- 1Computer Science Department, CICESE, Ensenada, Baja California, Mexico
- 2Department of Computing and Design, Sonora Institute of Technology (ITSON), Ciudad Obregon, Sonora, Mexico
The integration of technology into cooking and eating routines often leads to distractions, hindering mindfulness. Mindful eating involves awareness of the present moment and attention to sensory experiences. While mindful eating has been promoted, mindful cooking remains underexplored. As conversational agents become increasingly popular in diverse fields like education, healthcare, and e-commerce, we are leveraging this technology to promote mindfulness practices that enhance the cooking and eating experience. This paper introduces MyndFood, a conversational agent designed to promote mindful cooking and eating. We describe the development process of MyndFood and present the outcomes from a user experiment involving 40 participants who engaged with the system while preparing sushi. The study employed a between-subjects design featuring two conditions for the agent: mindful and non-mindful. Results indicate that participants in the mindful condition experienced greater enjoyment and heightened food awareness compared to those in the non-mindful condition. These findings suggest that conversational agents can enhance hedonic experiences and improve food awareness by promoting mindful practices.
1 Introduction
Individuals often use devices like smartphones, tablets, and laptops during meals. This includes eating as well as cooking. These devices are used for watching videos, browsing social media, texting, reading, or working. Even though these devices provide entertainment, this behavior leads to reduced attention to the meal, which affects meal satisfaction and creates negative eating habits such as overeating (Lemke and Schifferstein, 2021; Ogden et al., 2013).
In light of the distraction caused by technology during meals, exploring alternative strategies becomes meaningful. One such approach is mindfulness. According to Kabat-Zinn (2003), mindfulness is the awareness that emerges through paying attention on purpose, in the present moment, and non-judgmentally to the unfolding experience moment by moment. By implementing mindful eating techniques, this practice can be applied while interacting with food, such as eating or cooking. Mindful eating is characterized by being fully present during meals, attentively observing how food affects our senses, and acknowledging the physical and emotional sensations that arise while eating (Warren et al., 2017). There is growing evidence of the positive impact of this practice on people's lives, such as reduced impulsive eating, lower calorie consumption, and healthier snack choices (Jordan et al., 2014; Mercado et al., 2021; Zhang et al., 2021).
In recent years, there has been a surge in the use of mindfulness applications, with evidence supporting their beneficial impact, such as dealing with work stress (Bostock et al., 2019; Azṕıroz-Dorronsoro et al., 2023). Nevertheless, maintaining sustained user engagement with mindfulness apps presents challenges (Linardon, 2023). Online mindfulness applications such as headspace.com typically provide guided meditation in audio format but lack interactive components allowing personalized user engagement or feedback. In contrast, a conversational agent is designed to simulate a conversation with human users, offering customized responses and feedback (Adamopoulou and Moussiades, 2020). Building on this, there is potential for the development of virtual dining companions (Niewiadomski et al., 2022) that can offer personalized interactions depending on the context, such as the user's meal choice, location, and company.
In 2020, the COVID-19 pandemic led to changes in people's eating and cooking habits, some of which still prevail (Chan et al., 2023). Due to lockdown restrictions, individuals were compelled to cook and eat at home. During the early phases of the pandemic, people reported adopting unhealthy eating habits, such as mindless eating, which may lead to overeating (Parra et al., 2021). Worsening eating habits are more associated with having a specific dependence on a mobile phone or staying at home and not leaving except for emergencies, persisting even after the pandemic (Prieto et al., 2023). However, the exceptional circumstances of the lockdown also presented a positive opportunity for some to improve the quality of their diet by dedicating more time to cooking (Sarda et al., 2022). Regularly preparing meals at home is linked to eating a healthier diet, regardless of whether weight loss is a goal (Wolfson and Bleich, 2015).
Meanwhile, as ubiquitous computing becomes a reality and computing becomes part of our daily lives, there has been growing interest in the HCI community in exploring the role of interactive technologies associated with food-related activities, such as eating and cooking (Spence et al., 2019; Khot and Mueller, 2019). For instance, Computational Commensality extends to research involving technology that explores diverse social dimensions of food, including aspects of cooking and dining experiences (Niewiadomski et al., 2019). These fields and the broader scientific literature have proposed a few interactive systems to promote mindful eating. However, the design and evaluation of technologies to support mindful cooking remain largely unexplored.
Therefore, in this work, we designed, implemented, and evaluated a conversational agent to enhance the cooking activity using mindfulness practices to increase awareness. This paper introduces MyndFood, a conversational agent in a smart environment for mindful cooking. We present the results of an evaluation of the agent with users, comparing the hedonic experience and level of mindfulness under two conditions: mindful and non-mindful. The outcomes of this research contribute to the domain of Computational Commensality by assessing the feasibility of using an innovative mindful conversational agent designed to enhance the cooking and eating experience.
2 Related work
This section explores the intricate relationship between technology, mindfulness, and food behaviors. We begin by introducing the concepts of mindfulness and mindful eating, presenting research examples that apply these principles. Following this, we investigate the concept of computational commensality, outlining its scope and highlighting related work in the context of food. Lastly, we present examples of conversational agents designed to assist the cooking process, illustrating their potential to enhance the cooking experience.
2.1 Mindfulness and food behaviors
Mindfulness is a capacity of attention and awareness oriented to the present moment that varies in degree within and between individuals and can be assessed empirically (Black and Slavich, 2016). Similarly, mindful eating is defined as being aware of the present moment when one is eating, paying attention to the effect of the food on the senses, and recognizing both the physical and emotional sensations in response to eating (Kristeller and Wolever, 2014). Reviews have examined the efficacy of practicing mindful eating (Warren et al., 2017; Zhang et al., 2021), where mindfulness-based methods seem to be most successful in addressing binge eating (Godfrey et al., 2015; Mercado et al., 2021; Giannopoulou et al., 2020), emotional eating (Beccia et al., 2020), and could be a practical approach to weight control (Fuentes Artiles et al., 2019).
External factors can impact the way we eat and the quantities we consume. These factors include aspects like the size and shape of our plates (Wansink et al., 2006), whether we dine alone or with others (Meegahapola et al., 2020), and the portion sizes indicated on food packaging (Wansink, 2004), among others. To address this, practicing mindfulness while eating, including preparing food, can be highly effective. Introducing mindfulness right from the food preparation moment could also influence the conscious eating process. For instance, Tylka et al. (2013) indicate that engaging children in food preparation can effectively promote mindful eating by encouraging them to pay attention to their hunger and satiety cues. Furthermore, studies of mindful eating practices highlight the importance of using all senses to appreciate more food. For instance, a study with Mexican-American parents suggests that food-related cultural practices, such as cooking and food traditions, may enhance mindful eating and mindful food parenting (Méndez et al., 2020).
Mindfulness-Based Therapies have shown potential as effective strategies in treating patients with a wide range of mental and physical health problems, supported by a growing body of empirical evidence and clinical integration of therapies with mindful principles (Khoury et al., 2013; Querstret et al., 2020; Ramos et al., 2022; Moqbel et al., 2023). Recently, with the ubiquity of mobile devices such as smartphones and smart speakers, applications have been developed to promote mindfulness practices. However, most of these technologies are commercial apps that have yet to be validated by research to demonstrate their effectiveness (Mani et al., 2015; Schultchen et al., 2021).
2.2 Mindfulness systems and computational commensality
In recent years, the intersection of technology and mindfulness has gained increasing interest as researchers explore innovative ways to leverage technological advancements to promote mindful practices. For instance, Mindful Garden (Liu et al., 2022) is an augmented reality (AR) lens that facilitates co-located mindfulness experiences. Mindful Garden transforms individuals' environments into relaxing spaces and uses biosignal sensors to represent one person's inner state as flowers in a shared AR environment. This research offers novel possibilities for enhancing mindfulness practices. In addition to AR applications, Inner Garden (Roo et al., 2017) presents a novel approach to mindfulness support, wherein the authors introduce an augmented sandbox that enables users to create a living miniature world by manipulating sand, which reflects their real-time physiological states, such as breathing. These studies demonstrate the diversity and promise of technology-driven mindfulness interventions. Recently, chatbot-based systems have also shown promise in facilitating mindfulness-based stress reduction (MBSR) practices. For example, Li et al. (2024) developed and piloted a rule-based chatbot delivering MBSR sessions to university students with depressive symptoms, demonstrating improvements in mindfulness, depression, anxiety, and stress. These studies demonstrate the diversity and promise of technology-driven mindfulness interventions.
Commensality is the act of eating together and is one of the most commonly shared practices among human beings (Jönsson et al., 2021). Digital Commensality is a set of practices that combine food and interactive digital agents, such as robots (Spence et al., 2019). Computational Commensality extends to research involving technology that explores diverse social dimensions of food, including aspects of cooking and dining experiences (Niewiadomski et al., 2019). These concepts, along with Human-Food Interaction (Khot and Mueller, 2019), cover various research and systems, each targeting different facets of the dining experience. This includes technologies designed to amplify food flavors (Bruijnes et al., 2016), tools for virtually connecting with loved ones during meals (Foley-Fisher et al., 2010), and methods for recognition of eating activities (Zhang et al., 2020). Other works are dedicated to creating virtual assistants or companions to accompany individuals during meals (Niewiadomski et al., 2022). However, few of these prototypes are designed with mindful eating in mind (Khot et al., 2022), and notably, mindful cooking has not been addressed at all.
Few prototypes that promote mindful eating practices have been developed. One such prototype is an intelligent spoon designed to assist individuals in staying attentive to their food (Khot et al., 2020). Similarly, Epstein et al. (2016) present a novel approach to fostering mindful eating through their concept of daily challenges called Crumbs. The idea of Crumbs involves completing daily food challenges by consuming a specific food that meets the challenge criteria, such as “something high in Omega-3.” To examine the effectiveness of Crumbs, the Food4Thought app was deployed in a 3-week field study with 61 participants. The study demonstrated that the app successfully promoted engagement and mindfulness, providing valuable opportunities for participants to learn about various food choices.
The work of Zhang et al. (2023) introduces a voice assistant designed to facilitate mindful eating with two approaches: friend and counselor. They demonstrate the potential of these assistants in promoting healthier eating habits and mindful consumption. However, they only focus on eating and do not address cooking.
These applications highlight an opportunity to develop technologies that promote mindful eating practices. Nonetheless, these apps or prototypes concentrate solely on eating, disregarding food preparation, an essential component of our food-related behaviors. As a result, there is an opportunity to explore the design of technologies that can serve as mindful guides during cooking and eating activities.
2.3 Conversational agents for food-related activities
A smart environment is a digital setting that actively and intelligently assists individuals in their daily activities (Augusto et al., 2010). Incorporating dialogue into a smart environment improves the interaction between humans and computers and is a key factor for a friendly system (Minker et al., 2009). A conversational agent (i.e., a chatbot, digital assistant, smart bot) is an artificial intelligence (AI) program designed to simulate conversation with human users. Conversational agents find applications in diverse fields, including education (Winkler and Söllner, 2018), healthcare (Tudor Car et al., 2020), e-commerce (Li and Wang, 2023), and therapeutic contexts (Catania and Garzotto, 2023). They have also been employed in scenarios such as hospitality counseling (Wang et al., 2024) and in supporting executive functions (Pergantis et al., 2025).
In recent years, the integration of conversational agents into the cooking domain has garnered attention. This trend is driven by the widespread adoption of virtual assistants such as Alexa, Siri, Cortana, and other similar devices (Hoy, 2018). These developments have led to research on recipe recommendation systems utilizing conversational agents (Barko-Sherif et al., 2020). Additionally, chatbots have been developed to assist the general process of following a recipe while cooking in the kitchen (Gottardi et al., 2022).
Other conversational agents present features such as meal recommendations and food image analysis. For instance, Foodie Fooderson (Angara et al., 2017) is a conversational agent built using IBM Watson technology that assists families in making better use of groceries while promoting healthy eating habits. Another example is Lucy (Parra et al., 2018), a conversational agent developed to provide users with dietary guidance, examining their diet with images, and answering their nutrition-related questions. Lastly, Kuoppamäki et al. (2023) designed a multimodal conversational agent specifically tailored to address the unique needs and challenges encountered by older adults in the kitchen.
Despite the existence of conversational agents designed to assist with cooking, the majority of these systems primarily present cooking steps without prioritizing the enhancement of the overall user experience. This includes fostering an enjoyable cooking activity and promoting healthy habits, such as mindfulness about food. To our knowledge, interactive technologies (or conversational agents) have yet to be developed or evaluated to assist in mindful cooking. Next, we present the methodology for designing and evaluating a conversational agent for mindful cooking and eating.
3 Methods and materials
We followed a user-centered approach (Abras et al., 2004) to design and develop MyndFood. This involved conducting an exploratory study, followed by an iterative process of defining, prototyping, and testing a conversational agent to enhance the food experience. We next explain each phase of the process:
1. Exploratory Study: This phase comprises interviews with individuals to uncover food behaviors and eating experiences at home.
2. Design Sessions: This step entails sessions with participants and experts for generating ideas, conceptualizing, and outlining potential design ideas to enhance individuals' food experiences.
3. Technology Implementation: Translating the chosen design ideas into high-fidelity and working prototypes of interactive systems.
4. User Experiments: This involves formative evaluations and experiments to refine the design, obtain user feedback, and test some hypotheses.
5. Results & Reports: This involves refining the design ideas, obtaining research insights, and a deeper understanding of the problem.
Upon concluding the initial cycle encompassing steps 2 to 5, the procedure circles back to step 2. This facilitates the incorporation of user input and the examination of novel concepts and potentials. The iterative nature of this process guarantees an ongoing advancement and enhancement of the system.
We next describe the activities carried out in each of the phases.
3.1 Exploratory study
A preliminary qualitative study was conducted to understand individuals' eating and cooking behaviors at home (Parra et al., 2021). The study started in 2020 during the COVID-19 pandemic, which allowed us to highlight instances of people's food experiences isolated in their homes. For this purpose, we interviewed 14 young professionals (aged 26 on average), covering topics related to lifestyle, eating and cooking behaviors, and technology usage. We verbatim transcribed the interviews, which were then analyzed using thematic analysis.
The results of Parra et al. (2021) show how the pandemic caused changes in people's eating behaviors, and most of these changes had a negative impact, particularly during the initial stages of the pandemic. For example, the stress and anxiety caused by the pandemic led some people to engage in behaviors such as overeating. Similarly, isolation caused anxiety in others due to being away from their loved ones. On the other hand, some people took this opportunity to cook meals at home instead of buying fast food. These behaviors were triggered for some by the confinement situation, but it helped highlight cases to understand how people eat and cook at home regularly. From these findings, four primary themes surfaced, each presenting unique design opportunities:
1. Negative eating behaviors: Technology is being used to distract during mealtime and can lead to overeating in some individuals. Design opportunities include creating systems that prevent these behaviors or promote healthy eating habits such as mindful eating.
2. Increased home cooking and eating: People were compelled to eat at home for an extended period due to lockdown and social confinement measures. This circumstance led to a notable rise in home cooking among people. This presents an opportunity to enrich the cooking experience and dining alone at home.
3. Surge motivation to improve health: During this period, some participants increased their motivation to achieve better health and actively sought assistance from others. People usually sought advice from their closest peers, such as family or friends, requested recommendations on nutrition or exercise, and stayed in touch regarding their process. Thus, systems for nutritional advice would require a feature for connection with others.
4. Reduce food socialization: A decrease in food-related socialization was a widespread trend among most people. The restrictions imposed by the lockdown appear to have altered the nature of social interactions during meals. Therefore, design strategies should explore alternative forms of socialization technology facilitates, such as a virtual environment, to connect with others while eating.
After identifying potential design opportunities, we uncovered two primary avenues for developing future technologies. The first path relates to themes 1 and 2. Creating interactive technologies that promote mindfulness while eating and cooking at home would decrease negative behaviors such as overeating. On the other hand, there is a second path involving themes 3 and 4, which focus on creating solutions to connect with people and loved ones during mealtimes. This second path has been explored more, with prototypes reported in the literature to address digital commensality (Spence et al., 2019).
3.2 Design sessions
Following a user-centered design approach (Abras et al., 2004), we organized design brainstorming sessions to develop innovative technological solutions to enhance individuals' food experiences. The initial phase of brainstorming involved the authors of this paper, focusing on conceptualizing primary ideas and frameworks. Following this, we conducted three online design sessions with technology professionals, each lasting 1 h. The first session involved three software engineers. For the second session, we recruited two experienced application designers with user interface design and user experience expertise. The third session involved two web designers and one data analyst working in the software industry.
The brainstorming sessions yielded various innovative and creative application ideas, each tailored to address the unique design opportunities identified in the realm of food experiences from the qualitative study. In these sessions, we first briefed participants on the outcomes of the qualitative study. Then, we presented specific problem scenarios as starting points for brainstorming. The task for each participant was to generate a range of potential solutions collaboratively and, subsequently, refine and pick the most promising idea by dot-voting. We next provide an overview of each idea, including the problem scenarios they address, and feature the idea solutions that garnered the most support among the participants:
Scenario 1: Mindless overeating. Miguel struggles with compulsive eating due to increased home confinement and tech distractions. Our solution, “Just Eating,” is a conversational agent for smart speakers designed to provide audio guidance that promotes mindful eating habits. This agent reduces distractions, fosters awareness, and encourages slower eating, addressing Miguel's challenges directly.
Scenario 2: Cooking and loneliness. Mrs. Dorita, who enjoys cooking for her family, feels isolated due to reduced family visits during the pandemic. The “Sharing My Food” app connects home cooks with extra food to individuals seeking homemade meals, particularly students. This platform supports meal sharing, enables community building through in-app conversations, and helps Mrs. Dorita regain social fulfillment in cooking.
Scenario 3: Eating alone. Susy feels lonely during meals after her roommates left due to pandemic restrictions. The “Table For 3” app allows users to join a virtual audio room with two other individuals during mealtimes, fostering connection and reducing isolation. This application includes healthy eating tips and recipe-sharing features, addressing Susy's need for companionship at meals.
Scenario 4: Missing social dining experiences. Joaquin misses dining out and trying new restaurants with friends, limited by pandemic-related restrictions. Our solution, “Restaurante En Línea” (Online Restaurant), provides a virtual environment where users navigate with avatars, meeting others in proximity via video and audio. This tool recreates the social dynamics of dining out, with an option for synchronized food delivery, fulfilling Joaquin's need for exploration and social dining.
Following design sessions with technology experts, we reviewed their ideas and explored state-of-the-art research related to the identified design opportunities. While technology has been used to facilitate remote communal dining with loved ones or to connect people during meals through digital commensality (Pereira-Castro et al., 2022), a unique opportunity lies in developing more interactive solutions that integrate mindfulness into the eating experience. Moreover, incorporating mindfulness into the cooking process remains largely unexplored, steering our focus toward creating solutions that enhance both eating and cooking through mindful practices. Consequently, we chose to further develop this mindfulness-centered idea to create an interactive system that holistically enhances both the eating and cooking experience.
3.3 MyndFood: a mindful cooking conversational agent
We decided to explore using a conversational agent as an orchestrator of a smart environment aimed at promoting mindful cooking. We selected sushi as the dish to prepare since it provides a variety of ingredients and requires a relatively straightforward and quick preparation process, suitable even for beginner cooks. Additionally, sushi represents a compelling choice within the Mexican cultural context, as it is a cuisine where expertise is not widely prevalent. The following scenario explains the functionality and the potential benefits of creating this type of technology:
Sofia, a graduate student living away from home, feels lonely and stressed, leading to changes in her eating behavior and rapid weight gain. She seeks help and consults a nutritionist who suggests incorporating mindful eating techniques and cooking her meals rather than ordering or eating out. However, Sofia struggles to stay focused during meals due to multiple distractions since she often watches TV or uses her smartphone while cooking and eating. The nutritionist recommends MyndFood, a smart conversational environment that utilizes mindfulness techniques during cooking and eating. Sofia starts using the system and finds it helpful in preparing dishes such as sushi. MyndFood guides her through the process, encouraging her to be present and mindful. It provides step-by-step instructions, reminding her to appreciate the ingredients' textures, aromas, and flavors. The system also prompts Sofia to chew slowly and savor each bite, allowing her to experience greater satisfaction and enjoyment from her meals. By using MyndFood, Sofia not only improves her cooking skills but, more importantly, develops healthier eating habits and finds joy in interacting with the system a couple of times a week.
3.3.1 System requirements
Based on the findings of the exploratory study and following the insights and scenarios from the design sessions, we next present the system requirements of MyndFood:
• (R1) Recipe instructions: The system should offer clear, step-by-step guidance for preparing the chosen dish. This includes ingredient preparation, rolling techniques, and serving suggestions. The language should be simple and easy to follow for all skill levels.
• (R2) Voice instructions: The primary mode of interaction should be voice-based due to its ease of use and since the user will often have her sight and hands focused on the food. Thus, the system should read aloud recipe instructions to users. The voice should be pleasant and easy to understand.
• (R3) Recipe videos: Videos are helpful for beginners, especially for complex tasks like sushi rolling. Short, concise videos demonstrating key steps can provide users with a clear visual reference. These videos should focus on the techniques and be easily played and paused by users' voice commands.
• (R4) Voice navigation: The user should be able to navigate the recipe steps easily using simple voice commands. The system should understand and respond to conventional voice commands like “next,” “back,” and “repeat,” among others.
• (R5) Mindfulness integration: This unique feature can enhance the cooking experience by encouraging users to engage their senses and be present in the moment. The system should encourage users to pay attention to the textures, scents, and sounds in the cooking process, even leading to brief mindful moments before tasting.
• (R6) Audio feedback: In response to user queries or comments, the system should provide relevant audio responses to user queries and input.
• (R7) Activity tracking: This advanced feature involves the system detecting and responding to user actions. The system should detect and track user food preparation and consumption activities. For example, detecting when a person finishes taking a bite to ask about its taste.
3.3.2 MyndFood prototype
The MyndFood prototype is a smart conversational environment that utilizes mindful techniques to guide individuals in food preparation. The agent has a speaker and a screen as its primary interface. For this prototype, the agent's capabilities are focused on the predefined process of preparing sushi, accompanied by specially designed audio and video support. Nevertheless, this agent can be modified or scaled for other meals and recipes.
For this initial prototype, the first three requirements (R1: Recipe Instructions, R2: Voice Instructions, and R3: Recipe Videos) were fully implemented, all focused on preparing a specific food item, in this case, sushi. As for requirement R4 (Voice Navigation), a Wizard of Oz approach was used (Dow et al., 2005), in which a person remotely listens to user interactions and selects the next navigation steps.
Regarding requirement R5 (Mindfulness Integration), we created guidance texts and audio for a mindful cooking approach, encouraging users to engage their attention and senses in the cooking process fully. We used Amazon Polly Voices text-to-speech tools (https://aws.amazon.com/es/polly/) for this purpose. These audio instructions were designed based on sample audio transcripts containing the kind of phrases and language commonly used in this context. For requirement R6 (Audio Feedback), we employed the same Amazon Polly Voices tool to generate audio responses to user comments, such as “well done” or “interesting comment.” A wizard responsible for guiding the system's navigation remotely plays these audio feedback cues. Lastly, for R7 (Activity Tracking), we decided to defer implementation in this initial prototype since the primary focus is to validate the impact of the system using mindfulness practice. The implementation of R7 is planned for future work.
This initial version of MyndFood serves as a means to validate the concept of integrating mindfulness techniques into cooking and eating behaviors before developing a fully autonomous system. Furthermore, it helps us to prevent possible setbacks or errors with external tools that need to be tested.
Figure 1 shows a selection of images that users encounter while interacting with MyndFood. The graphical user interface follows a simple and minimalist design, allowing individuals to focus on their meals. We also include video playback for specific recipe steps to help users better understand the food preparation process. These videos were carefully recorded, compiled, and edited to demonstrate particular actions, such as a video explaining the proper technique for cutting surimi for sushi.
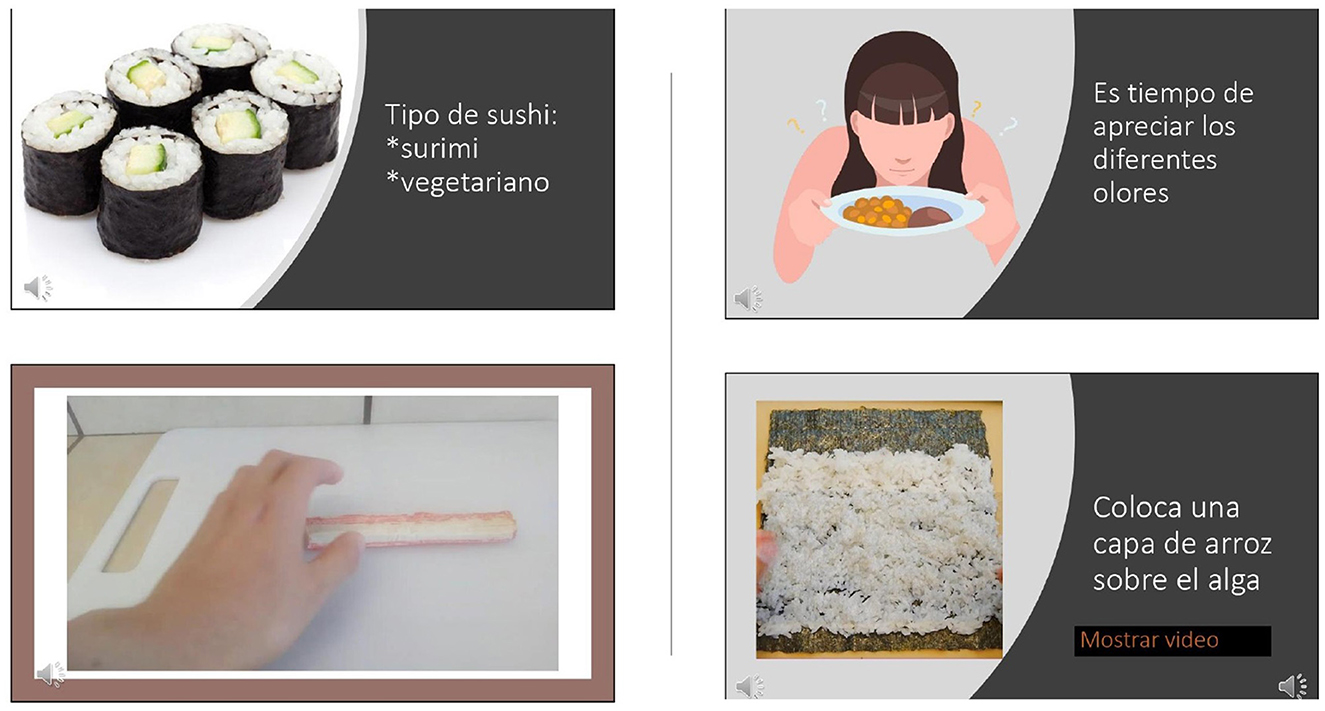
Figure 1. Graphical Interface MyndFood. Example of four images/videos displayed to the user on the monitor. Translation to English: (top-left) sushi type: surimi, vegetarian; (top-right) it's time to appreciate the different aromas; (bottom-right) place a layer of rice over the seaweed (show video).
3.3.3 Sample interaction with MyndFood
The interaction with MyndFood follows a script that displays the recipe steps with general instructions for preparing sushi. Special guided instructions are integrated during the interaction to incorporate the mindful aspect. As an illustration, the initial stages of the interaction with the agent are as follows, using the following terminology: [GI] for General Instruction, [MI] for Mindful Instruction, and [U] for the user's expected response.
• [GI] Hello! Welcome. I am your virtual assistant, and today, I will help you prepare sushi. Are you ready to start?
• [U] “Yes, I'm ready”
• [MI] Today, we are going to prepare a maki-style sushi roll. We will do it using a mindful cooking technique, and during this experience, I will guide you to use all your senses so you can fully enjoy your food. Before we begin cooking, let's take a moment to breathe deeply. Try to follow the image while inhaling and exhaling. Let me know when you're ready to continue.
• [U] The user briefly follows a breathing rhythm image. “I'm ready”
• [GI] To start, we will select the type of sushi. You can choose between surimi sushi or vegetarian sushi. Which option would you like to choose?
Likewise, the instructions for preparing sushi ingredients contain guided steps to motivate users to be mindful of their senses throughout the preparation process. For instance, after each general instruction to cut the cucumber and surimi, users are encouraged to center their attention on their senses and are prompted to describe their sensory perceptions, following standard dialogue feedback [DF] from the agent:
• [GI] Cut one-third of the cucumber into thin strips. If you need extra help, please feel free to ask me to show you a video on how to do it.
• [MI] While doing this process, focus all your senses on the food. Observe the color of the cucumber and notice how its aroma enters your nose.
• [U] “Done, next step, please”
• [GI] Next step, cut the surimi into thin rectangles (show video command available).
• [MI] As you do this step, pay full attention to the food and notice how its strong smell enters your nose. Then, let me know what you think about the smell of the surimi.
• [U] “it smells like fresh seafood.”
• [DF] Interesting response.
Furthermore, once the ingredients are prepared, the agent poses questions about the user's sensory experiences and motivates them to articulate aloud all they perceive. For instance, this interaction unfolds in the following manner:
• [MI] Now, let's take a moment to appreciate the ingredients. Please take a few seconds to observe them carefully. Could you tell me the colors you can see?
• [U] “I see white in the rice and cream cheese, orange in the carrot, a combination of white and orange in the surimi, green in the cucumber, and black in the seaweed.”
• [DF] Well done.
• [MI] Now, take a few seconds to appreciate the different aromas. You can take the ingredients and bring them closer to your nose. Finally, please tell me which smell you like the most or can quickly identify.
• [U] “The strongest smell I perceive is that of the surimi.”
• [DF] Interesting response.
• [MI] For the next steps, please be sure to pay attention to your sense of touch. Could you try to feel the texture of the ingredients and tools you're going to use? Are you ready to continue with the preparation?
• [U] “Yes, I'm ready.”
Lastly, at the end of the preparation instructions, the agent prompts the user to arrange the sushi pieces on a plate for tasting. At this point, the user is given a couple of minutes to taste their meal and briefly comment on its flavor to the agent. Following this exchange, the interaction comes to an end. This closing is carried out as follows:
• [GI] Place the sushi pieces on a plate to enjoy them. I would suggest accompanying it with soy sauce or any dressing you prefer.
• [U] “Done.”
• [MI] Please remember to observe the sushi carefully. Notice the smells as it approaches your nose. Feel the texture inside your mouth. And when you taste it, let me know what you think about its flavor.
• [U] The user takes a few minutes to savior the sushi. “It tastes very good.”
• [GI] Thank you very much for following the process. “I leave you to enjoy the rest of your sushi freely!”
3.4 User experiment design
We present the design of a user experiment to assess the agent's performance for mindful cooking and eating. To accomplish this, we designed an experiment to measure and compare variables related to the individual's mindfulness level during system interaction and assess the overall user experience following the interaction. Consequently, two distinct versions of the agent are introduced: one emphasizing the mindful attributes of the system and another non-mindful version.
This experiment aims to test the next two hypotheses:
• H1: Participants will perceive a higher level of awareness while cooking and eating when using a mindful conversational agent to assist in food preparation than a non-mindful conversational agent.
• H2: Participants will enjoy the experience more when using a mindful conversational agent to assist in cooking and eating than a non-mindful conversational agent.
The experiment's independent variable is the conversational agent, specifically with two types of interaction: mindful and non-mindful. The mindful agent will guide the users (their senses) when preparing and tasting the food and show explanatory videos. On the other hand, the non-mindful agent will not use mindful audio but will offer access to the same support videos.
• Mindful agent: An agent that guides the user through the entire sushi preparation process using a mindful cooking and eating technique.
• Non-mindful agent: An agent that provides general instructions for making sushi without including mindful instructions.
The dependent variables of the experiment are:
• Mindfulness: Measures the level of awareness during the food experience and is divided into two activities separately: cooking and eating. The Mindful Eating Scale instrument (Hulbert-Williams et al., 2014), adapted for cooking, is used with two constructs:
- Awareness: measures the level of attention a person gives to their food by focusing on her senses.
- Act with awareness: measures the level of distraction in a person's mind while engaging in their activity with food.
• Hedonic experience: measures the level of enjoyment experienced when interacting with the agent during food preparation. We utilize the Likeability construct from the SASSI instrument (Hone and Graham, 2000). Additionally, we employed the remaining constructs of the instrument to assess the general characteristics of the agent:
- Likeability: refers to the user's ratings of the systems as useful, pleasant, and friendly.
- Response accuracy: refers to the user's perceptions of the system as accurate and, therefore, doing what they expect.
- Cognitive demand: refers to the perceived effort needed to interact with the system and the feelings resulting from this effort.
- Annoyance: refers to the extent to which users rate the system as repetitive, boring, irritating, and frustrating.
- Habitability: refers to the extent to which the user knows what to do and knows what the system is doing
- Speed: refers to how quickly the system responds to user inputs.
3.4.1 Experimental setup
Figure 2 shows the setup for the study where the system is used to prepare and taste sushi. For this study, the agent is controlled through the Wizard of Oz method for navigation. We provided participants with all the necessary ingredients for preparing surimi or vegetarian sushi. These ingredients include cucumber, carrot, cream cheese, seaweed, sushi rice, oil, and several accompanying dressings. We also provided participants with all the kitchen utensils and tools required to prepare this dish, such as plates, makisu (sushi rolling mat), cutting board, knives, fork, spoon, cloth, and napkin.
The experiment took place in the institutional department kitchenette. This kitchenette has been equipped and designated to host participants so they can interact with the system. We allocated one hour for each participant to interact with the system. This location provided a controlled environment to minimize distractions that could affect the system's experience. Experimenting at home would require autonomous and portable technology that each participant could install and additional challenges in terms of experimental control.
3.4.2 Experimental procedure
The experimental procedure is illustrated in Figure 3. At the beginning of the experiment, participants interacted with an Amazon Echo device to familiarize themselves with the commands and cooking applications designed for smart speakers. Subsequently, participants are assigned to one of the two experimental conditions: mindful and non-mindful agent, where the latter does not provide mindful audio. At the end of the session, participants completed two questionnaires to assess their level of awareness during the food experience and their overall experience when interacting with the voice agent. The entire session lasted approximately 45 min.
We next provide a breakdown of the activities during the session:
• Instruction & consent: A researcher provides the participant with general session instructions and requests their consent by explaining the experiment's details, accompanied by a consent form.
• Alexa tasks: Participants are instructed to interact with an Amazon Echo device for 5 min, providing commands to Alexa like “Search for a surimi sushi recipe,” “Ask for the next step,” and other similar tasks. This exercise aims to familiarize the person with using a conversational agent and identify the typical commands. The Amazon Echo device was not used in the subsequent tasks.
• Cooking & eating experience: We allocate participants in one of the two conditions. The assignment is determined by the participant's arrival time for the session, meaning they do not know which type of agent they will be assigned to. This exercise is the core part of the experiment, during which the participant is guided through the preparation of sushi and is encouraged by the agent to eat the sushi at his own pace.
• First impressions: After interacting with the agent, a researcher asks open-ended questions about the participant's overall experience. Depending on the responses, the researcher asks a couple of follow-up questions to gather more feedback on their first impressions.
• Questionnaires (MES \& SASSI): Participants complete the questionnaires designed to assess their attention to food, perception of the agent's likability, and other pertinent attributes using the SASSI instrument for evaluating conversational agents.
3.4.3 Participant recruitment
To recruit participants for this study, we sent an invitation email through an institutional student mailing list. We focused on young adults as it was the age group with which we conducted the initial interviews. Therefore, inclusion criteria required candidates to be between 20 and 39 years old and have little or no previous experience in sushi preparation. On the other hand, exclusion criteria were set for individuals with severe food allergies or hearing impairments, as they could not participate in the study due to their condition. The invitation email included a flyer detailing these requirements and encouraging people to learn how to prepare sushi with the conversational agent that was implemented. Interested people had to fill out a form with their contact details and availability regarding dates and times for attending a 1-h session.
3.4.4 Data analysis
The questionnaires employed in the experiment utilize a 7-point Likert scale (completely disagree = 1; completely agree = 7). Each instrument evaluates constructs comprising multiple items, the values of which are subsequently averaged. Following confirmation of a normal distribution in the dataset, a one-tailed t-student test is applied to assess group differences, with a significance level of p < 0.05, to accept the experimental hypotheses. These computations are conducted in Python (version 3.11.3), utilizing the SciPy library (version 1.11.1).1
Additionally, to complement the quantitative data, we provide an open-ended question at the end of the questionnaire to participants to write their comments regarding their experience with the agent. We also consider the comments about their first impressions after the interaction. Since the answers to these questions are short, brief, and optional, they serve primarily to complement the quantitative data gathered during the experiment.
4 Results
The experiment lasted approximately one month, starting the third week of February 2023. Each participant was allocated an hour for the interaction, and separate schedules were arranged for independent kitchenette use on certain days throughout the experiment. All participants were able to prepare a sushi roll following the agent's instructions. However, most chose to eat only a tiny portion of the sushi and took the rest with them to eat later.
4.1 Participants
A total of 40 people participated in the study (see Table 1): 30 women and 10 men. Of the 20 people assigned to the mindful condition, 14 were women, and 6 were men. In the non-mindful condition, 16 participants were women, and 4 were men. A chi-square test for independence showed no difference in the distribution of males and females from both groups (, df = 1, p = 0.715). Regarding the participants' age, the overall average age was 28.52 years, with 29.3 for participants in the mindful condition and 27.75 for the non-mindful condition. A Student's t-test showed no significant difference in the mean age between both conditions (t = 1.4244, p = 0.1642).
Regarding the average duration of interaction with the system, participants in the mindful group took more time (mean = 17:58 min) than in the non-mindful condition (mean = 13:54 min). The mean difference was significant (t = 4.419, p = 0.00008).
4.2 Mindfulness
Table 2 presents the data for mindfulness on participants' behavior during cooking and eating, as assessed by the adapted Mindful Eating Scale (MES) questionnaire. Results indicate that during the cooking phase, participants in the mindful group demonstrated a higher level of Awareness (mean = 6.56) compared to those in the non-mindful group (mean = 5.98). Additionally, the Act with Awareness construct also showed a significant difference, with the mindful group scoring lower (mean = 1.55) than the non-mindful group (mean = 1.95), indicating reduced distraction or a more mindful approach to cooking in the former group. These findings support Hypothesis 1 (H1), confirming that mindfulness guidance increases participants' levels of awareness during food preparation.
The impact of mindfulness extended beyond the cooking process into the eating phase. The Awareness construct during Eating showed a positive difference, with the mindful group scoring 6.48 against the Non-mindful group's 6.19. This difference is statistically significant, suggesting that the mindful practices imparted during the cooking stage had a lasting effect, influencing the participants' awareness while eating. However, the mean difference was not significant for the Act with Awareness construct during eating.
Furthermore, Table 3 presents data more specific for mindfulness during cooking, differentiating between individuals who cook with mindfulness and those who do not. This table shows a series of items focusing on various aspects of Cooking Awareness. Among these, two items stand out due to their differences. The first item, “I noticed flavors and textures when cooking my food,” reveals a marked disparity in the level of sensory engagement between the two groups. Participants' scores were higher (mean = 6.55) in the mindful group than in the non-mindful group (mean = 5.85), indicating a heightened awareness while cooking in the former.
Similarly, a second item, “I noticed the smell and aromas of food while cooking,” also shows a significant difference, with the mindful group again scoring higher (mean = 6.55) than the non-mindful group (mean = 4.95). The other items assessed, such as general awareness of food, attention to food appearance, and ease of concentration on the cooking process, did not show significant differences between the groups. This indicates that while mindfulness boosts specific sensory perceptions (aroma), it does not equally influence other general aspects of cooking awareness. However, the scores for both conditions is high, indicating that regardless of the agent, the fact that the activity was guided and no distractions were present, promoted a focus on the task at hand.
4.3 Hedonic experience
Table 4 provides a detailed assessment of the participant responses to the SASSI questionnaire. A significant finding from this table is the difference in the Likeability construct. The mindful group scored a mean of 6.6278, which is higher compared to the 6.4556 of the non-mindful group. The significance of this difference (t = 1.9762, p-value = 0.0244) supports Hypothesis 2. Conversely, the other constructs measured by the SASSI questionnaire (Response Accuracy, Cognitive Demand, Annoyance, Habitability, and Speed) did not show significant differences between the two groups.
Furthermore, Table 5 offers a more granular look at the Likeability construct, breaking down the participants' responses to various aspects of their experience with the voice agent. The table lists nine items related to the likability of the agent. Among these, two items demonstrate significant differences, offering insights into how the agent was perceived differently in the mindful condition compared to the non-mindful one.
First, in item 3, the mindful group rated the system more friendly (mean = 6.67) than the non-mindful group (mean = 6.2). This mean difference was significant. This result suggests that mindfulness techniques positively influenced how users perceived the friendliness of the voice agent.
Second, also showing a significant difference between the means, the enjoyment of using the system (item 5) is markedly higher in the mindful group (mean = 6.95) compared to the non-mindful group (mean = 6.5). This finding reveals that mindfulness practices significantly improve the overall enjoyment and user satisfaction with the system.
The remaining aspects of Likeability were not statistically different: the system's perceived usefulness, pleasantness, ease of error recovery, clarity in communication, ease of learning, willingness to use, and feeling of control during interaction. Nevertheless, the data from Tables 4, 5 collectively offer valuable insights into the impact of mindfulness techniques on user experience with a conversational agent.
4.4 Participants' comments
Upon concluding their interaction with the system, participants briefly shared their impressions of their overall experience. They were encouraged to openly discuss any aspect of the experiment that resonated with them. Participants commented on three predominant topics: overall impressions, mindfulness and awareness, and recommendations for prototype improvement.
4.4.1 Overall impressions
Both groups described the agent as easy to use, intuitive, interactive, and helpful. However, participants who engaged with the mindful agent also described it as friendly and capable of providing companionship. For instance, a participant from the mindful group mentioned, “With the voice agent, I felt accompanied and secure during [food] preparation.” Similarly, another participant stated, “I quite liked it; it was concise and friendly, and at times, it even brought a smile to my face.” These supplementary remarks help explain why individuals in the mindful group rated the agent higher regarding Likeability. Nevertheless, subjects from the non-mindful group also enjoyed their interaction, albeit to a lesser extent.
Additionally, participants provided feedback on the videos presented by the agent. For instance, one participant from the non-minful group remarked, “The videos were quite good. The video on how to roll, which I think is one of the trickiest parts, was quite accurate for me.” Generally, most comments regarding the videos highlighted their helpfulness and ease of following.
4.4.2 Mindfulness and awareness
According to our participants' comments regarding the use of mindfulness while cooking, we noticed the following: the majority of them enjoyed incorporating this practice and found it to be a relaxing experience. For instance, one participant remarked, “The dynamic of sensing your food was relaxing. During that time I spent on the task, I was able to put a pause in my day.” However, only one participant from this group indicated that she did not consider it necessary as it distracted her from the process.
On the other hand, reinforcing the notion that the mindful agent effectively increased levels of awareness, one participant said, “I think the questions about the smell, color, and texture of the ingredients helped me become conscious of these factors that I would have overlooked otherwise.” This insight highlights the agent's role in facilitating mindfulness during cooking. Furthermore, another participant expressed intentions of integrating this practice into their daily life, stating, “I really liked the aspect of pausing at each step; I'm going to incorporate that into my life.”
4.4.3 Recommendations for prototype improvement
Participants also provided feedback regarding potential enhancements that could be integrated into the prototype. For example, some participants commented on the voice used, suggesting it might benefit from improvement as it occasionally sounded robotic. One participant even expressed a preference for a female voice. Additionally, some participants indicated that the agent provided more explicit instructions regarding recipe proportions in case they use it at home. This was because participants were provided with exact ingredient quantities during the experiment.
On the other hand, a couple of participants from the non-mindful group suggested the idea of incorporating music. For instance, one participant remarked, “If you could tell it while you're cooking to play music, I mean, while you're doing something. To open, like Spotify at the same time as this app, I mean, that's already looking ahead. But it would be cool if it could play [music].” Recalling that participants in the non-mindful group were provided only with basic instructions rather than extended mindful instructions.
5 Discussion
The evaluation of the MyndFood prototype shows promising results, with mindful dialogue enhancing participants' cooking experience and awareness. Participants in the mindful group spent more time interacting with the agent, which was anticipated. Additional instructions for engaging with the mindful agent were important, as they guided participants in savoring their food and prompted users to utilize all their senses fully during meal preparation.
The mindful group particularly enjoyed the instructions more, finding they helped them concentrate on preparing their meals, which aligns with research highlighting mindfulness's positive influence on eating habits and food choices (Jordan et al., 2014). Additionally, mindfulness results indicated that mindful instruction from the system impacts olfactory awareness, enhancing the participants' ability to notice and appreciate the aromas emanating during cooking. These findings are supported by user feedback, with some participants expressing that the mindful instructions helped them relax during the activity and sparked a desire to incorporate similar practices into their daily lives.
Likewise, results regarding hedonic experience indicate that incorporating mindfulness techniques positively influenced participants' overall perception and enjoyment, enhancing the experience's likability. While mindfulness techniques distinctly increased the likability of the user experience, they did not significantly impact other aspects, such as cognitive demand, response accuracy, or speed. This outcome is promising, as it shows that mindful interactions can be incorporated without negatively affecting these performance factors, even with increased user engagement. Ultimately, mindfulness significantly enhances user experience, particularly in terms of perceived friendliness and overall enjoyment. This is further supported by participants' impressions, as some found the agent friendly and noted that its way of delivering instructions occasionally made them smile.
Moreover, participants in the mindfulness group reported a unique sense of companionship with the agent, a feeling not observed in the non-mindful group. This sense of connection suggests that these agents can extend beyond mere informational or task-focused support, providing emotional comfort and fostering a sense of social interaction, as noted in Niewiadomski et al. (2022).
Furthermore, the addition of recipe guidance videos was well-received by participants, suggesting potential for deeper integration of instructional videos across various recipe steps. By incorporating video instructions that guide users through each phase of the cooking process, the system could offer clearer, more supportive assistance, enhancing user confidence and satisfaction. Additionally, based on user feedback, allowing individuals to choose when to engage with mindfulness practices during food preparation could make the system more adaptable and user-friendly. This option would empower users to integrate mindfulness techniques at moments that feel most natural and beneficial to them, potentially fostering a stronger connection to the cooking experience. Incorporating such personalization features, grounded in evidence-based research, could significantly amplify the system's impact and boost user engagement (Kocaballi et al., 2019).
Ultimately, this new perspective on mindful conversational agents allows us to view technology as a beneficial aid in our interaction with food rather than merely a distraction contributing to overeating (Lemke and Schifferstein, 2021; Ogden et al., 2013). The MyndFood prototype has helped us identify ways and activities through which technology can effectively encourage healthier practices, such as mindful cooking and eating while enhancing the overall experience of its users. Finally, the development of MyndFood contributes to the field of Computational Commensality (Niewiadomski et al., 2019) by providing the design and evaluation of a system that enhances the experience of food-related activities, including cooking and eating.
5.1 Limitations
While our study aimed to provide insights into the effectiveness of a mindful conversational agent for food preparation, certain limitations must be acknowledged, which could impact the generalization of our findings. First, the controlled setting may not accurately represent the dynamic and varied nature of cooking in real-world home environments. Additionally, including a broader selection of recipes could help improve the applicability and generalization of the findings. The study was conducted with a relatively homogeneous participant group, which may limit the depth and breadth of the insights obtained.
Further, our study employed the Wizard of Oz technique, wherein a human operator controlled the conversational agent's interaction. While this approach allowed us to simulate interactions effectively, it needs to account for the potential errors of the autonomy of the technology. Certain features, such as full voice navigation and activity tracking, were not implemented in this version of the prototype and were left for future work. As a result, the system serves primarily as a proof of concept rather than a fully developed solution.
Lastly, in terms of qualitative data analysis, we primarily relied on participants' initial impressions and responses to open-ended questions within the questionnaires rather than conducting in-depth interviews. Nevertheless, some research has reported the value of these open-ended questions, as they can yield insights that closed questionnaires might not capture (Etz et al., 2018).
6 Conclusions and future work
The MyndFood prototype was considered helpful and easy to use by the participants. It provided an intuitive design for interacting and navigating through voice during the intervention. The results show that participants who interacted with the mindful conversational agent reported a higher level of awareness during the food experience than those who interacted with the non-mindful agent. Moreover, participants who interacted with the mindful agent also reported a more positive experience when interacting with the voice agent than the non-mindful group. These findings demonstrate how a mindful conversational agent can enhance users' experience in cooking and eating. Furthermore, this study could lay the groundwork for developing more advanced and mindful voice assistants to improve our relationship with food and technology.
For future work, we are developing an autonomous agent to facilitate cooking and eating mindfully within the smart environment. The new prototype incorporates large language models (LLM) and activity recognition to adjust the agent's responses according to the individual's tasks. Future experiments should involve participants from diverse age groups and backgrounds to evaluate the new prototypes in varied contexts. Experience-driven scenarios such as wine tasting sessions may help foster engaging interactions between users and the agent. Additionally, assessing the long-term impact of mindful conversational agents will require extended studies to understand their influence over time.
Data availability statement
The datasets presented in this article are not readily available because no restrictions apply. The dataset consists of anonymized questionnaire responses and is included in the article. Requests to access the datasets should be directed to bWFyaW9wYXJyYUBjaWNlc2UuZWR1Lm14.
Ethics statement
The studies involving humans were conducted in accordance with the local legislation and institutional requirements. The participants provided their written informed consent to participate in this study. Written informed consent was obtained from the individual(s) for the publication of any potentially identifiable images or data included in this article.
Author contributions
MP: Data curation, Formal analysis, Software, Writing – original draft, Writing – review & editing, Investigation. JF: Conceptualization, Supervision, Writing – original draft, Writing – review & editing. LC: Conceptualization, Supervision, Writing – original draft, Writing – review & editing.
Funding
The author(s) declare that financial support was received for the research and/or publication of this article. This work was partially funded by CONAHCYT through a graduate scholarship provided by CONAHCYT to MP. Also, this research was partially funded by the Sonora Institute of Technology (ITSON) through the PROFAPI program.
Conflict of interest
The authors declare that the research was conducted in the absence of any commercial or financial relationships that could be construed as a potential conflict of interest.
Generative AI statement
The author(s) declare that no Gen AI was used in the creation of this manuscript.
Publisher's note
All claims expressed in this article are solely those of the authors and do not necessarily represent those of their affiliated organizations, or those of the publisher, the editors and the reviewers. Any product that may be evaluated in this article, or claim that may be made by its manufacturer, is not guaranteed or endorsed by the publisher.
Footnotes
References
Abras, C., Maloney-Krichmar, D., and Preece, J. (2004). “User-centered design,” in Encyclopedia of Human-Computer Interaction, ed. W. Bainbridge (Thousand Oaks: Sage Publications), 445–456.
Adamopoulou, E., and Moussiades, L. (2020). Chatbots: history, technology, and applications. Mach. Learn. Applic. 2:100006. doi: 10.1016/j.mlwa.2020.100006
Angara, P., Jiménez, M., Agarwal, K., Jain, H., Jain, R., Stege, U., et al. (2017). “Foodie fooderson a conversational agent for the smart kitchen,” in CASCON, 247–253.
Augusto, J. C., Nakashima, H., and Aghajan, H. (2010). “Ambient intelligence and smart environments: a state of the art,” in Handbook of Ambient Intelligence and Smart Environments, 3–31. doi: 10.1007/978-0-387-93808-0_1
Azpíroz-Dorronsoro, C., Fernández-Muñiz, B., Montes-Peón, J. M., and Vázquez-Ordás, C. J. (2023). Technostress and work-family conflict in ict-user employees during the covid-19 pandemic: the role of social support and mindfulness. Behav. Inf. Technol. 43, 1531–1553. doi: 10.1080/0144929X.2023.2220051
Barko-Sherif, S., Elsweiler, D., and Harvey, M. (2020). “Conversational agents for recipe recommendation,” in Proceedings of the 2020 Conference on Human Information Interaction and Retrieval, 73–82. doi: 10.1145/3343413.3377967
Beccia, A. L., Ruf, A., Druker, S., Ludwig, V. U., and Brewer, J. A. (2020). Women's experiences with a mindful eating program for binge and emotional eating: a qualitative investigation into the process of change. J. Altern. Compl. Med. 26, 937–944. doi: 10.1089/acm.2019.0318
Black, D. S., and Slavich, G. M. (2016). Mindfulness meditation and the immune system: a systematic review of randomized controlled trials. Ann. N. Y. Acad. Sci. 1373, 13–24. doi: 10.1111/nyas.12998
Bostock, S., Crosswell, A. D., Prather, A. A., and Steptoe, A. (2019). Mindfulness on-the-go: effects of a mindfulness meditation app on work stress and well-being. J. Occup. Health Psychol. 24:127. doi: 10.1037/ocp0000118
Bruijnes, M., Huisman, G., and Heylen, D. (2016). “Tasty tech: human-food interaction and multimodal interfaces,” in Proceedings of the 1st Workshop on Multi-sensorial Approaches to Human-Food Interaction, 1–6. doi: 10.1145/3007577.3007581
Catania, F., and Garzotto, F. (2023). A conversational agent for emotion expression stimulation in persons with neurodevelopmental disorders. Multimed. Tools Appl. 82, 12797–12828. doi: 10.1007/s11042-022-14135-w
Chan, X. W., Shang, S., Brough, P., Wilkinson, A., and Lu, C.-q. (2023). Work, life and covid-19: a rapid review and practical recommendations for the post-pandemic workplace. Asia Pacific J. Hum. Resour. 61, 257–276. doi: 10.1111/1744-7941.12355
Dow, S., MacIntyre, B., Lee, J., Oezbek, C., Bolter, J. D., and Gandy, M. (2005). Wizard of OZ support throughout an iterative design process. IEEE Perv. Comput. 4, 18–26. doi: 10.1109/MPRV.2005.93
Epstein, D. A., Cordeiro, F., Fogarty, J., Hsieh, G., and Munson, S. A. (2016). “Crumbs: lightweight daily food challenges to promote engagement and mindfulness,” in Proceedings of the 2016 CHI Conference on Human Factors in Computing Systems, 5632–5644. doi: 10.1145/2858036.2858044
Etz, R. S., Gonzalez, M. M., Eden, A. R., and Winship, J. (2018). Rapid sense making: a feasible, efficient approach for analyzing large data sets of open-ended comments. Int. J. Qualit. Methods 17:1609406918765509. doi: 10.1177/1609406918765509
Foley-Fisher, Z., Tsao, V., Wang, J., and Fels, S. (2010). “Netpot: easy meal enjoyment for distant diners,” in Entertainment Computing-ICEC 2010: 9th International Conference, ICEC 2010, Seoul, Korea (Springer), 446–448. doi: 10.1007/978-3-642-15399-0_56
Fuentes Artiles, R., Staub, K., Aldakak, L., Eppenberger, P., Rühli, F., and Bender, N. (2019). Mindful eating and common diet programs lower body weight similarly: systematic review and meta-analysis. Obesity Rev. 20, 1619–1627. doi: 10.1111/obr.12918
Giannopoulou, I., Kotopoulea-Nikolaidi, M., Daskou, S., Martyn, K., and Patel, A. (2020). Mindfulness in eating is inversely related to binge eating and mood disturbances in university students in health-related disciplines. Nutrients 12:396. doi: 10.3390/nu12020396
Godfrey, K. M., Gallo, L. C., and Afari, N. (2015). Mindfulness-based interventions for binge eating: a systematic review and meta-analysis. J. Behav. Med. 38, 348–362. doi: 10.1007/s10865-014-9610-5
Gottardi, A., Ipek, O., Castellucci, G., Hu, S., Vaz, L., Lu, Y., et al. (2022). Alexa, let's work together: introducing the first alexa prize taskbot challenge on conversational task assistance. arXiv:2209.06321.
Hone, K. S., and Graham, R. (2000). Towards a tool for the subjective assessment of speech system interfaces (sassi). Nat. Lang. Eng. 6, 287–303. doi: 10.1017/S1351324900002497
Hoy, M. B. (2018). Alexa, siri, cortana, and more: an introduction to voice assistants. Med. Ref. Serv. Q. 37, 81–88. doi: 10.1080/02763869.2018.1404391
Hulbert-Williams, L., Nicholls, W., Joy, J., and Hulbert-Williams, N. (2014). Initial validation of the mindful eating scale. Mindfulness 5, 719–729. doi: 10.1007/s12671-013-0227-5
Jönsson, H., Michaud, M., and Neuman, N. (2021). What is commensality? A critical discussion of an expanding research field. Int. J. Environ. Res. Public Health 18:6235. doi: 10.3390/ijerph18126235
Jordan, C. H., Wang, W., Donatoni, L., and Meier, B. P. (2014). Mindful eating: trait and state mindfulness predict healthier eating behavior. Pers. Individ. Dif. 68, 107–111. doi: 10.1016/j.paid.2014.04.013
Kabat-Zinn, J. (2003). Mindfulness-based interventions in context: past, present, and future. Clin. Psychol. 10, 144–156. doi: 10.1093/clipsy.bpg016
Khot, R. A., Aggarwal, D., and Pasumarthy, N. (2022). “Understanding screen-based dining practices through the lens of mindful eating,” in Proceedings of the 2022 CHI Conference on Human Factors in Computing Systems, 1–19. doi: 10.1145/3491102.3517651
Khot, R. A., and Mueller, F. (2019). Human-food interaction. Found. Trend Hum. Comput. Inter. 12, 238–415. doi: 10.1561/1100000074
Khot, R. A., Yi, J.-Y., and Aggarwal, D. (2020). “Swan: designing a companion spoon for mindful eating,” in Proceedings of the Fourteenth International Conference on Tangible, Embedded, and Embodied Interaction, 743–756. doi: 10.1145/3374920.3375009
Khoury, B., Lecomte, T., Fortin, G., Masse, M., Therien, P., Bouchard, V., et al. (2013). Mindfulness-based therapy: a comprehensive meta-analysis. Clin. Psychol. Rev. 33, 763–771. doi: 10.1016/j.cpr.2013.05.005
Kocaballi, A. B., Berkovsky, S., Quiroz, J. C., Laranjo, L., Tong, H. L., Rezazadegan, D., et al. (2019). The personalization of conversational agents in health care: systematic review. J. Med. Internet Res. 21:e15360. doi: 10.2196/15360
Kristeller, J. L., and Wolever, R. Q. (2014). Mindfulness-based eating awareness training for treating binge eating disorder: the conceptual foundation. Eating Disor. Mindf. 19, 93–105. doi: 10.1016/B978-0-12-416031-6.00006-2
Kuoppamäki, S., Jaberibraheem, R., Hellstrand, M., and McMillan, D. (2023). Designing multi-modal conversational agents for the kitchen with older adults: a participatory design study. Int. J. Soc. Robot. 15, 1507–1523. doi: 10.1007/s12369-023-01055-4
Lemke, M., and Schifferstein, H. N. (2021). The use of ICT devices as part of the solo eating experience. Appetite 165:105297. doi: 10.1016/j.appet.2021.105297
Li, M., and Wang, R. (2023). Chatbots in e-commerce: the effect of ChatBot language style on customers' continuance usage intention and attitude toward brand. J. Retail. Cons. Serv. 71:103209. doi: 10.1016/j.jretconser.2022.103209
Li, Y., Chung, T. Y., Lu, W., Li, M., Ho, Y. W. B., He, M., et al. (2024). Chatbot-based mindfulness-based stress reduction program for university students with depressive symptoms: intervention development and pilot evaluation. J. Am. Psychiatr. Nurses Assoc. 20:10783903241302092. doi: 10.1177/10783903241302092
Linardon, J. (2023). Rates of attrition and engagement in randomized controlled trials of mindfulness apps: systematic review and meta-analysis. Behav. Res. Ther. 170:104421. doi: 10.1016/j.brat.2023.104421
Liu, L. H., Lu, X., Martinez, R., Wang, D., Liu, F., Monroy-Hernández, A., et al. (2022). “Mindful garden: supporting reflection on biosignals in a co-located augmented reality mindfulness experience,” in Companion Publication of the 2022 Conference on Computer Supported Cooperative Work and Social Computing, 201–204. doi: 10.1145/3500868.3559708
Mani, M., Kavanagh, D. J., Hides, L., and Stoyanov, S. R. (2015). Review and evaluation of mindfulness-based iphone apps. JMIR mHealth uHealth 3:e4328. doi: 10.2196/mhealth.4328
Meegahapola, L., Ruiz-Correa, S., and Gatica-Perez, D. (2020). “Alone or with others? Understanding eating episodes of college students with mobile sensing,” in Proceedings of the 19th International Conference on Mobile and Ubiquitous Multimedia, 162–166. doi: 10.1145/3428361.3428463
Méndez, R., Goto, K., Song, C., Giampaoli, J., Karnik, G., and Wylie, A. (2020). Cultural influence on mindful eating: traditions and values as experienced by mexican-american and non-hispanic white parents of elementary-school children. Glob. Health Promot. 27, 6–14. doi: 10.1177/1757975919878654
Mercado, D., Robinson, L., Gordon, G., Werthmann, J., Campbell, I. C., and Schmidt, U. (2021). The outcomes of mindfulness-based interventions for obesity and binge eating disorder: a meta-analysis of randomised controlled trials. Appetite 166:105464. doi: 10.1016/j.appet.2021.105464
Minker, W., López-Cózar, R., and McTear, M. (2009). The role of spoken language dialogue interaction in intelligent environments. J. Ambient Intell. Smart Environ. 1, 31–36. doi: 10.3233/AIS-2009-0004
Moqbel, M., Alshare, K., Erskine, M. A., and Bartelt, V. (2023). Whatsapp social media addiction and mental health: mindfulness and healing use interventions. Behav. Inf. Technol. 43, 2511–2524. doi: 10.1080/0144929X.2023.2253932
Niewiadomski, R., Bruijnes, M., Huisman, G., Gallagher, C. P., and Mancini, M. (2022). Social robots as eating companions. Front. Comput. Sci. 4:909844. doi: 10.3389/fcomp.2022.909844
Niewiadomski, R., Ceccaldi, E., Huisman, G., Volpe, G., and Mancini, M. (2019). Computational commensality: from theories to computational models for social food preparation and consumption in HCI. Front. Robot. AI 6:119. doi: 10.3389/frobt.2019.00119
Ogden, J., Coop, N., Cousins, C., Crump, R., Field, L., Hughes, S., et al. (2013). Distraction, the desire to eat and food intake. Towards an expanded model of mindless eating. Appetite 62, 119–126. doi: 10.1016/j.appet.2012.11.023
Parra, M. O., Castro, L. A., and Favela, J. (2021). Understanding changes in behavior during the COVID-19 pandemic: opportunities to design around new eating experiences. Avan. Inter. Hum. Comput. 11, 11–17. doi: 10.47756/aihc.y6i1.79
Parra, M. O., Favela, J., Castro, L. A., and Morales, A. (2018). Monitoring eating behaviors for a nutritionist e-assistant using crowdsourcing. Computer 51, 43–51. doi: 10.1109/MC.2018.1731078
Pereira-Castro, M. R., Pinto, A. G., Caixeta, T. R., Monteiro, R. A., Bermúdez, X. P. D., and Mendonça, A. V. M. (2022). Digital forms of commensality in the 21st century: a scoping review. Int. J. Environ. Res. Public Health 19:16734. doi: 10.3390/ijerph192416734
Pergantis, P., Bamicha, V., Skianis, C., and Drigas, A. (2025). AI chatbots and cognitive control: enhancing executive functions through chatbot interactions: a systematic review. Brain Sci. 15:47. doi: 10.3390/brainsci15010047
Prieto, D. C., Lanza-León, P., Moreno, P., Blázquez-Fernández, C., Lera, J., Gonzalez-Diego, F.-J., et al. (2023). Change in eating habits during the Spanish COVID-19 pandemic lockdown: evidence for a sample of university community. PeerJ 11:e14244. doi: 10.7717/peerj.14244
Querstret, D., Morison, L., Dickinson, S., Cropley, M., and John, M. (2020). Mindfulness-based stress reduction and mindfulness-based cognitive therapy for psychological health and well-being in nonclinical samples: a systematic review and meta-analysis. Int. J. Stress Manag. 27:394. doi: 10.1037/str0000165
Ramos, G., Aguilera, A., Montoya, A., Lau, A., Wen, C. Y., Torres, V. C., et al. (2022). App-based mindfulness meditation for people of color who experience race-related stress: protocol for a randomized controlled trial. JMIR Res. Protoc. 11:e35196. doi: 10.2196/35196
Roo, J. S., Gervais, R., Frey, J., and Hachet, M. (2017). “Inner garden: connecting inner states to a mixed reality sandbox for mindfulness,” in Proceedings of the 2017 CHI Conference on Human Factors in Computing Systems, 1459–1470. doi: 10.1145/3025453.3025743
Sarda, B., Delamaire, C., Serry, A.-J., and Ducrot, P. (2022). Changes in home cooking and culinary practices among the French population during the COVID-19 lockdown. Appetite 168:105743. doi: 10.1016/j.appet.2021.105743
Schultchen, D., Terhorst, Y., Holderied, T., Stach, M., Messner, E.-M., Baumeister, H., et al. (2021). Stay present with your phone: a systematic review and standardized rating of mindfulness apps in European app stores. Int. J. Behav. Med. 28, 552–560. doi: 10.1007/s12529-020-09944-y
Spence, C., Mancini, M., and Huisman, G. (2019). Digital commensality: eating and drinking in the company of technology. Front. Psychol. 10:2252. doi: 10.3389/fpsyg.2019.02252
Tudor Car, L., Dhinagaran, D. A., Kyaw, B. M., Kowatsch, T., Joty, S., Theng, Y.-L., et al. (2020). Conversational agents in health care: scoping review and conceptual analysis. J. Med. Internet Res. 22:e17158. doi: 10.2196/17158
Tylka, T. L., Eneli, I. U., Van Diest, A. M. K., and Lumeng, J. C. (2013). Which adaptive maternal eating behaviors predict child feeding practices? An examination with mothers of 2-to 5-year-old children. Eating Behav. 14, 57–63. doi: 10.1016/j.eatbeh.2012.10.014
Wang, Y.-C., Chi, O. H., Saito, H., and Lu, Y. D. (2024). Conversational AI chatbots as counselors for hospitality employees. Int. J. Hospit. Manag. 122:103861. doi: 10.1016/j.ijhm.2024.103861
Wansink, B. (2004). Environmental factors that increase the food intake and consumption volume of unknowing consumers. Annu. Rev. Nutr. 24, 455–479. doi: 10.1146/annurev.nutr.24.012003.132140
Wansink, B., Van Ittersum, K., and Painter, J. E. (2006). Ice cream illusions: bowls, spoons, and self-served portion sizes. Am. J. Prev. Med. 31, 240–243. doi: 10.1016/j.amepre.2006.04.003
Warren, J. M., Smith, N., and Ashwell, M. (2017). A structured literature review on the role of mindfulness, mindful eating and intuitive eating in changing eating behaviours: effectiveness and associated potential mechanisms. Nutr. Res. Rev. 30, 272–283. doi: 10.1017/S0954422417000154
Winkler, R., and Söllner, M. (2018). “Unleashing the potential of chatbots in education: a state-of-the-art analysis,” in Academy of Management Proceedings (Academy of Management Briarcliff Manor, NY), 15903. doi: 10.5465/AMBPP.2018.15903abstract
Wolfson, J. A., and Bleich, S. N. (2015). Is cooking at home associated with better diet quality or weight-loss intention? Public Health Nutr. 18, 1397–1406. doi: 10.1017/S1368980014001943
Zhang, D., Lee, E. K., Mak, E. C., Ho, C., and Wong, S. Y. (2021). Mindfulness-based interventions: an overall review. Br. Med. Bull. 138, 41–57. doi: 10.1093/bmb/ldab005
Zhang, M., Papachristos, E., and Merritt, T. (2023). “Facilitating mindful eating with a voice assistant,” in Proceedings of the 5th International Conference on Conversational User Interfaces, 1–6. doi: 10.1145/3571884.3604311
Keywords: conversational agents, computational commensality, digital commensality, mindful cooking, human-computer interaction
Citation: Parra MO, Favela J and Castro LA (2025) MyndFood: a conversational agent for enhancing the cooking and eating experience. Front. Comput. Sci. 7:1566940. doi: 10.3389/fcomp.2025.1566940
Received: 26 January 2025; Accepted: 10 April 2025;
Published: 30 April 2025.
Edited by:
Jérémie Topin, Université Côte d'Azur, FranceReviewed by:
Pantelis Pergantis, University of the Aegean, GreeceJoyeta Ghosh, Amity University Kolkata, India
Copyright © 2025 Parra, Favela and Castro. This is an open-access article distributed under the terms of the Creative Commons Attribution License (CC BY). The use, distribution or reproduction in other forums is permitted, provided the original author(s) and the copyright owner(s) are credited and that the original publication in this journal is cited, in accordance with accepted academic practice. No use, distribution or reproduction is permitted which does not comply with these terms.
*Correspondence: Luis A. Castro, bHVpcy5jYXN0cm9AYWNtLm9yZw==