- 1Genomic Prediction Inc., North Brunswick, NJ, United States
- 2Genomic Prediction Clinical Laboratory, North Brunswick, NJ, United States
- 3Department of Physics and Astronomy, Michigan State University, East Lansing, MI, United States
For over 2 decades preimplantation genetic testing (PGT) has been in clinical use to reduce the risk of miscarriage and genetic disease in patients with advanced maternal age and risk of transmitting disease. Recently developed methods of genome-wide genotyping and machine learning algorithms now offer the ability to genotype embryos for polygenic disease risk with accuracy equivalent to adults. In addition, contemporary studies on adults indicate the ability to predict polygenic disorders with risk equivalent to monogenic disorders. Existing biobanks provide opportunities to model the clinical utility of polygenic disease risk reduction among sibling adults. Here, we provide a mathematical model for the use of embryo screening to reduce the risk of type 1 diabetes. Results indicate a 45–72% reduced risk with blinded genetic selection of one sibling. The first clinical case of polygenic risk scoring in human preimplantation embryos from patients with a family history of complex disease is reported. In addition to these data, several common and accepted practices place PGT for polygenic disease risk in the applicable context of contemporary reproductive medicine. In addition, prediction of risk for PCOS, endometriosis, and aneuploidy are of particular interest and relevance to patients with infertility and represent an important focus of future research on polygenic risk scoring in embryos.
Introduction
Contemporary preimplantation genetic testing (PGT) is a well-established method for reducing the risk of adverse outcomes in in vitro fertilization (IVF). PGT-A (aneuploidy screening) reduces the risk of miscarriage, implantation failure, and multiples without compromising success rates (1, 2). Given the maternal age-related increase in aneuploidy, PGT-A has also been shown to be particularly important in older women (3). PGT-SR (structural rearrangements) is also widely used by couples which carry a balanced translocation or other structural rearrangement, in order to reduce the risk of miscarriage and to prevent disease associated with an unbalanced karyotype (4). PGT-M (monogenic disease) has also helped prevent many serious conditions in children born to at risk parents (5). Finally, as Edwards and Schulman predicted in 1996, testing embryos for polygenic disorders is now possible (6). As with any new application in reproductive medicine, the recent introduction of PGT-P (for polygenic disease risk reduction) has been met with both criticism and enthusiasm.
A recent study suggested that screening embryos for polygenic traits has limited utility (7). The authors elected to define utility as expected gain in trait value. However, there may also be utility in eliminating negative (disease) outcomes, which is arguably a more ethical and practical application of polygenic risk scoring in human embryos. In other words, utilization of PGT-P to reduce disease risk, as opposed to improving upon a desired trait, may be more ethically justifiable, as well as more likely to be successful. Interestingly, an American Society for Reproductive Medicine (ASRM) Practice Committee opinion on PGT for adult onset conditions of lesser severity states that “testing is not available for multifactorial diseases” (8).
The first step in making such testing (PGT-P) possible, is demonstrating the ability to accurately obtain genome-wide genotypes from an embryo biopsy. This step has been completed, with >99% concordance between limited quantities of DNA when compared to large quantities of DNA (9). As a result of this advance, it is now possible to equate performance of polygenic risk scoring in adult populations to performance on embryos produced during IVF. Khera et al. has already shown that many polygenic diseases, including breast cancer, type 2 diabetes, coronary artery disease, and atrial fibrillation can be accurately predicted in adults, “with risk equivalent to monogenic mutations” (10). DNA databases with sibling cohorts are also now available, and allow for analysis of the risk reduction provided by genetic testing for selection against polygenic disease.
For example, 2,601 type 1 diabetes affected/unaffected, sibling-pair families are available from the type 1 diabetes consortium (T1DGC) (11). These data provide an opportunity to evaluate relative risk reduction by comparing randomly selected siblings to siblings selected based on having the lowest polygenic risk score. Herein we report performance of PGT-P in the context of diabetes risk reduction by genetic testing of multiple siblings. We also provide initial observations from the first clinical application of PGT-P in families with a history of complex disease.
Materials and Methods
Mathematical Model
The liability threshold model is widely used for binary disease traits in genetics. In this model, disease status is completely determined by a continuous liability score exceeding a threshold. To generate a realistic model for a group of siblings, a correction for sibling relatedness was performed. Recent papers have shown that using logistic regression on HLA risk haplotypes in addition to other genome-wide significant loci show strong out-of-sample predictive validity for type 1 diabetes (12, 13). These constructed genomic risk scores achieved a 0.85–0.92 area under the curve for type 1 diabetes out-of-sample validation on the UK biobank (14).
Here, 2 additional predictors were implemented to test the affected sibling pair cohort of the type 1 diabetes consortium (T1DGC). Oram et al. (13), which uses the Barker et al. (12) methodology to determine the high-risk HLA MHC (DR3/DR4-DQ8, DR3/DR3, etc.) haplotypes (DRB1-DQA1-DQB1 gene locus), and Sharp et al. (14), which uses SNPs with high r2 to tag for the specific haplotypes as well as modeling interaction effects between haplotypes.
SNPs were lifted over to hg19/GRcH37 using liftOver (15) and SNP flips were handled using conform-gt (https://faculty.washington.edu/browning/conform-gt.html). Samples were imputed using BEAGLE v5 to 1KG data (16). In addition, affected sibling pair samples were sent to University of Michigan's HRC imputation server (https://imputationserver.sph.umich.edu/index.html#!) and imputed. Missing genotypes from the 1KG imputation were filled in with the HRC imputation.
For relative risk reduction analysis, siblings were randomly sampled (100 times) and compared with taking the lowest polygenic risk score sibling. The T1DGC first collected affected sibling pair families from four geographic networks (Asia-Pacific, Europe, North America, United Kingdom), and ancestry analysis was performed upon this cohort, confirming a mixture of Caucasian and East Asian ancestry components. In addition, type 1 diabetes cases and controls were ascertained from existing and de novo collections. The T1DGC assembled 2,601 type 1 diabetes affected sibling pair families. Additional information can be found in Supplementary Methods.
Clinical Case
A same-sex couple was referred to Genomic Prediction Clinical Laboratory (CLIA#31D2152380) for PGT-P. The couple reported a family history of heart disease and stroke in one set of grandparents in one male partner (age 37), and a history of breast cancer (mother and paternal grandmother), and type 2 diabetes (maternal grandmother, paternal grandmother, and paternal aunt) in the other partner (age 35). The couple otherwise denied a personal or family history of other polygenic conditions available for testing at Genomic Prediction Clinical Laboratory. The couple denied a history of multiple pregnancy loss, parental chromosome rearrangements, previous pregnancies, or family history of aneuploidy. Standard pedigree nomenclature (17) was used in Figure 1. The couple chose to use an oocyte donor (age 23) and to fertilize half with one partner's sperm and half with the others. The couple consented to research.
Genetic Counseling
The following text describes information provided to patients during genetic counseling and prior to obtaining informed consent for performing PGT-P.
PGT-P refers specifically to screening embryos for one or more polygenic disorders; diseases influenced by genetic variants in more than one gene. The purpose of this testing is to identify which embryos have an increased lifetime risk of developing specific disease conditions, such as type I diabetes and coronary artery disease. Embryo biopsy samples are evaluated for hundreds of thousands of single nucleotide polymorphisms (SNPs) to produce data that can be used to define a polygenic risk score for the specific disease(s) of interest. Each PGT-P predictor is trained on a large repository of hundreds of thousands of genomes with associated clinical phenotypes, as part of validating a polygenic risk score (PRS) model.
Validation on positive controls demonstrated a diagnostic accuracy of 94% for PGT-P on the polygenic trait of type 1 diabetes, and a variant concordance with the controls which exceeded 99% for PGT-P genotyping. The genotyping concordance refers to the ability of the test to correctly characterize genetic variants using the PGT-P genotyping platform. The clinical positive predictive value (prediction of a clinical diagnosis of a given disease) varies from disease trait to disease trait.
Saliva samples from both biological parents are required as part of this analysis to aid in data interpretation for improved accuracy.
A “high risk” result indicates that the polygenic risk score computed from the embryo's genotype suggests a high risk for the disease whose risk is being assessed. Empirical studies of individuals with equivalent polygenic risk scores indicate a lifetime disease risk where the average population-matched individual with this score is in the top 2% of risk.
A “normal risk” result indicates that the polygenic score computed from that embryo's genome does not indicate risk exceeding the top 2% of genomes in that individual's population. Each embryo is in a “risk percentile,” which describes the fraction of other genomes in their population which is lower than them in polygenic risk. In this way, a 98th percentile embryo is in the top 2% of highest risk. Generally, a lower risk (and a lower percentile) is preferable.
An “inconclusive” result indicates that the data could not be interpreted to determine polygenic risk scores. The chance for an embryo to be designated as inconclusive is <1%. A “no amp” result indicates that the no DNA was detected in the sample. The chance for an embryo to be designated as no amp is <5%. In these scenarios, a repeat biopsy is usually recommended to complete the analysis.
PGT-P results are based upon polygenic risk scores (PRS) for each given disease trait. PGT-P is a screening tool, designed to provide a risk estimate only. This is not a diagnostic test. PRS are not a guarantee of the presence or absence of disease. PGT-P is designed to provide a PRS only for the specific condition(s) requested by the ordering provider. Additional PRS for other polygenic diseases are not included in analysis. In demographics different from the Caucasian training set, sensitivity will be reduced.
Disease risk is adjusted to the sex of the embryo and the familial disease history, where available. For most polygenic conditions, disease risk will be additionally influenced by environmental, and other non-genetic factors. PGT-P does not address these factors as part of the analysis. Less frequently, conditions that are generally considered polygenic may be highly affected by rare, monogenic variants, which are inherited in certain families. If these variants are rare, the polygenic risk score may not take these into account, and the polygenic risk score accuracy may perform with severely reduced prediction of true disease risk. Based on these limitations, testing for the polygenic disorder(s) in individuals conceived following PGT-P testing is recommended according to standard clinical criteria.
PGT-P is not a replacement for PGT-M and is not capable of detecting monogenic causes of disease. For cases with known monogenic variants causing a Mendelian inheritance pattern of disease, these variants should be addressed using PGT-M.
Additional polygenic disorders, monogenic disorders, microdeletion and microduplication syndromes, segmental aneuploidies associated with parental chromosome rearrangements, and other genetic and non-genetic disorders, are outside the scope of PGT-P screening, and will not be detected by this test.
Results
Type 1 Diabetes Risk Reduction
A type 1 diabetes high risk cohort (families with history of the disease) was evaluated. There were families with 2, 3, 4, and 5 siblings, all fully grown adults, with and without mature (final) type 1 disease status. The probability of randomly selecting a sibling with type 1 diabetes was compared to the probability after ranking siblings by genetic testing for polygenic risk. A reduction of 45% for 2 siblings, 55% for 3, 71% for 4, and 72% for 5 was observed (Figure 2).
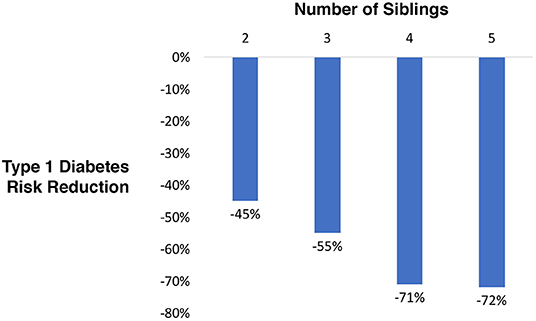
Figure 2. Relative type 1 diabetes risk reduction when selecting a sibling using blinded genetic testing for polygenic scoring compared to random selection.
PGT-P Case
In the first application of PGT for polygenic disease risk, 6 embryos were tested for aneuploidy and for lifetime risk of type 1 diabetes, type two diabetes, breast cancer, testicular cancer, prostate cancer, basal cell carcinoma, malignant melanoma, heart attack, atrial fibrillation, coronary artery disease, hypertension, high cholesterol. One embryo gave inconclusive results due to failed amplification. A repeat biopsy was recommended. All five remaining embryos were euploid, and two displayed a high risk for breast cancer (Figure 3). The couple elected to perform another cycle before proceeding with embryo transfer to a gestational carrier.
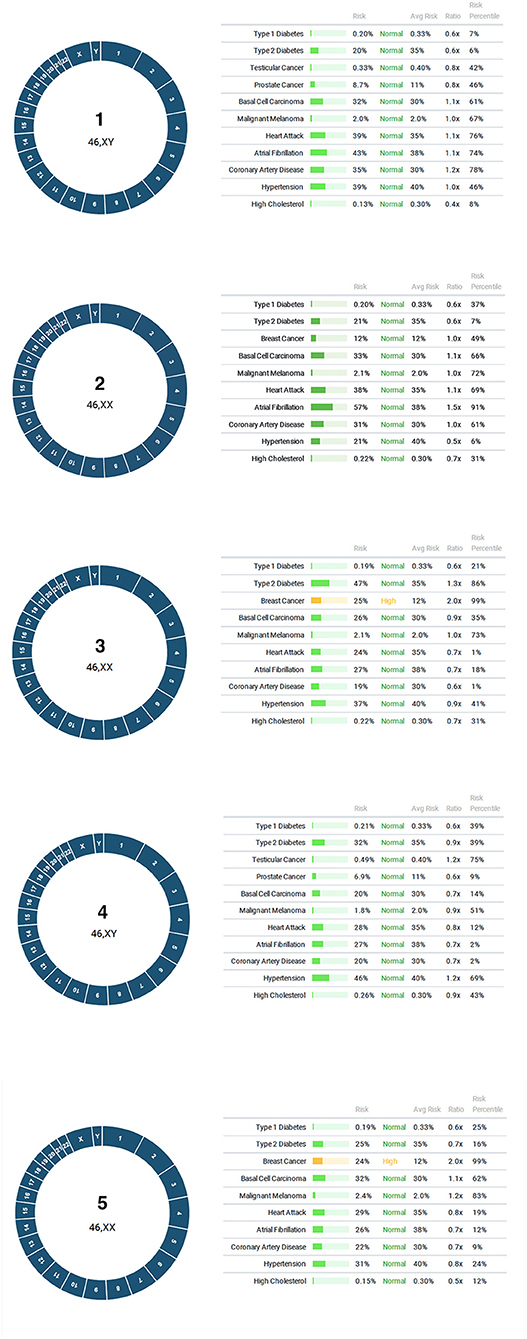
Figure 3. Results of PGT-P. All five embryos were euploid, with two displaying high risk for breast cancer.
Discussion
This study demonstrates the clinical utility of PGT for type 1 diabetes risk reduction, ranging from 45 to 72% in families with an affected individual. The model accounts for having 2–5 siblings available for genetic testing. Interestingly, patients utilizing PGT-M commonly produce ~6–7 embryos following IVF (18). In addition, patients utilizing PGT-M often do so based on family history (19). The model presented here is analogous to this situation, in that diabetes risk reduction was applied by genetic testing of siblings where the family was known to have a history of type 1 diabetes.
It is also reasonable to expect that patients seeking infertility treatment for other indications may produce 2 or more euploid embryos. In the first case involving PGT-P, the couple produced five euploid blastocysts and presented with a family history of several polygenic disorders, including breast cancer. Two embryos were found to have a high risk of breast cancer (top 99th percentile of risk, or higher) with >2X the average risk. A unique feature of the PGT methodology performed in this study is the ability to perform testing in all four major categories (PGT-A, -M, -SR, and -P) using the same platform. As a result of this capability, the additional information on polygenic risk (i.e., cancer), could be performed at no additional cost to the patient. This illustrates an important opportunity for patients to elect to obtain PGT-P results after they have determined the number of euploid blastocysts available for transfer.
Despite widespread application and demonstrated clinical utility, PGT has spurred numerous debates regarding the ethics of its use (6, 8, 20–22). Controversial applications include savior siblings, social sexing, adult onset disease testing, and testing for diseases of lesser severity and penetrance.
Data on PGT for social sexing (PGT-SS), also referred to as family balancing, was recorded by the European Society for Human Reproduction and Embryology PGT Consortium for over a decade before the procedure was deemed “ethically unacceptable” (23). PGT-SS involves elective IVF (i.e., treatment of patients without infertility), embryo biopsy, genetic testing to determine each embryos sex, and selection of the desired sex for embryo transfer. While the percentage of PGT-SS was routinely reported to represent only 2% of all PGT applications, many patients are able to choose the sex of the embryo for transfer as a result of incidental testing during conventional PGT.
Although some outlying countries and IVF programs still debate clinical utility, it is generally accepted that patients with advanced maternal age be offered the option of PGT-A to reduce the risk of miscarriage due to aneuploidy. An ethics committee of the American Society for Reproductive Medicine has recently published an opinion on disclosing an embryo's sex when incidentally revealed as part of the PGT process (22). Essentially, the committee argues that each clinic should create a formal policy, and that patients should be specifically informed of that policy prior to initiating treatment with PGT. Actual practice across individual clinics vary considerably but fall within these guidelines.
For example, a large IVF program in the United States recently performed a comparison of implantation rates between patients using PGT-A that elected to either transfer an embryo based on its sex or based on its morphological quality (24). The report indicated that 48% of patients in the study chose which embryo to transfer based upon its sex, illustrating that PGT-SS within PGT is a relatively common practice. PGT-P may be applied in a similar fashion. That is, patients may elect to select amongst available euploid embryos based upon additional information; the risk of polygenic disease.
ASRM has also released an ethics opinion on the use of PGT for adult onset conditions, indicating that reproductive liberty arguments ethically allow for testing conditions that are highly treatable, of lesser severity, or exhibit reduced penetrance (8). For example, alpha 1 antitrypsin, hereditary hemochromatosis, non-classic 21 hydroxylase deficiency, biotinidase deficiency, and familial Mediterranean fever all fit this category. These disorders are amongst the most frequently identified in the clinical setting through expanded carrier screening (25). In addition, mutations in the BRCA1/2 genes are a common indication for PGT-M, recently reported to represent ~4% of all PGT-M cases at a large reference laboratory (26). BRCA mutations account for ~5–6% of breast cancer (27, 28). However, polygenic origins may account for 10–15% given the frequency of familial breast cancer (29).
Remaining questions regarding the clinical utility of screening embryos for polygenic disease risk reduction include to what extent family history is required. Polygenic risk scoring in adults is known to benefit from availability of data on age, sex, and clinical risk (30, 31). Although the sex of an embryo is easily predicted, age, and clinical risk data are not available, which may necessitate family history as a surrogate (32).
While prior research focused on expected gain in polygenic trait value (height and intelligence) with embryo selection (7), the present study demonstrates the clinical utility of embryo selection for polygenic disease risk reduction. As new databases are developed, new predictors will continue to become available, and existing predictors may be further improved (Table 1). Prediction of risk for PCOS, endometriosis, and aneuploidy are of particular interest and relevance to patients with infertility, and represent an important focus of future research on polygenic risk scoring in embryos.
Data Availability Statement
The datasets generated for this study will not be made publicly available due to maintaining confidentiality of identifiable patient data.
Ethics Statement
Ethical review and approval was not required for the study on human participants in accordance with the local legislation and institutional requirements. The patients/participants provided their written informed consent to participate in this study. Written informed consent was obtained from the individual(s) for the publication of any potentially identifiable images or data included in this article.
Author Contributions
NT, SH, EB, JH, and LT designed the study. JE and KP counseled the patient. NT, LT, EB, JE, LL, RZ, BR, and AS analyzed the data. RZ and BR performed the laboratory work. NT, JE, LL, and LT wrote the manuscript.
Conflict of Interest
All authors are employees or founders of Genomic Prediction Inc.
Acknowledgments
This study has been performed with UK Biobank data under application 15326.
Supplementary Material
The Supplementary Material for this article can be found online at: https://www.frontiersin.org/articles/10.3389/fendo.2019.00845/full#supplementary-material
References
1. Forman EJ, Hong KH, Ferry KM, Tao X, Taylor D, Levy B, et al. In vitro fertilization with single euploid blastocyst transfer: a randomized controlled trial. Fertil Steril. (2013) 100:100–7.e101. doi: 10.1016/j.fertnstert.2013.02.056
2. Scott RT Jr, Ferry K, Su J, Tao X, Scott K, Treff NR. Comprehensive chromosome screening is highly predictive of the reproductive potential of human embryos: a prospective, blinded, nonselection study. Fertil Steril. (2012) 97:870–5. doi: 10.1016/j.fertnstert.2012.01.104
3. Rubio C, Bellver J, Rodrigo L, Castillon G, Guillen A, Vidal C, et al. In vitro fertilization with preimplantation genetic diagnosis for aneuploidies in advanced maternal age: a randomized, controlled study. Fertil Steril. (2017) 107:1122–9. doi: 10.1016/j.fertnstert.2017.03.011
4. Morin SJ, Eccles J, Iturriaga A, Zimmerman RS. Translocations, inversions and other chromosome rearrangements. Fertil Steril. (2017) 107:19–26. doi: 10.1016/j.fertnstert.2016.10.013
5. Simpson JL, Kuliev A, Rechitsky S. Overview of preimplantation genetic diagnosis (PGD): historical perspective and future direction. Methods Mol Biol. (2019) 1885:23–43. doi: 10.1007/978-1-4939-8889-1_2
6. Schulman JD, Edwards RG. Preimplantation diagnosis in disease control, not eugenics. Hum Reprod. (1996) 11:463–4. doi: 10.1093/HUMREP/11.3.463
7. Karavani E, Zuk O, Zeevi D, Atzmon G, Barzilai N, Stefanis NC, et al. Screening human embryos for polygenic traits has limited utility. Cell. (2019). doi: 10.1016/j.cell.2019.10.033. [Epub ahead of print].
8. ASRM Practice-Committee. Use of preimplantation genetic testing for monogenic defects (PGT-M) for adult-onset conditions: an Ethics Committee opinion. Fertil Steril. (2018) 109:989–92. doi: 10.1016/j.fertnstert.2018.04.003
9. Treff NR, Zimmerman R, Bechor E, Hsu J, Rana B, Jensen J, et al. Validation of concurrent preimplantation genetic testing for polygenic and monogenic disorders, structural rearrangements, and whole and segmental chromosome aneuploidy with a single universal platform. Eur J Med Genet. (2019) 62:103647. doi: 10.1016/j.ejmg.2019.04.004
10. Khera AV, Chaffin M, Aragam KG, Haas ME, Roselli C, Choi SH, et al. Genome-wide polygenic scores for common diseases identify individuals with risk equivalent to monogenic mutations. Nat Genet. (2018) 50:1219–24. doi: 10.1038/s41588-018-0183-z
11. Burren OS, Adlem EC, Achuthan P, Christensen M, Coulson RM, Todd JA. T1DBase: update 2011, organization and presentation of large-scale data sets for type 1 diabetes research. Nucleic Acids Res. (2011) 39:D997–1001. doi: 10.1093/nar/gkq912
12. Barker JM, Triolo TM, Aly TA, Baschal EE, Babu SR, Kretowski A, et al. Two single nucleotide polymorphisms identify the highest-risk diabetes HLA genotype: potential for rapid screening. Diabetes. (2008) 57:3152–5. doi: 10.2337/db08-0605
13. Oram RA, Patel K, Hill A, Shields B, McDonald TJ, Jones A, et al. A type 1 diabetes genetic risk score can aid discrimination between type 1 and type 2 diabetes in young adults. Diabetes Care. (2016) 39:337–44. doi: 10.2337/dc15-1111
14. Sharp SA, Rich SS, Wood AR, Jones SE, Beaumont RN, Harrison JW, et al. Development and standardization of an improved type 1 diabetes genetic risk score for use in newborn screening and incident diagnosis. Diabetes Care. (2019) 42:200–7. doi: 10.2337/dc18-1785
15. Gao B, Huang Q, Baudis M. segment_liftover : a Python tool to convert segments between genome assemblies. F1000Res. (2018) 7:319. doi: 10.12688/f1000research.14148.2
16. Baele G, Ayres DL, Rambaut A, Suchard MA, Lemey P. High-performance computing in bayesian phylogenetics and phylodynamics using BEAGLE. Methods Mol Biol. (2019) 1910:691–722. doi: 10.1007/978-1-4939-9074-0_23
17. Bennett RL, French KS, Resta RG, Doyle DL. Standardized human pedigree nomenclature: update and assessment of the recommendations of the National Society of Genetic Counselors. J Genet Couns. (2008) 17:424–33. doi: 10.1007/s10897-008-9169-9
18. Grace J, El-Toukhy T, Scriven P, Ogilvie C, Pickering S, Lashwood A, et al. Three hundred and thirty cycles of preimplantation genetic diagnosis for serious genetic disease: clinical considerations affecting outcome. BJOG. (2006) 113:1393–401. doi: 10.1111/j.1471-0528.2006.01143.x
19. Eccles JM, Iturriaga A, Jalas C, Behrens A, Kleinman E, Scott RTJ, et al. Experiences in single gene disorder (SGD) preimplantation genetic diagnosis (PGD): a focus on indication for testing, family member availability and its influence on test design paradigms. Fertil Steril. (2016) 106. doi: 10.1016/j.fertnstert.2016.07.254
20. ASRM Practice-Committee. Use of reproductive technology for sex selection for nonmedical reasons. Fertil Steril. (2015) 103:1418–22. doi: 10.1016/j.fertnstert.2015.03.035
21. ASRM Practice-Committee. Transferring embryos with genetic anomalies detected in preimplantation testing: an Ethics Committee Opinion. Fertil Steril. (2015) 107:1130–5. doi: 10.1016/j.fertnstert.2017.02.121
22. ASRM Practice-Committee. Disclosure of sex when incidentally revealed as part of preimplantation genetic testing (PGT): an Ethics Committee opinion. Fertil Steril. (2018) 110:625–7. doi: 10.1016/j.fertnstert.2018.06.019
23. De Rycke M, Goossens V, Kokkali G, Meijer-Hoogeveen M, Coonen E, Moutou C. ESHRE PGD consortium data collection XIV-XV: cycles from January 2011 to December 2012 with pregnancy follow-up to October 2013. Hum Reprod. (2017) 32:1974–94. doi: 10.1093/humrep/dex265
24. Whitehead CV, Metzgar TL, Rana B, Lopes CF, Juneau CR, Morin SJ, et al. Is there a difference in sustained implantation rate in patients who elect a gender preference versus those who select the best quality embryo for transfer when utilizing preimplantation genetic screening? Fertil Steril. (2017) 108:e100. doi: 10.1016/j.fertnstert.2017.07.305
25. Lazarin GA, Haque IS, Nazareth S, Iori K, Patterson AS, Jacobson JL, et al. An empirical estimate of carrier frequencies for 400+ causal Mendelian variants: results from an ethnically diverse clinical sample of 23,453 individuals. Genet Med. (2013) 15:178–86. doi: 10.1038/gim.2012.114
26. Rechitsky S, Kuliev A. Preimplantation genetic testing for non-traditional indications. Reprod Biomed Online. (2019) 38:e3. doi: 10.1016/j.rbmo.2019.03.006
27. Buys SS, Sandbach JF, Gammon A, Patel G, Kidd J, Brown KL, et al. A study of over 35,000 women with breast cancer tested with a 25-gene panel of hereditary cancer genes. Cancer. (2017) 123:1721–30. doi: 10.1002/cncr.30498
28. Tung N, Lin NU, Kidd J, Allen BA, Singh N, Wenstrup RJ, et al. Frequency of germline mutations in 25 cancer susceptibility genes in a sequential series of patients with breast cancer. J Clin Oncol. (2016) 34:1460–8. doi: 10.1200/JCO.2015.65.0747
29. Economopoulou P, Dimitriadis G, Psyrri A. Beyond BRCA: new hereditary breast cancer susceptibility genes. Cancer Treat Rev. (2015) 41:1–8. doi: 10.1016/j.ctrv.2014.10.008
30. Forgetta V, Keller-Baruch J, Forest M, Durand A, Bhatnagar SR, Kemp JP, et al. Machine learning to predict osteoporotic fracture risk from genotypes. biorix. (2019). doi: 10.1101/413716
31. Lello L, Raben T, Yong S, Tellier L, Hsu S. Genomic prediction of 16 complex disease risks including heart attack, diabetes, breast and prostate cancer. Sci Rep. (2019) 9:15286. doi: 10.1038/s41598-019-51258-x
32. Chatterjee N, Shi J, Garcia-Closas M. Developing and evaluating polygenic risk prediction models for stratified disease prevention. Nat Rev Genet. (2016) 17:392–406. doi: 10.1038/nrg.2016.27
33. Wunnemann F, Sin Lo K, Langford-Avelar A, Busseuil D, Dube MP, Tardif JC, et al. Validation of genome-wide polygenic risk scores for coronary artery disease in french canadians. Circ Genom Precis Med. (2019) 12:e002481. doi: 10.1161/CIRCGEN.119.002481
34. Mavaddat N, Michailidou K, Dennis J, Lush M, Fachal L, Lee A, et al. Polygenic risk scores for prediction of breast cancer and breast cancer subtypes. Am J Hum Genet. (2019) 104:21–34. doi: 10.1016/j.ajhg.2018.11.002
35. Black MH, Li S, LaDuca H, Chen J, Hoiness R, Gutierrez S, et al. Validation of a prostate cancer polygenic risk score for clinical use. White Paper. (2018). Available online at: https://www.ambrygen.com/file/material/view/985/AmbryScore_Prostate_WhitePaper_0918_final.pdf
Keywords: polygenic risk score, preimplantation genetic testing, advanced maternal age, aneuploidy, type 1 diabetes
Citation: Treff NR, Eccles J, Lello L, Bechor E, Hsu J, Plunkett K, Zimmerman R, Rana B, Samoilenko A, Hsu S and Tellier LCAM (2019) Utility and First Clinical Application of Screening Embryos for Polygenic Disease Risk Reduction. Front. Endocrinol. 10:845. doi: 10.3389/fendo.2019.00845
Received: 15 July 2019; Accepted: 19 November 2019;
Published: 04 December 2019.
Edited by:
Katja Teerds, Wageningen University, NetherlandsReviewed by:
Hakan Yarali, Anatolia IVF Center, TurkeyRossella Mazzilli, Sapienza University of Rome, Italy
Copyright © 2019 Treff, Eccles, Lello, Bechor, Hsu, Plunkett, Zimmerman, Rana, Samoilenko, Hsu and Tellier. This is an open-access article distributed under the terms of the Creative Commons Attribution License (CC BY). The use, distribution or reproduction in other forums is permitted, provided the original author(s) and the copyright owner(s) are credited and that the original publication in this journal is cited, in accordance with accepted academic practice. No use, distribution or reproduction is permitted which does not comply with these terms.
*Correspondence: Nathan R. Treff, nathan@genomicprediction.com