- 1Institute of Science and Technology for Brain-Inspired Intelligence, Fudan University, Shanghai, China
- 2Department of Neurology, Shangrao Municipal Hospital, Shangrao, Jiangxi, China
- 3Institute of Longevity and Aging Research, Zhongshan Hospital, Fudan University, Shanghai, China
- 4Shanghai Key Laboratory of Medical Epigenetics, Institutes of Biomedical Sciences, Fudan University, Shanghai, China
- 5Department of Neurosurgery, Renmin Hospital of Wuhan University, Wuhan, Hubei, China
- 6Department of Emergency Medicine, Shanghai Pudong New Area Gongli Hospital, Shanghai, China
Background: Ischemic stroke (IS) represents a significant contributor to morbidity and mortality globally. The relationship between IS and mitochondrial unfolded protein response
Methods: In GSE58294, differentially expressed genes (DEGs) were obtained, which were overlapped with key module genes of
Results: MCEMP1, CACNA1E, and CLEC4D were identified as biomarkers and subsequently validated by RT-qPCR. RT-qPCR revealed that CLEC4D is the most sensitive biomarker. The nomogram analysis revealed that these biomarkers possess strong diagnostic value. Immune infiltration analysis indicated that all three biomarkers are strongly correlated with neutrophils. Additionally, in the single-cell transcriptome data, these biomarkers were predominantly enriched in neutrophils. Compared to the sham group, the middle cerebral artery occlusion (MCAO) group exhibited enhanced immune-inflammatory responses. Virtual knockout experiments provide preliminary evidence that CLEC4D functions as a regulatory molecule in neutrophil-mediated inflammation, rather than serving merely as a passive marker.
Conclusion: CLEC4D was identified as the most sensitive biomarker for IS related to
1 Introduction
Ischemic stroke (IS) poses a significant burden on society and families due to its high incidence, mortality, and disability rates. The economic and disease burden associated with IS remains heavy (Feigin et al., 2014; Tao et al., 2020; Rochmah et al., 2021). Current treatment approaches include intravenous thrombolysis, endovascular therapy, pharmacological treatments, complication management, rehabilitation, and lifestyle modifications (Bathla et al., 2023; Maalouf et al., 2023). Although these interventions can improve outcomes for some patients, clinical treatment efficacy remains poor due to the narrow therapeutic window, potential bleeding risks, and subsequent reperfusion injury (Yang et al., 2021). Effective methods for curing brain dysfunction in IS patients are yet to be developed. Therefore, early exploration of key genes related to ischemia is crucial. Understanding the complex molecular mechanisms underlying these factors may provide new possibilities for identifying novel therapeutic targets for IS, effectively preventing severe complications and mortality associated with the disease.
During the occurrence of ischemic stroke, the interruption of blood supply impairs mitochondrial oxidative phosphorylation, leading to a sharp decline in energy production (An et al., 2021; Zhou et al., 2021; Chavda et al., 2022). Additionally, the hypoxic environment disrupts the mitochondrial electron transport chain (ETC), generating excessive reactive oxygen species (ROS) that further damage mitochondrial structure and function. In this environment, proteins within the mitochondria fail to fold correctly, or previously folded proteins undergo denaturation due to oxidative stress. When the mitochondrial protein-folding mechanisms can no longer cope with this stress, the mitochondrial stress response—known as the mitochondrial unfolded protein response
The neuroprotective role of
In this study, we aimed to identify potential biomarkers for IS by integrating transcriptomic data with single-cell RNA sequencing analysis. Differentially expressed genes (DEGs) from the GSE58294 dataset were cross-referenced with key
2 Materials and methods
2.1 Data source
Ischemic stroke related transcriptomic data was obtained from the Gene Expression Omnibus (GEO) database. Specifically, GSE58294 (GPL570) includes 69 whole blood samples from IS patients (IS group) and 23 whole blood samples from healthy individuals (control group). In addition, GSE16561 (GPL6883) consists of 39 whole blood samples from IS patients (IS group) and 24 whole blood samples from healthy individuals (control group). To further validate the bulk RNA-seq results at the single-cell resolution, we utilized mouse data due to the lack of available human stroke data. Specifically, GSE225948 (GPL19057) includes 4 whole blood samples from middle cerebral artery occlusion (MCAO) mice (IS group) and 4 whole blood samples from sham surgery controls (control group). GSE154396 (GPL21273) includes 4 brain cell samples from MCAO mice (IS group) and 2 brain cell samples from sham surgery controls (control group). The description of the dataset is provided in Supplementary Table 1. In addition, 35
2.2 Identification and analysis of candidate genes
Differentially expressed genes were obtained from the GSE58294 (IS vs. control) using the “limma” with
To further identify key genes associated with
2.3 Functional analysis of candidate genes
To investigate the biological functions and signaling pathways associated with the candidate genes in the pathogenesis of IS, we first performed Gene Ontology (GO) enrichment analysis using the R package “clusterProfiler.” This analysis focused on three key aspects: biological processes (BP), cellular components (CC), and molecular functions (MF), with a significance threshold of
Next, to explore the potential interactions between the candidate genes at the protein level, we constructed a protein-protein interaction (PPI) network using the Search Tool for the Retrieval of Interacting Genes (STRING) database.
2.4 Identification of biomarkers via machine learning
We employed the Least Absolute Shrinkage and Selection Operator (LASSO) regression and Random Forest (RF) models to identify genes that significantly contribute to the accurate prediction of IS group from the identified candidate genes. Specifically, we used the candidate genes as independent variables to predict the IS group, with the weights of the candidate genes serving as training parameters. LASSO regression was applied to identify genes whose weights were not penalized to zero. In the RF model, the top eight genes ranked by importance were selected for further analysis. The intersection of the genes identified by both models was considered as the final set of candidate key genes.
Expression validation of candidate key genes was conducted in the datasets GSE58294 and GSE16561 using the Wilcoxon rank-sum test. Candidate key genes that exhibited significant differential expression
To identify biomarkers, receiver operating characteristic (ROC) curves were generated for all hub genes. The area under the curve (AUC) values were calculated to assess the diagnostic accuracy for IS. Hub genes with an AUC
2.5 Construction of nomogram
To facilitate patient assessment in medical research and clinical practice, we constructed a regression model using the expression levels of biomarkers to predict the probability of disease in patients. The calibration curve of the nomogram was evaluated for prediction accuracy using the “regplot” package in R. The reliability of the nomogram was assessed through decision curve analysis (DCA) using the “rmda” package. Additionally, the “pROC” package was used to generate the ROC curve for the nomogram, with the AUC serving as a measure of diagnostic accuracy for IS. An AUC value greater than 0.7 was considered indicative of good performance.
2.6 Gene set enrichment analysis
To investigate the biological roles associated with the biomarkers and the differences in the signaling pathways involved, we performed the GSEA analysis. We used the “stats” package in R to calculate the Spearman correlation coefficients between the biomarkers and all other genes. Subsequently, genes were ranked based on their correlation coefficients, generating a list of genes correlated with each key gene. Enrichment analysis was then performed using the GSEA function from the “clusterProfiler” package in R. The background gene set was derived from the “c2. cp.kegg.v2023.1. Hs.symbols.gmt” file in the MsigDB database.
2.7 Immune infiltration analysis
To further assess the differences in immune status between the IS and control groups, immune cell infiltration was analyzed using the ssGSEA algorithm from the “GSVA” package in R. This analysis generated immune scores for each of the 28 immune cell types. The Wilcoxon rank-sum test function from the “stats” package in R was used to evaluate the differences in immune cell infiltration between the IS and control groups, with differential immune cells being selected based on a significance threshold of
2.8 Analysis of biomarker on single cell
To validate the bulk RNA sequencing results, we analyzed the expression of biomarkers at the single-cell level. We clustered the single-cell RNA sequencing (scRNA-seq) data to identify distinct cell types and examined the differences in cell type proportions and biomarker expression between the IS and control groups within each cell type. Additionally, we employed the CellPhoneDB tool to explore the intercellular communication network. To further explore the biological function of the biomarker in specific cell populations, we conducted virtual knockout (in silico KO) analyses. Single-cell RNA sequencing datasets derived from the peripheral blood and brain cells of stroke model mice were independently analyzed using a computational knockout approach (scTenifoldpy).
2.9 Participants
This study was carried out in accordance with the Declaration of Helsinki established by the World Medical Association and approved by the Institutional Review Board of Shanghai Pudong New Area Gongli Hospital (GLYYls 2024-078). The inclusion criteria were as follows: patients with no history of traumatic brain injury, brain tumors, stroke, or obvious neurological deficits within the past 3 months; no use of antiplatelet drugs, anticoagulants, or neuroprotective agents. The stroke onset in patients was within 3 days. A total of 21 stroke patients were recruited based on diagnosis by experienced neurologists and supporting imaging evidence, including 11 females and 10 males, with an average age of 68.67 years (SD = 9.92). The control group consisted of 26 participants, including 13 females and 13 males, with an average age of 66.15 years (SD = 8.70). All participants, or their legal representatives, provided written informed consent. Baseline characteristics of participants is in Table 1.
2.10 Real-time, fluorescence-based quantitative PCR
Peripheral blood samples from all participants were collected in EDTA anticoagulant tubes. GAPDH was used as the internal control gene, and the expression levels of three target genes (primer information can be found in Supplementary Table 2) were detected using SYBR GREEN I to analyze the target gene expression in the submitted samples. The primary instruments used were the analytikjena-qTOWER2.2 real-time PCR system (Germany), SCILOGEX D3024R centrifuge (United States), analytikjena-Easycycler PCR machine (Germany), scandrop100 microvolume nucleic acid and protein quantifier (Germany), pipettes (Bio-Rad, United States), and the M1 q-PCR automatic pipetting system (Sichuan Kejin). The main reagents and consumables used were the TUREscript 1st Strand cDNA Synthesis Kit (Aidelai, China),
2.11 Statistical analysis
Data analysis was performed using GraphPad Prism 10. Normality tests were conducted first. For data that followed a normal distribution, Student’s t-test was applied. Data that did not pass the normality test were analyzed using the nonparametric unpaired Mann-Whitney U test.
3 Results
3.1 A total of 17 candidate genes (differential -RGs) are identified
In the GSE58294 dataset, we identified 42 DEGs in the IS group compared to the control group, of which 22 genes were upregulated and 20 genes were downregulated (Figure 1A). Additionally, the
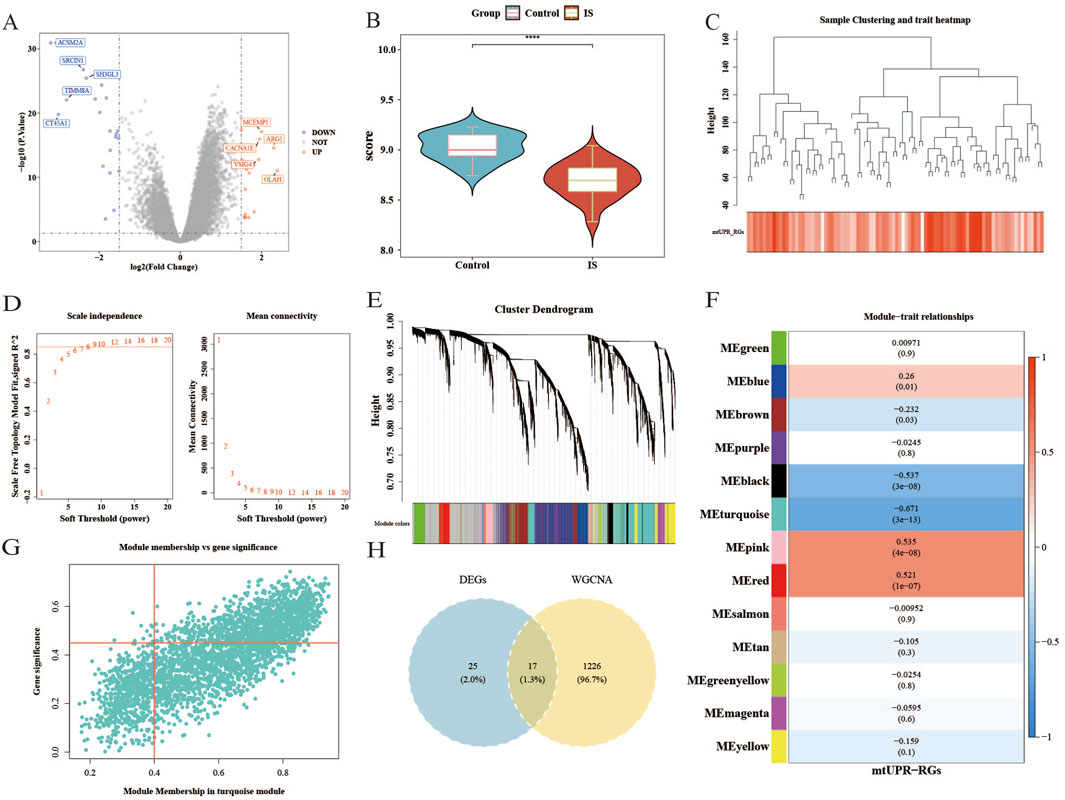
Figure 1. The gene expression profiles of the control group and the IS group, and weighted gene co-expression network analysis in GSE58294. (A) Volcano map of upregulated and downregulated genes in the IS group compared to the control group. (B) The differences in ssGSEA scores of
The results from the WGCNA indicated that the optimal soft threshold
3.2 Immune defense, apoptosis, and metabolic regulation are the primary processes associated with the candidate genes
A total of 249 GO biological functions, including 201 biological process (BP), 32 molecular function (MF), and 16 cellular component (CC) were enriched for candidate genes (Figure 2A). Functional enrichment analysis showed that in the BP, these candidate genes were primarily associated with positive regulation of myeloid leukocyte mediated immunity, neutrophil mediated immunity, myeloid leukocyte activation, defense response to fungus; In the CC, these candidate genes were mainly involved in specific granule, tertiary granule membrane, specific granule membrane, tertiary granule, secretory granule membrane; In the MF, these candidate genes were mainly involved in oxalate transmembrane transporter activity, cysteine-type endopeptidase activity involved in apoptotic signaling pathway, complement component C3b binding, high voltage-gated calcium channel activity, hydrolase activity, acting on carbon-nitrogen (but not peptide) bonds, in linear amidines.
The PPI network constructed based on candidate genes contained 35 interactions of 17 proteins. Notably, the CLEC4D and MCEMP1 interact with most of the proteins in the network (Figure 2B).
3.3 MCEMP1, CACNA1E and CLEC4D are identified as biomarkers
Utilizing LASSO analysis, the candidate genes with the smallest error (lambda.min = 0.0026) were selected, resulting in eight candidate characterization genes (Figures 3A,B). Additionally, Random Forest (RF) analysis (“ntree = 83”) identified another set of eight candidate characterization genes (Figures 3C,D). The intersection of these two gene sets resulted in four key candidate genes: LILRA5, MCEMP1, CACNA1E, and CLEC4D. To validate the expression levels of these four selected genes, we conducted an analysis in the GSE58294 and GSE16561 datasets. The results revealed significantly higher expression of these genes in the IS group compared to the control group, with consistent expression trends (***
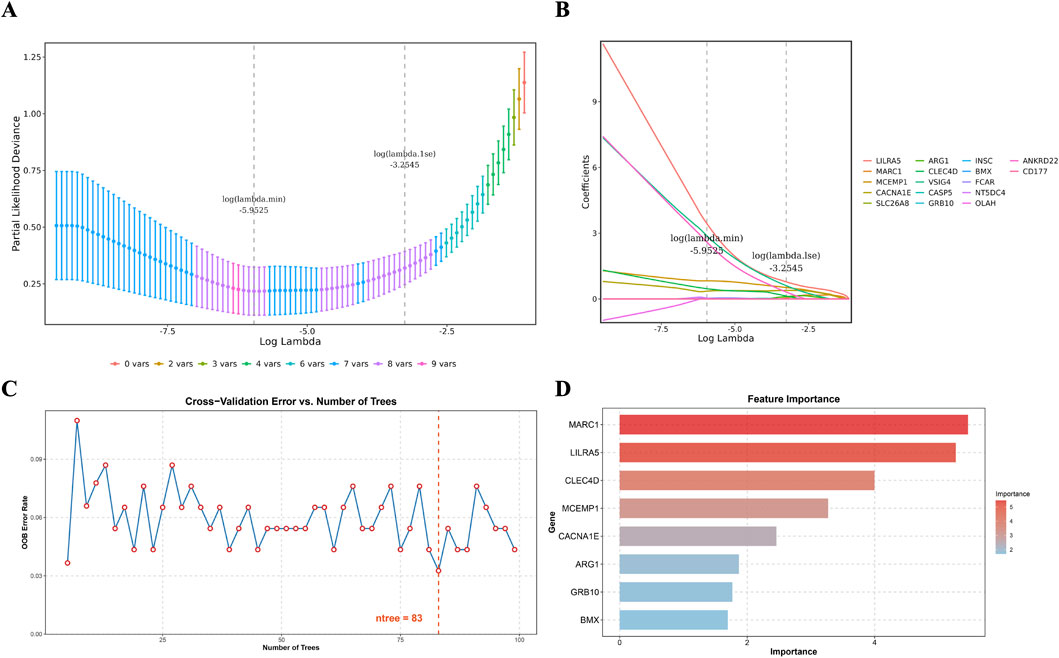
Figure 3. Candidate key genes were identified by machine learning. (A) Fitting deviation against the log(lambda) sequence. (B) The LASSO coefficients of the 17 candidate genes. (C) The relationship between the out-of-bag (OOB) error rate and the number of trees (ntree) in the random forest model. (D) Importance ranking of differential
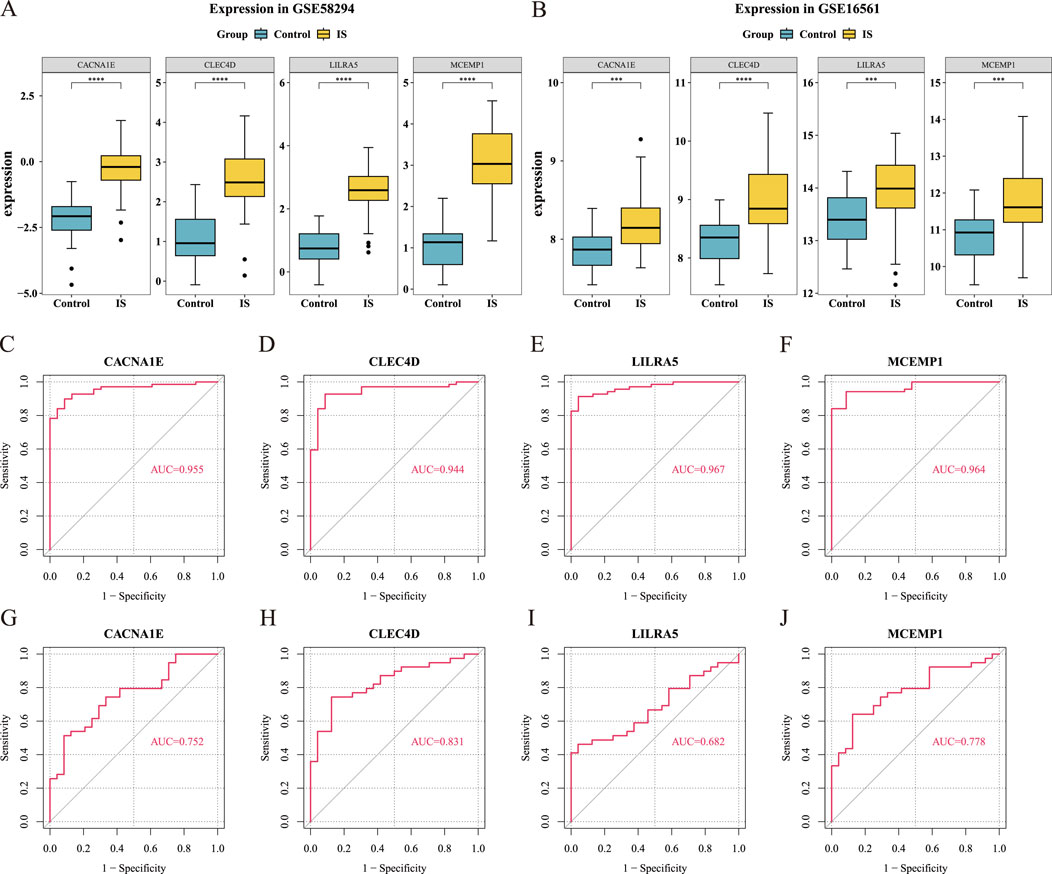
Figure 4. Validation of hub gene expression levels (Wilcoxon test, ***
To evaluate the diagnostic potential of these genes in distinguishing IS from control groups, we used ROC curve analysis. The results showed that the AUC values for MCEMP1, CACNA1E, and CLEC4D were greater than 0.7 in both GSE58294 and GSE16561, indicating that these three hub genes are potential biomarkers (Figures 4C–J). Comparison with well-established ischemic stroke biomarkers revealed that CLEC4D consistently achieved the highest average AUC (0.944 in GSE58294 and 0.831 in GSE16561), outperforming all classical biomarkers. Notably, MMP9 exhibited relatively high AUC values (0.863 in GSE58294 and 0.862 in GSE16561), but still slightly lower than CLEC4D (Supplementary Figure 3).
3.4 CLEC4D is a sensitive biomarker validated by RT-qPCR
To validate the expression levels of the identified biomarkers (CLEC4D, CACNA1E, and MCEMP1) in IS, we performed RT-qPCR analysis using peripheral blood samples from recruited participants. The RT-qPCR results (Figure 5; Supplementary Table 2) revealed that CLEC4D expression was significantly upregulated in the IS group compared to the healthy controls (**
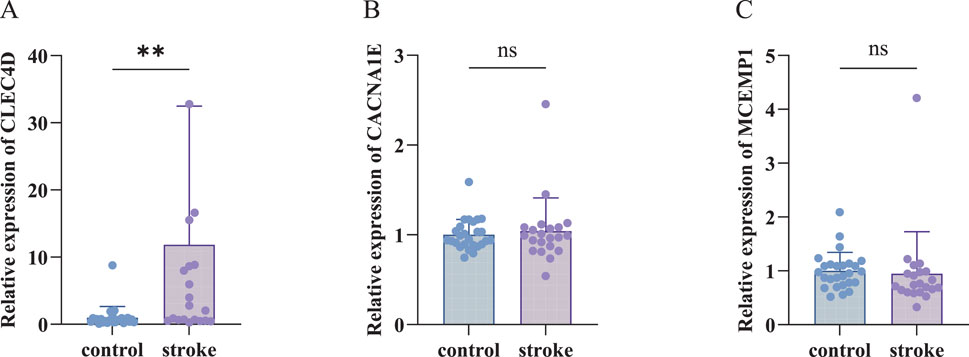
Figure 5. RT-qPCR Validation of biomarkers. (A) Relative expression of CLEC4D (nonparametric unpaired Mann-Whitney U test, **
3.5 The nomogram based on biomarkers is a valuable clinical assessment scale
In order to predict the probability of having IS by integrating biomarker, we constructed a nomogram. The nomogram assigns a point to each sample in GSE58294 based on the expression levels of the biomarkers. Each biomarker corresponds to a specific point, and the total point is obtained by summing the individual points of all biomarkers. The total point is then used to infer the likelihood of ischemic stroke (IS), with a higher point indicating an increased risk of IS. (Figure 6A). The good consistency of the calibration curve to the ideal curve indicated that the nomogram was more accurate
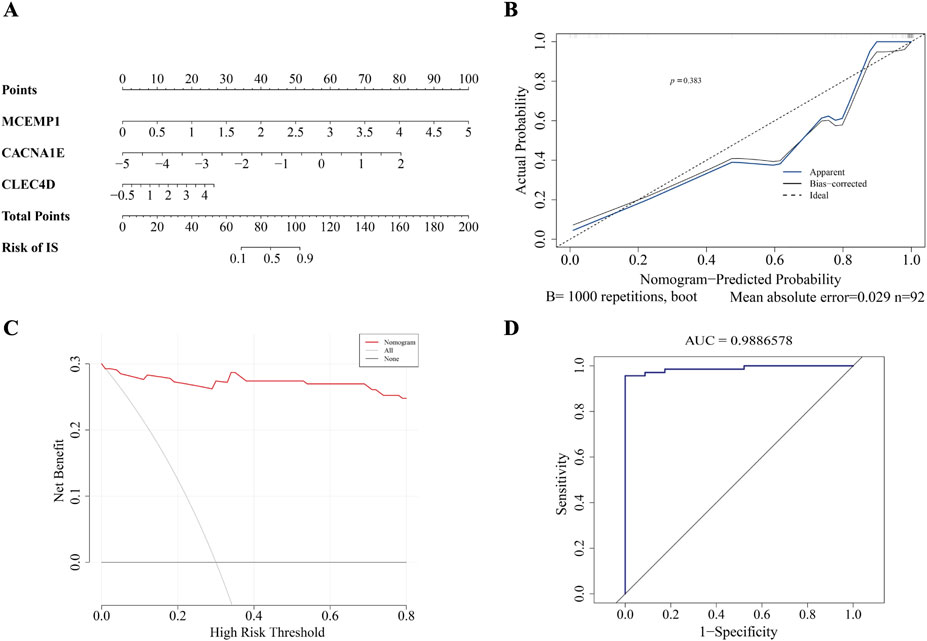
Figure 6. The construction of nomogram. (A) Nomogram. (B) Calibration curve. (C) DCA curve. (D) ROC curve.
3.6 GSEA identifies CLEC4D linked to immunity and protein homeostasis pathways
GSEA showed that CLEC4D was enriched in 37 functional pathways, with the top three being chronic myeloid leukemia, ubiquitin-mediated proteolysis, and protein export signaling pathway (Figure 7; Supplementary Table 3).
3.7 Immune infiltration analysis reveals neutrophils as the dominant biomarker carriers
Immune cell infiltration analysis was performed in GSE58294 to obtain 28 immune cell infiltration scores between the IS and control groups (Figure 8A). Then, we compared the differences in immune cell infiltration between the IS and control groups, and we got 21 differential immune cells (Figure 8B). The correlation analysis between differential immune cells and biomarkers revealed a notable positive association between CACNA1E and neutrophils (cor = 0.55,
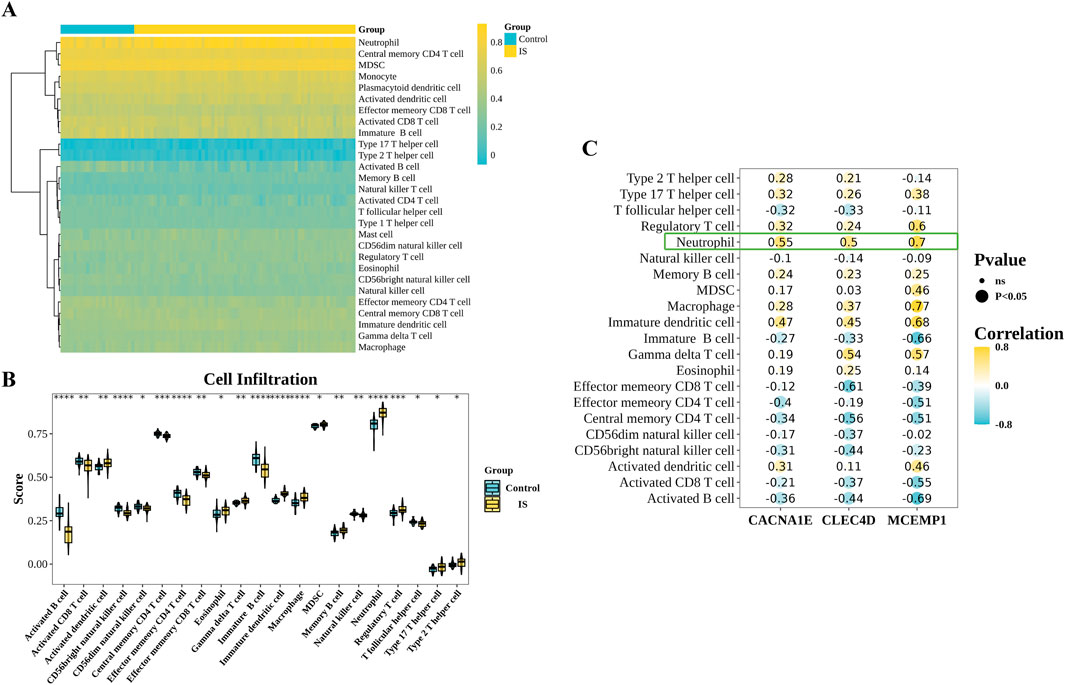
Figure 8. Immunoinfiltration analysis. (A) Immune cell abundance in the IS group and control group samples. (B) Differentially infiltrated immune cells between the IS group and control group samples (Wilcoxon test, ****
3.8 Single-cell analysis reveals neutrophils as key biomarker carriers
In the scRNA-seq dataset of mouse peripheral blood, we performed unsupervised clustering and uniform manifold approximation and projection (UMAP) to classify 43,269 cells into eight distinct clusters (Figure 9A). We observed a significant increase in the proportion of neutrophils in the MCAO group (Figure 9B). Furthermore, we validated the expression patterns of MCEMP1, CACNA1E and CLEC4D (Figures 9C–E) and found that these genes were predominantly enriched in neutrophils. Among them, CLEC4D exhibited significantly (****
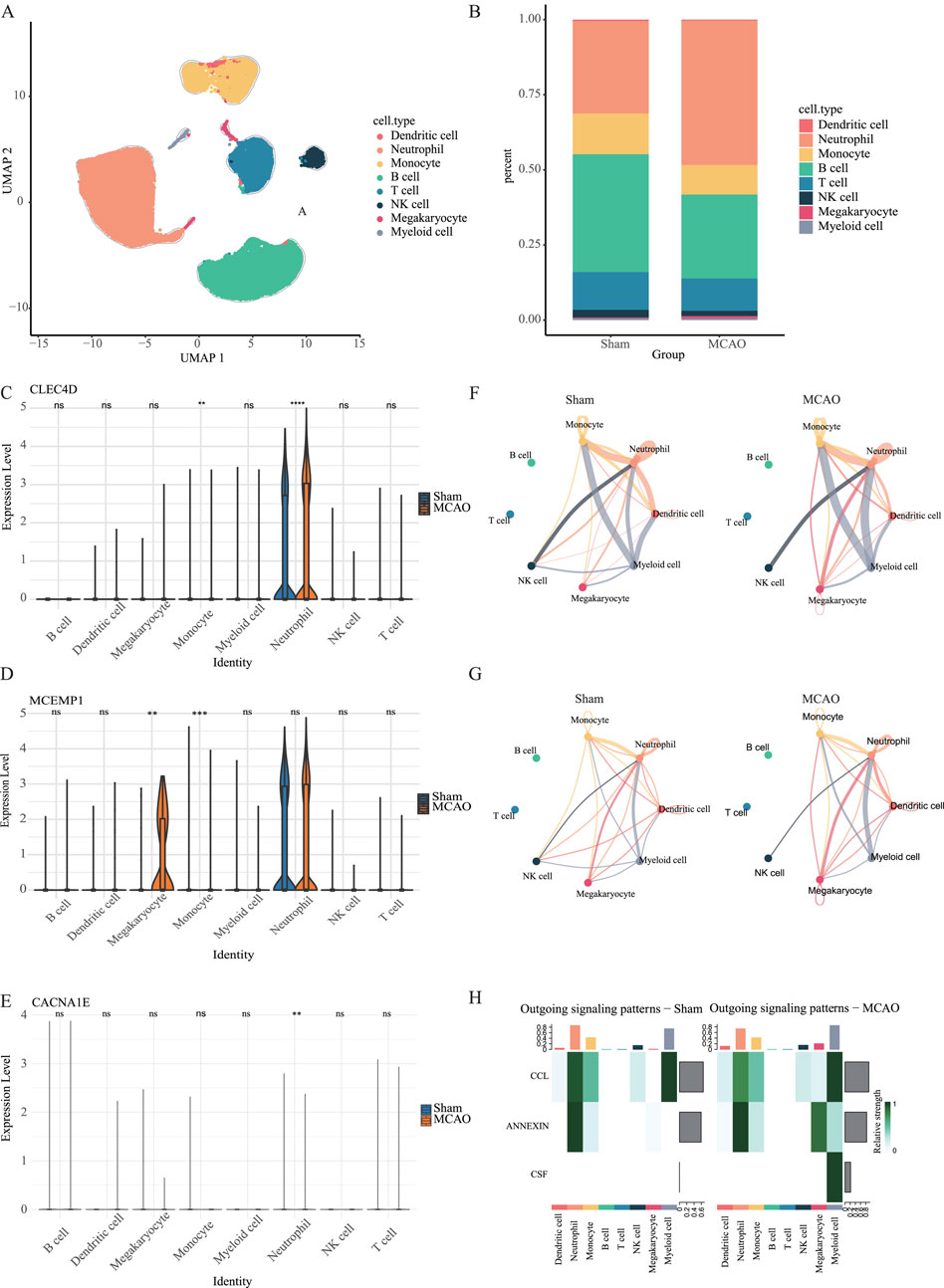
Figure 9. Single-cell analysis in the peripheral blood dataset. (A) UMAP of merged sham and MCAO groups. (B) The proportion of different cell types in the sham group and MCAO group. (C–E) The expression levels of CLEC4D, MCEMP1 and CACNA1E across different cell types in the sham group and MCAO group (ns
Additionally, we analyzed scRNA-seq data from brain cells. Using UMAP, we identified seven distinct cell clusters (Figure 10A). There is a significant increase of neutrophil proportion in the MCAO group within the first hour post-stroke
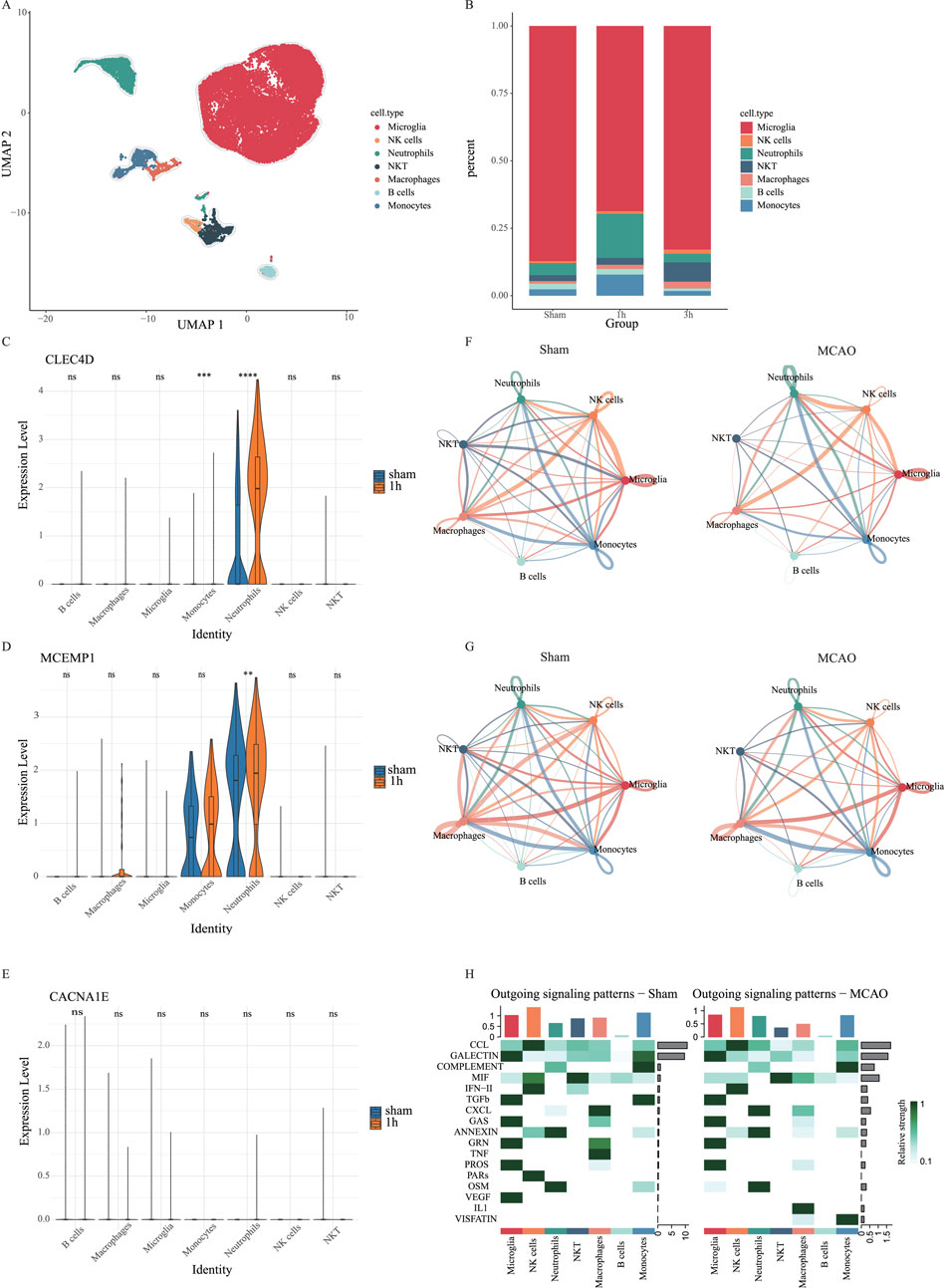
Figure 10. Single-cell analysis in the brain cell dataset. (A) UMAP of merged sham and MCAO groups. (B) The proportion of different cell types in the sham group, 1h-MCAO group and 3h-MCAO group. (C–E) The expression levels of CLEC4D, MCEMP1 and CACNA1E across different cell types in the sham group and 1h-MCAO group (ns
3.9 Neutrophil-mediated cell communication is enhanced
To further investigate the role of neutrophils in the post-stroke immune microenvironment, we conducted cell-cell communication analysis using CellPhoneDB. In the peripheral blood dataset, the results revealed a significant
Additionally, cell-cell communication analysis in brain tissue indicated a reduction in the number of intercellular communication pathways in the MCAO group due to cerebral infarction. However, interactions between neutrophils, macrophages, monocytes, and microglia were enhanced (Figure 10F), along with an increase in their communication pathways (Figure 10G). Compared to the sham group, the MCAO group exhibited vascular endothelial growth factor (VEGF) dysfunction in microglia, increased IL-1 signaling in macrophages, upregulated VISFATIN expression in monocytes, and enhanced CXCL signaling in neutrophils (Figure 10H). These findings suggest that neutrophils, macrophages, and monocytes may contribute to blood-brain barrier (BBB) disruption by intensifying their interactions with endothelial cells, ultimately exacerbating brain tissue damage. This study provides new insights into the neuroinflammatory response following stroke and suggests that targeting neutrophil-endothelial cell communication could be a potential therapeutic strategy for post-stroke inflammation.
3.10 Virtual knockout of CLEC4D reveals its regulatory role in neutrophil-mediated inflammation
In brain cell-derived immune cells, virtual knockout of CLEC4D in neutrophils led to significant transcriptional reprogramming. Differential gene expression analysis followed by enrichment analysis revealed prominent involvement of pathways associated with chromosome segregation, spindle elongation, Fc
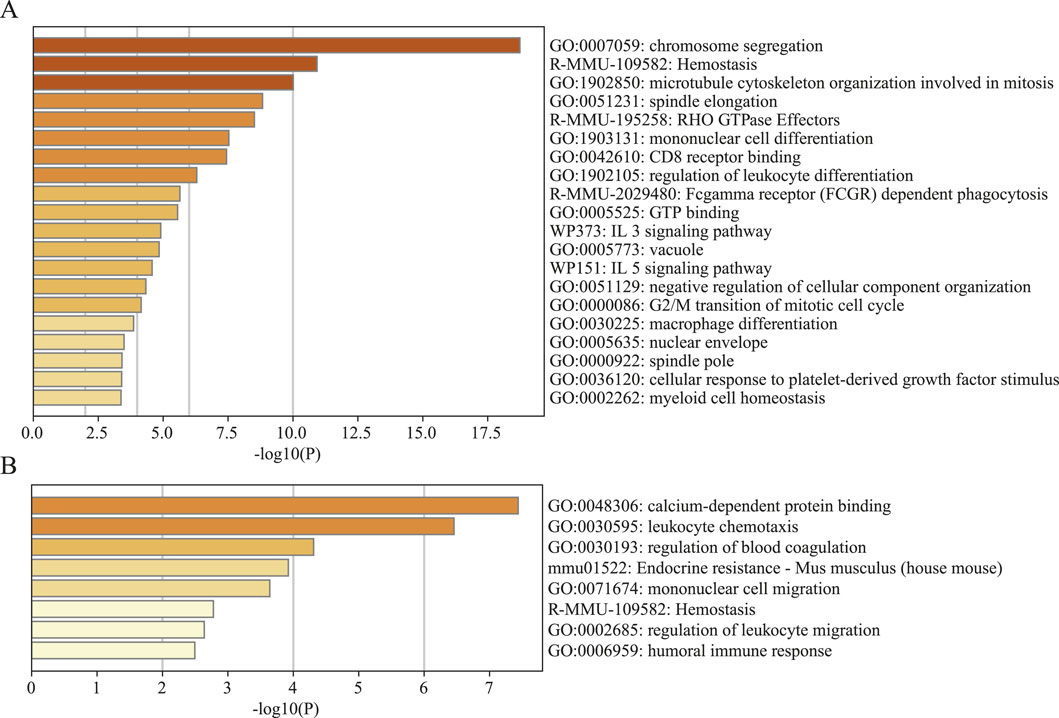
Figure 11. Gene set enrichment analysis (GSEA) of significantly altered genes by knocking out CLEC4D. (A) Brain cell sample. (B) Peripheral blood sample.
In contrast, the CLEC4D knockout in peripheral blood-derived cells induced a more limited response, with enrichment mainly in calcium-dependent protein binding, leukocyte chemotaxis, and regulation of blood coagulation (Figure 11B; Supplementary Table 3 GSEA of CLEC4D). This discrepancy highlights the tissue-specific functional dynamics of CLEC4D, suggesting a more active immunomodulatory role within the brain parenchyma following stroke.
Collectively, these data support the notion that CLEC4D is not merely a surface marker but likely contributes to the activation and effector functions of neutrophils in a context-dependent manner, particularly within the inflamed brain.
4 Discussion
We first identified key genes associated with IS and
IS leads to severe tissue damage due to the lack of blood supply, resulting in cell death and subsequent release of intracellular components. These released molecules, known as damage-associated molecular patterns (DAMPs), are crucial in triggering inflammation and immune responses. Major DAMPs involved in IS include high-mobility group box 1 (HMGB1) and peroxidases (PRX). After cell death, these molecules leak into the extracellular space and act as danger signals to the immune system (Nakamura et al., 2020; Simats and Liesz, 2022; Alsbrook et al., 2023).
DAMPs are released from ischemic cells and recognized by pattern recognition receptors (PRRs) expressed on immune cells such as microglia, macrophages, and neutrophils. For instance, HMGB1 increases the expression of matrix metalloproteinase 9 (MMP9), which contributes to blood-brain barrier (BBB) disruption and exacerbates inflammation (Li et al., 2024; Liao et al., 2024). Additionally, HMGB1 activates neutrophils and induces the release of neutrophil extracellular traps (NETs), further promoting the inflammatory cascade (Gao et al., 2024b). PRX, a protein that reduces oxidative stress by metabolizing hydrogen peroxide, is also released from ischemic cells and activates microglia and infiltrating macrophages. This activation promotes the release of pro-inflammatory cytokines such as TNF-
Based on our findings, one key PRR in this process is CLEC4D. CLEC4D is a C-type lectin receptor primarily expressed on myeloid cells, including neutrophils and monocytes. CLEC4D plays an essential role in pathogen recognition, phagocytosis, and the initiation of inflammatory responses (Guerrero-Juarez et al., 2023). Our GSEA further revealed that CLEC4D is significantly enriched in pathways such as chronic myelogenous leukemia (CML), involving critical signaling pathways like JAK-STAT, MAPK, and PI3K-AKT (Singh et al., 2021). These pathways also play pivotal roles in post-stroke inflammation. For instance, STAT3 has been shown to mediate endogenous IL-6 to prevent apoptosis of neurons in the acute phase of cerebral ischemia (Yamashita et al., 2005). Activation of the PI3K/AKT signaling pathway can suppress the expression of pro-inflammatory factors induced by NF-
The relationship between the inflammatory response triggered by DAMP-PRR interactions and
However, it is important to acknowledge several limitations in this study. First, the limited sample size and sources may affect the statistical significance and biological generalizability of the data. Future research should validate these findings through multi-center, longitudinal studies. Second, we were unable to perform protein-level validation of CLEC4D expression (e.g., Western blot) due to sample and technical limitations. However, relevant evidence from the literature provides indirect support for our findings. CLEC4E, a closely related C-type lectin family member, has been shown to be significantly upregulated at both the mRNA and protein levels in microglia following ischemic stroke, as demonstrated in a recent study by Liu et al. (2023). CLEC4E (encoding the Mincle receptor) mediates proinflammatory responses by binding to damage-associated molecular patterns (DAMPs), such as properdin, and subsequently activating downstream Syk/NF-
From a clinical translation perspective, CLEC4D and its associated signaling pathways represent promising therapeutic targets for ischemic stroke. As a myeloid C-type lectin receptor, CLEC4D interacts with Fc
5 Conclusion
In summary, this study not only identifies the abnormal expression of key genes such as CLEC4D in ischemic stroke through large-scale data mining but also proposes a hypothesis that CLEC4D may regulate the balance between inflammation activation and
Data availability statement
The original contributions presented in the study are included in the article/Supplementary Material, further inquiries can be directed to the corresponding authors.
Ethics statement
The studies involving humans were approved by the Institutional Review Board of Shanghai Pudong New Area Gongli Hospital (GLYYls2024-078). The studies were conducted in accordance with the local legislation and institutional requirements. The participants provided their written informed consent to participate in this study. Ethical approval was not required for the study involving animals in accordance with the local legislation and institutional requirements because We used public data and did not involve any animal testing. Written informed consent was obtained from the individual(s) for the publication of any potentially identifiable images or data included in this article.
Author contributions
LZ: Conceptualization, Data curation, Formal Analysis, Investigation, Methodology, Project administration, Software, Supervision, Validation, Visualization, Writing – original draft, Writing – review and editing. LY: Writing – review and editing. PJ: Writing – review and editing, Methodology. ZC: Conceptualization, Formal Analysis, Investigation, Methodology, Software, Visualization, Writing – original draft, Writing – review and editing. JL: Data curation, Funding acquisition, Project administration, Resources, Supervision, Writing – review and editing.
Funding
The author(s) declare that financial support was received for the research and/or publication of this article. Shanghai Pudong New Area Science and Technology Development Fund public institutions livelihood scientific research special program (PKJ2024-Y28). Discipline Construction Project of Shanghai Pudong New Area Health Commission (Key Discipline Group) (PWZxq2022-12). Clinical Characteristic Discipline Construction Project of Shanghai Pudong New Area Health Commission (PWYts2021-17).
Conflict of interest
The authors declare that the research was conducted in the absence of any commercial or financial relationships that could be construed as a potential conflict of interest.
Generative AI statement
The author(s) declare that no Generative AI was used in the creation of this manuscript.
Publisher’s note
All claims expressed in this article are solely those of the authors and do not necessarily represent those of their affiliated organizations, or those of the publisher, the editors and the reviewers. Any product that may be evaluated in this article, or claim that may be made by its manufacturer, is not guaranteed or endorsed by the publisher.
Supplementary material
The Supplementary Material for this article can be found online at: https://www.frontiersin.org/articles/10.3389/fcell.2025.1582252/full#supplementary-material
References
Allard, L., Lescuyer, P., Burgess, J., Leung, K.-Y., Ward, M., Walter, N., et al. (2004). Apoc-i and apoc-iii as potential plasmatic markers to distinguish between ischemic and hemorrhagic stroke. Proteomics 4, 2242–2251. doi:10.1002/pmic.200300809
Alsbrook, D. L., Di Napoli, M., Bhatia, K., Biller, J., Andalib, S., Hinduja, A., et al. (2023). Neuroinflammation in acute ischemic and hemorrhagic stroke. Curr. neurology Neurosci. Rep. 23, 407–431. doi:10.1007/s11910-023-01282-2
An, H., Zhou, B., Hayakawa, K., Durán Laforet, V., Park, J.-H., Nakamura, Y., et al. (2024). Atf5-mediated mitochondrial unfolded protein response (uprmt) protects neurons against oxygen-glucose deprivation and cerebral ischemia. Stroke 55, 1904–1913. doi:10.1161/STROKEAHA.123.045550
An, H., Zhou, B., and Ji, X. (2021). Mitochondrial quality control in acute ischemic stroke. J. Cereb. Blood Flow & Metabolism 41, 3157–3170. doi:10.1177/0271678X211046992
Bathla, G., Ajmera, P., Mehta, P., Benson, J., Derdeyn, C., Lanzino, G., et al. (2023). Advances in acute ischemic stroke treatment: current status and future directions. Am. J. Neuroradiol. 44, 750–758. doi:10.3174/ajnr.A7872
Chavda, V., Chaurasia, B., Garg, K., Deora, H., Umana, G. E., Palmisciano, P., et al. (2022). Molecular mechanisms of oxidative stress in stroke and cancer. Brain Disord. 5, 100029. doi:10.1016/j.dscb.2021.100029
Chavda, V., and Lu, B. (2023). Reverse electron transport at mitochondrial complex i in ischemic stroke, aging, and age-related diseases. Antioxidants 12, 895. doi:10.3390/antiox12040895
Cuadrado, E., Rosell, A., Penalba, A., Slevin, M., Alvarez-Sabín, J., Ortega-Aznar, A., et al. (2009). Vascular mmp-9/timp-2 and neuronal mmp-10 up-regulation in human brain after stroke: a combined laser microdissection and protein array study. J. proteome Res. 8, 3191–3197. doi:10.1021/pr801012x
El Jamal, S. M., Taylor, E. B., Abd Elmageed, Z. Y., Alamodi, A. A., Selimovic, D., Alkhateeb, A., et al. (2016). Interferon gamma-induced apoptosis of head and neck squamous cell carcinoma is connected to indoleamine-2, 3-dioxygenase via mitochondrial and er stress-associated pathways. Cell Div. 11, 11–14. doi:10.1186/s13008-016-0023-4
Feigin, V. L., Forouzanfar, M. H., Krishnamurthi, R., Mensah, G. A., Connor, M., Bennett, D. A., et al. (2014). Global and regional burden of stroke during 1990–2010: findings from the global burden of disease study 2010. lancet 383, 245–254. doi:10.1016/s0140-6736(13)61953-4
Gao, L., Peng, L., Wang, J., Zhang, J. H., and Xia, Y. (2024a). Mitochondrial stress: a key role of neuroinflammation in stroke. J. Neuroinflammation 21, 44. doi:10.1186/s12974-024-03033-7
Gao, X., Zhao, X., Li, J., Liu, C., Li, W., Zhao, J., et al. (2024b). Neutrophil extracellular traps mediated by platelet microvesicles promote thrombosis and brain injury in acute ischemic stroke. Cell Commun. Signal. 22, 50. doi:10.1186/s12964-023-01379-8
Guerrero-Juarez, C. F., Schilf, P., Li, J., Zappia, M. P., Bao, L., Patel, P. M., et al. (2023). C-type lectin receptor expression is a hallmark of neutrophils infiltrating the skin in epidermolysis bullosa acquisita. Front. Immunol. 14, 1266359. doi:10.3389/fimmu.2023.1266359
Hahn, W. S., Kuzmicic, J., Burrill, J. S., Donoghue, M. A., Foncea, R., Jensen, M. D., et al. (2014). Proinflammatory cytokines differentially regulate adipocyte mitochondrial metabolism, oxidative stress, and dynamics. Am. J. Physiology-Endocrinology Metabolism 306, E1033–E1045. doi:10.1152/ajpendo.00422.2013
He, X., Li, Y., Deng, B., Lin, A., Zhang, G., Ma, M., et al. (2022). The pi3k/akt signalling pathway in inflammation, cell death and glial scar formation after traumatic spinal cord injury: mechanisms and therapeutic opportunities. Cell Prolif. 55, e13275. doi:10.1111/cpr.13275
Inigo, J. R., and Chandra, D. (2022). The mitochondrial unfolded protein response (uprmt): shielding against toxicity to mitochondria in cancer. J. Hematol. & Oncol. 15, 98. doi:10.1186/s13045-022-01317-0
Jelinek, M., Jurajda, M., and Duris, K. (2021). Oxidative stress in the brain: basic concepts and treatment strategies in stroke. Antioxidants 10, 1886. doi:10.3390/antiox10121886
Johns, A., Higuchi-Sanabria, R., Thorwald, M. A., and Vilchez, D. (2023). A tale of two pathways: regulation of proteostasis by uprmt and mdps. Curr. Opin. Neurobiol. 78, 102673. doi:10.1016/j.conb.2022.102673
Joshi, A., Orset, C., Engelhardt, B., Baumgart-Vogt, E., Gerriets, T., Vivien, D., et al. (2015). Deficiency of factor vii activating protease alters the outcome of ischemic stroke in mice. Eur. J. Neurosci. 41, 965–975. doi:10.1111/ejn.12830
Li, J., Wang, Z., Li, J., Zhao, H., and Ma, Q. (2024). Hmgb1: a new target for ischemic stroke and hemorrhagic transformation. Transl. Stroke Res., 1–26. doi:10.1007/s12975-024-01258-5
Li, M., Zhang, R., Li, J., and Li, J. (2022). The role of c-type lectin receptor signaling in the intestinal microbiota-inflammation-cancer axis. Front. Immunol. 13, 894445. doi:10.3389/fimmu.2022.894445
Liao, Y., Hu, J., Guo, C., Wen, A., Wen, L., Hou, Q., et al. (2024). Acteoside alleviates blood–brain barrier damage induced by ischemic stroke through inhibiting microglia hmgb1/tlr4/nlrp3 signaling. Biochem. Pharmacol. 220, 115968. doi:10.1016/j.bcp.2023.115968
Liu, P.-y., Li, H.-q., Dong, M.-q., Gu, X.-y., Xu, S.-y., Xia, S.-n., et al. (2023). Infiltrating myeloid cell-derived properdin markedly promotes microglia-mediated neuroinflammation after ischemic stroke. J. Neuroinflammation 20, 260. doi:10.1186/s12974-023-02946-z
Lopez, M. F., Sarracino, D. A., Prakash, A., Athanas, M., Krastins, B., Rezai, T., et al. (2012). Discrimination of ischemic and hemorrhagic strokes using a multiplexed, mass spectrometry-based assay for serum apolipoproteins coupled to multi-marker roc algorithm. PROTEOMICS–Clinical Appl. 6, 190–200. doi:10.1002/prca.201100041
Maalouf, E., Hallit, S., Salameh, P., and Hosseini, H. (2023). Eating behaviors, lifestyle, and ischemic stroke: a lebanese case-control study. Int. J. Environ. Res. Public Health 20, 1487. doi:10.3390/ijerph20021487
Muñoz-Carvajal, F., and Sanhueza, M. (2020). The mitochondrial unfolded protein response: a hinge between healthy and pathological aging. Front. Aging Neurosci. 12, 581849. doi:10.3389/fnagi.2020.581849
Nakamura, A., Otani, K., and Shichita, T. (2020). Lipid mediators and sterile inflammation in ischemic stroke. Int. Immunol. 32, 719–725. doi:10.1093/intimm/dxaa027
Pellegrino, M. W., Nargund, A. M., Kirienko, N. V., Gillis, R., Fiorese, C. J., and Haynes, C. M. (2014). Mitochondrial upr-regulated innate immunity provides resistance to pathogen infection. Nature 516, 414–417. doi:10.1038/nature13818
Rochmah, T. N., Rahmawati, I. T., Dahlui, M., Budiarto, W., and Bilqis, N. (2021). Economic burden of stroke disease: a systematic review. Int. J. Environ. Res. public health 18, 7552. doi:10.3390/ijerph18147552
Simats, A., and Liesz, A. (2022). Systemic inflammation after stroke: implications for post-stroke comorbidities. EMBO Mol. Med. 14, e16269. doi:10.15252/emmm.202216269
Singh, P., Kumar, V., Gupta, S. K., Kumari, G., and Verma, M. (2021). Combating tki resistance in cml by inhibiting the pi3k/akt/mtor pathway in combination with tkis: a review. Med. Oncol. 38, 10–16. doi:10.1007/s12032-021-01462-5
Sun, G.-w., Ding, T.-y., Wang, M., Hu, C.-l., Gu, J.-j., Li, J., et al. (2022). Honokiol reduces mitochondrial dysfunction and inhibits apoptosis of nerve cells in rats with traumatic brain injury by activating the mitochondrial unfolded protein response. J. Mol. Neurosci. 72, 2464–2472. doi:10.1007/s12031-022-02089-5
Sun, J., and Nan, G. (2016). The mitogen-activated protein kinase (mapk) signaling pathway as a discovery target in stroke. J. Mol. Neurosci. 59, 90–98. doi:10.1007/s12031-016-0717-8
Tan, X., Chen, J., Keep, R. F., Xi, G., and Hua, Y. (2020). Prx2 (peroxiredoxin 2) as a cause of hydrocephalus after intraventricular hemorrhage. Stroke 51, 1578–1586. doi:10.1161/STROKEAHA.119.028672
Tao, T., Liu, M., Chen, M., Luo, Y., Wang, C., Xu, T., et al. (2020). Natural medicine in neuroprotection for ischemic stroke: challenges and prospective. Pharmacol. & Ther. 216, 107695. doi:10.1016/j.pharmthera.2020.107695
Torres, A. K., Fleischhart, V., and Inestrosa, N. C. (2024). Mitochondrial unfolded protein response (uprmt): what we know thus far. Front. Cell Dev. Biol. 12, 1405393. doi:10.3389/fcell.2024.1405393
Xiaowei, X., Qian, X., and Dingzhou, Z. (2023). Sirtuin-3 activates the mitochondrial unfolded protein response and reduces cerebral ischemia/reperfusion injury. Int. J. Biol. Sci. 19, 4327–4339. doi:10.7150/ijbs.86614
Yamashita, T., Sawamoto, K., Suzuki, S., Suzuki, N., Adachi, K., Kawase, T., et al. (2005). Blockade of interleukin-6 signaling aggravates ischemic cerebral damage in mice: possible involvement of stat3 activation in the protection of neurons. J. Neurochem. 94, 459–468. doi:10.1111/j.1471-4159.2005.03227.x
Yan, J., Zhang, X., Wang, H., Jia, X., Wang, R., Wu, S., et al. (2024). Macrophage nrf1 promotes mitochondrial protein turnover via the ubiquitin proteasome system to limit mitochondrial stress and inflammation. Cell Rep. 43, 114780. doi:10.1016/j.celrep.2024.114780
Yang, C., Hawkins, K. E., Doré, S., and Candelario-Jalil, E. (2019). Neuroinflammatory mechanisms of blood-brain barrier damage in ischemic stroke. Am. J. Physiology-Cell Physiology 316, C135–C153. doi:10.1152/ajpcell.00136.2018
Yang, D., Elner, S. G., Bian, Z.-M., Till, G. O., Petty, H. R., and Elner, V. M. (2007). Pro-inflammatory cytokines increase reactive oxygen species through mitochondria and nadph oxidase in cultured rpe cells. Exp. eye Res. 85, 462–472. doi:10.1016/j.exer.2007.06.013
Yang, L., Qian, J., Yang, B., He, Q., Wang, J., and Weng, Q. (2021). Challenges and improvements of novel therapies for ischemic stroke. Front. Pharmacol. 12, 721156. doi:10.3389/fphar.2021.721156
Yu, Y., Wang, R.-R., Miao, N.-J., Tang, J.-J., Zhang, Y.-W., Lu, X.-R., et al. (2022). Pd-l1 negatively regulates antifungal immunity by inhibiting neutrophil release from bone marrow. Nat. Commun. 13, 6857. doi:10.1038/s41467-022-34722-7
Zhang, S., Guo, H., Wang, H., Liu, X., Wang, M., Liu, X., et al. (2024). A novel mitochondrial unfolded protein response-related risk signature to predict prognosis, immunotherapy and sorafenib sensitivity in hepatocellular carcinoma. Apoptosis 29, 768–784. doi:10.1007/s10495-024-01945-6
Zhou, Q., Zhu, L., Qiu, W., Liu, Y., Yang, F., Chen, W., et al. (2022). Erratum: nicotinamide riboside enhances mitochondrial proteostasis and adult neurogenesis through activation of mitochondrial unfolded protein response signaling in the brain of ALS SOD1G93A mice: erratum. Int. J. Biol. Sci. 18, 2181–2183. doi:10.7150/ijbs.71902
Keywords: bioinformation, biomarker, bulk RNA-seq, ischemic stroke, single cell, mitochondrial unfolded protein response, neutrophils, virtual knockout experiments
Citation: Zhang L, Yue L, Jia P, Cheng Z and Liu J (2025) Comprehensive transcriptomic analysis integrating bulk and single-cell RNA-seq with machine learning to identify and validate mitochondrial unfolded protein response biomarkers in patients with ischemic stroke. Front. Cell Dev. Biol. 13:1582252. doi: 10.3389/fcell.2025.1582252
Received: 24 February 2025; Accepted: 07 April 2025;
Published: 17 April 2025.
Edited by:
Shikai Yu, Tongji University, ChinaReviewed by:
Virginia Actis Dato, University of California San Diego, United StatesBaohua Hou, Henan Polytechnic University, China
Copyright © 2025 Zhang, Yue, Jia, Cheng and Liu. This is an open-access article distributed under the terms of the Creative Commons Attribution License (CC BY). The use, distribution or reproduction in other forums is permitted, provided the original author(s) and the copyright owner(s) are credited and that the original publication in this journal is cited, in accordance with accepted academic practice. No use, distribution or reproduction is permitted which does not comply with these terms.
*Correspondence: Lu Zhang, bHV6aGFuZzE5QGZ1ZGFuLmVkdS5jbg==; Ziqi Cheng, Y2hlbmd6aXFpQHdodS5lZHUuY24=; Jiwen Liu, c2VhMTczMTFAaG90bWFpbC5jb20=