- School of Communication Engineering, Hangzhou Dianzi University, Hangzhou, China
As one of the important statistical methods, quantile regression (QR) extends traditional regression analysis. In QR, various quantiles of the response variable are modeled as linear functions of the predictors, allowing for a more flexible analysis of how the predictors affect different parts of the response variable distribution. QR offers several advantages over standard linear regression due to its focus on estimating conditional quantiles rather than the conditional mean of the response variable. This paper investigates QR over sensor networks, where each node has access to a local dataset and collaboratively estimates a global QR model. QR solves a non-smooth optimization problem characterized by a piecewise linear loss function, commonly known as the check function. We reformulate this non-smooth optimization problem as the task of finding a saddle point of a convex–concave objective and develop a distributed primal–dual hybrid gradient (dPDHG) algorithm for this purpose. Theoretical analyses guarantee the convergence of the proposed algorithm under mild assumptions, while experimental results show that the dPDHG algorithm converges significantly faster than subgradient-based schemes.
1 Introduction
Distributed signal processing (Hovine and Bertrand, 2024; Cattivelli and Sayed, 2010; Schizas et al., 2009) in wireless sensor networks addresses the challenges of limited energy, processing power, and communication range of individual sensors. By applying collaborative computational algorithms, sensors can operate as a distributed signal processor, overcoming individual limitations and improving energy efficiency. Distributed signal processing is particularly used in various applications where distributed algorithms can enhance the power efficiency by avoiding data centralization, including environmental monitoring, healthcare, and military surveillance. However, the complexity of real-world sensor data often involves non-linear relationships, heteroscedasticity, and outliers. In such environments, traditional distributed methods like least squares regression may fail to provide robust estimates due to the influence of extreme values or noise.
Quantile regression (QR) (Waldmann, 2018) offers a solution by estimating conditional quantiles of the data distribution, rather than just the mean, making it more robust to outliers and better suited for modeling heterogeneous data sources. Quantile regression has gained significant attention as a robust approach to regression analysis, particularly in situations where the distribution of the response variable is not symmetric or when outliers are present, and has applications in various fields, including ecology, economics, and industry (Cade and Noon, 2003; Ben Taieb et al., 2016; Wan et al., 2017). Some efficient numerical methods, including alternating direction method of multipliers (ADMM) (Mirzaeifard et al., 2024; Bazzi and Chafii, 2023), majorize–minimize (MM) (Kai et al., 2023; Cheng and Kuk, 2024), and machine learning (Patidar et al., 2023; Hüttel et al., 2022), were used for solving the optimization problem associated with quantile regression. Recent research has focused on distributed quantile regression (dQR) in sensor networks. In distributed sensor networks, where data from different sensors can vary significantly in terms of noise and variability, quantile regression can be applied at the local level to estimate the distributional characteristics of the data at each sensor node. Wang and Li (2018) proposed a diffusion-based distributed strategy [including a variant for sparse models (Bazzi et al., 2017)] for quantile regression over wireless sensor networks. Wang and Lian (2023), Lee et al. (2018), and Lee et al. (2020) introduced several consensus-based dQR methods for sensor networks. These methods overcome challenges in distributed settings, including limited storage and transmission power, while maintaining statistical robustness. They offer promising solutions for quantile-based analyses in decentralized sensor networks across diverse applications.
It should be noted that quantile regression involves a non-differentiable optimization problem with a piecewise linear loss function, also known as the check function. Most existing quantile regression algorithms rely on subgradient methods, which typically exhibit sublinear convergence rates. Although these methods have certain merits, they often struggle with slow convergence when tackling the non-differentiability of the optimization problem. Alternatively, techniques such as MM (Kai et al., 2023; Cheng and Kuk, 2024) mitigate the non-differentiability issue by minimizing a smooth majorizer of the check function instead of the function itself. However, these methods can introduce additional computational complexity and may not fully exploit the structure of distributed settings. As a data-driven approach, the machine learning-based methods (Patidar et al., 2023; Hüttel et al., 2022; Delamou et al., 2023; Njima et al., 2022) can circumvent the non-differentiability issue. However, it relies heavily on the availability of large datasets, which may not always be feasible or efficient in certain scenarios.
In this paper, we propose a novel approach for diffusion-based distributed quantile regression, leveraging the primal–dual hybrid gradient method to find a saddle point of a convex–concave objective. This strategy accelerates convergence and significantly enhances the efficiency of the quantile regression process.
2 Network model and problem formulation
2.1 Preliminaries
In this section, we present a brief introduction to quantile regression. Let
for
In the quantile regression model,
Here,
This function adjusts the loss asymmetrically depending on whether the residual
2.2 Network model and problem formulation
Consider a network consisting of
where
The aim of this study is to develop a distributed quantile regression algorithm to estimate
where
Here,
This condition (Equation 5) is explicitly satisfied by widely used weight rules in the distributed optimization literature (Cattivelli and Sayed, 2010; Tu and Sayed, 2011). In addition,
We assume that the underlying network operates under ideal and stable communication conditions. Transient link or node failures—well-studied in the distributed network literature (Gao et al., 2022; Swain et al., 2018)—are effectively handled using established engineering solutions, such as fault-tolerant protocols, redundancy, and consensus mechanisms, thereby ensuring system reliability.
3 Distributed primal–dual hybrid gradient algorithm
This section first introduces a distributed quantile regression framework and formulates our problem as a saddle-point optimization problem. Subsequently, a distributed primal–dual hybrid gradient algorithm (dPDHG) for quantile regression is proposed, and its convergence is analyzed.
3.1 Diffusion-based distributed estimation framework
Since the main task of quantile regression is to estimate the parameter vector
By definition in Equation 4, the local cost function of node
Based on this idea, we will use a distributed strategy in the following subsections to solve the problem (Equation 3).
This study focuses on a diffusion-based framework for decentralized quantile regression. The integration of consensus-based strategies with primal–dual hybrid gradient methods for distributed quantile regression, which presents significant algorithmic challenges, is left for future investigation.
3.2 Dual problem and saddle point optimization
By definition, we split
Using the conjugate function
This suggests that
where
We can obtain a dual problem associated with the original function minimization problem
This indicates that the global optimization problem (Equation 3) can be formulated as
which has a standard saddle-point optimization problem expression with the primal variable
3.3 Algorithmic principles and derivations of the dPDHG
This section presents the algorithmic principles and derivations of the proposed dPDHG to solve Equation 9. Its basic framework includes the following steps (a)–(d), which involve iterative updates of the primal and dual variables
(a) Update the primal variable
where
with
(b) Update the auxiliary variable
(c) Each node
(d) Update the dual variable
where one can deduce that the proximal operator
(e) Assign global
The algorithm continues iterating until the stopping criterion is met, typically based on the difference between successive iterates or the duality gap. Finally, we summarize the proposed dPDHG algorithm in Table 1.
3.4 Selection of the primal–dual step sizes
In the above steps,
Note that, for each
where
4 Simulation examples
We consider a connected network with
where
We begin by evaluating the performance of the distribution estimation for
Figures 1–3 illustrate the transient-network mean-square deviation (MSD) performance of our proposed dPDHG algorithm, compared with three benchmark algorithms: the subgradient-based algorithm (Subgrad) (Wang and Li, 2018), the majorization–minimization algorithm (MM) (Kai et al., 2023), accelerated proximal-based gradient methods(APG) (Chen and Ozdaglar, 2012), and the least mean squares (LMS) algorithm (Liu et al., 2012). The transient network MSD is defined as
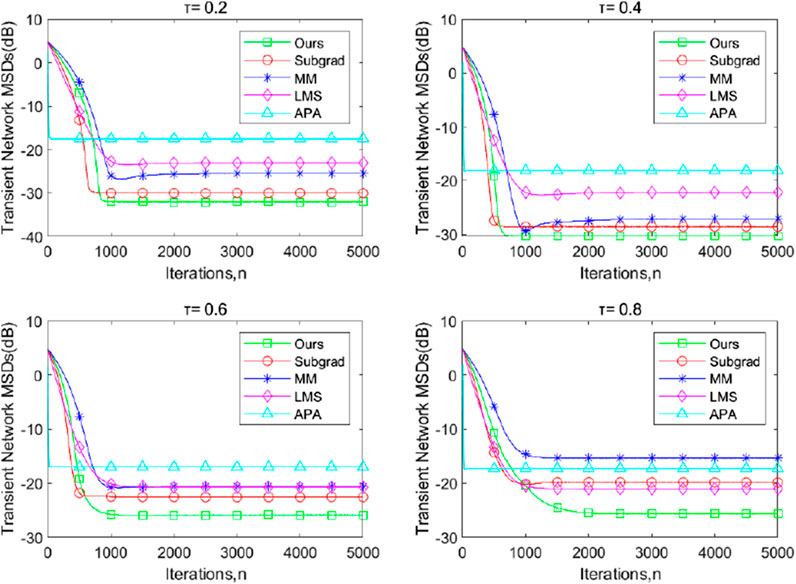
Figure 1. The transient network MSDs of the proposed dPDHG algorithm compared with three benchmark distributed algorithms: the Subgrad, the MM, and the LMS, and the APA algorithms for estimating
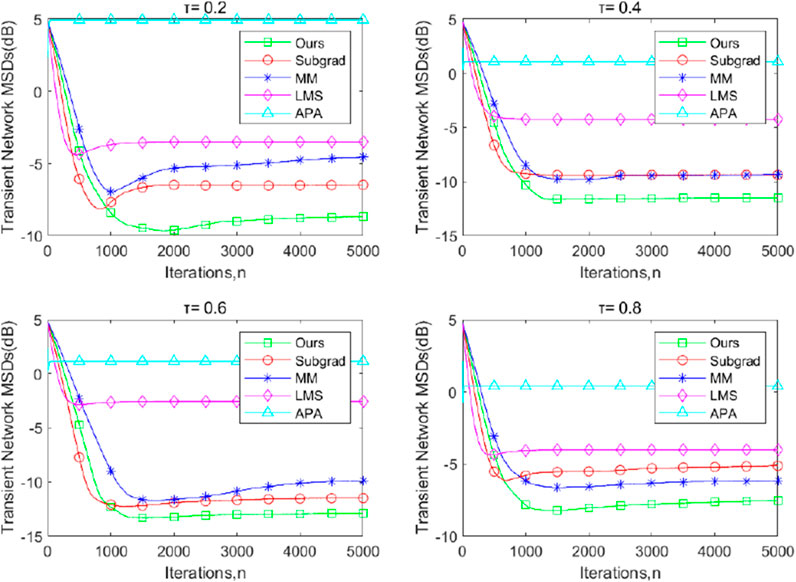
Figure 2. The transient-network MSDs of the proposed dPDHG algorithm compared with three benchmark distributed algorithms: the Subgrad, the MM, and the LMS, and the APA algorithms for estimating
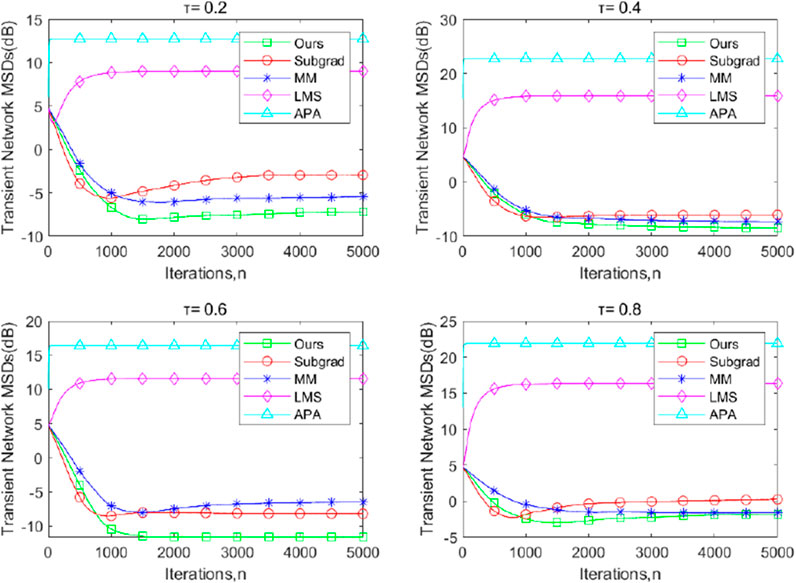
Figure 3. The transient network MSDs of the proposed dPDHG algorithm compared with three benchmark distributed algorithms: the Subgrad, the MM, and the LMS, and the APA algorithms for estimating
Across all quantile levels, our algorithm demonstrates superior convergence properties, achieving the lowest MSD values at steady state, compared to the other algorithms. The APG and LMS algorithms exhibit the highest MSD, indicating poor adaptation to the beta-distributed noise,
We further consider a practical application in spectrum estimation for a narrow-band source. A peaky spectrum can be modeled by an
where the MA coefficients
Figures 4–6 compare the true source spectrum with the estimated results averaged across
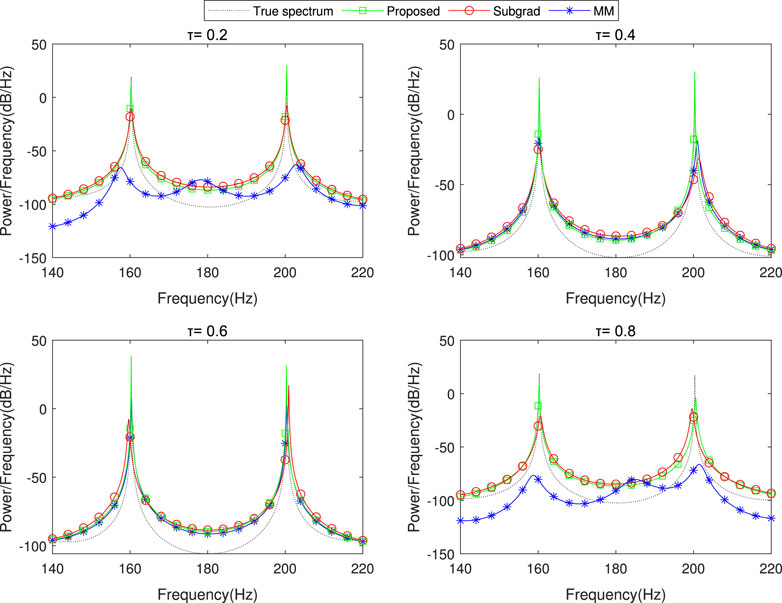
Figure 4. The spectrum estimation results of the proposed dPDHG algorithm compared with two benchmark distributed algorithms: Subgrad and MM. The comparison is conducted in the presence of beta-distributed noise
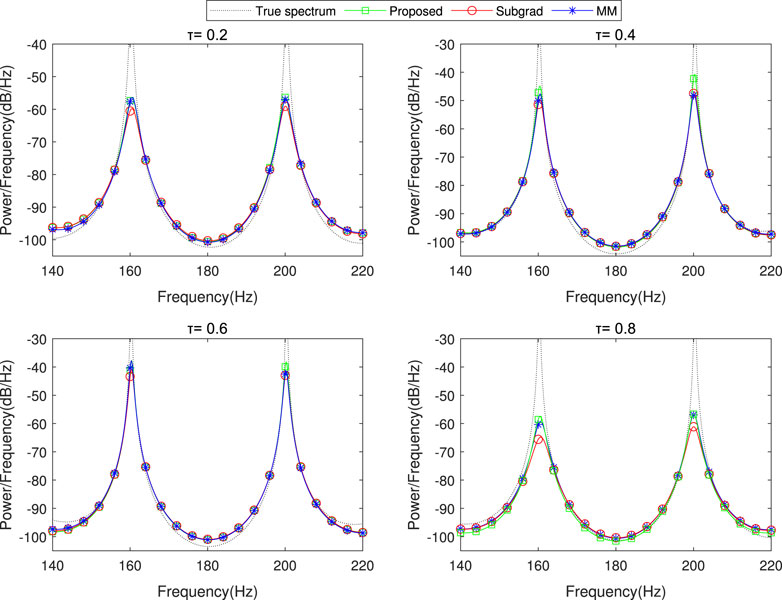
Figure 5. The spectrum estimation results of the proposed dPDHG algorithm compared with two benchmark distributed algorithms: Subgrad and MM. The comparison is conducted in the presence of t-distributed noise
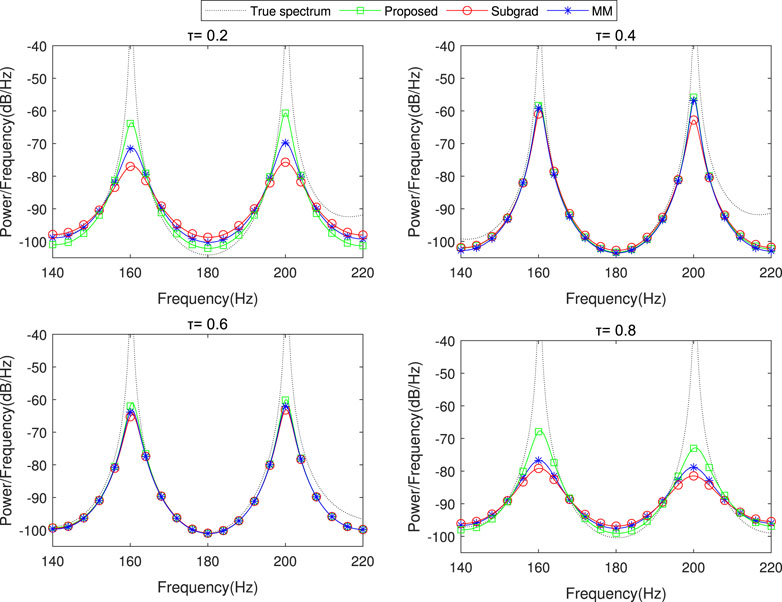
Figure 6. The spectrum estimation results of the proposed dPDHG algorithm compared with two benchmark distributed algorithms: Subgrad and MM. The comparison is conducted in the presence of Cauchy noise
As shown in these figures, our algorithm closely matches the true spectrum across all tested quantile levels, achieving higher estimation accuracy than the benchmark algorithms. These results highlight the robustness and precision of the proposed dPDHG algorithm in estimating the spectrum of narrow-band sources under challenging noise conditions.
5 Conclusion
This paper investigated distributed robust estimation in sensor networks and introduced a distributed quantile regression algorithm based on the primal–dual hybrid gradient method. The proposed algorithm effectively addresses the challenge of non-differentiability in the optimization problem by iteratively identifying the saddle point of a convex–concave objective. Additionally, it mitigates the issue of slow convergence commonly associated with such problems. The method demonstrates robustness, scalability, and suitability for processing large-scale data distributed across sensor networks.
Data availability statement
The raw data supporting the conclusions of this article will be made available by the authors, without undue reservation.
Author contributions
ZQ: Writing – review and editing. ZL: Writing – original draft.
Funding
The author(s) declare that no financial support was received for the research and/or publication of this article.
Conflict of interest
The authors declare that the research was conducted in the absence of any commercial or financial relationships that could be construed as a potential conflict of interest.
Generative AI statement
The author(s) declare that no Generative AI was used in the creation of this manuscript.
Publisher’s note
All claims expressed in this article are solely those of the authors and do not necessarily represent those of their affiliated organizations, or those of the publisher, the editors and the reviewers. Any product that may be evaluated in this article, or claim that may be made by its manufacturer, is not guaranteed or endorsed by the publisher.
References
Bazzi, A., and Chafii, M. (2023). On integrated sensing and communication waveforms with tunable papr. IEEE Trans. Wirel. Commun. 22, 7345–7360. doi:10.1109/TWC.2023.3250263
Bazzi, A., Slock, D. T. M., and Meilhac, L. (2017). “A Newton-type forward backward greedy method for multi-snapshot compressed sensing,” in 2017 51st asilomar conference on signals, systems, and computers, 1178–1182. doi:10.1109/ACSSC.2017.8335537
Ben Taieb, S., Huser, R., Hyndman, R. J., and Genton, M. G. (2016). Forecasting uncertainty in electricity smart meter data by boosting additive quantile regression. IEEE Trans. Smart Grid 7, 2448–2455. doi:10.1109/TSG.2016.2527820
Cade, B. S., and Noon, B. R. (2003). A gentle introduction to quantile regression for ecologists. Front. Ecol. Environ. 1, 412–420. doi:10.1890/1540-9295(2003)001[0412:agitqr]2.0.co;2
Cattivelli, F. S., and Sayed, A. H. (2010). Diffusion LMS strategies for distributed estimation. IEEE Trans. Signal Process. 58, 1035–1048. doi:10.1109/tsp.2009.2033729
Chambolle, A., Delplancke, C., Ehrhardt, M. J., Schönlieb, C.-B., and Tang, J. (2024). Stochastic primal–dual hybrid gradient algorithm with adaptive step sizes. J. Math. Imaging Vis. 66, 294–313. doi:10.1007/s10851-024-01174-1
Chen, A. I., and Ozdaglar, A. (2012). “A fast distributed proximal-gradient method,” in 2012 50th annual allerton Conference on communication, control, and computing (allerton) (IEEE).
Cheng, Y., and Kuk, A. Y. C. (2024). MM algorithms for statistical estimation in quantile regression
Delamou, M., Bazzi, A., Chafii, M., and Amhoud, E. M. (2023). “Deep learning-based estimation for multitarget radar detection,” in 2023 IEEE 97th vehicular technology conference (VTC2023-Spring), 1–5. doi:10.1109/VTC2023-Spring57618.2023.10200157
Esser, E., Zhang, X., and Chan, T. F. (2010). A general framework for a class of first order primal-dual algorithms for convex optimization in imaging science. SIAM J. Imaging Sci. 3, 1015–1046. doi:10.1137/09076934x
Gao, M., Niu, Y., and Sheng, L. (2022). Distributed fault-tolerant state estimation for a class of nonlinear systems over sensor networks with sensor faults and random link failures. IEEE Syst. J. 16, 6328–6337. doi:10.1109/jsyst.2022.3142183
Goldstein, T., Li, M., and Yuan, X. (2015). “Adaptive primal-dual splitting methods for statistical learning and image processing,” in Proceedings of the 29th international conference on neural information processing systems - volume 2 (Cambridge, MA, USA: MIT Press), 2089–2097.
Hovine, C., and Bertrand, A. (2024). A distributed adaptive algorithm for non-smooth spatial filtering problems in wireless sensor networks. IEEE Trans. Signal Process. 72, 4682–4697. doi:10.1109/TSP.2024.3474168
Hüttel, F. B., Peled, I., Rodrigues, F., and Pereira, F. C. (2022). Modeling censored mobility demand through censored quantile regression neural networks. IEEE Trans. Intelligent Transp. Syst. 23, 21753–21765. doi:10.1109/TITS.2022.3190194
Kai, B., Huang, M., Yao, W., and Dong, Y. (2023). Nonparametric and semiparametric quantile regression via a New MM algorithm. J. Comput. Graph. Stat. 32, 1613–1623. doi:10.1080/10618600.2023.2184374
Lee, J., Tepedelenlioglu, C., and Spanias, A. (2018). Consensus-based distributed quantile estimation in sensor networks. arXiv Prepr. arXiv:1805.00154.
Lee, J., Tepedelenlioglu, C., Spanias, A., and Muniraju, G. (2020). ngermanDistributed quantiles estimation of sensor network measurements. Int. J. Smart Secur. Technol. 7, 38–61. doi:10.4018/ijsst.2020070103
Liu, Y., Li, C., and Zhang, Z. (2012). Diffusion sparse least-mean squares over networks. IEEE Trans. Signal Process. 60, 4480–4485. doi:10.1109/tsp.2012.2198468
Mirzaeifard, R., Venkategowda, N. K. D., Gogineni, V. C., and Werner, S. (2024). Smoothing admm for sparse-penalized quantile regression with non-convex penalties. IEEE Open J. Signal Process. 5, 213–228. doi:10.1109/OJSP.2023.3344395
Njima, W., Bazzi, A., and Chafii, M. (2022). Dnn-based indoor localization under limited dataset using gans and semi-supervised learning. IEEE Access 10, 69896–69909. doi:10.1109/ACCESS.2022.3187837
Patidar, V. K., Wadhvani, R., Shukla, S., Gupta, M., and Gyanchandani, M. (2023). “Quantile regression comprehensive in machine learning: a review,” in 2023 IEEE international students’ conference on electrical, electronics and computer science (SCEECS), 1–6. doi:10.1109/SCEECS57921.2023.10063026
Schizas, I. D., Mateos, G., and Giannakis, G. B. (2009). Distributed LMS for consensus-based in-network adaptive processing. IEEE Trans. Signal Process. 57, 2365–2382. doi:10.1109/tsp.2009.2016226
Swain, R., Khilar, P. M., and Dash, T. (2018). Fault diagnosis and its prediction in wireless sensor networks using regressional learning to achieve fault tolerance. Int. J. Commun. Syst. 31. doi:10.1002/dac.3769
Tu, S.-Y., and Sayed, A. H. (2011). Mobile adaptive networks. IEEE J. Sel. Top. Signal Process. 5, 649–664. doi:10.1109/jstsp.2011.2125943
Waldmann, E. (2018). Quantile regression: a short story on how and why. Stat. Model. 18, 203–218. doi:10.1177/1471082x18759142
Wan, C., Lin, J., Wang, J., Song, Y., and Dong, Z. Y. (2017). Direct quantile regression for nonparametric probabilistic forecasting of wind power generation. IEEE Trans. Power Syst. 32, 2767–2778. doi:10.1109/TPWRS.2016.2625101
Wang, H., and Li, C. (2018). Distributed quantile regression over sensor networks. IEEE Trans. Signal Inf. Process. over Netw. 4, 338–348. doi:10.1109/TSIPN.2017.2699923
Wang, Y., and Lian, H. (2023). On linear convergence of ADMM for decentralized quantile regression. IEEE Trans. Signal Process. 71, 3945–3955. doi:10.1109/TSP.2023.3325622
Appendix
Appendix A spectrum estimation
Since
where
both vectors having a length of
Appendix Equation A.1 expresses
Keywords: primal–dual, quantile regression, sensor networks, distribution estimation, robustness
Citation: Qin Z and Liu Z (2025) Distributed quantile regression over sensor networks via the primal–dual hybrid gradient algorithm. Front. Commun. Netw. 6:1604850. doi: 10.3389/frcmn.2025.1604850
Received: 02 April 2025; Accepted: 19 May 2025;
Published: 17 June 2025.
Edited by:
Ramoni Adeogun, Aalborg University, DenmarkReviewed by:
Ahmed Aftan, Middle Technical University, IraqAhmad Bazzi, New York University Abu Dhabi, United Arab Emirates
Copyright © 2025 Qin and Liu. This is an open-access article distributed under the terms of the Creative Commons Attribution License (CC BY). The use, distribution or reproduction in other forums is permitted, provided the original author(s) and the copyright owner(s) are credited and that the original publication in this journal is cited, in accordance with accepted academic practice. No use, distribution or reproduction is permitted which does not comply with these terms.
*Correspondence: Zhaoting Liu, bGl1emhhb3RpbmdAMTYzLmNvbQ==