- 1Institute of Urology, Lanzhou University Second Hospital, Lanzhou University, Lanzhou, China
- 2Institute of Urology, The Affiliated Luohu Hospital of Shenzhen University, Shenzhen University, Shenzhen, China
- 3Institute of Urology, South China Hospital, Health Science Center, Shenzhen University, Shenzhen, China
Background: Previous observational researchers have found an inverse bidirectional link between Alzheimer’s disease (AD) and prostate cancer (PCa); yet, the causative nature of this link remains unclear. To investigate the causal interactions between AD and PCa, a bidirectional Mendelian randomization (MR) analysis was conducted.
Methods: This study comprised two Genome-Wide Association Study (GWAS) summary statistics for AD (17,008 cases and 37,154 controls) and PCa (79,148 cases and 61,106 controls) in individuals of European ancestry. The inverse-variance weighted (IVW) method was employed as the primary approach, while MR-Egger, weighted median, weighted mode, and simple mode served as supplementary methods for estimating the causal effect. To assess pleiotropy, the MR-PRESSO global test and MR-Egger regression were used. Cochran’s Q test was adopted to check heterogeneity, MR Steiger test and the leave-one-out analysis was performed to confirm the robustness and reliability of the results.
Results: The causal association genetically inferred of AD on PCa was found using IVW (OR = 0.974, 95% CI = 0.958-0.991, p = 0.003) in forward MR analysis and the causal association genetically inferred of PCa on AD was not found using IVW (OR = 1.000, 95% CI: 0.954-1.049, P = 0.988) in reverse MR analysis. The sensitivity analysis showed that no pleiotropy and heterogeneity was observed. The leave-one-out analysis showed that the findings were not inordinately affected by any instrumental variables.
Conclusion: The results of this study demonstrated an absence of bidirectional causality between AD and PCa among the European population, suggested that a genetically predicted possibility of decreased PCa risk in AD patients, and no significant genetically predicted causal effect of PCa on AD.
1 Introduction
Prostate cancer (PCa) represents a prevalent malignancy in elderly males, especially in Western countries, and ranks as the second most frequent cause of cancer-related mortality in the male population (1). Despite advancements in the therapeutic approaches for PCa, there is considerable geographic variability in its occurrence, with all regions experiencing a consistent annual surge in cases (2). Variations in the disease’s progression are attributed to the pathological diversity and the heterogeneity present within the cancerous cells (3). At the moment of PCa diagnosis, nearly 90% of affected individuals exhibit locoregional advancement of the tumor, which often disqualifies them from undergoing surgical interventions (4). Established risk determinants include age, genetic predisposition, and racial or ethnic origins, while the significance of other potential causative factors is still a subject of debate (5). Considering the significant impact of PCa on a global scale, it is imperative to investigate both protective and risk elements of PCa and to implement prompt interventions, aiming to enhance the prognosis for those diagnosed with the disease.
Alzheimer’s disease (AD) stands as the predominant neurodegenerative condition in the aging population, clinically manifesting through memory-related cognitive decline and pathologically distinguished by the presence of extracellular plaques rich in β-amyloid (Aβ) and intracellular neurofibrillary tangles composed of tau protein. The principal risk factor for AD is advanced age (6–8). Recent research has unveiled a reciprocal negative correlation between Alzheimer’s disease and cancer incidence. Studies have indicated that individuals with AD have their cancer risk halved, while a 35% decrease in the likelihood of AD has been noted among individuals who have had or recovered from cancer (9, 10). Prior observational research has suggested that those afflicted with AD may have a diminished probability of developing prostate cancer (11). And, Sherzai et al. observed that patients with PCa have a lower probability of developing AD (12). Such findings highlight a potential association between AD and PCa.
The majority of research examining the link between AD and PCa has utilized cross-sectional or retrospective designs, with a scarcity of prospective studies conducted. Observational studies have not been able to thoroughly investigate the causal relationship between AD and PCa. These studies often face challenges such as limited participant numbers and the absence of randomization. Mendelian randomization (MR) employs genetic variations, usually in the form of single-nucleotide polymorphisms (SNPs), as tools for evaluating the causal influence of an exposure on an outcome (13–15). The MR approach mirrors the principles of a randomized controlled trial, is structured to circumvent biases stemming from confounders that are not accounted for and to prevent biases due to reverse causality (15, 16). And genetic data for this method are frequently sourced from extensive genome-wide association studies (GWAS). As a result, MR offers a time-efficient and economical strategy for discovering potential causal links (17). In this study, a bidirectional two-sample MR approach was utilized to explore the causal relationships between AD and PCa within European demographic groups.
2 Methods
2.1 Study design and ethics statement
Figure 1 illustrated the schematic of our study design along with the three critical assumptions inherent in the MR study. The first assumption asserts that the instrumental variables (IVs) have a robust association with exposure (AD or PCa). The second assumption contends that the IVs are not affected by confounding variables. The third assumption holds that the IVs exert an effect on the likelihood of exposure exclusively through their interaction with outcome, without any indirect routes (18). Since our data were derived from previously conducted studies or databases available to the public, there was no requirement to obtain additional ethical approval from an ethics committee. In our research, we consistently followed the STROBE-MR guidelines (19, 20), with Supplementary Material presenting a checklist that illustrates our adherence to these protocols.
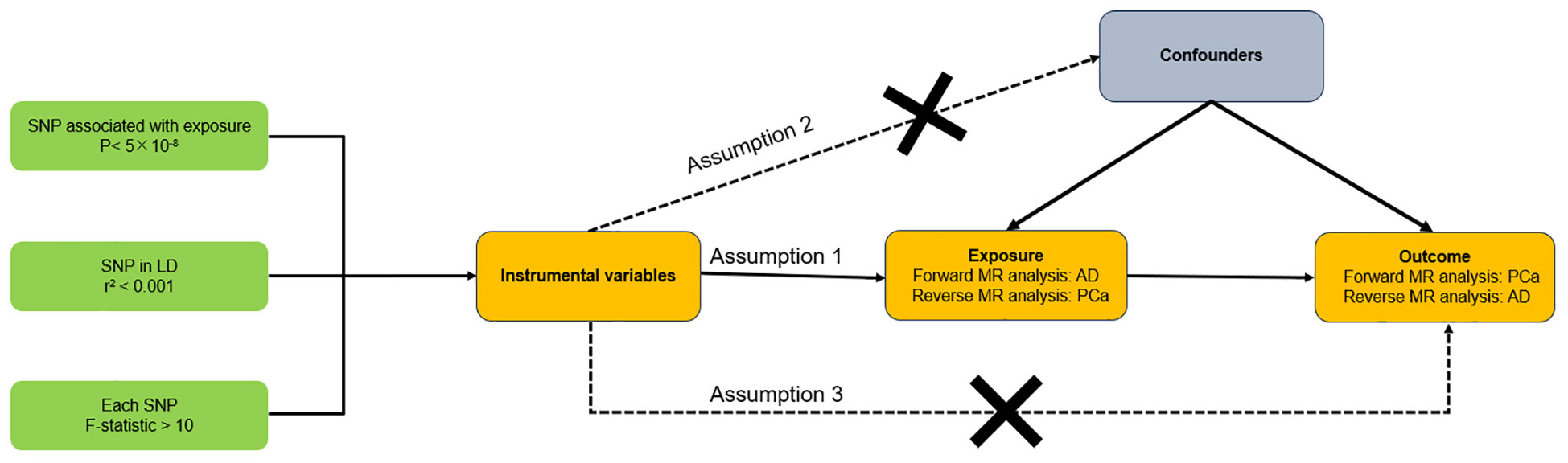
Figure 1 Overall design of this bidirectional Mendelian randomization analysis. AD, Alzheimer’s disease; PCa, Prostate cancer.
2.2 Data source
Our research utilized subjects of European descent from the Integrative Epidemiology Unit (IEU) GWAS database (https://gwas.mrcieu.ac.uk/). All data were extracted from the IEU GWAS database. We identified genetic variants associated with AD by analyzing AD GWAS data (ebi-a-GCST002245) comprising a cohort of 54,162 individuals (21). To avoid overlap between the populations assessed for exposure and outcome, we obtained summary data for prostate cancer from the largest GWAS meta-analysis conducted by the PRACTICAL consortium, which included 79,148 cases and 61,106 controls of European descent (22). The average age of participants with prostate cancer in the PRACTICAL study was 66 years. The distribution of disease stages among these patients was as follows: low aggression, characterized by T0 or T1, Gleason Score ≤6, and PSA <10, accounted for 12.1%; intermediate aggression, defined by T2 or Gleason Score = 7 or PSA between 10 and 20, comprised 37.9%; high aggression, indicated by T3, T4, N1, M1, Gleason Score ≥8, or PSA >20, made up 26.8%; and advanced stages, which included Gleason Score 8+, metastatic disease, PSA >100, or prostate cancer as cause of death according to the previous aggressiveness definition from iCOGS and accessible phenotype data, constituted 20.1% (22). Table 1 provides comprehensive details about the two sets of GWAS summary data.
2.3 Selection of instrumental variables
Utilizing the aforementioned GWAS summary data, a rigorous method was adopted to select appropriate SNPs as instrumental variables (IVs). The selection process began with the identification of SNPs that were strongly associated with the exposure, demonstrated by a genome-wide significant P-value threshold of less than 5×10-8. To prevent skewed results due to linkage disequilibrium (LD), we applied a clumping procedure with a stringent r2 threshold of 0.001 and a 10,000 kb window size. For further refinement, the Phenoscanner database (http://www.phenoscanner.medschl.cam.ac.uk/) was employed to filter out genetic variants linked to potential confounders. In cases where the identified SNPs were absent in the outcome GWAS dataset, we sought substitute proxy SNPs that exhibited a high LD (r2 > 0.8) with the initial SNPs. Furthermore, to eliminate confounding from palindromic and ambiguous SNPs with mismatching alleles, we harmonized the exposure and outcome data to ensure consistency in the effect alleles between them. In addition, to address the concern of bias due to weak IVs, we assessed the strength of the IVs by calculating the F-statistic, with an F-statistic significantly greater than 10 indicating a reduced risk of weak instrument bias (23, 24).
2.4 Statistical analysis
In this study, various techniques were employed to examine and measure the causal relationships and effects between the exposure and the outcome. These techniques encompassed the inverse-variance weighted (IVW) (25), MR-Egger (26), weighted median (27), simple mode (28) and weighted mode (29). The IVW approach, commonly used in MR analyses, has both random-effects and fixed-effects models. It acts as a meta-analytic tool that aggregates the individual Wald ratio estimates from each IV to provide a consolidated estimate of the effect of exposure on the outcome. The IVW method’s estimates are most credible in the absence of heterogeneity and pleiotropy (30). A random-effects model was implemented when substantial heterogeneity was detected among IVs (p < 0.05); otherwise, a fixed-effect model was chosen. The MR-Egger regression is designed to yield reliable estimates even in the presence of pleiotropy among IVs (31). The weighted median technique is capable of producing causal effect estimates provided that less than half of the IVs breach essential MR preconditions (27). The weighted mode method is suitable for MR causal analysis when the majority of the IVs are valid (29). The simple mode approach offers a less robust alternative to IVW. All these analyses were performed and graphically represented using R version 4.3.1, leveraging the “MRPRESSO” and “TwoSampleMR” R packages. A p-value of less than 0.05 was considered statistically significant.
2.5 Sensitivity analysis
We utilized the MR-PRESSO global test and the MR-Egger regression to evaluate pleiotropy in the instrumental variables, with pleiotropy indicated by a p-value of less than 0.05 (26, 32). We assessed heterogeneity through Cochran’s Q statistic, deeming it significant at p < 0.05. The MR Steiger test was used to verify the directionality that exposure causes the outcome (33). Additionally, the “leave-one-out” sensitivity analysis was performed to ascertain whether any single SNP might exert undue influence on the aggregate causal inference.
3 Results
3.1 Selection of instrumental variables
In accordance with rigorous criteria for selecting instrumental SNPs, we chose suitable SNPs as IVs that conformed to three essential assumptions. We pinpointed 13 SNPs with a high correlation to AD, and 89 SNPs with a high correlation to PCa. These SNPs acted as IVs for exposure (AD or PCa), and each SNP worked out as F-statistic greater than 10, suggesting a low chance of weak IV bias. Detailed information of included SNPs was showed in Supplementary Tables 1, 2.
3.2 Causal effects of Alzheimer’s disease on prostate cancer
The outcomes of forward MR analysis of the causality of AD on PCa were shown in Table 2. IVW revealed a statistically significant negative causal impact of AD on PCa (OR = 0.974, 95% CI = 0.958-0.991, p = 0.003). Simultaneously, a relationship following the same trend was discerned through MR Egger method (OR = 0.974, 95% CI =0.953-0.996, p = 0.039), the weighted median method (OR = 0.975, 95% CI = 0.957-0.993, p = 0.007) and Weighted mode method (OR = 0.975, 95% CI = 0.957-0.993, p = 0.020). These results were graphically represented in both the forest plot (Supplementary Figure 1) and the scatter plot (Figure 2). The forest plot visually displayed the effect estimates alongside their confidence intervals (CI) for each SNP, whereas the scatter diagram graphically portrayed the association between the exposure (AD) and the outcome (PCa) utilizing the IVs. Given the simple mode approach offers a less robust alternative to IVW, the outcomes from the MR analysis provide support for a causal association between AD and PCa.
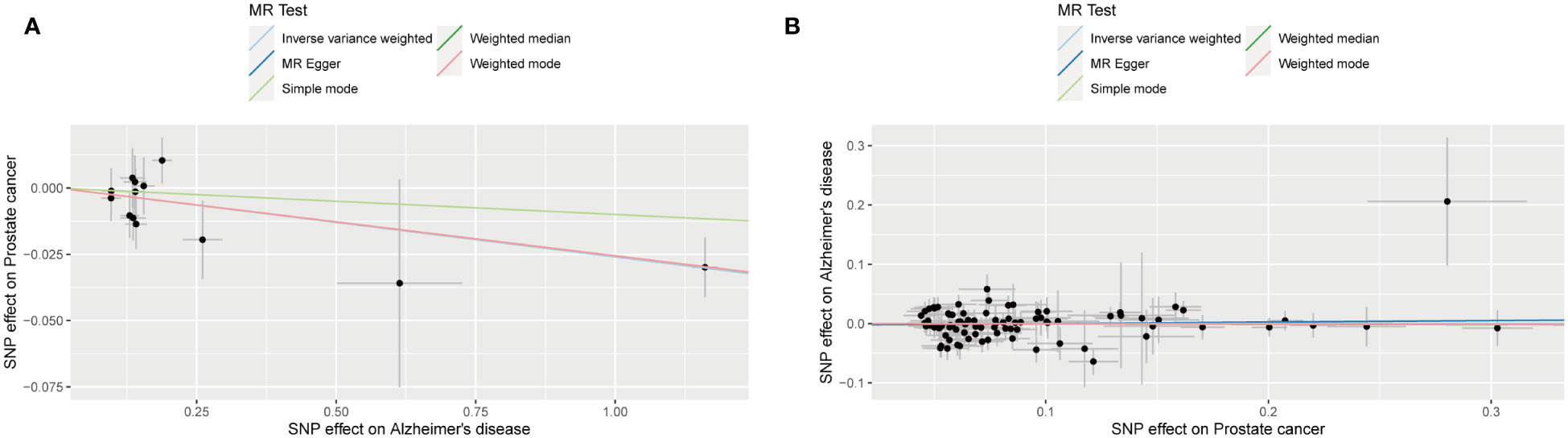
Figure 2 Scatter plot for the causality of Alzheimer’s disease on prostate cancer risk in forward MR analysis (A) and Scatter plot for the causality of prostate cancer on Alzheimer’s disease risk in reverse MR analysis (B). The regression slopes of the lines represent the magnitude of the causal effect.
3.3 Causal effects of prostate cancer on Alzheimer’s disease
The outcomes of reverse MR analysis of the causality of PCa on AD were shown in Table 3. The findings from the IVW analysis indicated that PCa did not causally influence AD (OR = 1.000, 95% CI: 0.954-1.049, P = 0.988), with the MR-Egger, Weighted Median, Weighted mode and Simple Mode approaches yielding congruent outcomes. The forest plot and the scatter plot were shown in Supplementary Figure 1 and Figure 2. Thus, our results showed that there is no significant causal relationship of PCa on AD.
3.4 Sensitivity analysis
Pleiotropy of IVs was examined through the application of MR-Egger regression and MR-PRESSO global test. The MR-Egger regression inferred that no pleiotropy in IVs (p = 0.994 in forward MR analysis, p = 0.597 in reverse MR analysis, Table 4), a conclusion that was further corroborated by the MR-PRESSO global test (p = 0.877 in forward MR analysis, p = 0.132 in reverse MR analysis, Table 3). Cochran’s Q test was employed to identify heterogeneity in IVs. The study revealed no heterogeneity in forward MR analysis and reverse MR analysis by the Cochran Q-test (p = 0.729 for MR-Egger, p = 0.799 for IVW in forward MR analysis, p = 0.122 for MR-Egger, p = 0.133 for IVW in reverse MR analysis, Table 4) and funnel plots (Supplementary Figure 2). The MR Steiger test identified no evidence of reverse causality, and the causal direction was reliable (Table 4). A leave-one-out analysis was conducted, sequentially excluding each SNP to assess the impact on the results (Supplementary Figure 3). This analysis in forward MR analysis indicated that no single SNP significantly influenced the causal inference, suggesting that the observed overall causal link between AD and PCa was not propelled by any particular SNP, underlining the robustness of the results. These outcomes provide confidence in the validity and robustness of the causal inference derived from the MR analysis.
4 Discussion
Previous epidemiological research examining the link between AD and PCa suggests that those diagnosed with AD exhibit a reduced occurrence of PCa, while survivors of PCa show a decreased incidence of AD. Such epidemiological investigations are primarily hypothesis-generating regarding causation and are inevitably constrained by biases and confounding variables. We employed a bidirectional two-sample MR technique to examine the potential causal link between AD and PCa. Analyses using MR methodologies revealed an absence of bidirectional causality between AD and PCa. In particular, our analysis indicated a possibility of decreased PCa risk in AD patients, yet no significant causal effect of PCa on AD was observed. These results offer important perspectives regarding the prospective causal dynamics between the two disorders.
With the rise in the elderly population, there has been a corresponding increase in the prevalence of conditions like cancer and neurodegenerative diseases. Past research focusing mainly on white demographics has found an association between AD and a reduced cancer risk, ranging from 36% to 80%, though this reduction rate differs according to the study design (34–37). Similarly, a retrospective cohort study in Shanghai, China, and a population-based longitudinal study in South Korea have indicated a potential reduced risk for several cancers, included prostate cancer, in patients with AD (11, 38). The results of our study are consistent with these earlier findings.
Several studies have demonstrated an inverse correlation between cancer and AD. An earlier study from Italy indicated that the prevalence of AD did not increase in PC patients (36), while research from Taiwan noted a negligible rise in AD prevalence among PC patients compared to the broader population (39). Individuals who have survived certain cancers appear to have a diminished likelihood of developing AD, rather than other age-related ailments, which suggests that the reduced AD diagnoses are not solely attributable to biases. It has been posited that cancer therapy may be linked to a lower risk of AD (40). Our study showed that no genetic causal association of PCa on AD by MR analysis.
Utilizing discharge information sourced from the National Inpatient Sample (NIS) spanning 1999 to 2008, this subsequent analysis of existing data additionally revealed a reverse correlation between Alzheimer’s disease (AD) and ten types of cancer, where prostate, ovarian, and lung cancers showed the most significant reverse associations (12). Drawing on a cohort study conducted in Northern Italy involving over 1 million residents, it was found that the incidence of cancer in individuals with AD dementia was reduced by half, while the likelihood of developing AD dementia in cancer patients decreased by 35%. The incidence rates for cancers originating from various tissues in individuals with AD dementia were consistently below expected levels; this decrease in risk was statistically significant for cancers arising from epithelial tissues, as well as those from non-epithelial, mesenchymal, blood, or nervous tissues. The number of AD dementia cases among the five common cancer locations (breast, lung, bladder, prostate, colorectal) always fell short of expectations. Nonetheless, the relative risk (RR) showed a significant decline only in individuals with colorectal cancer or with cancers of non-epithelial, mesenchymal, blood, or nervous tissues. The frequency of the five cancer types ((breast, lung, bladder, prostate, colorectal)) in AD dementia patients was below anticipated levels, though the observed prostate cancer cases were nearly the same as expected. Notably, the risk for developing lung and colorectal cancers, along with tumors of non-epithelial, mesenchymal, blood, or nervous tissues, was significantly lower (36). A retrospective cohort study conducted in Shanghai indicates that individuals with AD may have a reduced likelihood of developing various cancers, such as lung, prostate, and testicular cancer. Concurrently, an increased incidence of lymphoma was found to be positively associated with AD (11).
Yuan et al. investigated the bidirectional causality between AD and colorectal cancer (CRC) using a two-sample MR approach. The MR findings indicated a lower likelihood of AD in individuals with CRC, alongside a marginally increased probability of CRC in those diagnosed with AD. However, the reliability of the latter finding is compromised by the effect of overlapping samples (41). Sahba Seddighi and colleagues utilized MR to investigate the causal link between cancer and AD. They found that cancers related to smoking, as predicted genetically, were linked to a 5.2% decrease in the likelihood of AD (OR 0.95, 95% CI 0.92 -0.98, p = 0.0026) for every 1-unit increase in log odds of cancer. Among these, only lung cancer predicted genetically, showing a 9.0% reduction, had a significant association with AD (OR 0.91, 95% CI 0.84-0.99, p = 0.019) per 1-unit increase in log odds of cancer. Cancers not related to smoking, as predicted genetically, were linked to a 1.9% decrease in the odds of AD (OR 0.98, 95% CI 0.97-0.995, p = 0.0091) for each 1-unit increase in log odds of cancer. Within this category, prostate cancer, melanoma, lymphoma, and ovarian cancer, as predicted genetically, showed no significant association with the odds of developing AD. Conversely, leukemia predicted genetically was associated with a 2.4% reduction in AD odds (OR 0.98, 95% CI 0.96- 0.995, p = 0.012) per 1-unit increase in log odds of cancer, and breast cancer was linked to a 5.9% lower likelihood of AD (OR 0.94, 95% CI 0.89-0.99, p = 0.028) for each 1-unit increase in log odds of cancer. Combining genetic predictors for all types of cancer considered in the study, the overall association pointed to a 2.5% reduction in the odds of AD (OR 0.98, 95% CI 0.96-0.99, p = 0.00027) for every 1-unit increase in log odds of cancer (42).
An intriguing theory accounting for the inverse relationship between cancer and AD posits a common etiology that exerts divergent effects on carcinogenesis and neurodegenerative processes (43). Proposed biological pathways include proteins that inhibit tau accumulation and amyloid-β aggregation, as well as those that control cell cycle events (44, 45), common epigenetic alterations (46), and aging-associated disruptions in cell metabolism (47). This hypothesized common etiology offers a significant chance to enhance our understanding of the underlying mechanisms of both carcinogenesis and neurodegenerative conditions (43, 48, 49).
The association between cancer and AD seems to related to disruptions in the regulation of the cell cycle (50). Proteins such as P53, Wnt, and Pin1 are involved in managing the cell cycle for both diseases. The Pin1 gene plays a role in cancer and AD by influencing cell cycle control, signal transduction, DNA damage response, as well as the management of tau and β-amyloid (Aβ) precursor proteins (37, 51). In AD patient brain tissues, Pin1 expression is typically diminished, while in several cancer forms, such as prostate cancer, an increased expression of Pin1 is observed (12, 51–54). Research involving animals indicates that the absence of Pin1 hinders the development of oncogenes and inhibits both tumor and cellular proliferation (12, 53). Simultaneously, a reduced expression of Pin1 may contribute to enhanced neurodegeneration in AD (55). Experiments with animals have shown that elevated Pin1 levels in postnatal neurons can counteract neurodegeneration (51, 56, 57). The depletion of Pin1 in animals results in tau and Aβ-related pathological changes that occur in an age-dependent fashion, mirroring those in AD (12). Due to Pin1’s direct involvement with both cancer and AD, it has garnered considerable interest as a potential therapeutic target and warrants further exploration.
This investigation presented multiple strengths. First, this study utilized MR analysis, a method in genetic epidemiology that applies instrumental variables to assess the causal impact of exposures (AD or PCa) on outcomes (PCa or AD). An earlier MR study reported that prostate cancer did not affect AD (42). However, the bidirectional analysis conducted in this research represents the novelty of the study. Second, the principles of MR analysis ensured resistance to confounder-induced biases and forestalled reverse causality in the MR analysis. The use of published GWAS data provided access to a large cohort and extensive information on genetic diversity. Consequently, the outcomes of this study suggested a potential reduction in PCa risk among individuals with AD, while no significant causal relationship was found from PCa towards AD, implying a notable causal effect of increased AD risk leading to a lower PCa risk. The robustness of these results was further validated through sensitivity analysis. Lastly, the selection of study participants from a European demographic reduced the likelihood of biases related to population stratification.
Nonetheless, this study was subject to certain limitations. First, the GWAS summary statistics were restricted to individuals with European ancestry, hence our conclusions may be primarily applicable to European cohorts. Therefore, extending the application of our findings to racially and ethnically different populations should be done with caution. Second, constraints in our data sources precluded the possibility of performing analyses that were stratified or adjusted for other variables. More exhaustive investigations, such as future prospective randomized controlled trials, could provide deeper insights to the inferences made in this study. Third, the GWAS data for PCa are derived exclusively from males. Unfortunately, GWAS data for AD with gender stratification are not currently available. Consequently, the GWAS data for AD used in this study include both males and females. Ideally, using male-only GWAS data for traits related to AD would be preferable. Lastly, the capability of the MR approach was confined to examining causal links; it did not permit the exploration of the underlying mechanisms behind these associations. Elucidating these mechanisms would necessitate more detailed research.
5 Conclusion
In summary, we demonstrated an absence of bidirectional causality between AD and PCa among the European population, employing a bidirectional two-sample MR analysis. Our findings suggested that a genetically predicted possibility of decreased PCa risk in AD patients, and no significant genetically predicted causal effect of PCa on AD. Our results provided new evidence for discovering potential relationship between AD and PCa. Further studies focused on mechanisms are necessary to clarify the complex relationship between AD and PCa.
Data availability statement
The original contributions presented in the study are included in the article/Supplementary Material. Further inquiries can be directed to the corresponding authors.
Author contributions
RL: Conceptualization, Data curation, Formal analysis, Investigation, Software, Writing – original draft. LP: Conceptualization, Data curation, Formal analysis, Investigation, Software, Writing – original draft. DD: Formal analysis, Software, Writing – original draft. GL: Funding acquisition, Methodology, Project administration, Resources, Supervision, Writing – review & editing. SW: Funding acquisition, Methodology, Project administration, Resources, Supervision, Writing – review & editing.
Funding
The author(s) declare financial support was received for the research, authorship, and/or publication of this article. This work was partially supported by the Medical Scientific Research Foundation of Guangdong Province (A2022024), the National Natural Science Foundation of China (61731018), Sanming Project of Medicine in Shenzhen (SZSM202211009) and Shenzhen Science and Technology Innovation Commission (RCJC20200714114557005).
Conflict of interest
The authors declare that the research was conducted in the absence of any commercial or financial relationships that could be construed as a potential conflict of interest.
Publisher’s note
All claims expressed in this article are solely those of the authors and do not necessarily represent those of their affiliated organizations, or those of the publisher, the editors and the reviewers. Any product that may be evaluated in this article, or claim that may be made by its manufacturer, is not guaranteed or endorsed by the publisher.
Supplementary material
The Supplementary Material for this article can be found online at: https://www.frontiersin.org/articles/10.3389/fendo.2024.1354528/full#supplementary-material
References
1. Siegel RL, Miller KD, Fuchs HE, Jemal A. Cancer statistics, 2022. CA Cancer J Clin. (2022) 72:7–33. doi: 10.3322/caac.21708
2. Kimura T, Sato S, Takahashi H, Egawa S. Global trends of latent prostate cancer in autopsy studies. Cancers (Basel). (2021) 13. doi: 10.3390/cancers13020359
3. Mo F, Lin D, Takhar M, Ramnarine VR, Dong X, Bell RH, et al. Stromal gene expression is predictive for metastatic primary prostate cancer. Eur Urol. (2018) 73:524–32. doi: 10.1016/j.eururo.2017.11.031
4. Li W, Middha M, Bicak M, Sjoberg DD, Vertosick E, Dahlin A, et al. Genome-wide scan identifies role for AOX1 in prostate cancer survival. Eur Urol. (2018) 74:710–9. doi: 10.1016/j.eururo.2018.06.021
5. Zi H, He SH, Leng XY, Xu XF, Huang Q, Weng H, et al. Global, regional, and national burden of kidney, bladder, and prostate cancers and their attributable risk factors, 1990-2019. Mil Med Res. (2021) 8:60. doi: 10.1186/s40779-021-00354-z
6. Knopman DS, Amieva H, Petersen RC, Chetelat G, Holtzman DM, Hyman BT, et al. Alzheimer disease. Nat Rev Dis Primers. (2021) 7:33. doi: 10.1038/s41572-021-00269-y
7. Eratne D, Loi SM, Farrand S, Kelso W, Velakoulis D, Looi JC. Alzheimer’s disease: clinical update on epidemiology, pathophysiology and diagnosis. Australas Psychiatry. (2018) 26:347–57. doi: 10.1177/1039856218762308
8. Scheltens P, De Strooper B, Kivipelto M, Holstege H, Chetelat G, Teunissen CE, et al. Alzheimer’s disease. Lancet. (2021) 397:1577–90. doi: 10.1016/S0140-6736(20)32205-4
9. Rogers NK, Romero C, SanMartin CD, Ponce DP, Salech F, Lopez MN, et al. Inverse relationship between alzheimer’s disease and cancer: how immune checkpoints might explain the mechanisms underlying age-related diseases. J Alzheimers Dis. (2020) 73:443–54. doi: 10.3233/JAD-190839
10. Poddar NK, Agarwal D, Agrawal Y, Wijayasinghe YS, Mukherjee A, Khan S. Deciphering the enigmatic crosstalk between prostate cancer and Alzheimer’s disease: A current update on molecular mechanisms and combination therapy. Biochim Biophys Acta Mol Basis Dis. (2022) 1868:166524. doi: 10.1016/j.bbadis.2022.166524
11. Ren RJ, Huang Q, Xu G, Gu K, Dammer EB, Wang CF, et al. Association between Alzheimer’s disease and risk of cancer: A retrospective cohort study in Shanghai, China. Alzheimers Dement. (2022) 18:924–33. doi: 10.1002/alz.12436
12. Sherzai AZ, Parasram M, Haider JM, Sherzai D. Alzheimer disease and cancer: A national inpatient sample analysis. Alzheimer Dis Assoc Disord. (2020) 34:122–7. doi: 10.1097/WAD.0000000000000369
13. Smith GD, Ebrahim S. ‘Mendelian randomization’: can genetic epidemiology contribute to understanding environmental determinants of disease? Int J Epidemiol. (2003) 32:1–22. doi: 10.1093/ije/dyg070
14. Lee K, Lim CY. Mendelian randomization analysis in observational epidemiology. J Lipid Atheroscler. (2019) 8:67–77. doi: 10.12997/jla.2019.8.2.67
15. Emdin CA, Khera AV, Kathiresan S. Mendelian randomization. JAMA. (2017) 318:1925–6. doi: 10.1001/jama.2017.17219
16. Smith GD, Ebrahim S. Mendelian randomization: prospects, potentials, and limitations. Int J Epidemiol. (2004) 33:30–42. doi: 10.1093/ije/dyh132
17. Sekula P, Del Greco MF, Pattaro C, Kottgen A. Mendelian randomization as an approach to assess causality using observational data. J Am Soc Nephrol. (2016) 27:3253–65. doi: 10.1681/ASN.2016010098
18. Lawlor DA, Harbord RM, Sterne JA, Timpson N, Davey Smith G. Mendelian randomization: using genes as instruments for making causal inferences in epidemiology. Stat Med. (2008) 27:1133–63. doi: 10.1002/sim.3034
19. Skrivankova VW, Richmond RC, Woolf BAR, Yarmolinsky J, Davies NM, Swanson SA, et al. Strengthening the reporting of observational studies in epidemiology using mendelian randomization: the STROBE-MR statement. JAMA. (2021) 326:1614–21. doi: 10.1001/jama.2021.18236
20. Skrivankova VW, Richmond RC, Woolf BAR, Davies NM, Swanson SA, VanderWeele TJ, et al. Strengthening the reporting of observational studies in epidemiology using mendelian randomisation (STROBE-MR): explanation and elaboration. BMJ. (2021) 375:n2233. doi: 10.1136/bmj.n2233
21. Lambert JC, Ibrahim-Verbaas CA, Harold D, Naj AC, Sims R, Bellenguez C, et al. Meta-analysis of 74,046 individuals identifies 11 new susceptibility loci for Alzheimer’s disease. Nat Genet. (2013) 45:1452–8. doi: 10.1038/ng.2802
22. Schumacher FR, Al Olama AA, Berndt SI, Benlloch S, Ahmed M, Saunders EJ, et al. Association analyses of more than 140,000 men identify 63 new prostate cancer susceptibility loci. Nat Genet. (2018) 50:928–36. doi: 10.1038/s41588-018-0142-8
23. Staiger D, Stock JH. Instrumental variables regression with weak instruments. Econometrica. (1997) 65:557–86. doi: 10.2307/2171753
24. Xiong Y, Zhong X, Zhang F, Wang W, Zhang Y, Wu C, et al. Genetic evidence supporting a causal role of snoring in erectile dysfunction. Front Endocrinol (Lausanne). (2022) 13:896369. doi: 10.3389/fendo.2022.896369
25. Burgess S, Dudbridge F, Thompson SG. Combining information on multiple instrumental variables in Mendelian randomization: comparison of allele score and summarized data methods. Stat Med. (2016) 35:1880–906. doi: 10.1002/sim.6835
26. Bowden J, Davey Smith G, Burgess S. Mendelian randomization with invalid instruments: effect estimation and bias detection through Egger regression. Int J Epidemiol. (2015) 44:512–25. doi: 10.1093/ije/dyv080
27. Bowden J, Davey Smith G, Haycock PC, Burgess S. Consistent estimation in mendelian randomization with some invalid instruments using a weighted median estimator. Genet Epidemiol. (2016) 40:304–14. doi: 10.1002/gepi.21965
28. Zhang K, Li A, Zhou J, Zhang C, Chen M. Genetic association of circulating C-reactive protein levels with idiopathic pulmonary fibrosis: a two-sample Mendelian randomization study. Respir Res. (2023) 24:7. doi: 10.1186/s12931-022-02309-x
29. Hartwig FP, Davey Smith G, Bowden J. Robust inference in summary data Mendelian randomization via the zero modal pleiotropy assumption. Int J Epidemiol. (2017) 46:1985–98. doi: 10.1093/ije/dyx102
30. Burgess S, Bowden J, Fall T, Ingelsson E, Thompson SG. Sensitivity analyses for robust causal inference from mendelian randomization analyses with multiple genetic variants. Epidemiology. (2017) 28:30–42. doi: 10.1097/EDE.0000000000000559
31. Burgess S, Thompson SG. Interpreting findings from Mendelian randomization using the MR-Egger method. Eur J Epidemiol. (2017) 32:377–89. doi: 10.1007/s10654-017-0255-x
32. Verbanck M, Chen CY, Neale B, Do R. Detection of widespread horizontal pleiotropy in causal relationships inferred from Mendelian randomization between complex traits and diseases. Nat Genet. (2018) 50:693–8. doi: 10.1038/s41588-018-0099-7
33. Hemani G, Tilling K, Davey Smith G. Orienting the causal relationship between imprecisely measured traits using GWAS summary data. PloS Genet. (2017) 13:e1007081. doi: 10.1371/journal.pgen.1007081
34. Roe CM, Behrens MI, Xiong C, Miller JP, Morris JC. Alzheimer disease and cancer. Neurology. (2005) 64:895–8. doi: 10.1212/01.WNL.0000152889.94785.51
35. Roe CM, Fitzpatrick AL, Xiong C, Sieh W, Kuller L, Miller JP, et al. Cancer linked to Alzheimer disease but not vascular dementia. Neurology. (2010) 74:106–12. doi: 10.1212/WNL.0b013e3181c91873
36. Musicco M, Adorni F, Di Santo S, Prinelli F, Pettenati C, Caltagirone C, et al. Inverse occurrence of cancer and Alzheimer disease: a population-based incidence study. Neurology. (2013) 81:322–8. doi: 10.1212/WNL.0b013e31829c5ec1
37. Driver JA, Beiser A, Au R, Kreger BE, Splansky GL, Kurth T, et al. Inverse association between cancer and Alzheimer’s disease: results from the Framingham Heart Study. BMJ. (2012) 344:e1442. doi: 10.1136/bmj.e1442
38. Lee JE, Kim D, Lee JH. Association between alzheimer’s disease and cancer risk in South Korea: an 11-year nationwide population-based study. Dement Neurocogn Disord. (2018) 17:137–47. doi: 10.12779/dnd.2018.17.4.137
39. Chung SD, Lin HC, Tsai MC, Kao LT, Huang CY, Chen KC. Androgen deprivation therapy did not increase the risk of Alzheimer’s and Parkinson’s disease in patients with prostate cancer. Andrology. (2016) 4:481–5. doi: 10.1111/andr.12187
40. Frain L, Swanson D, Cho K, Gagnon D, Lu KP, Betensky RA, et al. Association of cancer and Alzheimer’s disease risk in a national cohort of veterans. Alzheimers Dement. (2017) 13:1364–70. doi: 10.1016/j.jalz.2017.04.012
41. Yuan C, Liu S, Yang K, Xie F, Li Y, Guo Y, et al. Causal association between colorectal cancer and Alzheimer’s disease: a bidirectional two-sample mendelian randomization study. Front Genet. (2023) 14:1180905. doi: 10.3389/fgene.2023.1180905
42. Seddighi S, Houck AL, Rowe JB, Pharoah PDP. Evidence of a causal association between cancer and alzheimer’s disease: a mendelian randomization analysis. Sci Rep. (2019) 9:13548. doi: 10.1038/s41598-019-49795-6
43. Ospina-Romero M, Glymour MM, Hayes-Larson E, Mayeda ER, Graff RE, Brenowitz WD, et al. Association between alzheimer disease and cancer with evaluation of study biases: A systematic review and meta-analysis. JAMA Netw Open. (2020) 3:e2025515. doi: 10.1001/jamanetworkopen.2020.25515
44. Li Q, Dong Z, Lin Y, Jia X, Li Q, Jiang H, et al. The rs2233678 polymorphism in PIN1 promoter region reduced cancer risk: a meta-analysis. PloS One. (2013) 8:e68148. doi: 10.1371/journal.pone.0068148
45. Ma SL, Tang NL, Tam CW, Lui VW, Lam LC, Chiu HF, et al. A PIN1 polymorphism that prevents its suppression by AP4 associates with delayed onset of Alzheimer’s disease. Neurobiol Aging. (2012) 33:804–13. doi: 10.1016/j.neurobiolaging.2010.05.018
46. Tremolizzo L, Rodriguez-Menendez V, Brighina L, Ferrarese C. Is the inverse association between Alzheimer’s disease and cancer the result of a different propensity to methylate DNA? Med Hypotheses. (2006) 66:1251–2. doi: 10.1016/j.mehy.2005.12.022
47. Driver JA. Inverse association between cancer and neurodegenerative disease: review of the epidemiologic and biological evidence. Biogerontology. (2014) 15:547–57. doi: 10.1007/s10522-014-9523-2
48. Ganguli M. Cancer and dementia: it’s complicated. Alzheimer Dis Assoc Disord. (2015) 29:177–82. doi: 10.1097/WAD.0000000000000086
49. Bennett DA, Leurgans S. Is there a link between cancer and Alzheimer disease? Neurology. (2010) 74:100–1. doi: 10.1212/WNL.0b013e3181cbb89a
50. Nagy Z. The dysregulation of the cell cycle and the diagnosis of Alzheimer’s disease. Biochim Biophys Acta. (2007) 1772:402–8. doi: 10.1016/j.bbadis.2006.11.001
51. Behrens MI, Lendon C, Roe CM. A common biological mechanism in cancer and Alzheimer’s disease? Curr Alzheimer Res. (2009) 6:196–204. doi: 10.2174/156720509788486608
52. Lu PJ, Wulf G, Zhou XZ, Davies P, Lu KP. The prolyl isomerase Pin1 restores the function of Alzheimer-associated phosphorylated tau protein. Nature. (1999) 399:784–8. doi: 10.1038/21650
53. Liou YC, Sun A, Ryo A, Zhou XZ, Yu ZX, Huang HK, et al. Role of the prolyl isomerase Pin1 in protecting against age-dependent neurodegeneration. Nature. (2003) 424:556–61. doi: 10.1038/nature01832
54. Ryan KM, Vousden KH. Cancer: pinning a change on p53. Nature. (2002) 419:795, 7. doi: 10.1038/419795a
55. Segat L, Pontillo A, Annoni G, Trabattoni D, Vergani C, Clerici M, et al. PIN1 promoter polymorphisms are associated with Alzheimer’s disease. Neurobiol Aging. (2007) 28:69–74. doi: 10.1016/j.neurobiolaging.2005.11.009
56. Driver JA, Lu KP. Pin1: a new genetic link between Alzheimer’s disease, cancer and aging. Curr Aging Sci. (2010) 3:158–65. doi: 10.2174/1874609811003030158
Keywords: Mendelian randomization, Alzheimer’s disease, prostate cancer, GWAS, causal analysis
Citation: Li R, Peng L, Deng D, Li G and Wu S (2024) Causal relationship between Alzheimer’s disease and prostate cancer: a bidirectional Mendelian randomization analysis. Front. Endocrinol. 15:1354528. doi: 10.3389/fendo.2024.1354528
Received: 12 December 2023; Accepted: 16 February 2024;
Published: 13 March 2024.
Edited by:
Guangliang Chen, Fudan University, ChinaReviewed by:
Shigeo Horie, Juntendo University, JapanAhmed Dawoud, University Hospital Southampton NHS Foundation Trust, United Kingdom
Copyright © 2024 Li, Peng, Deng, Li and Wu. This is an open-access article distributed under the terms of the Creative Commons Attribution License (CC BY). The use, distribution or reproduction in other forums is permitted, provided the original author(s) and the copyright owner(s) are credited and that the original publication in this journal is cited, in accordance with accepted academic practice. No use, distribution or reproduction is permitted which does not comply with these terms.
*Correspondence: Song Wu, c29uZ3d1X2x6dUAxMjYuY29t; Guangzhi Li, Z3Vhbmd6aGlsaUBlbWFpbC5zenUuZWR1LmNu
†These authors have contributed equally to this work