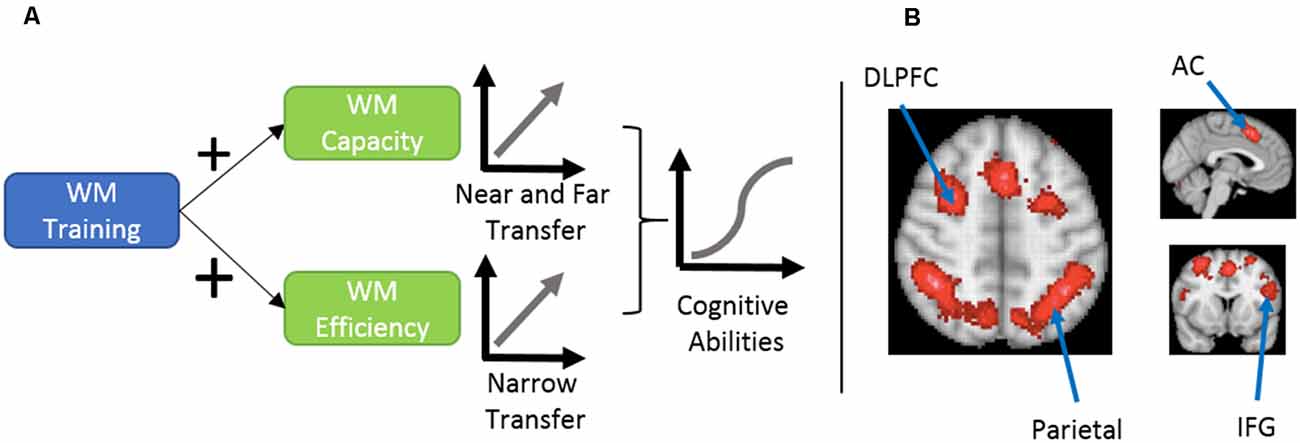
Figure 1. (A) Proposed mechanism of cognitive ability enhancement using working memory training, as explained by von Bastian et al. (2013). (B) An association-test map displaying brain regions that were consistently reported in 1091 studies investigating working memory. Working memory training engages executive and dorsal attentional networks. This involves brain regions like dorsolateral prefrontal cortex (DLPFC), anterior cingulate (AC), inferior frontal gyrus (IFG), and parietal regions. Map prepared using Neurosynth meta-analysis of the term working memory (Yarkoni et al., 2011). Similar neural networks show decreased signals in attention deficit hyperactivity disorder (ADHD) patients performing working memory tasks.
Two general mechanisms explain the effects of working memory training (von Bastian and Oberauer, 2014). The first is increased working memory capacity, which enables people to hold more items in their working memory, and the second is increased efficiency in using available working memory capacity.
At the behavioural level, an increase in working memory capacity results in performance improvements in tasks on which the person was not trained, but that share some variance with the training tasks (Klingberg, 2010). Given that working memory capacity significantly correlates with several cognitive abilities, these improvements should manifest in several measures which are independent of the precise materials and structure of the tasks (Schmiedek et al., 2010). In general, two terms are used to define the transfer of training: (1) near-transfer which refers to gains in abilities directly related to the training task; and (2) far-transfer, which are gains in different but related abilities.
Meiran, Dreisbach, and von Bastian noted in 2019 that while meta-analyses assessing the existence of far-transfer benefits of working memory training have found negative (Melby-Lervåg et al., 2016), limited or short-lived positive results (Au et al., 2015), performance gains on similar tasks are substantial, and trainees typically performing above average after training. For example, in one study, young adults could recall twice as many items from a list as the average healthy adult after 20 sessions of working memory training (von Bastian and Oberauer, 2014).
Understanding the neural correlates of training-induced improvements would help guide and monitor training strategies. However, this is challenging given the existence of many parallel behavioural changes that occur during working memory training (Klingberg, 2010). There are, however, studies that show a positive correlation between working memory capacity and brain activity in different task-relevant areas. For example, inter-individual differences in working memory capacity positively correlate with activity in the IPC (Gray et al., 2003; Todd and Marois, 2004; Vogel and Machizawa, 2004; Lee et al., 2006; McNab and Klingberg, 2008) and increases in working memory capacity during childhood are positively correlated with brain activity in the prefrontal cortex and intraparietal sulcus (Klingberg et al., 2002; Kwon et al., 2002; Ciesielski et al., 2006; Crone et al., 2006; Scherf et al., 2006; Olesen et al., 2007). On the other hand, the decline in working memory during ageing is associated with decreased activity in certain prefrontal areas (Rajah and D’Esposito, 2005; Persson and Nyberg, 2006).
To assess the effects of working memory training on the brain, most neuroimaging studies have relied on recording changes in the activity of brain regions during working memory task performance before and after training (Constantinidis and Klingberg, 2016). Working memory training is associated with changes in the neuronal activity of brain regions that are activated during working memory tasks before training (Constantinidis and Klingberg, 2016). This finding suggests that improvements in task performance during working memory training reflect improved working memory capacity (Constantinidis and Klingberg, 2016).
Earlier research with healthy adults using fMRI suggests that working memory training has a direct effect on the prefrontal cortex, posterior parietal cortex, and dopamine receptor binding. Studies have shown that working memory training results in enhanced neuronal activity in the prefrontal cortex and the posterior parietal cortex, which are areas linked to working memory processing (Green et al., 2012). Working memory training also increases the density of D1 receptors in these brain regions (Green et al., 2012) and increases activity in the striatum (Constantinidis and Klingberg, 2016). Although this change in striatal activity is not consistent, it is predictive of working memory capacity changes during development (Constantinidis and Klingberg, 2016).
Several studies of the brain’s functional connectivity have shown that stronger functional connectivity between frontal and parietal cortices plays a significant role in working memory improvement with training (Constantinidis and Klingberg, 2016). A study using TMS of the parietal cortex during training on higher-demand working memory tasks revealed stronger functional connectivity between the frontal cortex and the parietal cortex, and between the parietal cortex and the occipital cortex (Constantinidis and Klingberg, 2016). Furthermore, a magnetoencephalography study of functional connectivity changes during, before, and after working memory training found increased connectivity between frontal, parietal and lateral occipital cortex, which was associated with working memory improvements (Constantinidis and Klingberg, 2016).
An increase in synaptic connection and myelin density in axons connecting the involved regions might be the mechanism underlying training-related functional connectivity changes (Constantinidis and Klingberg, 2016). As previously mentioned, dopamine plays an important role during working memory training (Constantinidis and Klingberg, 2016). Positron emission tomography studies in humans and other animals have shown that working memory training results in changes in the release of dopamine as well as the density of dopamine receptors (Constantinidis and Klingberg, 2016). Changes in striatal dopamine release might also enable cortical plasticity (Constantinidis and Klingberg, 2016).
Working Memory Training in ADHD
Several studies have provided evidence for the efficacy of working memory training in ADHD. A meta-analysis of 54 studies investigating a range of CCT programs (including most of the ones mentioned above) showed that they were associated with improvements in working memory, as well as other elements of executive functioning, in people with ADHD (Veloso et al., 2019). Importantly, the majority of studies involving longitudinal follow-up showed that these improvements were maintained over time.
The reason CWMT attracts more attention compared to most other CCT programs likely stems from the fact that its effectiveness has been established by several studies (Simons et al., 2016). Furthermore, studies investigating the effects of CWMT tend to have a better experimental design, often including active controls. These randomised controlled trials of CWMT provide strong evidence that it enhances performance on other working memory tasks that have similar processing demands. While other CCT programs have been shown to improve working memory, evidence for this from randomised controlled trials is often lacking. Furthermore, when randomised controlled trials of these interventions are conducted, they are often compared with passive control groups (Simons et al., 2016). Due to its widespread use, popularity, and strong evidence for its efficacy, the following section will focus on studies utilising CWMT.
Several studies have found significant clinical effects on executive functions in ADHD individuals following CWMT intervention (Table 5). Klingberg et al. (2005) conducted the first randomised controlled trial of CWMT in 53 children with ADHD who were randomised to either adaptive (adjusted to the user’s performance) or non-adaptive CWMT (not adjusted to the user’s performance) This research showed that adaptive CWMT resulted in significant improvement of working memory, inhibition control, complex reasoning, and a reduction in ADHD symptoms on the parent-rating scale; these effects were maintained at a post-training 3 months follow-up. Gray et al. (2012) evaluated the impact of CWMT on working memory in a sample of 60 youth who were diagnosed with ADHD and learning disability. The main finding was that CWMT improved visuospatial working memory and visual working memory, and also led to gains in attention, math, and reading.
Consistent with the findings of previous studies, Holmes et al. (2010) found that CWMT produced significant clinical effects in a sample of 25 children with ADHD, who were assessed before and after training as well as on and off medication. The researchers concluded that, although medication significantly improved visuospatial working memory, CWMT led to significant gains on multiple memory tasks such as visuospatial working memory, visual working memory, visuospatial short term memory, and verbal short term memory. The improvements lasted for at least 6 months after training and were larger than the effect of the medication alone.
In a different study, Beck et al. (2010) found that children with ADHD who underwent CWMT showed an improvement in working memory and a reduction in inattention along with an increase in positive behaviours compared to age-typical children in the wait-list group. These improvements were still noticed by their parents 4 months later. However, the study reported no positive effects on hyperactivity/impulsivity symptoms rated by their patients and teachers (Beck et al., 2010). Similarly, Green et al. (2012) reported that adaptive-CWMT in ADHD children led to significant gains in working memory tasks, but there was no effect on ADHD symptoms rated by the parents (Gray et al., 2012).
There is some evidence regarding the beneficial effects of CWMT on fluid intelligence, which may play a significant role in educational achievement (Kaufman et al., 2009). Bergman Nutley et al. (2011) found significant improvements on measures of fluid intelligence in children who trained on a non-verbal reasoning task. This finding was also replicated on working memory training tasks other than CogMed (i.e., N-Back), and lasted for at least 3 months post-training (Jaeggi et al., 2011). Söderqvist and Bergman Nutley (2015) reported that CWMT in typical learners led to higher academic achievement in math and reading 2 years after training.
Other studies have found less promising results with regards to CWMT outcomes. These studies concluded that, although CWMT led to improvements in certain aspects of working memory and executive function, these improvements failed to generalise to academic achievement (Chacko et al., 2013; Bigorra et al., 2016). Similarly, a previous meta-analysis that examined the effectiveness of cognitive training in children with ADHD concluded that there is no significant effect of training on the cognitive, behavioural, and/or academic abilities of these children (Rapport et al., 2013). However, the researchers stated that their findings might be due to methodological limitations across the reviewed studies. In line with the previous meta-analysis, Melby-Lervåg and Hulme (2013) reported that working memory training resulted in short-term gains in working memory skills, but these gains do not generalise to other skills or persist for long periods.
With regards to the transfer effects of cognitive training, it has been theorised that training-based improvements in working memory capacity and attention generalise to other functioning domains. However, the results across the literature are inconsistent (Sala et al., 2019). CWMT has shown near-transfer effects in children diagnosed with ADHD, poor working memory, and/or attention deficits (Rossignoli-Palomeque et al., 2018). Far-transfer effects were reported on measures of reasoning and inhibition (Klingberg et al., 2005) and executive function (Holmes et al., 2010; Bigorra et al., 2016). Far-transfer effects were also reported on ADHD symptoms (Beck et al., 2010; Bigorra et al., 2016) as well as on academic abilities such as math (Holmes et al., 2010; Dahlin, 2013; Egeland et al., 2013) and reading (Egeland et al., 2013). The effects of training on near-transfer were short-lived, and on far-transfer ranged from 4 to 8 months (Rossignoli-Palomeque et al., 2018).
The inconsistent results regarding the effectiveness of cognitive training on improving ADHD cognition and behaviour may reflect differences in training strategy (exact training protocols, trained populations, concomitant treatment, etc). The heterogeneity of training strategies used in previous studies may itself reflect a lack of understanding of the mechanisms that underlie the response (or lack thereof) to cognitive interventions.
Factors Potentially Influencing The Effect of Working Memory Training
Several factors are known to influence the outcomes of CCT interventions, including characteristics of the training plan (such as the intensity, duration, adaptivity of the training task), as well as individual differences in age, cognitive abilities, biological factors, personality factors, motivational factors, and emotional factors (von Bastian and Oberauer, 2014; Barkus, 2020; Dentz et al., 2020). Awareness of the role of these factors may help optimise CCT strategies in the future.
Characteristics of the Training Plan
Most commercially available CCT programs target a mix of different cognitive skills. While this was thought to lead to more transfer effects than targeting single skills, empirical evidence for this – in the form of comprehensive direct comparisons between these strategies – is lacking. The little evidence that is available in this regard suggests that programs that provide intensive practise of one aspect of a cognitive function like working memory are probably more effective at achieving transfer effects than those that involve multiple cognitive skills (von Bastian et al., 2013).
There is considerable heterogeneity in the CCT literature regarding the number and duration of training sessions (Luis-Ruiz et al., 2020; Wiest et al., 2020; Grinberg et al., 2021). Importantly, few studies have attempted to directly determine the optimal length and intensity of CCT. Nonetheless, several studies have shown that the effect of such interventions is dose-dependent, meaning the length and intensity of these programs influence outcomes (Jaeggi et al., 2008; Alloway et al., 2013). Although many commercially available CCT products implement this, the influence of adapting to the individual trainee’s performance to maintain a level of difficulty that is challenging on performance gains is also controversial (von Bastian and Oberauer, 2014).
Characteristics of the Trainee
Several factors potentially contribute to the relatively high between-person variability in performance gains and transfer effects following working memory training. Working memory training programs tend to generally be more effective in younger than older individuals, with evidence suggesting that the relationship between age and training is linear throughout the lifespan (Wass et al., 2012; Melby-Lervåg and Hulme, 2013). Characteristics such as intrinsic motivation (finding enjoyment or satisfaction in engaging in a particular behaviour) are known to correlate with working memory performance (Brose et al., 2010; Duckworth et al., 2011). Whether or not such characteristics directly influence the performance gains following CCT interventions has not yet been established. On the other hand, personality traits like neuroticism (related to higher excitability and emotional responsiveness) are associated with lower performance gains after working memory training (Studer-Luethi et al., 2012).
Twin studies show an estimated heritability of working memory capacity of around 50% (Ando et al., 2001). As previously mentioned, the importance of dopamine in this context is evidenced by the finding that working memory training increases dopaminergic receptor density and dopaminergic pathway activity (Green et al., 2012; Constantinidis and Klingberg, 2016). In addition, working memory performance appears to be significantly influenced by dopamine-relevant genes (Bäckman and Nyberg, 2013). Carriers of the DAT1 9/10-repeat allele benefit more from working memory training compared to carriers of the DAT1 10-repeat allele (Brehmer et al., 2012). This difference may be explained by the fact that 10-repeat carriers have increased gene expression, which leads to a higher level of dopamine reuptake, and, consequently, fewer active dopaminergic pathways available (Swanson et al., 2000).
Another genetic factor that contributes to the availability of dopamine is the allelic variations in the LIM homeobox transcription factor 1 alpha (LMX1A; Nakatani et al., 2010). A study conducted by Colzato et al. (2011) showed that two single nucleotide polymorphisms (SNP) that influence the number of dopamine neurons in the midbrain have a significant relationship with verbal working memory training.
Finally, brain-derived neurotrophic factor (BDNF), which is involved in hippocampal plasticity, may also have a role in working memory training (Loprinzi and Frith, 2019). One example of this is the Val66 Met SNP in the BDNF gene. In comparison to Val homozygotes, carriers with the Met allele perform poorer in certain memory tasks (Hariri et al., 2003) and have reduced hippocampal volume (Bueller et al., 2006). A comparison of Val/Val homozygotes with carriers of the Met allele (Colzato et al., 2011) showed that, while both groups improved during CCT, only the Val/Val homozygous individuals showed far-transfer to a divided attention task.
Discussion
ADHD has a complex and heterogeneous disorder and a one-size-fits-it-all treatment approach is likely to provide limited results in many cases. The present study provides a review of the neurocognitive mechanisms that underlie changes in working memory and how these mechanisms may influence the response to working memory training.
Cognitive training programs that target working memory are a potentially useful therapeutic option in ADHD. Response to working memory training may reflect changes in the neuronal activity of brain regions that are activated during working memory tasks before training (Constantinidis and Klingberg, 2016) and multiple factors may explain individual differences in response (Ando et al., 2001), such as the settings of the training regime and several individual factors.
Given that working memory capacity significantly correlates with several cognitive abilities, improvements in working memory capacity should manifest in several measures which are independent of the materials and structure of the tasks (Schmiedek et al., 2010; Rosenberg et al., 2020). The possibility of achieving near-transfer and far-transfer following working memory training arguably adds additional support in favour of researching and improving working memory training programs for ADHD.
Although pharmacological interventions such as stimulants provide a substantial improvement in ADHD cognitive symptoms, this comes at the cost of a higher risk of side effects. Cognitive training provides additional benefits above and beyond those of pharmacological interventions in ADHD, though additional studies of higher methodological quality comparing these two treatment strategies are necessary.
Author Contributions
MA-S, BA-J and AA: literature search, writing, editing, reviewing. All authors contributed to the article and approved the submitted version.
Conflict of Interest
The authors declare that the research was conducted in the absence of any commercial or financial relationships that could be construed as a potential conflict of interest.
References
Alloway, T. P., Bibile, V., and Lau, G. (2013). Computerized working memory training: can it lead to gains in cognitive skills in students? Comput. Hum. Behav. 29, 632–638. doi: 10.1016/j.chb.2012.10.023
Ando, J., Ono, Y., and Wright, M. J. (2001). Genetic structure of spatial and verbal working memory. Behav. Genet. 31, 615–624. doi: 10.1023/a:1013353613591
Au, J., Sheehan, E., Tsai, N., Duncan, G. J., Buschkuehl, M., and Jaeggi, S. M. (2015). Improving fluid intelligence with training on working memory: a meta-analysis. Psychon. Bull. Rev. 22, 366–377. doi: 10.3758/s13423-014-0699-x
Azami, S., Moghadas, A., Sohrabi-Esmrood, F., Nazifi, M., Mirmohamad, M., Hemmati, F., et al. (2016). A pilot randomized controlled trial comparing computer-assisted cognitive rehabilitation, stimulant medication and an active control in the treatment of ADHD. Child Adolesc. Ment. Health 21, 217–224. doi: 10.1111/camh.12157
Bäckman, L., and Nyberg, L. (2013). Dopamine and training-related working-memory improvement. Neurosci. Biobehav. Rev. 37, 2209–2219. doi: 10.1016/j.neubiorev.2013.01.014
Baddeley, A. D. (2017). Modularity, working memory and language acquisition. Second Lang. Res. 33, 299–311. doi: 10.1177/0267658317709852
Barkus, E. (2020). Effects of working memory training on emotion regulation: transdiagnostic review. PsyCh J. 9, 258–279. doi: 10.1002/pchj.353
Beck, S. J., Hanson, C. A., Puffenberger, S. S., Benninger, K. L., and Benninger, W. B. (2010). A controlled trial of working memory training for children and adolescents with ADHD. J. Clin. Child Adolesc. Psychol. 39, 825–836. doi: 10.1080/15374416.2010.517162
Bélanger, S. A., Andrews, D., Gray, C., and Korczak, D. (2018). ADHD in children and youth: part 1-Etiology, diagnosis, and comorbidity. Paediatr. Child Health 23, 447–453. doi: 10.1093/pch/pxy109
Benyakorn, S., Riley, S. J., Calub, C. A., and Schweitzer, J. B. (2016). Current state and model for development of technology-based care for attention deficit hyperactivity disorder. Telemed. J. E Health 22, 761–768. doi: 10.1089/tmj.2015.0169
Bergman Nutley, S., Söderqvist, S., Bryde, S., Thorell, L. B., Humphreys, K., and Klingberg, T. (2011). Gains in fluid intelligence after training non-verbal reasoning in 4-year-old children: a controlled, randomized study: fluid intelligence gains after training non-verbal reasoning. Dev. Sci. 14, 591–601. doi: 10.1111/j.1467-7687.2010.01022.x
Bigorra, A., Garolera, M., Guijarro, S., and Hervás, A. (2016). Long-term far-transfer effects of working memory training in children with ADHD: a randomized controlled trial. Eur. Child Adolesc. Psychiatry 25, 853–867. doi: 10.1007/s00787-015-0804-3
Brehmer, Y., Westerberg, H., and Bäckman, L. (2012). Working-memory training in younger and older adults: training gains, transfer, and maintenance. Front. Hum. Neurosci. 6:63. doi: 10.3389/fnhum.2012.00063
Briars, L., and Todd, T. (2016). A review of pharmacological management of attention-deficit/hyperactivity disorder. J. Pediatr. Pharmacol. Ther. 21, 192–206. doi: 10.5863/1551-6776-21.3.192
Brose, A., Schmiedek, F., Lövdén, M., Molenaar, P. C. M., and Lindenberger, U. (2010). Adult age differences in covariation of motivation and working memory performance: contrasting between-person and within-person findings. Res. Hum. Dev. 7, 61–78. doi: 10.1080/15427600903578177
Bueller, J. A., Aftab, M., Sen, S., Gomez-Hassan, D., Burmeister, M., and Zubieta, J.-K. (2006). BDNF Val66Met allele is associated with reduced hippocampal volume in healthy subjects. Biol. Psychiatry 59, 812–815. doi: 10.1016/j.biopsych.2005.09.022
Bunford, N., Brandt, N. E., Golden, C., Dykstra, J. B., Suhr, J. A., and Owens, J. S. (2015). Attention-deficit/hyperactivity disorder symptoms mediate the association between deficits in executive functioning and social impairment in children. J. Abnorm. Child Psychol. 43, 133–147. doi: 10.1007/s10802-014-9902-9
Castellanos, F. X., and Proal, E. (2012). Large-scale brain systems in ADHD: beyond the prefrontal-striatal model. Trends Cogn. Sci. 16, 17–26. doi: 10.1016/j.tics.2011.11.007
Catalá-López, F., Hutton, B., Núñez-Beltrán, A., Page, M. J., Ridao, M., Macías Saint-Gerons, D., et al. (2017). The pharmacological and non-pharmacological treatment of attention deficit hyperactivity disorder in children and adolescents: a systematic review with network meta-analyses of randomised trials. PLoS One 12:e0180355. doi: 10.1371/journal.pone.0180355
Chacko, A., Feirsen, N., Bedard, A.-C., Marks, D., Uderman, J. Z., and Chimiklis, A. (2013). Cogmed working memory training for youth with ADHD: a closer examination of efficacy utilizing evidence-based criteria. J. Clin. Child Adolesc. Psychol. 42, 769–783. doi: 10.1080/15374416.2013.787622
Chai, W. J., Abd Hamid, A. I., and Abdullah, J. M. (2018). Working memory from the psychological and neurosciences perspectives: a review. Front. Psychol. 9:401. doi: 10.3389/fpsyg.2018.00401
Christophel, T. B., Klink, P. C., Spitzer, B., Roelfsema, P. R., and Haynes, J.-D. (2017). The distributed nature of working memory. Trends Cogn. Sci. 21, 111–124. doi: 10.1016/j.tics.2016.12.007
Ciesielski, K. T., Lesnik, P. G., Savoy, R. L., Grant, E. P., and Ahlfors, S. P. (2006). Developmental neural networks in children performing a categorical N-back task. NeuroImage 33, 980–990. doi: 10.1016/j.neuroimage.2006.07.028
Coghill, D. R., Seth, S., and Matthews, K. (2014). A comprehensive assessment of memory, delay aversion, timing, inhibition, decision making and variability in attention deficit hyperactivity disorder: advancing beyond the three-pathway models. Psychol. Med. 44, 1989–2001. doi: 10.1017/S0033291713002547
Colzato, L. S., van Muijden, J., Band, G. P. H., and Hommel, B. (2011). Genetic modulation of training and transfer in older adults: BDNF ValMet polymorphism is associated with wider useful field of view. Front. Psychol. 2:199. doi: 10.3389/fpsyg.2011.00199
Constantinidis, C., and Klingberg, T. (2016). The neuroscience of working memory capacity and training. Nat. Rev. Neurosci. 17, 438–449. doi: 10.1038/nrn.2016.43
Crone, E. A., Wendelken, C., Donohue, S., van Leijenhorst, L., and Bunge, S. A. (2006). Neurocognitive development of the ability to manipulate information in working memory. Proc. Natl. Acad. Sci. U S A 103, 9315–9320. doi: 10.1073/pnas.0510088103
Dahlin, K. I. E. (2013). Working memory training and the effect on mathematical achievement in children with attention deficits and special needs. J. Stat. Educ. 2, 118–133. doi: 10.5539/jel.v2n1p118
Danielson, M. L., Bitsko, R. H., Ghandour, R. M., Holbrook, J. R., Kogan, M. D., and Blumberg, S. J. (2018). Prevalence of parent-reported ADHD diagnosis and associated treatment among U.S. children and adolescents, 2016. J. Clin. Child Adolesc. Psychol. 47, 199–212. doi: 10.1080/15374416.2017.1417860
Davis, N. O., Bower, J., and Kollins, S. H. (2018). Proof-of-concept study of an at-home, engaging, digital intervention for pediatric ADHD. PLoS One 13:e0189749. doi: 10.1371/journal.pone.0189749
Dentz, A., Guay, M.-C., Parent, V., and Romo, L. (2020). Working memory training for adults with ADHD. J. Atten. Disord. 24, 918–927. doi: 10.1177/1087054717723987
Duckworth, A. L., Quinn, P. D., Lynam, D. R., Loeber, R., and Stouthamer-Loeber, M. (2011). Role of test motivation in intelligence testing. Proc. Natl. Acad. Sci. U S A 108, 7716–7720. doi: 10.1073/pnas.1018601108
Egeland, J., Aarlien, A. K., and Saunes, B.-K. (2013). Few effects of far transfer of working memory training in ADHD: a randomized controlled trial. PLoS One 8:e75660. doi: 10.1371/journal.pone.0075660
Ekman, M., Fiebach, C. J., Melzer, C., Tittgemeyer, M., and Derrfuss, J. (2016). Different roles of direct and indirect frontoparietal pathways for individual working memory capacity. J. Neurosci. 36, 2894–2903. doi: 10.1523/JNEUROSCI.1376-14.2016
Emch, M., von Bastian, C. C., and Koch, K. (2019). Neural correlates of verbal working memory: an fMRI meta-analysis. Front. Hum. Neurosci. 13:180. doi: 10.3389/fnhum.2019.00180
Fang, X., Zhang, Y., Zhou, Y., Cheng, L., Li, J., Wang, Y., et al. (2016). Resting-state coupling between core regions within the central-executive and salience networks contributes to working memory performance. Front. Behav. Neurosci. 10:27. doi: 10.3389/fnbeh.2016.00027
Farr, O. M., Zhang, S., Hu, S., Matuskey, D., Abdelghany, O., Malison, R. T., et al. (2014). The effects of methylphenidate on resting-state striatal, thalamic and global functional connectivity in healthy adults. Int. J. Neuropsychopharmacol. 17, 1177–1191. doi: 10.1017/S1461145714000674
Franke, B., Michelini, G., Asherson, P., Banaschewski, T., Bilbow, A., Buitelaar, J. K., et al. (2018). Live fast, die young? A review on the developmental trajectories of ADHD across the lifespan. Eur. Neuropsychopharmacol. 28, 1059–1088. doi: 10.1016/j.euroneuro.2018.08.001
Friedman, L. M., Rapport, M. D., Orban, S. A., Eckrich, S. J., and Calub, C. A. (2018). Applied problem solving in children with ADHD: the mediating roles of working memory and mathematical calculation. J. Abnorm. Child Psychol. 46, 491–504. doi: 10.1007/s10802-017-0312-7
Gallo, E. F., and Posner, J. (2016). Moving towards causality in attention-deficit hyperactivity disorder: overview of neural and genetic mechanisms. Lancet Psychiatry 3, 555–567. doi: 10.1016/S2215-0366(16)00096-1
Gray, J. R., Chabris, C. F., and Braver, T. S. (2003). Neural mechanisms of general fluid intelligence. Nat. Neurosci. 6, 316–322. doi: 10.1038/nn1014
Gray, S. A., Chaban, P., Martinussen, R., Goldberg, R., Gotlieb, H., Kronitz, R., et al. (2012). Effects of a computerized working memory training program on working memory, attention, and academics in adolescents with severe LD and comorbid ADHD: a randomized controlled trial. J. Child Psychol. Psychiatry 53, 1277–1284. doi: 10.1111/j.1469-7610.2012.02592.x
Green, C. T., Long, D. L., Green, D., Iosif, A.-M., Dixon, J. F., Miller, M. R., et al. (2012). Will working memory training generalize to improve off-task behavior in children with attention-deficit/hyperactivity disorder? Neurotherapeutics 9, 639–648. doi: 10.1007/s13311-012-0124-y
Grinberg, A., Egglefield, D. A., Schiff, S., Motter, J. N., and Sneed, J. R. (2021). Computerized cognitive training: a review of mechanisms, methodological considerations, and application to research in depression. J. Cogn. Enhanc. [Epub ahead of print].doi: 10.1007/s41465-021-00209-4
Halperin, J. M., Trampush, J. W., Miller, C. J., Marks, D. J., and Newcorn, J. H. (2008). Neuropsychological outcome in adolescents/young adults with childhood ADHD: profiles of persisters, remitters and controls. J. Child Psychol. Psychiatry 49, 958–966. doi: 10.1111/j.1469-7610.2008.01926.x
Hampson, M., Driesen, N., Roth, J. K., Gore, J. C., and Constable, R. T. (2010). Functional connectivity between task-positive and task-negative brain areas and its relation to working memory performance. Magn. Reson. Imaging 28, 1051–1057. doi: 10.1016/j.mri.2010.03.021
Hariri, A. R., Goldberg, T. E., Mattay, V. S., Kolachana, B. S., Callicott, J. H., Egan, M. F., et al. (2003). Brain-derived neurotrophic factor val66met polymorphism affects human memory-related hippocampal activity and predicts memory performance. J. Neurosci. 23, 6690–6694. doi: 10.1523/JNEUROSCI.23-17-06690.2003
Holbrook, J. R., Cuffe, S. P., Cai, B., Visser, S. N., Forthofer, M. S., Bottai, M., et al. (2016). Persistence of parent-reported ADHD symptoms from childhood through adolescence in a community sample. J. Atten. Disord. 20, 11–20. doi: 10.1177/1087054714539997
Holmes, J., Gathercole, S. E., Place, M., Dunning, D. L., Hilton, K. A., and Elliott, J. G. (2010). Working memory deficits can be overcome: impacts of training and medication on working memory in children with ADHD. Appl. Cogn. Psychol. 24, 827–836. doi: 10.1002/acp.1589
Hovik, K. T., Saunes, B.-K., Aarlien, A. K., and Egeland, J. (2013). RCT of working memory training in ADHD: long-term near-transfer effects. PLoS One 8:e80561. doi: 10.1371/journal.pone.0080561
Hudec, K. L., Alderson, R. M., Patros, C. H. G., Lea, S. E., Tarle, S. J., and Kasper, L. J. (2015). Hyperactivity in boys with attention-deficit/hyperactivity disorder (ADHD): the role of executive and non-executive functions. Res. Dev. Disabil. 45–46, 103–109. doi: 10.1016/j.ridd.2015.07.012
Jaeggi, S. M., Buschkuehl, M., Jonides, J., and Perrig, W. J. (2008). Improving fluid intelligence with training on working memory. Proc. Natl. Acad. Sci. U S A 105, 6829–6833. doi: 10.1073/pnas.0801268105
Jaeggi, S. M., Buschkuehl, M., Jonides, J., and Shah, P. (2011). Short- and long-term benefits of cognitive training. Proc. Natl. Acad. Sci. U S A 108, 10081–10086. doi: 10.1073/pnas.1103228108
Johnstone, S. J., Roodenrys, S., Phillips, E., Watt, A. J., and Mantz, S. (2010). A pilot study of combined working memory and inhibition training for children with AD/HD. Atten. Defic. Hyperact. Disord. 2, 31–42. doi: 10.1007/s12402-009-0017-z
Karalunas, S. L., Gustafsson, H. C., Dieckmann, N. F., Tipsord, J., Mitchell, S. H., and Nigg, J. T. (2017). Heterogeneity in development of aspects of working memory predicts longitudinal attention deficit hyperactivity disorder symptom change. J. Abnorm. Psychol. 126, 774–792. doi: 10.1037/abn0000292
Kasper, L. J., Alderson, R. M., and Hudec, K. L. (2012). Moderators of working memory deficits in children with attention-deficit/hyperactivity disorder (ADHD): a meta-analytic review. Clin. Psychol. Rev. 32, 605–617. doi: 10.1016/j.cpr.2012.07.001
Kaufman, A. S., Kaufman, J. C., Liu, X., and Johnson, C. K. (2009). How do educational attainment and gender relate to fluid intelligence, crystallized intelligence, and academic skills at ages 22–90 years? Arch. Clin. Neuropsychol. 24, 153–163. doi: 10.1093/arclin/acp015
Klingberg, T. (2010). Training and plasticity of working memory. Trends Cogn. Sci. 14, 317–324. doi: 10.1016/j.tics.2010.05.002
Klingberg, T., Fernell, E., Olesen, P. J., Johnson, M., Gustafsson, P., Dahlström, K., et al. (2005). Computerized training of working memory in children with ADHD—a randomized, controlled trial. J. Am. Acad. Child Adolesc. Psychiatry 44, 177–186. doi: 10.1097/00004583-200502000-00010
Klingberg, T., Forssberg, H., and Westerberg, H. (2002). Increased brain activity in frontal and parietal cortex underlies the development of visuospatial working memory capacity during childhood. J. Cogn. Neurosci. 14, 1–10. doi: 10.1162/089892902317205276
Kofler, M. J., Rapport, M. D., Bolden, J., Sarver, D. E., and Raiker, J. S. (2010). ADHD and working memory: the impact of central executive deficits and exceeding storage/rehearsal capacity on observed inattentive behavior. J. Abnorm. Child Psychol. 38, 149–161. doi: 10.1007/s10802-009-9357-6
Kofler, M. J., Sarver, D. E., Austin, K. E., Schaefer, H. S., Holland, E., Aduen, P. A., et al. (2018a). Can working memory training work for ADHD? Development of central executive training and comparison with behavioral parent training. J. Consult. Clin. Psychol. 86, 964–979. doi: 10.1037/ccp0000308
Kofler, M. J., Sarver, D. E., Harmon, S. L., Moltisanti, A., Aduen, P. A., Soto, E. F., et al. (2018b). Working memory and organizational skills problems in ADHD. J. Child Psychol. Psychiatry 59, 57–67. doi: 10.1111/jcpp.12773
Kofler, M. J., Sarver, D. E., Spiegel, J. A., Day, T. N., Harmon, S. L., and Wells, E. L. (2017). Heterogeneity in ADHD: neurocognitive predictors of peer, family, and academic functioning. Child Neuropsychol. 23, 733–759. doi: 10.1080/09297049.2016.1205010
Kofler, M. J., Singh, L. J., Soto, E. F., Chan, E. S. M., Miller, C. E., Harmon, S. L., et al. (2020). Working memory and short-term memory deficits in ADHD: a bifactor modeling approach. Neuropsychology 34, 686–698. doi: 10.1037/neu0000641
Kofler, M. J., Spiegel, J. A., Soto, E. F., Irwin, L. N., Wells, E. L., and Austin, K. E. (2019). Do working memory deficits underlie reading problems in attention-deficit/hyperactivity disorder (ADHD)? J. Abnorm. Child Psychol. 47, 433–446. doi: 10.1007/s10802-018-0447-1
Konrad, K., and Eickhoff, S. B. (2010). Is the ADHD brain wired differently? A review on structural and functional connectivity in attention deficit hyperactivity disorder. Hum. Brain Mapp. 31, 904–916. doi: 10.1002/hbm.21058
Kwon, H., Reiss, A. L., and Menon, V. (2002). Neural basis of protracted developmental changes in visuo-spatial working memory. Proc. Natl. Acad. Sci. U S A 99, 13336–13341. doi: 10.1073/pnas.162486399
Lambez, B., Harwood-Gross, A., Golumbic, E. Z., and Rassovsky, Y. (2020). Non-pharmacological interventions for cognitive difficulties in ADHD: a systematic review and meta-analysis. J. Psychiatr. Res. 120, 40–55. doi: 10.1016/j.jpsychires.2019.10.007
Lee, K. H., Choi, Y. Y., Gray, J. R., Cho, S. H., Chae, J.-H., Lee, S., et al. (2006). Neural correlates of superior intelligence: stronger recruitment of posterior parietal cortex. NeuroImage 29, 578–586. doi: 10.1016/j.neuroimage.2005.07.036
Lopez, P. L., Torrente, F. M., Ciapponi, A., Lischinsky, A. G., Cetkovich-Bakmas, M., Rojas, J. I., et al. (2018). Cognitive-behavioural interventions for attention deficit hyperactivity disorder (ADHD) in adults. Cochrane Database Syst. Rev. 3:CD010840. doi: 10.1002/14651858.CD010840.pub2
Loprinzi, P. D., and Frith, E. (2019). A brief primer on the mediational role of BDNF in the exercise-memory link. Clin. Physiol. Funct. Imaging 39, 9–14. doi: 10.1111/cpf.12522
Luis-Ruiz, S., Caldú, X., Sánchez-Castañeda, C., Pueyo, R., Garolera, M., and Jurado, M. Á. (2020). Is cognitive training an effective tool for improving cognitive function and real-life behaviour in healthy children and adolescents? A systematic review. Neurosci. Biobehav. Rev. 116, 268–282. doi: 10.1016/j.neubiorev.2020.06.019
Magnus, W., Nazir, S., Anilkumar, A. C., and Shaban, K. (2021). “Attention deficit hyperactivity disorder,” in StatPearls [Internet], (Treasure Island, FL: StatPearls Publishing). Available online at: https://www.ncbi.nlm.nih.gov/books/NBK441838/.
McNab, F., and Klingberg, T. (2008). Prefrontal cortex and basal ganglia control access to working memory. Nat. Neurosci. 11, 103–107. doi: 10.1038/nn2024
Melby-Lervåg, M., and Hulme, C. (2013). Is working memory training effective? A meta-analytic review. Dev. Psychol. 49, 270–291. doi: 10.1037/a0028228
Melby-Lervåg, M., Redick, T. S., and Hulme, C. (2016). Working memory training does not improve performance on measures of intelligence or other measures of “far transfer”: evidence from a meta-analytic review: evidence from a meta-analytic review. Perspect. Psychol. Sci. 11, 512–534. doi: 10.1177/1745691616635612
Minder, F., Zuberer, A., Brandeis, D., and Drechsler, R. (2018). Informant-related effects of neurofeedback and cognitive training in children with ADHD including a waiting control phase: a randomized-controlled trial. Eur. Child Adolesc. Psychiatry 27, 1055–1066. doi: 10.1007/s00787-018-1116-1
Nakatani, T., Kumai, M., Mizuhara, E., Minaki, Y., and Ono, Y. (2010). Lmx1a and Lmx1b cooperate with Foxa2 to coordinate the specification of dopaminergic neurons and control of floor plate cell differentiation in the developing mesencephalon. Dev. Biol. 339, 101–113. doi: 10.1016/j.ydbio.2009.12.017
Nesayan, A., Asadi Gandomani, R., and Moin, N. (2019). Effect of neurofeedback on perceptual organization, visual and auditory memory in children with attention deficit/hyperactivity disorder. Iran. J. Child Neurol. 13, 75–82.
Oldrati, V., Corti, C., Poggi, G., Borgatti, R., Urgesi, C., and Bardoni, A. (2020). Effectiveness of computerized cognitive training programs (CCTP) with game-like features in children with or without neuropsychological disorders: a meta-analytic investigation. Neuropsychol. Rev. 30, 126–141. doi: 10.1007/s11065-020-09429-5
Olesen, P. J., Macoveanu, J., Tegnér, J., and Klingberg, T. (2007). Brain activity related to working memory and distraction in children and adults. Cereb. Cortex 17, 1047–1054. doi: 10.1093/cercor/bhl014
Pan, C.-Y., Chu, C.-H., Tsai, C.-L., Lo, S.-Y., Cheng, Y.-W., and Liu, Y.-J. (2016). A racket-sport intervention improves behavioral and cognitive performance in children with attention-deficit/hyperactivity disorder. Res. Dev. Disabil. 57, 1–10. doi: 10.1016/j.ridd.2016.06.009
Persson, J., and Nyberg, L. (2006). Altered brain activity in healthy seniors: what does it mean? Prog. Brain Res. 157, 45–56. doi: 10.1016/s0079-6123(06)57004-9
Polyzoi, M., Ahnemark, E., Medin, E., and Ginsberg, Y. (2018). Estimated prevalence and incidence of diagnosed ADHD and health care utilization in adults in Sweden—a longitudinal population-based register study. Neuropsychiatr. Dis. Treat. 14, 1149–1161. doi: 10.2147/NDT.S155838
Rabiner, D. L., Murray, D. W., Skinner, A. T., and Malone, P. S. (2010). A randomized trial of two promising computer-based interventions for students with attention difficulties. J. Abnorm. Child Psychol. 38, 131–142. doi: 10.1007/s10802-009-9353-x
Rahi, V., and Kumar, P. (2021). Animal models of attention-deficit hyperactivity disorder (ADHD). Int. J. Dev. Neurosci. 81, 107–124. doi: 10.1002/jdn.10089
Raiker, J. S., Rapport, M. D., Kofler, M. J., and Sarver, D. E. (2012). Objectively-measured impulsivity and attention-deficit/hyperactivity disorder (ADHD): testing competing predictions from the working memory and behavioral inhibition models of ADHD. J. Abnorm. Child Psychol. 40, 699–713. doi: 10.1007/s10802-011-9607-2
Rajah, M. N., and D’Esposito, M. (2005). Region-specific changes in prefrontal function with age: a review of PET and fMRI studies on working and episodic memory. Brain 128, 1964–1983. doi: 10.1093/brain/awh608
Rapport, M. D., Orban, S. A., Kofler, M. J., and Friedman, L. M. (2013). Do programs designed to train working memory, other executive functions and attention benefit children with ADHD? A meta-analytic review of cognitive, academic, and behavioral outcomes. Clin. Psychol. Rev. 33, 1237–1252. doi: 10.1016/j.cpr.2013.08.005
Ros, T., Baars, B. J., Lanius, R. A., and Vuilleumier, P. (2014). Tuning pathological brain oscillations with neurofeedback: a systems neuroscience framework. Front. Hum. Neurosci. 8:1008. doi: 10.3389/fnhum.2014.01008
Rosenberg, M. D., Martinez, S. A., Rapuano, K. M., Conley, M. I., Cohen, A. O., Cornejo, M. D., et al. (2020). Behavioral and neural signatures of working memory in childhood. J. Neurosci. 40, 5090–5104. doi: 10.1523/JNEUROSCI.2841-19.2020
Rossignoli-Palomeque, T., Perez-Hernandez, E., and González-Marqués, J. (2018). Brain training in children and adolescents: is it scientifically valid? Front. Psychol. 9:565. doi: 10.3389/fpsyg.2018.00565
Rubia, K. (2018). Cognitive neuroscience of attention deficit hyperactivity disorder (ADHD) and its clinical translation. Front. Hum. Neurosci. 12:100. doi: 10.3389/fnhum.2018.00100
Sala, G., Aksayli, N. D., Tatlidil, K. S., Tatsumi, T., Gondo, Y., and Gobet, F. (2019). Near and far transfer in cognitive training: a second-order meta-analysis. Collabra Psychol. 5:18. doi: 10.1525/collabra.203
Salari, R., Bohlin, G., Rydell, A.-M., and Thorell, L. B. (2017). Neuropsychological functioning and attachment representations in early school age as predictors of ADHD symptoms in late adolescence. Child Psychiatry Hum. Dev. 48, 370–384. doi: 10.1007/s10578-016-0664-1
Scherf, K. S., Sweeney, J. A., and Luna, B. (2006). Brain basis of developmental change in visuospatial working memory. J. Cogn. Neurosci. 18, 1045–1058. doi: 10.1162/jocn.2006.18.7.1045
Schmiedek, F., Lövdén, M., and Lindenberger, U. (2010). Hundred days of cognitive training enhance broad cognitive abilities in adulthood: findings from the COGITO study. Front. Aging Neurosci. 2:27. doi: 10.3389/fnagi.2010.00027
Sciberras, E., Mulraney, M., Silva, D., and Coghill, D. (2017). Prenatal risk factors and the etiology of ADHD—review of existing evidence. Curr. Psychiatry Rep. 19:1. doi: 10.1007/s11920-017-0753-2
Shabanpour, A., Mohammadyfar, M. A., TalePesand, S., and Rezaei, A. M. (2017). Comparison of the effectiveness of three methods of cognitive-behavioral therapy (individual counseling, group counseling and parent education) on reduction of ADHD syndrome. Eur. J. Biosci. 11, 63–71. doi: 10.1016/j.brat.2015.10.008
Sharma, A., Gerbarg, P. L., and Brown, R. P. (2015). Non-pharmacological treatments for ADHD in youth. Adolesc. Psychiatry 5, 84–95. doi: 10.2174/221067660502150430154937
Shinaver, C. S. III., Entwistle, P. C., and Söderqvist, S. (2014). Cogmed working memorytraining: reviewing the reviews. Appl. Neuropsychol. Child 3, 163–172. doi: 10.1080/21622965.2013.875314
Simons, D. J., Boot, W. R., Charness, N., Gathercole, S. E., Chabris, C. F., Hambrick, D. Z., et al. (2016). Do “brain-training” programs work? Psychol. Sci. Public Interest 17, 103–186. doi: 10.1177/1529100616661983
Sinnari, D., Krause, P., and Abulkhair, M. (2019). “Effectiveness and usability of a developed collaborative online tool for children with ADHD,” in Interactivity, Game Creation, Design, Learning, and Innovation. ArtsIT 2018, DLI 2018. Lecture Notes of the Institute for Computer Sciences, Social Informatics and Telecommunications Engineering, Vol. 265, eds A. Brooks, E. Brooks, and C. Sylla, (Cham: Springer). doi: 10.1007/978-3-030-06134-0_52
Söderqvist, S., and Bergman Nutley, S. (2015). Working memory training is associated with long term attainments in math and reading. Front. Psychol. 6:1711. doi: 10.3389/fpsyg.2015.01711
Sonuga-Barke, E., Brandeis, D., Holtmann, M., and Cortese, S. (2014). Computer-based cognitive training for ADHD: a review of current evidence. Child Adolesc. Psychiatr. Clin. N. Am. 23, 807–824. doi: 10.1016/j.chc.2014.05.009
Steiner, N. J., Frenette, E. C., Rene, K. M., Brennan, R. T., and Perrin, E. C. (2014). Neurofeedback and cognitive attention training for children with attention-deficit hyperactivity disorder in schools. J. Dev. Behav. Pediatr. 35, 18–27. doi: 10.1097/DBP.0000000000000009
Studer-Luethi, B., Jaeggi, S. M., Buschkuehl, M., and Perrig, W. J. (2012). Influence of neuroticism and conscientiousness on working memory training outcome. Pers. Individ. Dif. 53, 44–49. doi: 10.1016/j.paid.2012.02.012
Swanson, J. M., Flodman, P., Kennedy, J., Spence, M. A., Moyzis, R., Schuck, S., et al. (2000). Dopamine genes and ADHD. Neurosci. Biobehav. Rev. 24, 21–25. doi: 10.1016/s0149-7634(99)00062-7
Todd, J. J., and Marois, R. (2004). Capacity limit of visual short-term memory in human posterior parietal cortex. Nature 428, 751–754. doi: 10.1038/nature02466
van der Donk, M., Hiemstra-Beernink, A.-C., Tjeenk-Kalff, A., van der Leij, A., and Lindauer, R. (2015). Cognitive training for children with ADHD: a randomized controlled trial of cogmed working memory training and ‘paying attention in class’. Front. Psychol. 6:1081. doi: 10.3389/fpsyg.2015.01081
van der Oord, S., Ponsioen, A. J. G. B., Geurts, H. M., Ten Brink, E. L., and Prins, P. J. M. (2014). A pilot study of the efficacy of a computerized executive functioning remediation training with game elements for children with ADHD in an outpatient setting: outcome on parent- and teacher-rated executive functioning and ADHD behavior. J. Atten. Disord. 18, 699–712. doi: 10.1177/1087054712453167
van Dongen-Boomsma, M., Vollebregt, M. A., Buitelaar, J. K., and Slaats-Willemse, D. (2014). Working memory training in young children with ADHD: a randomized placebo-controlled trial. J. Child Psychol. Psychiatry 55, 886–896. doi: 10.1111/jcpp.12218
Van Doren, J., Arns, M., Heinrich, H., Vollebregt, M. A., Strehl, U., and K Loo, S. (2019). Sustained effects of neurofeedback in ADHD: a systematic review and meta-analysis. Eur. Child Adolesc. Psychiatry 28, 293–305. doi: 10.1007/s00787-018-1121-4
van Lieshout, M., Luman, M., Twisk, J. W. R., van Ewijk, H., Groenman, A. P., Thissen, A. J. A. M., et al. (2016). A 6-year follow-up of a large European cohort of children with attention-deficit/hyperactivity disorder-combined subtype: outcomes in late adolescence and young adulthood. Eur. Child Adolesc. Psychiatry 25, 1007–1017. doi: 10.1007/s00787-016-0820-y
Veloso, A., Vicente, S. G., and Filipe, M. G. (2019). Effectiveness of cognitive training for school-aged children and adolescents with attention deficit/hyperactivity disorder: a systematic review. Front. Psychol. 10:2983. doi: 10.3389/fpsyg.2019.02983
Vogel, E. K., and Machizawa, M. G. (2004). Neural activity predicts individual differences in visual working memory capacity. Nature 428, 748–751. doi: 10.1038/nature02447
von Bastian, C. C., Langer, N., Jäncke, L., and Oberauer, K. (2013). Effects of working memory training in young and old adults. Mem. Cogn. 41, 611–624. doi: 10.3758/s13421-012-0280-7
von Bastian, C. C., and Oberauer, K. (2014). Effects and mechanisms of working memory training: a review. Psychol. Res. 78, 803–820. doi: 10.1007/s00426-013-0524-6
Wass, S. V., Scerif, G., and Johnson, M. H. (2012). Training attentional control and working memory—is younger, better? Dev. Rev. 32, 360–387. doi: 10.1016/j.dr.2012.07.001
Wiest, D. J., Wong, E. H., Bacon, J. M., Rosales, K. P., and Wiest, G. M. (2020). The effectiveness of computerized cognitive training on working memory in a school setting. Appl. Cogn. Psychol. 34, 465–471. doi: 10.1002/acp.3634
Wolraich, M. L., Hagan, J. F. Jr., Allan, C., Chan, E., Davison, D., Earls, M., et al. (2019). Clinical practice guideline for the diagnosis, evaluation, and treatment of attention-deficit/hyperactivity disorder in children and adolescents. Pediatrics 144:e20192528. doi: 10.1542/peds.2019-2528
Keywords: working memory training (WMT), working memory (WM), ADHD (attention deficit hyperactivity disorder), computerised cognitive training (CCT), neurodevelopmental disorders
Citation: Al-Saad MSH, Al-Jabri B and Almarzouki AF (2021) A Review of Working Memory Training in the Management of Attention Deficit Hyperactivity Disorder. Front. Behav. Neurosci. 15:686873. doi: 10.3389/fnbeh.2021.686873
Received: 28 March 2021; Accepted: 28 June 2021;
Published: 21 July 2021.
Edited by:
Chia-Liang Tsai, National Cheng Kung University, TaiwanReviewed by:
Mustapha Muzaimi, Universiti Sains Malaysia Health Campus, MalaysiaTao Tan, Texas A&M University, United States
Copyright © 2021 Al-Saad, Al-Jabri and Almarzouki. This is an open-access article distributed under the terms of the Creative Commons Attribution License (CC BY). The use, distribution or reproduction in other forums is permitted, provided the original author(s) and the copyright owner(s) are credited and that the original publication in this journal is cited, in accordance with accepted academic practice. No use, distribution or reproduction is permitted which does not comply with these terms.
*Correspondence: Abeer F. Almarzouki, YWZtYWxtYXJ6b3VraUBrYXUuZWR1LnNh