- 1Complications Research, Steno Diabetes Center Copenhagen, Herlev, Denmark
- 2Department of Epidemiology, Erasmus Medical Center, University Medical Center Rotterdam, Rotterdam, Netherlands
- 3Department of Data Science & Biostatistics, Julius Global Health, University Medical Center Utrecht, Utrecht, Netherlands
- 4Department of Clinical Sciences, Lund University, Malmö, Sweden
- 5Centre for Public Health, Queen’s University Belfast, Belfast, United Kingdom
- 6GENYO Centre for Genomics and Oncological Research, Pfizer-University of Granada-Andalusian Regional Government, Granada, Spain
- 7Instituto de Investigación Biosanitaria de Granada (ibs.GRANADA), Granada, Spain
- 8Department of Internal Medicine, Erasmus Medical Center, University Medical Center Rotterdam, Rotterdam, Netherlands
- 9deCODE Genetics, Amgen, Inc., Reykjavik, Iceland
- 10University of Bordeaux, Institut National de la Santé et de la Recherche Médicale (INSERM), Bordeaux Population Health Research Center, Bordeaux, France
- 11Nantes Université, Centre Hospitalier Universitaire Nantes, Centre National de la Recherche Scientifique, INSERM, l’institut du thorax, Nantes, France
- 12Faculty of Medicine, School of Health Sciences, University of Iceland, Reykjavik, Iceland
- 13Institute of Human Genetics, University Hospital Heidelberg, Heidelberg, Germany
- 14Department of Clinical Medicine, University of Copenhagen, Copenhagen, Denmark
- 15The Bioinformatics Center, Department of Biology, University of Copenhagen, Copenhagen, Denmark
Aim: Rare genetic variants in the CUBN gene encoding the main albumin-transporter in the proximal tubule of the kidneys have previously been associated with microalbuminuria and higher urine albumin levels, also in diabetes. Sequencing studies in isolated proteinuria suggest that these variants might not affect kidney function, despite proteinuria. However, the relation of these CUBN missense variants to the estimated glomerular filtration rate (eGFR) is largely unexplored. We hereby broadly examine the associations between four CUBN missense variants and eGFRcreatinine in Europeans with Type 1 (T1D) and Type 2 Diabetes (T2D). Furthermore, we sought to deepen our understanding of these variants in a range of single- and aggregate- variant analyses of other kidney-related traits in individuals with and without diabetes mellitus.
Methods: We carried out a genetic association-based linear regression analysis between four CUBN missense variants (rs141640975, rs144360241, rs45551835, rs1801239) and eGFRcreatinine (ml/min/1.73 m2, CKD-EPIcreatinine(2012), natural log-transformed) in populations with T1D (n ~ 3,588) or T2D (n ~ 31,155) from multiple European studies and in individuals without diabetes from UK Biobank (UKBB, n ~ 370,061) with replication in deCODE (n = 127,090). Summary results of the diabetes-group were meta-analyzed using the fixed-effect inverse-variance method.
Results: Albeit we did not observe associations between eGFRcreatinine and CUBN in the diabetes-group, we found significant positive associations between the minor alleles of all four variants and eGFRcreatinine in the UKBB individuals without diabetes with rs141640975 being the strongest (Effect=0.02, PeGFR_creatinine=2.2 × 10-9). We replicated the findings for rs141640975 in the Icelandic non-diabetes population (Effect=0.026, PeGFR_creatinine=7.7 × 10-4). For rs141640975, the eGFRcreatinine-association showed significant interaction with albuminuria levels (normo-, micro-, and macroalbuminuria; p = 0.03). An aggregated genetic risk score (GRS) was associated with higher urine albumin levels and eGFRcreatinine. The rs141640975 variant was also associated with higher levels of eGFRcreatinine-cystatin C (ml/min/1.73 m2, CKD-EPI2021, natural log-transformed) and lower circulating cystatin C levels.
Conclusions: The positive associations between the four CUBN missense variants and eGFR in a large population without diabetes suggests a pleiotropic role of CUBN as a novel eGFR-locus in addition to it being a known albuminuria-locus. Additional associations with diverse renal function measures (lower cystatin C and higher eGFRcreatinine-cystatin C levels) and a CUBN-focused GRS further suggests an important role of CUBN in the future personalization of chronic kidney disease management in people without diabetes.
1 Introduction
Urine albumin or albuminuria is one of the most important biomarkers of kidney damage in individuals with or without diabetes. In healthy individuals, the glomerular filter in the kidneys retains most of the albumin, although a small amount can usually pass through to the tubular system (1). Reabsorption of albumin is facilitated by the kidney’s proximal tubular cells (PTCs), ensuring that almost no albumin is excreted in urine under normal conditions (2, 3). Elevated excretion of albumin in the urine - initially coined as “microalbuminuria” - is one of the earliest signs of chronic kidney disease (CKD) and may be the kidney-related manifestation of general endothelial damage, where scarring of the glomerulus causes chronic leakiness through the filter of albumin and other proteins (4).
Over the past decades, the number of people with diabetes mellitus has more than doubled to a global prevalence of 537 million in 2021 (5), with serious consequences for the healthcare system and society. According to a recent European study (6), one in four hospitalized patients has diabetes. Up to 40% of individuals with diabetes develop diabetic kidney disease (DKD), which is associated with elevated cardiovascular morbidity and mortality and progresses to dependency on kidney replacement therapies such as dialysis and transplantation and is a leading cause of CKD (7).
In the recent years, studies have begun to unravel genetic aspects of albuminuria. Recently, we and others identified that genetic variants (single nucleotide variants (SNVs)) in the gene encoding for cubilin (CUBN) – the main albumin-transporter in PTCs (1, 8) – are associated with microalbuminuria and higher urine albumin levels in populations with and without diabetes (8–14). Four variants in the C-terminal end of cubilin have been of particular interest (rs141640975 (c.5069C>T; p.Ala1690Val), rs144360241 (c.6469A>G; p.Asn2157Asp), rs45551835 (c.8741C>T; p.Ala2914Val), and rs1801239 (c.8950A>G, p.Ile2984Val)); these are functional (missense) variants that have been proposed to alter the function of cubilin, leading to a form of albuminuria that may reflect a lack of tubular reabsorption of albumin (i.e., tubular albuminuria) (8). In silico structural and damage prediction analyses of the variants indicate their potential to change secondary or even tertiary structure(s) in the cubilin protein and to have different degrees of damaging effects on protein function, disease, or both (8). Our recent study further suggests that the effect of some of these variants on urine albumin levels is 2-3 times higher in diabetes compared to non-diabetes (11).
However, the role of these CUBN variants in relation to estimated glomerular filtration rate (eGFR), a clinically used marker of kidney function, is largely unexplored, and most genetic studies have focused on the general population (8, 9, 11). Recent efforts to uncover the role of these variants specifically in diabetes – and to clearly separate the effect seen here from the effect in the non-diabetes-proportion of the general population – have been performed as relatively small secondary analyses without including rs144360241 or diabetes subtypes (8). Thus far, only rs45551835 has been connected to higher levels of eGFR in type 2 diabetes and rs141640975 in non-diabetes (8). Therefore, we investigated the relationship between the four CUBN variants and eGFR in different contexts: First, we meta-analyzed studies of SNV-eGFRcreatinine regressions in Europeans with type 1 (T1D) or type 2 diabetes mellitus (T2D). We then examined single- and aggregate-variant associations separately in diabetes and non-diabetes populations of a large, nationally representative cohort facilitating application of identical phenotype definitions, including the dependency of albuminuria-stage in SNV-eGFRcreatinine associations, generation of a CUBN-specific genetic risk score (GRS), and identification of associations between individual SNVs and cystatin C-based measures of kidney function. Together, these analyses both seek to replicate previous associations in DM and NDM populations and to provide novel insights into the link between CUBN and eGFR.
2 Methods
2.1 Study design and cohorts
For the genetic association meta-analysis in diabetes mellitus (DM), we included data collected via three approaches (Figure 1): First, we acquired summary statistics from up to 15,200 individuals of European origin with either type 1 diabetes (T1D) or type 2 diabetes (T2D) subsetted from six cohorts: AfterEU (T1D) (15–18), Rotterdam (T2D) (19), DiaGene (T2D) (20), UK-ROI (T1D) (21), Genesis (T1D) (22) and ANDIS (T2D) (23). These studies (hereafter referred to as “DM cohorts”) were invited to the study and given a harmonized analysis plan provided that any subset of the requested genetic variants was available. A description of each cohort can be found in the Supplemental text.
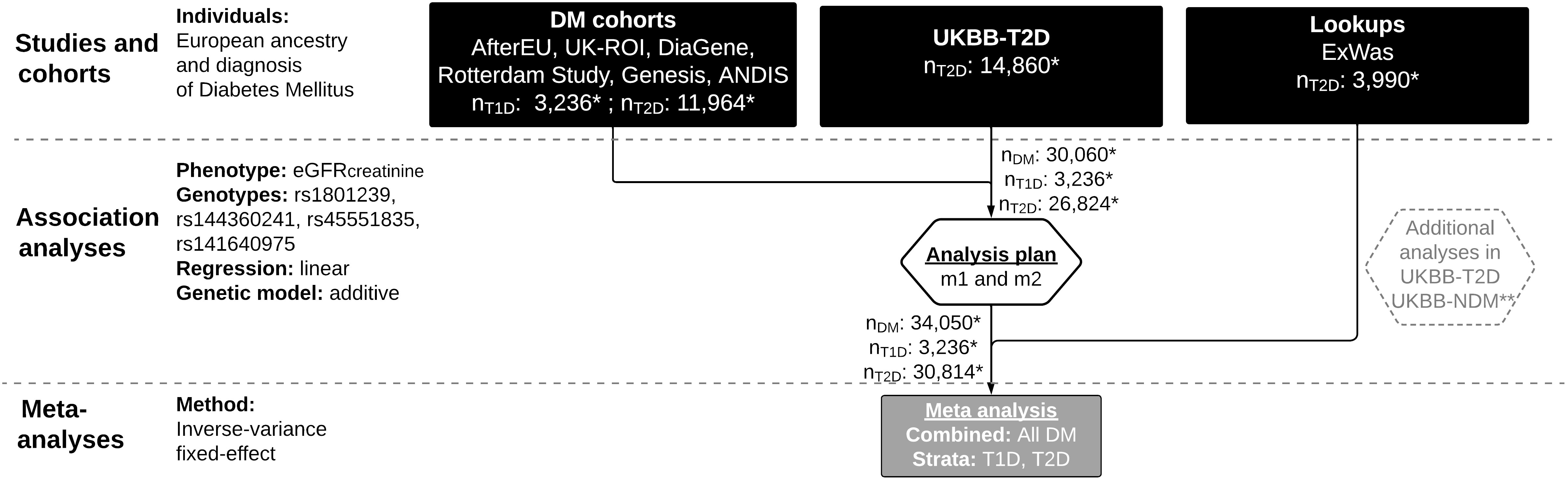
Figure 1 Flow chart of SNV-eGFRcreatinine meta-analyses in Diabetes. UKBB, UK Biobank; T2D, Type 2 diabetes; DM, diabetes mellitus; T1D, Type 1 diabetes; NDM, without diabetes mellitus; SNV, single nucleotide variant; eGFRcreatinine, Estimated glomerular filtration rate, natural log-transformed; PCs, Principal components of population structure; HbA1C, hemoglobin A1C; SBP, Systolic blood pressure; m1: model 1 (eGFRcreatinine ~ genotype + sex + age + 0-10 PCs); m2: model 2 (m1 + HbA1c + SBP + diabetes duration); * Sample sizes (n) reflect the maximal number of individuals (out of the total number of individuals in Table 1) available for rs45551835, model 1. ** See Supplementary Figure 1 for a flow chart of additional analyses. Figure made with LucidChart (lucid.app).
Second, we applied the same analysis plan to a subset of individuals with T2D (n ~ 14,860) from the UK Biobank (24) (henceforth referred to as “UKBB-T2D”). The approach we used to extract the T2D subset has been described previously (25, 26).
Third, we did a lookup in a subset of an exome-wide association study (henceforth referred to as “ExWas”) that included 3,990 individuals with T2D from three Danish studies (Inter99, Vejle biobank and Addition-DK) described previously (11).
We also searched the Type 2 Diabetes Knowledge Portal [at time of search: www.type2diabetesgenetics.org, now: https://t2d.hugeamp.org/ (27)] for large-scale studies with publicly available summary statistics fulfilling the following criteria: Summary statistics should a) be readily available through the knowledge portal or a direct link to a study website; b) be available for diabetes-stratified and European-only populations; c) include at least one target genotype; d) be based on natural log-transformed eGFR values rather than non-transformed eGFR values; and e) be based on regression models with covariate adjustments comparable to those in the other cohorts in this study. However, as of 10 July 2020, no studies in the portal fulfilled our criteria, and no additional studies were included.
For additional analyses, we used 1) a group of individuals without diabetes from UKBB (n ~ up to 370,000 individuals), henceforth referred to as “UKBB-NDM”) and 2) the UKBB-T2D group, which was also part of the meta-analysis (Supplementary Figure 1). 127,090 non-diabetes individuals from the Icelandic study deCODE participated as the replication cohort (Supplemental text).
This research work was conducted in accordance with the Helsinki Declaration. Ethical approval was previously obtained locally for individual studies. All participants gave written informed consent before participating.
2.2 Phenotype details
For the DM cohorts and UKBB (both NDM and T2D groups), we calculated the creatinine-based estimated glomerular filtration rate (eGFRcreatinine) with the Chronic Kidney Disease Epidemiology Collaboration creatinine equation (CKD-EPIcreatinine(2012), ml/min/1.73 m2 (28), natural log-transformed). We included it here as a continuous variable. Other measures of kidney function were also calculated for UKBB; see section 2.4.2.4.
2.3 Genotyping, imputation, quality control and variant selection
We obtained information on genotyping, imputation, and quality control of each cohort and summarized it in Supplementary Tables 1, 2.
Four variants were selected for further analysis: rs141640975 (Chromosome (chr) 10, position (pos) 16992011 (genome-build GRCh37.p13)) with minor allele frequency (MAF) 0.002-0.009; rs144360241 (chr 10, pos 16967417) with MAF 0.006-0.010; rs45551835 (chr 10, pos 16932384) with MAF 0.016-0.021; and rs1801239 (chr 10, pos 16919052) with MAF 0.097-0.114. For the deCODE study, the MAFs were in the same range except rs144360241 (MAF: 0.002). The minor alleles of these variants (A, C, A, and C, respectively) were used as effect alleles.
We used LDlink version 5.1 (29) with the European (CEU + GBR) reference panel to confirm the independent relationship (Linkage Disequilibrium (LD) r2< 0.1) between these SNVs.
The SNVs were first used in single-variant analyses and were then combined into a genetic risk score (GRS; see description below).
2.4 Statistical methods
A flow chart of the meta-analyses is shown in Figure 1, and one of the additional analyses is shown in Supplementary Figure 1.
2.4.1 Study-level SNV-eGFRcreatinine association analysis in diabetes and subsequent meta-analysis
In each DM cohort and UKBB-T2D, associations between eGFRcreatinine and genetic variants were assessed assuming an additive genetic model. We used natural log-transformed eGFRcreatinine in a linear regression model (model 1) adjusted for traditional clinical and genetic factors, i.e. age, gender, and study-specific covariates (i.e., 0-10 principal components of population structure to account for population stratification). To control for potential bias on kidney function in the diabetes population, another model was further adjusted for HbA1C, systolic blood pressure (a proxy for medication with Angiotensin receptor blockers (ARBs) or Angiotensin-converting enzyme inhibitor (ACEi) frequently used in diabetes treatment) and diabetes duration (model 2). Some of the cohorts used summary statistics calculated prior to our query, so we allowed minor deviations in the included covariates (Supplementary Table 3). A list of software used for association analysis can be found in Supplementary Table 1. Each study dealt with missing data separately. Once all summary results were collected, we performed study-level quality control. Summary results were meta-analyzed using a fixed-effect inverse-variance method in the “Metagen” package in R (version 3.6.3). We report results in any diabetes mellitus subtype (denoted “combined”) and in T1D and T2D subsets. Significant heterogeneity (Phet< 0.05) indicated variation across studies. Effect sizes (betas) are presented with 95% confidence intervals. We evaluate statistical significance at an FDR-corrected level of 0.05/4 = 0.0125 considering the number of tested SNVs.
2.4.2 Additional analyses in UKBB populations with diabetes and non-diabetes
To explore the interplay between CUBN-variants and kidney-related traits in more detail, we did a range of additional linear regressions in the UKBB NDM and T2D groups. Further, we also applied a combined genetic risk score (GRS). We based the analyses on model 1 and model 3. The latter was very similar to model 2, in that it included adjustment for model 1 and SBP but not HbA1c and diabetes duration. The last two adjustments were absent from this model because they are less relevant in non-diabetes. We applied the same models in DM and NDM to provide consistency. Individuals were excluded if they had missing data for any variable.
2.4.2.1 SNV-eGFRcreatinine association analysis in the UKBB population without diabetes and replication in the deCODE study
We examined SNV-eGFRcreatinine associations in the UKBB NDM and T2D populations. It was advantageous to use the UKBB dataset here as it is a well-powered, phenotypically homogenous dataset (n ~ up to 370,000 individuals without diabetes). Since effects are based on natural log-transformed eGFR (trait) values, we also calculated the percental difference in mean, non-transformed eGFR per added effect allele for significant effects as follows: % difference =(ebeta−1)*100%. . Again, we evaluated statistical significance at an FDR-corrected level of 0.0125.
SNV-eGFRcreatinine associations identified in the UKBB NDM group were also examined in the Icelandic deCODE study (nNDM=127,090) applying model 3.
2.4.2.2 Interaction with albuminuria
In order to examine whether the SNVs associated with eGFRcreatinine in an albuminuria-dependent fashion, we assessed albuminuria-SNV interactions in SNV-eGFRcreatinine regression models in individuals with T2D (nT2D = 7,777) and without DM (nNDM = 107,276) for whom continuous urine albumin levels were available (derived from the UKBB “microalbumin” field). The interaction term in the regression models included albuminuria groups as a factor defined from these albumin levels as follows: i) normoalbuminuria: =< 30 mg/L (nDM = 5,566, nNDM = 93,728), ii) microalbuminuria: 30-300 mg/L (incl. lower but not upper threshold, nNDM = 1,954, nNDM = 12,690), and iii) macroalbuminuria: >300 mg/L (incl. lower threshold, nDM = 257, nNDM = 858). We used regression models based on model 1 and 3 (i.e., model 1: ln(eGFRcreatinine) ~ SNV + albuminuria group + age + sex + SNV*albuminuria group and model 3: model 1 + SBP). A significant p-value (< 0.05) for the SNV*albuminuria interaction term was considered evidence for interaction. Interaction analysis was done whenever primary SNV-eGFRcreatinine analyses were well-powered.
2.4.2.3 Genetic risk score association with microalbuminuria and eGFRcreatinine
We estimated an albuminuria genetic risk score (GRS) using the four albuminuria-associated CUBN missense SNVs. The GRS was generated for each study participant using the sum of individual SNV effect alleles in the UKBB dataset. We then examined the associations between GRSCUBN and continuous urine microalbumin levels (mg/L) and eGFRcreatinine.
2.4.2.4 SNV vs. other kidney function-related traits in UKBB
We examined the associations between the study SNVs and 1) circulating serum Cystatin C levels (mg/L) and 2) the more recent eGFRcreatinine-cystatin C equation (30) that uses both serum creatinine and cystatin C levels and applies to all ethnicities.
2.4.3 Power calculations
We used Quanto (version 1.2.4) (31) to calculate post-hoc power for main SNV-eGFRcreatinine associations in DM and NDM groups.
For all power calculations in Quanto, we: a) chose a continuous design for independent individuals; b) assumed a gene-only hypothesis; c) assumed an additive inheritance mode; and d) set the two-sided type I error-rate to 0.05.
For the remaining options in Quanto, we typed in information specific to each variant and population (Supplementary Tables 13-14): For each variant, we used allele frequencies of the effect allele; for meta-analyses, this was done as a range of calculations spanning the frequencies reported by individual cohorts. We used effect sizes obtained through DM and NDM SNV-eGFRcreatinine association analyses (main effect). Means and standard deviations of ln(eGFRcreatinine) were derived from UKBB subsets. Unless otherwise specified, total DM sample sizes were used.
3 Results
3.1 Clinical characteristics
Up to 34,743 individuals with diabetes mellitus (type 1 diabetes (T1D), n ~ 3,588, or type 2 diabetes (T2D), n ~ 31,155) and up to 370,061 without diabetes participated in the current study (Figure 1 and Supplementary Figure 1). Clinical characteristics of participating studies can be found in Table 1 and Supplementary Tables 4–7.
3.2 CUBN variants are not associated with eGFRcreatinine in a diabetes meta-analysis
The effect of rs144360241 on eGFRcreatinine was studied in 32,904 individuals with diabetes. The variant was not available in UK-ROI (Supplementary Figures 2, 6). All eight studies contributed to the 34,050 individuals analyzed for rs45551835 (Supplementary Figures 3 and 7). The rs141640975 variant was available for 32,993 individuals and was unavailable in UK-ROI (Supplementary Figures 4, 8). The common variant, rs1801239, was available in all eight studies in 34,070 individuals (Supplementary Figures 2, 9).
After meta-analysis, none of the four CUBN variants were significantly positively associated with eGFRcreatinine in the DM group, neither in the T1D or T2D subgroup [Table 2 (Model 1) and Table 3 (Model 2)]. However, the positive directionality of the effect for the T2D group was consistent with the directionality of effect for the combined group for all variants with non-zero effects. The T2D group carried the largest weight in the combined meta-analyses and UKBB carried the largest weight within the T2D group (Supplementary Figures 2–5). There was no evidence of heterogeneity across studies, except in model 2 for rs45551835 and rs1801239 (Table 3).
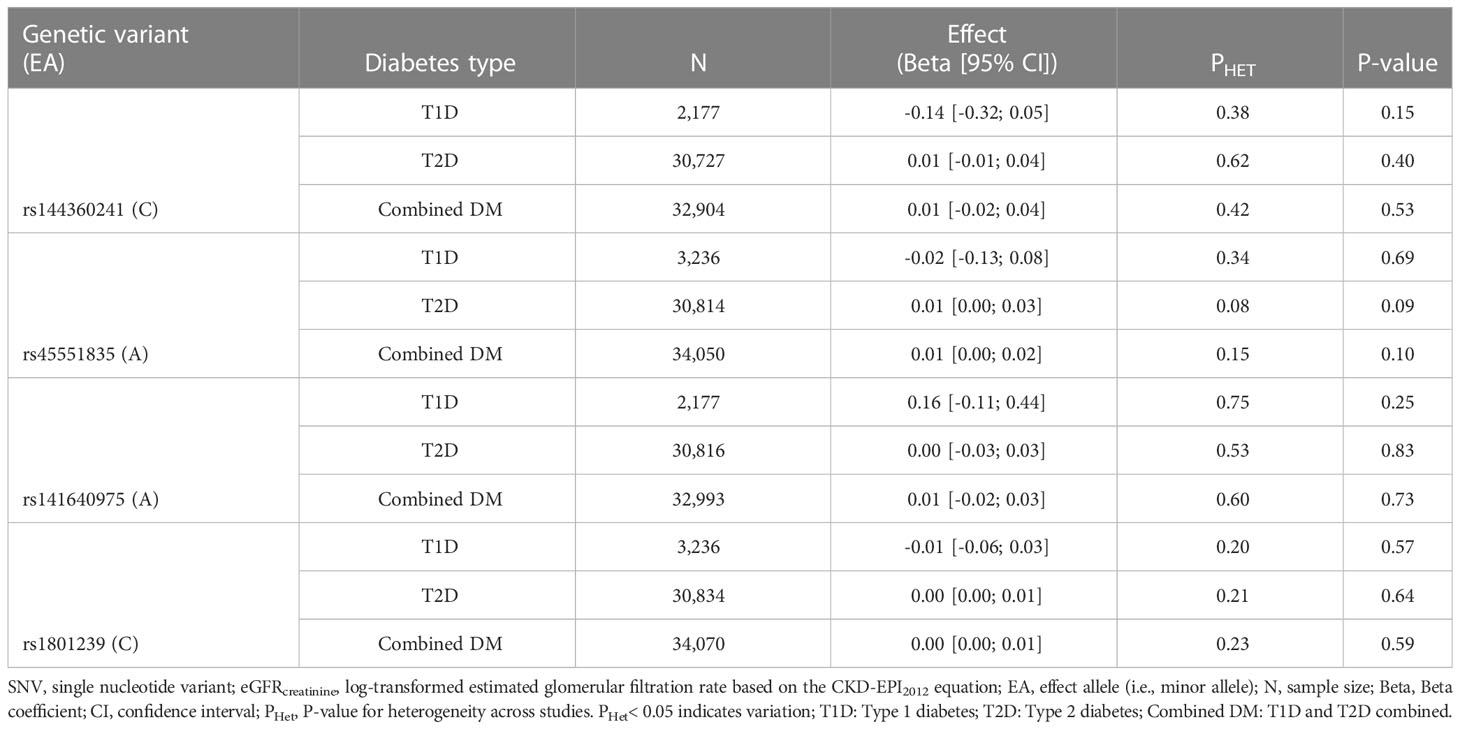
Table 2 meta-analysis of SNV-eGFRcreatinine summary data in diabetes mellitus and its subtypes (model 1).
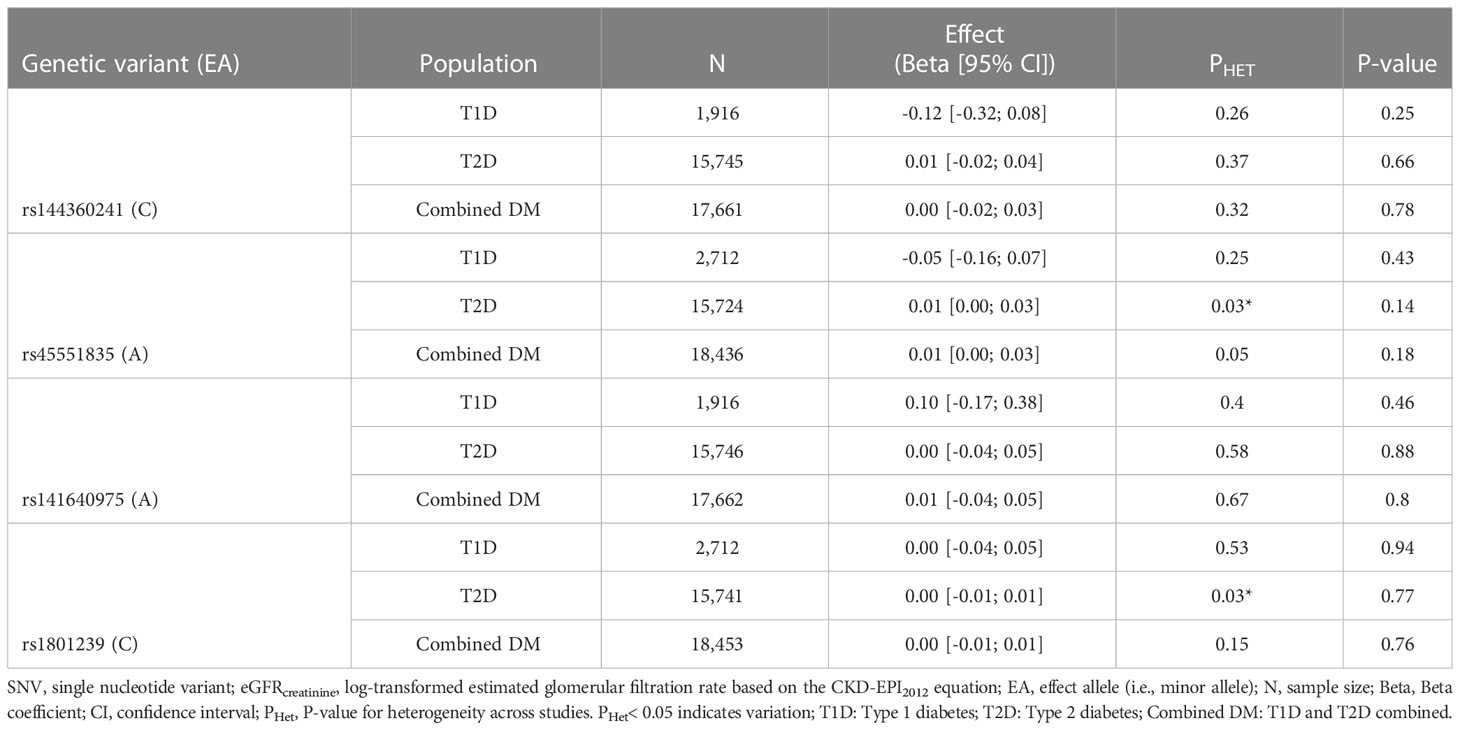
Table 3 meta-analysis of SNV-eGFRcreatinine summary data in diabetes mellitus and its subtypes (model 2).
3.3 CUBN variants are associated with higher eGFRcreatinine in non-diabetes
In UKBB-NDM, we observed larger eGFRcreatinine-levels for minor alleles compared to major alleles for all four CUBN variants in both models, except for rs1801239 in NDM, model 3 (Table 4 and Supplementary Table 8): The effect and standard deviation of rs144360241 was, for model 1 (model 3), 0.008 ± 0.002 (0.007 ± 0.002), corresponding to a difference of +0.8% (+0.7%) in mean eGFRcreatinine (ml/min/1.73 m2) for each additional copy of the affect allele, C. For rs45551835, the effect was 0.005 ± 0.001 (0.004 ± 0.001), corresponding to a difference of +0.5% (+0.4%) in mean eGFRcreatinine per copy of the A-allele. rs141640975 had the largest effect size, 0.02 ± 0.003 (0.02 ± 0.003), corresponding to a +2.02% (+2.02%) difference in mean eGFRcreatinine for each additional A-allele. The common variant, rs1801239, had the smallest effect size of 0.001 ± 0.0005, corresponding to a +0.1% difference in eGFRcreatinine for each C-allele. We replicated the finding that rs141640975 was significantly associated with higher eGFRcreatinine in non-diabetes in an Icelandic study (deCODE, n = 127,090, effect = 0.026, SE = 0.007, PeGFR_creatinine = 7.7 × 10-4, model 3, Supplementary Table 8). None of the other SNVs were replicated (data not shown). Meta-analysis for the rs141640975-eGFR-association in the NDM studies (UKBB and deCODE) is depicted in Supplementary Figure 10.
In UKBB-T2D, none of the variants had statistically significant associations with eGFRcreatinine, although the effects of three of the variants (except rs141640975) were in the same direction as in NDM (Table 4 and Supplementary Table 8).
3.4 Associations of rs141640975 with eGFRcreatinine depend on albuminuria-status in non-diabetes
To examine whether the SNVs are associated with eGFRcreatinine in an albuminuria-dependent fashion, we included albuminuria*SNV interactions in two regression models. For the first model, we observed significant interaction for rs141640975 in UKBB-NDM (Pinteraction = 0.03, Table 5). This was also observed in the other model (Pinteraction = 0.04, Supplementary Table 9). An interaction plot showed that for the eGFR-SNV-association, the effect on eGFR was even higher for more elevated albuminuria-levels (Supplementary Figure 11).
3.5 A CUBN-based GRS for albuminuria is associated with eGFRcreatinine in non-diabetes
We combined the four CUBN variants into a genetic risk score for albuminuria, verified its associations with continuous urine albumin levels and tested it against eGFRcreatinine in UKBB-T2D and UKBB-NDM. The GRS was associated with higher levels of both traits, except for eGFR in T2D (Tables 6, 7).
3.6 rs141640975 is associated with additional markers of kidney function in non-diabetes
We examined the associations between the study SNVs and two additional markers of kidney function. The SNV rs141640975 was associated with higher levels of eGFRcreatine-cystatin C [a more recent ethnicity-independent GFR-estimator (28)] and lower levels of cystatin C, both observed in NDM (Supplementary Tables 10–12). The eGFRcreatinine-cystatin C association of rs144360241 was borderline significant in NDM.
3.7 Estimated power
3.7.1 Meta-analysis (diabetes mellitus)
Given the ranges of EAFs obtained from individual studies participating in meta-analyses, we reached a power level of 35-43% for rs45551835, 16-23% for rs1444360241, and 9-21% for rs141640975 in the DM group (Supplementary Table 14). Effect sizes were assumed from the individual meta-analysis eGFRcreatinine-associations of each SNV. We did not calculate power for rs1801239 as the effect in the DM meta-analysis was 0.0.
3.7.2 Association of SNVs with eGFR (UKBB population without diabetes)
In NDM, the power for main eGFRcreatinine analyses was between 70-99% for the four variants (Supplementary Table 15).
4 Discussion
Recently, we demonstrated that individuals carrying the minor allele of the CUBN missense variant rs141640975 had higher albuminuria-levels than non-carriers. The effect of this variant was stronger in individuals with diabetes (DM) compared to those without diabetes (NDM) (11). In continuation of these findings, Bedin et al. (8) performed secondary lookups for CUBN-variants in the CKDGen eGFR GWAS study population, reporting that missense variants in CUBN may also be associated with higher levels of eGFR in the general population. Our current large-scale study aimed to examine the effect of minor alleles of three rare CUBN missense variants (rs144360241 (c.6469A>G; p.Asn2157Asp), rs45551835 (c.8741C>T; p.Ala2914Val) and rs141640975 (c.5069C>T; p.Ala1690Val)) and one common variant (rs1801239 (c.8950A>G; p.Ile2984Val)) on eGFRcreatinine levels separately in people with and without diabetes (nDM ~ 34,000 individuals, nNDM ~ 370,000 individuals), including stratification for diabetes-type and supplemented by tests on circulating cystatin C levels, the recently updated eGFR-equation based on creatinine and cystatin C (30), and aggregate-variant tests. We were able to replicate the association between creatinine-based eGFR and rs141640975 in NDM and report new insightful connections with the alternative measures of kidney function for all four SNVs.
Previously, a borderline association between rs45551835 and higher eGFR-levels has been reported in a smaller type 2 diabetes (T2D) population from Denmark (8, 11), a finding which we could not replicate in our meta-analysis of up to 34,432 individuals with diabetes and its subtypes. Like the initial study (8), we could not establish a link between eGFR and the three other variants within the diabetes group. As for rs45551835, it was surprising to be unable to replicate the earlier findings as the current study has a larger sample size compared to earlier efforts. Our post-hoc power assessment indicated that insufficient power might be at play, even with a larger sample size for the diabetes group (8). We also speculated whether the apparent lack of association between CUBN and eGFR in our diabetes meta-analysis could be due to use of Angiotensin receptor blockers (ARBs) or Angiotensin-converting enzyme inhibitor (ACEi) medication which is frequently used in diabetes treatment. As part of our sensitivity analyses, we included models adjusted for systolic blood pressure (a proxy for such medication) and did not find evidence that this could explain why no association was found in the diabetes group. Another reason could be the allele frequency of the variants may differ between Danish and UK populations. We need further validation in well-powered populations to confirm the relationship between the rs45551835 and eGFR in diabetes, especially in T2D. In case of a true lack of association, CUBN may be associated with higher levels of urine albumin (11) with no pleiotropic effect to eGFR in this population.
We proceeded to single- and aggregate-variant analyses in the UK Biobank (UKBB), shifting focus to non-diabetes populations. For all four CUBN variants, we report significantly higher eGFRcreatinine-levels in individuals without diabetes harboring more copies of the minor alleles compared to individuals with fewer or no copies of the minor alleles in the same group. For rs141640975, we observed the strongest association with eGFRcreatinine (P = 2.2 × 10-9) with replication in the Icelandic study (deCODE, P = 7.7 × 10-4), confirming what has previously been observed for this SNV in NDM (8) – but also a significant interaction between the SNV and albuminuria stages (PINT< 0.05). Taken together with the already known associations of the minor alleles with higher albuminuria (11), this not only demonstrates genetic pleiotropy of CUBN for albuminuria and eGFR in non-diabetes but also implies that these two associations are intertwined for this SNV, where the effect on eGFR is even higher for more elevated albuminuria-levels. Here, CUBN demonstrates a classic genetic pleiotropy phenomenon where a DNA variant influences multiple traits, usually in the same domain with concordant or sometimes discordant effects as observed earlier in complex disorders (32). Further validation of independent biological or related causal effects might be required in additional follow up studies.
This finding is unusual as there is no obvious clinical or pathophysiological explanation for such an albuminuria-eGFR pattern in the context of non-diabetes. It has been suggested that the tubular albuminuria observed in presence of C-terminal variants in CUBN has a benign or even slightly protective effect on kidney function in chronic kidney disease if glomerular albuminuria is also present (8, 33, 34). Another recent study on chronic isolated proteinuria suggests that different C-terminal CUBN variants uncouple proteinuria from glomerular filtration barrier through declined cubilin expression accompanied by aberrant amnionless (AMN) localization in renal tubules. AMN is part of the receptor complex (along with cubilin and megalin) necessary for tubular reabsorption of albumin. This is suggested to create a benign condition, not requiring any further proteinuria lowering treatment (35). In non-diabetes, where the population can be assumed to consist mostly of healthy individuals, a concept of such protectiveness is less relevant. However, it is possible that an undetected subpopulation with relevant comorbidities exists in the non-diabetes group.
Our CUBN aggregate-variant method – which was defined as a genetic risk score (GRS) combining the four variants – showed that a higher number of C-terminal CUBN risk alleles is associated with higher urine albumin and eGFRcreatinine levels and confirms both the single-variant association with higher urine albumin levels reported previously in diabetes and non-diabetes (11, 14), and the consistency of the overall effects on urine albumin levels being greater in diabetes compared to non-diabetes (10, 11). Through GRSCUBN, we also saw that a higher number of minor alleles across the four variants was associated with higher eGFRcreatinine-levels in the UKBB population without diabetes, which is in line with our single-variant findings and the previous findings for rs45551835 (8). Using aggregate-variant methods is an optimal way to examine combined genetic effects and has been used extensively for polygenic traits (13, 36). Using GRS is highly relevant here as three of the four variants are rare and mostly present as heterozygous variants in our populations. This might substantiate with some additional power to detect effects and adds further certainty to the presence of a CUBN-eGFR relationship in non-diabetes. Nevertheless, we still do not find an association with eGFR in T2D, even when the variants are combined in a GRS.
Finally, we examined the association between the study SNVs and two alternative markers of kidney function. In non-diabetes, the minor alleles of rs141640975 and rs144360241 were associated with higher levels of eGFRcreatinine-cystatin C. This measure was estimated using a recent update to the equation, CKD-EPI2021, which does not include ethnicity and is a more precise indicator of kidney function in comparison to the CKD-EPIcreatinine(2012) equation which is based only on creatinine. Our results using the conventional eGFRcreatinine equation are concordant with our results from the updated equation in terms of directionality of effect and with our finding that rs141640975 is associated with lower cystatin C levels, which is another indicator of kidney function. It should be noted, though, that considering Table 1 and Supplementary Tables 4–6, the 0.1% – 2.02% higher mean eGFR we report for each minor allele is modest and may reflect that individual harboring these genetic variants have normal kidney function rather than a better kidney function.
A strength of our study is the restriction to specifically diabetes- and non-diabetes-only subgroups so that effects from mixed diabetes-status are minimized. Heterogeneity is likely to be present in meta-analyses of a diverse set of cohorts originally used for different research purposes. Indeed, some of the cohorts included in our meta-analyses differ regarding available covariates and/or kidney disease status. However, we did not observe heterogeneity in our meta-analyses. In addition to this, we could minimize heterogeneity in the remainder of our analyses by using data from the UKBB, which is a nationally representative cohort facilitating application of identical phenotype definitions across subgroups. Another strength is the broad spectrum of additional analyses that we explored in the UKBB population to nuance our findings on the relationship between eGFR and CUBN. The judicious use of UKBB leveraging individual-level genotype information to investigate interaction-analyses based on albuminuria groupings is a great strength of the current study, especially for rare variants.
A major limitation is that we did not have sufficient statistical power for our meta-analyses in the diabetes group due to the limited availability of suitable datasets. Consequently, interpretations of T2D findings should not be overstated and we thus could not demonstrate, nor disprove, the presence of a CUBN-eGFR relationship in this population. Although we demonstrate that C-terminal missense variants in CUBN are associated with different measures of normal (or even higher) kidney function in non-diabetes, we emphasize that the current study is insufficient to establish causality. Finally, using multiple-testing-corrected significance thresholds might be too conservative when testing a very small number of variants from the same locus as it may remove true associations. In genome-wide studies, a conservative threshold of 5 × 10−8 is generally agreed upon for novel associations. There is less consensus on when and how to appropriately apply multiple testing correction in smaller-scale genetic studies dealing with a mixture of new and known associations. Nevertheless, we deemed that it would be fair to apply FDR-correction of the significance threshold to our primary analyses in DM and NDM.
In conclusion, the current study identifies the existence of pleiotropic genetic effects of CUBN on two facets of kidney function – albuminuria and eGFR – by reporting SNV-eGFR associations in a large study population without diabetes. The interaction between rs141640975 and albuminuria-status on eGFRcreatinine in this population and its associations with lower cystatin C and higher levels of eGFRcreatinine-cystatin C expands our knowledge of these variants in relation to measures of kidney function. The demonstration of a CUBN-focused GRS in relation to albuminuria and eGFRcreatinine further suggests an important role of CUBN-variants in the future personalization of chronic kidney disease management.
Data availability statement
The original contributions presented in the study are included in the article/Supplementary Material. Further inquiries can be directed to the corresponding author.
Ethics statement
The studies involving human participants were reviewed and approved by Ethical approval has previously been obtained locally for each individual study. The patients/participants provided their written informed consent to participate in this study.
Author contributions
NU, MS, PR, and TA contributed to conception and design of the study. NU wrote the first draft of the manuscript.NU, MC-G, CH, AN, AO, SS, D-AT, EA, MH, AM, EJS, MS, PR, and TA contributed to manuscript revision. D-AT, VS, KS, EA, MH, AM, PR and TA acquired data. NU, FA, MC-G, CH, AN, SS, LS, D-AT, and TA performed statistical analysis. NU, MC-G, LS, D-AT, AM, MS, PR, and TA contributed to interpretation of data. TA and MS acquired funding and TA administered this project. PR and TA supervised the project. LC and MG had other roles. All authors contributed to the article and approved the submitted version.
Funding
NU, MS, and TA were supported by the Novo Nordisk Foundation, Steno Collaborative Grant (NNF18OC0052457). TA and PR were supported by Steno Diabetes Center Copenhagen, Herlev, Denmark. Funding of individual studies contributing to this project can be found in Supplementary Table 16.
Acknowledgments
This research has been conducted using data from UK Biobank database (www.ukbiobank.ac.uk), project ID numbers, 32683 and 71699. Acknowledgements of individual studies can be found in Supplementary Table 16.
Conflict of interest
Author MC-G was employed by Pfizer-University of Granada-Andalusian Regional Government. Authors AO, VS, and KS were employed by Amgen, Inc. PR reports personal fees from Bayer during the conduct of the study. He has received research support and personal fees from AstraZeneca and Novo Nordisk, and personal fees from Astellas Pharma, Boehringer Ingelheim, Eli Lilly, Gilead Sciences, Mundipharma, Sanofi, and Vifor Pharma. All fees are given to Steno Diabetes Center Copenhagen.
The remaining authors declare that the research was conducted in the absence of any commercial or financial relationships that could be construed as a potential conflict of interest.
Publisher’s note
All claims expressed in this article are solely those of the authors and do not necessarily represent those of their affiliated organizations, or those of the publisher, the editors and the reviewers. Any product that may be evaluated in this article, or claim that may be made by its manufacturer, is not guaranteed or endorsed by the publisher.
Supplementary material
The Supplementary Material for this article can be found online at: https://www.frontiersin.org/articles/10.3389/fendo.2023.1081741/full#supplementary-material
Abbreviations
ACEi, Angiotensin-converting enzyme inhibitors; AER, Albumin excretion rate (mg/24 hours); ALB, Urinary albumin level (mg/L); ARBs, Angiotensin receptor blockers; CKD, Chronic kidney disease; CKD-EPI, CKD Epidemiology Collaboration; CUBN, The gene encoding cubilin; DM, Diabetes mellitus; eGFR, Estimated glomerular filtration rate (ml/min/1.73 m2); GRS, Genetic risk score; NDM, Non-diabetes; T1D, Type 1 diabetes; T2D, Type 2 diabetes; UACR, Urinary albumin-creatinine ratio (mg/mmol); UKBB, UK Biobank.
References
1. Amsellem S, Gburek J, Hamard G, Nielsen R, Willnow TE, Devuyst O, et al. Cubilin is essential for albumin reabsorption in the renal proximal tubule. J Am Soc Nephrol (2010) 21:1859–67. doi: 10.1681/ASN.2010050492
2. Yang J, Xu Y, Deng L, Zhou L, Qiu L, Zhang Y, et al. CUBN gene mutations may cause focal segmental glomerulosclerosis (FSGS) in children. BMC Nephrol (2022) 23:15. doi: 10.1186/s12882-021-02654-x
3. Christensen EI, Nielsen R, Birn H. From bowel to kidneys: The role of cubilin in physiology and disease. Nephrol Dial Transplant (2013) 28:274–81. doi: 10.1093/ndt/gfs565
4. Deckert T, Feldt-Rasmussen B, Borch-Johnsen K, Jensen T, Kofoed-Enevoldsen A. Albuminuria reflects widespread vascular damage. Steno hypothesis Diabetologia (1989) 32:219–26. doi: 10.1007/BF00285287
5. International Diabetes Federation. IDF Diabetes Atlas, 10th edn. Brussels, Belgium: International Diabetes Federation. (2021), 2021.
6. Kufeldt J, Kovarova M, Adolph M, Staiger H, Bamberg M, Haring HU, et al. Prevalence and distribution of diabetes mellitus in a maximum care hospital: Urgent need for HbA1c-screening. Exp Clin Endocrinol Diabetes (2018) 126:123–9. doi: 10.1055/s-0043-112653
7. Alicic RZ, Rooney MT, Tuttle KR. Diabetic kidney disease: Challenges, progress, and possibilities. Clin J Am Soc Nephrol (2017) 12:2032–45. doi: 10.2215/CJN.11491116
8. Bedin M, Boyer O, Servais A, Li Y, Villoing-Gaude L, Tete MJ, et al. Human c-terminal CUBN variants associate with chronic proteinuria and normal renal function. J Clin Invest (2020) 130:335–44. doi: 10.1172/JCI129937
9. Boger CA, Chen MH, Tin A, Olden M, Kottgen A, de Boer IH, et al. CUBN is a gene locus for albuminuria. J Am Soc Nephrol (2011) 22:555–70. doi: 10.1681/ASN.2010060598
10. Casanova F, Tyrrell J, Beaumont RN, Ji Y, Jones SE, Hattersley AT, et al. A genome-wide association study implicates multiple mechanisms influencing raised urinary albumin-creatinine ratio. Hum Mol Genet (2019) 28:4197–207. doi: 10.1093/hmg/ddz243
11. Ahluwalia TS, Schulz CA, Waage J, Skaaby T, Sandholm N, van Zuydam N, et al. A novel rare CUBN variant and three additional genes identified in europeans with and without diabetes: Results from an exome-wide association study of albuminuria. Diabetologia (2019) 62:292–305. doi: 10.1007/s00125-018-4783-z
12. Haas ME, Aragam KG, Emdin CA, Bick AG, International Consortium for Blood P, Hemani G, et al. Genetic association of albuminuria with cardiometabolic disease and blood pressure. Am J Hum Genet (2018) 103:461–73. doi: 10.1016/j.ajhg.2018.08.004
13. Teumer A, Li Y, Ghasemi S, Prins BP, Wuttke M, Hermle T, et al. Genome-wide association meta-analyses and fine-mapping elucidate pathways influencing albuminuria. Nat Commun (2019) 10:4130. doi: 10.1038/s41467-019-11576-0
14. Teumer A, Tin A, Sorice R, Gorski M, Yeo NC, Chu AY, et al. Genome-wide association studies identify genetic loci associated with albuminuria in diabetes. Diabetes (2016) 65:803–17. doi: 10.2337/db15-1313
15. Charmet R, Duffy S, Keshavarzi S, Gyorgy B, Marre M, Rossing P, et al. Novel risk genes identified in a genome-wide association study for coronary artery disease in patients with type 1 diabetes. Cardiovasc Diabetol (2018) 17:61. doi: 10.1186/s12933-018-0705-0
16. Dahlstrom EH, Saksi J, Forsblom C, Uglebjerg N, Mars N, Thorn LM, et al. The low-expression variant of FABP4 is associated with cardiovascular disease in type 1 diabetes. Diabetes (2021) 70:2391–401. doi: 10.2337/db21-0056
17. Winther SA, Ollgaard JC, Tofte N, Tarnow L, Wang Z, Ahluwalia TS, et al. Utility of plasma concentration of trimethylamine n-oxide in predicting cardiovascular and renal complications in individuals with type 1 diabetes. Diabetes Care (2019) 42:1512–20. doi: 10.2337/dc19-0048
18. Lajer M, Jorsal A, Tarnow L, Parving HH, Rossing P. Plasma growth differentiation factor-15 independently predicts all-cause and cardiovascular mortality as well as deterioration of kidney function in type 1 diabetic patients with nephropathy. Diabetes Care (2010) 33:1567–72. doi: 10.2337/dc09-2174
19. Ikram MA, Brusselle G, Ghanbari M, Goedegebure A, Ikram MK, Kavousi M, et al. Objectives, design and main findings until 2020 from the Rotterdam study. Eur J Epidemiol (2020) 35:483–517. doi: 10.1007/s10654-020-00640-5
20. van Herpt TTW, Lemmers RFH, van Hoek M, Langendonk JG, Erdtsieck RJ, Bravenboer B, et al. Introduction of the DiaGene study: Clinical characteristics, pathophysiology and determinants of vascular complications of type 2 diabetes. Diabetol Metab Syndr (2017) 9:47. doi: 10.1186/s13098-017-0245-x
21. McKnight AJ, Patterson CC, Pettigrew KA, Savage DA, Kilner J, Murphy M, et al. A GREM1 gene variant associates with diabetic nephropathy. J Am Soc Nephrol (2010) 21:773–81. doi: 10.1681/ASN.2009070773
22. Hadjadj S, Pean F, Gallois Y, Passa P, Aubert R, Weekers L, et al. Different patterns of insulin resistance in relatives of type 1 diabetic patients with retinopathy or nephropathy: the genesis France-Belgium study. Diabetes Care (2004) 27:2661–8. doi: 10.2337/diacare.27.11.2661
23. Mansour Aly D, Dwivedi OP, Prasad RB, Karajamaki A, Hjort R, Thangam M, et al. Genome-wide association analyses highlight etiological differences underlying newly defined subtypes of diabetes. Nat Genet (2021) 53:1534–42. doi: 10.1038/s41588-021-00948-2
24. Bycroft C, Freeman C, Petkova D, Band G, Elliott LT, Sharp K, et al. The UK biobank resource with deep phenotyping and genomic data. Nature (2018) 562:203–9. doi: 10.1038/s41586-018-0579-z
25. Eastwood SV, Mathur R, Atkinson M, Brophy S, Sudlow C, Flaig R, et al. Algorithms for the capture and adjudication of prevalent and incident diabetes in UK biobank. PloS One (2016) 11:e0162388. doi: 10.1371/journal.pone.0162388
26. Noordam R, Lall K, Smit RAJ, Laisk T, Estonian Biobank Research T, Metspalu A, et al. Stratification of type 2 diabetes by age of diagnosis in the UK biobank reveals subgroup-specific genetic associations and causal risk profiles. Diabetes (2021) 70:1816–25. doi: 10.2337/db20-0602
27. Common Metabolic Diseases Knowledge Portal. Available at: https://hugeamp.org/. Accessed 10 Jul 2020. 2020.
28. Inker LA, Schmid CH, Tighiouart H, Eckfeldt JH, Feldman HI, Greene T, et al. Investigators, Estimating glomerular filtration rate from serum creatinine and cystatin C. N Engl J Med (2012) 367:20–9.
29. Machiela MJ, Chanock SJ. LDlink: A web-based application for exploring population-specific haplotype structure and linking correlated alleles of possible functional variants. Bioinformatics (2015) 31:3555–7. doi: 10.1093/bioinformatics/btv402
30. Inker LA, Eneanya ND, Coresh J, Tighiouart H, Wang D, Sang Y, et al. New creatinine- and cystatin c-based equations to estimate GFR without race. N Engl J Med (2021) 385:1737–49. doi: 10.1056/NEJMoa2102953
31. Gauderman WJ. Sample size requirements for matched case-control studies of gene-environment interaction. Stat Med (2002) 21:35–50. doi: 10.1002/sim.973
32. Gratten J, Visscher PM. Genetic pleiotropy in complex traits and diseases: implications for genomic medicine. Genome Med (2016) 8:78. doi: 10.1186/s13073-016-0332-x
33. Beenken A, Barasch JM, Gharavi AG. Not all proteinuria is created equal. J Clin Invest (2020) 130:74–6. doi: 10.1172/JCI133250
34. Quinlan C. CUBN variants uncouple proteinuria from kidney function. Nat Rev Nephrol (2020) 16:135–6. doi: 10.1038/s41581-019-0242-4
35. Gan C, Zhou X, Chen D, Chi H, Qiu J, You H, et al. Novel pathogenic variants in CUBN uncouple proteinuria from renal function. J Transl Med (2022) 20:480. doi: 10.1186/s12967-022-03706-y
Keywords: genetics, CUBN, cubilin, kidney function, eGFR, diabetes, non-diabetes, chronic kidney disease (CKD)
Citation: Uglebjerg N, Ahmadizar F, Aly DM, Cañadas-Garre M, Hill C, Naber A, Oddsson A, Singh SS, Smyth L, Trégouët D-A, Chaker L, Ghanbari M, Steinthorsdottir V, Ahlqvist E, Hadjadj S, Van Hoek M, Kavousi M, McKnight AJ, Sijbrands EJ, Stefansson K, Simons M, Rossing P and Ahluwalia TS (2023) Four missense genetic variants in CUBN are associated with higher levels of eGFR in non-diabetes but not in diabetes mellitus or its subtypes: A genetic association study in Europeans. Front. Endocrinol. 14:1081741. doi: 10.3389/fendo.2023.1081741
Received: 27 October 2022; Accepted: 07 February 2023;
Published: 28 February 2023.
Edited by:
Anandwardhan Hardikar, Western Sydney University, AustraliaReviewed by:
Sameet Mehta, Yale University, United StatesMarija Petkovic, Roskilde University, Denmark
Jalal Taneera, University of Sharjah, United Arab Emirates
Copyright © 2023 Uglebjerg, Ahmadizar, Aly, Cañadas-Garre, Hill, Naber, Oddsson, Singh, Smyth, Trégouët, Chaker, Ghanbari, Steinthorsdottir, Ahlqvist, Hadjadj, Van Hoek, Kavousi, McKnight, Sijbrands, Stefansson, Simons, Rossing and Ahluwalia. This is an open-access article distributed under the terms of the Creative Commons Attribution License (CC BY). The use, distribution or reproduction in other forums is permitted, provided the original author(s) and the copyright owner(s) are credited and that the original publication in this journal is cited, in accordance with accepted academic practice. No use, distribution or reproduction is permitted which does not comply with these terms.
*Correspondence: Tarunveer S. Ahluwalia, dGFydW4udmVlci5zaW5naC5haGx1d2FsaWFAcmVnaW9uaC5kaw==
†ORCID: Nicoline Uglebjerg, orcid.org/0000-0001-8874-0895
Dina M. Aly, orcid.org/0000-0002-9510-4691
Marisa Cañadas-Garre, orcid.org/0000-0003-1103-5658
Claire Hill, orcid.org/0000-0002-2481-5162
Annemieke Naber, orcid.org/0000-0002-2875-9858
Asmundur Oddsson, orcid.org/0000-0002-4606-5163
Laura Smyth, orcid.org/0000-0001-6419-4826
David-Alexandre Trégouët, orcid.org/0000-0001-9084-7800
Valgerdur Steinthorsdottir, orcid.org/0000-0003-1846-6274
Emma Ahlqvist, orcid.org/0000-0002-6513-2384
Mandy Van Hoek, orcid.org/0000-0002-2957-5436
Amy Jayne McKnight, orcid.org/0000-0002-7482-709X
Eric J. Sijbrands, orcid.org/0000-0001-8857-7389
Kari Stefansson, orcid.org/0000-0003-1676-864X
Peter Rossing, orcid.org/0000-0002-1531-4294
Tarunveer S. Ahluwalia, orcid.org/0000-0002-7464-3354