- 1Cancer Center, The First Hospital of Jilin University, Changchun, China
- 2Department of Anesthesiology, The First Hospital of Jilin University, Changchun, China
Background: Metabolic syndrome is a cluster of metabolic disorders, including obesity, hypertension, hyperglycemia, and abnormal lipid levels. However, researches on the association between overall dietary quality measured by the Healthy Eating Index-2015 (HEI-2015) and the risk of metabolic syndrome is still lacking.
Methods: This study utilized data from four cycles (2011-2018) of the National Health and Nutrition Examination Survey (NHANES) database, including 17,582 participants. Logistic regression analysis was employed to explore the correlation between HEI and the risk of metabolic syndrome. Additionally, mediation analysis was conducted to examine the effects of Systemic Immune-Inflammation Index (SII) and serum uric acid as potential mediators between HEI and metabolic syndrome risk. Weighted quantile sum (WQS) regression evaluated the composite exposure impact of the 13 components of the HEI on metabolic syndrome, as well as the proportion of their weights.
Results: Higher dietary quality measured by HEI-2015 (at the 75th percentile) was negatively correlated with the risk of metabolic syndrome (OR=0.80, 95%CI=0.72-0.89, P=0.003). Higher SII and serum uric acid levels were identified as risk factors for metabolic syndrome (P for trend<0.001). Approximately 37.5% of the effect of HEI on metabolic syndrome occurrence was mediated by SII (Indirect effect=-0.002, 95%CI (-0.003,-0.001), Direct effect=-0.022, 95%CI (-0.0273,-0.015)). Additionally, 25% of the effect of HEI on metabolic syndrome occurrence was mediated by serum uric acid levels (Indirect effect=-0.006, 95%CI (-0.010,-0.012), Direct effect=-0.024, 95%CI (-0.041,-0.009)). WQS regression analysis revealed the highest weighted proportions for seafood and plant proteins (25.20%) and sodium (17.79%), while the weight for whole fruit was the lowest (0.25%).
Conclusion: Better dietary quality measured by HEI-2015 was associated with a lower likelihood of metabolic syndrome. Higher SII and serum uric acid levels were identified as risk factors for metabolic syndrome and potential mediators.
1 Introduction
The metabolic syndrome (MetS) refers to a cluster of metabolic abnormalities that increase the risk of cardiovascular disease and type 2 diabetes mellitus (1). The key components of MetS include central obesity, hypertension, dyslipidemia and hyperglycemia (2). With the global epidemic of obesity, the prevalence of MetS has increased substantially over the past few decades. It is estimated that 20-30% of the adult population worldwide has MetS (3). The underlying mechanisms are complex and not yet fully understood, but diet and nutrition have been identified as modifiable factors that may help prevent MetS (4).
The Healthy Eating Index (HEI) developed by the United States Department of Agriculture (USDA) is a measure of diet quality and adherence to the Dietary Guidelines for Americans. The index categorizes food components or nutrients into 13 elements, comprising 9 adequacy components and 4 moderation components, emphasizing a high intake of total vegetables, vegetables and legumes, whole grains, total fruits, whole fruits, total protein foods, plant-based proteins, seafood, and fatty acids and limiting the intake of saturated fatty acids, refined grains, sodium, and added sugars (5). Higher HEI scores indicate closer alignment with key recommendations and better diet quality. Previous studies have shown that higher HEI scores are associated with lower risks of obesity, hypertension, dyslipidemia and diabetes (6–9). However, there is limited research on the association between overall diet quality measured by HEI and risk of MetS, especially in the US population.
Systemic inflammation has been implicated in the pathogenesis of MetS. Chronic, sustained, low-grade inflammation promotes the release of a multitude of inflammatory mediators and cytokines, predisposing the organism to insulin resistance, dyslipidemia, and other metabolic dysregulations (10). Systemic immune-inflammation index (SII), based on peripheral neutrophil, lymphocyte and platelet counts, is a novel composite inflammatory biomarker. Recent evidence suggests that higher SII is associated with increased risks of diabetes, hypertension and metabolic disorders (11–13). Serum uric acid is also linked to systemic inflammation, and hyperuricemia predicts MetS risk (14). Uric acid may mediate the effects of diet on MetS, but few studies have examined its potential mediating role.
In this study, we aimed to investigate the association between HEI and risk of MetS and examine whether SII and serum uric acid levels mediate the association in US adults based on the National Health and Nutrition Examination Survey (NHANES) 2011-2018. The findings will provide novel evidence on the link between diet quality, inflammation and MetS among Americans.
2 Methods
2.1 Study population
In this study, our data was obtained from the publicly available National Health and Nutrition Examination Survey (NHANES) database (https://www.cdc.gov/nchs/nhanes/index.htm). NHANES is a nationally representative cross-sectional survey conducted by the National Institutes of Health and the Centers for Disease Control and Prevention, which aims to comprehensively investigate the health and dietary status of the U.S. population. The database includes modules on demographics, questionnaires, physical examinations, and laboratory tests (including blood and urine samples). For this study, we downloaded four consecutive datasets (2011-2012, 2013-2014, 2015-2016, and 2017-2018) from the website to accurately assess the relationship between a healthy diet index and metabolic syndrome. A total of 39,156 participants’ information was extracted.The following criteria were applied for inclusion and exclusion of participants: Inclusion criteria (1): All participants from 2011 to 2018; (2) Participants who cooperated with the follow-up and provided informed consent. Exclusion criteria: (1) Age< 18 years (n=15,331); (2) Participants with indeterminate MetS status (n=252), including individuals with missing data on blood pressure, total cholesterol, fasting blood glucose, glycated hemoglobin, and body mass index (BMI); (3) Individuals with incomplete or unreasonable dietary data that prevented the calculation of the healthy diet index (n=5,722). After applying these criteria, a total of 17,528 participants were included in this study. The specific details can be shown in Figure 1. This NHANES study was approved by the NCHS (National Center for Health Statistics) Ethics Review Board, and informed consent was obtained from all participants.
2.2 Data collection
The dietary data for this study was obtained from two 24-hour dietary recall surveys using the Automated Multiple-Pass Method (AMPM) standardized by the USDA (15). In the NHANES survey, trained interviewers conducted face-to-face interviews with participants to collect information on the nutrient composition and intake of various foods and beverages consumed in the past 24 hours. Based on the data from two 24-hour dietary recall surveys, equivalent data grouping transformations and relevant calculations were performed using the USDA Food and Nutrient Database.
The Healthy Eating Index-2015 (HEI-2015) utilizes the population ratio method based on dietary intake data from the entire polulation to assess the reasonableness of the population’s average dietary quality, as well as the adherence to healthy dietary patterns by researchers. This index was jointly developed by the USDA and the Department of Health and Human Services (HHS) in the United States and consists of 13 components including total fruit, whole fruit, total vegetables, greens and beans, dairy, whole grains, protein foods, fats, added sugars, seafood and plant proteins, saturated fats and unsaturated fats, refined grains, and added sugars. These indicators evaluate the diet quality and health of individuals or populations in terms of whole grains, vegetables and fruits, dairy, fats, and sugars, among others. Each component is scored according to specific criteria, and an overall score out of 100 can be calculated. A higher total score indicates greater adherence to the Dietary Guidelines for Americans (DGA) and better overall diet quality (16). The index was calculated and constructed using dietary intake data collected from participants’ two 24-hour dietary recalls, which were combined with USDA Food Patterns equivalent data. We utilized SAS code provided by the National Cancer Institute, and calculated the HEI-2015 score using the HEI scoring algorithm provided by the NHANES database. Detailed components, algorithms, and scoring criteria of HEI-2015 are available in the provided Supplementary Table 1.
In this study, participants diagnosed with MetS needed to meet three or more of the following conditions: 1) hypertriglyceridemia: serum triglyceride levels ≥150 mg/dL (1.7 mmol/L), or the use of lipid-lowering medication; 2) low high-density lipoprotein cholesterol levels: HDL<40 mg/dL (1.03 mmol/L) for men, HDL<50 mg/dL (1.29 mmol/L) for women; 3) high blood glucose levels: fasting blood glucose ≥100 mg/dL (5.6 mmol/L), or the use of glucose-lowering medication; 4) hypertension: systolic blood pressure ≥130 mmHg or diastolic blood pressure ≥85 mmHg, or the use of antihypertensive medication; 5) central obesity: waist circumference ≥102 cm for men, ≥88 cm for women (1).
The data for blood samples were measured and recorded by professional researchers utilizing automated hematological analyzers, including platelet count, neutrophil count, lymphocyte count, and uric acid levels. We calculated the Systemic Immune-Inflammation Index (SII), defined as (platelet count × neutrophil count)/lymphocyte count (17). Additionally, we gathered data on gender (female and male), age, race (Mexican American, Non-Hispanic Black, Non-Hispanic White, and other), poverty income ratio (≤1, 1–3, >3), education level (low high school, high school, college or above), smoking status, and alcohol consumption. Current smoker was defined as ‘more than 100 cigarettes in lifetime and currently smoking all or some days’, former smoker as ‘more than 100 cigarettes in lifetime but not currently smoking’, and never smoker as ‘fewer than 100 cigarettes in lifetime’. In terms of alcohol consumption, heavy drinker was defined as ‘weekly alcohol intake exceeding 14 standard drinks for women and 21 standard drinks for men’, moderate drinker as ‘weekly alcohol intake between 8-14 standard drinks for women and between 8-21 standard drinks for men’, and mild drinker as ‘weekly alcohol intake between 1-7 standard drinks’, with one standard drink equivalent to 14 grams of pure alcohol. The HEI-2015 was categorized based on quartiles, Quartile 1 (Q1):<25th percentile, Quartile 2 (Q2): ≥25 to 50th percentile, Quartile 3 (Q3): ≥50 to 75th percentile, and Quartile 4 (Q4): ≥75th percentile. Additionally, both SII and uric acid levels were categorized into quartiles (Q1, Q2, Q3, Q4) using the same approach.
2.3 Statistical analysis
Given the complex, multi-stage, stratified sampling design of the NHANES database, we conducted a weighted analysis in accordance with the database’s weighting recommendations. The analysis was weighted. Weighting factors included two-year examination weights (WTMEC2YR) and two-day dietary interview weights (WTDR2D), strata (SDMVSTRA), and primary sampling units (SDMVPSU) were considered to account for the complex survey design. Besides,we employed the data processing method of deleting missing values and accurately documented the number of cases where missing variables were removed. In this study, continuous variables were described using the weighted mean ± standard deviation (Mean ± SD) or the median and interquartile range (M, IQR), statistical differences were assessed using the weighted Student’s t-test or the Kruskal-Wallis rank sum test. Categorical variables were described by the number of samples (weighted percentage), with statistical differences evaluated using Pearson’s Chi-squared test.
For non-normally distributed continuous variables like HEI-2015, SII, and uric acid levels, they were transformed into categorical variables (quartiles) and three multivariable logistic regression models were established to preliminarily assess the relationship between HEI and MetS.These models included: Crude model - not adjusting for any variables; Model 1 - adjusting for gender, age, and race; Model 2 - adjusting for gender, age, race, education level, poverty income ratio, smoking status, and drinking status. Additionally, a second multivariable logistic regression model was used to evaluate the relationship between SII, uric acid levels, and the outcome variable metabolic syndrome. Furthermore, we employed mediation analysis, a method used to determine whether the association between variables can be partially explained by the influence of a third or mediating variable. In this study, the method was conducted to determine whether SII and uric acid levels mediated the relationship between a healthy eating index and MetS. The total effect (TE) represents the direct relationship between HEI-2015 and MetS, unaffected by the mediating factors. The indirect effect (IE) refers to the influence of SII and uric acid levels on MetS through HEI-2015. The direct effect (DE) represents the effect of HEI-2015 on MetS after controlling for SII and uric acid levels. A significant IE indicates the presence of a mediating effect. Besides, We employed sub-group analysis to investigate the relationship between HEI-2015 and MetS across different age groups, genders, races, educational levels, poverty-income ratios and smoking status, and assessed the significance of interaction effects by using p-values of the product terms between HEI-2015 components and stratification factors.
The relationship between mixed exposure of the 13 components constituting HEI and MetS, along with the weight proportion of each component, was analyzed using the WQS regression model.The basic weighted index model is as follows:
denotes any differentiable link function, represents the intercept, represents the regression coefficient, denotes the count of HEI components included in the analysi, denotes the matrix of covariates, Φ represents the coefficients for these covariates, denotes the sum of weighted quantiles for (c) components. represents the weighted index, where each index ranges from 0 to 1 , and the total sum of the weighted indices equals 1. represents the quartiles of the concentration for each chemical compound, where (i = 0,1,2,3) corresponds to the 1st, 2nd, 3rd, or 4th quartile, respectively (18).
All data analyses in this study were performed using R 4.2.2. All statistical tests were two-sided, and significance was set at P<0.05.
3 Results
3.1 Baseline characteristics
Participants were grouped based on the presence or absence of MetS, and detailed study characteristics are shown in Table 1. A total of 17,528 participants aged over 18 years were included, with 8,269 male participants (47.18%) and 9,259 female participants (52.82%). Among them, approximately 5,901 participants had MetS, while 11,951 participants did not. Compared to the group without metabolic syndrome, individuals with MetS were more likely to be in the age range of 18-65 years, female, non-Hispanic White, with a poverty income ratio (PIR) less than 3, college-educated or higher, non-smokers, drinkers, lower HEI and had higher SII and uric acid levels (p<0.050). For more specific details, please refer to Table 1.
3.2 Association between HEI and metabolic syndrome
Three multivariable logistic regression models were established to further investigate the impact of HEI on MetS. Compared to the Q1 group, the Q4 group showed a negative correlation with the occurrence of metabolic syndrome. In Model 1, compared to the Q1 quartile, the risk of developing MetS in the Q4 quartile was reduced by 22% (OR 0.78, 95% CI 0.66-0.92, p=0.003). In Model 2, compared to the Q1 quartile, the risk of developing MetS in the Q4 quartile was reduced by 23% (OR 0.77, 95% CI 0.66-0.91, p=0.003). Further details can be seen in Table 2.
3.3 The relationship between SII, uric acid levels, and metabolic syndrome
In order to further investigate the relationship between SII, uric acid levels, and MetS, and the role of covariates in the model, we categorized SII and uric acid levels into quartiles. We adjusted for gender, age, race, education level, poverty-income ratio, smoking status, and alcohol consumption in logistic regression models. Compared to the Q1 quartile for SII, the risk of developing MetS in the Q2, Q3, and Q4 quartiles increased by 21% (OR 1.21, 95% CI 1.06-1.38, p=0.010), 35% (OR 1.35, 95% CI 1.16-1.57, p<0.001), and 66% (OR 1.66, 95% CI 1.44-1.91, p<0.001), respectively. Compared to the Q1 quartile for uric acid levels, the risk of developing MetS in the Q2, Q3, and Q4 quartiles increased by 92% (OR 1.92, 95% CI 1.64-2.25, p<0.001), 184% (OR 2.84, 95% CI 2.37-3.40, p<0.001), and 404% (OR 5.04, 95% CI 4.25-5.98, p<0.001), respectively. Therefore, both SII and uric acid levels can be considered important risk factors for the occurrence of MetS. More details can be observed by referring to Table 3.
3.4 Mediation analysis
To explore whether SII and uric acid mediate the relationship between HEI and MetS, we conducted a mediation analysis. In this mediation model, HEI was treated as the independent variable, MetS as the dependent variable, and SII and uric acid levels as the mediating variables. The results are shown in Figure 2: HEI has a significant indirect effect on the occurrence of MetS through serum uric acid levels, with an indirect effect of -0.006 (95%CI: -0.010, -0.012). This suggests that serum uric acid levels partially mediate the relationship between HEI and MetS. After controlling for serum uric acid levels, HEI still exerts a significant inhibitory effect on the occurrence of MetS, with a direct effect of -0.024 (95%CI: -0.041, -0.009). This indicates that there are both direct and indirect effects of HEI on the occurrence of MetS. Approximately 25% of the impact of HEI on the occurrence of MetS is mediated by serum uric acid levels.
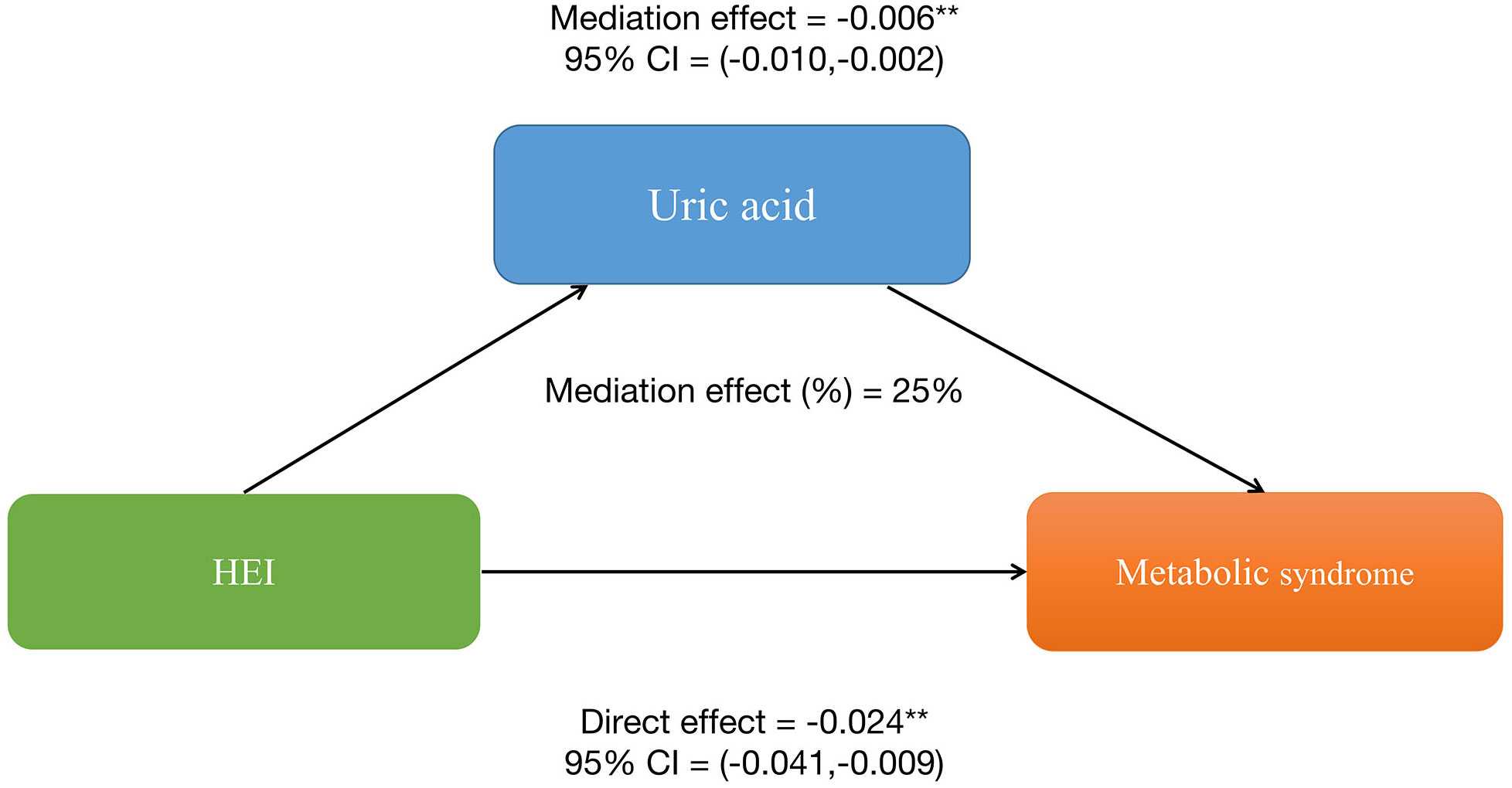
Figure 2 Serum uric acid levels partially mediates the relationship between healthy eating index-2015 and metabolic syndrome. *P<0.05, **P<0.01.
Similarly, HEI also has a significant indirect effect on the occurrence of MetS through SII, with an indirect effect of -0.002 (95%CI: -0.003, -0.001), suggesting a partial mediating effect of SII. After controlling for SII, HEI still shows a significant inhibitory effect on the occurrence of MetS, with a direct effect of -0.022 (95%CI: -0.0273, -0.015). This further indicates the presence of both direct and indirect effects of HEI on the occurrence of MetS, with approximately 37.5% of the impact of HEI on MetS occurrence being mediated by SII. More details can be shown in Figure 3. Therefore, both SII and serum uric acid levels can be considered important mediating factors in the relationship between HEI and the occurrence of MetS.
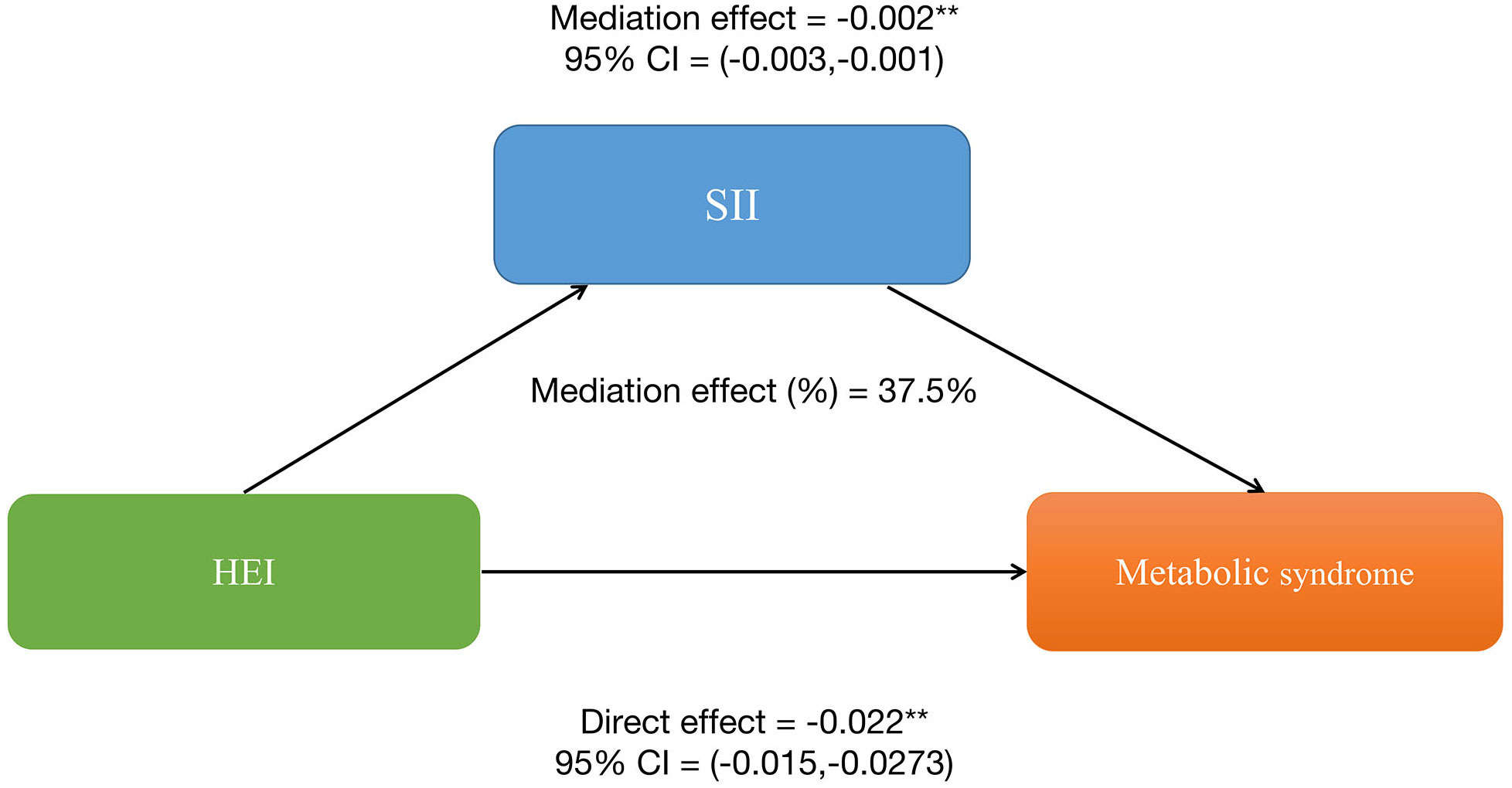
Figure 3 Systemic Immune-Inflammation Index (SII) partially mediates the relationship between healthy eating index-2015 and metabolic syndrome. *P<0.05, **P<0.01.
3.5 Subgroup analysis and interaction
The subgroup analysis revealed a significant interaction between HEI-2015 and age, gender, education level, PIR, and smoking status (p<0.05). The negative correlation between HEI-2015 and MetS was observed in older participants (aged > 65 years, OR 0.99, 95%CI (0.90,1.00)), female participants (OR 0.99, 95%CI (0.99,1.00)), non-Hispanic White participants (OR 0.99, 95%CI (0.99,0.99)), participants with a university or higher education level (OR 0.99, 95%CI (0.99,0.99)), and participants with a PIR≥3 (OR 0.99, 95%CI (0.99,0.99)). For more specific details, please refer to Figure 4.
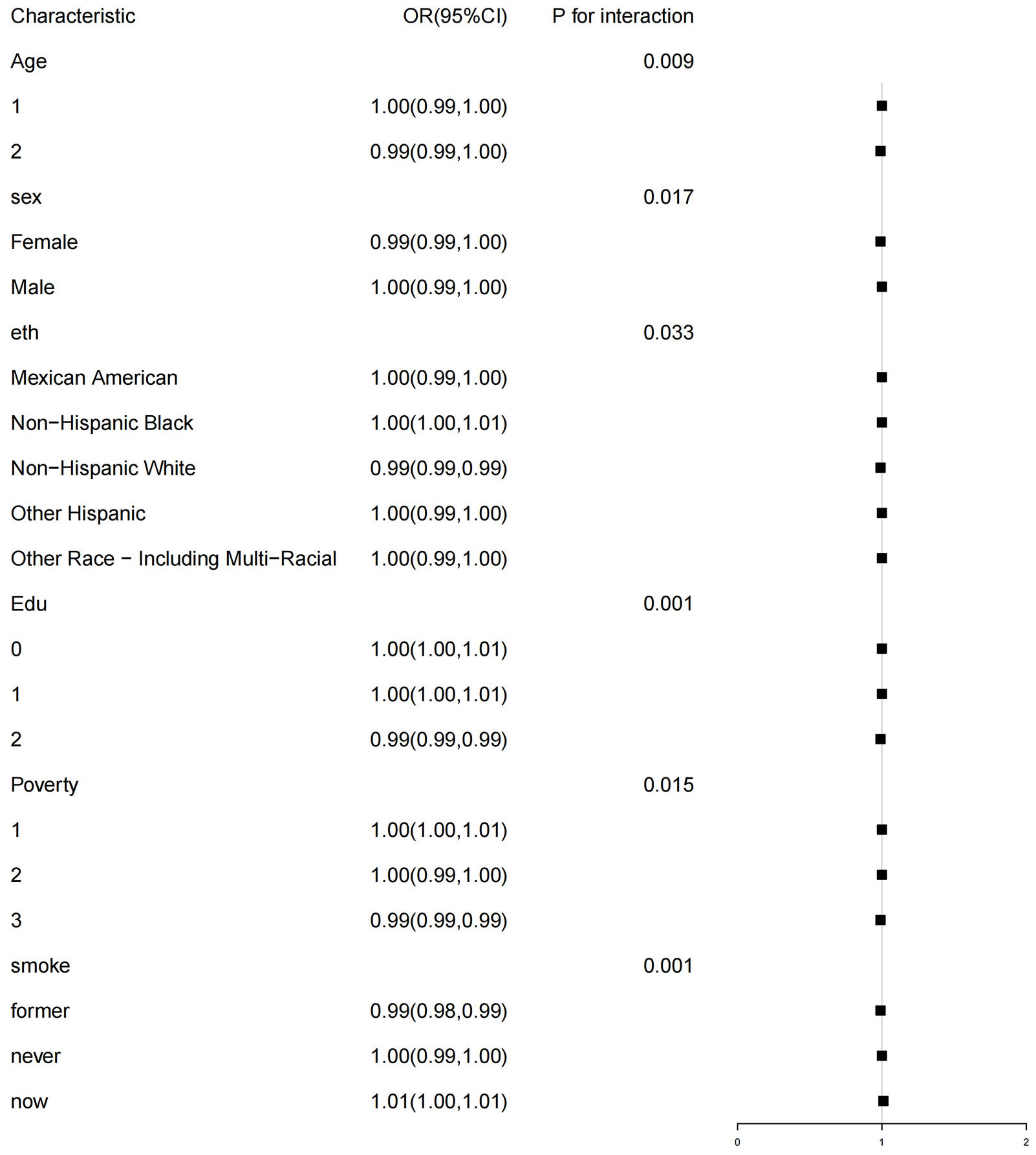
Figure 4 WQS model regression index weights for the metabolic syndrome (MetS), adjusted for sex,age, race/ethnicity, education attainment, poverty income ratio, smoking status, and alcohol drinking status.
3.6 Weighted quantile sum regression
The WQS regression model was employed to construct a weighted index for investigating the cumulative impact and proportional weights of the 13 components of the HEI on the risk of MetS. The findings illustrated a statistically significant inverse association between the overall HEI score and the prevalence of MetS (OR = -0.47, P< 0.001). Among the 13 components of the HEI, seafood and plant proteins (25.20%) along with sodium (17.79%) had the highest weights, whereas whole fruit had the lowest (0.25%). More detailed information can be obtained in Figure 5.
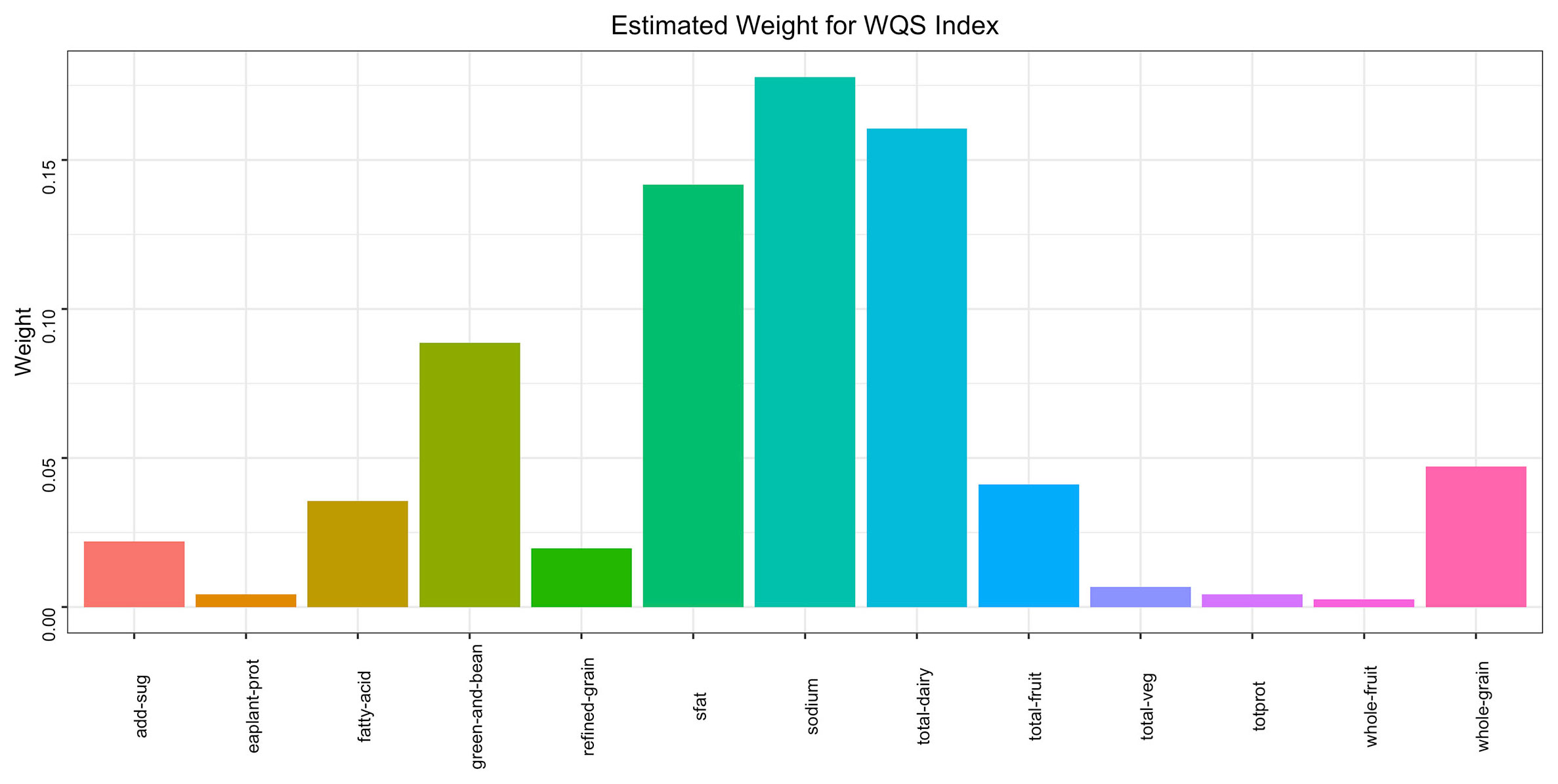
Figure 5 Subgroup analysis of the association of the HEI-2015 and the presence of metabolic syndrome (MetS). Each stratifcation was adjusted for age, sex, race/ethnicity, education attainment, poverty income ratio, smoking status. Age (1 aged 18-65 years; 2 ≥65years), Edu Education level (0 low high school; 1 high school; 2 College or above), Poverty (1<1; 2 [1, 3); 3 ≥3).
4 Discussion
In this large, nationally representative sample of US adults, we found that better diet quality measured by HEI-2015 was associated with lower odds of having MetS. The association remained significant after adjusting for demographics, socioeconomics, smoking and alcohol use. In addition, higher SII and serum uric acid levels were identified as risk factors for MetS. Mediation analysis further revealed that SII and uric acid partially mediated the association between HEI and MetS. The WQS regression model assessed the cumulative effects and weights of the 13 HEI components on MetS, with substantial consumption of seafood and plant proteins and moderate intake of sodium being most significantly associated with a reduced risk of MetS.
Our study provides new evidence that better adherence to the Dietary Guidelines for Americans and higher diet quality are associated with reduced Mets risk. The HEI-2015 assesses conformity to key dietary recommendations on fruits, vegetables, whole grains, dairy, protein, fatty acids, refined grains, sodium and empty calories (5). Meeting these targets may help prevent development of individual MetS components including central obesity, hypertension, dyslipidemia and hyperglycemia. In alignment with our findings, previous studies have linked higher HEI scores to lower prevalence of obesity, diabetes, hypertension and dyslipidemia (6–9). Two separate studies, one focusing on Korean adults and the other on Iranian adults, have also reported the associations between HEI scores and MetS risk (19, 20). Our research further confirmed the beneficial effects of diet quality in MetS among Americans. Interventions to improve diet quality should be a public health priority.
Chronic low-grade inflammation is involved in MetS pathogenesis (4). SII and uric acid are two emerging inflammatory markers associated with obesity, insulin resistance, diabetes and cardiovascular disease (11–14). Our study newly identified higher SII and uric acid as independent predictors of MetS after adjusting for confounders. Inflammation may be a key mechanism linking diet quality to MetS. Mediation analysis showed that the associations between HEI and MetS were partially mediated by SII and uric acid, suggesting both direct and indirect effects. A high-quality diet may help decrease systemic inflammation, thereby lowering MetS risk. The mediating roles of novel inflammatory markers warrant further investigation in diet and MetS research.
Compared to other diet quality indices, the HEI-2015 is unique as it quantifies conformance to federal dietary guidelines. However, associations between diet quality and inflammation/MetS likely reflect commonalities across indices like higher intakes of plant foods and unsaturated fats versus processed foods and saturated fats. For instance, the Mediterranean diet characterized by high intakes of fruits, vegetables, legumes, cereals, fish, and monounsaturated fatty acids has been linked to lower systemic inflammation and MetS prevalence (21, 22). The DASH diet targeting high intake of fruits, vegetables, low-fat dairy, whole grains, chicken, fish, nuts and low intake of red meats, sweets, and sugar-sweetened beverages also reduces CRP and other inflammatory markers (23). Overall diet quality appears more influential than individual components. Further research can continue examining how different diet quality indices relate to inflammation, MetS risks, and cardiometabolic health. Our study utilized the HEI to evaluate diet quality in relation to MetS risk among Americans, providing dietary strategies for MetS prevention.
Our research found that SII and uric acid partially mediated the relationship between HEI and MetS. The potential beneficial effects of diet quality on mitigating inflammation and MetS risk likely accumulate over time. Long-term adherence to high-quality diets emphases fruits, vegetables, fiber, plant proteins, and unsaturated fats while limiting red meats, saturated fats, processed foods, and sugars. These healthy dietary patterns characterized by high HEI scores have been associated with lower systemic inflammation marked by CRP, interleukin-6, E-selectin and other biomarkers in both cross-sectional and prospective studies (24–26). The compounding anti-inflammatory effects could alleviate insulin resistance, endothelial dysfunction, and dyslipidemia central to MetS pathogenesis (27). For example, the phytochemicals and antioxidants in plant foods may improve insulin signaling and glucose metabolism through regulating oxidative stress and inflammatory pathways (28). Omega-3 fatty acids can inhibit nuclear factor kappa B activation, suppressing production of inflammatory cytokines like tumor necrosis factor alpha and interleukin-6 (29). The high fiber content in whole grains, fruits and vegetables may also contribute by producing short-chain fatty acids upon fermentation, which hold immunomodulatory activities (30), lower levels of purines, thus reducing the production of uric acid. These plant-based foods are abundant in vitamins C and E, and marine foods are rich in polyphenols and flavonoids. The rich content of these bioactive compounds exerts potent anti-inflammatory and antioxidant effects, mitigating systemic immune-inflammatory responses, thereby lowering the SII (31, 32). Chronic inflammation can interfere with the normal function of insulin, leading to insulin resistance; the release of inflammatory cells and mediators contributes to dysregulated lipid metabolism, increasing the risk of MetS (11). Elevated uric acid levels may promote oxidative stress and cellular apoptosis, heightening the incidence of hypertension, gout, and cardiovascular diseases (33).In addition, higher HEI signifies more balanced nutrition intake, which can improve insulin sensitivity and reduce inflammatory cytokine production (21, 34). Future research is warranted to investigate the anti-inflammatory effects of high-quality diets and their impacts on MetS.
Our study boasts several innovative advantages, firstly, it is based on a large national NHANES sample, ensuring ample sample size and validity of the research outcomes. We constructed several weighted multivariate logistic regression models, adjusting for multiple variables to robustly assess the impact of HEI on the risk of incident MetS. Moreover, we utilized mediation analysis to further elucidate the potential mediating roles of SII and uric acid in the association between HEI and MetS. We employed the weighted quantile sum regression approach to test the cumulative effects of the 13 components of HEI on MetS and quantified the significant contributions of each component, substantially advancing the reliability and robustness of dietary characteristics associated with MetS.
This study has important public health implications regarding the role of diet quality in metabolic health. Our findings that better adherence to federal dietary guidelines assessed by HEI-2015 is associated with lower likelihood of MetS in Americans further highlights the value of the HEI as a diet quality monitoring tool. The index could help shape dietary recommendations and policies aimed at curbing the growing prevalence of obesity, diabetes, hypertension and related metabolic disorders. Specifically, the HEI-2015 components with the greatest weights in relation to MetS risk in our analysis—including seafood/plant proteins, sodium and empty calories—can inform targeted efforts to improve these aspects of diet quality at the population level. Future revisions to federal dietary guidelines and educational campaigns can also emphasize overall diet patterns aligned with HEI-2015 targets rather than individual nutrients or foods.
However, our study has some limitations. The cross-sectional analysis prevents causal determination of the relationship between diet quality and MetS. While the NHANES dataset is nationally representative, the self-reported dietary data may be subject to recall biases. We identified certain novel inflammatory biomarkers as mediators but did not measure an exhaustive profile. Future longitudinal cohorts could track participants’ diets using validated methods alongside periodic assessment of clinical parameters, MetS incidence and detailed inflammatory markers to elucidate long-term relationships. Examining how diet quality interacts with genetics, microbiome and other factors influencing MetS risks would also advance scientific understanding. Overall, our findings highlight diet quality as a modifiable factor and the utility of HEI-2015 to assess adherence to healthy dietary patterns for lowering metabolic disease burden.
5 Conclusion
In conclusion, we found that better diet quality assessed by HEI-2015 was associated with lower likelihood of having MetS in a nationally representative sample of US adults. Higher SII and serum uric acid levels were identified as risk factors for MetS and partial mediators. Our results highlight the importance of a high-quality diet and controlling inflammation in MetS prevention. The HEI-2015 index may be a useful tool to monitor diet quality at the population level. Our findings need to be confirmed by longitudinal studies elucidating the interrelationships between diet, inflammation and metabolic disorders. Nutrition strategies to improve diet quality, reduce inflammation and alleviate the growing burden of MetS warrant further investigation.
Data availability statement
The raw data supporting the conclusions of this article will be made available by the authors, without undue reservation.
Ethics statement
The studies involving humans were approved by the National Center for Health Statistics Research Ethics Review Board, duly approved by the ethical review committee (protocol #2011-17, #2018-01). The patients/participants provided their written informed consent to participate in this study.
Author contributions
LY: Data curation, Formal analysis, Visualization, Writing – original draft. YC: Data curation, Formal analysis, Visualization, Writing – original draft. HL: Data curation, Formal analysis, Writing – original draft. FL: Methodology, Project administration, Writing – original draft. QZ: Methodology, Project administration, Writing – original draft. XL: Conceptualization, Methodology, Supervision, Writing – original draft, Writing – review & editing. XC: Conceptualization, Supervision, Writing – review & editing. JC: Conceptualization, Methodology, Supervision, Writing – review & editing.
Funding
The author(s) declare that no financial support was received for the research, authorship, and/or publication of this article.
Acknowledgments
The authors express their gratitude to the NHANES project members for their extensive work in data collection and follow-up.
Conflict of interest
The authors declare that the research was conducted in the absence of any commercial or financial relationships that could be construed as a potential conflict of interest.
Publisher’s note
All claims expressed in this article are solely those of the authors and do not necessarily represent those of their affiliated organizations, or those of the publisher, the editors and the reviewers. Any product that may be evaluated in this article, or claim that may be made by its manufacturer, is not guaranteed or endorsed by the publisher.
Supplementary material
The Supplementary Material for this article can be found online at: https://www.frontiersin.org/articles/10.3389/fendo.2024.1293850/full#supplementary-material
References
1. Alberti KG, Eckel RH, Grundy SM, Zimmet PZ, Cleeman JI, Donato KA, et al. Harmonizing the metabolic syndrome: A joint interim statement of the international diabetes federation task force on epidemiology and prevention; National heart, lung, and blood institute; American Heart Association; World Heart Federation; international atherosclerosis society; and International association for the study of obesity. Circulation. (2009) 120:1640–5. doi: 10.1161/circulationaha.109.192644
2. Scott MG, Daniel B, Luther TC, Richard SC, Margo AD, Donald BH, et al. Executive summary of the third report of the national cholesterol education program (NCEP) expert panel on detection, evaluation, and treatment of high blood cholesterol in adults (Adult treatment panel III). Jama (2001) 285:2486–97. doi: 10.1001/jama.285.19.2486
3. O'Neill S, O'Driscoll L. Metabolic syndrome: A closer look at the growing epidemic and its associated pathologies. Obes Rev an Off J Int Assoc Study Obes (2015) 16:1–12. doi: 10.1111/obr.12229
4. Gustafson B. Adipose tissue, inflammation and atherosclerosis. J Atheroscl thrombosis. (2010) 17:332–41. doi: 10.5551/jat.3939
5. Krebs-Smith SM, Pannucci TE, Subar AF, Kirkpatrick SI, Lerman JL, Tooze JA, et al. Update of the healthy eating index: HEI-2015. J Acad Nutr Dietetics. (2018) 118:1591–602. doi: 10.1016/j.jand.2018.05.021
6. Zhang X, Guo Y, Yao N, Wang L, Sun M, Xu X, et al. Association between dietary inflammatory index and metabolic syndrome: Analysis of the NHANES 2005-2016. Front Nutr (2022) 9:991907. doi: 10.3389/fnut.2022.991907
7. Hiza HA, Casavale KO, Guenther PM, Davis CA. Diet quality of Americans differs by age, sex, race/ethnicity, income, and education level. J Acad Nutr Dietetics. (2013) 113:297–306. doi: 10.1016/j.jand.2012.08.011
8. Nicklas TA, O'Neil CE, Fulgoni VL 3rd. Diet quality is inversely related to cardiovascular risk factors in adults. J Nutr (2012) 142:2112–8. doi: 10.3945/jn.112.164889
9. Huffman FG, Maurcio DLC, Vaccaro JA, Zarini GG, Joel E, Deva G, et al. Healthy eating index and alternate healthy eating index among Haitian Americans and African Americans with and without type 2 diabetes. J Nutr Metab (2011) 2011:398324. doi: 10.1155/2011/398324
10. Grundy SM. Adipose tissue and metabolic syndrome: too much, too little or neither. Eur J Clin Invest (2015) 45:1209–17. doi: 10.1111/eci.12519
11. Chen JH, Zhai ET, Yuan YJ, Wu KM, Xu JB, Peng JJ, et al. Systemic immune-inflammation index for predicting prognosis of colorectal cancer. World J gastroenterology. (2017) 23:6261–72. doi: 10.3748/wjg.v23.i34.6261
12. Wang X, Bao W, Liu J, Ouyang YY, Wang D, Rong S, et al. Inflammatory markers and risk of type 2 diabetes: A systematic review and meta-analysis. Diabetes Care (2013) 36:166–75. doi: 10.2337/dc12-0702
13. Fu Y, Luo N, Klein RL, Garvey WT. Adiponectin promotes adipocyte differentiation, insulin sensitivity, and lipid accumulation. J Lipid Res (2005) 46:1369–79. doi: 10.1194/jlr.M400373-JLR200
14. Li Y, Xu C, Yu C, Xu L, Miao M. Association of serum uric acid level with non-alcoholic fatty liver disease: A cross-sectional study. J hepatology. (2009) 50:1029–34. doi: 10.1016/j.jhep.2008.11.021
15. Moshfegh AJ, Rhodes DG, Baer DJ, Murayi T, Clemens JC, Rumpler WV, et al. The US department of agriculture automated multiple-pass method reduces bias in the collection of energy intakes. Am J Clin Nutr (2008) 88:324–32. doi: 10.1093/ajcn/88.2.324
16. Reedy J, Lerman JL, Krebs-Smith SM, Kirkpatrick SI, Pannucci TE, Wilson MM, et al. Evaluation of the healthy eating index-2015. J Acad Nutr Dietetics. (2018) 118:1622–33. doi: 10.1016/j.jand.2018.05.019
17. Nøst TH, Alcala K, Urbarova I, Byrne KS, Guida F, Sandanger TM, et al. Systemic inflammation markers and cancer incidence in the UK Biobank. Eur J Epidemiol (2021) 36:841–8. doi: 10.1007/s10654-021-00752-6
18. Yorita Christensen KL, Carrico CK, Sanyal AJ, Gennings C. Multiple classes of environmental chemicals are associated with liver disease: NHANES 2003-2004. Int J hygiene Environ Health (2013) 216:703–9. doi: 10.1016/j.ijheh.2013.01.005
19. Park S, Kim K, Lee BK, Ahn J. A healthy diet rich in calcium and vitamin C Is inversely associated with metabolic syndrome risk in Korean adults from the KNHANES 2013-2017. Nutrients. (2021) 13:1300–12. doi: 10.3390/nu13041312
20. Saraf-Bank S, Haghighatdoost F, Esmaillzadeh A, Larijani B, Azadbakht L. Adherence to healthy eating index-2010 is inversely associated with metabolic syndrome and its features among Iranian adult women. Eur J Clin Nutr (2017) 71:425–30. doi: 10.1038/ejcn.2016.173
21. Esposito K, Marfella R, Ciotola M, Di Palo C, Giugliano F, Giugliano G, et al. Effect of a mediterranean-style diet on endothelial dysfunction and markers of vascular inflammation in the metabolic syndrome: A randomized trial. Jama. (2004) 292:1440–6. doi: 10.1001/jama.292.12.1440
22. Kastorini CM, Milionis HJ, Esposito K, Giugliano D, Goudevenos JA, Panagiotakos DB. The effect of Mediterranean diet on metabolic syndrome and its components: a meta-analysis of 50 studies and 534,906 individuals. J Am Coll Cardiol (2011) 57:1299–313. doi: 10.1016/j.jacc.2010.09.073
23. Saneei P, Salehi-Abargouei A, Esmaillzadeh A, Azadbakht L. Influence of Dietary Approaches to Stop Hypertension (DASH) diet on blood pressure: A systematic review and meta-analysis on randomized controlled trials. Nutrition metabolism Cardiovasc Dis NMCD. (2014) 24:1253–61. doi: 10.1016/j.numecd.2014.06.008
24. Ma Y, Hébert JR, Li W, Bertone-Johnson ER, Olendzki B, Pagoto SL, et al. Association between dietary fiber and markers of systemic inflammation in the Women's health initiative observational study. Nutr (Burbank Los Angeles County Calif). (2008) 24:941–9. doi: 10.1016/j.nut.2008.04.005
25. Lefevre M, Jonnalagadda S. Effect of whole grains on markers of subclinical inflammation. Nutr Rev (2012) 70:387–96. doi: 10.1111/j.1753-4887.2012.00487.x
26. Soltani S, Chitsazi MJ, Salehi-Abargouei A. The effect of dietary approaches to stop hypertension (DASH) on serum inflammatory markers: A systematic review and meta-analysis of randomized trials. Clin Nutr (Edinburgh Scotland). (2018) 37:542–50. doi: 10.1016/j.clnu.2017.02.018
27. Monteiro R, Azevedo I. Chronic inflammation in obesity and the metabolic syndrome. Mediators inflammation. (2010) 2010:1–10. doi: 10.1155/2010/289645
28. Wang Q, Yuan J, Yu Z, Lin L, Jiang Y, Cao Z, et al. FGF21 Attenuates High-Fat Diet-Induced cognitive impairment via metabolic regulation and Anti-inflammation of Obese Mice. Mol neurobiology. (2018) 55:4702–17. doi: 10.1007/s12035-017-0663-7
29. Li K, Huang T, Zheng J, Wu K, Li D. Effect of marine-derived n-3 polyunsaturated fatty acids on C-reactive protein, interleukin 6 and tumor necrosis factor α: A meta-analysis. PloS One (2014) 9:e88103. doi: 10.1371/journal.pone.0088103
30. Trompette A, Gollwitzer ES, Yadava K, Sichelstiel AK, Sprenger N, Ngom-Bru C, et al. Gut microbiota metabolism of dietary fiber influences allergic airway disease and hematopoiesis. Nat Med (2014) 20:159–66. doi: 10.1038/nm.3444
31. Casas R, Sacanella E, Estruch R. The immune protective effect of the Mediterranean diet against chronic low-grade inflammatory diseases. Endocrine Metab Immune Disord Drug targets. (2014) 14:245–54. doi: 10.2174/1871530314666140922153350
32. Ahmed MH, Byrne CD. Modulation of sterol regulatory element binding proteins (SREBPs) as potential treatments for non-alcoholic fatty liver disease (NAFLD). Drug Discovery Today (2007) 12:740–7. doi: 10.1016/j.drudis.2007.07.009
33. Copur S, Demiray A, Kanbay M. Uric acid in metabolic syndrome: Does uric acid have a definitive role? Eur J Internal Med (2022) 103:4–12. doi: 10.1016/j.ejim.2022.04.022
Keywords: healthy eating index, metabolic syndrome, mediation effect, NHANES, chronic inflammation
Citation: Yuguang L, Chang Y, Li H, Li F, Zou Q, Liu X, Chen X and Cui J (2024) Inflammation mediates the relationship between diet quality assessed by healthy eating index-2015 and metabolic syndrome. Front. Endocrinol. 15:1293850. doi: 10.3389/fendo.2024.1293850
Received: 13 September 2023; Accepted: 16 January 2024;
Published: 05 February 2024.
Edited by:
Sijung Yun, Predictiv Care, Inc., United StatesReviewed by:
Carol Johnston, Arizona State University, United StatesMiguel Rebollo-Hernanz, Autonomous University of Madrid, Spain
Copyright © 2024 Yuguang, Chang, Li, Li, Zou, Liu, Chen and Cui. This is an open-access article distributed under the terms of the Creative Commons Attribution License (CC BY). The use, distribution or reproduction in other forums is permitted, provided the original author(s) and the copyright owner(s) are credited and that the original publication in this journal is cited, in accordance with accepted academic practice. No use, distribution or reproduction is permitted which does not comply with these terms.
*Correspondence: Xiangliang Liu, ZHM5MjkxQHFxLmNvbQ==; Xiao Chen, Y2hlbnhpYW9Aamx1LmVkdS5jbg==; Jiuwei Cui, Y3VpandAamx1LmVkdS5jbg==
†These authors have contributed equally to this work and share first authorship