- 1IndividuALLytics, Inc., Ann Arbor, MI, United States
- 2Janssen, Brunswick, NJ, United States
- 3Mad*Pow, Boston, MA, United States
The technology currently available for quantifying various biometric, behavioral, emotional, cognitive, and psychological aspects of daily life has become increasingly diverse, accurate, and accessible as a result of ongoing and continuous improvements. These burgeoning technologies can and will profoundly alter the way lifestyle, health, wellness, and chronic diseases are managed in the future. For those pursuing the potential of such digital technologies in the creation of a compelling and effective connected healthcare experience, a number of new concepts have surfaced. We have taken these concepts (many of which originate in engineering) and extended them so they can be incorporated into managing health risk and health conditions via a blended digital health experience. For example, the advent of mobile technology for health has given rise to concepts, such as ecological momentary assessment and ecological momentary intervention that assess the person's (digital twin) status and delivers interventions as needed, when needed—perhaps even preemptively. For such concepts to be fully realized, the experience design of mobile health (mHealth) program(s) (aka connected care) should and now can actually guide end users through a series of self-experiments directed by data-driven feedback from a version of their digital twin. As treatment development and testing move toward the precision of individual differences inherent in every person and every treatment response (or non-response), group data and more recent big data approaches for generating new knowledge offer limited help to end users (including practitioners) for helping individuals evaluate their own digital twin–generated data and change over time under different conditions. This is the renaissance of N-of-1 or individual science. N-of-1 evaluation creates the opportunity to evaluate each individual uniquely. The rigor and logic of N-of-1 designs have been well articulated and expanded upon for over a half century. For the clinician, this revitalized form of scientific and behavioral interaction evaluation can help validate or reject the impact a given treatment has for a given patient with increased efficiency and accuracy. Further, N-of-1 can incorporate biological (genomic), behavioral, psychological, and digital health data such that users themselves can begin to evaluate the relationships of their own treatment response patterns and the contingencies that impact them. Thus, emerges the self-scientist.
What Happened to the “Quantified Self” Movement?
The vision of a “quantified self” really began with Gary Wolf and Kevin Kelly (then editors at Wired magazine) in 2007 (Wolf, 2007). Its original intention was to promote the value of self-monitoring facilitated by emerging mobile (and other) connected digital technologies (mobile apps, wearables, wireless peripherals, etc.) for data acquisition and self-reflection. The data covered a range of overt and covert behaviors (steps, mood, diet, stress, medication adherence, etc.) and biomarkers (sleep, heart rate, weight, etc.) of which the end user's ultimate goal was to gain greater self-insights and share those insights with others. The movement itself centered largely on the activity of self-measurement (aka tracking or self-monitoring) as the primary functional component of the experience. Much has changed since Wolf and Kelly originally coined this term [see (Heyen, 2020)]. The technology currently available for quantifying various biometric, behavioral, emotional, cognitive, and psychosocial factors of daily life has become increasingly diverse, accurate, and accessible. Many believe these burgeoning technologies can and will profoundly alter the way lifestyle, health, wellness, and chronic disease are managed in the future although, as Heyen (2020) notes, the quantified-self phenomenon has had minimal impact on the collective scientific knowledge to date. For those pursuing the potential of such digital technologies, several concepts have surfaced and/or resurfaced that when conceptually and practically integrated may help facilitate the as-yet-unrealized potential of connected care.
The first of these concepts is that of a digital twin. A digital twin is a digital representation of a real-world entity or system that offers information on the functional status of that system. The digital twin has its origins as an engineering paradigm for predictive problem solving of dynamic systems with early applications at NASA (Marr, 2017; Tao and Qi, 2019). The concept has been extended into many manufacturing- and process-related contexts to map out potential system failures. Gartner named the digital twin concept one of the Top 10 Strategic Technology Trends for 2017, 2018, 2019, and 2020 (Cearley et al., 2020).
(Call out box: The average consumer knows more about the operation of their car than their own bodies. Today's automobile is equipped with more than 50 sensors/minicomputers that continuously monitor the functioning (i.e., health) of the car. The driver is signaled when one of these key functions falls outside a specific set of parameters via simplified and displayed dashboards. Such information allows drivers to determine the current functional state of their vehicle and intervene to avoid more extensive and costly problems prior to catastrophe. When serious trouble arises, these integrated computers alert the dealership to diagnostic issues and even contact emergency services based on continuously collected data from the car).
Imagine if people had access to a similar set of self-generated biobehavioral information via a dashboard connected to the increasingly sophisticated and diverse set of commercially available devices, biosensors, technologies, and related data representing their own operational health and lifestyle. These digital twins could be the by-product of a networked set of biosensors, wearables, peripherals, smart pill dispensers, smart inhalers, ingestible smart pills, implantable devices (e.g., implantable cardio defibrillators), smart injectors, smartphone applications, and/or smart speakers all connected to an intelligent home ecosystem. Data emanating from these varied sources and sensors would be rendered back to the person, reflecting everything from their ongoing blood pressure to degree of hydration. The rendered data, with supporting content, would drive personalized and actionable health choices and behavior change guidance to each person uniquely throughout the day based on their own configuration and biobehavioral readings.
The technology for creating a usable digital twin largely exists today for addressing wellness, prevention, and ongoing management of focused health conditions. Figure 1 lays out a conceptual (albeit incomplete) digital health technology map as it might be applied to the range of monitoring possibilities by organ system based on commercially available digital technology. The map also attempts to display likely clinical goals, potential digital health tools, and the biometric and behavioral data gathered from them to be used for clinical purposes.
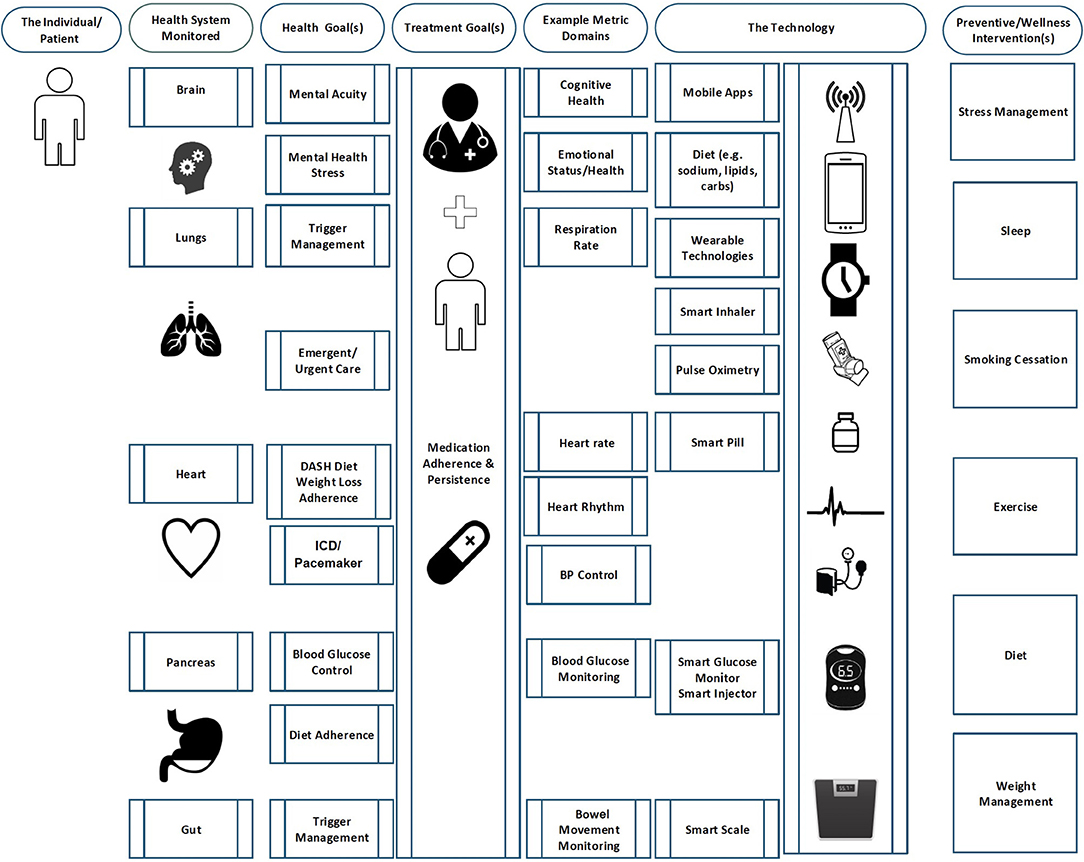
Figure 1. This map describes one set of possibilities for configuring a digital twin with existing technologies.
If the concept of a digital twin is currently conceivable with existing commercially ready digital health and therapeutics technologies, then data derived from such enhanced self-monitoring technology represents the individual's digital phenotype (Onnela and Rauch, 2016; Huckvale et al., 2019). As such, this digital phenotype is the sum of an individual's ad libitum behavior expressed through digital media (sensors, tools, devices, apps, and related software, such as machine learning or artificial intelligence, etc.) in vivo and in situ. Today, these digital phenotypes do not necessarily reflect an a priori attempt by the individual to make use of their digital information as reflected in their phenotype. However, the collected data, when organized, has the potential to typify an individual's behaviors, lifestyle, and related baseline biomarkers as they relate to targeted risks and health end points consistent with what is now being referred to as P4 (predictive, preventive, personalized, participatory; Flores et al., 2013; Sanger et al., 2016).
The link between the digital twin and the digital phenotype would likely be a set of algorithms patterned off the current scientific knowledge base. For example, findings from the ongoing Framingham Heart Study have been used to establish a 10-year coronary risk prediction algorithm (D'Agostino et al., 2008). If an individual has contributed the necessary input data of age, diabetes diagnosis, smoking status, treated and untreated systolic blood pressure, total cholesterol, HDL cholesterol, and/or body mass index (BMI) from any combination of sources, including consumer devices, clinical records, or self-report, then the system could provide real-time feedback that also ties to a risk score for cardiovascular health as well as related evidence-based insights for cardiovascular risk modification. Additional algorithms could provide similar scores for other biological functions. Collectively, the dashboard could demonstrate changing future risk based on real-time, present-time performance. This can also prompt individuals to connect other data sources if key variables are missing from certain algorithms.
One concern, which we address in more detail in the conclusion, is basing digital twin technology on data sets that capture patterns of bias. Because the technology, by nature, hones in on patterns, training the algorithm with flawed data can exacerbate and perpetuate those flaws. For example, a study examining an algorithm used by Optum to assign risk levels to patients systematically under-risked Black patients, likely due to the use of data that included racially based care disparities (Obermeyer et al., 2019). The resulting algorithm treated less care tendered to Black patients as indicative of lower need rather than less access.
Several considerations are important here. First is the integrity of the data itself as is true of all data operations. Second, any data included in the digital twin technology should be critically reviewed to identify and remediate issues that perpetuate historical bias. Third, and most importantly (and often confused with the second consideration), is the interpretive lens. Reliable and valid data do indicate biases in healthcare because they truly exist (and are now commonly and collectively referred to as the “social determinants of health”). What is deeply needed and will not be addressed by data or analytics alone is the strong interpretive lens, and that is about values (NEJM Catalyst, 2017). The history of intelligence testing and the eugenics movement is a sober reminder (Gould, 1981) of what happens when the interpretive lens is not considered.
Commercially Available Digital Health Technology
Digital health technology exists within and beyond the boundaries of the formal medical system. Although some healthcare sectors have enthusiastically pursued this technology, adoption by practitioners and patients has lagged. Recent current events prompted by the COVID-19 pandemic have altered both the public and practitioner perception of the value of remote patient monitoring by means of digital technology. There are growing impressions that digitally enabled remote patient monitoring is no longer just an interesting innovation or “nice to have” but rather a clinical delivery infrastructure imperative.
Any given individual has likely generated an extensive digital footprint already in their lifetime from multiple data sources, of which most fall into one or more of these four broad and potentially overlapping categories:
• Clinically generated data. This is the full spectrum of data generated by a person's interactions with the formal healthcare system, including electronic medical records, lab test results, pharmacy data, and health insurance claims, etc.
• Commercial real-world health data. This information is data generated by programs focused on population health management that complement traditional healthcare, including wellness and disease management, to targeted populations intended to improve risk pools.
• Consumer digital health device–generated data. The increasingly sophisticated array of commercially available connected digital technologies are now available to leverage in the care and well-being of key patient populations and generate clinically relevant data from non-clinical sources.
• Health-suggestive data. Digital data is generated by people from a variety of non-health, non-clinical data that are not explicitly tied to health but do reflect other aspects of lifestyle and secondarily can provide additional insights into health (social determinants such as zip code, local weather, buying habits, etc.).
Creating the Digital Twin
To bring the digital twin concept to life, people must have access to an integrated set of tools, content, and services all existing within a single internally consistent live and digital experience that helps both patient and practitioner make data-based health choices. These data create the person's health data repository. There must also be a mechanism for people to access the resultant insights. In one model, people could create personalized accounts via a website or downloaded app. It is also possible that entities, such as health systems or regional or national governments, might create the digital twin system for enrolling their members or citizens.
Once an account is created for an individual, including unique identity markers, the user could permission various data sources to interface with the digital twin to avoid potential data misuse or abuse. Once data sources are connected to the system, the individual would then return to the account to view insights and feedback over time. The value of the system is 4-fold: 1. data capture, 2. communication, 3. intervention delivery, and 4. outcome evaluation. As with any digital technology, there is also the opportunity to design deliberate outreach to users. For example, users might receive a cellphone alert if their digital twin data indicates an acute health issue or, on the positive side, if their data indicates behavioral changes are leading to risk reduction.
To be explicit, the typical user of digital twin technology is not required to have the health or science expertise to form conclusions about behavioral responses based on their own data. In many circumstances, with better information, people themselves are in the best position to weigh the costs vs. the benefits of a given treatment. Therefore, a core component of the digital twin must be coaching or feedback to guide users through the “so what” of their data insights. By the nature of the basis of that personalized coaching and feedback, the digital twin harnesses the strengths of tailored interventions, which are consistently seen to produce more sustained changes than static or generic health education (Noar et al., 2007; Strecher et al., 2008).
Current Healthcare and the Clinical Trial
Clinical trials have been the primary mechanism for generating clinical knowledge and depend on measures of central tendency for assessing a treatment's benefits and side effects/risks relative to some comparator (i.e., true control or standard of care). This produces reliable and valid findings centered on group averages and variation around those averages. As the reigning gold standard in clinical research, the randomized control trial (RCT) has had great success demonstrating efficacy of treatment for most common conditions, syndromes, and diseases, yielding a portfolio of effective, evidence-based treatments that most helps the most people. This group lens and the supporting deductive inference provide a forest view of clinical outcomes relative to determining the greatest overall good. However, no matter how rigorously the group data is derived, using group statistics alone can never fully address the need to treat individuals uniquely.
Small Data and N-of-1 Individual Science
Putting all the value, promise, and hype of Big Data aside, the digital twin for health has a small data requirement. It is becoming increasingly clear that RCT and group methods, although still quite valuable, are insufficient as treatments and testing move toward the precision of inherent individual differences, which are reflected in every person and every treatment response (positive or negative) (Gagne et al., 2014; Richter et al., 2015; Hilgers et al., 2016; McMenamin et al., 2018). The quantified self, with the “self” as the primary unit of analysis, was always intended for the individual to benefit from the added detail of self-observation. If self-quantification is to promote health and help manage chronic conditions, the data generated from a specifically constructed digital twin must be processed into a consumable and actionable form. Importantly, this information is embedded in every treated patient (if data were collected and analyzed properly) but remains largely latent. N-of-1 captures that value by rigorously evaluating each user, which provides the tree level of observation and evaluation.
A variety of authors from diverse disciplines have spoken about the value of N-of-1 research (Guyatt et al., 1986; Lillie et al., 2011; Barnett et al., 2012; Parker and Vannest, 2012; Dallery et al., 2013; Duan et al., 2013; Kravitz and Duan, 2014; Schork, 2015; Strathmann, 2015; Vohra et al., 2015; De Groot and Martin-Sanchez, 2017; Lobo et al., 2017; Mirza et al., 2017). The U.S. Department of Health's Agency for Healthcare Research and Quality has even published its own user guide to N-of-1 trials (AHRQ, 2014), and CONSORT has issued reporting guidelines (Vohra et al., 2015). N-of-1 evaluation creates the opportunity to evaluate each individual uniquely, which complements the existing evidence-based framework. The rigor and logic of these designs have been well articulated and expanded upon for over a half century (Mirza et al., 2017). By using more refined, time-ordered data to optimize individual-level understanding via N-of-1 analysis, more timely feedback can be provided to the patient (end user) that indicates what in their specific lives is influencing their behavior and biobehavioral health outcomes. The use of N-of-1 approaches is more true to life and clinical practice by providing individualized feedback to each patient and clinician about the quality and strength of their unique response to a given course of treatment. Further, as with more traditional approaches, N-of-1 can incorporate biological (genomic), behavioral, psychological, and digital health data such that users themselves can begin to evaluate the relationships of their own treatment response patterns and the contingencies that impact them in context. The approach can also evaluate and inform the combined treatments for comorbid condition management for which there are virtually no randomized clinical trials. For the clinician, this revitalized form of scientific and behavioral interaction evaluation methodology can help confirm or reject the impact of any given intervention for any given patient with increased efficiency and accuracy and greater insight to lifestyle: hence, the execution of precision medicine.
Because the classic clinical trial cannot fully answer all the relevant clinical questions or address the variants of an individual patient's treatment response, it must be coupled with other rigorous and valid clinical evaluation methods appropriate for the individual patient level of analysis. An N-of-1 perspective does not challenge the value of the RCT or Big Data, but rather complements it. This allows for personalization through a different lens and strategizes around time-ordered data within a single patient with multiple attributes. Further, through the use of the N-of-1 methodology, time-ordered data gets optimized by providing a new simple-to-interpret metric from the growing deluge of time-ordered data now coming from the advances in ecological momentary assessment and intervention coming from new and expanding measurement technologies (wearable devices, nanotechnology, pervasive wireless connectivity, Internet of Things, improved personal privacy and data protection technology, etc.) (Smyth and Stone, 2003; Kuntsche and Labhart, 2013; Runyan and Steinke, 2015; Spoelmann et al., 2016; Versluis et al., 2016; Dai and Bikdash, 2017).
Current standard of care for assessing treatment response to many chronic conditions takes place with limited frequency. Secondary clinical objectives (weight, diet, adherence to prescribed medicine, sleep, etc.) are almost never or only superficially addressed. Time and cost constraints do not allow for this type of care in our current healthcare delivery model. By bringing in scalable digital technology, the frequency of assessment can be increased while algorithms based on aggregate science offer evidence-based feedback. The architecture and metric strategy of any digital program that structures and optimizes time-ordered data with this technology can arrive at a user value level that is, by definition, personalized and without the classic paradox of requiring big data. One way to consider the digital twin is as a tool to pinpoint the specific individual response within the known variance of the aggregate responses, essentially locating the individual user within the distribution of the broader sample treatment responses.
Design Considerations for the Digital Twin
For the digital twin concept to produce its promised benefits, the technology must be designed to engage one or more end user groups in a meaningful way. The concept of N-of-1 must be operationalized in a way that a user can actually engage with and learn from it, including granting permission for data sources to be gathered and analyzed, viewing their personal data against the digital twin, and taking action based on insights from the comparison.
Any design of an actual N-of-1 product should take the needs of both provider and patient or caregiver user groups into consideration. Ideally, the group designing any interface will conduct original primary research with the intended users with the specific aim of informing the design. That said, prior experience with creating data-driven health interfaces for consumer use suggests several best practices that are likely to be relevant here. The design needs for a provider end user group vary somewhat from those for a patient and caregiver end user group due to the context in which they might access the system and the level of expertise they bring to interpret the data. As long as medically expert users are able to opt out of or skip instructional content that may lengthen their workflow without adding value, it is better to design the entire system to be appropriate for the patient than to take on a provider as the primary design target. Designers can then modify only with regards to the specific practical clinical care needs of the practitioner. Accordingly, the suggestions below focus on the patient and caregiver user audience.
We assume that an interface or dashboard will be created as the primary mechanism through which users interact with the N-of-1 system. This interface should accommodate the following.
Clear Data Visualization
The N-of-1 process draws from an enormous volume of data. Any user interface must offer a way of cutting through the noise to amplify the signal. Importantly, visual inspection of the data has been a critical part of the N-of-1 framework from its conception. Consider that, even if providers can discern meaningful trends in large volumes of data, they are unlikely to have the time to do so. Therefore, N-of-1–enabled P4 medicine requires a data-processing step to optimize accuracy and efficiency without the practitioner losing autonomy to set a treatment plan with the patient. Patients and caregivers may have the time but likely lack the expertise and require a processing step that helps make the data more understandable and actionable (Fisher et al., 2003; Parker et al., 2011a,b). A presentation layer that prioritizes meaningful information and makes trends easily visible—perhaps through use of colors, icons, or other visual elements—is a prerequisite for adoption of the digital twin.
Plain Language
For patients and caregivers, an additional level of interpretation is likely to be needed for the digital twin concept to be useful. Although many patients do become experts in their own condition over time, the average health literacy and numeracy levels in the United States are quite low (see Kirsch, 1993; U.S. Department of Health Human Services., 2010). Particularly for people engaging with the digital twin prophylactically, plain language explanations of health phenomena and clear directives about action to take will increase the odds that they take positive action based on their data.
Access
Access refers to the ability of individuals to acquire and use technology and may be limited by either financial or geographical concerns. Additionally, in some countries, such as the United States, there is often a large cost burden on the patient for health care utilization that may affect whether and what data exists for a given individual. There is a role for organizations, such as health plans, governments, or employers, to subsidize or provide devices and internet access to facilitate people's use of the digital twin, but designers can also minimize the access burden wherever possible. One example is to offer options between data collected by often costly connected devices and web-based self-report; this will also make the digital twin more palatable to people who are not early adopters of technology and may not yet use connected devices. Another example is to design interfaces to require minimal data downloads so as to not max out limited cell phone plans or be unusable in areas with slow connectivity.
Accessibility
Accessibility best practices, such as those put forth in the Web Accessibility Initiative Worldwide Web Consortium (3Wc) (2019) should be observed in the design of the digital twin. The World Health Organization estimates that about 15% of the global population, or one billion people, have at least one disability World Health Organization (2020).
Although certainly demographic attributes can be important in personalizing an approach, we do not see pervasive demographically based needs. For example, research suggests that, despite stereotypes, older adults are increasingly likely to own and regularly use smartphones and computers (see Yoon et al., 2020). There is heterogeneity in technology usage among older adults as with all age groups with more educated and affluent people being more likely to use technology skillfully and regularly (Hargittai et al., 2019). However, as people age and experience normal physical and mental declines, they often benefit from the accommodations included in general accessibility standards, such as high color contrast between fonts and backgrounds and larger clickable areas on websites. Accessible design can, therefore, benefit multiple user groups. Importantly, it is always best practice to research the target users of any technology to understand and design for any limitations they are likely to experience.
Prioritization of Interventions
As the self-scientist identifies needs for intervention—for example, as data trends suggest an increased possibility of a health event without a change in behavior or medication—it will be important to offer a clear order of operations to follow (i.e., call to action and action steps). For health optimization situations, such as healthy self-scientists seeking to attain greater well-being, the order of steps to try may be entirely based upon their N-of-1 data and prioritize those activities most likely to produce the desired result. For more serious medical issues, the suggested steps could include the professional care team on the part of the patient and then focus on the provider as the audience for any other prioritized suggestions.
Ease of Adding and Removing Data Sources
The N-of-1 dashboard should make it simple for people to choose which self-generated data sources to include in their profile. Just as Mint (a financial budgeting program in North America) allows users to select financial accounts to connect with the service, the N-of-1 should allow a self-scientist to log into their consumer health apps, workplace wellness programs, and health risk assessments so that all relevant health data can be included. At the same time, it should be easy for any self-scientist to exclude a data source as they wish. Although theoretically optimal results come from including more data rather than less, there may be compelling reasons for a person to sever their relationship with a particular data source (e.g., privacy breaches or known errors in the data).
Ability to Add Context
A potential frustration with the N-of-1 approach is that some data may be better interpreted by both humans and algorithms with context. For example, a prolonged trend of low physical activity and weight gain would be interpreted differently if it happened during a stressful work period, a high-risk pregnancy, or without any precipitating life factors. Allowing users to specify contextual events that may have influenced their data would be helpful. In some cases, the context might have been provided by data that is not available for some reason. In the example above, a medical record would have revealed a pregnancy co-occurring with the activity cluster, but it may be that the electronic medical record (EMR) does not integrate with the N-of-1 system yet despite being technically possible and necessary for system-level optimization.
Integration Into Clinical Workflow
The more accessible the N-of-1 interface during the normal clinical workflow, the greater the chances of its wide adoption by providers. Within a clinical setting, providers' technology usage might be limited to an EMR on an intranet. The technological challenge in that case is facilitating access to the digital twin dashboard within the clinic, whether through EMR integration or offering new access to other systems. It is also worth pursuing policy-level inclusion of the N-of-1 approach in value-based reimbursement models. Providers are unlikely to dedicate significant time to a tool that does not contribute to their success metrics.
Logical Longitudinal Use of Phase Shifts
Because the individual and their time-series data are paramount in this framework, the experience is best designed around treatment phases (Pertschuk et al., 1978; Hayes et al., 1999; Dallery et al., 2013; Pham et al., 2016; Michie et al., 2017) and changes in those phases based on a new (novel) treatment circumstance. There exist a number of designs, such as ABAB reversal designs, that when strung together over the course of treatment can enhance the causal logic for associating a change in a monitored outcome with a specific treatment phase. Hayes et al. (1999) have described the clinical value of such approaches in detail.
Persona Use Cases
To bring clinical and consumer design realism to the discussion, the use of personas represents a common and useful methodology when designing a user-centered experience or program. By way of example, we wish to consider two personas (father and son) and their digital twins for what a risk modification health experience might look like today. Each has a connected care configuration based on their health profiles and life circumstances. In addition, we speculate on each persona with regards to what their health experience might look like in 5 years.
Raymond (father) and Josh (son): Current Connected Care Experiences
Call Out Box. Raymond (age 59).
□ Firefighter Retired Secondary to Type II Diabetes Complications
□ Type II Diabetes Treatment Metformin
□ BMI 29.4
□ Hypertension Treatment ACE inhibitor
□ Spotty Medication Adherence
□ Moderately Active
□ Health Risk Assessment (HRA) Predicted Health
□ Technology Suboptimizer1.
Background
Raymond lives with his wife Jeanne of 38 years. The couple have resided in the same suburban neighborhood their entire marriage. They raised their son Joshua (age 35) and daughter Casandra (age 24) in that home. Raymond retired 3 years ago following complications secondary to poorly controlled diabetes including retinopathy. Since that time, he has occupied himself with his garden, his grandkids, and general work around the home.
Health Status
Diagnosed with hypertension 10 years ago and type II diabetes 7 years ago, Raymond has struggled to keep his HbA1c and blood pressure levels in the range his doctor has recommended. Unfortunately, he has also struggled keeping both under control due to poor medication adherence and an unhealthy diet. As a result, Raymond has never been able to sustain a healthy blood pressure or HbA1c. Consultation with his internist indicated he may soon need to switch to injectable medications to better manage his blood glucose.
Psychographics
Raymond has always prided himself on his independence and being “a man's man.” He finds it hard to ask for help and is much more comfortable caring for others than being cared for. He admits to some conflict with his wife over his health, diet, and activity level. He never liked the idea of being dependent on medication and would prefer to handle his health issues with diet and exercise. But he admits to dietary weaknesses, including a strong sweet tooth. His love of gardening and chasing around grandchildren are his primary sources of exercise, but he admits to very little physical activity in the last 4 months, which, in turn, raised his weight another 10 pounds. His history of habitual exercise as a firefighter and desire to retain his independence are potential strengths.
Technographics
Raymond can best be described as a technology “suboptimizer” (i.e., someone who uses current technology but not to its fullest potential). He uses a smartphone, laptop, and tablet, but does not get the most out of all the technology could provide. He uses apps on his phone for a limited number of practical activities, such as banking, scheduling, and checking the weather. He never uses the health apps that came preinstalled on the phone. He does use several online social sites.
Raymond's Connected Care Configuration
Raymond and his physician have agreed to try several technological tools to help him take his medication as directed as the primary and most immediate goal. Raymond's doctor pointed out that Raymond needs to monitor his health, including his blood pressure and blood sugar, more regularly just as he once needed to monitor his firefighting equipment for proper functioning. Based on a match between Raymond's needs and the available technology, they agree to the following tool set.
• A wearable device that can help monitor basic biobehavioral functions, such as sleep, activity (steps), and heart rate.
• A smart bottle that can message Raymond and select others when medication is to be taken and/or when a dose is missed.
• A smart scale that can assess weight as well as other secondary metrics, such as level of hydration, percent body fat, and body mass index.
• A mobile app that helps connect his support system, which includes his wife, children, and sister.
• Home smart glucometer that tracks blood glucose, aids in decision making, connects with the other technology (wearable and smart scale), and connects to support and practitioners.
Figure 2 displays Raymond's treatment plan laid out in a series of three consecutive treatment phases. Each phase represents a new unique set of treatment conditions. The A phase of Raymond's experience represents a baseline period of management as usual. In Raymond's case this includes a combination of historical data (past blood glucose values and other lab values, rough estimates of medication adherence, etc.) with 2 weeks of biobehavioral baseline (i.e., run in) data (blood sugars, medication adherence, activity level), average daily steps. The B phase represents the starting intervention that is focused on supporting medication adherence for both hypertension and type II diabetes by way of increased monitoring and intervention via smart bottle–driven reminders and increased coordination of family support via a support network app. There is also a secondary goal of increasing his average daily steps by 10% (from 5,800 to 6,380). The choices made for phase C are dependent on the phase B response data. They are presented in these personas as a “happy path” in which phase C builds on positive gains made in phase B. Importantly, the methodology would also allow for early detection of a non- or negative response to inform clinical decision making. Therefore, in our examples, the phase C has defined new goals for continued improvement in medication adherence and more programmatic increases in physical activity and diet (which now incorporate his wife Jeanne as the primary grocery shopper and cook). Each of these interventions is also tied to biometrics of blood pressure and blood sugar, the data of which is regularly collected and shared among patient, practitioner, and primary support (wife).
Call Out Box. Josh (age 35).
Employed Community College Math Instructor
BMI 27.6
Prehypertension Considering Start of ACE Inhibitor
Moderately Active
HRA Health Prediction
Technology Optimizer2.
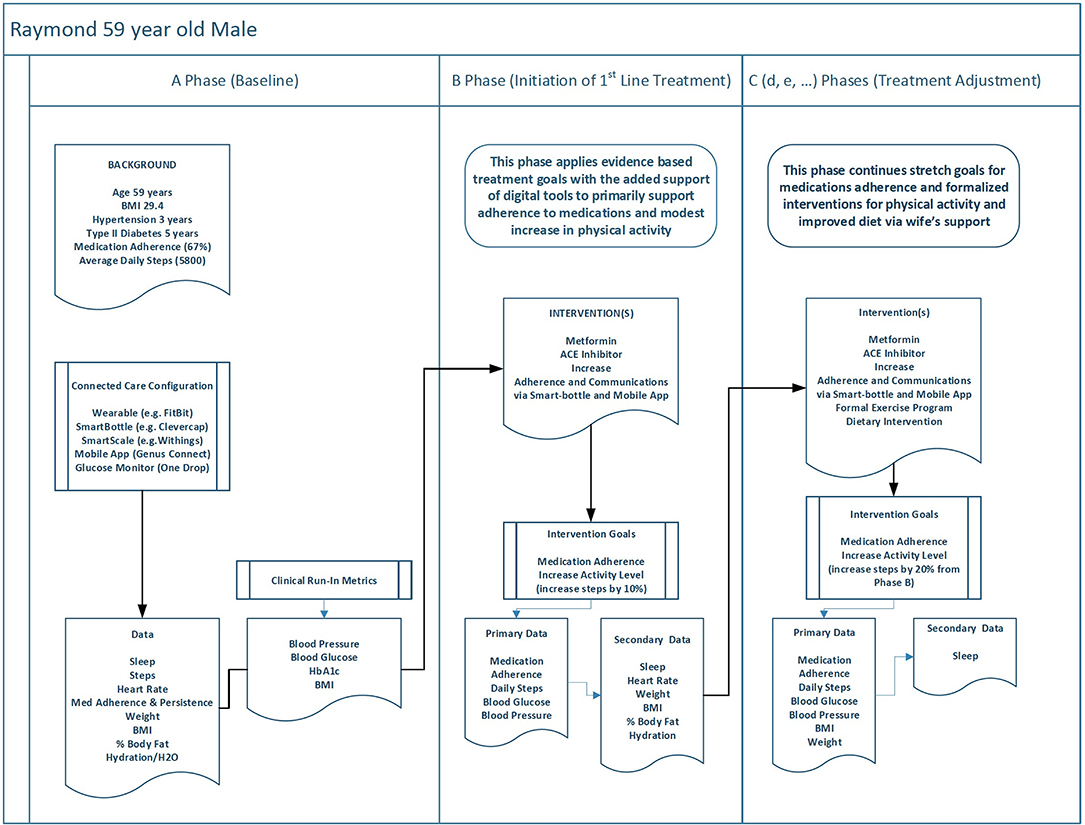
Figure 2. This figure describes how Raymond's personal health data might be used with a digital twin across three phases of behavior change to achieve increasingly tighter outcome goals.
Background
Josh is Raymond's son and oldest child. He lives with his second wife, Adrian, of 3 years, and he has no children. The couple live in a condo not far from where Josh and Adrian work. Josh is employed as a math instructor at a community college, and Adrian works as a real estate broker. The couple maintain an active personal and professional life and have recently considered starting a family. They are particularly close with Raymond and Jeanne who live nearby. Josh decides to partner with his father on health to support him and spend more quality time together.
Health Status
Josh has always been athletic and still plays softball once a week during the season, but his regular exercise routine has become increasingly less regular and his expanding waistline reflects it. His doctor says that, unless he can get his blood pressure under control with diet, exercise, and weight loss, he will need to start medication. Having seen his father struggle with weight, high blood pressure, and diabetes, Josh is determined to avoid medication and get back to a healthier level of activity and eating. The prospect of fatherhood is an added motive.
Psychographics
Josh has always viewed himself as fit and athletic and, prior to marrying Adrian, was just that. It was a classic example of domestic comfort and contentment. The recent weight gain and increased blood pressure have him concerned but also motivated. He does not see himself as overweight, but his BMI says otherwise. His wife is very supportive of his plan to eat better and get more active and is eager to help him to develop a system that fits his busy and stressful schedule. Josh intends to begin with increased daily walking and biking at least once a week. Strong intrinsic motivation is a potential strength for Josh.
Technographics
Josh grew up with technology. He got his first X-Box at age 12 and his first cellphone when he obtained his driver's license. He uses a variety of technology, including a multitude of apps for managing personal affairs, work, and entertainment. He has recently purchased an updated fitness tracker and has used one off and on for the last several years.
The A phase of Josh's experience (see Figure 3) also has a baseline that parallels his father's. It combines data, such as blood pressure measures and historical data from his wearable, with the “run in” biobehavioral data (i.e., steps, sleep, BMI, etc.). Josh's B phase is designed to prevent and reverse his progression toward hypertension by way of lifestyle change alone. Therefore, his B phase is focused on weight loss by way of increased activity level and initial diet changes. He will increase his coordination of family support by better connecting with Adrian and Raymond as well as his mother and sister. Activity goals are to increase his average daily steps by 20% (from 8,900 to 10,680) and return to his old habit of biking a minimum of 1 but no more than 2 days per week. As with Raymond, the choices for phase C as presented here are a “happy path” in which phase C builds on positive gains made in phase B. Therefore, phase C has defined phase goals for continued improvement in physical activity and the addition of the DASH diet framework into his lifestyle (which now incorporates his wife as the primary support for dietary change which she will share). Each of these interventions is also tied to primary biometrics of blood pressure and BMI.
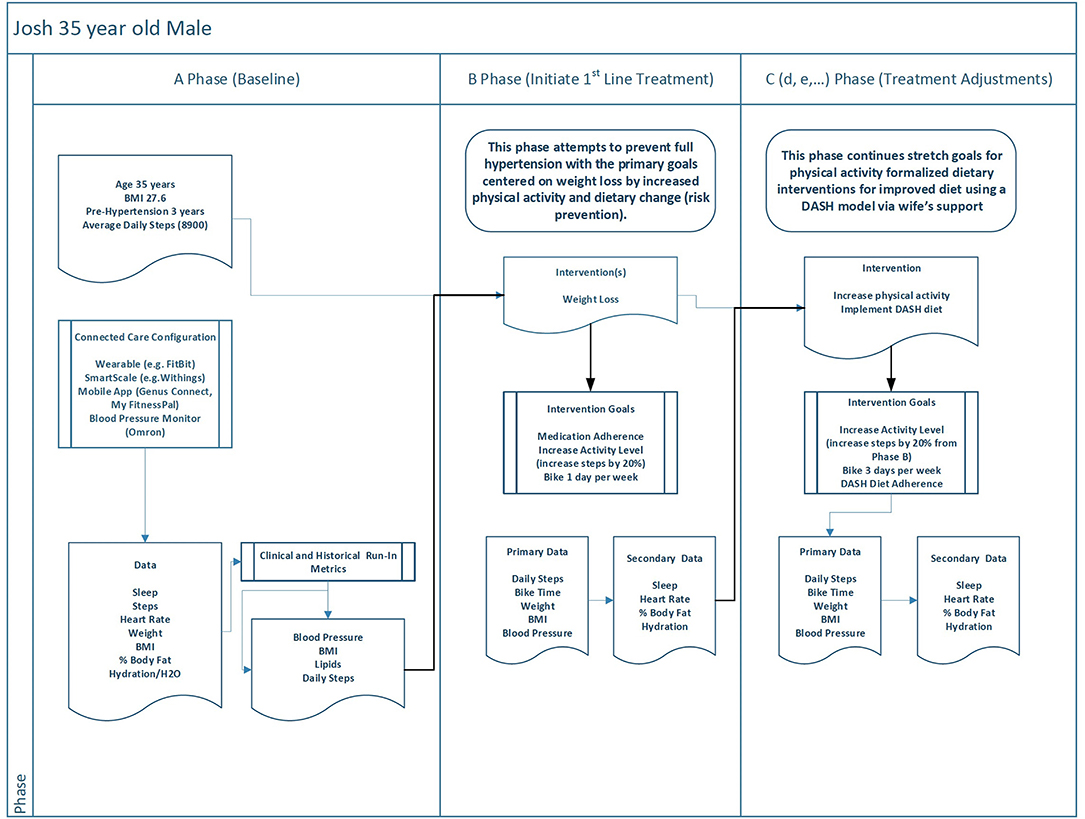
Figure 3. This figure offers a contrast to Figure 2 by showing how a similar phased digital twin process might unfold for a relatively healthier individual in order to accomplish health goals.
Raymond and Josh—Fast Forward 5 years
Figure 4 displays the health status, connected care configuration, and health goals for both Raymond and Josh as they might look 5 years into the future. Again, it is based on a digital twin model that helped both father and son achieve their baseline primary and secondary health goals and established healthier lifestyle habits (with their wives as participatory supporters).
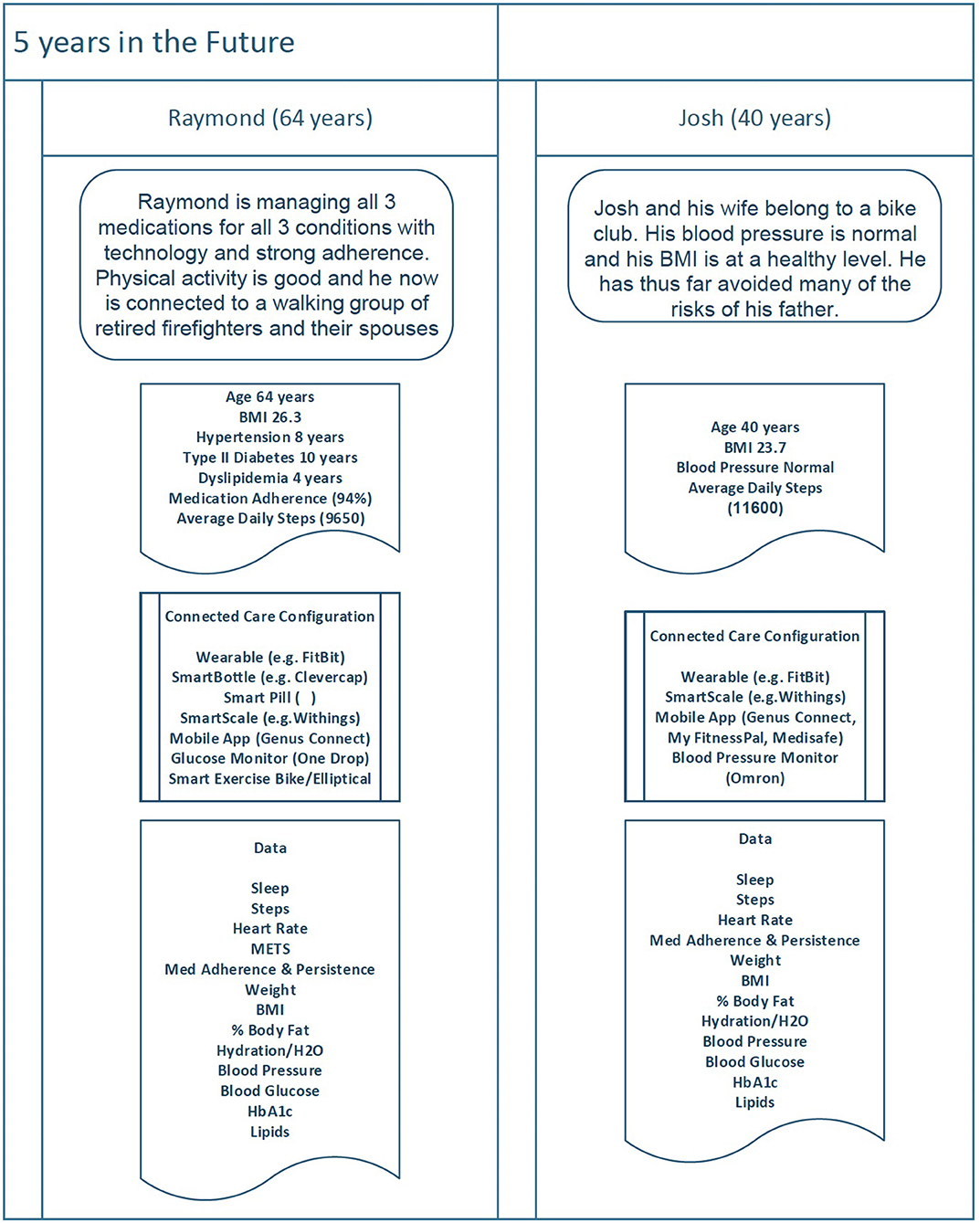
Figure 4. As technology improves and both Raymond and Josh transition to maintenance of goals, they continue to monitor their data using a variety of connected devices and make behavioral adjustments in order to achieve desired outcomes.
Raymond Health Status (Age 64)
Raymond continues to manage both hypertension and type II diabetes and has been well controlled on both for 4+ years. His retinopathy has slowed, but visual impairment has been a challenge. The basics for what he must do to manage his health are the same (i.e., stay physically active, watch what he eats, and take his medications as prescribed) but how he does it (the tools he uses) has changed over time. Now, due to visual impairment, he brings his exercise indoors and uses an exercise bike and an elliptical machine, both connected to specific live and on-demand training programs. Data collected by the machines are also now available as part of his digital twin with full details (METS, etc.) for each bout of exercise.
Josh Health Status (Age 40)
Josh also continues to manage his health. He has, thus far, avoided hypertension but developed dyslipidemia a year and a half ago. Josh now takes a statin and has been well controlled. Josh's goal is to stay physically active and watch what he eats.
Persona Summary
Connected care in this model included selected use of currently commercially available digital health and therapeutic technologies that will likely be more enhanced 5 years in the future. In both the current and future configurations, the assumption is that interconnectivity and visualization of individual patient data back to the patient, practitioner, and caregiver network can be accomplished and integrated from a technical and practical perspective. The data in these examples is integrated with more traditionally collected healthcare data (i.e., labs, utilization data, etc.) and evaluated using established N-of-1 methods and presented back with design considerations that make the personal data accurate (valid), easy to understand quickly, and with clear relevance for clinical decision making.
The Expert Patient Problem
It is now generally recognized that regardless of the health condition(s) being managed, individuals are a rich source of experiential information about the signs and symptoms of disease, common and unique responses to interventions, and successes and failures for self-managing health, all within the context of everyday living. These expert patients (Tattersall, 2002; Cordier, 2014) and their primary caregivers are the ultimate source of information for patient-centered processes and outcomes as they are shaped by each individual's experience of illness, social circumstances, attitudes to risk, values, preferences, and problem solving.
The challenge is in how to best facilitate and leverage that collective experience for the benefit of the whole health community, including practitioners. If the original intent of the quantified self movement was to gain greater self-insights and share those insights with others, then what better way to leverage them at the personal and community level than to use data and digital phenotypes? This aggregated N-of-1–level data can then facilitate and accelerate (e.g., crowdsource) the intelligence that is latent within the collective expert patient community (Levy, 2005; Eysenbach, 2008; Buecheler et al., 2010; McAfee, 2010; Li et al., 2012; Ranard et al., 2013; Crequit et al., 2018).
Accelerated Patient Insights
Digital technology today provides the mechanism to harness patients' (expert or not) latent collective wisdom with the potential to provide value and self-insights for the collective patient-consumer experience (e.g., patientslikeme, a patient-focused social network for crowdsourcing treatment options and clinical trials). Research supports the notion that large groups, when properly facilitated and applied to a topic, including health problems, will self-organize in ways that are well-suited to developing behavioral patterns that, with proper analysis can generate a greater number of novel and valid insights and alternative conclusions.
Although Von Hippel (1986) lead user concept suggests that expert patients may push the edges of the digital twin technology to discover issues and opportunities to be incorporated into the design, less expert patients also contribute to learning. Indeed, many people using digital twin technologies will, especially at the outset, have low levels of health knowledge and may not be able to extract meaningful insights without coaching or support. Fortunately, the individual group members do not necessarily need subject matter expertise for the aggregated group to display this form of emergent or collective intelligence (Roskams and Popovic, 2016; Khatib et al., 2019). The digital twin technology can capture and sort the data provided by such users to provide expert researchers with information that can jump-start their research as well as provide visibility to expert patients to help provide that coaching and support. Such connected care can realize the quantified-self goals of greater self-insights (and very likely improve health literacy as well).
The Self-Scientist
One of the historical shortcomings of the quantified-self movement was an overemphasis on tracking (i.e., self-monitoring) functionality within the technology (which still exists in many apps today) as the primary action. Practically speaking, health data and behavioral tracking generally suffered from two fundamental challenges in converting such data into insights and actions.
1. Nearly all methods for tracking were tedious and burdensome to the end user (e.g., patient or practitioner), making compliance to data collection problematic.
2. Perhaps more importantly, it has been repeatedly demonstrated that tracking in isolation does not produce sustained behavior change. Tracking must be coupled with analysis-driven intervention and feedback. This latter component is largely based on the overall experience design, functionality, and visualization.
What is known today from behavioral science research is that self-monitoring is a necessary but not sufficient condition for sustained engagement and subsequent behavior change (Karkar et al., 2016; Wicks, 2018). Insights and behavior change will not simply blossom out of self-quantification. The experience design and generated data must facilitate valid and practical insights with clear, well-timed, and motivating calls to action through the integration of an evidence-based approach.
Digital health and therapeutic technologies are increasingly solving the first problem by limiting or eliminating the end users' input requirements, thereby refining the digital twin and phenotypes. Consequently, the streaming data from these technologies can now become a rich source of time series data (aka repeated measures, trends) such that single case and small sample research designs can now be integrated into the analytical space to drive the ability of the end-user to draw valid conclusions about themselves and the contextual factors that influence their health behaviors. When the overall user experience is well designed around small case design and clinical reasoning, only then can technology-enabled experience go beyond tracking and actually enable end users to become more rigorous “naturalistic observers” of themselves…self-scientists (Parker and Vannest, 2012; Strathmann, 2015; Karkar et al., 2016; De Groot and Martin-Sanchez, 2017; Wicks, 2018).
The advent of mobile technology for health has also given rise to concepts, such as ecological momentary assessment and ecological momentary intervention. By combining and integrating data from the assessed person's (digital twin) status, technology can be used to deliver interventions as needed, when needed (even preemptively). For such concepts to be fully realized, the experience design of mobile health (mHealth) program(s) (connected care) should actually guide the end user through a series of self-experiments directed by data-driven feedback from a version of their digital twin and provide ongoing feedback about the experiments' outcomes.
Pulling It All Together for the Greater Good (Crowdsourcing N-of-1)
The implications of the digital twin for healthcare at the individual and collective levels are exciting from both an outcome and an experience perspective. A clear benefit to successful implementation of the digital twin is the more rapid identification of treatment needs to ultimately improve health outcomes and patient quality of life with ancillary benefits across the ecosystem.
The digital twin also offers the opportunity to create an empirically validated understanding of how medical treatments (e.g., medications, surgical procedures) might be enhanced or supported by behavior change interventions, such as formally or informally crafted lifestyle management programs. These programs have yielded a mixed bag of outcomes without a strong shared understanding of how they might be developed and implemented most effectively. For example, it is only in 2019 that a group of experts put forth their consensus statement on basic standards for digital mental health applications (Torous et al., 2019). The digital twin concept would enable more rapid capture of usage of such apps and related outcomes to leapfrog our current understanding of when and how to leverage them and how to identify best-in-class options.
Anticipating that digital twin technology will follow a standard diffusion of innovation curve (Rogers, 2003), we urge teams working on developing the digital twin to consider the lead user concept first advanced by Von Hippel (1986). Lead users are very early adopters of new technologies who, as the first users, are also the first people to encounter problems and unmet needs. By including lead users as part of a beta test group in which feedback is actively sought, designers will be better prepared with a useful and usable product when a less savvy segment of the population begins to set up digital twins. Research has shown that leveraging lead users as part of a product development process accelerates both volume and variety of projects completed (Ho-Dac, 2020).
A consumer-facing outcome of digital twin could be an algorithm-driven recommendation engine. The combination of individual and aggregate data at the heart of digital twin mimics the model used by companies, such as Amazon and Netflix to surface suggestions for purchases or entertainment. Such an engine could support shared decision-making tools, improve the quality of information within digital health apps, and reduce the frequency of patient panic when a search engine suggests dire but unlikely health outcomes.
Aggregation of N-of-1 replications (replications being those self-experiments that have serially been proven to work for N of x number of people) based on a set of common contextual attributes allows for a bottom-up approach to developing new knowledge (i.e., inductive reasoning) that combines and leverages the concept of the expert patient. Development of recommendation engines based on accumulating replications with ongoing evaluation of group based significance grows knowledge from direct experience accelerated by N-of-1 (Levy, 2005; Eysenbach, 2008; Buecheler et al., 2010; McAfee, 2010; Li et al., 2012; Ranard et al., 2013; Crequit et al., 2018).
Ethical and Policy Considerations
As we consider a future in which each individual can cross-reference their digital twin against their own personalized health data, it is important to note a few potential ethical issues with the intention of preempting them with patient protections. It is feasible that entities with access to patient data may use insights from the digital twin concept in ways that are against patients' best interests. For example, employers could use digital twin insights to deny offers to employees who are likely to incur high healthcare costs in the next few years3. Or insurance plans may raise premiums on people whose health data suggests an impending negative event, ironically making care more difficult to access at just the time when it is most critical. These would be misuses of the digital twin concept. We suggest three principles to safeguard against them.
Patients Own Their Data
Patients are the source of their data. When the patient is the primary entity associated with the data, it enables the digital twin concept to work. When data is scattered amongst tens or hundreds of databases under the control of organizations that are not talking to each other, the burden of compiling an individual's digital twin data set becomes enormous. Maintaining patient data ownership across the life span helps to solve for that (Dorey et al., 2018). It also creates an opportunity for new economic models that permit patients to monetize the use of their data if they choose to share it with third-party entities for research and development or other purposes. It offers patients a mechanism to protect the privacy of their data against misuse by other entities by maintaining ownership and granting or withholding access to specific data in a granular fashion.
Patients Must Provide Explicit Informed Consent for the Use of Their Data
There are many legitimate reasons why third parties may want to use patient-generated data for research or investigation. It's also clear that there may be a public health benefit to this type of data usage that we would not want to disrupt. Informing patients in plain language about the potential ways in which their data may be used—and offering them regular opportunities to review that information and change their consent—provides them the agency to participate or not, similar to the code of ethics used in human subjects research. As patterns emerge in what people are willing to consent to and not, it also may be possible to determine whether and when it's appropriate to compensate patients for use of their data.
Advocacy Efforts Should Enshrine Patient Data Ownership and Access Into Law
Research shows that although physicians and researchers are sympathetic to the notion that patients should have a say in the use of their data, they are also skeptical that patients can make appropriate choices based on the potential benefits of research to society (Dorey et al., 2018). It cannot be assumed that physicians and researchers will honor patient data ownership in practice whether because they truly believe the positive impact of their work is sufficiently large to override patient rights or because they are able to convince patients to consent when they otherwise might not through their position authority. The potential for data misuse or abuse speaks to the need for advocacy for strong patient protection laws and a role for policy advocacy from subject matter experts and organizations (e.g., HIMSS, Xcertia, etc.). The forms this advocacy might take will differ depending on the form of government and existing data protections in place in a given region as will any resultant policy (see the GDPR in the European Union as an example).
In the United States, some of the specific advocacy targets to enable the digital twin concept to work include establishing a national standard data format, which will enable interoperability and easier sharing of data across platforms. The Standard Health Record (http://standardhealthrecord.org/) and the Fast Healthcare Interoperability Resources (FHIR) are two examples of U.S.-based projects to accomplish a standard format.
There is also a call to make patient data open source, meaning it is available to be used for free. With appropriate privacy protections in place (e.g., deidentification of individual data in public data sets, securing individual informed consent for data inclusion in the data set), having clinically generated health data made open source would facilitate integration into a digital twin system.
Product Designers Must Critically Evaluate Data Sources
The success of the digital twin relies on building a data model against which any individual's data can be compared. Unfortunately, many existing data sets incorporate racial (see Obermeyer et al., 2019), gender (see Criado Perez, 2019), or other bias. Using those data sets without correction can perpetuate and intensify the bias, which would result in the digital twin making suboptimal or inappropriate recommendations for anyone not fitting the “right” demographic profile. Designers must do a deliberate and critical review of candidate data to identify potential sources of bias, such as the following:
• Historical inequities in care
• Studies that exclude women, non-white people, or members of other groups
• Biased or motivated data-collection methods
• Understand potential interpretive biases and the values that shape access to resources, technology, and information
Any identified biased data should be excluded from the digital twin, adjusted and corrected, or specified to apply only to the relevant demographic groups. Designers should also specifically question any assumptions in using one piece of data as a proxy for another (as was the case in the (Obermeyer et al., 2019), study in which prior access to care was used as a proxy for need, obscuring a history of discrimination that limited access among Black patients).
Future Work
In many ways the future is here. The technology to create the beginnings of a digital twin to support the care and management of individuals with multiple chronic conditions exists today. The next challenge is for adventurous companies and institutions with quality technologies and subject matter expertise to start testing such systems (albeit in limited form to start) in real environments to address the following problems:
1. Data integration, quality, and security across platforms and systems.
2. Iterative development of the connected care experience that includes practitioners and caregivers.
3. Iterative development of the data visualization feedback to each stakeholder.
4. Broaden the N-of-1 library of analytical approaches designed for specific clinical data circumstances.
5. Application of the continuously improving design to high value patient populations to evaluate impact on engagement, clinical outcomes, utilization of services and costs.
6. Develop a mechanism to compile N-of-1 learnings to detect aggregate trends and insights and bring them into the shared scientific knowledge.
7. Identify, within regional or national subject matter expert coalitions, the appropriate political advocacy targets and organize lobbying efforts toward making health data accessible and usable for tools such as the digital twin.
Assuming the ability to build out success for the first four short-term goals above, the more distal goals would be to experiment with the aggregation and dissemination approaches back to key audiences to better foster the value of N-of-1 insights; continuously improve the experience and design of the digital twin; and create replications for the relevant communities in ways that leverage and reinforce collective intelligence of the group.
Author Contributions
All authors listed have made a substantial, direct and intellectual contribution to the work, and approved it for publication.
Conflict of Interest
SS was employed by IndividuALLytics and Diplomat. KW was employed by Janssen. AB was employed by Mad*Pow. BB was employed by IndividuALLytics.
Footnotes
1. ^Person who uses some but not all and does not optimize the features of current digital connected technologies.
2. ^Person who fully uses all the features of current digital connected technologies.
3. ^Indeed, some employers already prohibit employees from using tobacco and require a biometric test before confirming employment offers.
References
Barnett, S. D., Heinemann, A. W., Libin, A., Houts, A. C., Gassaway, J., Sen-Gupta, S., et al. (2012). Small N designs for rehabilitation research. J. Rehabil. Res. Dev. 49, 175–186. doi: 10.1682/JRRD.2010.12.0242
Buecheler, T., Sieg, J. H., Füchslin, R. M., and Pfeifer, R. (2010). Crowdsourcing: open innovation and collective intelligence in the scientific method: a research agenda and operational framework. Proc. Alife XII Conf. Odense Denmark 2010.
Cearley, D., Burke, B., Smith, D., Jones, N., Chandrasekaran, A., and Lu, C. K. (2020). Gartner Top 10 Strategic Trends for 2020. Available online at: https://www.gartner.com/smarterwithgartner/gartner-top-10-trends-in-data-and-analytics-for-2020/
Cordier, J. F. (2014). The expert patient: towards a novel definition. Euro. Respir J. 44, 853–857. doi: 10.1183/09031936.00027414
Crequit, P., Mansouri, G., Benchoufi, M., Vivot, A., and Ravaud, P. (2018). Mapping of crowdsourcing in health: systematic review. J. Med. Internet Res. 5:e187. doi: 10.2196/preprints.9330
Criado Perez, C. (2019). Invisible Women: Exposing Data Bias in a World Designed for Men. New York, NY: Abrams.
D'Agostino, R. B. Sr., Vasan, R. S., Pencina, M. J., Wolf, P. A., Cobain, M., Massaro, J. M., et al. (2008). General cardiovascular risk profile for use in primary care: the Framingham Heart Study. Circulation 117, 743–753. doi: 10.1161/CIRCULATIONAHA.107.699579
Dai, X., and Bikdash, M. (2017). Trend analysis of fragmented time series for mHealth apps: Hypothesis testing based on adaptive spline filtering method with importance weighting. IEEE Access. 5, 27767–27776.
Dallery, J., Cassidy, R. N., and Raiff, B. R. (2013). Single-case experimental designs to evaluate novel technology-based health interventions. JMIR 15:e22. doi: 10.2196/jmir.2227
De Groot, M., and Martin-Sanchez, F. (2017). Single-subject (N-of-1) research design, data processing and personal science. Methods Med. 56, 416–418. doi: 10.3414/ME17-03-0001
Dorey, C. M., Baumann, H., and Biller-Adorno, N. (2018). Patient data and patient rights: Swiss healthcare stakeholders' ethical awareness regarding large patient data sets - a qualitative study. BMC Medical Ethics 19:20. doi: 10.1186/s12910-018-0261-x
Duan, N., Kravitz, R. L., and Schmid, C. H. (2013). Single-patient (n-of-1) trials: a pragmatic clinical decision methodology for patient-centered comparative effectiveness research. J. Clin. Epidemiol. 66, s21–s28. doi: 10.1016/j.jclinepi.2013.04.006
Eysenbach, G. (2008). Medicine 2.0: social networking, collaboration, participation, apomediation, and openness. J. Med. Internet Res. 10:e22. doi: 10.2196/jmir.1030
Fisher, W. W., Kelley, M. E., and Lomas, J. E. (2003). Visual aids and structured criteria for improving visual inspection and interpretation of single-case designs. J. Appl. Behav. Analysis 36, 387–406. doi: 10.1901/jaba.2003.36-387
Flores, M., Glusman, G., Brogaard, K., Price, P. D., and Hood, L. (2013). P4 medicine how systems medicine will transform the healthcare sector and society. Personal. Med. 10, 565–576. doi: 10.2217/pme.13.57
Gagne, J., Thompson, L., O'Keefe, K., and Kesselheim, A. S. (2014). Innovative research methods for studying treatments for rare disease: methodological review: BMJ 349:g6802. doi: 10.1136/bmj.g6802
Guyatt, E., Sackett, D., Taylor, D. W., Chong, J., Roberts, R., Pugsley, S., et al. (1986). Determining optimal therapy – randomized trials in individual patients. NEJM 314, 889–892. doi: 10.1056/NEJM198604033141406
Hargittai, E., Piper, A. M., and Morris, M. R. (2019). From internet access to internet skills: digital inequality among older adults. Univ. Access Inform. Soc. 18, 881–890. doi: 10.1007/s10209-018-0617-5
Hayes, S. C., Barlow, D. H., and Nelson-Gray, R. (1999). The Scientist Practitioner: Research and Accountability in the Age of Managed Care. Needham Heights, MA: Allyn and Bacon.
Heyen, N. B. (2020). From self-tracking to self-expertise: the production of self-related knowledge by doing personal science. Public Unders. Sci. 29, 124–183. doi: 10.1177/0963662519888757
Hilgers, R. D., Konig, F., Molenberghs, G., and Senn, S. (2016). Design and analysis of clinical trials for small rare disease populations. J. Rare Disease Res. Treatm. 1, 53–60. doi: 10.29245/2572-9411/2016/3.1054
Ho-Dac, N. N. (2020). The value of online user generated content in product development. J. Business Res. 112, 136–146. doi: 10.1016/j.jbusres.2020.02.030
Huckvale, K., Venkatesh, S., and Christensen, H. (2019). Toward clinical digital phenotyping: a timely opportunity to consider purpose, quality and safety. Digital Med. 2:88. doi: 10.1038/s41746-019-0166-1
Karkar, R., Zia, J., Vilardaga, R., Mishra, S. R., Fogarty, J., Munson, S. A., et al. (2016). A framework for self-experimentation in personalized health. J. Amer Info Assoc. 23, 440–448. doi: 10.1093/jamia/ocv150
Khatib, F., Desfosses, A., Players, F., Koepnick, B., Flatten, J., Popović, Z., et al. (2019). Building de novo cryo-electron microscopy structures collaboratively with citizen scientists. PLoS Biol. 17:e3000472. doi: 10.1371/journal.pbio.3000472
Kirsch, I. S. (1993). Adult Literacy in America: A First Look at the Results of the National Adult Literacy Survey. US Government Printing Office, Superintendent of Documents. Washington, DC 20402 (Stock No. 065-000-00588-3).
Kravitz, R. I., and Duan, N., (eds.) (2014). The DEcIDIE Methods Center N-of-1 Guidance Panel. (2014. Design and implementation of N-of-1 Trials: A User's Guide. AHRQ Pub No 13(14)-EHC122-EF. Rockville, MD: Agency for Healthcare Research and Quality. Available online at: www.effectivehealthcare.ahrq/gov/N-of-1Trials.cfm
Kuntsche, E., and Labhart, F. (2013). Using personal cell phones for ecological momentary assessment: an overview of current developments. Eur. Psychol. 18, 3–11. doi: 10.1027/1016-9040/a000127
Levy, R. (2005). Collective intelligence, a civilization: towards a method of positive interpretation. Int. J. Polit. Cult. Soc. 18, 189–198. doi: 10.1007/s10767-006-9003-z
Li, B., Lee-Urban, S., Scott Appling, D., and Riedl, M. O. (2012). Crowdsourcing narrative intelligence. Adv. Cog. Systems 1, 1–18.
Lillie, E. O., Patay, B., Diamant, J., Issell, B., Topol, E. J., Schork, N. J., et al. (2011). The n-of-1 clinical trial: the ultimate strategy for individualizing medicine? Person. Med. 8, 161–173. doi: 10.2217/pme.11.7
Lobo, M. A., Moeyaert, M., Cunha, A. B., and Babik, I. (2017). Single-case design, analysis, and quality assessment for intervention research. J. Neurol. Phys. Ther. 41:187–197. doi: 10.1097/NPT.0000000000000187
Marr, B. (2017). What is Digital Twin Technology and Why is it so Important. Forbes, Available online at: https://www.forbes.com/sites/bernardmarr/2017/03/06/what-is-digital-twin-technology-and-why-is-it-so-important/#7c3690342e2a
McAfee, A. (2010). Why Crowdsourcing Works. Available online at: http://forbes.com/2010/08/23/science-proteins-foldit-technology-breakthrough-crowdsourcing.html
McMenamin, M., Berglind, A., and Wason, J. M. S. (2018). Improving the analysis of composite endpoints in rare disease trials. Orphanet J. Rare Diseases 13:81. doi: 10.1186/s13023-018-0819-1
Michie, S., Yardley, L., West, R., Patrick, K., and Greaves, F. (2017). Developing and evaluating digital interventions to promote behavior change in health and health care: recommendations resulting from an international workshop. JMIR 19:e232. doi: 10.2196/jmir.7126
Mirza, R. D., Punja, S., Vohra, S., and Guyatt, G. (2017). The history and development of the N-of-1 trials. J. R. Soc. Med. 110, 330–340. doi: 10.1177/0141076817721131
Noar, S. M., Benac, C. N., and Harris, M. S. (2007). Does tailoring matter? Meta-analytic review of tailored print health behavior change interventions. Psychol. Bull. 133, 673–693. doi: 10.1037/0033-2909.133.4.673
Obermeyer, Z., Powers, B., Vogeli, C., and Mullainathan, S. (2019). Dissecting racial bias in an algorithm used to manage the health of populations. Science 366, 447–453. doi: 10.1126/science.aax2342
Onnela, J. P., and Rauch, S. L. (2016). Harnessing smartphone-based digital phenotyping to enhance behavioral and mental health. Neuropsychopharmacology 41, 1691–1696. doi: 10.1038/npp.2016.7
Parker, R. I., and Vannest, K. J. (2012). Bottom-up analysis of single-case research designs. J. Behav. Educ. 21, 254–265. doi: 10.1007/s10864-012-9153-1
Parker, R. I., Vannest, K. J., and Davis, J. L. (2011a). Effect side in single-case research: a review of nine nonoverlap techniques. Behav. Modif. 4, 1–20. doi: 10.1177/0145445511399147
Parker, R. I., Vannest, K. J., Davis, J. L., and Sauber, S. B. (2011b). Combining Nonoverlap and trend for single case research: Tau-U. Behav. Therapy 42, 284–299. doi: 10.1016/j.beth.2010.08.006
Pertschuk, M. J., Edwards, N., and Pomerleau, O. F. (1978). A multiple-baseline approach to behavioral intervention in anorexia nervosa. Behav. Therapy 9, 368–376. doi: 10.1016/S0005-7894(78)80080-X
Pham, Q., Wiljer, D., and Cafazzo, J. A (2016). Beyond the randomized controlled trial: a review of alternatives in mhealth clinical trial methods. JMIR 4:e107. doi: 10.2196/mhealth.5720
Ranard, B., Ha, Y., Meisel, Z., Asch, D., Hill, S., Becker, L., et al. (2013). Crowdsourcing – Harnessing the masses to advance health and medicine, a systematic review. J. General Internal Med. 29, 187–203. doi: 10.1007/s11606-013-2536-8
Richter, T., Nestler-Parr, S., Babela, R., Khan, Z. M., Tesoro, T., Molsen, E., et al. (2015). Rare disease terminology and definitions—a systematic global review: report of the ISPOR rare disease special interest group. Value Health. 18, 906–914. doi: 10.1016/j.jval.2015.05.008
Roskams, J., and Popovic, Z. (2016). Power to the people: addressing big data challenges in neuroscience by creating a new cadre of citizen neuroscientists. Neuron 92:45. doi: 10.1016/j.neuron.2016.10.045
Runyan, J. D., and Steinke, E. G. (2015). Virtues, ecological momentary assessment/intervention and smartphone technology. Front. Psychol. 6:481. doi: 10.3389/fpsyg.2015.00481
Sanger, M., McNeil, A., Puska, P., Auffray, C., Price, N. D., Hood, L., et al. (2016). The P4 health spectrum–A perspective, preventive, personalized, and participatory continuum for promoting healthspan. Progress Cardiov. Diseases 59, 506–521. doi: 10.1016/j.pcad.2016.08.002
Smyth, J. M., and Stone, A. A. (2003). Ecological momentary assessment research in behavioral medicine. J. Happiness Stud. 4, 35–52. doi: 10.1023/A:1023657221954
Spoelmann, W. A., Bonten, T. N., de Waal, M. W. M., Drenthen, T., Smeele, I. J. M., Nielen, M. M. J., et al. (2016). Effect of an evidence-based website on healthcare usage: an interrupted time-series study. BMJ Open 6:e013166. doi: 10.1136/bmjopen-2016-013166
Strathmann, F. G. (2015). N-of-1 clinical trials: removing the hay to find the needle. Clin. Chem. 61:5928. doi: 10.1373/clinchem.2015.245928
Strecher, V. J., McClure, J., Alexander, G., Chakraborty, B., Nair, V., Konkel, J., et al. (2008). Web-based smoking cessation program: results of a randomized trial. Am. J. Preventive Med. 34, 373–381. doi: 10.1016/j.amepre.2007.12.024
Tao, F., and Qi, Q. (2019). Make more digital twins: virtual models boost smart manufacturing by simulating decisions and optimization, from design to operations. Nature 573, 274–277. doi: 10.1038/d41586-019-02849-1
Tattersall, R. (2002). The expert patient: a new approach to chronic disease management for the twenty-first century. Clin. Med. 2, 227–229. doi: 10.7861/clinmedicine.2-3-227
Torous, J., Andersson, G., Bertagnoli, A., Christensen, H., Cuijpers, P., Firth, J., et al. (2019). Towards a consensus around standards for smartphone apps and digital mental health. World Psychiatry 18, 97–98. doi: 10.1002/wps.20592
U.S. Department of Health Human Services. (2010). Office of Disease Prevention and Health Promotion. National Action Plan to Improve Health Literacy. Washington, DC. Available online at: https://health.gov/communication/hlactionplan/pdf/Health_Literacy_Action_Plan.pdf
Versluis, A., Verkuil, B., Spinhoven, P., van der Ploeg, M. M., Brosschot, J. F., et al. (2016). Changing mental health and positive psychological well-being using ecological momentary interventions: a systematic review and meta-analysis. JMIR 18:e152. doi: 10.2196/jmir.5642
Vohra, S., Shamseer, L., Sampson, M., Bukutu, C., Schmid, C. H., Tate, R., et al. (2015). CONSORT extension for reporting N-of-1 trials (CENT) 2015 Statement. BMJ. 350:h1738. doi: 10.1136/bmj.h1738
Von Hippel, E. (1986). Lead users: a source of novel product concepts. Managem. Sci. 32, 791–805. doi: 10.1287/mnsc.32.7.791
Wolf, G. (2007). Quantified Self . Available online at: https://www.webcitation.org/66TEHdz4d
World Health Organization (2020). Disability Inclusion. Available online at: https://www.worldbank.org/en/topic/disability
Worldwide Web Consortium (3Wc) (2019). Web Content Accessibility Guidelines (WCAG) 2.1. Available online at: https://www.w3.org/TR/WCAG21/
Keywords: digital health, digital twin, digital phenotype, self-scientist, mHealth, small data, N-of-1
Citation: Schwartz SM, Wildenhaus K, Bucher A and Byrd B (2020) Digital Twins and the Emerging Science of Self: Implications for Digital Health Experience Design and “Small” Data. Front. Comput. Sci. 2:31. doi: 10.3389/fcomp.2020.00031
Received: 29 November 2019; Accepted: 06 July 2020;
Published: 15 October 2020.
Edited by:
Eric Hekler, University of California, San Diego, United StatesCopyright © 2020 Schwartz, Wildenhaus, Bucher and Byrd. This is an open-access article distributed under the terms of the Creative Commons Attribution License (CC BY). The use, distribution or reproduction in other forums is permitted, provided the original author(s) and the copyright owner(s) are credited and that the original publication in this journal is cited, in accordance with accepted academic practice. No use, distribution or reproduction is permitted which does not comply with these terms.
*Correspondence: Steven M. Schwartz, c3RldmVAaW5kaXZpZHVhbGx5dGljcy5jb20=