- 1Genetics and Bioinformatics Unit, Dasman Diabetes Institute, Kuwait City, Kuwait
- 2Doctoral Program in Population Health, Faculty of Medicine, University of Helsinki, Helsinki, Finland
Despite dedicated nation-wide efforts to raise awareness against the harmful effects of fast-food consumption and sedentary lifestyle, the Arab population continues to struggle with an increased risk for metabolic disorders. Unlike the European population, the Arab population lacks well-established genetic risk determinants for metabolic disorders, and the transferability of established risk loci to this population has not been satisfactorily demonstrated. The most recent findings have identified over 240 genetic risk loci (with ~400 independent association signals) for type 2 diabetes, but thus far only 25 risk loci (ADAMTS9, ALX4, BCL11A, CDKAL1, CDKN2A/B, COL8A1, DUSP9, FTO, GCK, GNPDA2, HMG20A, HNF1A, HNF1B, HNF4A, IGF2BP2, JAZF1, KCNJ11, KCNQ1, MC4R, PPARγ, SLC30A8, TCF7L2, TFAP2B, TP53INP1, and WFS1) have been replicated in Arab populations. To our knowledge, large-scale population- or family-based association studies are non-existent in this region. Recently, we conducted genome-wide association studies on Arab individuals from Kuwait to delineate the genetic determinants for quantitative traits associated with anthropometry, lipid profile, insulin resistance, and blood pressure levels. Although these studies led to the identification of novel recessive variants, they failed to reproduce the established loci. However, they provided insights into the genetic architecture of the population, the applicability of genetic models based on recessive mode of inheritance, the presence of genetic signatures of inbreeding due to the practice of consanguinity, and the pleiotropic effects of rare disorders on complex metabolic disorders. This perspective presents analysis strategies and study designs for identifying genetic risk variants associated with diabetes and related traits in Arab populations.
Background
Discovery of oil reserves in the Arabian Gulf since the 1930s increased overall wealth in these countries. Rapid socioeconomic transitions in Arab countries in the rich post-oil era marked changes in the nutritional patterns and food habits, including a shift from locally grown natural products to a Western diet and change from nomadic way to urbanized life. These resulted in an increasingly sedentary lifestyle and wide-spread obesity (1–3) and an increased prevalence of diabetes (4, 5), and metabolic syndrome (6) in the last few decades. Diabetes is sweeping through Middle East; as per the International Diabetes Federation Atlas 8th Edition for 2017, the age-adjusted comparative prevalence of diabetes (18–99 years) in the Middle East and North Africa region is 10.5%, which is the second highest after the North America and Caribbean region. Up to 30% of native adult hospital visitors in Kuwait are afflicted with T2DM (4). The prevalence among adults in the countries from the Peninsula (Kingdom of Saudi Arabia 31.6%, Oman 29%, Kuwait 25.4%, Bahrain 25.0%, and United Arab Emirates 25.0%) are significantly associated with high per capita GDP (gross domestic product) and energy consumption (7). T2DM results from a complex interplay of adverse lifestyle exposures and genetic predisposition. Despite the high prevalence of T2DM, the Arab population lacks convincingly determined T2DM genetic risk variants and reports that sufficiently demonstrate the replication of established risk variants.
Identified T2DM Risk Loci in Arab Population Compared With Established Gene Loci
Since the advent of genome-wide association (GWA) studies, many T2DM risk loci have been globally identified, which concentrated mainly on the European and Asian populations. A recent study by Mahajan et al. (8), that combined data from 32 European-descent GWAS imputed to high-density reference panels, identified 243 genome-wide significant T2D-risk loci with 403 independent association signals. Therefore, it is important to understand the relevance and performance of these established loci in the Arab population and how often such novel risk loci are identified in this population. Published reports on T2DM genetics in the Arab population originate from Kuwait, Lebanon, Saudi Arabia, Qatar, UAE, Oman, and Tunisia (Table 1). Some of these studies illustrate certain overlaps with established T2DM risk loci, some illustrate the complete absence of any overlap (Table 1), and some identify novel risk loci. Approximately 25 established T2DM loci (ADAMTS9, ALX4, BCL11A, CDKAL1, CDKN2A/B, COL8A1, DUSP9, FTO, GCK, GNPDA2, HMG20A, HNF1A, HNF1B, HNF4A, IGF2BP2, JAZF1, KCNJ11, KCNQ1, MC4R, PPARγ, SLC30A8, TCF7L2, TFAP2B, TP53INP1, and WFS1) and few established obesity loci (ADIPOQ, FTO, RFX7, and USP37) are observed to replicate in the Arab population (Table 1). The replicated T2DM risk loci fall into three categories (Table S1): (i) those that impact the T2DM risk through impaired β-cell function (ALX4, BCL11A, CDKAL1, CDKN2A/B, GCK, HNF1A, HNF1B, HNF4A, IGF2BP2, JAZF1, KCNJ11, KCNQ1, SLC30A8, TCF7L2, WFS1); (ii) those that act through modulating insulin action (ADAMTS9, DUSP9, PPARγ); and (iii) those that were primarily associated with BMI, obesity and adiposity but subsequently identified also as affecting T2DM risk through insulin action (FTO, GNPDA2, MC4R, TFAP2B).
Studies illustrating a replication of established risk loci in Arab population are generally based on targeted genotyping, whereas those failing to observe the established loci are GWA-based. GWA studies for quantitative traits in the Arab population in Kuwait were unsuccessful to demonstrate established loci at genome-wide significance but instead led to identification of novel risk loci (Table 2); these identified novel loci are often reported in literature as associated with biological processes relating to the T2D traits (Table S2). Moreover, even at nominal p-values, very few established markers are identified in the data sets in the Kuwaiti population (Table 2—Items V–VI). The exemplary susceptibility gene loci namely, PPARγ, KCNJ11, TCF7L2, SLC30A, ABCC8, HHEX, CDKN2A, IGF2BP2, CDKAL1, and FTO, known to be well-replicated in other ethnic population groups were not identified in our studies on the Kuwaiti population. Similarly, only two established T2DM risk gene loci namely (CDKAL1 and TCF7L2) were identified using an imputed data set of 5,000,000 single nucleotide polymorphisms (SNP) in the Lebanese population, which has a higher affinity to European populations compared with other Middle Eastern populations (21). It was also observed that the established markers do not necessarily replicate among inter-Arabic population groups. For example, Mtiraoui et al. (17) (Table 1) illustrated differences between the Levant Arabs (from Lebanon) and north African Arabs (from Tunisia) by demonstrating the association of TCF7L2 in both groups, IGF2BP2 and PPARγ exclusively in the Lebanese group, and KCNJ11 and SLC30A8 exclusively in the Tunisian group.
The partial overlap of established markers and differential replication of established markers in inter-Arabic populations along with the identification of novel loci are also observed in case of other complex disorders, such as rheumatoid arthritis, myocardial infarction/coronary artery disease, prostate cancer, and breast cancer (37–40). It is highly interesting to perform genetic association studies in ethnic populations such as Arabs, not only to re-confirm findings from other ethnic groups but since they may also lead to identification of novel T2DM risk loci.
Possible Causes for Observed Differences in the Risk Loci Profiles
Study Cohorts
The above-mentioned observations in Arab populations are associated with the size of study cohorts used in the studies, which is considerably lower than those used conventionally in global GWA studies (Table 1). This deficit affects the strength of the studies and leads to issues such as non-consideration of markers that have become rare in Arab populations. Other shortcomings of the study designs are inconsistencies (such as in age) between case and control cohorts and the failure to include in the set of markers tested for replication those that are in LD with established markers.
Differences in Phenotype Profiles Between Arab and Global Populations
While most of the well-characterized T2DM genes appear to be associated with β-cell dysfunction, diabetes observed in the Arab population is supposedly associated with obesity. This is underlined by the following observations in the Arab population: the prevalence of obesity in T2DM patients is high (41), Arab studies often find obesity-related genetic loci (e.g., ADIPOQ gene) to contribute to the genetic risk of T2DM in Arab populations (10, 23, 42, 43), and established T2DM-related SNPs are seen associated with obesity phenotypes in Arabs (44).
High Rate of Consanguinity and Prevalence of Rare, Mendelian, and Familial Disorders in Arab Populations
Marriages in Arab populations are traditionally often consanguineous (45, 46). An increased risk of T2DM has been observed among the offspring of such consanguineous marriages in Saudi Arabia and Qatar (47, 48). The familial clustering of T2DM has been reported in Arab populations from Morocco (49), Tunisia (50), Oman (51), and Qatar (52). Additionally, Arab populations exhibit many rare, Mendelian, and familial genetic disorders. Blair et al. (53) identified thousands of associations between Mendelian and complex diseases in the medical records of over 110 million patients from USA and revealed a non-degenerate, phenotypic code that links a complex disorder to a unique collection of Mendelian loci. Using GWA studies, they further demonstrated that common variants associated with complex diseases are enriched in the genes indicated by this “Mendelian code.” Thalassemia, cystic fibrosis, Huntington's disease, and Friedreich's ataxia are examples of rare disorders that increase patient's pre-disposition to diabetes (54–58). Examples of T2DM risk genetic loci, which are also associated with rare recessive disorders, are LIPC (Hepatic lipase deficiency), PDX1 (Pancreatic agedness 1), ENPP1 (Hypophosphatemic rickets, also associated with obesity), WFS1 (Wolfram syndrome 1), and SLC2A2 (Fanconi-Bickel syndrome). Table 2 summarizes OMIM disease annotations, from the OMIM (Online Mendelian Inheritance in Man) catalog of human genes and genetic disorders (59), for risk loci that were identified for metabolic traits in Kuwaiti Arab populations. The genetic locus OTX2-AS1 associated with hypogonadism is particularly interesting as literature reports have provided hints regarding the connection between hypogonadism and T2DM in Arab consanguineous families (60, 61). Al Hayek et al. reported that 36.5% men with T2DM from Jordan had low serum testosterone levels; 17% of such T2DM patients with low serum testosterone levels had primary hypogonadism, whereas the remaining had secondary hypogonadism (62). A study based on Saudi Arabian population revealed a significantly higher positive family history of schizophrenia in patients with first or second cousin parents (63). An increased prevalence of T2DM has been observed in patients with schizophrenia (SCZ); the co-occurrence of SCZ and T2DM may partly be driven by shared genetic factors (64) such as cell adhesion molecules (65, 66). Furthermore, the QDiabetes-2018 model (used within the UK National Health Service) (67) now includes schizophrenia, learning disabilities, use of atypical antipsychotics, treated hypertension, and polycystic ovary syndromes as additional risk factors.
Analysis Strategies for GWA Studies in Arab Populations
The current analysis strategies for GWA studies in Arab populations and suggested extensions are presented in Figure 1 and are discussed below.
Participant Recruitment and Sample Size
The sample size has a linear relationship with the number of identified risk loci; a plateau has not been observed for any trait to date (68). Recruiting the required large number of participants from the intended sample population continues to be challenging in Arab countries (69, 70). Clinical research in Arab countries experiences a lack of public outreach capabilities and coordination between research institutes and hospitals or Ministries of Health. This challenge can be circumvented by understanding public perception and attitude toward medical research and by seeking out means to increase public trust and awareness of clinical research in the Arab population.
The optimum sample size is determined by various factors including homogeneity seen in the population, prevalence of the disorder, variance in the trait measurements, genetic models used in the association tests, number of markers tested in the study, allele frequencies of the risk variants, effect sizes, genome control inflation rates, desired Type I error rates, type of study design (quantitative trait association, case-control studies with unrelated individuals or family-based trios, or sibling case-control designs) (71, 72). A number of tools are available to calculate the optimum sample size; some of the tools often used include Genetic Association Study (GAS) Power Calculator for one-stage genetic association studies http://csg.sph.umich.edu/abecasis/cats/gas_power_calculator/; (73), CaTS Power calculator http://csg.sph.umich.edu//abecasis/CaTS/index.html; (74) for two stage genome wide association studies; Quanto for various study designs (including the matched case-control, case-sibling, case-parent, and case-only designs) http://biostats.usc.edu/Quanto.html; (75), and QpowR for two-stage study design with unrelated individuals https://msu.edu/~steibelj/JP_files/QpowR.html.
Differences in the Extent of Inbreeding Among Subgroups in the Arab Population
The rate of consanguinity and the extent of resultant inbreeding differ among Arab countries as well within a single country. For example, among the three genetic substructures of Kuwaiti Arab population (76–79), the subgroups of Saudi Arabian tribe ancestry and Persian ancestry exhibit high inbreeding coefficients (0.04226 and 0.025742, respectively) indicating endogamy, whereas the nomadic Bedouin subgroup exhibits lower inbreeding coefficient (0.00274), indicating heterogamy (76). Similar observations have been made with population substructures in Qatar (80). The genetic heterogeneity between the subgroups warrants subgroup-specific genetic association analysis using large sample sizes, particularly for those with higher rates of inbreeding.
Comorbid Conditions as Confounders
Diabetes is often comorbid with other complex chronic disorders (81); Most prevalent comorbid disorders, that occur in “concordant” form wherein both disorders represent parts of the overall identical pathophysiological risk profile, include hypertension, coronary artery disease and peripheral vascular disease (82). An example for comorbid disorders being influenced by unique environmental factors is an increased risk for diabetes in patients with learning disabilities, physical or sensory disabilities, and mental health problems. The co-occurrence of schizophrenia and diabetes may partly be driven by shared genetic factors (64). Comorbidities can also be consequences of hyperglycemia. Tests for genetic associations need to consider comorbid conditions. For advancements in metabolic disorder research in the Arabian Peninsula, the identification of overlaps between metabolic disorders and rare genetic as well as common disorders from existing literature and the formation of a catalog of causal factors/markers for overlapping traits are immediately required. A careful and extensive phenotyping in relation to rare and less frequent disorders must be prioritized during sampling. These variables can be further used as covariates in association tests.
Genetic Models Based on Recessive Mode of Inheritance for Association Tests
Consanguinity in successive generations cumulatively increases inbreeding levels, recessive alleles, and the proportion of homozygous gene regions in Arab populations (76, 83). An overwhelming proportion (63%) of the disorders documented in the Catalog for Transmission Genetics in Arabs (CTGA) (84) follows a recessive mode of inheritance. Therefore, it is not surprising to observe that the risk variants reported in our studies appeared when the genetic model based on a recessive mode of inheritance was used (Table 2), and majority of the risk variants were observed to be harbored in the runs of homozygosity (ROH) segments (24). However, when established markers appeared in the Kuwaiti population data sets, it was invariably when the association tests used additive models. It is obvious that genetic pre-disposition of T2DM is not a recessive disorder, although in certain populations homozygosity of susceptibility alleles can further increase the population prevalence of the disease. It is recommended that population genetics in the Arab region use recessive models in addition to additive models, especially when large number of homozygous segments and/or of recessive risk genotypes are observed in sufficiently large number of individuals in the study cohort.
Estimation of Acceptable Effect Size
Large sample sizes enable the identification of causal variants with small effect size in GWA studies. Although the estimation of sample sizes for a 2-stage design (discovery phase followed by replication) is generally strictly followed in GWA studies, the estimation of a true effect size explainable by sample size is not strictly followed in several studies; the proper selection of associated variants in the discovery phase is not usually difficult, mainly because the use of additive models (wherein both heterozygote + rare homozygous genotypes are tested against reference homozygous genotypes with additive effect) does not unusually inflate the effect size values for common markers. However, in case of the recessive model, variants that show ≥5% frequency (i.e., associated with few rare homozygous genotypes) can show unusually large effect sizes. Therefore, an estimation of the acceptable effect size for a desired percentage of variance in a given trait (mean ± SD) and sample size at 80% power is pivotal for restricting SNP associations from undesirable high effect size and inappropriate p-values resulting from recessive effect.
Joint Analysis—Combining Results From Discovery and Replication Phases
GWA studies follow a 2-stage design irrespective of addressing quantitative or binary trait association. A strategy involving the joint analysis of data from both stages, which is currently increasingly used, has been advocated to result in an increased capability of detecting genetic associations (74). Such a meta-analysis increases the capacity for detecting weak genotypic effects. An example of the resultant increased power is observed in the case of three established markers from CETP (rs3764261, rs1864163, and rs1800775), which do not attain genome-wide significance in discovery cohort of our studies but in joint analysis (Table 2—Item V).
Heteroscedasticity and Trait Transformation
Quantitative traits (associated with complex disorders) used in association studies often violate the assumption of normality. Methods commonly used to handle non-normal traits include natural logarithm and rank-based inverse-normal transformations (85). Although the merits of such transformations are questionable (86), their use has been increasing. Elaborate assessments regarding the extent to which such transformations mask the true phenotype variability are lacking in literature. Owing to high inbreeding and prevalence of autosomal recessive disorders in the Arab population (87), the segregation of rare homozygous alleles may exert relatively larger effects on quantitative trait variations. Hence, earmarking any outlying data dispersion as heteroscedastic may conceptually be incorrect. Furthermore, performing trait transformations may adversely mask the actual effects of such loci on the variation of quantitative traits. Thus, to avoid any false positive or negative associations, it is advised to perform association tests, using appropriate genetic models, with both the raw and transformed traits, and to simultaneously adjust the models for disorders (rare and common) that overlap with the metabolic syndrome.
Relatedness and Loss of Samples
The imprecise modeling of genetic relatedness and population stratification among study subjects results in a substantial inflation of test statistics and spurious associations. Moreover, randomized sample sets in the Arab population exhibit rich relatedness due to the prevalent practices of polygamy and consanguinity. Therefore, detailed quality control procedures for relatedness and admixture must be performed in case of Arab studies. Relevantly, our studies are performed using the following steps: (i) assessment of relatedness among participants to the extent of third-degree relatives and removal of one sample per pair of related participants, (ii) performing ancestry estimations using ADMIXTURE (88) and removal of samples with abnormal deviations to the extent of component ancestry elements that have been previously established for the three Kuwaiti population subgroups (76), and (iii) delineation of principal components using EIGENSTRAT (89) and removal of outlying samples. These exhaustive steps, aimed at reducing false positive findings, lead to huge loss of samples. Thus, although the use of unrelated individuals in GWA studies is a norm, the use of recent sophisticated algorithms [such as BLOT-LMM (90) FaST-LMM (91), EMMAX (92)], which account for kinship structures and ancestries within a sample set, may offer larger power to the study by retaining more samples.
Quantitative Trait Association Studies Using “All” Diabetic Cohort
Quantitative trait association studies are usually conducted in population-based cohorts comprising both people with diabetes and diabetes-free individuals at the time of participant recruitment. Association tests are usually adjusted for obesity, diabetes, and medication regimen. However, it is important to extend the studies to cohorts comprising entirely of diabetic or prediabetic participants so that the prospects for use of identified genetic determinants in diabetes care and treatment become promising (93).
Case for Whole-Genome Homozygosity Association (WGHA) Methodologies
High inbreeding within the Arab population renders it a promising repository for providing a large scope for discovery of ROH and segments of identity by descent (IBD). ROH indicates an ancient shared common ancestry, whereas IBD indicates a recent ancestry. Both traits are reportedly effective in delineating population demography and recessive components of Mendelian and complex phenotypes (76). The risk loci identified in our studies often overlay ROH regions, some of which are “novel.” Currently, a promising new concept of “whole-genome homozygosity association (WGHA) methodology” in identifying genetic susceptibility loci harboring recessive variants (94) is being developed. Exemplary works include the identification of “risk ROH” for schizophrenia (95) and adult height (96). Tools such as LOHAS (97), which use either whole-genome sequence or genotype data in cohorts of either related or unrelated individuals, are now available for performing WGH association tests under the study designs for both case–control and quantitative trait association tests.
Case for Family-Based Genetic Association Studies in Arab Population
The large number of T2DM genetic loci identified to date using unrelated people explains only a relatively small proportion of observed heritability (familial clustering) of T2DM (8, 98). Possible explanations for “missing heritability” may originate from the role of rare variants, copy number variants, indels and more complex rearrangements, gene-environment interactions and epigenetics (98–102). Family-based designs allow the segregation of rare variants in a pedigree; multiple copies of such rare variants facilitate the detection of their effects. Family-based studies require a fewer number of samples than population-based studies and offer advantages in terms of quality control, robustness to population stratification, and uniformity in exposure to environmental factors or lineage-specific diseases. They offer the potential to combine linkage and association data. Arab population, which is largely consanguineous, offers a large potential for family-based designs as the population can show familial gene clustering for diabetes and metabolic traits. However, except for few studies, such as the “Oman Family Study” (103–106) and the study on an extended family from the UAE by Al Safar et al. (22), no notable familial study for diabetes risk loci has been reported on the Arab population. Both the abovementioned studies confirmed well-established gene loci, but failed to identify any novel “rare” variants. Considerable attention needs to be paid to appropriate study designs as family data continue to provide important information in the search for trait loci (107). It is ideal if the recruitment of large-pedigrees/extended families, particularly those containing several sub-families suitable for both parent-offspring design or for sibling design, with high inbreeding and roots traceable up to at least six generations with deduced consanguinity data is possible.
Epigenetic Mechanisms of T2DM Genetic Risk Factors and Environmental Factors in Arab Population
As mentioned earlier, the post-oil era witnessed in Arab population a rapid shift in the eating and physical activity habits. Environmental and lifestyle factors (including diet, obesity, physical activity, tobacco smoking and environmental pollutants) can influence epigenetic mechanisms, such as DNA methylation, histone acetylation, and microRNA expression; these modifications can result in altered gene expression with effects on regulation of specific genes. Epigenome-wide association studies (EWASs) that examine the role of epigenetic modifications in the etiology and progression of metabolic disorders (108–112) and diabetes (113–116) have recently emerged. Most of such EWASs with T2D and obesity are focused on Caucasian populations; however, a study emerged recently on Arab population from Qatar (117), which identified one novel CpG association at DQX1 at genome-wide significance for T2D and replicated eight previously reported associations involving TXNIP for T2D and SOCS3, SREBF1, SBNO2, CPT1A, PRR5L, LY6G6E, and an intergenic region on chromosome 17 for BMI albeit at suggestive p-values.
An Integrative Approach as Direction of Future T2DM Genetics Research in Arab Populations
It has increasingly become evident that epigenetics, genetics, and environment are likely to interact with one another to define an individual's risk of diabetes and obesity (118). Integration of data on expression quantitative trait loci (eQTL), which represent regulatory loci, with genetic variants identified from GWA studies can give new insights into identification of causal genes for T2DM (119, 120). The ability of epigenetic modifications and expression of miRNA (and largely the non-coding RNAs) to manipulate gene expression has enabled incorporation of such data in research on pathogenesis of T2DM (121–123). Consideration of expression data and epigenome data along with large-scale GWA data on genotyped and imputed SNPs and copy number variations in association studies for T2DM is depicted as future directions for diabetes research (Figure 2).
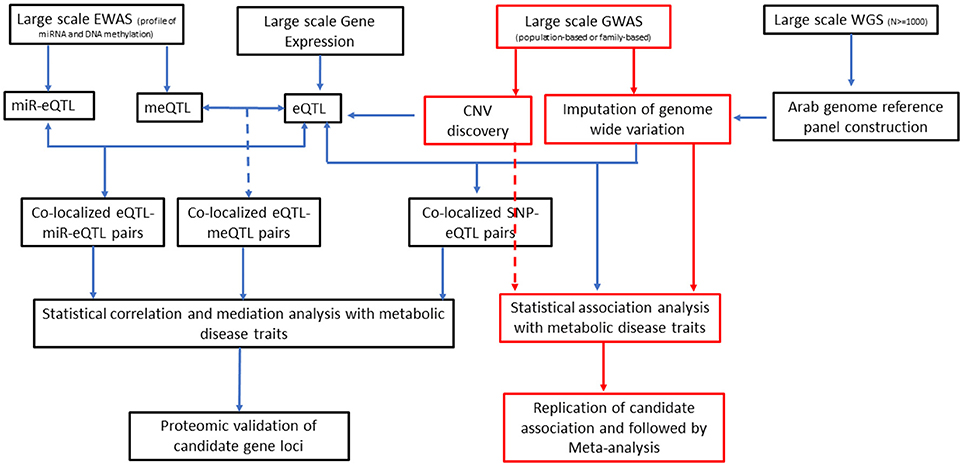
Figure 2. An integrative approach as direction of future T2DM genetics research in Arab populations. EWAS, epigenome-wide association studies; GWAS, genome-wide association studies; WGS, whole-genome sequencing; miR-Eqtl, microRNA expression quantitative trait loci; meQTL, methylation quantitative trait loci; eQTL, expression quantitative trait loci; CNV, copy number variation; SNP, single nucleotide polymorphism.
Conclusion
The failure to convincingly replicate a large number of Euro-centric risk variants for T2DM in Arab populations may have resulted from several aspects, including study design and strength, low prevalence of causative Euro-centric risk variants in the Arab population, or from the gene–environment interactions that masked the effect of the Euro-centric risk variants. However, epidemiological studies have illustrated the deficit of global risk assessment tools fitted to the Arab population (124). The performance of global genetic risk assessment tools (based on Euro-centric markers) in other populations is also questionable (125). The discrepancy of marker relevance in the applicability of Euro-centric genetic risk variants to Arab population could be resolved by performing large-scale genome-wide surveys (a combination of GWAS, exome, and genome sequencing and imputation) of the Arab population with diabetes. Detailed functional assessments of loci identified in the Arab population to interact with Euro-centric risk loci as part of common gene networks or physiologic processes should also be performed.
Author Contributions
TT and FA-M conceptualized the study design. TT and PH developed the manuscript. All the authors participated in discussions. JA, MA-F, JT, and FA-M critically reviewed the manuscript.
Funding
The study was funded by the Kuwait Foundation for Advancement of the Sciences (KFAS) (Dasman Diabetes Institute project number RA 2016-026).
Conflict of Interest Statement
The authors declare that the research was conducted in the absence of any commercial or financial relationships that could be construed as a potential conflict of interest.
Supplementary Material
The Supplementary Material for this article can be found online at: https://www.frontiersin.org/articles/10.3389/fendo.2019.00008/full#supplementary-material
References
2. Badran M, Laher I. Obesity in arabic-speaking countries. J Obes. (2011) 2011:686430. doi: 10.1155/2011/686430
3. Ng M, Fleming T, Robinson M, Thomson B, Graetz N, Margono C, et al. Global, regional, and national prevalence of overweight and obesity in children and adults during 1980–2013: a systematic analysis for the Global Burden of Disease Study 2013. Lancet (2014) 384:766–81. doi: 10.1016/S0140-6736(14)60460-8
4. Channanath AM, Farran B, Behbehani K, Thanaraj TA. State of diabetes, hypertension, and comorbidity in Kuwait: showcasing the trends as seen in native versus expatriate populations. Diabetes Care (2013) 36:e75. doi: 10.2337/dc12-2451
5. Klautzer L, Becker JS, Mattke S. The curse of wealth - Middle Eastern countries need to address the rapidly rising burden of diabetes. Int J Health Pol Manag. (2014) 2:109–14. doi: 10.15171/ijhpm.2014.33
6. Ansarimoghaddam A, Adineh HA, Zareban I, Iranpour S, HosseinZadeh A, Kh F. Prevalence of metabolic syndrome in Middle-East countries: meta-analysis of cross-sectional studies. Diabet Metabol Syndr. (2018) 12:195–201. doi: 10.1016/j.dsx.2017.11.004
7. Meo SA, Usmani AM, Qalbani E. Prevalence of type 2 diabetes in the Arab world: impact of GDP and energy consumption. Eur Rev Med Pharmacol Sci. (2017) 21:1303–12.
8. Mahajan A, Taliun D, Thurner M, Robertson NR, Torres JM, Rayner NW, et al. Fine-mapping type 2 diabetes loci to single-variant resolution using high-density imputation and islet-specific epigenome maps. Nat Genet. (2018) 50:1505–13. doi: 10.1038/s41588-018-0241-6
9. Li-Gao R, Wakil SM, Meyer BF, Dzimiri N, Mook-Kanamori DO. Replication of Type 2 diabetes-associated variants in a Saudi Arabian population. Physiol Genom. (2018) 50:296–7. doi: 10.1152/physiolgenomics.00100.2017
10. Osman W, Tay GK, Alsafar H. Multiple genetic variations confer risks for obesity and type 2 diabetes mellitus in arab descendants from UAE. Int J Obes. (2018) 42:1345–53. doi: 10.1038/s41366-018-0057-6
11. Cauchi S, Ezzidi I, El Achhab Y, Mtiraoui N, Chaieb L, Salah D, et al. European genetic variants associated with type 2 diabetes in North African Arabs. Diab Metabol. (2012) 38:316–23. doi: 10.1016/j.diabet.2012.02.003
12. O'Beirne SL, Salit J, Rodriguez-Flores JL, Staudt MR, Abi Khalil C, Fakhro KA, et al. Type 2 diabetes risk allele loci in the qatari population. PLoS ONE (2016) 11:e0156834. doi: 10.1371/journal.pone.0156834
13. Al-Daghri NM, Alkharfy KM, Alokail MS, Alenad AM, Al-Attas OS, Mohammed AK, et al. Assessing the contribution of 38 genetic loci to the risk of type 2 diabetes in the Saudi Arabian population. Clin Endocrinol. (2014) 80:532–7. doi: 10.1111/cen.12187
14. Tomei S, Mamtani R, Al Ali R, Elkum N, Abdulmalik M, Ismail A, et al. Obesity susceptibility loci in Qataris, a highly consanguineous Arabian population. J Transl Med. (2015) 13:119. doi: 10.1186/s12967-015-0459-3
15. Almawi WY, Nemr R, Keleshian SH, Echtay A, Saldanha FL, AlDoseri FA, et al. A replication study of 19 GWAS-validated type 2 diabetes at-risk variants in the Lebanese population. Diabet Res Clin Prac. (2013) 102:117–22. doi: 10.1016/j.diabres.2013.09.001
16. Al-Sinani S, Woodhouse N, Al-Mamari A, Al-Shafie O, Al-Shafaee M, Al-Yahyaee S, et al. Association of gene variants with susceptibility to type 2 diabetes among Omanis. World J Diabet. (2015) 6:358–66. doi: 10.4239/wjd.v6.i2.358
17. Mtiraoui N, Turki A, Nemr R, Echtay A, Izzidi I, Al-Zaben GSN, et al. Contribution of common variants of ENPP1, IGF2BP2, KCNJ11, MLXIPL, PPARgamma, SLC30A8 and TCF7L2 to the risk of type 2 diabetes in Lebanese and Tunisian Arabs. Diab Metabol. (2012) 38:444–9. doi: 10.1016/j.diabet.2012.05.002
18. El Hajj Chehadeh SW, Jelinek HF, Al Mahmeed WA, Tay GK, Odama UO, Elghazali GE, et al. Relationship between MTHFR C677T and A1298C gene polymorphisms and complications of type 2 diabetes mellitus in an Emirati population. Meta Gene (2016) 9:70–5. doi: 10.1016/j.mgene.2016.04.002
19. Al-Safar H, Hassoun A, Almazrouei S, Kamal W, Afandi B, Rais N. Association of the Genetic polymorphisms in transcription factor 7-Like 2 and peroxisome proliferator-activated receptors- gamma 2 with Type 2 diabetes mellitus and is interaction with obesity status in emirati population. J Diab Res. (2015) 2015:129695. doi: 10.1155/2015/129695
20. Khan SM, El Hajj Chehadeh S, Abdulrahman M, Osman W, Al Safar H. Establishing a genetic link between FTO and VDR gene polymorphisms and obesity in the Emirati population. BMC Med Genet. (2018) 19:11. doi: 10.1186/s12881-018-0522-z
21. Ghassibe-Sabbagh M, Haber M, Salloum AK, Al-Sarraj Y, Akle Y, Hirbli K. J., et al. T2DM GWAS in the Lebanese population confirms the role of TCF7L2 and CDKAL1 in disease susceptibility. Sci Rep. (2014) 4:7351. doi: 10.1038/srep07351
22. Al Safar HS, Cordell HJ, Jafer O, Anderson D, Jamieson SE, Fakiola M, et al. A genome-wide search for type 2 diabetes susceptibility genes in an extended Arab family. Ann Hum Genet (2013) 77:488–503. doi: 10.1111/ahg.12036
23. Zadjali F, Al-Yahyaee S, Hassan MO, Albarwani S, Bayoumi RA. Association of adiponectin promoter variants with traits and clusters of metabolic syndrome in Arabs: family-based study. Gene (2013) 527:663–9. doi: 10.1016/j.gene.2013.06.057
24. Hebbar P, Nizam R, Melhem M, Alkayal F, Elkum N, John SE, et al. Genome-wide association study identifies novel recessive genetic variants for high TGs in an Arab population. J Lipid Res. (2018). doi: 10.1194/jlr.P080218
25. Hebbar P, Alkayal F, Nizam R, Melhem M, Elkum N, John SE, et al. The TCN2 variant of rs9606756 [Ile23Val] acts as risk loci for obesity-related traits and mediates by interacting with Apo-A1. Obesity (2017) 25:1098–108. doi: 10.1002/oby.21826
26. Hebbar P, Elkum N, Alkayal F, John SE, Thanaraj TA, Alsmadi O. Genetic risk variants for metabolic traits in Arab populations. Sci Rep. (2017) 7:40988. doi: 10.1038/srep40988
27. Alsmadi O, Melhem M, Hebbar P, Thareja G, John SE, Alkayal F, et al. Leptin in association with common variants of MC3R mediates hypertension. Am J Hyperten. (2014) 27:973–81. doi: 10.1093/ajh/hpt285
28. Soliman AT, De Sanctis V, Elalaily R, Yassin M. Insulin-like growth factor- I and factors affecting it in thalassemia major. Indian J Endocrinol Metabol. (2015) 19:245–51. doi: 10.4103/2230-8210.131750
29. Willer CJ, Schmidt EM, Sengupta S, Peloso GM, Gustafsson S, Kanoni S, et al., Discovery and refinement of loci associated with lipid levels. Nat Genet. (2013) 45:1274–83. doi: 10.1038/ng.2797
30. Spracklen CN, Chen P, Kim YJ, Wang X, Cai H, Li S, et al. Association analyses of East Asian individuals and trans-ancestry analyses with European individuals reveal new loci associated with cholesterol and triglyceride levels. Hum Mol Genet. (2017) 26:1770–84. doi: 10.1093/hmg/ddx062
31. Willer CJ, Sanna S, Jackson AU, Scuteri A, Bonnycastle LL, Clarke R, et al. Newly identified loci that influence lipid concentrations and risk of coronary artery disease. Nat Genet. (2008) 40:161–9. doi: 10.1038/ng.76
32. Ridker PM, Pare G, Parker AN, Zee RY, Miletich JP, Chasman DI. Polymorphism in the CETP gene region, HDL cholesterol, and risk of future myocardial infarction: genomewide analysis among 18 245 initially healthy women from the Women's Genome Health Study. Circul Cardiovasc Genet. (2009) 2:26–33. doi: 10.1161/CIRCGENETICS.108.817304
33. Dupuis J, Langenberg C, Prokopenko I, Saxena R, Soranzo N, Jackson AU, et al., New genetic loci implicated in fasting glucose homeostasis and their impact on type 2 diabetes risk. Nat Genet. (2010) 42:105–16. doi: 10.1038/ng.520
34. Saxena R, Hivert MF, Langenberg C, Tanaka T, Pankow JS, Vollenweider P, et al., Genetic variation in GIPR influences the glucose and insulin responses to an oral glucose challenge. Nat Genet. (2010) 42:142–8. doi: 10.1038/ng.521
35. Heid IM, Boes E, Muller M, Kollerits B, Lamina C, Coassin S, et al. Genome-wide association analysis of high-density lipoprotein cholesterol in the population-based KORA study sheds new light on intergenic regions. Circul Cardiovasc Genet. (2008) 1: 10–20. doi: 10.1161/CIRCGENETICS.108.776708
36. Suhre K, Shin SY, Petersen AK, Mohney RP, Meredith D, Wagele B, et al. Human metabolic individuality in biomedical and pharmaceutical research. Nature (2011) 477:54–60. doi: 10.1038/nature10354
37. Saxena R, Plenge RM, Bjonnes AC, Dashti HS, Okada Y, Gad El Haq W, et al. A multinational arab genome-wide association study identifies new genetic associations for rheumatoid arthritis. Arthritis Rheumatol. (2017) 69:976–85. doi: 10.1002/art.40051
38. Wakil SM, Ram R, Muiya NP, Mehta M, Andres E, Mazhar N, et al. A genome-wide association study reveals susceptibility loci for myocardial infarction/coronary artery disease in Saudi Arabs. Atherosclerosis (2016) 245:62–70. doi: 10.1016/j.atherosclerosis.2015.11.019
39. Shan J, Al-Rumaihi K, Rabah D, Al-Bozom I, Kizhakayil D, Farhat K, et al. Genome scan study of prostate cancer in Arabs: identification of three genomic regions with multiple prostate cancer susceptibility loci in Tunisians. J Transl Med. (2013) 11:121. doi: 10.1186/1479-5876-11-121
40. Shan J, Mahfoudh W, Dsouza SP, Hassen E, Bouaouina N, Abdelhak SA, et al. Genome-wide association studies (GWAS) breast cancer susceptibility loci in Arabs: susceptibility and prognostic implications in Tunisians. Breast Cancer Res Treat. (2012) 135:715–24. doi: 10.1007/s10549-012-2202-6
41. Abuyassin B, Laher I. Obesity-linked diabetes in the Arab world: a review. East Mediterr Health J. (2015) 21:420–39. doi: 10.26719/2015.21.6.420
42. Alkhateeb A, Al-Azzam S, Zyadine R, Abuarqoub D. Genetic association of adiponectin with type 2 diabetes in Jordanian Arab population. Gene (2013) 512:61–3. doi: 10.1016/j.gene.2012.09.095
43. Mtiraoui N, Ezzidi I, Turki A, Chaieb A, Mahjoub T, Almawi WY. Single-nucleotide polymorphisms and haplotypes in the adiponectin gene contribute to the genetic risk for type 2 diabetes in Tunisian Arabs. Diabetes Res Clin Pract. (2012) 97:290–7. doi: 10.1016/j.diabres.2012.02.015
44. Al-Daghri NM, Alkharfy KM, Al-Attas OS, Krishnaswamy S, Mohammed AK, Albagha OM, et al. Association between type 2 diabetes mellitus-related SNP variants and obesity traits in a Saudi population. Mol Biol Rep. (2014) 41:1731–40. doi: 10.1007/s11033-014-3022-z
45. Al-Awadi SA, Moussa MA, Naguib KK, Farag TI, Teebi AS, el-Khalifa M., et al. Consanguinity among the Kuwaiti population. Clin Genet. (1985) 27:483–6. doi: 10.1111/j.1399-0004.1985.tb00236.x
46. Warsy AS, Al-Jaser MH, Albdass A, Al-Daihan S, Alanazi M. Is consanguinity prevalence decreasing in Saudis?: a study in two generations. African Health Sci. (2014) 14:314–21. doi: 10.4314/ahs.v14i2.5
47. Gosadi IM, Goyder EC, Teare MD. Investigating the potential effect of consanguinity on type 2 diabetes susceptibility in a Saudi population. Hum Heredity. (2014) 77:197–206. doi: 10.1159/000362447
48. Bener A, Hussain R. Consanguineous unions and child health in the State of Qatar. Paediatr Perinat Epidemiol. (2006) 20:372–8. doi: 10.1111/j.1365-3016.2006.00750.x
49. Benrahma H, Arfa I, Charif M, Bounaceur S, Eloualid A, Boulouiz R, et al. Maternal effect and familial aggregation in a type 2 diabetic Moroccan population. J Commun Health (2011) 36:943–8. doi: 10.1007/s10900-011-9393-3
50. Arfa I, Abid A, Malouche D, Ben Alaya N, Azegue TR, Mannai I, et al. Familial aggregation and excess maternal transmission of type 2 diabetes in Tunisia. Postgrad Med J. (2007) 83:348–51. doi: 10.1136/pgmj.2006.053744
51. Al-Sinani S, Al-Shafaee M, Al-Mamari A, Woodhouse N, Al-Shafie O, Hassan M, et al. Familial clustering of type 2 diabetes among omanis. Oman Med J. (2014) 29:51–4. doi: 10.5001/omj.2014.11
52. Bener A, Yousafzai MT, Al-Hamaq AO, Mohammad AG, Defronzo RA. Parental transmission of type 2 diabetes mellitus in a highly endogamous population. World J Diabetes 4 (2013) 40–6. doi: 10.4239/wjd.v4.i2.40
53. Blair DR, Lyttle CS, Mortensen JM, Bearden CF, Jensen AB, Khiabanian H, et al. A non-degenerate code of deleterious variants in Mendelian loci contributes to complex disease risk. Cell (2013) 155:70–80. doi: 10.1016/j.cell.2013.08.030
54. De Sanctis V, Zurlo MG, Senesi E, Boffa C, Cavallo L, Di Gregorio F. Insulin dependent diabetes in thalassaemia. Arch Dis childh. (1988) 63:58–62. doi: 10.1136/adc.63.1.58
55. Podolsky S, Leopold NA, Sax DS. Increased frequency of diabetes mellitus in patients with Huntington's chorea. Lancet (1972) 1:1356–8. doi: 10.1016/S0140-6736(72)91092-6
56. Ristow M. Neurodegenerative disorders associated with diabetes mellitus. J Mol Med. (2004) 82:510–29. doi: 10.1007/s00109-004-0552-1
57. Cutting GR. Modifier genes in mendelian disorders: the example of cystic fibrosis. Ann NY Acad Sci. (2010) 1214:57–69. doi: 10.1111/j.1749-6632.2010.05879.x
58. Hu Y, Liang J, Yu S. High prevalence of diabetes mellitus in a five-generation Chinese family with Huntington's disease. J Alzheimer's Dis. (2014) 40:863–8. doi: 10.3233/JAD-131847
59. Amberger J, Bocchini CA, Scott AF, Hamosh A. McKusick's online mendelian inheritance in man (OMIM). Nucleic Acids Res. (2009) 37:D793–6. doi: 10.1093/nar/gkn665
60. Al-Gazali L, Hamamy H. Consanguinity and dysmorphology in Arabs. Hum Heredity (2014) 77:93–107. doi: 10.1159/000360421
61. Bo-Abbas Y, Acierno JS, Shagoury JK, Crowley WF, Seminara SB. Autosomal recessive idiopathic hypogonadotropic hypogonadism: genetic analysis excludes mutations in the gonadotropin-releasing hormone (GnRH) and GnRH receptor genes. J Clin Endocrinol Metabol. (2003) 88:2730–7. doi: 10.1210/jc.2002-021948
62. Al Hayek AA, Khader YS, Jafal S, Khawaja N, Robert AA, Ajlouni K. Prevalence of low testosterone levels in men with type 2 diabetes mellitus: a cross-sectional study. J Fam Commun Med. (2013) 20:179–86. doi: 10.4103/2230-8229.122006
63. Chaleby K, Tuma TA. Cousin marriages and schizophrenia in Saudi Arabia. Br J Psychiatr (1987) 150:547–9. doi: 10.1192/bjp.150.4.547
64. Lin PI, Shuldiner AR. Rethinking the genetic basis for comorbidity of schizophrenia and type 2 diabetes. Schizophr Res. (2010) 123:234–43. doi: 10.1016/j.schres.2010.08.022
65. Shaltout TE, Alali KA, Bushra S, Alkaseri AM, Jose ED, Al-Khainji M, et al. Significant association of close homologue of L1 gene polymorphism rs2272522 with schizophrenia in Qatar. Asia Pac Psychiatry (2013) 5:17–23. doi: 10.1111/appy.12014
66. Pankow JS, Decker PA, Berardi C, Hanson NQ, Sale M, Tang W, et al. Circulating cellular adhesion molecules and risk of diabetes: the Multi-Ethnic Study of Atherosclerosis (MESA). Diabet Med. (2016) 33:985–91. doi: 10.1111/dme.13108
67. Hippisley-Cox J, Coupland C. Development and validation of QDiabetes-2018 risk prediction algorithm to estimate future risk of type 2 diabetes: cohort study. BMJ (2017) 359:j5019. doi: 10.1136/bmj.j5019
68. Visscher PM, Wray NR, Zhang Q, Sklar P, McCarthy MI, Brown MA, Yang J. 10 Years of GWAS discovery: biology, function, and translation. Am J Hum Genet. (2017) 101:5–22. doi: 10.1016/j.ajhg.2017.06.005
69. El-Azami-El-Idrissi M, Lakhdar-Idrissi M, Ouldim K, Bono W, Amarti-Riffi A, Hida M, et al. Improving medical research in the Arab world. Lancet (2013) 382:2066–7. doi: 10.1016/S0140-6736(13)62692-6
70. El Obaid Y, Al Hamiz A, Abdulle A, Hayes RB, Sherman S, Ali R. Perceptions and attitudes towards medical research in the United Arab Emirates: results from the Abu Dhabi Cohort Study (ADCS) focus group discussions. PLoS ONE (2016) 11:e0149609. doi: 10.1371/journal.pone.0149609
71. Pfeiffer RM, Gail MH. Sample size calculations for population- and family-based case-control association studies on marker genotypes. Genet Epidemiol. (2003) 25:136–48. doi: 10.1002/gepi.10245
72. Hong EP, Park JW. Sample size and statistical power calculation in genetic association studies. Genom Info. (2012) 10:117–22. doi: 10.5808/GI.2012.10.2.117
73. Purcell S, Cherny SS, Sham PC. Genetic power calculator: design of linkage and association genetic mapping studies of complex traits. Bioinformatics (2003) 19:149–50. doi: 10.1093/bioinformatics/19.1.149
74. Skol AD, Scott LJ, Abecasis GR, Boehnke M. Joint analysis is more efficient than replication-based analysis for two-stage genome-wide association studies. Nat Genet (2006) 38:209–13. doi: 10.1038/ng1706
75. Gauderman WJ. Sample size requirements for association studies of gene-gene interaction. Am J Epidemiol. (2002) 55:478–84. doi: 10.1093/aje/155.5.478
76. Alsmadi O, Thareja G, Alkayal F, Rajagopalan R, John SE, Hebbar P, et al. Genetic substructure of Kuwaiti population reveals migration history. PLoS ONE (2013) 8:e74913. doi: 10.1371/journal.pone.0074913
77. Alsmadi O, John SE, Thareja G, Hebbar P, Antony D, Behbehani K, et al. Genome at juncture of early human migration: a systematic analysis of two whole genomes and thirteen exomes from Kuwaiti population subgroup of inferred Saudi Arabian tribe ancestry. PLoS ONE (2014) 9:e99069. doi: 10.1371/journal.pone.0099069
78. Thareja G, John SE, Hebbar P, Behbehani K, Thanaraj TA, Alsmadi O. Sequence and analysis of a whole genome from Kuwaiti population subgroup of Persian ancestry. BMC Genom. (2015) 16:92. doi: 10.1186/s12864-015-1233-x
79. John SE, Thareja G, Hebbar P, Behbehani K, Thanaraj TA, Alsmadi O. Kuwaiti population subgroup of nomadic bedouin ancestry-whole genome sequence and analysis. Genom Data (2015) 3:116–27. doi: 10.1016/j.gdata.2014.11.016
80. Hunter-Zinck H, Musharoff S, Salit J, Al-Ali KA, Chouchane L, Gohar A, et al. Population genetic structure of the people of Qatar. Am J Hum Genet. (2010) 87:17–25. doi: 10.1016/j.ajhg.2010.05.018
81. Adriaanse MC, Drewes HW, van der Heide I, Struijs JN, Baan CA. The impact of comorbid chronic conditions on quality of life in type 2 diabetes patients. Qual Life Res. (2016) 25:175–82. doi: 10.1007/s11136-015-1061-0
82. Piette JD, Kerr EA. The impact of comorbid chronic conditions on diabetes care. Diabetes Care (2006) 29:725–31. doi: 10.2337/diacare.29.03.06.dc05-2078
83. Scott EM, Halees A, Itan Y, Spencer EG, He Y, Azab MA, et al. Gleeson, characterization of greater middle eastern genetic variation for enhanced disease gene discovery. Nat Genet. (2016) 48:1071–6. doi: 10.1038/ng.3592
84. Tadmouri GO, Al Ali MT, Al-Haj Ali S, Al Khaja N. CTGA: the database for genetic disorders in Arab populations. Nucleic Acids Res. (2006) 34:D602–6. doi: 10.1093/nar/gkj015
85. Beasley TM, Erickson S, Allison DB. Rank-based inverse normal transformations are increasingly used, but are they merited? Behav Genet. (2009) 39:580–95. doi: 10.1007/s10519-009-9281-0
86. Buzkova P. Linear regression in genetic association studies. PLoS ONE (2013) 8:e56976. doi: 10.1371/journal.pone.0056976
87. Teebi AS. Autosomal recessive disorders among Arabs: an overview from Kuwait. J Med Genet. (1994) 1:224–33. doi: 10.1136/jmg.31.3.224
88. Alexander DH, Novembre J, Lange K. Fast model-based estimation of ancestry in unrelated individuals. Genome Res. (2009) 19:1655–64. doi: 10.1101/gr.094052.109
89. Price AL, Patterson NJ, Plenge RM, Weinblatt ME, Shadick NA, Reich D. Principal components analysis corrects for stratification in genome-wide association studies. Nat Genet. (2006) 38:904–9. doi: 10.1038/ng1847
90. Loh PR, Tucker G, Bulik-Sullivan BK, Vilhjalmsson BJ, Finucane HK, Salem RM, et al. Efficient Bayesian mixed-model analysis increases association power in large cohorts. Nat Genet. (2015) 47:284–90. doi: 10.1038/ng.3190
91. Lippert C, Listgarten J, Liu Y, Kadie CM, Davidson RI, Heckerman D. FaST linear mixed models for genome-wide association studies. Nat Methods (2011) 8:833–5. doi: 10.1038/nmeth.1681
92. Kang HM, Sul JH, Service SK, Zaitlen NA, Kong SY, Freimer NB, et al. Variance component model to account for sample structure in genome-wide association studies. Nat Genet. (2010) 42:348–54. doi: 10.1038/ng.548
93. Soranzo N. Genetic determinants of variability in glycated hemoglobin [HbA(1c] in humans: review of recent progress and prospects for use in diabetes care. Curr Diabet Rep. (2011) 11:562–9. doi: 10.1007/s11892-011-0232-9
94. Ku CS, Naidoo N, Teo SM, Pawitan Y. Regions of homozygosity and their impact on complex diseases and traits. Hum Genet. (2011)129:1–15. doi: 10.1007/s00439-010-0920-6
95. Lencz T, Lambert C, DeRosse P, Burdick KE, Morgan TV, Kane JM, et al. Runs of homozygosity reveal highly penetrant recessive loci in schizophrenia. Proc Natl Acad Sci USA (2007) 104:19942–7. doi: 10.1073/pnas.0710021104
96. Yang TL, Guo Y, Zhang LS, Tian Q, Yan H, Papasian CJ, et al. Runs of homozygosity identify a recessive locus 12q21.31 for human adult height. J Clin Endocrinol Metabol. (2010) 95:3777–82. doi: 10.1210/jc.2009-1715
97. Yang HC, Li HW. Analysis of homozygosity disequilibrium using whole-genome sequencing data. BMC Proc. (2014) 8:S15. doi: 10.1186/1753-6561-8-S1-S15
98. Manolio TA, Collins FS, Cox NJ, Goldstein DB, Hindorff LA, Hunter DJ, et al. Finding the missing heritability of complex diseases. Nature (2009) 461:747–53. doi: 10.1038/nature08494
99. Cousminer DL, Grant SFA. Public resources aid diabetes gene discovery. Nat Genet (2018) 50:1499–500. doi: 10.1038/s41588-018-0242-5
100. Eichler EE, Flint J, Gibson G, Kong A, Leal SM, Moore JH, Nadeau JH. Missing heritability and strategies for finding the underlying causes of complex disease. Nat Rev Genet. (2010) 11:446–50. doi: 10.1038/nrg2809
101. Gibson G. Rare and common variants: twenty arguments. Nat Rev Genet. (2012) 13:135–45. doi: 10.1038/nrg3118
102. Zuk O, Hechter E, Sunyaev SR, Lander ES. The mystery of missing heritability: genetic interactions create phantom heritability. Proc Natl Acad Sci USA (2012) 109:1193–8. doi: 10.1073/pnas.1119675109
103. Riad B, Al-Yahyaee S, Albarwani S, Jaju D, Comuzzie A, Lopez-Alvarenga J, et al. Chapter 4: Some Genetic Determinants of Obesity, Type 2 Diabetes and Dyslipidemias in Oman Family Study. Dubai: CTGA (2008).
104. Hassan MO, Albarwani S, Al Yahyaee S, Al Haddabi S, Rizwi S, Jaffer A, et al. A family study in Oman: large, consanguineous, polygamous Omani Arab pedigrees. Commun Genet. (2005) 8:56–60. doi: 10.1159/000083341
105. Bayoumi RA, Al-Yahyaee SA, Albarwani SA, Rizvi SG, Al-Hadabi S, Al-Ubaidi FF, et al. Heritability of determinants of the metabolic syndrome among healthy Arabs of the Oman family study. Obesity (2007) 15:551–6. doi: 10.1038/oby.2007.555
106. Hassan MO, Jaju D, Albarwani S, Al-Yahyaee S, Al-Hadabi S, Lopez-Alvarenga JC, et al. Non-dipping blood pressure in the metabolic syndrome among Arabs of the Oman family study. Obesity (2007) 15:2445–53. doi: 10.1038/oby.2007.290
107. Wijsman EM. Family-based approaches: design, imputation, analysis, and beyond. BMC Genet. (2016) 17(Suppl 2):9. doi: 10.1186/s12863-015-0318-5
108. Wahl S, Drong A, Lehne B, Loh M, Scott WR, Kunze S, et al., Epigenome-wide association study of body mass index, and the adverse outcomes of adiposity. Nature (2017) 541:81–6. doi: 10.1038/nature20784
109. Kvaloy K, Page CM, Holmen TL. Epigenome-wide methylation differences in a group of lean and obese women - A HUNT Study. Sci Rep. (2018) 8:16330. doi: 10.1038/s41598-018-34003-8
110. Demerath EW, Guan W, Grove ML, Aslibekyan S, Mendelson M, Zhou YH, et al. Epigenome-wide association study (EWAS) of BMI, BMI change and waist circumference in African American adults identifies multiple replicated loci. Hum Mol Genet. (2015) 24:4464–79. doi: 10.1093/hmg/ddv161
111. Dick KJ, Nelson CP, Tsaprouni L, Sandling JK, Aissi D, Wahl S, et al. DNA methylation and body-mass index: a genome-wide analysis. Lancet (2014) 383:1990–8. doi: 10.1016/S0140-6736(13)62674-4
112. Feinberg AP, Irizarry RA, Fradin D, Aryee MJ, Murakami P, Aspelund T, et al. Personalized epigenomic signatures that are stable over time and covary with body mass index. Sci Transl Med. (2010) 2:49ra67. doi: 10.1126/scitranslmed.3001262
113. Chambers JC, Loh M, Lehne B, Drong A, Kriebel J, Motta V, et al. Epigenome-wide association of DNA methylation markers in peripheral blood from Indian Asians and Europeans with incident type 2 diabetes: a nested case-control study. Lancet Diab Endocrinol. (2015) 3:526–34. doi: 10.1016/S2213-8587(15)00127-8
114. Dayeh T, Volkov P, Salo S, Hall E, Nilsson E, Olsson AH, et al. Genome-wide DNA methylation analysis of human pancreatic islets from type 2 diabetic and non-diabetic donors identifies candidate genes that influence insulin secretion. PLoS Genet. (2014) 10:e1004160. doi: 10.1371/journal.pgen.1004160
115. Toperoff G, Aran D, Kark JD, Rosenberg M, Dubnikov T, Nissan B, et al. Genome-wide survey reveals predisposing diabetes type 2-related DNA methylation variations in human peripheral blood. Hum Mol Genet. (2012) 21:371–83. doi: 10.1093/hmg/ddr472
116. Kulkarni H, Kos MZ, Neary J, Dyer TD, Kent JW, Goring HH, et al. Novel epigenetic determinants of type 2 diabetes in Mexican-American families. Hum Mol Genet. (2015) 24:5330–44. doi: 10.1093/hmg/ddv232
117. Al Muftah WA, Al-Shafai M, Zaghlool SB, Visconti A, Tsai PC, Kumar P, Spector T, et al. Epigenetic associations of type 2 diabetes and BMI in an Arab population. Clin Epigenet. (2016) 8:13. doi: 10.1186/s13148-016-0177-6
118. Drong AW, Lindgren CM, McCarthy MI. The genetic and epigenetic basis of type 2 diabetes and obesity. Clin Pharmacol Therapeut. (2012) 92:707–15. doi: 10.1038/clpt.2012.149
119. Liang X, He A, Wang W, Liu L, Du Y, Fan Q, et al. Integrating genome-wide association and eQTLs studies identifies the genes and gene sets associated with diabetes. BioMed Res Int. (2017) 2017:1758636. doi: 10.1155/2017/1758636
120. Nicolae DL, Gamazon E, Zhang W, Duan S, Dolan ME, Cox NJ. Trait-associated SNPs are more likely to be eQTLs: annotation to enhance discovery from GWAS. PLoS Genet. (2010) 6:e1000888. doi: 10.1371/journal.pgen.1000888
121. Kameswaran V, Bramswig NC, McKenna LB, Penn M, Schug J, Hand NJ, et al. Epigenetic regulation of the DLK1-MEG3 microRNA cluster in human type 2 diabetic islets. Cell Metab. (2014) 19:135–45. doi: 10.1016/j.cmet.2013.11.016
122. Muhonen P, Holthofer H. Epigenetic and microRNA-mediated regulation in diabetes. Nephrol Dial Transplant. (2009) 24:1088–96. doi: 10.1093/ndt/gfn728
123. Pradhan P, Upadhyay N, Tiwari A, Singh LP. Genetic and epigenetic modifications in the pathogenesis of diabetic retinopathy: a molecular link to regulate gene expression. New Front Ophthalmol. (2016) 2:192–204. doi: 10.15761/NFO.1000145
124. Farran B, Channanath AM, Behbehani K, Thanaraj TA. Predictive models to assess risk of type 2 diabetes, hypertension and comorbidity: machine-learning algorithms and validation using national health data from Kuwait–a cohort study. BMJ Open (2013) 3. doi: 10.1136/bmjopen-2012-002457
Keywords: Arab population, type 2 diabetes, genome-wide association studies, risk loci, Kuwait, Euro-centric risk variants, genetics, epigenetics
Citation: Hebbar P, Abubaker JA, Abu-Farha M, Tuomilehto J, Al-Mulla F and Thanaraj TA (2019) A Perception on Genome-Wide Genetic Analysis of Metabolic Traits in Arab Populations. Front. Endocrinol. 10:8. doi: 10.3389/fendo.2019.00008
Received: 05 November 2018; Accepted: 09 January 2019;
Published: 28 January 2019.
Edited by:
Ondřej Šeda, Charles University, CzechiaReviewed by:
Zhichao Feng, Albert Einstein College of Medicine, United StatesNirav Dhanesha, The University of Iowa, United States
Copyright © 2019 Hebbar, Abubaker, Abu-Farha, Tuomilehto, Al-Mulla and Thanaraj. This is an open-access article distributed under the terms of the Creative Commons Attribution License (CC BY). The use, distribution or reproduction in other forums is permitted, provided the original author(s) and the copyright owner(s) are credited and that the original publication in this journal is cited, in accordance with accepted academic practice. No use, distribution or reproduction is permitted which does not comply with these terms.
*Correspondence: Fahd Al-Mulla, ZmFoZC5hbG11bGxhQGRhc21hbmluc3RpdHV0ZS5vcmc=
Thangavel Alphonse Thanaraj, YWxwaG9uc2UudGhhbmdhdmVsQCBkYXNtYW5pbnN0aXR1dGUub3Jn
†Present Address: Jaakko Tuomilehto, Department of Public Health, University of Helsinki, Helsinki, Finland