- 1Fashion Design College of Istituto Marangoni Zhejiang Sci-Tech University (International Institute of Fashion Technology), Zhejiang Sci-Tech University, Hangzhou, China
- 2Silk and Fashion Culture Research Center, Zhejiang Sci-Tech University, Hangzhou, China
- 3School of Fashion Design and Engineering, Zhejiang Sci-Tech University, Hangzhou, China
Online shopping has become common, but fitting clothes purchased online remains a challenge for both consumers and merchants. Two digital solutions are available: virtual fitting to try on clothes or using a 2D anthropometric measurement system to select the correct size. However, consumers’ experiences and perceptions of 2D measurement systems vary. To understand consumers’ willingness to adopt 2D anthropometric systems, this study reviewed the current use of these systems in China and abroad and created a model to identify the factors that influence adoption. The model was based on the Technology Acceptance Model (TAM) and the Theory of Planned Behavior (TPB), with consumer perception as the mediator. Hypotheses were proposed based on this framework. A questionnaire survey was conducted to test these factors. A total of 410 valid responses were analyzed using SPSS to confirm the reliability and validity of the measurement dimensions. Then, structural equation modeling was used to test the hypotheses, and the results showed good model fit. Finally, path analysis and mediation tests revealed that consumer perceptions mediate the relationship between perceived usefulness, perceived ease of use, and adoption attitudes. These findings highlight the importance of these factors in predicting consumer adoption of new systems. Fashion managers and designers should adjust marketing strategies and technology development based on factors like perceived usefulness, ease of use, and consumer perceptions (e.g., enjoyment, value, and risk) to encourage adoption.
1 Introduction
With the rapid spread of the Internet and mobile devices, consumers’ online shopping behavior is becoming increasingly frequent as stated by Bölen and Üstün (2020). In online shopping, many factors affect consumers’ shopping intention, such as fit, comfort, convenience, consumer reviews, shopping experience, etc. The issue of “clothing fit” is a key indicator for consumers to evaluate shopping satisfaction as stated by Sheth and Nisheeth (2019), and the most important factors affecting “clothing fit” are the accuracy of the clothing’s fit in terms of shape and size in research conducted by Liu et al. (2022).
There are two main solutions to the problem of body size accuracy in online apparel shopping. One is virtual fitting, where consumers try on clothes digitally, but this method relies on mathematical models and often fails to provide a completely accurate fit, as noted by Liu et al. (2018). The other method involves choosing a size based on personal measurements, which can be done manually, with 3D measuring systems, or with 2D anthropometric systems. Manual measurement is cheap but often inaccurate, as consumers lack the knowledge and tools for precise measurements. 3D systems, while quick and convenient, are expensive and not ideal for everyday shopping (Xia S. et al., 2022; Bhat et al., 2021). In contrast, 2D anthropometric systems are affordable, accurate, and offer reliable data, helping to reduce errors in online purchases and improve the shopping experience, as mentioned by Ertürk and Karnaşak (2022) and Ballester et al. (2016). For online merchants, accurate body measurements can lower return rates and save time on customer support, according to Lin and Wang (2011).
The 2D image-based anthropometric system is commonly used to collect human body measurement data. This data is used for clothing design, product customization, medical research, and more reported in these regions (Viviani et al., 2018; Tao and Yun, 2019). 2D anthropometric measurement is a non-contact method that uses two-dimensional images of the front and side of the human body captured by a camera. A transparent body contour map is obtained through human image denoising and contour edge detection, including width, thickness, and length. Luo (2017) found that accurate body measurements are then derived by curve fitting, regression analysis, and other methods. Key steps in processing 2D images are to use technology such as convolutional neural network models to remove the background from the 2D images and extract the human body contours, identify the key measurement locations (measurements of the hips, chest, waist, shoulders, and other related areas.) through chain code algorithms, and Canny edge detection in research conducted by Guiming et al. (2015).
The next steps involve algorithms like curve fitting and regression analysis to calculate body measurements. Using image data, the 2D anthropometric measurement system can estimate 3D body measurements, as shown by Ballester et al. (2016). For example, the Bodygram software, developed in Japan, lets users measure their body by taking a photo with their phone. The measurements are accurate and offer a convenient experience. The system has two measurement modes: In the first mode, the user takes a photo from a height of 1.8–2 m to ensure the body fits within the measurement range, helping to remove the background and accurately capture key areas. In the second mode, another person helps position the phone to ensure the user’s whole body is in view. The process then follows the same steps as the first mode, as shown in Figure 1.
The demand for anthropometric measurements has increased due to consumers’ problems with the fit of clothing purchased online. 2D anthropometric systems provide simple measurements of human body dimensions, but these systems are not currently commercially available in China as stated by Lin and Wang (2011), and are mainly used for personalization and e-commerce in the apparel industry. Current research is focused on measurement technology development, and measurement garment improvement reported in these regions (Ertürk and Karnaşak, 2022; Meunier and Yin, 2000; Li et al., 2022), consumer use of the technology is still in the early stages of development; the technology does not have enough impact, and willingness to adopt is not apparent.
This study constructs an integrated research model based on the technology acceptance model (TAM), the theory of planned behavior (TPB), and consumer perceptions measured using a questionnaire. Research hypotheses are formulated to investigate consumer willingness to adopt 2D anthropometric systems. Marketing and management recommendations are provided for fashion managers and app designers based on the results.
1.1 The need for research
The research necessity of this paper is categorized into the following three points. First, traditional body measurement methods rely on manual operation, which has problems such as low efficiency, large error, and privacy concerns. Especially in the context of the growing demand for health management and personalized consumption, non-contact, high-precision 2D body measurement systems (e.g., based on digital image processing technology) have become an important direction of technological innovation. The application scenarios of such systems cover fitness management, clothing customization, and healthcare, but their acceptance by consumers has not yet been fully studied.
Second, as a new technology, the consumer adoption of 2D anthropometric systems is complex, and the adoption process is influenced by a variety of factors. A single model may not be able to fully explain consumers’ adoption intentions, and a model that integrates TAM and TPB (TAM-TPB) can synthesize technological attributes and psychosocial factors to more comprehensively explain consumers’ acceptance mechanisms of emerging technologies. Finally, consumers’ perceptions of technology in the use of 2D measurement systems, including perceived usefulness and perceived ease of use, have an important impact on their adoption intentions.
1.2 The novelty of the study
The novelty of this study can be explored in terms of both research perspectives and applications. First, from a research perspective, researchers such as Ajzen (1991) have widely applied the Technology Acceptance Model (TAM) and the Theory of Planned Behavior (TPB) to industries such as information technology, but they have rarely been extended to the field of apparel technology. Recent research suggests that while Pfaff et al. (2021) started these models in applications such as healthcare and education, their application to apparel and related technology domains remains underexplored. This gap in the literature highlights the novelty of applying these models to the apparel industry.
In addition, traditional technology acceptance studies have typically focused on objective technology characteristics such as functionality and ease of use, while ignoring the role of subjective consumer experiences and perceptions. Although Li et al. (2022) later applied sportswear elements to two-dimensional measurement tights and transformed them into a fashionable apparel product, they only considered technical elements and failed to perceive subjective factors such as user acceptance. In contrast, this study incorporates subjective factors such as perceived value and attitude into the model, and by emphasizing the impact of individual consumer differences on technology acceptance, this study provides a broader and more nuanced understanding of the influencing factors of technology adoption, thus contributing a new research perspective to the existing body of knowledge.
This research stands out because it goes beyond theoretical studies and focuses on real user needs. By using a questionnaire, it gathers insights into consumer acceptance and application demands, helping businesses understand consumer preferences, optimize product features, and refine marketing strategies. This, in turn, promotes the technology’s market acceptance and supports the sustainable development of 2D measurement technology in the apparel industry.
By combining the TAM-TPB model with consumer perception as a mediator, this study uncovers the complex factors influencing the willingness to adopt 2D anthropometric systems. The theoretical contribution of this work lies in challenging the assumptions of traditional models, while its practical value provides businesses with a solid foundation for improving technology and user experience. Future research could explore how cultural differences impact the adoption of virtual measurement technology, especially in metaverse environments.
2 Background and theoretical framework
Current research on measurement systems is divided into three main modules. The first module is the technical aspect, which mainly explores the innovation and improvement of the system technology. Applying color-coded clothing and using it with a neural network model, Xia S. et al. (2022) found that such measurements processed the images well enough to extract measurements. This level of research focuses on the technical aspects of the measurement system, which improves the accuracy of the measurement system to a certain extent. However, its limitation is that the sample data is only applicable to specific studies and lacks some practical validation.
The second module focuses on designing and improving measurement garments to enhance the accuracy of data for users. Li et al. (2022) integrated sportswear elements into 2D measurement tights, turning them into fashionable apparel that not only addresses privacy concerns but also boosts user acceptance and usage of the measurement technology. However, the research has limitations, including a sample that may not represent all young female consumers, as most participants were college students interested in new technology. Additionally, the measurement garments were tested only with certain 2D measurement systems and need further testing on other systems to verify the accuracy and applicability of the results.
The third level examines how consumers use measurement systems. Wren (2024) conducted a study and found that the measurement guide provided by online shopping can influence consumers’ accuracy and experience. Research on the utility and validity of measurement systems from a consumer perspective is still limited and narrow. Since 2D measurement systems are experience-based technology, this paper will explore users’ attitudes and willingness to use these systems from both a technical experience and consumer perception viewpoint. The aim is to address the limitations of previous research by offering suggestions for improving future technical practices.
In summary, research on 2D measurement systems often focuses too much on technology, overlooking the diversity of consumer groups and relying on a single theoretical framework. This paper combines the technology acceptance model and the theory of planned behavior, using consumer perception as an intermediary. It explores the system from the technical, external environmental, and psychological perspectives, aiming to broaden the research scope and consider more diverse group differences.
2.1 Technology acceptance model (TAM)
Over the past decades, scholars have proposed various models to describe the architecture and influences behind technology adoption, such as the TAM and the unified theory of acceptance and use of technology (UTAUT). TAM is used as a theoretical basis for predicting body composition from 2D smartphone app images and can be used as an alternative to the BIA scale for at-home body composition assessment in research conducted by Nana et al. (2022).
The UTAUT model is difficult to test because the determinants of the theory (e.g., technology performance, expectations, social influence, and facilitating conditions) are easily influenced by the test conditions and the voluntary nature of technology use in research conducted by Scherer et al. (2019). In contrast, the TAM is designed to study users’ adoption of new technologies reported by these regions (Yuan et al., 2021; Davis et al., 1992), which can help researchers understand and explain users’ attitudes toward technology and adoption intention found by George (2004), and some scholars have also adopted the TAM to explain the impact of technology on user experience as stated by Chang and Wildt (1994).
The model explains users’ behavioral intention (BI) through three factors: perceived usefulness (PU), perceived ease of use (PEOU), and Attitude (ATT) in research conducted by Yuan et al. (2021). Perceived usefulness is “the degree to which a user believes that the use of a particular technology or system will improve his or her job performance” in research conducted by Davis (1989). Perceived ease of use is defined as “the degree to which a user believes that the use of a particular system is effortless” in research conducted by Davis et al. (1992), and adoption attitude is a positive or negative consumer evaluation of the behavior of adopting a particular technology. (Figure 2).
The study of consumers’ willingness to adopt innovative systems based on the TAM model is an important reference for judging the innovativeness of a system. It explains the factors influencing users’ willingness to adopt innovative technologies as stated by Wu and Sheng (2022). 2D anthropometric measurement is a continuously evolving technology that has begun to be applied in e-commerce. When consumers experience the measurement system, PU and PEOU become the main drivers for consumers to adopt the system. Therefore, this study uses the TAM to investigate consumers’ willingness to adopt 2D anthropometric systems.
In a study by Cheon et al. (2012), the TAM model suggests that technology that is easy to use and useful positively affects consumer attitudes and encourages adoption. In this study, we define perceived usefulness (PU) as the extent to which consumers believe the 2D measurement system improves efficiency, stability, and convenience. Perceived ease of use (PEOU) refers to how simple and easy consumers find the system to use, and adoption attitude reflects their positive view of the system. Both PU and PEOU influence consumer attitudes. Previous research on technology adoption shows that PEOU may influence PU, making it a prerequisite for adoption, rather than directly determining system adoption (Saadé and Bouchaib, 2005; He et al., 2018). If a system is convenient and easy to use, consumers will also perceive the system as useful, indirectly affecting their attitude toward the adoption of the system. Therefore, this study proposes the following hypotheses:
Hypothesis 1: PU positively affects consumer attitudes toward adopting 2D anthropometric systems.
Hypothesis 2: PEOU positively affects consumer attitudes toward adopting 2D anthropometric systems.
Hypothesis 3: PEOU positively affects consumer attitudes toward adopting 2D image anthropometric systems through PU.
Hypothesis 4: ATT toward adopting 2D anthropometric systems will positively influence BI.
2.2 Theory of planned behavior (TPB)
The TPB was developed from the theory of reasoned action (TRA). Ajzen added a new variable, perceived behavioral control, to the TRA model and removed the limitations of the TRA model to deal with behaviors that are not fully voluntarily controlled by the consumer, and Ajzen (1991) found the TPB was applied to explain and predict user behavior between different information technologies. TPB suggests three main primitive constructs that influence behavioral intentions, namely attitude (ATT), Subjective Norm (SN), and Perceived Behavioral Control (PBC) as shown in Figure 3.
In this study, SN refers to whether consumer adoption of a 2D anthropometric system, is subject to social pressure, such as whether influential individuals or elders influence the consumers’ attitudes and willingness to adopt the system. PBC refers to the degree of difficulty consumers perceive in adopting the 2D anthropometric system, i.e., consumers determine whether to adopt the system by perceiving the degree of control they have over the system, which motivates consumer BI and directly predicts adoption behavior in research conducted by Xia Y. et al. (2022). Adoption attitudes are consumers’ positive or negative evaluations of the 2D anthropometric system.
Taherdoost (2018) identified two main factors influencing consumer adoption intention: external factors, such as social influence, and internal factors, like self-efficacy. Social influence refers to the effect of external pressures, such as media, on consumer behavior, as noted by Chen and Jia-Lun (2019). Self-efficacy is the internal belief in one’s ability to perform certain tasks, such as understanding and using a 2D anthropometric system. Social influence affects social norms (SN), while self-efficacy is related to perceived behavioral control (PBC). Terry and O’Leary argued that SN is influenced by external factors, whereas PBC is connected to internal factors (Davis et al., 1992; Kiriakidis, 2017; Zhou et al., 2022).
Davis and Mathieson suggested that self-efficacy impacts perceived ease of use (PEOU), and both internal and external factors significantly affect PEOU and perceived usefulness (PU), as shown in Kim et al.’s research (Kim et al., 2007). In this context, self-efficacy is a key internal factor influencing the intention to use system services, while PBC, which is linked to self-efficacy, determines PEOU, as Shaw and Sergueeva (2019) mentioned.
When examining consumer attitudes toward adopting new technology, it’s clear that consumers not only consider the technology’s features but are also influenced by factors like social influence, social norms (SN), and self-efficacy, according to research by Lee et al. (2003). Some studies have added social influence as a factor in the Technology Acceptance Model (TAM), suggesting that if one person believes a system is useful, others are likely to agree (Venkatesh and Fred, 2000; Yang et al., 2013), aligning with the concept of SN. Other research has found that SN, as a form of social influence, shapes how consumers perceive the system’s usefulness.
Lai (2017) found that social influence is positively related to PU and concluded that SN impacts PU. However, in research conducted by Hansen et al. (2018) if consumers perceive the system to be easier to use, but believe that they do not have full control and access to it, their willingness to adopt the system may decrease, from which it can be deduced that PEOU positively influences willingness to use the services of a 2D anthropometric system for measurement and that PBC is in turn affected by PEOU to a certain degree, that is, changes in PEOU change the effect of PBC on willingness to adopt. Therefore, in this study, SN and PBC were used as antecedents of PU and PEOU in research conducted by Davis et al. (1992). As a result, the following hypotheses are proposed:
Hypothesis 5: SN has a positive effect on PU.
Hypothesis 6: PBC positively affects PEOU.
The TAM and TPB models are commonly used to study e-technology use and e-service acceptance. However, Lee (2009) found that neither model consistently predicts adoption attitudes or behaviors. The TPB considers both personal and environmental factors, addressing some limitations of the TAM, which focuses only on technology and overlooks subjective consumer behavior, as noted by Taylor and Peter (1995). The TAM mainly looks at perceived usefulness and ease of use but may miss other factors like emotions and socio-cultural background. While the TPB includes subjective norms, it is less flexible in handling cultural differences. Research has shown that combining TPB and TAM provides a stronger prediction of IT acceptance. For example, Zhou et al. (2022) created a TAM-TPB model with motivation theory to study users’ long-term willingness to use travel apps.
As a result, more and more studies have focused on integrating them to study e-technology use and e-service acceptance, and the results show that the integrated TAM-TPB model has better exploratory power to better analyze consumers’ attitudes and intentions to adopt technology than the TAM model and TPB model alone. Therefore, the TAM-TPB model will be used in this study to investigate consumer adoption intentions for 2D anthropometric systems.
2.3 Consumer perceptions
The innovative 2D anthropometric technology is intertwined with social systems and individual characteristics. Consequently, research into consumers’ willingness to adopt the system should not only consider the technology itself but also take the subjective factor of consumers into account. Therefore, we integrated TAM and TPB into the research framework to examine consumer attitudes and willingness to adopt 2D anthropometric systems.
First, Beldad et al. (2010) highlighted that research on user adoption of business and e-technology has increasingly focused on trust, perceived risk, and their drivers in online environments. Since 2D anthropometric systems are used online, perceived risk is a key factor. The TAM model was criticized for overlooking the “impersonal nature of online environments, widespread IT use, and the uncertainty of the open Internet, “as noted by Pavlou and Mendel (2006). Benson et al. (2015) found that perceived risk can affect consumer adoption attitudes and ultimately influence their behavior.
Second, the 2D anthropometric system requires consumer participation and interaction. During the experience, consumers can perceive the benefits of the technology as well as the associated risks. Therefore, the benefits and risks are important in consumer decisions on whether to adopt the technology.
Additionally, since this study focuses on consumers’ willingness to adopt 2D anthropometric systems, it is necessary to understand consumer’ motivations for abandoning manual measurement methods and 3D measurement tools in favor of 2D anthropometric systems. Mook (1987) found motivation as the brain process that excites and directs behavior. Motivation is an important factor in explaining and understanding people’s behavior and is divided into two types: utilitarian and hedonic motives. Utilitarian motivation is where the system provides consumers with information and task-orientated values such as PU and PEOU. Hedonic motivation is related to emotional characteristics, such as perceived enjoyment, perceived value, etc.
Zhou et al. (2022) found that the TAM-TPB integrated model only focuses on utilitarian motivation, and it should also consider factors like perceived enjoyment and perceived value. Zhou Ting used the TAM-TPB model with perceived enjoyment as a mediating variable to study consumer willingness to use travel apps based on adoption motivation. The researchers also added perceived risk as a mediating variable to improve the model’s validity, as noted by Wallace and Steven (2014). Therefore, this study will incorporate characteristics of the 2D anthropometric system and use consumer perceptions, such as perceived risk, value, and enjoyment, as mediating variables to improve the model’s validity and comprehensiveness.
2.3.1 Perceived value
In research conducted by Xia et al. (2019) Perceived value (PV) refers to the consumer’s evaluation of a product or service, specifically the functional, service, and relational value perceived by consumers after purchasing a good or experiencing a service. It refers to the consumer’s overall assessment of a product’s utility based on perceptions of what is received and given (Kamiyama et al., 2020). In research conducted by Yu et al. (2017) PV refers to the overall consumer experience, including the quality (usefulness) and the perceived enjoyment of the system, and the cost of using the technology (money, time, and effort) and the technological mastery (PEOU) as the perceived sacrifices, which are subsequently assessed as a whole.
Many studies have shown that perceived value (PV) positively affects consumer adoption attitudes and intentions (Gordon et al., 2018; Altin et al., 2018; Abdullah et al., 2016). Kim et al. (2007) argued that PV influences users’ willingness to adopt different technologies. As a result, some researchers have used PV as a mediator in the TAM model to study consumer adoption. For example, Xia S. et al. (2022) developed a model to examine consumers’ willingness to use mobile phones for shopping, finding that PV positively impacts adoption. In the TAM model, Davis used cost–benefit theory to explain adoption attitudes, which are influenced by the effort needed to adopt (PEOU) and the outcome quality (PU) (Meunier and Yin, 2000; Li et al., 2022). In this context, adoption attitudes depend on the trade-off between the costs and benefits of using a 2D anthropometric system. Consumers are more likely to value the system if it is easy to use and provides accurate measurements; if it’s difficult to use and less accurate, PV decreases.
In this study, consumers measured PU and PEOU when adopting the 2D anthropometric system, which affected the PV and consumers’ attitudes and willingness to adopt the 2D anthropometric system. Therefore, this study hypothesizes that perceived value mediates PU, PEOU, and consumers’ attitudes to adoption.
In addition, in research conducted by Fagan et al. (2008) perceived enjoyment plays a significant positive role in PV and PV has been suggested as the main extrinsic motivator for determining behavioral intentions to use the technology again, leading to the following hypotheses:
Hypothesis 7: Consumers’ PV mediates the relationship between PU and ATT while using the 2D anthropometric system.
Hypothesis 8: Consumers’ PV mediates the relationship between PEOU and ATT while using the 2D anthropometric system.
Hypothesis 9: PV positively affects ATT toward adopting the 2D anthropometric system.
Hypothesis 10: Perceived enjoyment has a positive effect on PV.
2.3.2 Perceived risk
Mathieson (1991) found that Perceived risk (PR) is an important factor in consumer attitudes toward adopting innovative technologies. PR in this study is defined as uncertainty about factors such as privacy and security, system responsiveness, and unstable measurement results when using a 2D anthropometric system.
Risks may occur during measurement, especially on virtual shopping platforms. They may arise from incorrect results due to consumer error or inconvenience when the system malfunctions during the measurement process (e.g., failure to respond promptly, etc.), as well as possible privacy risks in using the system as stated by Lin and Wang (2011). In research conducted by Van Slyke et al. (2006) privacy is an important consideration for consumers in the context of e-commerce, and when using a 2D anthropometric system, the filming process may raise privacy concerns, and in research conducted by Zhou et al. (2022) consumers’ privacy concerns may limit their use of the technology, further affecting their judgment of the usefulness of the system as well as their attitude and willingness to adopt the system.
Recognizing this privacy risk, in research conducted by Xia S. et al. (2022) developed a 2D anthropometric system incorporating color coding to cover the tester’s body with blocks of colors to ensure the privacy and security of the person measuring without affecting the measurement results, thus addressing the privacy issue. In research conducted by Park et al. (2014) argued that PR impacts consumer adoption attitudes. In addition, in research conducted by Altin et al. (2018) demonstrated that PR directly affects PU in terms of consumers’ willingness to adopt technology. Thus, we propose the following hypotheses:
Hypothesis 11: Consumers’ PR mediates the relationship between PU and ATT in using the 2D anthropometric system.
Hypothesis 12: PR has a negative effect on ATT toward adopting a 2D measurement system as part of the online shopping process.
In research conducted by Xie et al. (2021) argued that the risk posed by the systems should be the cost incurred by the consumer in adopting the system, which is consistent with the concept of cost in PV, which in this study refers to the cost that the consumer has to pay for using the 2D anthropometric measurement system. Thus, the PR that consumers incur when adopting the system, such as unstable measurement results, failure of the measurement system to respond promptly, and lack of assurance of privacy and security, impact consumer perceived value. From this, the following hypothesis is formulated:
Hypothesis 13: Consumers’ PR has a negative impact on PV when using a 2D anthropometric system.
2.3.3 Perceived enjoyment
Several studies have emphasized the importance of perceived enjoyment (PE) in users’ acceptance of technology. Most customers accept new technologies and methods because they provide intrinsic benefits such as entertainment and enjoyment, but in research conducted by Hasan et al. (2021) has yet to explore the relationship between perceived enjoyment and adoption attitudes, and willingness to adopt. PE has been explained as the extent to which consumers feel pleasure when using technology as stated by Venkatesh and Fred (2000). In research conducted by Kevin et al. (2021) states that consumers who use sharing economy services are attracted to fun and engaging experiences. Whereas Airbnb provides entertainment and escapism, this experiential enjoyment leads to a desire to participate in the activity. The research study and data analysis yielded that authenticity only indirectly affects repurchase intentions through perceived enjoyment.
In contrast, family benefits, directly and indirectly, affect repurchase intentions through perceived enjoyment, and the findings add strong evidence to the literature on the mediating role of perceived fun between Airbnb stimulation and repurchase intentions. In research conducted by Giao et al. (2020) investigated the mediating role of PE on customer loyalty in online shopping. The study highlighted the mediating role of PE between PU, PEOU, ATT, and BI.
The relationship between technical features and consumer adoption intention is influenced by consumers’ perceived enjoyment (PE). Zhou et al. (2022) argued that this relationship is mediated by PE, showing that it positively impacts users’ intention to continue using travel apps. Davis suggested that PE is a key driver of consumer adoption behavior, as noted by Pfaff et al. (2021), and that PE influences perceived ease of use (PEOU) in the TAM model, as stated by Venkatesh and Fred (2000). PE also indirectly affects perceived usefulness (PU) through PEOU, significantly influencing consumer intentions. Abdullah et al. (2016) and Park et al. (2014) reported that PE significantly affects PU, PEOU, attitudes, and behavioral intentions. When using a 2D anthropometric system, consumers’ PE mediates the relationship between system quality and adoption attitude. Based on this, the following hypotheses are proposed:
Hypothesis 14: PE has a positive effect on ATT.
Hypothesis 15: Consumers’ PE mediates the relationship between PU and ATT while using the 2D anthropometric system.
Hypothesis 16: PE mediates the relationship between PEOU and ATT while using the 2D anthropometric system.
Based on the TAM-TPB theoretical framework, we developed a theoretical research framework (Figure 4) to investigate consumers’ willingness to adopt 2D anthropometric technologies with consumer perception as the mediating variable.
3 Methodology
3.1 Research design
To study the environmental factors of the Airbnb experience, Kevin et al. (2021) used purposive sampling, focusing on users who had stayed in Airbnb accommodations in the past year. They then used questionnaires to examine three attributes of Airbnb, perceived enjoyment, and repurchase intention. Purposive sampling is a common non-probability method used to select specific groups of people with certain characteristics relevant to the research. According to Saunders et al. (2019), this method helps gain insights into the attitudes or behaviors of the target group, directly related to the research question. In consumer behavior research, purposive sampling allows for a more focused study of specific segments, such as early adopters or high-income groups, which may have different adoption behaviors compared to the general population (Saunders et al., 2019).
Data collected through purposive sampling offers a good balance between flexibility and sample representativeness. To ensure the data’s credibility, depth, and relevance, and to better understand users’ attitudes and willingness to use the 2D anthropometric system, purposive sampling was used to select participants for the questionnaire.
The procedure for purposive sampling in this study involved identifying the target group and selection criteria. The sample included consumers who had used the Taobao 2D anthropometric system. The survey was conducted online, providing convenience and efficiency while ensuring a broad and accurate sample. Additionally, the focus was on the younger generation (ages 18–35), as they are more likely to adopt new technologies and represent future purchasing power.
The questionnaire uses the Taobao Intelligent Energy Body Measurement System as its experimental tool. This system uses a camera to take a 2D full-body photo of a user and analyzes it through deep learning to provide accurate measurements of the upper body, lower body, and foot size, as shown in Figure 5. It helps users find the right clothing size through four steps: data collection, processing, model matching, and size recommendation. The system offers several advantages: it’s convenient since no app download is needed—just open Taobao to use it; stores can recommend clothes in the exact size, improving the shopping experience and reducing returns. Additionally, the Taobao Smart Energy System is less known than other measurement systems, so users’ opinions are not influenced by prior experiences with other systems.
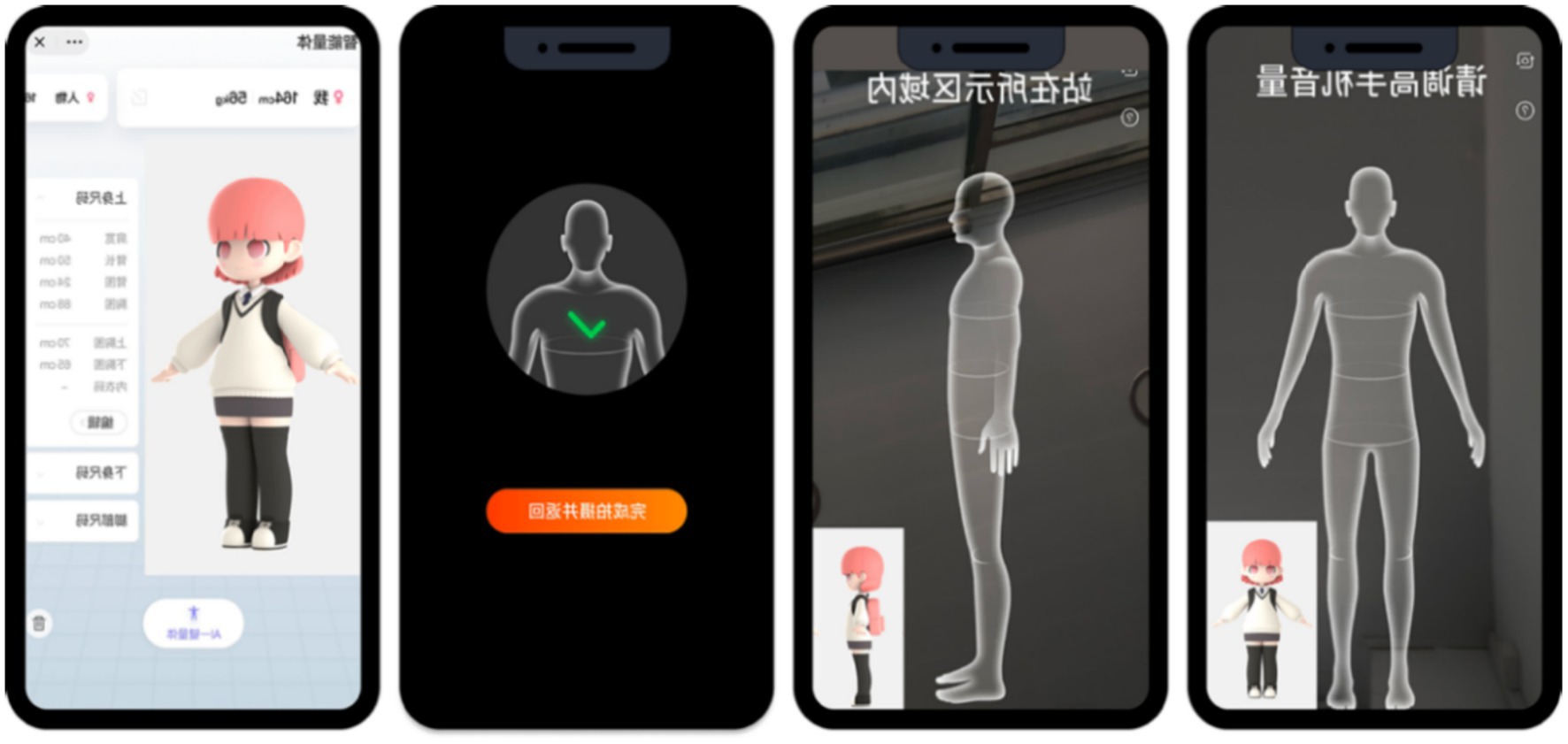
Figure 5. Schematic diagram of Taobao Intelligent Energy Body System operation (drawing from the author).
After determining the target group, selection criteria, and experimental tools, the sample composition was adjusted according to the needs of the study after the subsequent questionnaire data collection to ensure the representativeness and relevance of the sample.
3.2 Data acquisition
In this study, an online questionnaire was used to collect experimental data. The structure of the questionnaire design includes five aspects: the background of the questionnaire research, the introduction of the research content, the basic information of the target group, the core questions, and the scale criteria. In addition, before the questionnaire is formally distributed, a small pre-survey is conducted to test the validity and clarity of the questionnaire as well as the comprehensibility of the questions, and at the same time, the expression problems in the questionnaire can be adjusted according to the feedback to ensure that the questionnaire is expressed accurately and is easy to understand.
Before starting the survey, participants were asked if they had used the Taobao 2D anthropometric system. If they answered “yes, “they were shown a brief introduction to the system. After a mandatory 40-s wait to ensure they read the information, they could click “next” to continue. If they answered “no, “they were directed to a page saying the survey was closed and could not proceed. If they answered “I haven’t used it but want to try,” they were given a 10-min experience with the Taobao Smart Energy Body and could then continue the questionnaire. Question 33 was included as a reverse question to filter out invalid responses.
This step will be used for data validation and screening. Through screening and validation, questionnaires that have not experienced the measurement system as well as questionnaire options 33 and 32 with contradictory answers will be eliminated. This is to validate the data collected, check the completeness and consistency of the data, and eliminate data with obvious errors or inconsistencies to ensure the quality and validity of the data.
According to the sample size criteria developed by Devellis (1991), the sample size required for item analysis, exploratory factor analysis, and validation factor analysis is 5 to 10 times the number of entries in the compiled scale, and the official scale contains a total of 33 entries, and the sample size required for item analysis, exploratory factor analysis, and validation factor analysis is estimated to be 207–413 cases, taking into account the 20% nonresponse rate. A total of 410 valid responses were retained at the end of the paper (see Table 1), which satisfied the sample size required for both the validation factor analysis and the exploratory factor analysis, from which the next step of the analysis was conducted.
3.3 Measurement dimensions and measurement methods
In the development of the measurements, this study constructed measurement scales based on existing well-established and appropriate scales. This measurement scale was based on existing well-established scales, and a 5-point Likert scale ranging from 1 (strongly disagree) to 5 (strongly agree) was used for all questions. In addition, the scale for this study was divided into nine dimensions, namely SN, PBC as stated by Chen and Jia-Lun (2019), PU, PEOU in research conducted by Hansen et al. (2018), PR in research conducted by Altin et al. (2018), PE, PV in research conducted by Xie et al. (2021), ATT, and BI reported in these regions (Hagger et al., 2022; Zhu et al., 2022; Gardner et al., 2020), with three question items under each dimension.
3.4 Analysis of sample data
After collecting the data from the questionnaire, the first step is to analyze the basic information about the target group, which is the descriptive analysis. Table 1 shows there were more women respondents. Slightly more than half of the participants were between the ages of 24 and 29, followed by those between the ages of 18 and 23 and those between the ages of 30 and 35, respectively, both students and employees accounted for more than 30 percent of the total. More than half of the participants were undergraduates, followed by specialists and postgraduates and above. The majority of the participants indicated that they had used the Taobao 2D anthropometric system, and more than 80 percent of participants indicated that they frequently used or had used this system, and only a small percentage had not used it.
4 Results
4.1 Method bias analysis
Prior to the data reliability and validity analysis, this study will conduct a common method bias analysis in order to minimize measurement error. The most common method of detecting common method bias is the one-way test, which is easy to test and true and valid, especially when only self-reported data are available. The basic principle is that if all variables are affected by the same common method bias, a single factor may be formed centrally in the data.
In general, the risk of bias can be initially considered low if the first factor explains significantly less than 50% of the variance. According to Malhotra et al. (2006), the risk of common method bias can be considered manageable when the explained variance of the first factor in the Harman one-way test is less than 40% (Malhotra et al., 2006). The explained variance of the first factor in the unrotated factor analysis in this study was 37.308%, which is significantly lower than the conservative threshold of 40%; therefore, the data in this study has low bias and can be further tested for validity and reliability.
4.2 Measurement model evaluation
In empirical research, the level of reliability is usually used to indicate the stability, reliability and consistency of the measurements. It is generally accepted that when the Cronbach Alpha coefficient is greater than 0.8, the reliability of the questionnaire data is in a desirable state. The data were analyzed using SPSS 26.0, and the Cronbach’s alpha value was 0.934, which indicates that the consistency between the items is high, and the reliability of the questionnaire is excellent.
In empirical analysis, validity is often used to indicate the degree to which a measure correctly predicts a latent variable. Therefore, the level of validity can be used to indicate how well the results reflect the content of the latent variable. In order to ensure the authenticity and validity of validity, a combination of exploratory factor analysis (EFA) and validation factor analysis (CFA) is generally used for validity analysis. For the structural validity of the questionnaire, the Kaiser-Meyer-Olikin (KMO) measure with Bartlett’s test of sphericity was taken, and the KMO value was 0.917, a degree of freedom of 351, the significance less than 0.01. Therefore, the null hypothesis was rejected, and the sample was found suitable for factor analysis.
For principal component analysis, factors based on eigenvalues greater than 1 were extracted, resulting in nine male factors cumulatively explaining 75.23% of the variables. Through the orthogonal rotation method with Kaiser standardization, which converged after six iterations, each measurement dimension in the rotated component matrix has a high loading on its corresponding factor, which is able to distinguish the measurement of each dimension well. A scale with good structural validity can accurately reflect the traits of the object being measured, which can be evaluated by convergent validity and discriminant validity. Giao et al. (2020) and Fornell and Larcker (1981) noted that bootstrap critical ratios for each indicator were statistically significant at p < 0 0.001 and that convergent validity was indicated by an average variance extracted (AVE) greater than 0.50 for all constructs, with constituent reliabilities (CRs) exceeding 0.6.
In addition, Li et al. (2018) showed in his study that if most of the factors met the criteria, slightly lower individual factors were acceptable. Table 2 shows the factor loadings range from 0.708 to 0.830, reaching the level of significance, and the constituent reliability (CR) ranges from 0.6628 to 0.8348, indicating that the construct’s convergent validity is the average variance extracted (AVE) were >0.5, and the AVE value is greater than the average shared squared variance, indicating discriminant validity is achieved. See Table 3.
4.3 Structural equation modeling analysis
Structural equation modeling is a method used to analyze the relationship between variables. The data in this paper have been tested for reliability and validity and show good reliability and validity. Therefore, based on the research hypotheses, this paper constructed a structural equation modeling diagram using AMOS 26 to validate the relationship between the variables.
Consequently, to investigate the influences among interacting variables, we performed path analyses through structural equation modeling (SEM). The overall fit indices showed a satisfactory fit to the data: The chi-square divided by the degrees of freedom Ratio (χ2/df) = 2.195 < 3; the root mean square error of approximation (RMSEA) = 0.054 < 0.08, the comparative fit Index (CFI) = 0.933 > 0.9, goodness-of-fit index (GFI) = 0.897, incremental fit index (IFI) = 0.934 > 0.9, parsimonious normative fit index (PNFI) = 0.774 > 0.5, parsimonious adjustment comparative fit index (PCFI) = 0.816 > 0.5. All the indicators reached the fit criterion, except for the GFI, which is close to the standard. This indicates that the research model fits the data.
4.4 Hypothesis testing
Regression analysis is a common statistical method used to explore the relationship between one or more independent variables (independent variables) and dependent variables (dependent variables), and is also able to quantify the extent to which each of the independent variables affects the dependent variable, helping the researcher to understand the specific role of different factors on the target variable.
The SEM (Figure 6) and path analysis (Table 4) show there is a significant positive effect of SN on PU (β = 0.417, p < 0.001) and a significant positive effect of PBC on PEOU (β = 0.605, p < 0.001), supporting H5 and H6. There is a significant positive effect of PEOU on PU (β = 0.351, p < 0.001), supporting H3. PU had a significant positive effect on PR (β = 0.528, p < 0.001), PE (β = 0.381, p < 0.001), and PV (β = 0.282, p < 0.001), supporting H11, H15, and H7, but there is no significant positive effect of PU on ATT (β = 0.079, p = 0.301 > 0.05), and so hypothesis H1 is not supported. PEOU has a significant positive effect on PE, PV, and ATT, (β = 0.335, p < 0.001; β = 0.287, p < 0.001; β = 0.242, p < 0.001), supporting H16, H8, and H2. There was a significant positive effect of PE on PV (β = 0.255, p < 0.001), and thus, hypothesis H10 was supported.
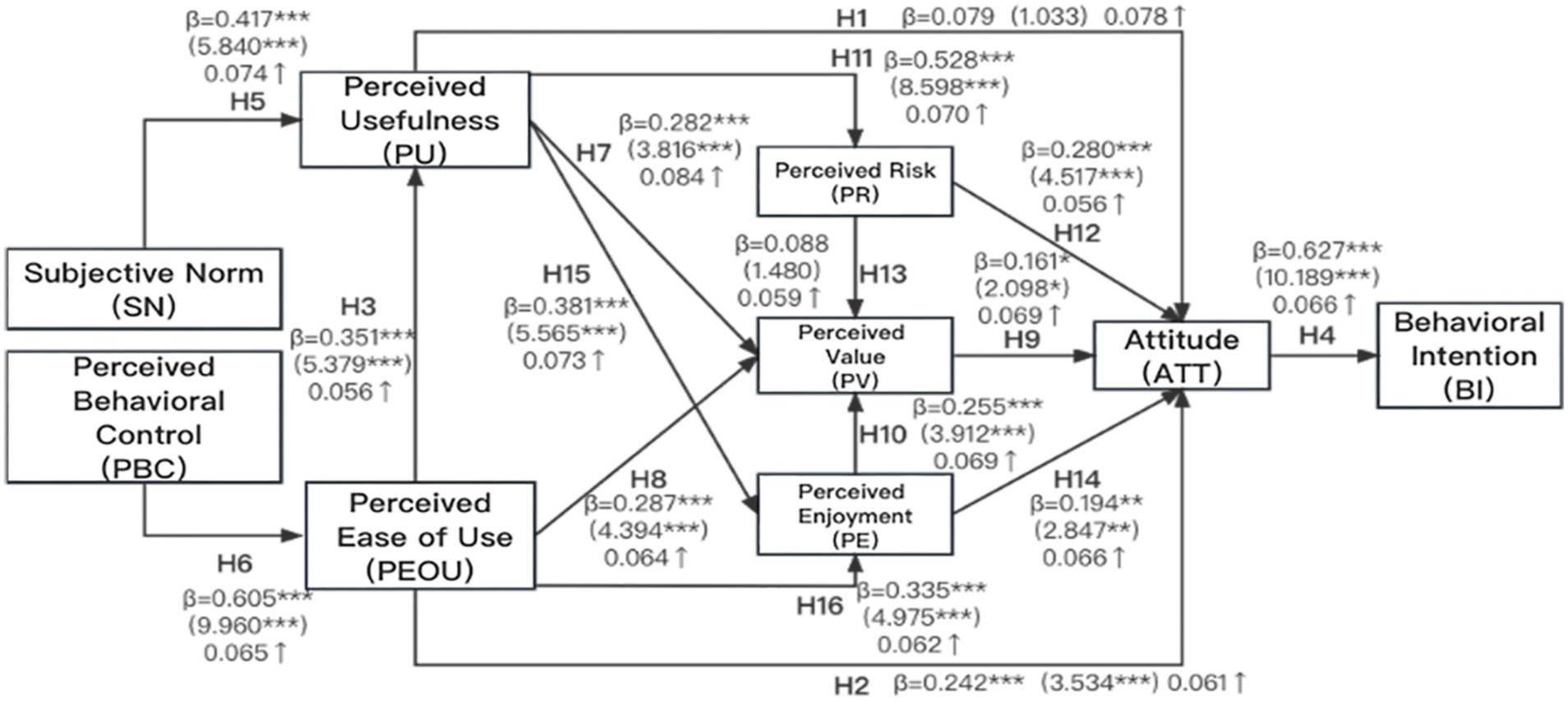
Figure 6. Structural equation model (drawing from the author). (1) t-values in parentheses (combined reliability); (2) *p < 0.05, **p < 0.01, ***p < 0.001; (3) ↑: path estimate; (4) β: standardized path coefficient.
However, the PR did not significantly contribute to the PV (β = 0.088, p > 0.0.5), and β > 0, so H13 was not supported. There was a positive effect of PV, PR, and PE on ATT (β = 0.161, p < 0.05; β = 0.280, p < 0.001; β = 0.194, p < 0.01), supporting H9 and H14. H12 was hypothesized to be a negative effect, but from the data results β > 0 indicated that PR has a positive effect on PV, therefore H12 was rejected. There is a significant positive effect of ATT on BI (β = 0.627, p < 0.001), supporting H4.
The reasons why H1 did not hold in this study are categorized as follows. First, when consumers have a high level of trust in a brand, the effect of perceived risk on perceived value may be greatly diminished. Second, if the product or service itself has significant perceived utility (e.g., in a highly technical 2D measurement system, where consumers place high value on its performance and efficiency), even if there is a certain amount of perceived risk, consumers are likely to ignore this risk and focus on the actual functionality and value of use of the product. In addition, individual differences in consumers (e.g., risk propensity, level of experience, dependence on purchasing decisions, etc.) may lead to differences in the impact of perceived risk on perceived value (Chaudhuri and Holbrook, 2001; Zeithaml, 1988; Mitchell and Greatorex, 1993).
At the level of the relationship between perceived usefulness and the influence of adoption attitudes, the reason for the hypothesis not being valid is that perceived usefulness may have strong interactions with other variables. For example, consumers may not only rely on their perceived usefulness when considering whether to adopt a particular technology, but also consider whether it is easy to get started, whether it is in line with societal trends, and so on. Thus, the role of a single factor on adoption attitudes may be diluted by the effects of other variables. Also, in emerging technologies, consumers may not fully understand the utility, or their interest and attitudes may be influenced more by the novelty of the technology and the marketing hype than by the actual perceived usefulness. Thus, perceived usefulness may play a weaker role in adoption attitudes in such cases (Venkatesh and Fred, 2000; Venkatesh et al., 2003).
4.5 Mediating effects
To investigate relationships between the variables, we conducted regression analysis through SEM. Table 5 shows the standardized estimates of the indirect paths at the 95% confidence interval for the mediating effects, which are significant if the 95% confidence interval does not contain zero. The results show that the 95% confidence intervals for all five indirect paths do not contain 0, indicating a significant mediation effect for all five paths. PU indirectly affects ATT through PR, PV, and PE, and PEOU indirectly affects ATT through PV and PE.
5 Discussion
5.1 Theoretical contribution
This study explored consumers’ willingness to adopt a 2D anthropometric measurement system using the TPB-TAM model and identified key factors influencing adoption. The study makes three main theoretical contributions. First, it examines factors that affect consumer adoption of the 2D anthropometric system and how these factors influence future behavior. The rise of online shopping in China has changed purchasing habits, making it easier for consumers to buy products online. One common challenge is selecting the correct clothing size, which 2D anthropometric systems can address more efficiently than 3D tools or manual methods. Despite their potential, there has been limited research on consumers’ willingness to adopt these systems.
Second, the study shows that consumer perceptions, such as perceived enjoyment (PE) and perceived value (PV), significantly affect attitudes and behavioral intentions (ATT and BI). This suggests that consumer perceptions act as a mediator in the adoption process, influenced by both internal and external factors (e.g., subjective norms [SN] and perceived behavioral control [PBC]). This provides a theoretical foundation for understanding the role of technology features and consumer perceptions in adoption.
Third, the study extends the TAM model by incorporating consumer perceptions as a mediator, making it more relevant for measuring willingness to use new technology. The results show that SN and PBC significantly impact technology characteristics (perceived usefulness [PU] and perceived ease of use [PEOU]), with consumer perceptions playing a key role in shaping adoption attitudes. The findings support the theoretical frameworks of TPB and TAM, while extending them to the emerging context of 2D image-based anthropometric systems, confirming the impact of consumer perceptions on ATT and BI.
5.2 Practical implications
This study offers several insights into how consumer adoption of 2D anthropometric systems can be improved. First, the findings suggest that influencing consumer perceptions and adoption intentions can help fashion managers, online platforms, and tech developers understand how clothing sizes affect consumers. This, in turn, impacts their willingness to adopt 2D anthropometric systems.
The study shows that subjective norms (SN) and perceived behavioral control (PBC) affect perceived usefulness (PU) and perceived ease of use (PEOU). Since SN shapes how users view the system, developers should create a personalized experience. For example, allowing users to customize the system to fit their body types or shopping habits can make the system feel more relevant, increasing its perceived usefulness. The study also highlights the importance of PBC, which relates to how easily users can use the system. Designers should simplify the system’s interface to make it easier to navigate. Clear instructions, intuitive design, and user-friendly features, like guides or tooltips, can reduce complexity and boost users’ confidence in using the system. Including features that give users control over their experience, such as real-time feedback or the ability to adjust measurements, can further increase the system’s ease of use. Providing easy access to support and help resources will also make users feel more secure.
On the other hand, fashion managers can partner with celebrities to promote the system and use terms like “convenient,” “fun,” “efficient,” and “easy” in their marketing to attract more shoppers to use the system. The study also suggests that PU and PEOU strongly influence consumer attitudes and intentions. Based on this, fashion managers and app designers should focus on making the system efficient, reliable, and convenient in their marketing efforts.
To make sure the system appeals to users, fashion managers should prioritize a simple, user-friendly design and highlight practical benefits, like personalized shopping experiences and time-saving features, in their marketing. Since perceived ease of use directly impacts consumer attitudes, developers should reduce the system’s complexity by providing clear instructions and tutorials, making it more intuitive and easier to adopt. Lastly, the study recommends that fashion managers and developers focus on creating an enjoyable and secure user experience. This can include adding fun elements like gamification or personalized styling suggestions, while also ensuring data protection to reduce perceived risk. By addressing these factors, fashion managers and developers can improve user satisfaction, build trust, and ultimately increase the adoption of 2D anthropometric systems among online shoppers.
Additionally, the results of the study show that the 2D body measurement system demonstrates its superiority and convenience for consumer online shopping by combining unique recognition through the Canny algorithm, efficient extraction using the convolutional neural network model, and accurate data measurements by curve fitting. Therefore, technology developers should utilize more convenient and efficient image extraction and data measurement techniques to improve the credibility of the technology, enhance the measurement and body identification functions, and provide consumers with a more reliable measurement method, so as to increase the willingness of consumers to adopt the measurement system by improving consumer perceptions.
Finally, the study shows that consumer perceptions play a key role in linking perceived usefulness (PU), perceived ease of use (PEOU), and attitudes (ATT). To improve the 2D anthropometric system, developers could add interactive features and enhance motion capture by integrating smart technologies like Artificial Intelligence (AI) or Augmented Reality (AR). These improvements would address issues like complex processes, make the system easier to use, and increase consumers’ perceived value and enjoyment. By incorporating AI, the system could create a more engaging and fun experience, while maintaining the core measurement functions of 2D anthropometry. Therefore, it is suggested that smaller fashion companies and consumers opt for 2D systems over 3D scanners to enhance user engagement.
6 Conclusion
In terms of consumers’ reactions to the 2D anthropometric system experience, consumer perceptions play a mediating role in consumers’ willingness to adopt the system; the usefulness and ease of use of the 2D anthropometric system affects consumers’ adoption attitudes through the mediating role of consumer perceptions. Therefore, the 2D anthropometric system technology development team should motivate consumers to adopt the 2D anthropometric system by enhancing the measurement functionality to make the measurement process more straightforward. Smoother interactions between consumers and the measurement system will immerse consumers in the measurement space. This means that enhancements to the usefulness and ease of use of the technology could build hedonistic and utilitarian attitudes toward the 2D anthropometric system, thus triggering consumer enjoyment.
6.1 Limitations
First, the study sample is limited to mainland China, which may affect the generalization of the findings to other regions or countries. Cultural, economic, and technological differences may lead to different consumer perceptions and adoption of new technologies. For example, consumer attitudes toward privacy, adoption of digital technologies, and online shopping habits vary widely across regions. Therefore, future research should consider including more diverse samples across regions or countries to explore cross-cultural differences and assess the generalizability of the findings globally.
Second, differences in device specifications and privacy settings also indirectly affect how consumers interact with the system. Different devices or settings can affect the experience of the technology and the extraction of results, and these factors may bias the results of the study because users’ experiences and perceptions are not solely based on the inherent functionality of the system, but are also influenced by the technical limitations of their devices. Future research should control for these device-related differences by ensuring that mobile device samples are more uniform or by collecting data on various device specifications.
In addition, the level of integration of new technologies with existing systems or infrastructure often hinders their adoption. For example, new technologies may require consumers to update their devices, operating systems or platforms, which may act as a barrier to adoption. Studies may not be able to adequately measure the impact of compatibility issues on users’ willingness to adopt, especially when users are highly invested in existing technologies or systems.
Third, questionnaires often rely on self-reporting by respondents, which can be problematic in terms of false reporting, overconfidence or memory bias. Respondents may not be able to accurately recall their own behaviors or attitudes, or may fail to provide truthful answers for personal reasons. Thus, self-report bias may lead to distorted results. Subsequent studies may use other data collection methods (e.g., behavioral observations, interviews, etc.) to validate self-reported data such as, using multiple indicators to cross-validate the data.
6.2 Scope for future research
This study provides valuable insights into the use of this relatively unexplored technology in fashion online shopping. Future research could conduct cross-cultural studies to validate the findings, which should include an equal number of male and female respondents and use larger samples and more diverse demographics to obtain more generalizable results to reduce gender and cultural bias. Future studies could also adopt a mixed-methods approach, using both qualitative and quantitative data to extend the existing model.
In addition, most of the current applications of 2D measurement systems are concentrated in traditional fields (e.g., manufacturing, quality inspection, etc.), and cross-industry applications have not yet been widely developed. In the future, the 2D measurement system can be applied to the fashion industry to explore the influencing factors in specific environments. For example, through online platforms and measurement systems, consumers can participate in measurement, design, customization, etc., to increase interaction with the brand and enhance their sense of participation and satisfaction.
Finally, since the current research mainly focuses on rational factors such as perceived usefulness and perceived ease of use, there is a bias in the research on the consumer perception level, and the influence of emotional factors on consumer decision-making is gradually recognized as crucial, emotional factors will be added in future research.
Data availability statement
The original contributions presented in the study are included in the article/supplementary material, further inquiries can be directed to the corresponding author.
Ethics statement
Ethical review and approval was not required for the study on human participants in accordance with the local legislation and institutional requirements. Written informed consent from the patients/participants or patients/participants’ legal guardian/next of kin was not required to participate in this study in accordance with the national legislation and the institutional requirements.
Author contributions
LL: Project administration, Resources, Supervision, Validation, Writing – review & editing. YL: Conceptualization, Formal analysis, Investigation, Methodology, Software, Visualization, Writing – original draft. HL: Data curation, Formal analysis, Methodology, Validation, Writing – review & editing.
Funding
The author(s) declare that financial support was received for the research and/or publication of this article. Support was received from Zhejiang Province “Leading Goose + X” Science and Technology Plan Project “Research on Key Technologies for Data-Driven Fashion Product Trend Guidance” (2025C03012); Central Government Guides Local Science and Technology Development Fund Project (2023ZY1029); Hangzhou Major Science and Technology Innovation Project (2022AIZD0153), and Scientific Research Fund of Zhejiang Provincial Education Department (Y202354122).
Acknowledgments
I would like to thank my supervisor, LL for her invaluable help, guidance, and patience throughout this project. Her unwavering support during our regular meetings and thought-provoking discussions helped me persist through challenging problems, making the project much enjoyable. Further, I would like to thank my third marker, HL, whose feedback during the initial stages of the project helped solidify the project’s direction. Finally, I would like to thank all my classmates, friends, and teachers who provided help and encouragement during the experimental process and the writing process, every researcher who never gave up, and the literature, information, and questionnaires cited in the thesis, the information and data provided by you are more comprehensive and detailed in my research, and this acknowledgment is to express my gratitude to you.
Conflict of interest
The authors declare that the research was conducted in the absence of any commercial or financial relationships that could be construed as a potential conflict of interest.
Publisher’s note
All claims expressed in this article are solely those of the authors and do not necessarily represent those of their affiliated organizations, or those of the publisher, the editors and the reviewers. Any product that may be evaluated in this article, or claim that may be made by its manufacturer, is not guaranteed or endorsed by the publisher.
Abbreviations
SN, subject norm; PBC, perceived behavioral control; PU, perceived usefulness; PEOU, perceived ease of use; PR, perceived risk; PE, perceived enjoyment; PV, perceived value; ATT, attitude; BI, behavioral intention.
References
Abdullah, F., Rupert, W., and Ejaz, A. (2016). Investigating the influence of the most commonly used external variables of TAM on students’ perceived ease of use (PEOU) and perceived usefulness (PU) of e-portfolios. Comput. Hum. Behav. 63, 75–90. doi: 10.1016/j.chb.2016.05.014
Ajzen, I. (1991). The theory of planned behavior. Org. Behav. Hum. Decis. Process. Theor. Cogn. Self Regul. 50, 179–211. doi: 10.1016/0749-5978(91)90020-T
Altin, G., Cigdem Aycan, K., and Erhan, O. (2018). “Determinants of mobile banking use: an extended TAM with perceived risk, mobility access, compatibility, perceived self-efficacy and subjective norms,” in Industrial engineering in the industry 4.0 era. Cham: Springer, 225–238.
Ballester, A., Parrilla, E., Piérola, A., Uriel, J., Pérez, C., Piqueras, P., et al. (2016). Data-driven three-dimensional reconstruction of human bodies using a mobile phone app. Int. J. Digit. Human 1, 361–385. doi: 10.1504/IJDH.2016.084581
Beldad, A., Menno, D. J., and Michaël, S. (2010). How shall I trust the faceless and the intangible? A literature review on the antecedents of online trust. Comput. Hum. Behav. Adv. Educ. Res. Comput. Supp. Collab. Learn. (CSCL) 26, 857–869. doi: 10.1016/j.chb.2010.03.013
Benson, V., George, S., and Hemamaali, T. (2015). Information disclosure of social media users: does control over personal information, user awareness and security notices matter? Inf. Technol. People 28, 426–441. doi: 10.1108/ITP-10-2014-0232
Bhat, S. S., Ananth, A., Dsouza, P., Sharanyalaxmi, K., and Tejasvini, S. (2021). “Human body measurement extraction from 2D images,” in Advances in VLSI, signal processing, power electronics, IOT, communication and embedded systems. Singapore: Springer. 752, 343–352.
Bölen, M., and Üstün, Ö. (2020). Understanding the factors affecting consumers’ continuance intention in mobile shopping: the case of private shopping clubs. Int. J. Mobile Commun. 18, 101–129. doi: 10.1504/IJMC.2020.104423
Chang, T.-Z., and Wildt, A. R. (1994). Price, product information, and purchase intention: an empirical study. J. Acad. Mark. Sci. 22, 16–27. doi: 10.1177/0092070394221002
Chaudhuri, A., and Holbrook, M. B. (2001). The chain of effects from brand trust and brand affect to brand performance: the role of brand loyalty. J. Mark. 65, 81–93. doi: 10.1509/jmkg.65.2.81.18255
Chen, C. C., and Jia-Lun, T. (2019). Determinants of behavioral intention to use the personalized location-based mobile tourism application: an empirical study by integrating TAM with ISSM. Futur. Gener. Comput. Syst. 96, 628–638. doi: 10.1016/j.future.2017.02.028
Cheon, J., Sangno, L., Crooks, S. M., and Jaeki, S. (2012). An investigation of mobile learning readiness in higher education based on the theory of planned behavior. Comput. Educ. 59, 1054–1064. doi: 10.1016/j.compedu.2012.04.015
Davis, F. D. (1989). Perceived usefulness perceived ease of use, and user acceptance of information technology. MIS Q. 13, 319–340. doi: 10.2307/249008
Davis, F. D., Bagozzi, R. P., and Warshaw, P. R. (1992). Extrinsic and intrinsic motivation to use computers in the workplace. J. Appl. Soc. Psychol. 22, 1111–1132. doi: 10.1111/j.1559-1816.1992.tb00945.x
Ertürk, R., and Karnaşak, M. (2022). “Anthropometric measurements with 2D images” in The international conference on computer science and engineering, 01–06.
Fagan, M. H., Stern, N., and Barbara, W. (2008). Exploring the intention to use computers: an empirical investigation of the role of intrinsic motivation, extrinsic motivation, and perceived ease of use. Mark. Theory. 48, 31–37. doi: 10.1080/08874417.2008.11646019
Fornell, C., and Larcker, D. F. (1981). Evaluating structural equation models with unobservable variables and measurement error. J. Mark. Res. 18, 39–50. doi: 10.2307/3151312
Gardner, B., Lally, P., and Rebar, A. (2020). Does habit weaken the relationship between intention and behaviour? Revisiting the habit-intention interaction hypothesis. Soc. Personal. Psychol. Compass 14, 1–24. doi: 10.1111/spc3.12553
George, J. F. (2004). The theory of planned behavior and internet purchasing. Internet Res. 14, 198–212. doi: 10.1108/10662240410542634
Giao, H., Bui, N. V., and Tran, Q. (2020). The influence of website quality on consumer’s e-loyalty through the mediating role of e-trust and e-satisfaction: an evidence from online shopping in Vietnam. Supply Chain Manag. 8, 351–370. doi: 10.5267/j.uscm.2019.11.004
Gordon, R., Sally, D., Christopher, M., Paul, C., and Gordon, W. (2018). Empirically testing the concept of value-in-behavior and its relevance for social marketing. J. Bus. Res. 82, 56–67. doi: 10.1016/j.jbusres.2017.08.035
Guiming, S., Qingtao, W., and Fansheng, M. (2015). Image edge detection algorithm based on canny operator. Modern Electron. Technol. 38:92–93+97. doi: 10.16652/j.issn.1004-373x.2015.12.040
Hagger, M. S., Cheung, M. W. -L., Ajzen, I., and Hamilton, K. (2022). Perceived behavioral control moderating effects in the theory of planned behavior: a meta-analysis. Health Psychol. 41, 155–167. doi: 10.1037/hea0001153
Hansen, J. M., George, S., and Vladlena, B. (2018). Risk, trust, and the interaction of perceived ease of use and behavioral control in predicting consumers’ use of social media for transactions. Comput. Hum. Behav. 80, 197–206. doi: 10.1016/j.chb.2017.11.010
Hasan, A., Shah, S., Md, I., and Mohammad, H. (2021). Factors influencing online shopping intentions: the mediating role of perceived enjoyment. Turkish J. Market. 6, 239–253. doi: 10.30685/tujom.v6i3.132
He, Y., Qimei, C., and Sakawrat, K. (2018). Regulatory focus and technology acceptance: perceived ease of use and usefulness as efficacy. Cogent Bus. Manag. 5, 1459–2006. doi: 10.1080/23311975.2018.1459006
Kamiyama, K., Koh, C. J., and Sato, Y. (2020). Systems and methods for weight measurement from user photos using deep learning networks [Internet]. U.S. Patent No. 20200319015A1. Washington, DC: U.S. Patent and Trademark Office.
Kevin, K. F., Hyunsu, K., and Haemoon, O. (2021). What makes Airbnb experiences enjoyable? The effects of environmental stimuli on perceived enjoyment and repurchase intention. J. Travel Res. 60, 1018–1038. doi: 10.1177/0047287520921241
Kim, H. W., Hock, C. C., and Sumeet, G. (2007). Value-based adoption of mobile internet: an empirical investigation. Decis. Supp. Syst. Mobile Commerce Strateg. Technol. Appl. 43, 111–126. doi: 10.1016/j.dss.2005.05.009
Kiriakidis, S. (2017). “Perceived behavioural control in the theory of planned behaviour: variability of conceptualization and operationalization and implications for measurement,” in Strategic innovative marketing, Springer Proceedings in Business and Economics. Cham: Springer, 197–202.
Lai, Y. H. (2017). “The social influence on the behavioral intention to use mobile electronic medical records,” in Advanced topics in intelligent information and database systems, Studies in Computational Intelligence. Cham: Springer. 710, 141–150.
Lee, M. C. (2009). Factors influencing the adoption of internet banking: an integration of TAM and TPB with perceived risk and perceived benefit. Electron. Commer. Res. Appl. 8, 130–141. doi: 10.1016/j.elerap.2008.11.006
Lee, J. S., Hichang, C., Geri, G., Barry, D., and Anthony, I. (2003). Technology acceptance and social networking in distance learning. J. Educ. Technol. Soc. 6, 50–61.
Li, S., Fan, M., and Wu, X. (2018). Effect of social capital between construction supervisors and workers on workers’ safety behavior. J. Constr. Eng. Manag. 31, 1947–1972. doi: 10.1108/ecam-02-2022-0180
Li, J., Xia, S., West, A. J., and Istook, C. L. (2022). Fashionable sportswear working as a body measurement collecting tool. Int. J. Cloth. Sci. Technol. 34, 589–604. doi: 10.1108/IJCST-07-2021-0090
Lin, Y., and Wang, M. (2011). Automated body feature extraction from 2D images. Exp. Syst. Appl. Int. J. 38, 2585–2591. doi: 10.1016/j.eswa.2010.08.048
Liu, K., Wu, H., Zhu, C., Wang, J., Zeng, X., Tao, X., et al. (2022). An evaluation of garment fit to improve customer body fit of fashion design clothing. Int. J. Adv. Manuf. Technol. 120, 2685–2699. doi: 10.1007/s00170-022-08965-z
Liu, K., Zeng, X., Bruniaux, P., Tao, X., Kamalha, E., and Wang, J. (2018). “Garment fit evaluation using machine learning technology,” in Artificial intelligence for fashion industry in the big data era, Springer Series in Fashion Business. Singapore: Springer, 273–288.
Luo, S.. (2017). Application research on 2D image-based non-contact anthropometric technology in clothing E-commerce. The 7th international conference on applied science, engineering and technology 112–118.
Malhotra, N. K., Kim, S. S., and Patil, A. (2006). Common method variance in IS research: a comparison of alternative approaches and a reanalysis of past research. Manag. Sci. 52, 1865–1883. doi: 10.1287/mnsc.1060.0597
Mathieson, K. (1991). Predicting user intentions: comparing the technology acceptance model with the theory of planned behavior. Inf. Syst. Res. 2, 173–191. doi: 10.1287/isre.2.3.173
Meunier, P., and Yin, S. (2000). Performance of a 2D image-based anthropometric measurement and clothing sizing system. Appl. Ergon. 31, 445–451. doi: 10.1016/S0003-6870(00)00023-5
Mitchell, V. W., and Greatorex, M. (1993). Risk perception and reduction in the purchase of consumer services. Serv. Ind. J. 13, 179–200. doi: 10.1080/02642069300000068
Mook, D. G. (1987). Motivation: the organization of action. New York: W.W. Norton. 3, 12–15. doi: 10.1016/j.tourman.2019.104041
Nana, A., Jonathan, M. D., Staynor, S., Arlai, A., El-Sallam, N. D., and Smith, M. (2022). Agreement of anthropometric and body composition measures predicted from 2D smartphone images and body impedance scales with criterion methods. Obes. Res. Clin. Pract. 16, 37–43. doi: 10.1016/j.orcp.2021.12.006
Park, E., Seoin, B., Jay, O., and Hyun, J. C. (2014). Determinants of player acceptance of mobile social network games: an application of extended technology acceptance model. Telematics Inform. 31, 3–15. doi: 10.1016/j.tele.2013.07.001
Pavlou, P., and Mendel, F. (2006). Understanding and predicting electronic commerce adoption: an extension of the theory of planned behavior. MIS Q. 30, 115–143. doi: 10.2307/25148720
Pfaff, N., Da Silva, A., Ozer, E., and Kaiser, S. (2021). Adolescent risk behavior screening and interventions in hospital settings: a scoping review. Pediatrics 147, 6–10. doi: 10.1542/peds.2020-020610
Saadé, R., and Bouchaib, B. (2005). The impact of cognitive absorption on perceived usefulness and perceived ease of use in on-line learning: an extension of the technology acceptance model. Inf. Manag. 42, 317–327. doi: 10.1016/j.im.2003.12.013
Saunders, M., Lewis, P., and Thornhill, A. (2019). Research methods for business students: Routledge. New York: Pearson, 56–58.
Scherer, R., Siddiq, F., and Tondeur, J. (2019). The technology acceptance model (TAM): a meta-analytic structural equation modeling approach to explaining teachers’ adoption of digital technology in education. Comput. Educ. 128, 13–35. doi: 10.1016/j.compedu.2018.09.009
Shaw, N., and Sergueeva, K. (2019). The non-monetary benefits of mobile commerce: extending UTAUT2 with perceived value. Int. J. Inf. Manag. 45, 44–55. doi: 10.1016/j.ijinfomgt.2018.10.024
Sheth, M, and Nisheeth, S. (2019). Predicting body size using mirror selfies. The international conference on intelligent human computer interaction, 151–162.
Taherdoost, H. (2018). A review of technology acceptance and adoption models and theories. Procedia manufacturing, 11th international conference interdisciplinarity in engineering 22, 960–967.
Tao, L., and Yun, C. (2019). Will virtual reality be a double-edged sword? Exploring the moderation effects of the expected enjoyment of a destination on travel intention. J. Destin. Mark. Manag. 12, 15–26. doi: 10.1016/j.jdmm.2019.02.003
Taylor, S., and Peter, T. (1995). Assessing IT usage: the role of prior experience. MIS Q. 19, 561–570. doi: 10.2307/249633
Van Slyke, C., Shim, J. T., Johnson, R., and Jiang, J. J. (2006). Concern for information privacy and online consumer purchasing. JAIS 7, 415–444. doi: 10.17705/1jais.00092
Venkatesh, V., and Fred, D. D. (2000). A theoretical extension of the technology acceptance model: four longitudinal field studies. Manag. Sci. 46, 186–204. doi: 10.1287/mnsc.46.2.186.11926
Venkatesh, V., Morris, M. G., Davis, G. B., and Davis, F. D. (2003). User acceptance of information technology: toward a unified view. MIS Q. 27, 425–478. doi: 10.2307/30036540
Viviani, C., Arezes, P. M., Bragança, S., Molenbroek, J., Dianat, I., and Castellucci, H. I. (2018). Accuracy, precision and reliability in anthropometric surveys for ergonomics purposes in adult working populations: a literature review. Int. J. Ind. Ergon. 65, 1–16. doi: 10.1016/j.ergon.2018.01.012
Wallace, L. G., and Steven, D. S. (2014). The adoption of software measures: a technology acceptance model (TAM) perspective. Inf. Manag. 51, 249–259. doi: 10.1016/j.im.2013.12.003
Wren, P. (2024). Consumers’ interaction with online fashion retailers’ body measurement guidance. J. Fash. Mark. Manag. 28, 1170–1196. doi: 10.1108/JFMM-05-2023-0137
Wu, L., and Sheng, Q. (2022). Research on smart wearable medical APP design based on TAM model. Des. Art Res. 12:109–113+138.
Xia, Y., Jiayu, S., Beibei, Z., Shouning, J., Yuqing, L., and Bingfei, G. (2022). Individualized generation of young women’s crotch curve based on body images. Int. J. Ind. Ergon. 90:103296. doi: 10.1016/j.ergon.2022.103296
Xia, S., Li, J., Istook, C. L., and West, A. J. (2022). A 2D image body measurement system developed with neural networks and a color-coded measurement garment. Int. J. Cloth. Sci. Technol. 34, 967–978. doi: 10.1108/IJCST-08-2021-0114
Xia, S., Siming, G., Jiayin, L., and Cynthia, I. (2019). Comparison of different body measurement techniques: 3D stationary scanner, 3D handheld scanner, and tape measurement. J. Textile Inst. 110, 1103–1113. doi: 10.1080/00405000.2018.1541437
Xie, J. L., Liying, Y., Wei, H., and Min, Y. (2021). Understanding FinTech platform adoption: impacts of perceived value and perceived risk. J. Theor. Appl. Electron. Commer. Res. 16, 1893–1911. doi: 10.3390/jtaer16050106
Yang, B., Youngchan, K., and Changjo, Y. (2013). The integrated mobile advertising model: the effects of technology-and emotion-based evaluations. J. Bus. Res. Adv. Res. Methods Market. 66, 1345–1352. doi: 10.1016/j.jbusres.2012.02.035
Yu, J., Hwansoo, L., Imsook, H., and Hangjung, Z. (2017). User acceptance of media tablets: an empirical examination of perceived value. Telematics Inform. 34, 206–223. doi: 10.1016/j.tele.2015.11.004
Yuan, Y., Myung-Ja, P., and Jun-Ho, H. (2021). A proposal for clothing size recommendation system using Chinese online shopping malls: the new era of data. Appl. Sci. 11, 112–115. doi: 10.3390/app112311215
Zeithaml, V. A. (1988). Consumer perceptions of price, quality, and value: a means-end model and synthesis of evidence. J. Mark. 52, 2–22. doi: 10.1177/002224298805200302
Zhou, T., Yao, S., and Ping, Z. (2022). Continued use intention of travel apps: from the perspective of control and motivation. Tech. Anal. Strat. Manag. 34, 703–716. doi: 10.1080/09537325.2021.1916457
Keywords: body anthropometric systems, online shopping, TAM-TPB model, consumer perceptions, adoption intention
Citation: Liu L, Li Y and Li H (2025) A study of consumer willingness to adopt two-dimensional anthropometric measurement systems. Front. Comput. Sci. 7:1389291. doi: 10.3389/fcomp.2025.1389291
Edited by:
Mohammed A. Al-Sharafi, University of Technology Malaysia, MalaysiaReviewed by:
Kamal Karkonasasi, Universiti Malaysia Kelantan, MalaysiaKandarp Singh, Bennett University, India
Copyright © 2025 Liu, Li and Li. This is an open-access article distributed under the terms of the Creative Commons Attribution License (CC BY). The use, distribution or reproduction in other forums is permitted, provided the original author(s) and the copyright owner(s) are credited and that the original publication in this journal is cited, in accordance with accepted academic practice. No use, distribution or reproduction is permitted which does not comply with these terms.
*Correspondence: Hao Li, bGgtY2FsdmluQHpzdHUuZWR1LmNu