- 1Department of Endocrinology, Xinhua Hospital, Shanghai Jiaotong University School of Medicine, Shanghai, China
- 2Department of Cardiology, Shanghai Tenth People’s Hospital, Tongji University School of Medicine, Shanghai, China
Background: Since 2020, longer stay-at-home time in response to the coronavirus disease 2019 (COVID-19) pandemic has changed the weight-related behaviors of Chinese population.
Objectives: To explore the demographic and basic characteristics of body fat scale users and to investigate the changes in obesity-related body composition of overweight and obese users during COVID-19. Further, we analyzed the factors associated with successful weight loss and improved body composition changes in overweight and obese people.
Methods: The study included 107,419 Chinese adults registered in the smart app connecting to the body fat scale in 2020 to describe the demographic characteristics of body fat scale users by Unpaired Student’s t-test and Chi-Square test. Subsequently, overweight and obese participants with body mass index (BMI) of more than 24 kg/m2 were screened to investigate the independent factors associated with effective weight loss and improved body composition changes by multivariable logistic regression analyses.
Results: During the pandemic, the number of body fat scale users increased markedly compared with pre-pandemic. Over half of the participants were women and with normal baseline BMI. Based on BMI classification, multivariable logistic regressions showed that age, gender, measurement frequency classification, baseline BMI, visceral adipose index and skeletal muscle rate were associated with weight loss and fat loss in the overweight and obese population, with the high-frequency measurement being the most important factor for effective weight and fat loss. In the population with normal BMI obesity, younger age was the most significant factor for effective fat loss.
Conclusion: During the COVID-19 pandemic, participation in self-monitored weight loss increased markedly compared with pre-pandemic, and women accounted for the majority. We found that many overweight and obese participants achieved weight loss goals by smart body fat scales, and the effectiveness of weight and fat loss was greater in obese participants than in overweight participants, both based on BMI and PBF classification. In addition, promoting the usage of smart body fat scales could contribute to more effective weight and fat loss in the overweight and obese population based on BMI classification. However, in the population with normal BMI obesity, young subjects might be easier to successfully lose fat compared with the elder. Digital self-management by smart body fat scales could become a promising approach for the obese population with high BMI to lose weight and keep healthy.
Introduction
Due to the rapid improvement of economic level and the westernization of dietary habits in China, the proportion of overweight and obese patients in the adult population climbed from 18.9% and 2.9% in 2002 to 34.3% and 16.4% in 2020, respectively (1). According to previous studies, obesity and metabolic syndrome were closely related to the occurrence and development of diabetes (2), hyperlipidemia, cardiovascular disease (3), cerebrovascular disease (4), and cancers (5). Obesity has become a critical metabolic disease threatening people’s health and is the most common comorbidity reported among patients with severe coronavirus disease 2019 (COVID-19) (6), thus the prevention and treatment of obesity care are crucial to avoid complications and a high rate of hospitalization. Several studies confirmed the potential efficacy of lifestyle interventions in reducing obesity in Caucasians (7, 8). These studies found that interventions, such as establishing appropriate weight loss goals, self-monitoring, and adjusting dietary intake and physical activity, could achieve the purpose of weight loss (9). Because of stay-at-home for more time than before, Chinese people have changed their daily behaviors including dietary intake and physical activity during the COVID-19 pandemic (10).
On account of the progression of the Internet and smartphones, various types of online health management software have appeared. Wang Y analyzed 17 reviews and found that health self-management depending on mobile apps was beneficial to the treatment of diabetes and obesity (11). In the meta-analysis by Flores M et al., patients using online methods had significantly greater reductions in body weight and body mass index (BMI) compared with patients using other traditional methods such as diary records (12). Notably, the frequency of self-weighing was higher in the group that uploaded weight data via Bluetooth compared with the group that entered weight data manually, and the percentage of participants who achieved weight-loss goals was also noticeably higher (13).
BMI is the most commonly used indicator for diagnosing obesity. At present, 24 kg/m2 and 28 kg/m2 of BMI values are used as the boundary values for being overweight and obese in China (14). However, it fails to determine whether the changes in BMI caused by fat mass (FM) or non-fat mass (FFM) (15). The concept of percentage of body fat (PBF) refers to the proportion of body fat mass in total body weight. Normal BMI obesity refers to people with normal BMI and high PBF. Previous studies found this specific population was susceptible to metabolic disorders and PBF could be used to help assess the metabolic risk (16–18). Thus, it is more reliable to establish PBF as an index to assess the improvement effect of body composition in this specific population. Nevertheless, most previous studies on the effect of weight loss were carried out in European Caucasians. Few studies focused on the effectiveness of self-managed weight loss via body fat scales in Chinese population, especially during the COVID-19 pandemic.
According to the aforementioned studies (11–13), online software-based self-management helped weight loss and alleviated diabetes and obesity. However, the effects of self-management based on online software and its independent factors during the COVID-19 pandemic are unclear, which are our current study concerns. Here, we conducted a cohort study based on the data of obesity-related anthropometric indices from the Qingniu Health app connecting to the body fat scale in overweight and obese people for at least one year self-monitoring, to test our hypotheses that online software might help self-management during the COVID-19 pandemic and measurement frequency would be an important factor.
Materials and methods
Subjects
The inclusion of participants were adult users aged 18 to 79 years in China, who signed up to use the Qingniu Health app connecting to the body fat scale in 2020 during the COVID-19 pandemic. Participants with extremely abnormal initial weight (below 30kg), BMI (below 14 kg/m2 and above 60 kg/m2) and PBF (below 10%) were excluded. Furthermore, participants whose annual measurement frequency was less than 2 times were also excluded. 107,419 subjects were included to describe the basic and demographic characteristics of body fat scale users registered in 2020. Then, 31,227 overweight participants with BMI of 24-27.9 kg/m2 and 15,509 obese participants with BMI ≥28 kg/m2 were followed up to investigate the improvement of obesity-related body composition indices for at least one year, which meant the time span from the first measurement to the last measurement was more than 1 year. Moreover, 13,724 participants with normal BMI but excessive PBF were also followed up (Figure 1). Electronic consent for the inclusion of participants was obtained. The study was approved by Xinhua Hospitals’ Ethics Committee.
Data collection
Data were collected in the Qingniu Health app, which contained the baseline information of participants at the time of initial registration, including gender, age, height, and residential city, as well as the measurements including weight, PBF, visceral adipose index (VAI), skeletal muscle rate (SMR) and fat-to-muscle ratio (FMR) by using the same brand, the same type of body fat scales (CS10C; Yolanda Technology Co., Ltd., Shenzhen, China). The measurement principle of the body fat scales is the multi-frequency bioelectrical impedance analysis (BIA), as same as the previous study (19). The participant’s body fat scales measurement data were synchronized to the Qingniu Health app platform via Bluetooth.
In the following analyses, participants were grouped by gender, age, BMI, PBF, measurement frequency, and the residential city level, respectively. Participants aged <40, 40 - 59, and ≥60 years were defined as the adults, middle-aged and elderly group. Participants of BMI <18.5 kg/m2, 18.5 - 23.9 kg/m2, 24 - 27.9 kg/m2, and ≥28 kg/m2 were considered as underweight, normal, overweight and obesity respectively. Male with PBF ≥25.0% and female with PBF ≥30.0% were regarded as obese (20, 21), and the others were regarded as non-obese subjects. Normal BMI obesity refers to people with normal BMI and high PBF. Participants were categorized into tertiles of measurement frequency as low, middle, and high-frequency groups. As the usage of body fat scales was irregular during the follow-up period, we adopted the last measurement indices at least more than 1 year from baseline as the end-point results. The annual changes in weight, PBF and SMR were calculated as follows: (last measurement value – initial measurement value)/time (month) * 12. A study showed weight loss by 5% of initial weight reduced the risk of obesity-related comorbidities (22). Therefore, we defined participants with a decrease of more than 5% of initial body weight as effective weight loss. Likewise, a decrease of more than 5% of initial PBF was considered effective fat loss (23). The annual growth rate of SMR increased by more than 5%, which was also considered beneficial muscle gain.
Statistical analyses
Continuous variables were described as mean and standard deviation (SD), measurement frequency was described as median and interquartile range (IQR), and categorical variables were described as count and percentage. Unpaired Student’s t-test or one-way ANOVA was used to analyze differences among groups, and differences in constituent ratios were analyzed by Chi-Square test. Univariable and multivariable logistic regression analyses were used to assess the independent influencing factors of the annual change rates of body weight, PBF, and SMR, respectively. Statistical analyses were performed using the Statistical Package for the Social Sciences (SPSS v.26.0, SPSS Inc.). Statistically significance was set at p value <0.05.
Results
General baseline characteristics of body fat scale users during the COVID-19 pandemic
The annual number of users registered in 2020 during the pandemic (N=107,419) was significantly higher than that in 2019 before the pandemic (N=73,307). During the pandemic, over half of the participants were women (84.7%) and with normal BMI. On average, participants were 32.87 ± 8.51 years and consisted of underweight (4.1%), normal (52.4%), overweight (29.1%), and obesity (14.4%) (Table 1). Participants in economically developed cities such as first-tier, new first-tier, and second-tier cities accounted for 86.5%. According to the users’ annual measurement frequency, participants were divided into three groups: low, middle, and high-frequency groups (13.33 IQR: 10.53; 42.03 IQR: 21.58; and 129.73 IQR: 101.61 times per year). At baseline, the average age of female users was younger than that of males (P <0.0001); the average weight of men was 79.13 ± 13.97 kg, and that of women was 61.62 ± 11.12 kg (P <0.0001); the average BMI of men was significantly higher than that of women (26.26 ± 4.20 vs 23.59 ± 4.00 kg/m2, P <0.0001), the proportion of overweight and obese users was also higher in men than in women (69.4% vs 38.9%, P <0.0001). Interestingly, the largest proportion of female users was in the normal BMI group (56.4%), and the average annual measurement frequency of female users was much higher than that of male users (44.44 IQR: 79.62 vs 30.89 IQR: 52.45 times per year, P <0.0001).
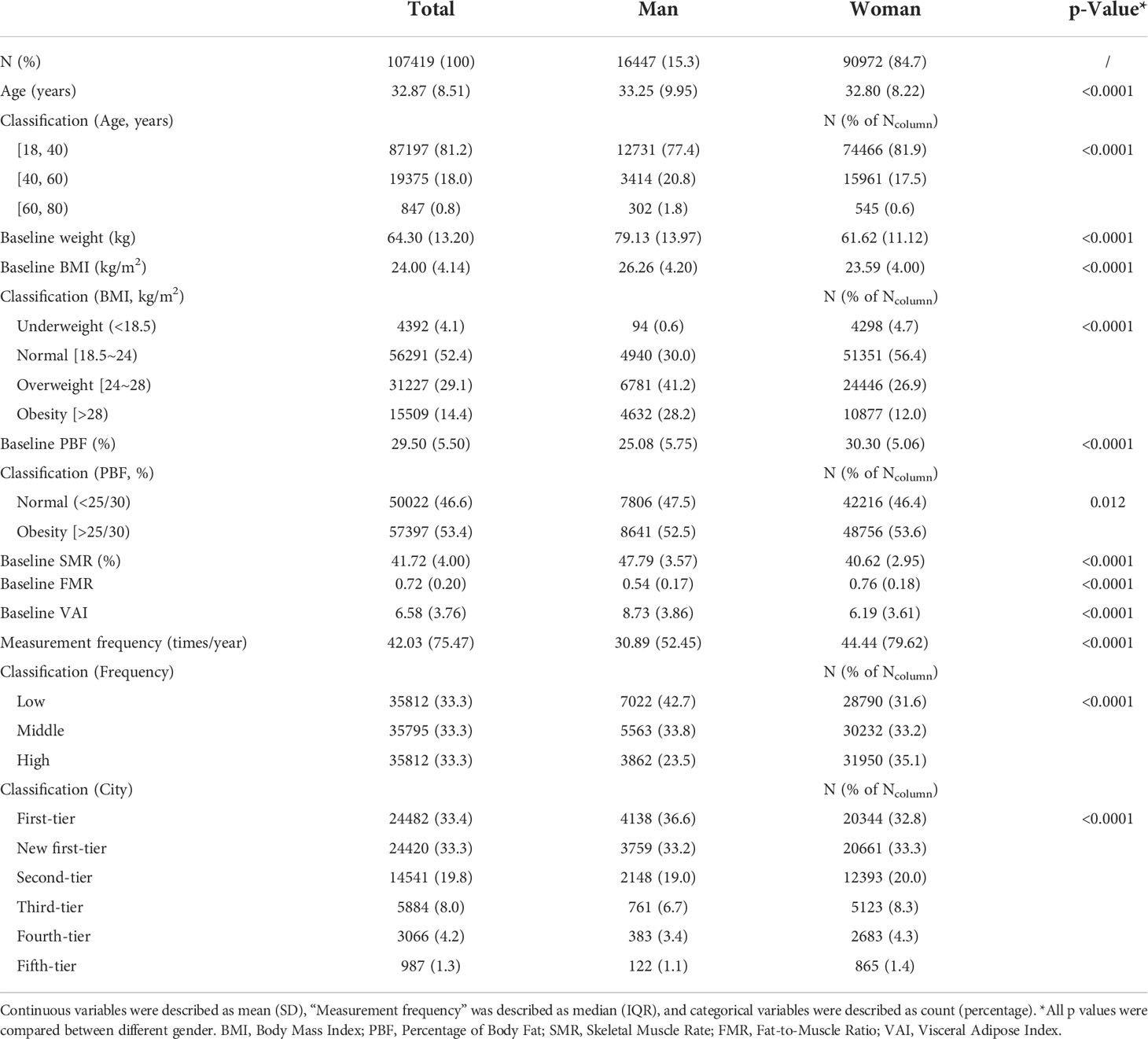
Table 1 Baseline characteristics of the participants grouped by gender during the COVID-19 pandemic.
Annual changes in weight and body composition for overweight and obese participants based on BMI classification
A total of 46,736 overweight and obese participants (N=31,227, 15,509 respectively) at baseline who had healthy needs of weight loss were followed up for longer than one year. There was no statistical difference in the annual weight loss of participants among different age groups. Nevertheless, the group aged 18-40 years experienced a greater decrease in PBF and a greater increase in SMR compared with the group aged 40-60 years. According to BMI classification, obese participants showed greater changes in all indices, including weight, BMI and PBF compared with overweight participants (P <0.0001). In obese men, on average, the annual body weight decreased by 3.90 ± 7.77 kg (Figure 2A), and the BMI decreased by 1.42 ± 2.59 kg/m2. In obese women, the annual weight loss was 4.74 ± 8.78 kg, and the BMI decreased by 1.80 ± 3.36 kg/m2. Grouped by PBF, obese participants showed greater changes in all indices than normal individuals as well (P <0.0001). Among different measurement frequency groups, the changes of all obesity-related indices in the high-frequency group were significantly greater than those of the low and middle-frequency groups (P <0.0001) (Figure 2B; Table 2).
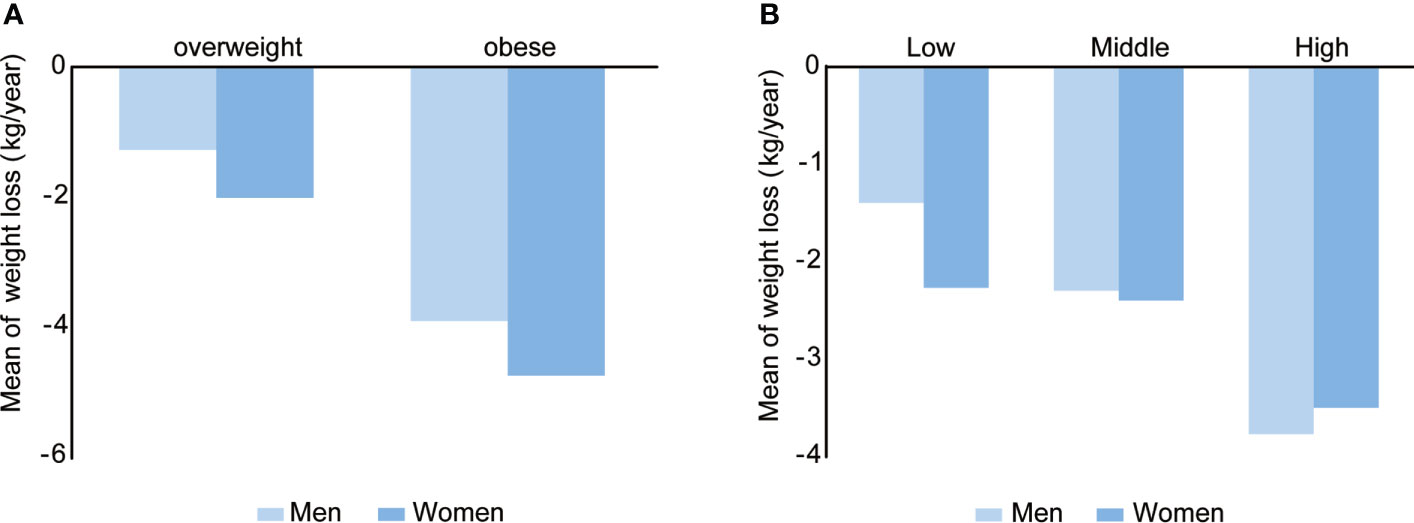
Figure 2 Mean of weight loss during the COVID-19 period in different BMI (A) and measurement frequency (B) groups. Overweight, men: n=6781, women: n=24446; obese, men: n=4632, women: n=10877. Low frequency, men: n=4475, women: n=8602; middle frequency, men: n=3900, women: n=11117; High frequency, men: n=3038, women: n=15604.
Independent factors associated with weight loss, fat loss, and muscle gain
Based on the above findings, we further performed univariable and multivariable logistic regression analyses to find the independent factors associated with successful weight, fat loss and muscle gain. Univariable logistic analysis showed that age, gender, measurement frequency classification, baseline weight, BMI, PBF, VAI and SMR were associated with weight loss (Table S1). Multivariable logistic regression models revealed that participants of the female sex, with lower age, lower VAI, higher baseline BMI, PBF, SMR and measurement frequency were more likely to succeed in losing weight (Table 3). As described in Bajaj NS’ study (24), the importance of each factor in the logistic regression model was measured as the partial chi-square statistic minus the predictor degrees of freedom (χ2 - df). High measurement frequency was the largest predictor for effective weight loss (OR = 2.101, 95% CI 1.997-2.211, χ2 - df = 818.81, P <0.0001) when other associated factors were corrected, including age, gender, baseline BMI, PBF, VAI and SMR (Figure 3A).
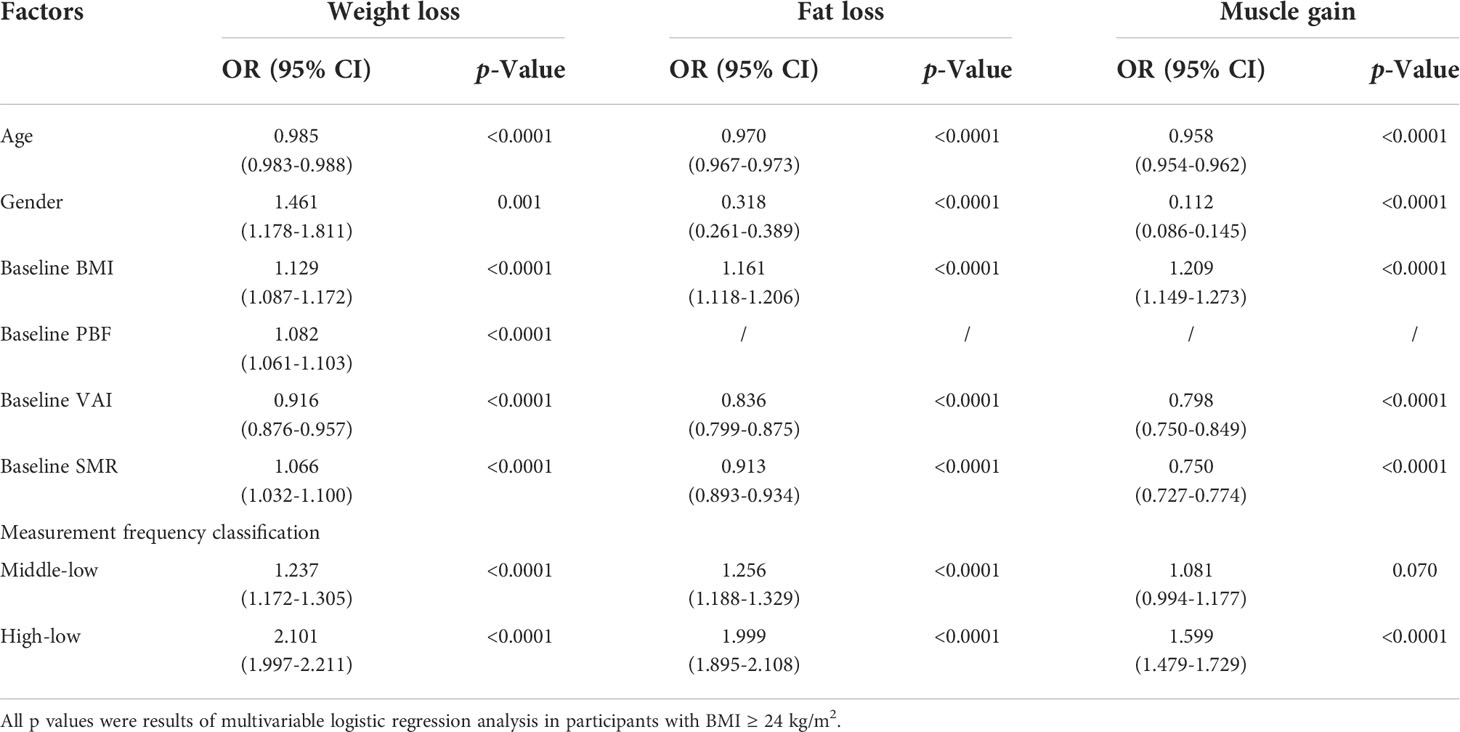
Table 3 Factors associated with the effective change of weight and obesity-associated body composition.
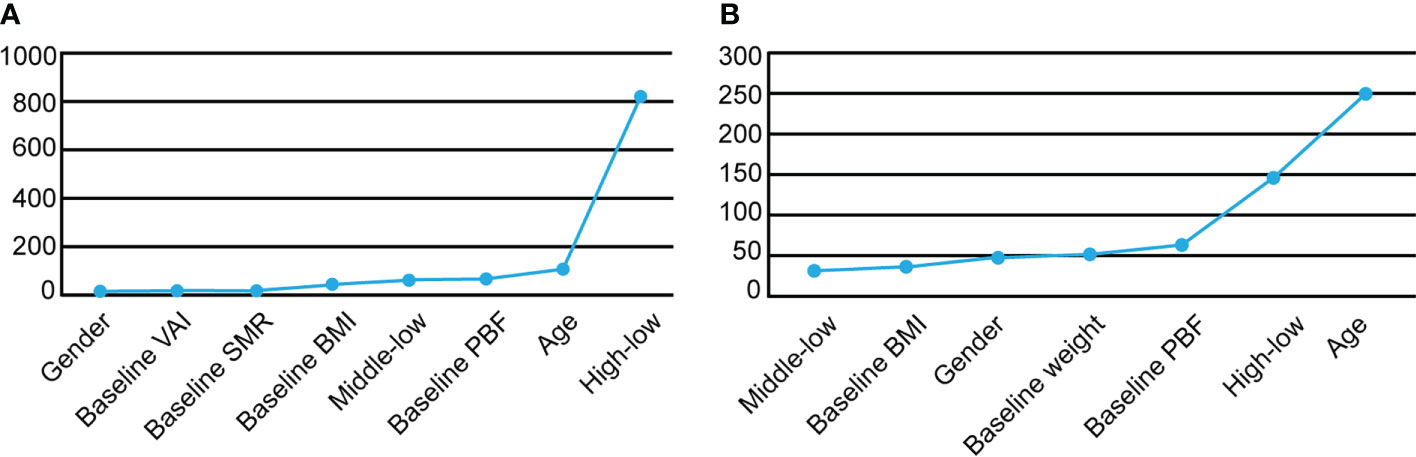
Figure 3 The importance of factors in logistic regression model which was measured as the partial chi-square statistic minus the predictor degrees of freedom (χ2 - df) was shown. (A) χ2 - df values for the prediction of weight loss success in the overweight and obese population with BMI ≥24. (B) χ2 - df values for the prediction of effective fat loss in the obese population with normal BMI.
Multivariable logistic regression analysis showed that lower age, male sex, lower baseline VAI, SMR, higher baseline BMI and measurement frequency were independent factors for effective fat loss (Table 3). Especially, high measurement frequency was a most contributing factor for success of fat loss (OR = 1.999, 95% CI 1.895-2.108, χ2 - df = 645.50, P <0.0001).
Table S1 showed age, gender, measurement frequency classification, baseline weight, BMI, PBF, VAI and SMR were associated with muscle gain. Multivariable logistic regression models showed age was a most significant factor for muscle gain (OR = 0.958, 95% CI 0.954-0.962, χ2 - df = 384.43, P <0.0001) when other factors were corrected. Other than lower age, significant factors associated with successful muscle gain were male sex, higher measurement frequency, higher baseline BMI, lower baseline VAI and SMR (Table 3).
Independent factors associated with fat loss in the population with normal BMI but excessive PBF
13,724 participants with normal BMI but excessive PBF were observed, and women accounted for 99.5% of this specific obese population. In the group with normal BMI, the measurement frequency was higher in people with excessive PBF than in people with normal PBF (51.51 IQR: 87.12 vs 33.87 IQR: 57.79, P <0.0001), which indicated that participants were not only concerned about changes in weight and BMI, but PBF management was also an important part of health monitoring (Table S2). Multivariable logistic regression analysis displayed male sex, lower age and BMI, higher baseline weight, PBF and measurement frequency were promoting factors for effective fat loss and muscle gain, among which, lower age was the most important contributing factor (Table 4; Figure 3B).
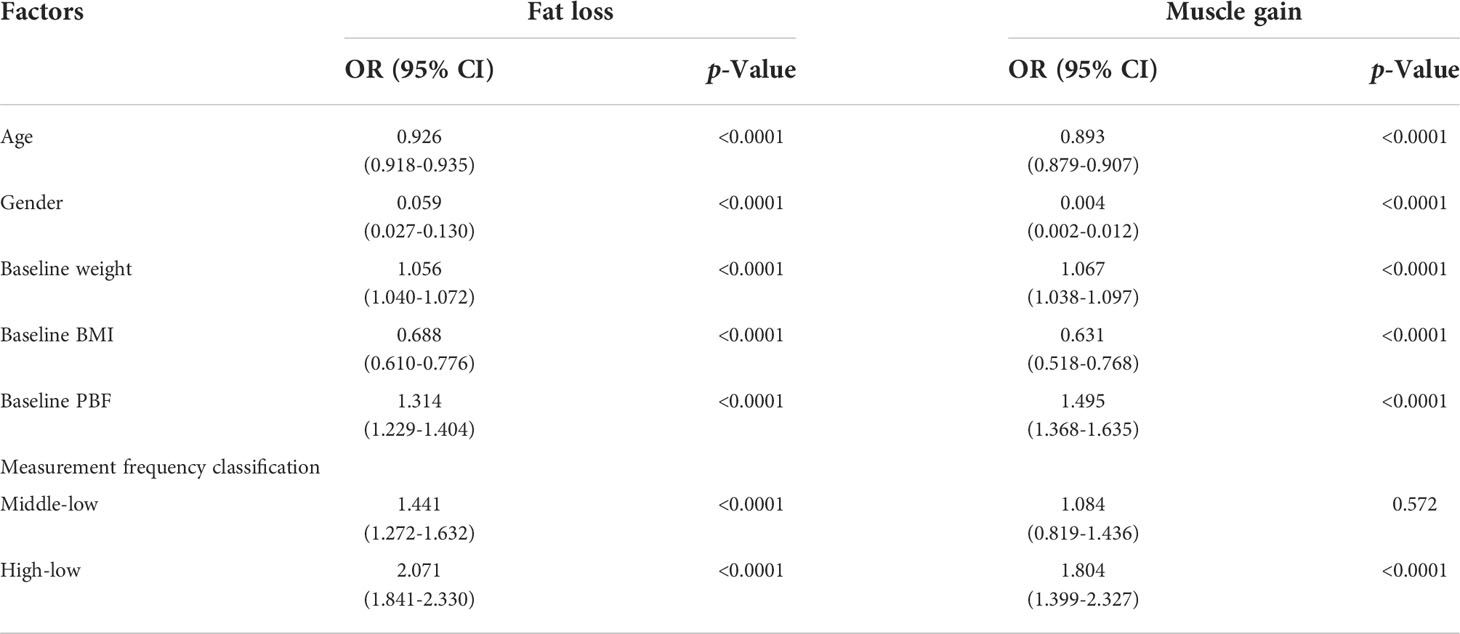
Table 4 Factors associated with the effective fat loss and muscle gain in participants with normal BMI but high PBF.
Discussion
Obesity, closely related to chronic metabolic diseases such as hypertension and diabetes, endangers the health of Chinese (25, 26). Recently, the harm of obesity has become more visible, and people have paid more attention to weight management (27). More and more body fat scales accompanied with their supporting weight management apps have appeared, and have been popularized in Chinese population because of convenience and economy (28). However, the role of self-managed weight loss through the use of smart body fat scales in Chinese during the COVID-19 pandemic is to be assessed. Our present study is a follow-up study based on the data of a Chinese commercial online weight management app connecting a smart body fat scale. We found online software might help self-management during the COVID-19 pandemic and measurement frequency would be an important factor.
The COVID-19 pandemic has resulted in overall changes to weight-related behaviors, including dietary intake and physical activity (29). Based on the baseline characteristics of all included users, we found that the young and middle-aged women were the main population using body fat scales during the COVID-19 pandemic, which suggested that they were more concerned about weight, body composition, and their health conditions compared with other groups. Furthermore, female users with normal BMI were more than half, which suggested that women placed more emphasis on overweight and obesity prevention than men, consistent with other research findings (28). Overweight persons predominated in male participants, nevertheless, their annual average measurement frequency was lower than female users. Thus, it was necessary to increase men’s awareness of preventing obesity in daily life through self-managed weight loss. In addition, participants in economically developed cities accounted for 86.5%, reflecting that the degree of urban development and economic level affected the usage of smart body fat scales.
Self-monitoring was the core of behavioral intervention for weight loss, including weight monitoring. Several studies found that self-monitoring was positively correlated with weight loss (30–33). However, the previous researches had some limitations, for example, the subjects of those study were a relative small sample size and confined to Caucasians. In the present study, we found the changes in weight, BMI and obesity-associated body composition were significantly different among different age, baseline BMI, PBF and measurement frequency groups in the overweight and obese participants. The changes in body composition were more obvious in the young, compared with the middle-aged person. The annual average weight loss of obese men was 3.90kg and that of obese women was 4.74kg, which implied that many overweight and obese participants based on BMI classification still achieved weight loss goals during the COVID-19 epidemic. According to PBF classification, the annual average weight loss of obese men was 2.83kg and that of obese women was 2.85kg, which showed a greater reduction than participants with normal PBF. At the same time, we found that the values of weight loss and fat loss were higher in obese participants than in overweight participants, both based on BMI and PBF classification, which indicated high PBF was also an important index, as in other studies (34). The current study provided evidence for the value of self-managed weight loss by using an online weight management app in the overweight and obese population.
A clinical study published by Carter et al. found that the participant’s compliance with weight monitoring by smartphone was significantly improved compared with paper diary and website monitoring (35). In Thomas’s study, participants with a BMI of 27-40 kg/m2 weighed using a commercial online smart scale and received active weight loss therapy simultaneously. This study showed that higher weight monitoring frequency was associated with better weight loss, which was consistent with numerous studies (13, 33). Bennett and colleagues found that the weight loss of participants with more frequent self-weighing was significantly higher than others in the 12-month digital obesity treatment (36). The sustainability of long-term self-monitoring was a strong predictor of weight loss success through lifestyle interventions (37), which was confirmed by our study that the high-frequency monitoring group had greater weight loss, fat loss, and muscle gain in the overweight and obese population classified by BMI. For successful weight loss and fat loss, high-frequency measurement was the most significant promoting factor in our study, while some other related factors were not included, like lifestyle changes. However, younger age was the most significant factor for effective muscle gain when other factors were corrected, it meant younger people gained muscle more easily than older people. Interestingly, we found that younger age was the most contributing factor for effective fat loss in the population with normal BMI obesity, and moderate self-management by using a smart body fat scale could contribute to successful fat loss and muscle gain in the young people, which might be related to higher metabolic rates in young people (38). This interesting finding from our study suggests that early weight-related intervention at a younger age may lead to more successful fat loss in normal BMI obese people.
Our study revealed the beneficial role of self-monitoring of weight and body composition by a smart body fat scale in self-managed weight loss and amelioration of body composition in Chinese overweight and obese people during the COVID-19 pandemic. Moreover, we indicated promoting the usage of smart body fat scales could contribute to weight and fat loss in the overweight and obese population with high BMI, thus promoting remote weight self-monitoring by smart body fat scales in clinical practice might help the progression of weight loss in obese patients. However, there were also some limitations in our study, one of which was that participants’ conditions were not taken into account, such as diabetes and thyroid-related disease, which might affect body composition. Moreover, other contributing factors of weight loss, such as dietary intake or physical activity were not included during the follow-up periods, and some accidental conditions might affect the accuracy of body fat measurements by BIA in our real-world study, such as the hydration status. A large randomized intervention trial could be performed to reinforce the results of this study. Nevertheless, this study analyzed a sizable population from the majority of Chinese urban cities, which implied the potential advantages of health economics through self-monitored weight loss by smart body fat scales.
In conclusion, during the COVID-19 pandemic, participation in self-monitored weight loss increased markedly compared with pre-pandemic, and women accounted for the majority. We found that many overweight and obese participants achieved weight loss goals by smart body fat scales, and the effectiveness of weight and fat loss was greater in obese participants than in overweight participants, both based on BMI and PBF classification. In addition, promoting the usage of smart body fat scales could contribute to more effective weight and fat loss in the overweight and obese population by BMI classification. However, in the population with normal BMI obesity, young subjects might be easier to successfully lose fat compared with the elder. Digital self-management by smart body fat scales could become a promising approach for the obese population with high BMI to lose weight and keep healthy.
Data availability statement
The datasets presented in this article are not readily available because restrictions apply to the availability of these data. Data were obtained from the Qingniu Health app and are available from the authors with the permission of the Qingniu Health app. Requests to access the datasets should be directed to Weixia Jian,amlhbndlaXhpYUB4aW5odWFtZWQuY29tLmNu.
Ethics statement
The studies involving human participants were reviewed and approved by Xinhua Hospitals’ Ethics Committee. Written informed consent for participation was not required for this study in accordance with the national legislation and the institutional requirements.
Author contributions
XH, ML, YS, HY, ZL, WK, BL, JS, WZ and WJ implemented the study. XH, ML, and WJ wrote the manuscript. YS, HY and ZL analyzed the data. All authors participated in the design of the studies, analysis of the data and review of the manuscript. All authors contributed to the article and approved the submitted version.
Funding
This research was funded by the Natural Science Foundation of Shanghai, grant number 20ZR1435300.
Acknowledgments
We would like to thank all participants in this study and the Qingniu Health app.
Conflict of interest
The authors declare that the research was conducted in the absence of any commercial or financial relationships that could be construed as a potential conflict of interest.
Publisher’s note
All claims expressed in this article are solely those of the authors and do not necessarily represent those of their affiliated organizations, or those of the publisher, the editors and the reviewers. Any product that may be evaluated in this article, or claim that may be made by its manufacturer, is not guaranteed or endorsed by the publisher.
Supplementary material
The Supplementary Material for this article can be found online at: https://www.frontiersin.org/articles/10.3389/fendo.2022.996814/full#supplementary-material
References
1. Pan XF, Wang L, Pan A. Epidemiology and determinants of obesity in China. Lancet Diabetes Endocrinol (2021) 9:373–92. doi: 10.1016/s2213-8587(21)00045-0
2. Piché ME, Tchernof A, Després JP. Obesity phenotypes, diabetes, and cardiovascular diseases. Circ Res (2020) 126:1477–500. doi: 10.1161/circresaha.120.316101
3. Powell-Wiley TM, Poirier P, Burke LE, Després JP, Gordon-Larsen P, Lavie CJ, et al. Obesity and cardiovascular disease: A scientific statement from the American heart association. Circulation (2021) 143:e984–e1010. doi: 10.1161/cir.0000000000000973
4. Marini S, Merino J, Montgomery BE, Malik R, Sudlow CL, Dichgans M, et al. Randomization study of obesity and cerebrovascular disease. Ann Neurol (2020) 87:516–24. doi: 10.1002/ana.25686
5. Avgerinos KI, Spyrou N, Mantzoros CS, Dalamaga M. Obesity and cancer risk: Emerging biological mechanisms and perspectives. Metabolism (2019) 92:121–35. doi: 10.1016/j.metabol.2018.11.001
6. Richardson S, Hirsch JS, Narasimhan M, Crawford JM, McGinn T, Davidson KW, et al. Presenting characteristics, comorbidities, and outcomes among 5700 patients hospitalized with COVID-19 in the new York city area. Jama (2020) 323:2052–9. doi: 10.1001/jama.2020.6775
7. van Rinsum CE, Gerards S, Rutten GM, van de Goor IAM, Kremers SPJ. The coaching on lifestyle (CooL) intervention for obesity, a study protocol for an action-oriented mixed-methods study. BMC Public Health (2018) 18:117. doi: 10.1186/s12889-017-5010-4
8. Hopstock LA, Deraas TS, Henriksen A, Martiny-Huenger T, Grimsgaard S. Changes in adiposity, physical activity, cardiometabolic risk factors, diet, physical capacity and well-being in inactive women and men aged 57-74 years with obesity and cardiovascular risk - a 6-month complex lifestyle intervention with 6-month follow-up. PloS One (2021) 16:e0256631. doi: 10.1371/journal.pone.0256631
9. Teixeira PJ, Carraça EV, Marques MM, Rutter H, Oppert JM, De Bourdeaudhuij I, et al. Successful behavior change in obesity interventions in adults: a systematic review of self-regulation mediators. BMC Med (2015) 13:84. doi: 10.1186/s12916-015-0323-6
10. Hu Z, Lin X, Chiwanda Kaminga A, Xu H. Impact of the COVID-19 epidemic on lifestyle behaviors and their association with subjective well-being among the general population in mainland China: Cross-sectional study. J Med Internet Res (2020) 22:e21176. doi: 10.2196/21176
11. Wang Y, Min J, Khuri J, Xue H, Xie B, Kaminsky AL, et al. Effectiveness of mobile health interventions on diabetes and obesity treatment and management: Systematic review of systematic reviews. JMIR Mhealth Uhealth (2020) 8:e15400. doi: 10.2196/15400
12. Flores Mateo G, Granado-Font E, Ferré-Grau C, Montaña-Carreras X. Mobile phone apps to promote weight loss and increase physical activity: A systematic review and meta-analysis. J Med Internet Res (2015) 17:e253. doi: 10.2196/jmir.4836
13. Thomas JG, Raynor HA, Bond DS, Luke AK, Cardoso CC, Wojtanowski AC, et al. Weight loss and frequency of body-weight self-monitoring in an online commercial weight management program with and without a cellular-connected 'smart' scale: a randomized pilot study. Obes Sci Pract (2017) 3:365–72. doi: 10.1002/osp4.132
14. WHO Expert Consultation. Appropriate body-mass index for Asian populations and its implications for policy and intervention strategies. Lancet (London England) (2004) 363:157–63. doi: 10.1016/s0140-6736(03)15268-3
15. Jin M, Du H, Zhang Y, Zhu H, Xu K, Yuan X, et al. Characteristics and reference values of fat mass index and fat free mass index by bioelectrical impedance analysis in an adult population. Clin Nutr (2019) 38:2325–32. doi: 10.1016/j.clnu.2018.10.010
16. Gómez-Ambrosi J, Silva C, Galofré JC, Escalada J, Santos S, Gil MJ, et al. Body adiposity and type 2 diabetes: increased risk with a high body fat percentage even having a normal BMI. Obes (Silver Spring Md.) (2011) 19:1439–44. doi: 10.1038/oby.2011.36
17. Oliveros E, Somers VK, Sochor O, Goel K, Lopez-Jimenez F. The concept of normal weight obesity. Prog Cardiovasc Dis (2014) 56:426–33. doi: 10.1016/j.pcad.2013.10.003
18. Zhu Y, Wang Z, Maruyama H, Onoda K, Huang Q. Body fat percentage and normal-weight obesity in the Chinese population: Development of a simple evaluation indicator using anthropometric measurements. Int J Environ Res Public Health (2022) 19:4238. doi: 10.3390/ijerph19074238
19. Chen W, Jiang H, Yang JX, Yang H, Liu JM, Zhen XY, et al. Body composition analysis by using bioelectrical impedance in a young healthy Chinese population: Methodological considerations. Food Nutr Bull (2017) 38:172–81. doi: 10.1177/0379572117697534
20. Lear SA, James PT, Ko GT, Kumanyika S. Appropriateness of waist circumference and waist-to-hip ratio cutoffs for different ethnic groups. Eur J Clin Nutr (2010) 64:42–61. doi: 10.1038/ejcn.2009.70
21. Sharma S, Batsis JA, Coutinho T, Somers VK, Hodge DO, Carter RE, et al. Normal-weight central obesity and mortality risk in older adults with coronary artery disease. Mayo Clinic Proc (2016) 91:343–51. doi: 10.1016/j.mayocp.2015.12.007
22. Magkos F, Fraterrigo G, Yoshino J, Luecking C, Kirbach K, Kelly SC, et al. Effects of moderate and subsequent progressive weight loss on metabolic function and adipose tissue biology in humans with obesity. Cell Metab (2016) 23:591–601. doi: 10.1016/j.cmet.2016.02.005
23. Beavers KM, Beavers DP, Newman JJ, Anderson AM, Loeser RF Jr., Nicklas BJ, et al. Effects of total and regional fat loss on plasma CRP and IL-6 in overweight and obese, older adults with knee osteoarthritis. Osteoarthritis cartilage (2015) 23:249–56. doi: 10.1016/j.joca.2014.11.005
24. Bajaj NS, Osborne MT, Gupta A, Tavakkoli A, Bravo PE, Vita T, et al. Coronary microvascular dysfunction and cardiovascular risk in obese patients. J Am Coll Cardiol (.(2018) 72:707–17. doi: 10.1016/j.jacc.2018.05.049
25. Manrique-Acevedo C, Chinnakotla B, Padilla J, Martinez-Lemus LA, Gozal D. Obesity and cardiovascular disease in women. Int J Obes (2005) (2020) 44:1210–26. doi: 10.1038/s41366-020-0548-0
26. Ng ACT, Delgado V, Borlaug BA, Bax JJ. Diabesity: the combined burden of obesity and diabetes on heart disease and the role of imaging. Nature reviews. Cardiology (2021) 18:291–304. doi: 10.1038/s41569-020-00465-5
27. Ghelani DP, Moran LJ, Johnson C, Mousa A, Naderpoor N. Mobile apps for weight management: A review of the latest evidence to inform practice. Front Endocrinol (2020) 11:412. doi: 10.3389/fendo.2020.00412
28. Senecal C, Widmer RJ, Larrabee BR, de Andrade M, Lerman LO, Lerman A, et al. A digital health weight loss program in 250,000 individuals. J Obes (2020) 2020:9497164. doi: 10.1155/2020/9497164
29. Zhang J, Zhao A, Ke Y, Huo S, Ma Y, Zhang Y, et al. Dietary behaviors in the post-lockdown period and its effects on dietary diversity: The second stage of a nutrition survey in a longitudinal Chinese study in the COVID-19 era. Nutrients (2020) 12:3269. doi: 10.3390/nu12113269
30. Brindal E, Hendrie GA, Freyne J, Noakes M. A mobile phone app designed to support weight loss maintenance and well-being (MotiMate): Randomized controlled trial. JMIR mHealth uHealth (2019) 7:e12882. doi: 10.2196/12882
31. Burke LE, Sereika SM, Parmanto B, Beatrice B, Cajita M, Loar I, et al. The SMARTER trial: Design of a trial testing tailored mHealth feedback to impact self-monitoring of diet, physical activity, and weight. Contemp Clin trials (2020) 91:105958. doi: 10.1016/j.cct.2020.105958
32. Turner-McGrievy GM, Beets MW, Moore JB, Kaczynski AT, Barr-Anderson DJ, Tate DF. Comparison of traditional versus mobile app self-monitoring of physical activity and dietary intake among overweight adults participating in an mHealth weight loss program. J Am Med Inf Assoc JAMIA (2013) 20:513–8. doi: 10.1136/amiajnl-2012-001510
33. Patel ML, Wakayama LN, Bennett GG. Self-monitoring via digital health in weight loss interventions: A systematic review among adults with overweight or obesity. Obes (Silver Spring Md.) (2021) 29:478–99. doi: 10.1002/oby.23088
34. Vuorinen AL, Helander E, Pietilä J, Korhonen I. Frequency of self-weighing and weight change: Cohort study with 10,000 smart scale users. J Med Internet Res (2021) 23:e25529. doi: 10.2196/25529
35. Carter MC, Burley VJ, Nykjaer C, Cade JE. Adherence to a smartphone application for weight loss compared to website and paper diary: pilot randomized controlled trial. J Med Internet Res (2013) 15:e32. doi: 10.2196/jmir.2283
36. Bennett GG, Steinberg D, Askew S, Levine E, Foley P, Batch BC, et al. Effectiveness of an app and provider counseling for obesity treatment in primary care. Am J Prev Med (2018) 55:777–86. doi: 10.1016/j.amepre.2018.07.005
37. Patel ML, Brooks TL, Bennett GG. Consistent self-monitoring in a commercial app-based intervention for weight loss: results from a randomized trial. J Behav Med (2020) 43:391–401. doi: 10.1007/s10865-019-00091-8
Keywords: COVID-19, weight loss, obesity, smart body fat scales, normal weight obesity
Citation: Huang X, Li M, Shi Y, Yao H, Lei Z, Kou W, Li B, Shi J, Zhang W and Jian W (2022) Self-managed weight loss by smart body fat scales ameliorates obesity-related body composition during the COVID-19 pandemic: A follow-up study in Chinese population. Front. Endocrinol. 13:996814. doi: 10.3389/fendo.2022.996814
Received: 18 July 2022; Accepted: 21 October 2022;
Published: 09 November 2022.
Edited by:
Jeff M. P. Holly, University of Bristol, United KingdomReviewed by:
Shaminie J. Athinarayanan, Virta Health Corp, United StatesMarcela Larissa Costa, University of São Paulo, Brazil
Brittanie Volk, Virta Health Corp, United States
Copyright © 2022 Huang, Li, Shi, Yao, Lei, Kou, Li, Shi, Zhang and Jian. This is an open-access article distributed under the terms of the Creative Commons Attribution License (CC BY). The use, distribution or reproduction in other forums is permitted, provided the original author(s) and the copyright owner(s) are credited and that the original publication in this journal is cited, in accordance with accepted academic practice. No use, distribution or reproduction is permitted which does not comply with these terms.
*Correspondence: Weixia Jian, amlhbndlaXhpYUB4aW5odWFtZWQuY29tLmNu
†These authors have contributed equally to this work and share first authorship