- 1School of Nursing, Chengdu Medical College, Chengdu, China
- 2Clinical Medical College of Chengdu Medical College, First Affiliated Hospital, Chengdu, China
- 3Department of Endocrinology and Metabolism, The First Affiliated Hospital of Chengdu Medical College, Chengdu, China
- 4Department of Rheumatology and Immunology, The First Affiliated Hospital of Chengdu Medical College, Chengdu, China
Aim: The present study aims to utilize structural equation modeling (SEM) to investigate the factors impacting long-term glycemic variability among patients afflicted with type 2 diabetes.
Method: The present investigation is a retrospective cohort study that involved the collection of data on patients with type 2 diabetes mellitus who received care at a hospital located in Chengdu, Sichuan Province, over a period spanning from January 1, 2013, to October 30, 2022. Inclusion criteria required patients to have had at least three laboratory test results available. Pertinent patient-related information encompassing general demographic characteristics and biochemical indicators was gathered. Variability in the dataset was defined by standard deviation (SD) and coefficient of variation (CV), with glycosylated hemoglobin variation also considering variability score (HVS). Linear regression analysis was employed to establish the structural equation models for statistically significant influences on long-term glycemic variability. Structural equation modeling was employed to analyze effects and pathways.
Results: Diabetes outpatient special disease management, uric acid variability, mean triglyceride levels, mean total cholesterol levels, total cholesterol variability, LDL variability, baseline glycated hemoglobin, and recent glycated hemoglobin were identified as significant factors influencing long-term glycemic variability. The overall fit of the structural equation model was found to be satisfactory and it was able to capture the relationship between outpatient special disease management, biochemical indicators, and glycated hemoglobin variability. According to the total effect statistics, baseline glycated hemoglobin and total cholesterol levels exhibited the strongest impact on glycated hemoglobin variability.
Conclusion: The factors that have a significant impact on the variation of glycosylated hemoglobin include glycosylated hemoglobin itself, lipids, uric acid, and outpatient special disease management for diabetes. The identification and management of these associated factors can potentially mitigate long-term glycemic variability, thereby delaying the onset of complications and enhancing patients’ quality of life.
1 Introduction
The prevalence of diabetes has increased threefold over the past 30 years (1), making it one of the most prevalent and rapidly growing illnesses. By 2045, it is projected that diabetes will affect 12.2% of the population (783.2 million) worldwide (2). In China, the prevalence rate of diabetes has reached 11.2% since 2015-2017, with type 2 diabetes accounting for over 90% of cases (3). Globally, diabetes is the ninth leading cause of mortality (1), accounting for 4.2 million deaths in 2019 alone (4), which is 2-3 times higher than in non-diabetic individuals (5). Morbidity and mortality in diabetes are mainly caused by cardiovascular disease, diabetic nephropathy, retinopathy, and neuropathy (6). Hospitalization costs for diabetic patients vary widely across different Asian countries and can range from 11% to 75% of per capita income, with three times higher costs for patients with complications (7). In 2019, the global cost of direct treatment for diabetes was estimated to be $760 billion, with China alone spending $109 billion (8). Moreover, hospitalization costs were found to be three times higher for diabetic patients with complications than for those without complications (7).
Glycosylated hemoglobin (HbA1c) is widely used as the benchmark for glycemic control and treatment of diabetic patients in clinical settings (3). The primary therapeutic goal for diabetic patients is to maintain blood glucose levels to prevent complications (9). However, diabetes experts agree that the extent of glycemic fluctuations among diabetic patients with similar HbA1c management may affect their risk of complications (10). Numerous studies have established a correlation between glycemic variability (GV) and diabetic complications (11–13), and it has been established that GV is a more clinically significant glycemic control indicator than HbA1c (14). Bergenstal et al. (15) demonstrated that GV refers to the fluctuations in blood glucose levels, primarily reflecting a decline in pancreatic β-cell function and is more detrimental to diabetic patients than persistent and stable hyperglycemia; it encompasses short-term and long-term glycemic variability, with short-term GV being characterized by intra-day or inter-day fluctuations in blood glucose that ultimately result in an increase in HbA1c (16). Long-term glycemic variability refers to the inconsistency of HbA1c values as they fluctuate between peaks and troughs (11). Therefore, optimal glycemic control for diabetic patients involves targeting HbA1c levels while also minimizing HbA1c fluctuation as much as possible (10).
In recent years, long-term glucose variability has garnered considerable attention from experts and clinicians (17). An increasing number of studies utilize it as a primary determinant of glycemic control quality (18). The glucose standard deviation (SD) and coefficient of variation (CV) are currently the most commonly employed measures (18). Recently, novel research has proposed a new metric, the HbA1c variability score (HVS), which calculates the proportion of HbA1c variations exceeding 0.5% from the previous measurement, divided by the total number of HbA1c measurements, to evaluate long-term glucose fluctuations (19, 20).
The degree of fluctuation in HbA1c, as opposed to absolute blood glucose levels, is strongly associated with unfavorable outcomes (21). Among diabetic patients, HbA1c variability is a risk factor for mortality and death due to cardiovascular disease (22, 23), and it is independent of HbA1c levels (24). In addition, numerous observational and randomized clinical trial studies have demonstrated that HbA1c variability is linked to the risk of both microvascular and macrovascular complications in diabetes patients, as well as increased occurrences of hypoglycemia (12, 19, 25–27). Furthermore, a meta-analysis revealed that HbA1c variability is connected with the incidence of dementia among diabetic patients (28). Therefore, the degree of HbA1c fluctuation may play a crucial role in the clinical assessment of risks related to complications and mortality.
Given the well-established connection between HbA1c variability and diabetic complications and mortality, reducing HbA1c variability should be a target of glycemic management for diabetic patients (29). To effectively reduce HbA1c variability in clinical practice, it is crucial to comprehend the factors that impact it in order to more successfully control HbA1c variability, delay the onset of complications, lower mortality rates in diabetic patients, and improve disease prognosis and quality of life. However, there is a dearth of trustworthy literature on the factors that influence HbA1c variability. Consequently, the purpose of this study is to perform a retrospective analysis to investigate the present status of HbA1c variability and its influencing factors, as well as to establish a reference basis for clinical concepts on strategies to decrease HbA1c variability in later stages.
2 Methods
2.1 Study design and participants
Clinical data of patients were obtained from the outpatient system of the Department of Endocrinology at a tertiary care hospital located in Chengdu for the purpose of collecting data from type 2 diabetes mellitus patients who were managed in the outpatient clinic from 2013 to 2022. Inclusion criteria were as follows: (1) meeting the diagnostic and classification criteria for type 2 diabetes according to the 1999 version of the World Health Organization (WHO); (2) age at the time of initial diagnosis was 18 years or older; and (3) at least three laboratory test findings were available. Exclusion criteria comprised: (1) the presence of other serious chronic diseases affecting the heart, liver, kidneys, brain, and lungs at the time of inclusion; (2) missing laboratory test data; (3) missing general data; and (4) presence of other endocrine diseases, such as thyroid, pituitary, and adrenal diseases, at the time of initial diagnosis.
2.2 Data collection
The medical records of patients receiving outpatient care at a tertiary hospital in Chengdu’s endocrinology department were reviewed to extract clinical data, including demographic variables such as gender and age, as well as uric acid levels, and other variables including triglycerides (TG), total cholesterol (TC), high-density lipoprotein (HDL), low-density lipoprotein (LDL), blood glucose levels, and glycated hemoglobin (HbA1c). The patients were also categorized based on their enrollment in the Outpatient Special Disease Management program, which involved regular HbA1C measurements, health education, medication prescriptions, and timely adjustments by a diabetes specialist. By organizing and analyzing the data, the variability and correlation values of each variable were determined.
2.3 Statistical analysis
The study data were exported from the EpiData (Chinese version) management software and subjected to analysis using IBM SPSS 26.0 and IBM SPSS AMOS 28.0 software. The Origin 2022 software was utilized for graphing purposes. Quantitative data were expressed in terms of mean ± standard deviation, while qualitative data were expressed as count and percentage (%). Linear regression analysis was employed to evaluate the impact of type 2 diabetes data on long-term glycemic variability. Additionally, structural equation modeling using AMOS 28.0 software was performed to identify the factors influencing long-term glycemic variability, and analyzed the power with power4SEM program. The goodness of fit index (GFI), incremental fit index (IFI), comparative fit index (CFI), standardized fit index (NFl), relative fit index (RFI), and non-normalized fit index (TLI) were used to evaluate the model fit. The values of IFI, CFI, NFI, RFI, and TLI were all greater than 0.9, while root mean square error of approximation (RMSEA) was less than 0.08, and χ2/df was less than 3 (30). Statistical significance was set at P<0.05.
2.4 Ethical considerations
The study was approved by the Ethics Committee of First Affiliated Hospital of Chengdu Medical College (approval no. 2021-07), and it was carried out in accordance with the Code of Ethics of the World Medical Association (Declaration of Helsinki).
3 Result
3.1 Participant characteristics
This study comprised 369 subjects, the majority of whom were 40 years of age or older (95.7%). They had a follow-up duration of at least 4 years for diabetes mellitus management (69.6%), and were treated as outpatient special diseases (69.9%). A considerable proportion of subjects had comorbidities (83.7%) and multiple complications (85.1%). Table 1 provides a comprehensive summary of the findings.
3.2 HbA1c variant distribution characteristics
In this study, the mean and standard deviation of HbA1C-HVS were 0.529 ± 0.285, HbA1C-SD were 0.833 ± 0.712, and HbA1C-CV were 0.109 ± 0.079. Detailed results can be found in Table 2. Specifically, 64.50% of HbA1C-HVS values ranged from 25.00% to 75.00%, 69.11% of HbA1c-SD values ranged from 0.35 to 1.50, and 58.14% of HbA1c-CV values were between 0.05 and 0.15. The distribution of each data type among different patients is illustrated in Figure 1.
3.3 Biochemical index variability in T2DM patients: descriptive statistics
In this investigation, the mean values and standard deviations for the variability of biochemical markers were determined as follows: UA-SD (51.509 ± 33.448), UA-CV (0.160 ± 0.106), TG-SD (0.977 ± 2.774), TG-CV (0.382 ± 0.349), TC-SD (0.677 ± 0.483), TC-CV (0.165 ± 0.088), LDL-SD (0.578 ± 0.548), LAL-CV (0.268 ± 0.154), HDL-CV (0.139 ± 0.196), HDL-SD (0.354 ± 3.509). Further elaboration of the results can be found in Table 3.
3.4 One-way logistic regression analysis of factors influencing HbA1c variants in T2DM patients
In this investigation, it was observed that specific outpatient disease management, changes in uric acid levels, average triglyceride and total cholesterol levels, variability of total cholesterol and LDL, as well as baseline and recent glycation, were significant contributing factors to the variation in HbA1C among patients with type 2 diabetes (P<0.05). The results of the study are presented in Table 4, while Table 5 provides the details of the variable assignments.
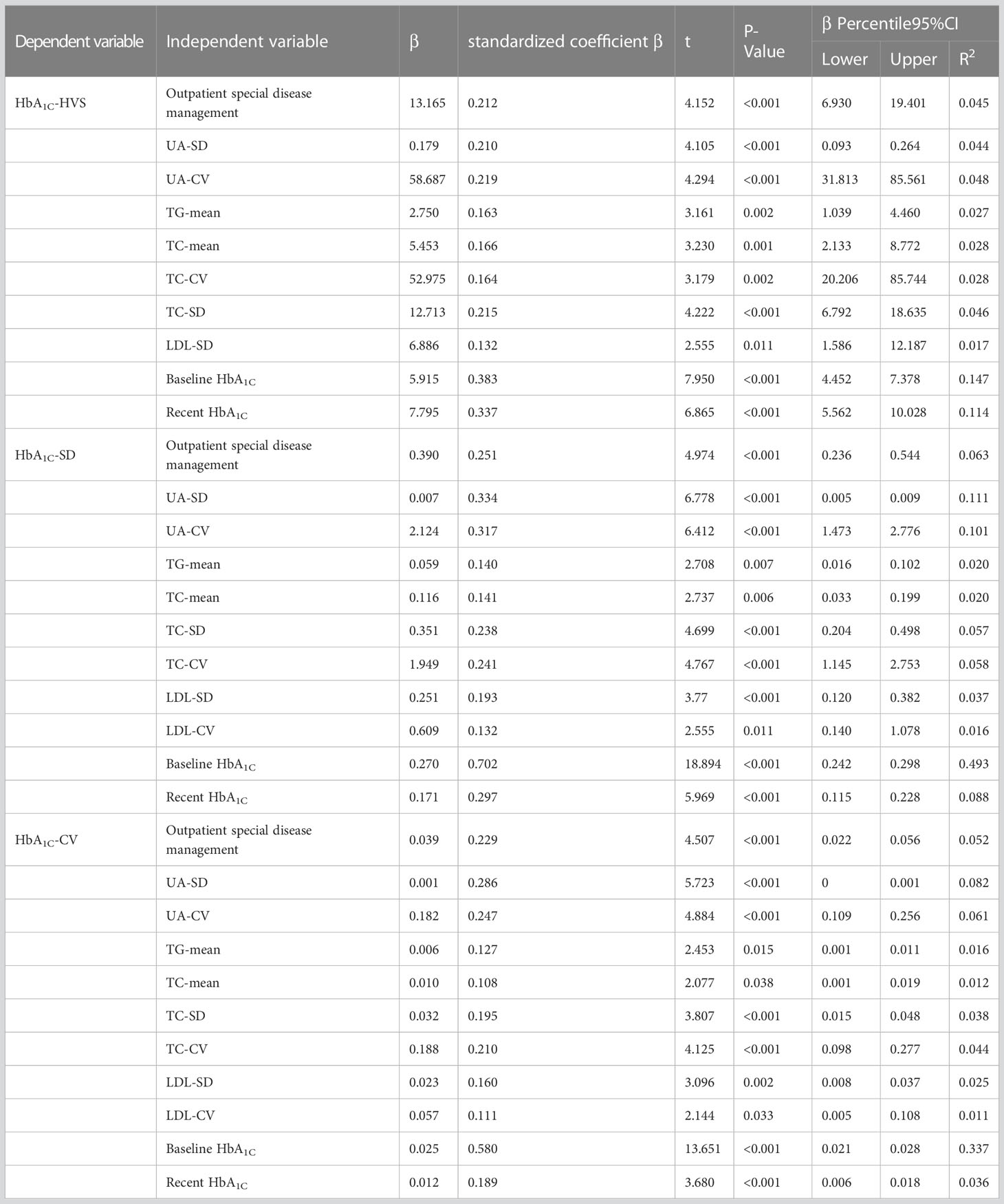
Table 4 Linear regression analysis of risk factors for HbA1C variants in patients with type 2 diabetes.
3.5 Structural equation model
Drawing on the literature on variability in HbA1C levels, the variables that demonstrated a significant impact on such variability were integrated into a structural equation model while taking into account the study’s objectives and domain-specific knowledge. The resulting model incorporated five observed variables - namely, outpatient-specific disease management, mean triglyceride, mean total cholesterol, baseline HbA1C, and recent HbA1C- along with four latent variables: uric acid variability, total cholesterol variability, LDL variability, and HbA1c variability. The final structural equation model is illustrated in Figure 2. The goodness-of-fit indices, which included a χ2/df of 2.698:3, RMSEA of 0.068:0.08, GFI of > 0.9, AGFI of 0.9, NFI of > 0.9, RFI of > 0.9, IFI of > 0.9, TLI of > 0.9, and CFI of > 0.9, revealed a good fit for the model. The model specific fits are detailed in Table 6. The power4SEM program and calculated the study efficacy of 0.969 for this study based on the study results RMSEA=0.068, DF=39, N=369, α=0.05. Further details are available in Figure 3.
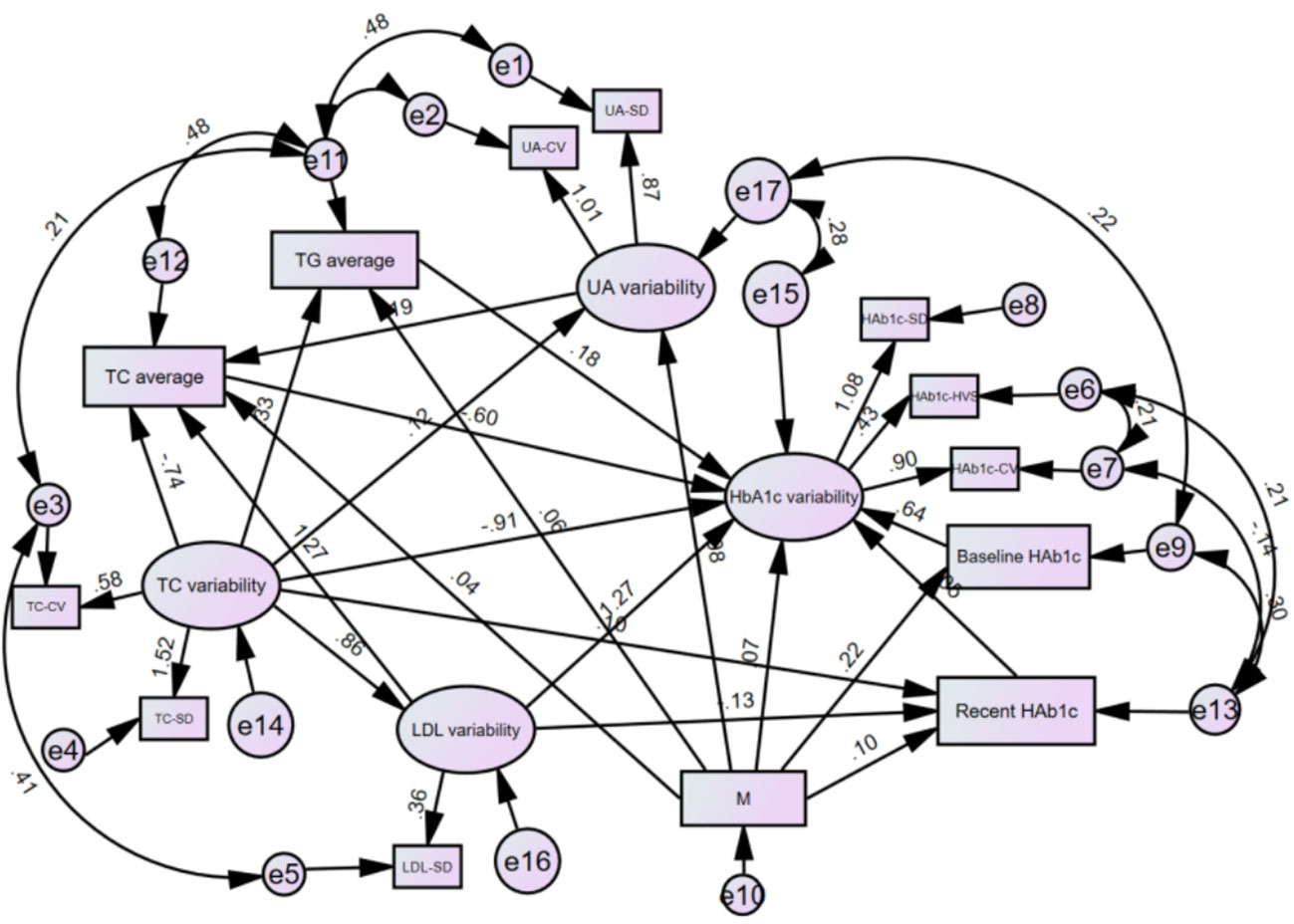
Figure 2 Modified model of structural equations for HbA1c variants in T2DM patients. M.Outpatient sppecial disease management. HbA1c Glycated hemoglobin; UA, Uric acid; TG, Triglycerides; TC, Total cholesterol; LDL, Low-density lipoprotein; CV, Coefficient of Variation; SD, Standard Deviation; HVS, HbA1c variability score.
3.6 Effect analysis of structural equation model variables
The present investigation discovered that TC-mean exerted a negative direct impact on HbA1c variability (β=-0.600, P=0.028). Baseline HbA1C exhibited the strongest positive direct influence on HbA1C variability (β=0.644, P=0.001), while TG-mean had a positive direct effect on HbA1C variability (β=0.176, P=0.042) and outpatient specific disease management had a positive direct effect on HbA1C variability (β=0.071, P=0.039). Further details are available in Table 7.
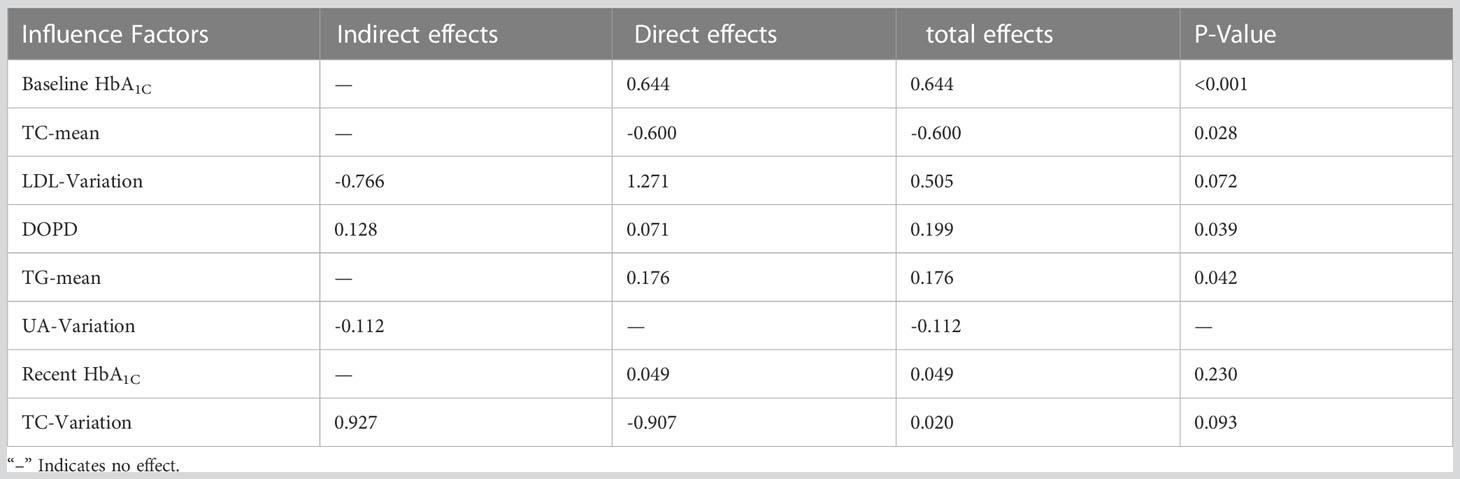
Table 7 Direct, indirect and total effects of each influencing factor on long-term blood glucose variability.
4 Discussion
In this study, the aim was to investigate the association between HbA1C variability and outpatient special disease management, uric acid, total cholesterol, triglycerides, HDL cholesterol, LDL cholesterol, and other relevant variables in patients with type 2 diabetes. The results indicated that each of the aforementioned variables had a varying degree of impact on HbA1C variability. This provides theoretical support for healthcare professionals to implement effective strategies to improve HbA1C variability in diabetic patients, thereby aiding in reducing the detrimental health outcomes associated with this disease and improving the quality of life of patients and their families, as well as minimizing the economic burden on society.
4.1 Current status of long-term glycemic variability in patients with T2DM
The results of this study indicated that the HbA1C high variability score (HbA1C-HVS) was below the threshold value (60%) reported in the study by Li et al. (19). This was potentially due to the majority of patients being enrolled in outpatient special disease management, which increased their compliance with treatment and resulted in a relatively low HVS. In contrast, the HbA1c standard deviation (HbA1C-SD) and coefficient of variation (HbA1C-CV) values were higher than those reported in the study by Sun et al. (31), who employed interventions to strengthen glycemic control and reduce glucose fluctuations. However, these values were lower than those reported in the study by Mo et al. (32) on the percentage of glucose variation in Chinese diabetic patients, with a coefficient (%CV) threshold value of 33%. These results suggest that the HbA1C fluctuations observed in this study were relatively stable, and that lower HbA1C variability in diabetic patients could potentially reduce or delay the occurrence of complications associated with diabetes (33). Moreover, lower levels of HbA1C variability in patients could potentially reduce the number of hypoglycemic events, alleviate economic and psychological stress, and improve their quality of life (27).
4.2 Risk factors for long-term glycemic variability in patients with T2DM
The present study employed structural equation modeling to investigate the predictors of long-term glycemic variation in patients with type 2 diabetes mellitus. The results indicated that outpatient special disease management, baseline HbA1c, mean total cholesterol (TC), and mean triglycerides (TG) had significant direct effects on long-term glycemic variation. Moreover, outpatient special disease management was found to have an indirect effect on long-term glycemic variation by influencing both baseline and recent HbA1c. However, long-term glucose variance was not significantly predicted by variance in low-density lipoprotein (LDL), uric acid (UA), recent HbA1c, or TC variance. Nevertheless, LDL variance and UA variance were found to have an indirect effect on long-term glucose variance through their effect on mean TC, while TC variance was also found to have an indirect effect on long-term glucose variance through its effect on mean TG.
Diabetes is a persistent, lifelong condition that necessitates frequent medication and monitoring of blood glucose levels, as well as regular assessments for worsening and complications to allow for timely intervention. Patients who meet the requisite criteria for diabetes management are encouraged to participate in outpatient special disease treatment as the diabetes management system improves. The proportion of patients in this study who were enrolled in the diabetes clinic’s special disease management program (69.92%) was higher than that reported by Luo Xiaolu et al. (34) for community-based diabetic patients in Chengdu (48.94%), possibly due to patients’ recognition of the importance of standardized diabetes management. Outpatient management of specific diseases can not only enhance patient health status, functional mobility, and combat obesity (35), but also improve glycemic control and reduce negative outcomes (36), shorten patient stays (37), and reduce medication usage and expenses (38), thereby reducing the financial burden on patients. Additionally, outpatient special disease management can improve patients’ blood glucose control, adherence to treatment, and decrease the incidence of adverse events (39). Greater adherence by patients results in better blood glucose control and timely detection of abnormal values, leading to timely intervention and hence reducing blood glucose fluctuations, ultimately leading to lower HbA1c levels (40), and in turn, reducing long-term bloating. Long-term blood glucose fluctuation can be minimized as a result. A study by Gill et al. (41) on the connection between healthcare expenses and glycemic control in diabetic patients revealed that diabetic patients managed by an endocrinologist specialist had lower healthcare costs, particularly those linked to microvascular and macrovascular complications, and improved HbA1c levels (41). Thus, eligible patients should be encouraged to participate in outpatient special disease care, which will not only minimize treatment expenses and family and societal obligations, but also improve glycemic control and diabetes outcomes, thereby improving patients’ quality of life.
Diabetes is a progressive and insidious condition, which may persist for a period of 4 to 6 years before its initial diagnosis (42). The presence of one or more complications highlights the urgency of early detection and management of glycemic aberrations, which can potentially minimize the severity and incidence of these complications. The baseline level of glycated hemoglobin (HbA1c) serves as a pivotal determinant of glucose-lowering therapies (43) and strongly correlates with HbA1c variability (44). Elevated baseline HbA1c levels significantly augment HbA1c decline following therapeutic interventions, precipitating increased variability (45). This variability escalates the risk of patient-related complications and mortality (46). Hence, it is imperative to fortify diabetes education programs and emphasize routine blood glucose monitoring, aimed at detecting and intervening early to minimize blood glucose fluctuations and improve HbA1c variability, thereby impeding the progression of complications and enhancing diabetes-related outcomes.
The present investigation also determined that lipid variability was strongly associated with HbA1c variability. Increased lipid oscillations have been linked to adverse outcomes such as cardiovascular disease and all-cause mortality (47, 48), as well as diabetic complications such as retinopathy, nephropathy, and neurological disease (49, 50). Moreover, the incidence of diabetes and lipid variability were positively correlated (51), suggesting that greater lipid variability is linked to an elevated risk of HbA1c abnormalities. This may be due to the lipotoxic effect of lipids on beta cells, which alters the structure of glucose-activating enzymes and lipid rafts on the cell membrane, leading to beta cell apoptosis (52). Consequently, insulin secretion is reduced and glucose oxidation and utilization are increased, further exacerbating HbA1c variability (53). According to previous research, dyslipidemia plays a pivotal role in the etiology and pathophysiology of diabetes and is strongly positively correlated with HbA1c (54–57). Elevated lipid levels enhance variability by causing beta cell dysfunction and apoptosis (52), leading to reduced insulin secretion and elevated HbA1c levels (56, 58). In addition, uric acid fluctuations have an indirect impact on HbA1c variations. Uric acid is involved in lipid regulation and is positively correlated with cholesterol (59), which can cause dyslipidemia and poor glucose tolerance by inducing hypothalamic inflammation and gliosis and reducing hypothalamic endocrine capacity (60). This leads to suboptimal lipid markers and elevated blood glucose levels, which combined contribute to poor glycemic control and HbA1c oscillations. Uric acid variability has been linked to diabetes (61), cardiovascular risk (62), renal function, and all-cause mortality (63). Thus, elevated levels of lipids and uric acid can impact not only HbA1c variability but also the severity of adverse outcomes and quality of life in diabetes patients. Improvements in the monitoring of lipid and uric acid levels in diabetic patients are necessary for early detection and management to enhance diabetes outcomes. Elevated levels of lipids and uric acid exert not only direct but also indirect effects on the variability of HbA1C, contributing to the exacerbation of adverse outcomes and diminished quality of life in individuals with diabetes. The management strategy for glycemic control in diabetic patients revolves around addressing the three principal components of glycemic dysregulation, namely chronic hyperglycemia, hypoglycemia, and glycemic variability. Heightened variability in HbA1C has been associated with numerous unfavorable prognostic factors in diabetes and currently stands as the strongest indicator of diabetic outcomes, rendering it of considerable clinical significance. Hence, early intervention to regulate blood glucose levels and maintain glycemic stability is imperative to enhance patient prognosis and improve their quality of life.
This study did not uncover a significant association between HbA1C variability and changes in follow-up duration. Upon thorough examination of the existing literature, it is evident that no study has thus far provided a specific threshold denoting the extent of risk posed by fluctuations in glycosylated hemoglobin levels for patients. While one study identified a correlation between prolonged variability in glycosylated hemoglobin and cardiovascular disease as well as mortality in individuals with diabetes, the long-term ramifications remain uncertain. Consequently, it is recommended to conduct follow-up investigations spanning a duration of 10 years or more, which may serve as a potential avenue for future research endeavors.
4.3 Limitations
This study possesses certain inherent limitations. Primarily, it is a retrospective study conducted at a single medical center, potentially resulting in an inadequate sample size. Secondly, it neglects to incorporate the influence of medications on long-term glycemic variation. It is anticipated that future investigations will employ multi-center surveys, expand the sample size, and undertake thorough exploration of the specific impact of medications on prolonged glucose variability in a prospective study. These endeavors will serve to augment the study’s depth and furnish a theoretical foundation for improved clinical treatment options, thereby enhancing the quality of care provided to patients.
5 Conclusions
Using structural equation modeling, we conducted an assessment of the factors that impact HbA1c variability in individuals with type 2 diabetes. Our findings revealed that outpatient special disease management, baseline HbA1c, lipids, and uric acid are significant risk factors for HbA1c variation. Consequently, it is recommended that health education programs be intensified for diabetic patients and those at risk, with emphasis on promoting treatment compliance and timely regulation of potential risk factors such as lipids and uric acid to maintain blood glucose stability. This approach is conducive to reducing HbA1c variability, improving adverse outcomes and mortality in diabetic patients, enhancing their quality of life, and reducing the economic burden on families and society.
Data availability statement
The raw data supporting the conclusions of this article will be made available by the authors, without undue reservation.
Ethics statement
Written informed consent was not obtained from the individual(s) for the publication of any potentially identifiable images or data included in this article.
Author contributions
YG, MC, ZX and HQ have determined the topic. LK, JW, and YP collected and organized data. XW, JZ, and XF did data analysis. YG and MC did manuscript writing and revision. ZX and HQ provided advice on draft revisions and finalized them. All authors contributed to the article and approved the submitted version.
Acknowledgments
The authors would like to thank all study participants as well as all participants participating in the study’s development, revision, and coaching. The final manuscript was read and approved by all writers.
Conflict of interest
The authors declare that the research was conducted in the absence of any commercial or financial relationships that could be construed as a potential conflict of interest.
Publisher’s note
All claims expressed in this article are solely those of the authors and do not necessarily represent those of their affiliated organizations, or those of the publisher, the editors and the reviewers. Any product that may be evaluated in this article, or claim that may be made by its manufacturer, is not guaranteed or endorsed by the publisher.
References
1. Zheng Y, Ley SH, Hu FB. Global aetiology and epidemiology of type 2 diabetes mellitus and its complications. Nat Rev Endocrinol (2018) 14(2):88–98. doi: 10.1038/nrendo.2017.151
2. Sun H, Saeedi P, Karuranga S, Pinkepank M, Ogurtsova K, Duncan BB, et al. IDF Diabetes Atlas: Global, regional and country-level diabetes prevalence estimates for 2021 and projections for 2045. Diabetes Res Clin Pract (2022) 183:109119. doi: 10.1016/j.diabres.2021.109119
3. Society CD. Guideline for the preventon and treatment of type 2 diabetes mellitus in China(2020 edition)(part 1). Chin J Pract Internal Med (2021) 41(08):668–95. doi: 10.19538/j.nk2021080106
4. Demir S, Nawroth PP, Herzig S, Ekim Ü.B. Emerging targets in type 2 diabetes and diabetic complications. Adv Sci (Weinh) (2021) 8(18):e2100275. doi: 10.1002/advs.202100275
5. Raghavan S, Vassy JL, Ho YL, Song RJ, Gagnon DR, Cho K, et al. Diabetes mellitus-related all-cause and cardiovascular mortality in a national cohort of adults. J Am Heart Assoc (2019) 8(4):e011295. doi: 10.1161/JAHA.118.011295
6. Cole JB, Florez JC. Genetics of diabetes mellitus and diabetes complications. Nat Rev Nephrol (2020) 16(7):377–90. doi: 10.1038/s41581-020-0278-5
7. Goldhaber-Fiebert JD, Li H, Ratanawijitrasin S, Vidyasagar S, Wang XY, Aljunid S, et al. Inpatient treatment of diabetic patients in Asia: evidence from India, China, Thailand and Malaysia. Diabetes Med (2010) 27(1):101–8. doi: 10.1111/j.1464-5491.2009.02874.x
8. Williams R, Karuranga S, Malanda B, Saeedi P, Basit A, Besançon S, et al. Global and regional estimates and projections of diabetes-related health expenditure: Results from the International Diabetes Federation Diabetes Atlas, 9th edition. Diabetes Res Clin Pract (2020) 162:162108072. doi: 10.1016/j.diabres.2020.108072
9. Cardoso C, Leite NC, Moram C, Salles GF. Long-term visit-to-visit glycemic variability as predictor of micro- and macrovascular complications in patients with type 2 diabetes: The Rio de Janeiro Type 2 Diabetes Cohort Study. Cardiovasc Diabetol (2018) 17(1):33. doi: 10.1186/s12933-018-0677-0
10. Endocrinology CSO. Expers consensus on management of glycemic variability of diabetes mellitus. Drug Eval (2017) 14(17):5–814. doi: 10.3969/j.issn.1672-2809.2017.17.001
11. Škrha J, Šoupal J, Škrha JJ, Prázný M. Glucose variability, HbA1c and microvascular complications. Rev Endocr Metab Disord (2016) 17(1):103–10. doi: 10.1007/s11154-016-9347-2
12. Ceriello A, Monnier L, Owens D. Glycaemic variability in diabetes: clinical and therapeutic implications. Lancet Diabetes Endocrinol (2019) 7(3):221–30. doi: 10.1016/S2213-8587(18)30136-0
13. Martinez M, Santamarina J, Pavesi A, Musso C, Umpierrez GE. Glycemic variability and cardiovascular disease in patients with type 2 diabetes. BMJ Open Diabetes Res Care (2021) 9(1):e002032. doi: 10.1136/bmjdrc-2020-002032
14. Devries JH. Glucose variability: where it is important and how to measure it. Diabetes (2013) 62(5):1405–8. doi: 10.2337/db12-1610
15. Bergenstal RM. Glycemic variability and diabetes complications: does it matter? Simply put, there are better glycemic markers!. Diabetes Care (2015) 38(8):1615–21. doi: 10.2337/dc15-0099
16. Home P. Contributions of basal and post-prandial hyperglycaemia to micro- and macrovascular complications in people with type 2 diabetes. Curr Med Res Opin (2005) 21(7):989–98. doi: 10.1185/030079905X49662
17. Klimontov VV, Saik OV, Korbut AI. Glucose variability: how does it work? Int J Mol Sci (2021) 22(15):7783. doi: 10.3390/ijms22157783
18. Rodbard D. Glucose variability: A review of clinical applications and research developments. Diabetes Technol Ther (2018) 20(S2):S25–S215. doi: 10.1089/dia.2018.0092
19. Li S, Nemeth I, Donnelly L, Hapca S, Zhou K, Pearson ER. Visit-to-visit hbA(1c) variability is associated with cardiovascular disease and microvascular complications in patients with newly diagnosed type 2 diabetes. Diabetes Care (2020) 43(2):426–32. doi: 10.2337/dc19-0823
20. Yang CY, Su PF, Hung JY, Ou HT, Kuo S. Comparative predictive ability of visit-to-visit HbA1c variability measures for microvascular disease risk in type 2 diabetes. Cardiovasc Diabetol (2020) 19(1):105. doi: 10.1186/s12933-020-01082-9
21. Forbes JM, Fotheringham AK. Vascular complications in diabetes: old messages, new thoughts. Diabetologia (2017) 60(11):2129–38. doi: 10.1007/s00125-017-4360-x
22. Wan EY, Fung CS, Fong DY, Lam CL. Association of variability in hemoglobin A1c with cardiovascular diseases and mortality in Chinese patients with type 2 diabetes mellitus - A retrospective population-based cohort study. J Diabetes Complications (2016) 30(7):1240–7. doi: 10.1016/j.jdiacomp.2016.05.024
23. Lee CL, Sheu WH, Lee IT, Lin SY, Liang WM, Wang JS, et al. Trajectories of fasting plasma glucose variability and mortality in type 2 diabetes. Diabetes Metab (2018) 44(2):121–8. doi: 10.1016/j.diabet.2017.09.001
24. Gorst C, Kwok CS, Aslam S, Buchan I, Kontopantelis E, Myint PK, et al. Long-term glycemic variability and risk of adverse outcomes: A systematic review and meta-analysis. Diabetes Care (2015) 38(12):2354–69. doi: 10.2337/dc15-1188
25. Hirakawa Y, Arima H, Zoungas S, Ninomiya T, Cooper M, Hamet P, et al. Impact of visit-to-visit glycemic variability on the risks of macrovascular and microvascular events and all-cause mortality in type 2 diabetes: the ADVANCE trial. Diabetes Care (2014) 37(8):2359–65. doi: 10.2337/dc14-0199
26. Zhou JJ, Schwenke DC, Bahn G, Reaven P. Glycemic variation and cardiovascular risk in the veterans affairs diabetes trial. Diabetes Care (2018) 41(10):2187–94. doi: 10.2337/dc18-0548
27. Long C, Tang Y, Huang J, Liu S, Xing Z. Association of long-term visit-to-visit variability of HbA1c and fasting glycemia with hypoglycemia in type 2 diabetes mellitus. Front Endocrinol (Lausanne) (2022) 13975468. doi: 10.3389/fendo.2022.975468
28. Song J, Bai H, Xu H, Xing Y, Chen S. HbA1c variability and the risk of dementia in patients with diabetes: A meta-analysis. Int J Clin Pract (2022) 2022:7706330. doi: 10.1155/2022/7706330
29. Jiaying N, Xiaojing M, Jian Z. Research progress of abnormal glycemic variability and diabetic complications. Chin J Diabetes (2022) 14(04):388–92. doi: 10.3760/cma.j.cn115791-20210510-00262
30. Hu LT, Bentler PM. Cutoff criteria for fit indexes in covariance structure analysis: Conventional criteria versus new alternatives. Struct equation modeling: Multidiscip J (1999) 6(1):1–55. doi: 10.1080/10705519909540118
31. Sun B, Gao Y, He F, Liu Z, Zhou J, Wang X, et al. Association of visit-to-visit HbA1c variability with cardiovascular diseases in type 2 diabetes within or outside the target range of HbA1c. Front Public Health (2022) 101052485. doi: 10.3389/fpubh.2022.1052485
32. Mo Y, Ma X, Lu J, Shen Y, Wang Y, Zhang L, et al. Defining the target value of the coefficient of variation by continuous glucose monitoring in Chinese people with diabetes. J Diabetes Investig (2021) 12(6):1025–34. doi: 10.1111/jdi.13453
33. Scott ES, Januszewski AS, O'connell R, Fulcher G, Scott R, Kesaniemi A, et al. Long-term glycemic variability and vascular complications in type 2 diabetes: post hoc analysis of the FIELD study. J Clin Endocrinol Metab (2020) 105(10):dgaa361. doi: 10.1210/clinem/dgaa361
34. Xiaolu L, Yanli H, Shuang F, Yu S. Effect of special disease Outpatient Mangement on Prevalence and Efficacy of Statin Treament in Type 2 Diabetic Patients:a Multicenter Community-based Study. Chin Gen Pract (2019) 22(11):1277–82. doi: 10.12114/j.issn.1007-9572.2019.00.068
35. Lipton BJ. Association between Health Insurance and Health among Adults with Diabetes: Evidence from Medicare. J Am Geriatr Soc (2020) 68(2):388–94. doi: 10.1111/jgs.16238
36. Brown A, Kressin N, Terrin N, Hanchate A, Suzukida J, Kher S, et al. The influence of health insurance stability on racial/ethnic differences in diabetes control and management. Ethn Dis (2021) 31(1):149–58. doi: 10.18865/ed.31.1.149
37. Zhang A, Quan J, Eggleston K. Association between the quality of primary care, insurance coverage, and diabetes-related health outcomes in a cohort of older adults in China: results from the China Health and Retirement Longitudinal Study. BMJ Open (2022) 12(9):e059756. doi: 10.1136/bmjopen-2021-059756
38. Guo L, Zheng J, Pan Q, Zhang Q, Zhou Y, Wang W, et al. Changes in direct medical cost and medications for managing diabetes in Beijing, China 2016 to 2018: Electronic Insurance Data Analysis. Ann Fam Med (2021) 19(4):332–41. doi: 10.1370/afm.2686
39. Hai-Li X, Hong G, Ming Z, Meng-Jie Y, Lei X. Analysis of the influence of pharmaceutical care on the health management of diabetic patients. Chin J Drug Appl Monit (2020) 17(01):56–9. doi: CNKI:SUN:YWYY.0.2020-01-021
40. Huaying G, Xiuying L, Lingli J, Qingqing W, Liping K. A study on self-monitoring compliance and factors influencing self-monitoring in elderly patients with type 2 diabetes mellitus. Chin J Health Stat (Chinese) (2022) 39(05):717–20.
41. Gill M, Chhabra H, Shah M, Zhu C, Lando H, Caldarella F. Association between provider specialty and healthcare costs and glycemic control for patients with diabetes. J Med Econ (2018) 21(7):704–8. doi: 10.1080/13696998.2018.1467324
42. Porta M, Curletto G, Cipullo D, Rigault DLLR, Trento M, Passera P, et al. Estimating the delay between onset and diagnosis of type 2 diabetes from the time course of retinopathy prevalence. Diabetes Care (2014) 37(6):1668–74. doi: 10.2337/dc13-2101
43. Jones AG, Lonergan M, Henley WE, Pearson ER, Hattersley AT, Shields BM. Should studies of diabetes treatment stratification correct for baseline HbA1c? PloS One (2016) 11(4):e0152428. doi: 10.1371/journal.pone.0152428
44. Giugliano D, Maiorino M, Bellastella G, Chiodini P, Esposito K. Relationship of baseline HbA1c, HbA1c change and HbA1c target of < 7% with insulin analogues in type 2 diabetes: a meta-analysis of randomised controlled trials. Int J Clin Pract (2011) 65(5):602–12. doi: 10.1111/j.1742-1241.2010.02619.x
45. Frias JP, Bonora E, Cox DA, Bethel MA, Kwan A, Raha S, et al. Glycaemic efficacy of an expanded dose range of dulaglutide according to baseline glycated haemoglobin (HbA1c) subgroup: Post hoc analysis of AWARD-11. Diabetes Obes Metab (2021) 23(12):2819–24. doi: 10.1111/dom.14533
46. Lee S, Liu T, Zhou J, Zhang Q, Wong WT, Tse G. Predictions of diabetes complications and mortality using HbA1c variability: a 10-year observational cohort study. Acta Diabetol (2021) 58(2):171–80. doi: 10.1007/s00592-020-01605-6
47. Wang MC, Li CI, Liu CS, Lin CH, Yang SY, Li TC, et al. Effect of blood lipid variability on mortality in patients with type 2 diabetes: a large single-center cohort study. Cardiovasc Diabetol (2021) 20(1):228. doi: 10.1186/s12933-021-01421-4
48. Li S, Hou L, Zhu S, Yi Q, Liu W, Zhao Y, et al. Lipid variability and risk of cardiovascular diseases and all-cause mortality: A systematic review and meta-analysis of cohort studies. Nutrients (2022) 14(12):2450. doi: 10.3390/nu14122450
49. Bjornstad P, Drews KL, Caprio S, Gubitosi-Klug R, Nathan DM, Tesfaldet B, et al. Long-term complications in youth-onset type 2 diabetes. N Engl J Med (2021) 385(5):416–26. doi: 10.1056/NEJMoa2100165
50. Lee S, Zhou J, Wong WT, Liu T, Wu W, Wong I, et al. Glycemic and lipid variability for predicting complications and mortality in diabetes mellitus using machine learning. BMC Endocr Disord (2021) 21(1):94. doi: 10.1186/s12902-021-00751-4
51. Cai Z, Chen Z, Fang W, Li W, Huang Z, Wang X, et al. Triglyceride to high-density lipoprotein cholesterol ratio variability and incident diabetes: A 7-year prospective study in a Chinese population. J Diabetes Investig (2021) 12(10):1864–71. doi: 10.1111/jdi.13536
52. Wen G, Jie L, Yamei W, Xiaoqian C, Haiyan S, Bing X. Different effects of lipid components on insulin secretion in patients with type 2 diabetes and subjects with normal glucose metabolism. Chin Jouurnal Endocrinol Metab (2015) 7:599–600. doi: 10.3760/cma.j.issn.1000-6699.2015.07.009
53. Sunil B, Ashraf AP. Dyslipidemia in pediatric type 2 diabetes mellitus. Curr Diabetes Rep (2020) 20(10):53. doi: 10.1007/s11892-020-01336-6
54. Martins RA, Jones JG, Cumming SP, Coelho ESM, Teixeira AM, Veríssimo MT. Glycated hemoglobin and associated risk factors in older adults. Cardiovasc Diabetol (2012) 11:13. doi: 10.1186/1475-2840-11-13
55. Wu L, Parhofer KG. Diabetic dyslipidemia. Metabolism (2014) 63(12):1469–79. doi: 10.1016/j.metabol.2014.08.010
56. Hsiung C, Chang Y, Lin C, Chang C, Chou W, Chu H, et al. The causal relationship of circulating triglyceride and glycated hemoglobin: A mendelian randomization study. J Clin Endocrinol Metab (2020) 105(3):908–19. doi: 10.1210/clinem/dgz243
57. Ting H, Yan X, Qing-Xing S. Analysis of the relationship between glycosylated hemoglobin and fasting blood glucose, blood lipids in patients with type 2Diabetes mellitus. J Med Inf (2022) 35(18):147–9.
58. Bulut T, Demirel F, Metin A. The prevalence of dyslipidemia and associated factors in children and adolescents with type 1 diabetes. J Pediatr Endocrinol Metab (2017) 30(2):181–7. doi: 10.1515/jpem-2016-0111
59. Peng TC, Wang CC, Kao TW, Chan JY, Yang YH, Chang YW, et al. Relationship between hyperuricemia and lipid profiles in US adults. BioMed Res Int (2015) 2015:127596. doi: 10.1155/2015/127596
60. Lu W, Xu Y, Shao X, Gao F, Li Y, Hu J, et al. Uric acid produces an inflammatory response through activation of NF-κB in the hypothalamus: implications for the pathogenesis of metabolic disorders. Sci Rep (2015) 5:12144. doi: 10.1038/srep12144
61. Su H, Liu T, Li Y, Fan Y, Wang B, Liu M, et al. Serum uric acid and its change with the risk of type 2 diabetes: A prospective study in China. Prim Care Diabetes (2021) 15(6):1002–6. doi: 10.1016/j.pcd.2021.06.010
62. Kim HK, Lee M, Lee YH, Lee BW, Cha BS, Kang ES. Uric acid variability as a predictive marker of newly developed cardiovascular events in type 2 diabetes. Front Cardiovasc Med (2021) 8775753. doi: 10.3389/fcvm.2021.775753
Keywords: type 2 diabetes mellitus, glycated hemoglobin, glycemic variability, influential factors, structural equation modeling
Citation: Gan Y, Chen M, Kong L, Wu J, Pu Y, Wang X, Zhou J, Fan X, Xiong Z and Qi H (2023) A study of factors influencing long-term glycemic variability in patients with type 2 diabetes: a structural equation modeling approach. Front. Endocrinol. 14:1216897. doi: 10.3389/fendo.2023.1216897
Received: 04 May 2023; Accepted: 10 July 2023;
Published: 31 July 2023.
Edited by:
Alessando Mattina, IRRCS ISMETT/UPMC Italy, ItalyReviewed by:
Elettra Mancuso, University Magna Graecia of Catanzaro, ItalyOscar Lorenzo, Health Research Institute Foundation Jimenez Diaz (IIS-FJD), Spain
Copyright © 2023 Gan, Chen, Kong, Wu, Pu, Wang, Zhou, Fan, Xiong and Qi. This is an open-access article distributed under the terms of the Creative Commons Attribution License (CC BY). The use, distribution or reproduction in other forums is permitted, provided the original author(s) and the copyright owner(s) are credited and that the original publication in this journal is cited, in accordance with accepted academic practice. No use, distribution or reproduction is permitted which does not comply with these terms.
*Correspondence: Hong Qi, MTUyOTgyMTE4NUBxcS5jb20=; Zhenzhen Xiong, eHp6NjIzMDg2MzFAMTYzLmNvbQ==
†These authors have contributed equally to this work and share first authorship
‡These authors have contributed equally to this work