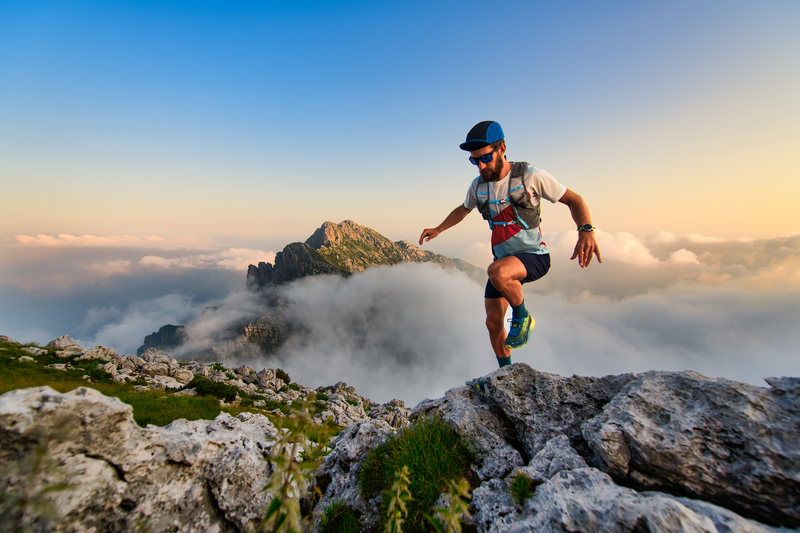
95% of researchers rate our articles as excellent or good
Learn more about the work of our research integrity team to safeguard the quality of each article we publish.
Find out more
ORIGINAL RESEARCH article
Front. Endocrinol. , 17 April 2024
Sec. Clinical Diabetes
Volume 15 - 2024 | https://doi.org/10.3389/fendo.2024.1377923
Background: We explore the effect of suboptimal glycemic control on the incidence of diabetic peripheral neuropathy (DPN) in both non-elderly and elderly patients with type 2 diabetes mellitus (T2DM).
Methods: A 6-year follow-up study (2013-2019) enrolled T2DM patients aged >20 without DPN. Participants were classified into two groups: those below 65 years (non-elderly) and those 65 years or older (elderly). Biochemical measurements, including glycated hemoglobin (HbA1C), were recorded regularly. DPN was diagnosed using the Michigan Neuropathy Screening Instrument examination. The outcome was DPN occurrence in 2019.
Results: In 552 enrollments (69% non-elderly), DPN occurred in 8.4% non-elderly and 24.0% elderly patients. A higher initial HbA1C level was significantly linked with a higher risk of future DPN in the non-elderly group (adjusted odds ratio [AOR] 1.46, 95% CI 1.13–1.89, p=0.004). In comparison, HbA1c at the end of the study period was not associated with DPN in the non-elderly group (AOR 1.17, 95% CI 0.72–1.90, p=0.526). In the elderly group, no statistical relationship was found between HbA1C levels and DPN, either in 2013 or in 2019.
Conclusion: Suboptimal glycemic control at baseline, rather than at the end of the study period, predicts an increased risk of future DPN in individuals with T2DM under age 65. This correlation is not seen in elderly patients. Therefore, we recommend implementing enhanced glycemic control early in middle-aged T2DM patients and propose individualized therapeutic strategies for diabetes in different age groups.
Diabetic peripheral neuropathy (DPN), which is one of the most common complication of diabetes mellitus (DM), is an important issue when it comes to DM care. It affects up to 50% of adults with type 2 DM (T2DM) during their lifetime (1). DPN contributes to numerous disabling morbidities, and DPN-related diabetic foot ulcerations are the most common cause of non-traumatic lower-limb amputations in most high-income countries (2, 3). Besides, DPN can also lead to impaired balance, gait disturbance, and distressing neuropathic pain, which result in decrease in quality of life, and are frequently resistant to conventional treatment (4). Therefore, early diagnosis and prevention the development of DPN is crucial.
In real-world practice, the diagnosis of DPN is typically based on patient medical history, clinical examinations, and validated scoring clinical assessments (such as the Michigan Neuropathy Screening Instrument (MNSI), the Toronto Clinical Neuropathy Score, and the United Kingdom Screening Test) (1, 5, 6). MNSI, which consists of assessing the physical appearance of feet, ulceration, ankle deep tendon reflexes, perception of light touch (using the Semmes-Weinstein 5.07 10-g monofilament), and distal vibration (using a 128-Hz tuning fork) (5), is the most widely used diagnostic tool.
Unfortunately, despite the severe complications and significant impact on patients’ daily lives and socio-economic status, DPN often begins insidiously. In the early stage, DPN typically present no discernible symptoms, making it hard to detect until it is well established, at which point it may become challenging to reverse (1). While DPN occurred, there is only disease-modifying therapy that targets the underlying nerve damage: intensive glycemic control in type 1 DM (T1DM) (7, 8), yet it is ineffective type 2 DM (T2DM) (9). The absence of disease-modifying therapy underscores the indispensability of preventive strategy prior to the onset of DPN in diabetes care, particularly in patients with T2DM. Good glycemic control has been demonstrated to reduce the occurrence rate of DPN in patients with T1DM. Interestingly, the same outcome has not been observed in patients with T2DM (7–9). However, in clinical setting, patients who suffer from complications of DM are mainly diagnosed with T2DM. Given that most patients diagnosed with DM complications predominantly have T2DM, there’s considerable research interest in exploring the link between poor glycemic control and subsequent DPN in T2DM. Despite several recent studies on the topic, definitive conclusions remain elusive.
We hypothesized that the definite effect of each predictor of DPN might be diverse in different age groups, especially glycemic control status. Therefore, we aim to investigate the effect of suboptimal glycemic control on the incidence of DPN in Taiwanese adults with T2DM, focusing on both non-elderly and elderly patients.
The study is a hospital-based 6-year follow-up study from a prospective DM registry at a comprehensive medical center located in central Taiwan. The study began in 2013, and its subjects include individuals over 20 years of age who had previously known or were newly diagnosed with type 2 diabetes. Each of the participants was been diagnosed by endocrinologists in the outpatient department of Taichung Veterans General Hospital in adherence to the criteria of American Diabetes Association (ADA). Relevant clinical data was collected at the time of enrollment and during subsequent follow up period, including patient’s medical records, laboratory test results, questionnaires and anthropometric measurements. Exclusion criteria were (1) patients had DPN at baseline; (2) patients having type 1 DM or gestational diabetes and (3) patients with missing data at baseline or at the end of study period.
Participants were monitored through clinical follow-up examinations and questionnaires. Our study was conducted until 2019, 6 years after its commencement. The primary outcome was the occurrence of DPN in 2019. Patients were classified into two age groups in 2013: (1) the elderly group, consisting of patients aged 65 years and above, and (2) the non-elderly group, comprising patients under 65 years old. We further defined the patients who developed DPN during follow-up period as the “incident DPN” sub-group. The sociodemographic factors and biochemical factors at baseline (2013) and at the end of study period (2019) between the patients with and without incident DPN were compared in each age group. The study mainly concentrated on the glycemic control status.
Patient’s information was anonymized through a computer system, and researchers were blinded to this data. Our study received approval from the Institutional Review Board at Taichung Veterans General Hospital (CG18082B-1). All participants volunteered for the current studies, and provided written informed consent prior to enrolment.
All study participants underwent a DPN assessment at enrollment and a second assessment after six years of follow-up by the same trained and certified nursing staff. DPN was evaluated based on MNSI examination (MNSIE), the second component of MNSI. In line with previous validated studies on adults, individuals whose MNSIE score > 2 were diagnosed with DPN (5).
Laboratory tests were conducted during outpatient follow-ups. Blood samples were collected in the morning after an overnight fasting period from the antecubital vein. Fasting plasma glucose (FPG; using standard enzymatic methods), glycated hemoglobin (HbA1c; using high-performance liquid chromatography), serum creatinine concentration and plasma lipid profiles (using standard enzymatic methods), including total cholesterol (TC), high-density lipoprotein (HDL), low-density lipoprotein (LDL), and triglyceride (TG). The blood test was performed at least once every three months.
We defined glycemic control status at baseline using the mean HbA1c level in 2013 and glycemic control status at the end of the study period with the mean HbA1c in 2019. All parameters were measured at least four times both in 2013 and 2019. After which, their respective averages were calculated.
All participants received anthropometric measurements, including height, weight, waist circumference, and blood pressure by our collaborated case-management nurse in 2013 and 2019, respectively. Smoking status and medication usage were also recorded. Current medication usage including oral hypoglycemic agent (OHA), insulin, antihypertensive drugs and lipid-lowering drugs such as statins and fibrates. The number of insulin used is defined as the number of individuals who use insulin divided by the total number of individuals within each age group, expressed as the median (interquartile range [IQR]). Comorbidities obtained from medical record and based on International Classification of Diseases, 9th revision Clinical Modification (ICD-9-CM) and 10th revision (ICD-10) which including hypertension (ICD-9-CM codes 401-405, ICD-10 codes I10-I15), cerebrovascular disease (ICD-9-CM codes 430-438, ICD-10 codes I60-I69), ischemic heart disease (ICD-9-CM codes 410-414, ICD-10 codes I20-I25), liver disease (ICD-9-CM codes 571-573, ICD-10 codes K70-K77). For the details of anthropometric measurements, please refers to our published study (10, 11).
Continuous data were presented as mean values ± standard deviation (SD) and categorical data as numbers with percentages, while discrete variables were expressed as median and IQR. We used Fisher’s exact test or the Chi-square test to analyze categorical variables. While discrete variable analyses were performed using the Mann-Whitney U test and continuous variable analyses were performed using the independent t-test. p values less than 0.05 were considered significant. The multivariable logistic regression analysis was performed in each age group to investigate the impact of each independently identified variable on the incident DPN. The multivariable regression model includes all confounders and the adjusted odds ratios (AOR) with 95% confidence interval (CI) were calculated between the comparison groups.
We conduct two multivariable regression models for each age group: applied parameters in 2013 and parameters in 2019, respectively. These two models adjusted all the confounders, besides, HbA1C would be included even if descriptive statistics revealed no statistical significant.
Because the relationship between HbA1c and FPG is hyperbolic, we do not adjust one for each other to statistic the interference between baseline glycemic control and the occurrence of DPN.
In 2013, we recruited 681 participants with DM as a baseline. Of those participants, 116 (17.0%) with DPN in their initial state and 13 (1.9%) with non-T2DM were excluded. Accordingly, 552 patients were considered eligible to participate in the study (Figure 1). That cohort includes 381 patients in the non-elderly group and 171 patients in the elderly group. In total cohort, the participants’ median age was 59.7 ± 10.7 years; the mean duration of DM was 15.2 ± 6.9 years, and the participants’ mean HbA1c level was 7.4 ± 1.3%. Seventy-three patients developed DPN during follow-up period, and the cumulative incidence of DPN over the 6 years of follow-up was 13.2%.
We compared the sociodemographic and biochemical factors at baseline for patients with and without incident DPN in both age groups (Table 1). In addition, the baseline sociodemographic and biochemical factors of our cohort were shown in Supplementary Table 1.
Table 1 Characteristics of patients with incident DPN and without incident DPN in non-elderly and elderly groups at baseline (2013).
Among non-elderly group, male gender (84.4% vs. 60.2%, p=0.007), body weight (75.3 ± 12.5 kg vs. 70.1 ± 13.4 kg, p< 0.05), HbA1C (8.6 ± 2.0% vs. 7.4 ± 1.3%, p=0.002), duration of diabetes (16.8 ± 7.7 years vs. 13.7 ± 6.1 years, p=0.009), number of insulin used (0.8 vs. 0.6, p=0.008) and serum creatinine (1.1 ± 0.4 mg/dL vs. 0.9 ± 0.3 mg/dL, p= 0.02) were significantly higher at baseline in patients with incident DPN than in those without incident DPN.
Among elderly group, male gender (78.1% vs. 46.9%, p<0.001), height (164.6 ± 7.8 cm vs. 159.9 ± 7.6 cm, p= 0.001) and body weight (69.9 ± 9.7 kg vs. 63.4 ± 11.3 kg, p= 0.001) were significantly higher at baseline in patients with incident DPN than in those without incident DPN. LDL (84.6 ± 26.4 mg/dL vs. 96.1 ± 23.7 mg/dL, p=0.014) and TC (84.6 ± 26.4 mg/dL vs. 163.3 ± 27.8 mg/dL, p=0.018) were significantly lower at baseline in patients with incident DPN than in those without incident DPN.
Table 2 summarizes the sociodemographic and biochemical factors of the patients with and without incident DPN in both age groups in 2019.
Table 2 Characteristics of patients with incident DPN and without incident DPN in non-elderly and elderly groups at the end of study period (2019).
As of 2019, among non-elderly group, male gender (84.4% vs. 60.2%, p=0.007), body weight (75.9 ± 12.7 kg vs. 69.3 ± 14.1 kg, p=0.011), waist circumference (93.9 ± 9.5 cm vs. 89.7 ± 11.0 cm, p=0.038), HbA1C (7.8 ± 0.8% vs. 7.4 ± 0.9%, p=0.016), duration of diabetes (22.8 ± 7.7 years vs. 19.7 ± 6.1 years, p=0.009), number of insulin used (0.8 vs. 0.6, p=0.008) and serum creatinine (1.5 ± 1.2 mg/dL vs. 1.0 ± 0.8 mg/dL, p= 0.041) were significantly higher in patients with incident DPN than in those without incident DPN.
Among elderly group, male gender (78.1% vs. 46.9%, p<0.001), height (162.8 ± 8.7 cm vs. 158.2 ± 8.0 cm, p=0.003), body weight (69.5 ± 10.1 kg vs. 61.4 ± 11.4 kg, p<0.001) and waist circumference (94.6 ± 9.4 cm vs. 87.5 ± 9.6 cm, p<0.001) were significantly higher in patients with incident DPN.
In Tables 3, 4, we present results from our multivariable logistic regression model which respectively applied the confounders and HbA1C levels from baseline and 2019; the data are presented as AOR for the risk factors of incident DPN.
In the non-elderly group, increased HbA1C level at baseline (AOR 1.46, 95% CI 1.13–1.89, p=0.004) was significantly associated with an increased risk of DPN. The duration of DM (AOR 1.07, 95% CI 1.00–1.14, p=0.041) and serum creatinine level (AOR 4.41, 95% CI 1.05–18.51, p=0.042) were significantly associated with a higher risk of DPN as well. On the other hand, male gender, body weight, mean number of insulin used at baseline and all parameters in 2019 revealed no statistically significant associations with the risk of DPN after adjustment for all confounding factors (Tables 3-1, 4-1).
In the elderly group, lower LDL at baseline (AOR 0.97, 95% CI 0.95–0.99, p=0.025) was significantly associated with an increased risk of DPN. On the other hand, HbA1C level, male gender, height, weight, TC at baseline and all parameters in 2019 revealed no statistically significant associations with the risk of DPN (Tables 3-2, 4-2).
To the best of our knowledge, our study is the first large-scale follow-up study to investigate risk factors for DPN across different age groups. The main findings of our study can be summarized as follows: (1) suboptimal glycemic control status at baseline is a predictor of future DPN in Taiwanese individuals with T2DM under the age of 65 but not in elderly populations, (2) glycemic control status at the end of study period did not significantly correlate with DPN risk in either age group, (3) the predictors of future DPN may differ among varying age populations.
In our study, the cumulative incidence of DPN was 8.4% and 24.0% in the non-elderly and elderly groups, respectively. Those data were based on 6 years of follow-up using the MNSIE for DPN diagnosis. The incidence of DPN in the present study is comparable to previous large-scale studies conducted in Han Chinese populations (n = 37,375, annual incidence of 3.2%) (12) but lower than that reported in Western populations (13, 14). The discrepancy might be attributed to the ethnic makeup of our study group and their relatively better control of blood sugar levels.
It is a topic of ongoing research whether intensive glycemic control can prevent patients with T2DM from developing DPN. Previous randomized controlled trials have demonstrated intensive glycemic control can substantially reduce the incidence of DPN in T1DM populations (7, 8). Furthermore, long-term poor glycemic control in T1DM patients is known to be a determining factor in rapid DPN development (15). In contrast, intensive glycemic control has revealed limited effectiveness in preventing DPN in T2DM patients (9). This observation remains true, regardless of whether the DPN was diagnosed using the MNSI (16) or nerve conduction study (17). Correspondingly, previous large-scale studies support the concept that baseline HbA1c levels are not an independent risk factor for DPN across all age groups (10, 18).
However, in clinical practice, T2DM is the most prevalent form of DM, and more than 90% of DPN cases can be attributed to T2DM (19). Therefore, it garners significant attention that whether suboptimal glycemic control is a predictor of future DPN in individuals with T2DM. Our study confirms this correlation in patients under the age of 65.
To date, the pathogenesis of neurotoxicity in patients with diabetes who have poor glycemic control is well-established. Hyperglycemia, by disrupting various metabolic pathways, gives rise to irregularities within nerve-related processes that specifically involve the polyol, hexosamine, and protein kinase C pathways (20). These irregularities serve as triggers for the release of proinflammatory cytokines, the accumulation of advanced glycation end products, and the generation of reactive oxygen species. Meanwhile, microangiopathic alterations in the vasa nervorum can lead to the condition of neuroischemia (21). This sequence of events results in oxidative stress in the nervous system and neuron apoptosis, which contribute to the development of DPN.
We posit that the pathogenesis of neurotoxicity generated by poor glycemic control should not differ depending on whether a patient has T1DM or T2DM. Therefore, we propose the hypothesis that suboptimal glycemic control contributing to DPN may vary depending on the individual with diabetes. Concurrently, our previous research findings have indicated that DPN in elderly patients may be attributed to multitude of factors, including advanced age itself and the relatively long duration of DM (10). In present study, we accordingly stratified our subjects at the age of 65 to assess the impact of glycemic control on the incidence of DPN among non-elderly and elderly patients.
Furthermore, our finding might be affected by the known link between age and HbA1c levels. One potential explanation is that older individuals physiologically have lower red blood cell counts, rendering HbA1c an inadequate indicator for evaluating glycemic control in a geriatric population (22, 23). When it comes to the glycemic control status at the end of the study period, there was no significant association between the 2019 HbA1c levels and DPN risk in either the non-elderly or elderly groups. This finding seems to imply that, as diabetes progresses over time, enhanced glycemic control becomes insufficient in preventing DPN. Our findings underscore the importance of early prompt intervention. Implementing enhanced glycemic control as a preventive strategy against DPN should commence as early as possible, particularly in non-elderly individuals.
Upon adjusting for potential confounding factors, our data revealed elevated risk of DPN was linked to the duration of DM and serum creatinine levels at baseline in the non-elderly group. In the elderly group, a lower baseline LDL was linked to an increased DPN risk. Our findings are consistent with previous literature and our published study (11, 18, 24, 25).
Regarding dyslipidemia, we also found that lower LDL at baseline was associated with a risk of DPN in the elderly group. Previous clinical studies have demonstrated inconsistent results in terms of a correlation between DPN and serum lipid profiles (26, 27). Nevertheless, the association between dyslipidemia and neurotoxicity is predominantly documented in preclinical research (28, 29). One possible explanation for the association between lower LDL levels and increased risk of incident DPN in the elderly group may stem from the fact that, in older adults, lower LDL levels might reflect underlying frailty, chronic illness, chronic inflammation, or malnutrition, thereby increasing their vulnerability to neuropathic complications (30, 31). Our study primarily investigates that whether glycemic control status is associated with incident DPN among different age groups. Further research on dyslipidemia is warranted to elucidate the underlying mechanisms and determine the clinical implications for DPN preventive strategies.
In addition, HbA1c and serum creatinine levels are crucial in clinical practice because of the “modifiable” characteristic and they are “commonly” available assessment. These properties make them suitable targets for therapeutic intervention. Therefore, we recommend early management of poor glycemic control and impaired renal function should be integral to diabetes-treatment strategies in middle-aged patients to prevent DPN. In addition, HbA1c and serum creatinine levels can serve as effective monitoring indicators.
The strengths of our study include the innovative age-stratified design, the large-scaled and unselected nature of the participants, the long-term follow-up period, and the inclusion of several potential risk factors at baseline. Nevertheless, our study has several limitations. First, we did not adhere to an interval-based examination protocol to assess the occurrence of DPN. We accordingly could not perform time-to-event analysis. Second, the inclusion of participants from a single hospital potentially restricted the generalizability of our findings. Third, the number of participants might be insufficient for powered subgroup analyses (for example, only 171 patients in elderly group). Fourth, we abstained from employing confirmatory diagnostic measures such as nerve conduction studies for detecting DPN. It is noteworthy, however, per ADA guidelines, DPN diagnosis usually relies on clinical evaluation – specifically, the highly sensitive and specific MNSI clinical screening tool. Lastly, our study did not include factors like alcohol consumption, heavy metal exposure, or non-hypoglycemic drugs, which are known confounding factors for neuropathy, thus representing a limitation. Despite these issues, similar prevalence rates of comorbidities between subgroups with and without incident DPN across both age groups may suggest no significant differences in routine medication usage among participants.
Suboptimal glycemic control at baseline is a predictor of future DPN in adult patients with T2DM who are less than 65 years old but not in elderly populations. Other risk factors include the duration of DM and serum creatinine levels at baseline in patients under the age of 65, as well as low LDL levels at baseline in elderly patients. Intensive glycemic control should be provided to middle-aged patients as soon as possible to prevent the occurrence of future DPN and HbA1c levels can serve as an appropriate and effective monitoring indicators. Furthermore, we recommend tailoring treatment strategies to the individual patient’s age group. Our findings provide valuable insights for researchers studying the pathogenesis of DPN, and we are hopeful they will encourage the development of disease-modifying treatments in the future.
The original contributions presented in the study are included in the article/Supplementary Material. Further inquiries can be directed to the corresponding authors.
C-SW: Conceptualization, Data curation, Formal Analysis, Methodology, Project administration, Resources, Writing – original draft, Writing – review & editing. Y-WP: Conceptualization, Investigation, Methodology, Project administration, Resources, Validation, Writing – review & editing. C-HL: Data curation, Formal Analysis, Resources, Writing – original draft, Visualization. I-TL: Data curation, Resources, Writing – review & editing. H-HC: Formal Analysis, Software, Writing – original draft. M-HC: Conceptualization, Funding acquisition, Investigation, Methodology, Project administration, Resources, Supervision, Validation, Visualization, Writing – review & editing.
The author(s) declare that financial support was received for the research, authorship, and/or publication of this article. This work was supported by the grant from the Taichung Veterans General Hospital (TCVGH 1093403C and 1103402C). The funding source had no role in the study’s design, conduct, and reporting.
The authors would like to thank all colleagues and students who contributed to our previous study. In addition, I am grateful to Professor Chang M. H. for getting me the opportunity to write this manuscript.
The authors declare that the research was conducted in the absence of any commercial or financial relationships that could be construed as a potential conflict of interest.
All claims expressed in this article are solely those of the authors and do not necessarily represent those of their affiliated organizations, or those of the publisher, the editors and the reviewers. Any product that may be evaluated in this article, or claim that may be made by its manufacturer, is not guaranteed or endorsed by the publisher.
The Supplementary Material for this article can be found online at: https://www.frontiersin.org/articles/10.3389/fendo.2024.1377923/full#supplementary-material
DPN, diabetic peripheral neuropathy; DM, diabetes mellitus; T2DM, type 2 DM; MNSI, Michigan Neuropathy Screening Instrument; MNSIE, MNSI examination; T1DM, type 1 DM; ADA, American Diabetes Association; FPG, fasting plasma glucose; HbA1c, glycated hemoglobin; TC, total cholesterol; HDL, high-density lipoprotein; LDL, low-density lipoprotein; TG, triglyceride; OHA, oral hypoglycemic agent; SD, standard deviation; AOR, adjusted odds ratio; CI, confidence interval
1. Selvarajah D, Kar D, Khunti K, Davies MJ, Scott AR, Walker J, et al. Diabetic peripheral neuropathy: advances in diagnosis and strategies for screening and early intervention. Lancet Diabetes Endocrinol. (2019) 7:938–48. doi: 10.1016/S2213-8587(19)30081-6
2. Margolis DJ, Malay DS, Hoffstad OJ, Leonard CE, MaCurdy T, de Nava KL, et al. Incidence of diabetic foot ulcer and lower extremity amputation among Medicare beneficiaries, 2006 to 2008. In: Data Points Publication Series. Agency for Healthcare Research and Quality (US, Rockville (MD (2011).
3. Gordois A, Scuffham P, Shearer A, Oglesby A, Tobian JA. The health care costs of diabetic peripheral neuropathy in the US. Diabetes Care. (2003) 26:1790–5. doi: 10.2337/diacare.26.6.1790
4. Callaghan BC, Cheng HT, Stables CL, Smith AL, Feldman EL. Diabetic neuropathy: clinical manifestations and current treatments. Lancet Neurol. (2012) 11:521–34. doi: 10.1016/S1474-4422(12)70065-0
5. Herman WH, Pop-Busui R, Braffett BH, Martin CL, Cleary PA, Albers JW, et al. Use of the Michigan Neuropathy Screening Instrument as a measure of distal symmetrical peripheral neuropathy in Type 1 diabetes: results from the Diabetes Control and Complications Trial/Epidemiology of Diabetes Interventions and Complications. Diabetes Med. (2012) 29:937–44. doi: 10.1111/j.1464-5491.2012.03644.x
6. Young MJ, Boulton AJ, MacLeod AF, Williams DR, Sonksen PH. A multicentre study of the prevalence of diabetic peripheral neuropathy in the United Kingdom hospital clinic population. Diabetologia. (1993) 36:150–4. doi: 10.1007/BF00400697
7. Linn T, Ortac K, Laube H, Federlin K. Intensive therapy in adult insulin-dependent diabetes mellitus is associated with improved insulin sensitivity and reserve: a randomized, controlled, prospective study over 5 years in newly diagnosed patients. Metabolism. (1996) 45:1508–13. doi: 10.1016/s0026-0495(96)90180-8
8. Nathan DM, Genuth S, Lachin J, Cleary P, Crofford O, Davise M, et al. The effect of intensive treatment of diabetes on the development and progression of long-term complications in insulin-dependent diabetes mellitus. N Engl J Med. (1993) 329:977–86. doi: 10.1056/NEJM199309303291401
9. Callaghan BC, Little AA, Feldman EL, Hughes RA. Enhanced glucose control for preventing and treating diabetic neuropathy. Cochrane Database Syst Rev. (2012) 6:Cd007543. doi: 10.1002/14651858.CD007543.pub2
10. Wang C-S, Pai Y-W, Lin C-H, Lee IT, Chang M-H. Renal impairment is one of appropriate predictors of future diabetic peripheral neuropathy: a hospital-based 6-year follow-up study. Sci Rep. (2022) 12:5240. doi: 10.1038/s41598-022-09333-3
11. Pai YW, Lin CH, Lee IT, Chang MH. Prevalence and biochemical risk factors of diabetic peripheral neuropathy with or without neuropathic pain in Taiwanese adults with type 2 diabetes mellitus. Diabetes Metab Syndr. (2018) 12:111–6. doi: 10.1016/j.dsx.2017.09.013
12. Yang CP, Lin CC, Li CI, Liu CS, Lin WY, Hwang KL, et al. Cardiovascular risk factors increase the risks of diabetic peripheral neuropathy in patients with type 2 diabetes mellitus: the Taiwan diabetes study. Med (Baltimore). (2015) 94:e1783. doi: 10.1097/MD.0000000000001783
13. Gaede P, Vedel P, Larsen N, Jensen GV, Parving HH, Pedersen O. Multifactorial intervention and cardiovascular disease in patients with type 2 diabetes. N Engl J Med. (2003) 348:383–93. doi: 10.1056/NEJMoa021778
14. Feldman EL, Callaghan BC, Pop-Busui R, Zochodne DW, Wright DE, Bennett DL, et al. Diabetic neuropathy. Nat Rev Dis Primers. (2019) 5(1):41. doi: 10.1038/s41572-019-0092-1
15. Hajas G, Kissova V, Tirpakova A. A 10-yr follow-up study for the detection of peripheral neuropathy in young patients with type 1 diabetes. Pediatr Diabetes. (2016) 17:632–41. doi: 10.1111/pedi.12382
16. Ismail-Beigi F, Craven T, Banerji MA, Basile J, Calles J, Cohen RM, et al. Effect of intensive treatment of hyperglycaemia on microvascular outcomes in type 2 diabetes: an analysis of the ACCORD randomised trial. Lancet. (2010) 376:419–30. doi: 10.1016/S0140-6736(10)60576-4
17. UK Prospective Diabetes Study (UKPDS) Group. Intensive blood-glucose control with sulphonylureas or insulin compared with conventional treatment and risk of complications in patients with type 2 diabetes (UKPDS 33). UK Prospective Diabetes Study (UKPDS) Group. Lancet. (1998) 352:837–53. doi: 10.1016/S0140-6736(98)07019-6
18. Andersen ST, Witte DR, Dalsgaard EM, Andersen H, Nawroth P, Fleming T, et al. Risk factors for incident diabetic polyneuropathy in a cohort with screen-detected type 2 diabetes followed for 13 years: ADDITION-Denmark. Diabetes Care. (2018) 41:1068–75. doi: 10.2337/dc17-2062
19. Abbott CA, Malik RA, van Ross ER, Kulkarni J, Boulton AJ. Prevalence and characteristics of painful diabetic neuropathy in a large community-based diabetic population in the U.K. Diabetes Care. (2011) 34:2220–4. doi: 10.2337/dc11-1108
20. Vincent AM, Callaghan BC, Smith AL, Feldman EL. Diabetic neuropathy: cellular mechanisms as therapeutic targets. Nat Rev Neurol. (2011) 7:573–83. doi: 10.1038/nrneurol.2011.137
21. Malik RA, Newrick PG, Sharma AK, Jennings A, Ah-See AK, Mayhew TM, et al. Microangiopathy in human diabetic neuropathy: relationship between capillary abnormalities and the severity of neuropathy. Diabetologia. (1989) 32:92–102. doi: 10.1007/BF00505180
22. Wu L, Lin H, Gao J, Li X, Xia M, Wang D, et al. Effect of age on the diagnostic efficiency of HbA1c for diabetes in a Chinese middle-aged and elderly population: The Shanghai Changfeng Study. PloS One. (2017) 12:e0184607. doi: 10.1371/journal.pone.0184607
23. Masuch A, Friedrich N, Roth J, Nauck M, Müller UA, Petersmann A. Preventing misdiagnosis of diabetes in the elderly: age-dependent HbA1c reference intervals derived from two population-based study cohorts. BMC Endocr Disord. (2019) 19:20. doi: 10.1186/s12902-019-0338-7
24. Papanas N, Ziegler D. Risk factors and comorbidities in diabetic neuropathy: an update 2015. Rev Diabetes Stud. (2015) 12:48–62. doi: 10.1900/RDS.2015.12.48
25. Won JC, Kim SS, Ko KS, Cha BY. Current status of diabetic peripheral neuropathy in Korea: report of a hospital-based study of type 2 diabetic patients in Korea by the diabetic neuropathy study group of the korean diabetes association. Diabetes Metab J. (2014) 38:25–31. doi: 10.4093/dmj.2014.38.1.25
26. Jende JME, Groener JB, Kender Z, Rother C, Hahn A, Hilgenfeld T, et al. Structural nerve remodeling at 3-T MR neurography differs between painful and painless diabetic polyneuropathy in type 1 or 2 diabetes. Radiology. (2020) 294:405–14. doi: 10.1148/radiol.2019191347
27. Cai Z, Yang Y, Zhang J. A systematic review and meta-analysis of the serum lipid profile in prediction of diabetic neuropathy. Sci Rep. (2021) 11:499. doi: 10.1038/s41598-020-79276-0
28. Vincent AM, Hayes JM, McLean LL, Vivekanandan-Giri A, Pennathur S, Feldman EL. Dyslipidemia-induced neuropathy in mice: the role of oxLDL/LOX-1. Diabetes. (2009) 58:2376–85. doi: 10.2337/db09-0047
29. Xie F, Fu H, Hou JF, Jiao K, Costigan M, Chen J. High energy diets-induced metabolic and prediabetic painful polyneuropathy in rats. PloS One. (2013) 8:e57427. doi: 10.1371/journal.pone.0057427
30. Liu Y, Coresh J, Eustace JA, Longenecker JC, Jaar B, Fink NE, et al. Association between cholesterol level and mortality in dialysis patients: role of inflammation and malnutrition. Jama. (2004) 291:451–9. doi: 10.1001/jama.291.4.451
Keywords: age, diabetic peripheral neuropathy, glycemic control, type 2 diabetes mellitus, risk factor
Citation: Wang C-S, Pai Y-W, Lin C-H, Lee I-T, Chen H-H and Chang M-H (2024) Diabetic peripheral neuropathy: age-stratified glycemic control. Front. Endocrinol. 15:1377923. doi: 10.3389/fendo.2024.1377923
Received: 28 January 2024; Accepted: 05 April 2024;
Published: 17 April 2024.
Edited by:
Chunjie Jiang, University of Texas MD Anderson Cancer Center, United StatesReviewed by:
Abilash Nair, Government Medical College, Thiruvananthapuram, IndiaCopyright © 2024 Wang, Pai, Lin, Lee, Chen and Chang. This is an open-access article distributed under the terms of the Creative Commons Attribution License (CC BY). The use, distribution or reproduction in other forums is permitted, provided the original author(s) and the copyright owner(s) are credited and that the original publication in this journal is cited, in accordance with accepted academic practice. No use, distribution or reproduction is permitted which does not comply with these terms.
*Correspondence: Ming-Hong Chang, Y21oNTAwODA5QGdtYWlsLmNvbQ==; Yen-Wei Pai, amFzbWluZXp4Y3ZAZ21haWwuY29t
†These authors contributed equally to this work and shared last and correspondence authorship
Disclaimer: All claims expressed in this article are solely those of the authors and do not necessarily represent those of their affiliated organizations, or those of the publisher, the editors and the reviewers. Any product that may be evaluated in this article or claim that may be made by its manufacturer is not guaranteed or endorsed by the publisher.
Research integrity at Frontiers
Learn more about the work of our research integrity team to safeguard the quality of each article we publish.