- Hangzhou TCM Hospital Affiliated to Zhejiang Chinese Medical University, Hangzhou, China
Background: Klotho is an anti-aging protein, and obesity and inflammation have been identified as having a relationship with Klotho. This study investigated the correlation between weight-adjusted-waist index(WWI), systemic inflammation index(SII) and soluble Klotho (sKlotho) and explored the potential mediating role of SII.
Methods: The association between WWI, SII, and sKlotho was investigated using weighted multivariate linear regression, subgroup analysis, and smoothed curve fitting in this cross-sectional study, which was based on NHANES data. The Bootstrap method was used to test for mediation effects. Covariate selection was validated using the variance inflation factor (VIF).
Results: The study involved a total of 9,506 participants. The results showed that WWI (β=-0.03; 95%CI:-0.04,-0.01; p<0.0001) was associated with SII (β=-0.00, 95%CI:-0.00,-0.00:p<0.001) and Ln-sKlotho.There was a negative correlation between both WWI and SII and sKlotho. Subgroup analysis showed that gender, age, education, marital status, family income, HEI, PA, hypertension, diabetes, CKD, alcohol use, smoking, and SII did not affect the negative correlation between WWI and sKlotho (FDR-corrected p>0.1). The mediation analysis showed that SII played a significant mediating effect in the role of WWI on sKlotho, with SII mediating 6.78% of the relationship between WWI and sKlotho.
Conclusion: There is a negative correlation between WWI and sKlotho, and SII may be an important mediator between WWI and sKlotho. Reducing inflammatory conditions in obese populations may increase sKlotho levels, which in turn may delay organismal aging in middle-aged and elderly people.
1 Introduction
With the increasing trend of population aging, the growing number of elderly people and the increasing number of age-related diseases, it is expected that the healthcare burden of age-related diseases will similarly increase (1). The Klotho gene was first noted in 1997 by Kuro-o et al. Defective expression of the Klotho gene in mice leads to syndromes similar to those seen in human aging, including a short lifespan, infertility, Atherosclerosis, skin atrophy, osteoporosis, and emphysema (2). Xu Y et al. showed that the Klotho gene is responsible for encoding a transmembrane protein (α-Klotho) (3). The extracellular structural domain of α-Klotho can be shed to form a soluble variant of the gene called soluble Klotho (sKlotho), which is widely found in urine, blood, and cerebrospinal fluid (CSF) (3–7). sKlotho possesses inflammation-regulating, antioxidant, and senescence-preventive functions, which make it a promising target for therapeutic development in aging-related diseases (8–11). Therefore, the study of the factors affecting the level of sKlotho will be of great significance in delaying aging and preventing aging-related diseases.
A growing number of studies have shown a relationship between obesity and aging. Daniela Frasca investigated the region of overlap between obesity and aging, suggesting that obesity may be a biomarker of accelerated aging in human B-cell function and antibody response (12). Valentina Salvestrini in her study suggested that obesity may accelerate the rate of aging affecting all aspects of physiology and thus shorten life expectancy and healthy lifespan (13). Santos AL et al. in their study illustrated the description of obesity as similar to the metabolic dysregulation observed in normal aging, suggesting that obesity may accelerate the aging process (14). The National Health and Nutrition Examination Survey (NHANES) database shows that weight gain over 10 years period promotes aging in adulthood (15). Charalampos Lampropoulos et al. showed that obesity is an inflammatory state, characterized with dysregulation of gastrointestinal hormones, such as GLP-1, ghrelin and PYY, leading also to impaired glucose homeostasis (16), all of which negatively impacts life expectancy. Abdominal obesity, which reflects the amount of visceral fat, is one of the important phenotypes of unhealthy metabolism (17–19). Previously, BMI and WC were commonly used as measures of obesity, but in recent years, studies have pointed out that BMI is unable to differentiate between lean body mass and fat mass, and WC does not take into account differences in height and is therefore insufficient in predicting obesity (20–23). Therefore, in 2018 researchers introduced a new obesity index called the weight-adjusted-waist index (WWI), which takes body weight into account and reflects weight-independent central obesity (24). A growing number of studies have shown that WWI has a higher accuracy in predicting metabolic diseases compared to other obesity indices, and Xiaowan Li et al. found that WWI was the best obesity index for predicting CKD and albuminuria when compared to other obesity indices (BMI, WHTR, WC, height, and body weight (25). To the best of our knowledge, no studies of WWI and sKlotho levels have been formally published in the literature.
Inflammation is an influential factor in sKlotho levels. Typiak MA-O et al. showed that lower sKlotho levels are associated with an increased risk of chronic inflammation (26). In addition, Martín-González CA-O et al. illustrated that higher Klotho levels were associated with improved immune system function and reduced oxidative stress, both of which play a key role in the development of inflammation (27). It is well known that there is a complex association between inflammation and obesity. On the one hand, obesity triggers a state of chronic low-grade inflammation, and the abnormal accumulation of macrophages (known as adipose tissue-associated macrophages or ATMs) in adipose depots and other immune cells is an important factor in obesity-induced inflammation (28, 29) On the other hand, chronic low-grade inflammation affects metabolism and may lead to an increase in food intake, thus exacerbating obesity (30, 31). The Systemic Immune Inflammation Index (SII) is considered a new and reliable index that can be used to comprehensively measure systemic immune and inflammation levels in subjects (32–34). Given the relationship between WWI, SII, and sKlotho, SII may play a role in the association between WWI and sKlotho.
Therefore, this study investigated the relationship between WWI, SII, and sKlotho in people over 45 years of age in the United States. To our knowledge, this is the first study to assess the relationship between WWI, SII, and sKlotho. In addition, this study hypothesized that SII plays a mediating role in the relationship between WWI and sKlotho. Based on exploring the relationship between WWI, SII and sKlotho, the role of SII in the relationship between WWI and sKlotho was analyzed.
2 Materials and methods
2.1 Survey description
The National Center for Health Statistics (NCHS) performed a countrywide research (NHANES) to evaluate the state of nutrition and health in the United States, which served as the basis for the cross-sectional data. Data on demographics, diet, examinations, lab work, and questionnaires were submitted by the participants. All full NHANES research designs and data are available to the public at www.cdc.gov/nchs/NHANEs/. The cross-sectional study complied with the Strengthening the Reporting of Observational Studies in Epidemiology (STROBE) reporting requirements.
2.2 Study population
After a comprehensive search and screening of the NHANES database, participants from 2007 through 2016 were included in this study (sKlotho data were not available for other years). Since the focus of this paper is to examine the relationship between WWI, SII, and sKlotho in a population of middle-aged and elderly Americans, exclusion criteria for this study included (a) participants with incomplete information on sKlotho(n = 36,824), (b) participants with incomplete information on WWI (n = 591) or SII(n = 62), (c) participants aged <45 years (n = 1,875), (d) participants with missing data on body mass index (BMI), education, marital status, family income, smoking, alcohol use, and Healthy Eating Index (HEI) (n= 1,730). A total of 9,506 individuals qualified for analysis, as shown in Figure 1.
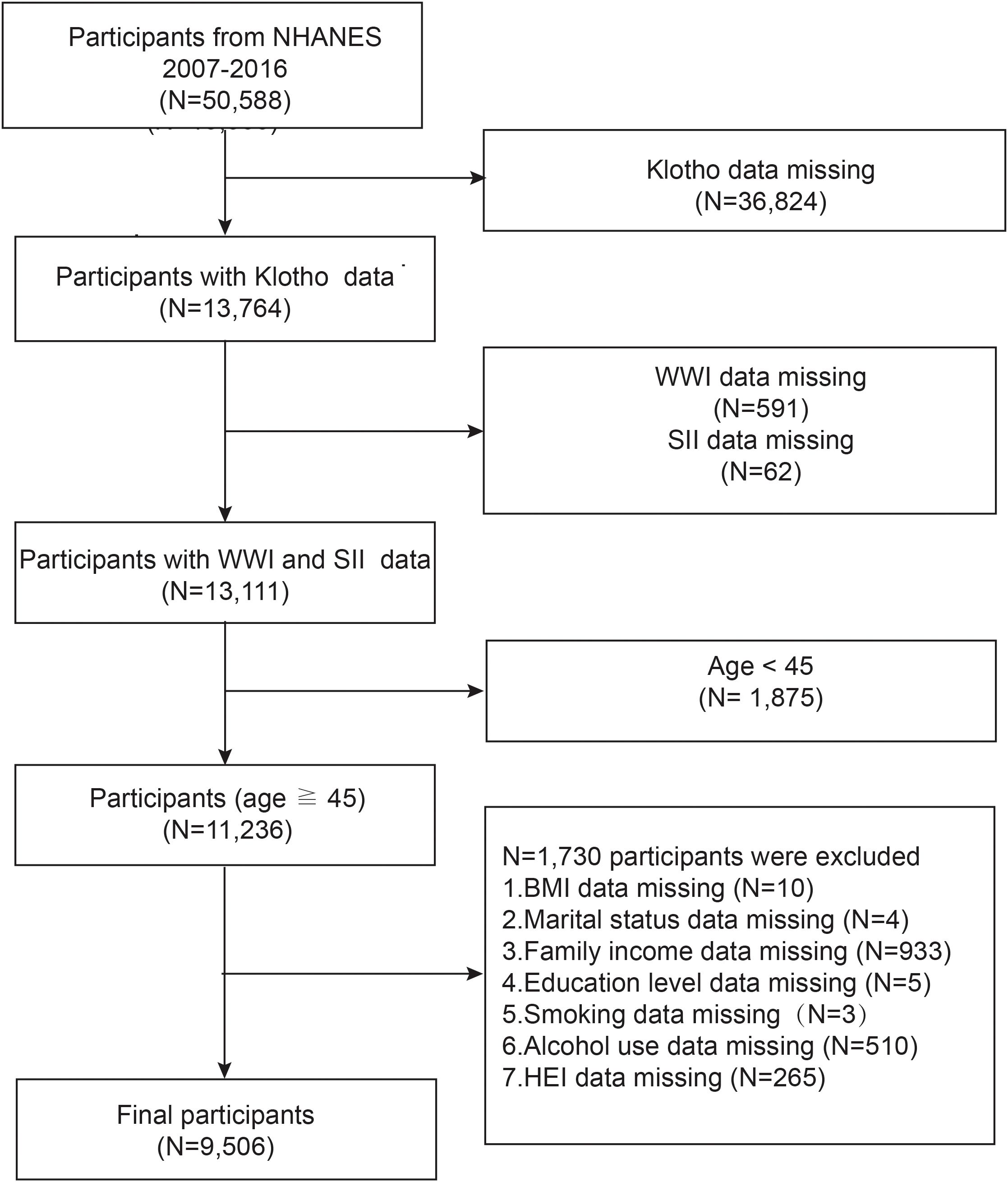
Figure 1. Flowchart of study population inclusion in 2007–2016 NHANES. NHANES, National Health and Nutrition Examination Survey; WWl, weight-adjusted-waist index; SII, systemic immune-inflammation index.
2.3 Measurement of sKlotho levels
Blood samples were collected from participants aged 40 to 79 years, transferred, and stored at -80°C during five cycles of the NHANES program. Serum Klotho concentrations were measured using commercially available ELISA kits from a CDC-accredited laboratory (IBL International, Japan) (35). All serum samples were analyzed twice for Klotho concentration, and the average of these two measured concentrations was used as the final measurement. Sample information, experimental measurement details, and quality control for Klotho concentration measurements can be found on the official NHANES Website.
2.4 Assessment of systemic immune-inflammation index
SII is an automated hematology analysis tool (CoulterDxH 800 analyzer) that is used to measure platelet count, neutrophil count, and lymphocyte count as a composite index. It is utilized as an exposure variable, and computed by multiplying by the neutrophil count after dividing the lymphocyte count by the platelet counts (36–39).
2.5 Assessment of covariates of interest
Demographic covariates in our study included gender, age, race, education level, marital status, and family income. Body measure covariates included BMI. Dietary covariates included energy and the Healthy Eating Index-2015(HEI-2015), HEI-2015 scores range from 0-100, with higher HEI scores reflecting better diet quality. We utilized Day 1 Total Nutrient Intake (DR1TOT) to calculate the 13 components of HEI-2015 and HEI-2015 scores of less than 50, between 50 and 70, and greater than 70 were categorized as insufficient, average, and optimal (40). Associated diseases included chronic kidney disease (CKD), diabetes, and hypertension. Participants with an eGFR ≤ 60 ml/min/1.73m2 or a urinary albumin/creatinine ratio ≥ 30 mg/g were defined as having CKD, where the eGFR was calculated by using the formula developed by the Chronic Kidney Disease-Epidemiology Collaboration (CKD-EPI) (41). Diabetes includes self-reported diabetes, use of antidiabetic medications or insulin, glycosylated hemoglobin (HbA1c) level ≥ 6.5%, and fasting blood glucose level ≥ 126 mg/dL. The 2017 American Heart Association/American College of Cardiology (AHA/ACC) guidelines recommend that individuals with SBP ≥130 mmHg and/or DBP ≥80 mmHg be defined as hypertensive (42). Participants were considered to have high blood pressure if they answered yes to any of the following questions: “Have you ever been told by a doctor or other health professional that you have hypertension, also called high blood pressure?”; “are you now taking prescribed medicine for high blood pressure?”; or if they had a high biological measurement value (systolic blood pressure ≥ 130 mm Hg and/or diastolic blood pressure ≥ 80 mm Hg). Questionnaire variables also included alcohol use, smoking, and physical activity (PA). NHANES participants self-reported their physical activity (PA) information using the Global Physical Activity Questionnaire (GPAQ), a validated PA monitoring tool (43). Participants who did not have any PA and were <600 MET per week were categorized as inactive (44), those participants with ≥600 METs per week were categorized as active. Individuals can access all comprehensive NHANES research designs and data at www.cdc.gov/nchs/NHANEs/.
2.6 Assessment of weight-adjusted waist circumference index
A sample of NHANES participants’ WWI was obtained from the examination data. The participant’s waist circumference was divided by their weight’s square root to calculate their WWI (45). Following computation using this formula, the study population’s WWI range was 8.59–14.79, with kilograms as the weight unit and centimeters as the waist circumference measurement unit.WWI was considered a continuous variable and was designed as an exposure variable in our study.
2.7 Statistical analysis
In descriptive analysis, the compound weighting method is the basis of data description and statistical analysis. Categorical characteristics are expressed as percentages, while continuous variables are expressed as means and standard errors (SE). We constructed three models for weighted multivariate linear regression analysis for different confounders to assess the relationship between sKlotho, WWI and SII in various models. Of these, Model 1: did not adjust for any confounders; Model 2: adjusted for age, gender, and ethnicity; and Model 3: addressed the issue of multicollinearity among the variables in the study and covariate selection, a variance inflation factor (VIF) was used for variable selection. A general rule of thumb is that a VIF value >5 indicates problematic covariance (46). The VIF values for gender, age, race, education level, marital status, family income, energy, BMI, alcohol use, smoking, PA, diabetes, HEI, CKD, and hypertension were all calculated to be less than 5, and therefore these variables were included in Model 3 for adjustment. In addition, we used directed acyclic graphs (www.dagitty.net/dags.html) to show the hypothesized relationships between variables (Figure 2). In this study, we observed that the sKlotho data were unevenly distributed and skewed. Considering that the logarithmic transformation helps to stabilize the variance and reduce the effect of outliers when dealing with skewed data, we used the natural logarithm (Ln)sKlotho to make it more suitable for our statistical analysis. This transformation may change the relationship between the variables and requires careful interpretation of the results. Therefore, when reporting the results, we also provide the results of the analyses with untransformed variables so that the reader can compare and assess the impact of the transformation. In the sensitivity analyses, a linear trend test was conducted using the WWI quartile set as the dependent variable to assess its robustness (47). Subgroup analyses were conducted using stratified multivariate regression models with stratification factors including gender, age, education, marital status, family income, HEI, PA, hypertension, diabetes, CKD, alcohol use, smoking, and SII quartile group. Smoothed curve fitting was used in this study to determine if there was a linear relationship between WWI and sKlotho. Finally, this study used Bootstrap to validate the analysis of the mediating role of SII between WWI and sKlotho (48). To maintain data and computational accuracy, this paper ensured that the samples used in the analysis were complete and accurate on all covariates by excluding missing values. All analyses were performed using EmpowerStats (http://www.empowerstats.com), SPSS27.0, and amos24.0. Statistical significance was assessed at a two-sided value of P<0.05. In subgroup analyses, to avoid increasing type I error due to multiple comparisons, false discovery rate control was used in this paper to adjust for multiple testing, and FDR-corrected p<0.1 was considered significant (49).
3 Results
3.1 Baseline characteristics of participants
A total of 9,506 participants who met the predefined inclusion and exclusion criteria were enrolled in this study, all over 45 years of age. Table 1 shows the baseline weighted characteristics of the included population stratified by sKlotho quartiles. Within the sKlotho quartiles, there were significant differences in age, gender, race, smoking, alcohol use, SII, HEI, hypertension, diabetes, CKD and education. However, no significant differences were observed in family income, BMI, PA, marital status, and energy.
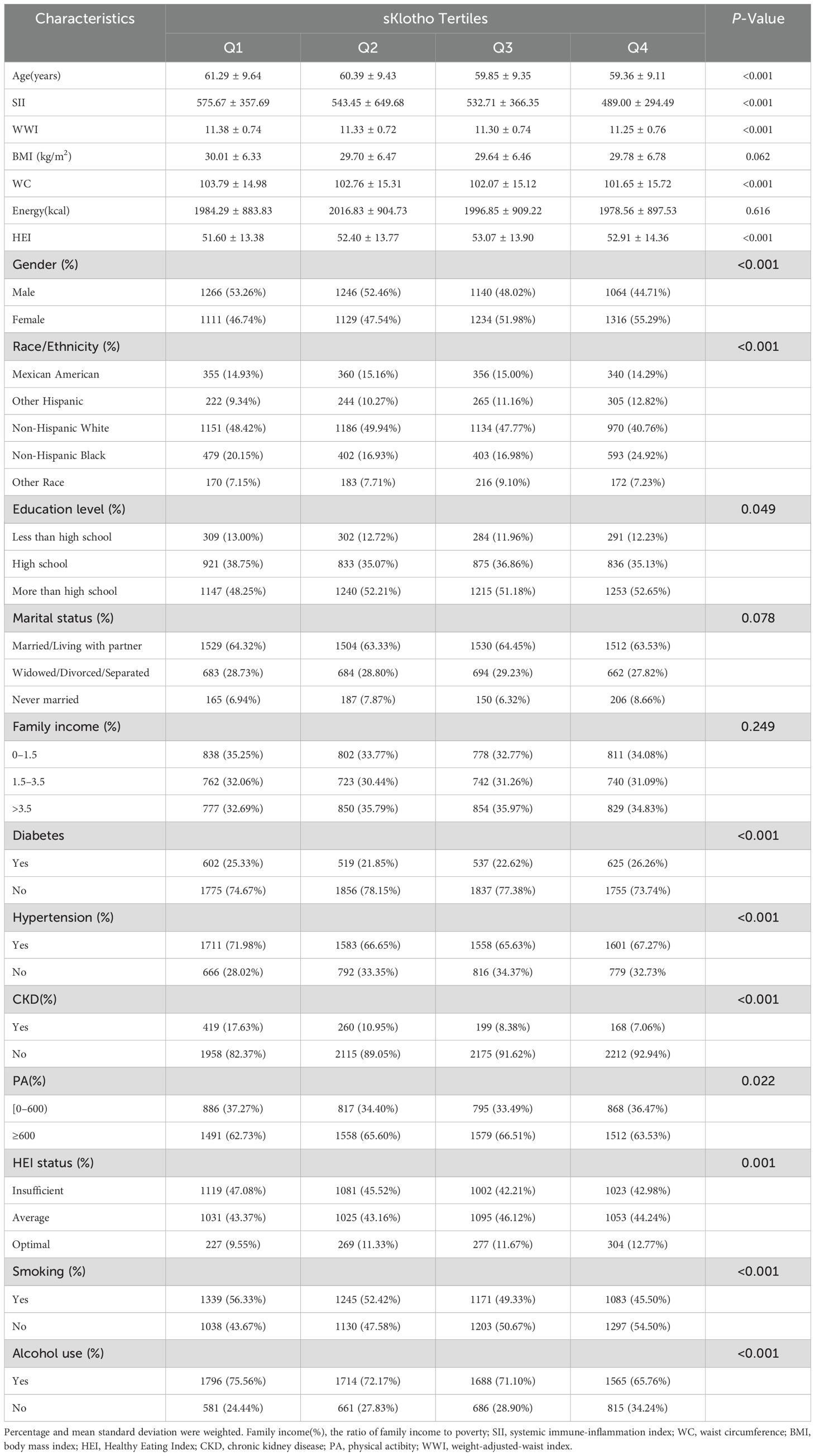
Table 1. Characteristics of included population in the NHANES based on sKlotho tertiles (N = 9,506).
3.2 The associations of SII and WWI with sKlotho
We first performed a weighted multivariate linear regression analysis of the relationship between WWI and Ln-sKlotho, and Table 2 shows that in the unadjusted model [β (95%CI) = -0.03 (-0.04, -0.02)], the minimally-adjusted model [-0.03 (-0.04, -0.02)] and the fully-adjusted model [-0.03 (-0.04, -0.01)], WWI was negatively correlated with Ln-sKlotho. Sensitivity analysis using WWI as a categorical variable (quartiles) showed that it was still statistically significant (β=-0.04, 95%CI: -0.06- -0.01, p=0.0016). Next, we analyzed the relationship between SII and Ln-sKlotho and Table 2 shows that SII was negatively correlated with Ln-sKlotho in unadjusted, minimally adjusted and fully adjusted models. We also analyzed the relationship between WWI, SII and sKlotho, and Table 3 shows that both WWI and SII were negatively correlated with sKlotho.
Based on this, subgroup analysis of the relationship between WWI and sKlotho (Table 4), gender, age, education, marital status, family income, HEI, PA, hypertension, diabetes, CKD, alcohol use, smoking, and SII did not affect the negative correlation between WWI and sKlotho (FDR-corrected p>0.1). Smooth curve fitting showed a negative correlation between WII and sKlotho (Figure 3).
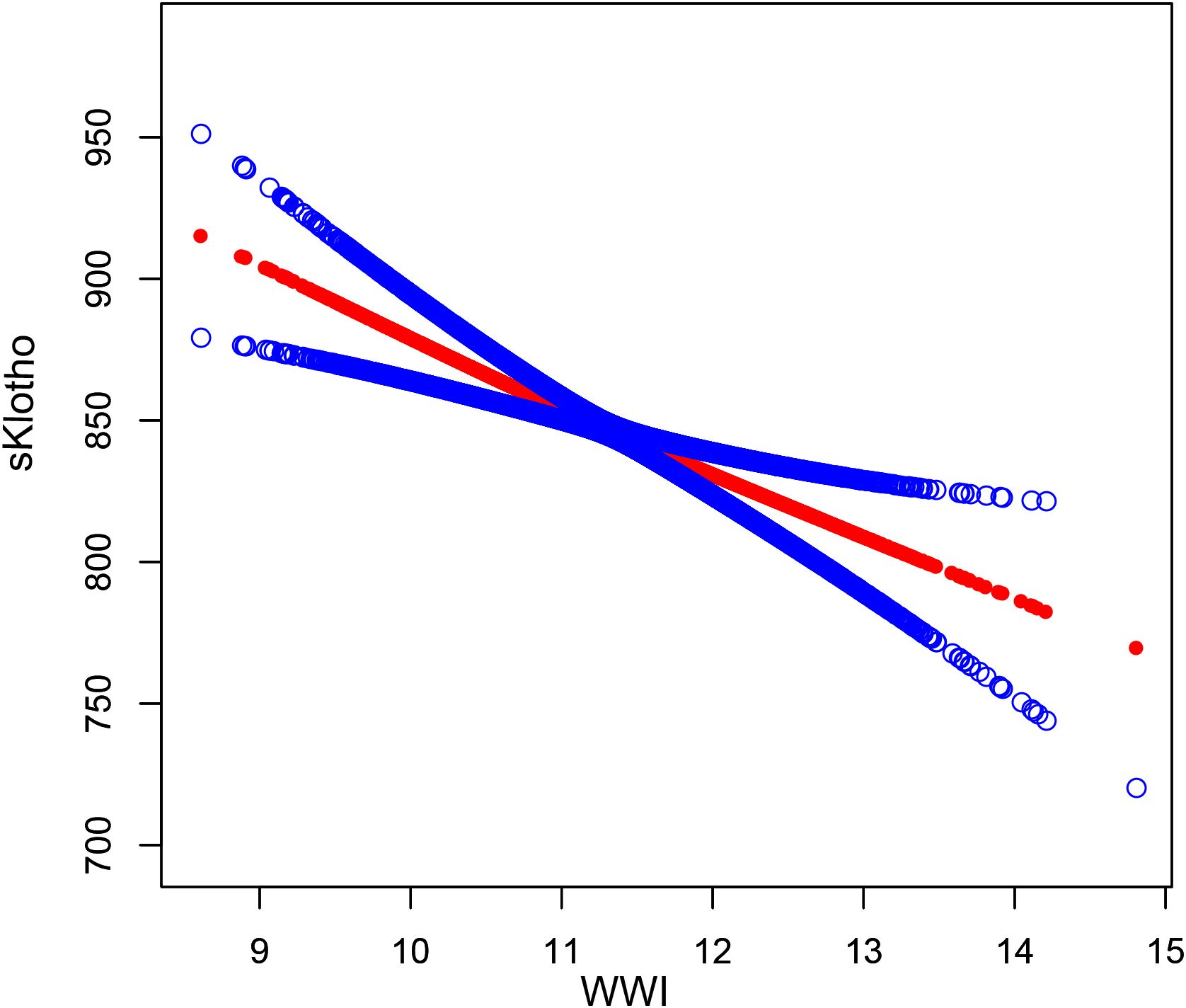
Figure 3. The association between WWl and sKlotho. The solid red line represents the smooth curve fit between variables. Blue bands represent the 95% confidence interval from the fit.
3.3 Analysis of the mediating effect of SII on the association between WWI and sKlotho
To explore the intrinsic mechanism of the significant negative effect of WWI on sKlotho, SII was further introduced as a mediating variable to substitute into the structural equation modeling in the study. The results are shown in Figure 4, in which it can be seen that WWI has a significant positive predictive effect on SII (β=0.06, t=2.58, p<0.01), and the 95% confidence intervals (hereafter collectively referred to as the “95% confidence intervals”) of the bias-corrected nonparametric percentile-based bootstrap method are [0.021, 0.105], SII had a significant negative predictive effect on sKlotho (β=-0.07, t=-4.79, p<0.001) with a 95% confidence interval of [-0.095, -0.046], while WWI had a significant negative predictive effect on sKlotho (β=-0.06, t=-5.50, p<0.001) with a 95% confidence interval of [-0.077, -0.035]. The mediating effect of SII in the effect of WWI on sKlotho was -0.004, with a 95% confidence interval of [-0.009, -0.001] excluding 0, indicating that the mediating effect of SII in the effect of WWI on sKlotho was significant. In summary, it can be concluded that the mediation model is partial. The specific results are shown in Table 5.
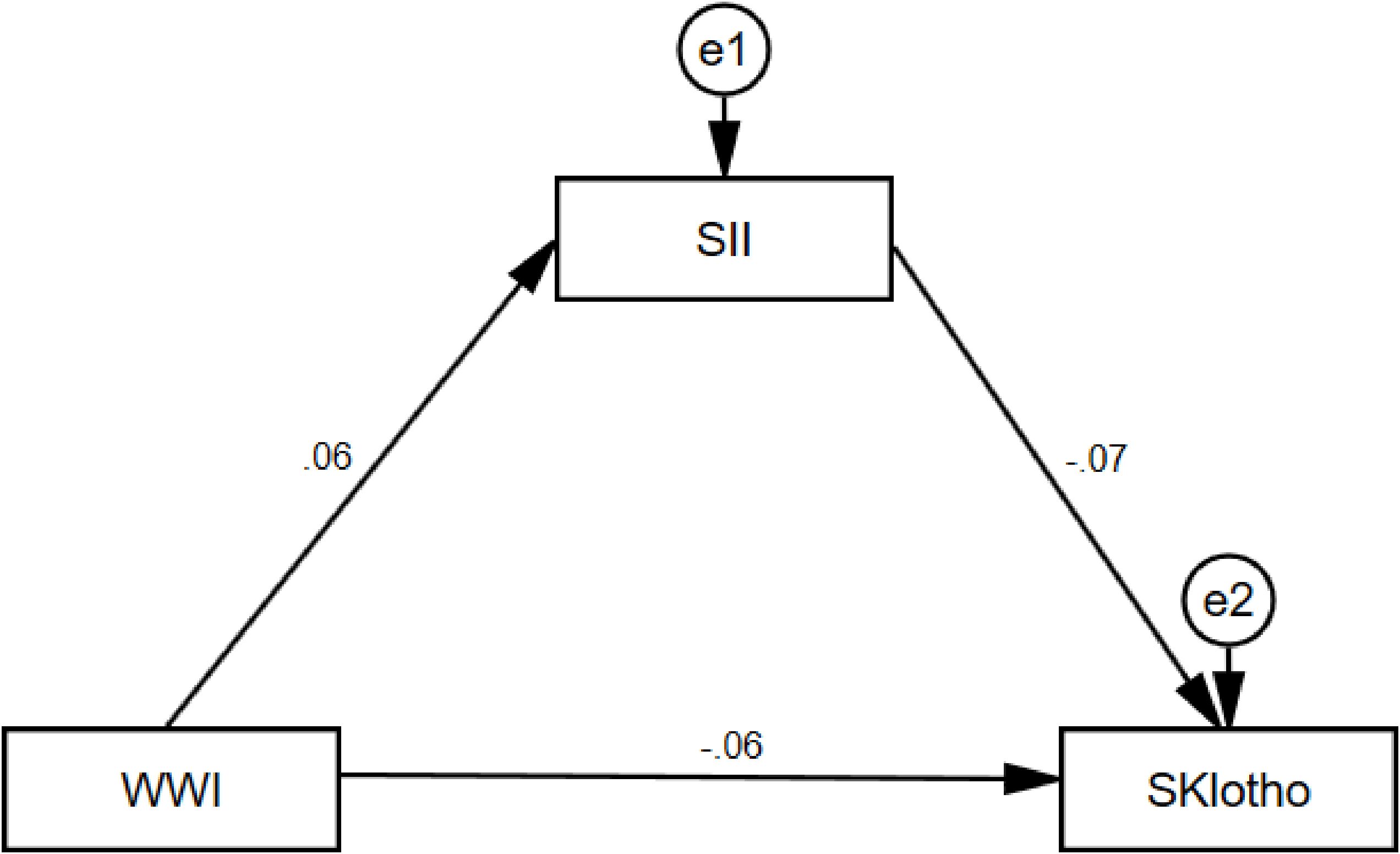
Figure 4. WWI, weight-adjusted-waist index; SII, systemic immune-inflammation index. Total effect -0.059; Direct effect -0.055; Indirect effect -0.004.
4 Discussion
This study investigated the relationship between WWI and SII and sKlotho in the United States over the age of 45. To our knowledge, this is the first study to assess the relationship between WWI and SII and sKlotho. We found that WWI was negatively associated with sKlotho in both crude and adjusted models. Sensitivity analyses with WWI as quartile also showed a correlation between WWI (β=-0.04, 95% CI: -0.06- -0.01, p=0.0016) and sKlotho. Subgroup analyses showed that gender, age, education, marital status, income, hypertension, diabetes, alcohol consumption, smoking status, and SII did not affect the negative correlation between WWI and sKlotho (FDR-corrected p>0.1) and that SII mediated the relationship between WWI and sKlotho. In future studies, we also recommend that other interested researchers measure sKlotho in patients who have lost weight through dieting or metabolic bariatric surgery to better analyze the relationship between obesity and aging.
The results of this study are similar to other studies, Jian Cui et al. showed a negative correlation between visceral obesity as measured by VAI and the anti-aging protein klotho (50). Taylor Landry et al. showed an inverse correlation between cerebrospinal fluid α-klotho and BMI (51). Multifactorial linear regression of Ping Chen et al. showed a multifactorial adjusted The multifactorial linear regression of Ping Chen et al. showed that high sKlotho levels were associated with low SII levels after multifactorial adjustment (β=-0.08,95% CI: -0.10- -0.05, p< 0.01) (52). Zhu Wen et al. also showed that SII levels were negatively correlated with serum klotho protein concentration in U.S. adults (53).
However, the mechanism behind the link between WWI and sKlotho remains unclear. Previous systematic assessments have shown that obesity may contribute to inflammation in several ways, on the one hand, adipose tissue is not only a storage of energy in the body, but is also capable of secreting a variety of adipokines (known as adipokines), including leptin, lipocalin, tumor necrosis factor-alpha (TNF-alpha), and interleukin-6 (IL-6), among others. In the state of obesity, the secretion of these adipokines is imbalanced, the expression of pro-inflammatory factors increases and the expression of anti-inflammatory factors decreases, leading to a state of chronic low-grade inflammation (54, 55). On the other hand, obese individuals often have insulin resistance, which can lead to elevated blood glucose levels, thus inducing inflammatory responses. Insulin resistance reduces the anti-inflammatory effect of insulin, leading to chronic inflammation (56). In addition, Ma TC et al. found a negative correlation between DII (Dietary Inflammatory Index) and sKlotho plasma levels and hypothesized that chronic inflammation induced by a pro-inflammatory dietary pattern could reduce plasma sKlotho level and that chronic inflammation induced by a pro-inflammatory dietary pattern could reduce serum klotho levels (57). In addition, Ma TC et al. found a negative correlation between DII (Dietary Inflammatory Index) and sKlotho plasma levels and hypothesized that chronic inflammation induced by a pro-inflammatory dietary pattern could reduce plasma sKlotho level and that chronic inflammation induced by a pro-inflammatory dietary pattern could reduce serum klotho levels (58). However, the underlying biological mechanisms between SII and sKlotho are not fully understood, and Moreno JA et al. showed that inflammatory cytokines (TWEAK and TNFα) downregulate Klotho expression by inflammatory cytokines including TWEAK and TNF through a transcription factor nuclear factor kappaB (NFκB)-dependent mechanism (59). Fitzpatrick EA et al. showed that downregulation of renal Klotho expression was associated with the amplification of inflammation in the kidney of diabetic mice (60) Whereas Klotho proteins have anti-inflammatory and anti-oxidative stress effects, a decrease in Klotho may lead to an increase in intracellular oxidative stress, which in turn promotes cellular senescence and tissue dysfunction. In our study, we found that the 6.78% relationship between WWI and sKlotho appeared to be mediated by SII. Although the mediating effect is numerically small, previous studies have shown that even if the mediating effect is small, it may still be significant. Sun Fei et al. found that SII mediated 5.08% of the correlation between WWI and depression (61). Jing Lin et al. found that 2.7% of the correlation between depression and premature coronary artery disease was mediated by SII (62). Therefore, it can be seen that SII has a certain mediating effect in the correlation of many diseases. These results suggest that SII may play a key mediating role in the relationship between WWI and sKlotho and that decreasing the inflammatory status of obese patients may increase sKlotho levels, which in turn delays the likelihood of obesity-induced aging. Of course this needs to be further verified in the clinic.
The strength of our study is that it is based on national data and the results are broadly applicable to the general population in the United States. Regression analyses adjusted for covariates, and the large sample size allowed us to perform sensitivity and subgroup analyses to confirm the robustness of the results. Finally, the mediating role of SII between WWI and sKlotho was validated. However, there are still some limitations that need to be declared. This is a cross-sectional study, which can only explore the relationship between WWI, SII and sKlotho to provide clues for etiologic studies, and cannot validate the causal relationship between disease and etiology. Based on the results of this study, we suggest that future longitudinal studies could focus on the long-term effects of WWI and SII on sKlotho levels, especially the differences in different age and sex groups. At the same time, Mendelian randomization analysis should be combined to verify whether these associations are causal in nature. This will not only help to reveal the roles of WWI and SII in aging-related diseases, but may also provide a theoretical basis for the development of new intervention strategies. Also although we have adjusted for several potential covariates, many factors still influence sKlotho, and we cannot completely rule out the influence of other potential confounders, such as other social, environmental, and weight loss drug use variables. Our study was based on U.S. adults age 45 and older, so it remains to be seen whether these results apply to young adults and other racial groups.
5 Conclusion
This study demonstrated an association between WWI, SII and sKlotho, and that SII plays an important role in mediating the relationship between WWI and sKlotho levels. These findings inform the improvement of sKlotho levels and the prevention of aging from the perspectives of obesity indicators and systemic inflammation indices, and clinical healthcare professionals should pay more attention to obesity and systemic inflammation levels in middle-aged and elderly populations to help them better prevent aging. However, due to the limitations of the study design, we were unable to determine the causal relationship between these variables. Further validation through longitudinal studies and Mendelian randomization analyses is needed in the future.
Data availability statement
Publicly available datasets were analyzed in this study. This data can be found here: www.cdc.gov/nchs/NHANEs/.
Ethics statement
The studies involving humans were approved by the National Center for Health Statistics (NCHS). The studies were conducted in accordance with the local legislation and institutional requirements. The participants provided their written informed consent to participate in this study. Written informed consent was obtained from the individual(s) for the publication of any potentially identifiable images or data included in this article.
Author contributions
MY: Conceptualization, Data curation, Formal Analysis, Methodology, Writing – original draft. QX: Conceptualization, Data curation, Formal Analysis, Supervision, Writing – review & editing. LH: Conceptualization, Data curation, Formal Analysis, Supervision, Writing – review & editing. LZ: Data curation, Formal Analysis, Methodology, Software, Supervision, Writing – review & editing.
Funding
The author(s) declare that no financial support was received for the research and/or publication of this article.
Acknowledgments
We thank the assistance of all authors in the manuscript preparation.
Conflict of interest
The authors declare that the research was conducted in the absence of any commercial or financial relationships that could be construed as a potential conflict of interest.
Generative AI statement
The author(s) declare that no Generative AI was used in the creation of this manuscript.
Publisher’s note
All claims expressed in this article are solely those of the authors and do not necessarily represent those of their affiliated organizations, or those of the publisher, the editors and the reviewers. Any product that may be evaluated in this article, or claim that may be made by its manufacturer, is not guaranteed or endorsed by the publisher.
References
1. Collaborators GA. Global, regional, and national burden of diseases and injuries for adults 70 years and older: systematic analysis for the Global Burden of Disease 2019 Study. Bmj. (2022) 376:e068208. doi: 10.1136/bmj-2021-068208
2. Kuro-o M, Matsumura Y, Aizawa H, Kawaguchi H, Suga T, Utsugi T, et al. Mutation of the mouse klotho gene leads to a syndrome resembling ageing. Nature. (1997) 390(6655):45–51. doi: 10.1038/36285
3. Jurado-Fasoli L, Castillo MJ, Amaro-Gahete FJ. Dietary Inflammatory Index and S-Klotho Plasma Levels in Middle-Aged Adults. Nutrients. (2020) 12(2):281. doi: 10.3390/nu12020281
4. Qian Y, Che L, Yan Y, Lu R, Zhu M, Xue S, et al. Urine klotho is a potential early biomarker for acute kidney injury and associated with poor renal outcome after cardiac surgery. BMC Nephrol. (2019) 20(1):268. doi: 10.1186/s12882-019-1460-5
5. Zimmermann M, Köhler L, Kovarova M, Lerche S, Schulte C, Wurster I, et al. The longevity gene Klotho and its cerebrospinal fluid protein profiles as a modifier for Parkinson´s disease. Eur J Neurol. (2021) 28(5):1557–65. doi: 10.1111/ene.14733
6. Prud'homme GJ, Kurt M, Wang Q. Pathobiology of the klotho antiaging protein and therapeutic considerations. Front Aging. (2022) 3:931331. doi: 10.3389/fragi.2022.931331
7. Matsumura Y, Aizawa H, Shiraki-Iida T, Nagai R, Kuro-o M, Nabeshima Y. Identification of the human klotho gene and its two transcripts encoding membrane and secreted klotho protein. Biochem Biophys Res Commun. (1998) 242(3):626–30. doi: 10.1006/bbrc.1997.8019
8. Yamamoto M, Clark JD, Pastor JV, Gurnani P, Nandi A, Kurosu H, et al. Regulation of oxidative stress by the anti-aging hormone klotho. J Biol Chem. (2005) 280(45):38029–34. doi: 10.1074/jbc.M509039200
9. Ikushima M, Rakugi H, Ishikawa K, Maekawa Y, Yamamoto K, Ohta J, et al. Anti-apoptotic and anti-senescence effects of Klotho on vascular endothelial cells. Biochem Biophys Res Commun. (2006) 339(3):827–32. doi: 10.1016/j.bbrc.2005.11.094
10. Ebert T, Pawelzik SC, Witasp A, Arefin S, Hobson S, Kublickiene K, et al. Inflammation and premature ageing in chronic kidney disease. Toxins (Basel). (2020) 12(4):227. doi: 10.3390/toxins12040227
11. Kanbay M, Demiray A, Afsar B, Covic A, Tapoi L, Ureche C, et al. Role of klotho in the development of essential hypertension. Hypertension. (2021) 77(3):740–50. doi: 10.1161/HYPERTENSIONAHA.120.16635
12. Frasca D. Several areas of overlap between obesity and aging indicate obesity as a biomarker of accelerated aging of human B cell function and antibody responses. Immun Ageing. (2022) 19(1):48. doi: 10.1186/s12979-022-00301-z
13. Salvestrini V, Sell C, Lorenzini A. Obesity may accelerate the aging process. Front Endocrinol (Lausanne). (2019) 10:266. doi: 10.3389/fendo.2019.00266
14. Santos AL, Sinha S. Obesity and aging: Molecular mechanisms and therapeutic approaches. Ageing Res Rev. (2021) 67:101268. doi: 10.1016/j.arr.2021.101268
15. Zhang Y, Xu Z, Yang Y, Cao S, Lyu S, Duan W. Association between weight change and leukocyte telomere length in U.S. adults. Front Endocrinol (Lausanne). (2021) 12:650988. doi: 10.3389/fendo.2021.650988
16. Lampropoulos C, Alexandrides T, Tsochatzis S, Kehagias D, Kehagias I. Are the changes in gastrointestinal hormone secretion necessary for the success of bariatric surgery? A critical review of the literature. Obes Surg. (2021) 31(10):4575–84. doi: 10.1007/s11695-021-05568-7
17. Jin X, Liu J, Cao Q, Lin J, Wu G, Liu L, et al. Normal-weight central obesity: implications for diabetes mellitus. Front Nutr. (2023) 10:1239493. doi: 10.3389/fnut.2023.1239493
18. Stefan N, Schick F, Häring HU. Causes, characteristics, and consequences of metabolically unhealthy normal weight in humans. Cell Metab. (2017) 26(2):292–300. doi: 10.1016/j.cmet.2017.07.008
19. Magkos F. Metabolically healthy obesity: what's in a name? Am J Clin Nutr. (2019) 110(3):533–9. doi: 10.1093/ajcn/nqz133
20. Wang X, Yang S, He G, Xie L. The association between weight-adjusted-waist index and total bone mineral density in adolescents: NHANES 2011-2018. Front Endocrinol (Lausanne). (2023) 14:1191501. doi: 10.3389/fendo.2023.1191501
21. Jackson AS, Stanforth PR, Gagnon J, Rankinen T, Leon AS, Rao DC, et al. The effect of sex, age and race on estimating percentage body fat from body mass index: The Heritage Family Study. Int J Obes Relat Metab Disord. (2002) 26(6):789–96. doi: 10.1038/sj.ijo.0802006
22. Lam BC, Koh GC, Chen C, Wong MT, Fallows SJ. Comparison of body mass index (BMI), body adiposity index (BAI), waist circumference (WC), waist-to-hip ratio (WHR) and waist-to-height ratio (WHtR) as predictors of cardiovascular disease risk factors in an adult population in Singapore. PloS One. (2015) 10(4):e0122985. doi: 10.1371/journal.pone.0122985
23. Browning LM, Hsieh SD, Ashwell M. A systematic review of waist-to-height ratio as a screening tool for the prediction of cardiovascular disease and diabetes: 0·5 could be a suitable global boundary value. Nutr Res Rev. (2010) 23(2):247–69. doi: 10.1017/S0954422410000144
24. Park Y, Kim NH, Kwon TY, Kim SG. A novel adiposity index as an integrated predictor of cardiometabolic disease morbidity and mortality. Sci Rep. (2018) 8(1):16753. doi: 10.1038/s41598-018-35073-4
25. Li X, Wang L, Zhou H, Xu H. Association between weight-adjusted-waist index and chronic kidney disease: a cross-sectional study. BMC Nephrol. (2023) 24(1):266. doi: 10.1186/s12882-023-03316-w
26. Typiak M, Piwkowska A. Antiinflammatory actions of klotho: Implications for Therapy of Diabetic Nephropathy. Int J Mol Sci. (2021) 22(2):956. doi: 10.3390/ijms22020956
27. Martín-González C, Espelosín-Ortega E, Abreu-González P, Fernández-Rodríguez C, Vera-Delgado VE, González-Navarrete L, et al. Klotho levels and their relationship with inflammation and survival among alcoholic patients. Biomolecules. (2022) 12(8):1151. doi: 10.3390/biom12081151
28. Weisberg SP, McCann D, Desai M, Rosenbaum M, Leibel RL, Ferrante AW Jr.. Obesity is associated with macrophage accumulation in adipose tissue. J Clin Invest. (2003) 112(12):1796–808. doi: 10.1172/JCI19246
29. Liu R, Nikolajczyk BS. Tissue immune cells fuel obesity-associated inflammation in adipose tissue and beyond. Front Immunol. (2019) 10:1587. doi: 10.3389/fimmu.2019.01587
30. Valdearcos M, Douglass JD, Robblee MM, Dorfman MD, Stifler DR, Bennett ML, et al. Microglial inflammatory signaling orchestrates the hypothalamic immune response to dietary excess and mediates obesity susceptibility. Cell Metab. (2018) 27(6):1356. doi: 10.1016/j.cmet.2018.04.019
31. Chen Y, Huang R, Mai Z, Chen H, Zhang J, Zhao L, et al. Association between systemic immune-inflammatory index and diabetes mellitus: mediation analysis involving obesity indicators in the NHANES. Front Public Health. (2023) 11:1331159. doi: 10.3389/fpubh.2023.1331159
32. Hu B, Yang XR, Xu Y, Sun YF, Sun C, Guo W, et al. Systemic immune-inflammation index predicts prognosis of patients after curative resection for hepatocellular carcinoma. Clin Cancer Res. (2014) 20(23):6212–22. doi: 10.1158/1078-0432.CCR-14-0442
33. Huang H, Liu Q, Zhu L, Zhang Y, Lu X, Wu Y, et al. Prognostic value of preoperative systemic immune-inflammation index in patients with cervical cancer. Sci Rep. (2019) 9(1):3284. doi: 10.1038/s41598-019-39150-0
34. Nie Y, Zhou H, Wang J, Kan H. Association between systemic immune-inflammation index and diabetes: a population-based study from the NHANES. Front Endocrinol (Lausanne). (2023) 14:1245199. doi: 10.3389/fendo.2023.1245199
35. Yamazaki Y, Imura A, Urakawa I, Shimada T, Murakami J, Aono Y, et al. Establishment of sandwich ELISA for soluble alpha-Klotho measurement: Age-dependent change of soluble alpha-Klotho levels in healthy subjects. Biochem Biophys Res Commun. (2010) 398(3):513–8. doi: 10.1016/j.bbrc.2010.06.110
36. Chen Y, Li Y, Liu M, Xu W, Tong S, Liu K. Association between systemic immunity-inflammation index and hypertension in US adults from NHANES 1999-2018. Sci Rep. (2024) 14(1):5677. doi: 10.1038/s41598-024-56387-6
37. Mangalesh S, Dudani S, Malik A. The systemic immune-inflammation index in predicting sepsis mortality. Postgrad Med. (2023) 135(4):345–51. doi: 10.1080/00325481.2022.2140535
38. Albany C. Systemic immune-inflammation index in germ-cell tumours: search for a biological prognostic biomarker. Br J Cancer. (2018) 118(6):761–2. doi: 10.1038/bjc.2018.7
39. Song Y, Guo W, Li Z, Guo D, Li Z, Li Y. Systemic immune-inflammation index is associated with hepatic steatosis: Evidence from NHANES 2015-2018. Front Immunol. (2022) 13:1058779. doi: 10.3389/fimmu.2022.1058779
40. De La Cruz N, Shabaneh O, Appiah D. The association of ideal cardiovascular health and ocular diseases among US adults. Am J Med. (2021) 134(2):252–9.e1. doi: 10.1016/j.amjmed.2020.06.004
41. Bundy JD, Mills KT, Anderson AH, Yang W, Chen J, He J, et al. Prediction of end-stage kidney disease using estimated glomerular filtration rate with and without race : a prospective cohort study. Ann Intern Med. (2022) 175(3):305–13. doi: 10.7326/M21-2928
42. Yuan M, He J, Hu X, Yao L, Chen P, Wang Z, et al. Hypertension and NAFLD risk: Insights from the NHANES 2017–2018 and Mendelian randomization analyses. Chin Med J (Engl). (2024) 137(4):457–64. doi: 10.1097/CM9.0000000000002753
43. Cleland CL, Hunter RF, Kee F, Cupples ME, Sallis JF, Tully MA. Validity of the global physical activity questionnaire (GPAQ) in assessing levels and change in moderate-vigorous physical activity and sedentary behaviour. BMC Public Health. (2014) 14:1255. doi: 10.1186/1471-2458-14-1255
44. Wu Y, Lei S, Li D, Li Z, Zhang Y, Guo Y. Relationship of Klotho with cognition and dementia: Results from the NHANES 2011–2014 and Mendelian randomization study. Transl Psychiatry. (2023) 13(1):337. doi: 10.1038/s41398-023-02632-x
45. Kim JY, Choi J, Vella CA, Criqui MH, Allison MA, Kim NH. Associations between weight-adjusted waist index and abdominal fat and muscle mass: multi-ethnic study of atherosclerosis. Diabetes Metab J. (2022) 46(5):747–55. doi: 10.4093/dmj.2021.0294
46. Marcoulides KM, Raykov T. Evaluation of variance inflation factors in regression models using latent variable modeling methods. Educ Psychol Meas. (2019) 79(5):874–82. doi: 10.1177/0013164418817803
47. VanderWeele TJ, Ding P. Sensitivity analysis in observational research: introducing the e-value. Ann Intern Med. (2017) 167(4):268–74. doi: 10.7326/M16-2607
48. Luo CY, Jiao P, Tu SM, Shen L, Sun YM. Mediating role of physical activity in the relationship between psychological distress and intimate relationships among stroke patients. World J Psychiatry. (2023) 13(12):1096–105. doi: 10.5498/wjp.v13.i12.1096
49. Shuai M, Zuo LS, Miao Z, Gou W, Xu F, Jiang Z, et al. Multi-omics analyses reveal relationships among dairy consumption, gut microbiota and cardiometabolic health. EBioMedicine. (2021) 66:103284. doi: 10.1016/j.ebiom.2021.103284
50. Cui J, Yang Z, Wang J, Yin S, Xiao Y, Bai Y, et al. A cross-sectional analysis of association between visceral adiposity index and serum anti-aging protein Klotho in adults. Front Endocrinol (Lausanne). (2023) 14:1082504. doi: 10.3389/fendo.2023.1082504
51. Landry T, Li P, Shookster D, Jiang Z, Li H, Laing BT, et al. Centrally circulating α-klotho inversely correlates with human obesity and modulates arcuate cell populations in mice. Mol Metab. (2021) 44:101136. doi: 10.1016/j.molmet.2020.101136
52. Chen P, Tang Y, Luo L, Chen H, He X. Lower serum klotho level and higher systemic immune-inflammation index: an inverse correlation. BMC Geriatr. (2023) 23(1):650. doi: 10.1186/s12877-023-04349-4
53. Wen Z, Liu X, Zhang T. L-shaped association of systemic immune-inflammation index (SII) with serum soluble α-Klotho in the prospective cohort study from the NHANES database. Sci Rep. (2024) 14(1):13189. doi: 10.1038/s41598-024-64050-3
54. Coelho M, Oliveira T, Fernandes R. Biochemistry of adipose tissue: an endocrine organ. Arch Med Sci. (2013) 9(2):191–200. doi: 10.5114/aoms.2013.33181
55. Ellulu MS, Patimah I, Khaza'ai H, Rahmat A, Abed Y. Obesity and inflammation: the linking mechanism and the complications. Arch Med Sci. (2017) 13(4):851–63. doi: 10.5114/aoms.2016.58928
56. Rehman K, Akash MS. Mechanisms of inflammatory responses and development of insulin resistance: how are they interlinked? J BioMed Sci. (2016) 23(1):87. doi: 10.1186/s12929-016-0303-y
57. Izquierdo MC, Perez-Gomez MV, Sanchez-Niño MD, Sanz AB, Ruiz-Andres O, Poveda J, et al. Klotho, phosphate and inflammation/ageing in chronic kidney disease. Nephrol Dial Transplant. (2012) 27 Suppl 4:iv6–10. doi: 10.1093/ndt/gfs426
58. Ma TC, Zhou J, Wang CX, Fang M, Gao F. Association between dietary inflammatory index and s-klotho plasma levels in middle-aged and elderly people. Front Nutr. (2022) 9:853332. doi: 10.3389/fnut.2022.853332
59. Moreno JA, Izquierdo MC, Sanchez-Niño MD, Suárez-Alvarez B, Lopez-Larrea C, Jakubowski A, et al. The inflammatory cytokines TWEAK and TNFα reduce renal klotho expression through NFκB. J Am Soc Nephrol. (2011) 22(7):1315–25. doi: 10.1681/ASN.2010101073
60. Fitzpatrick EA, Han X, Xiao Z, Quarles LD. Role of fibroblast growth factor-23 in innate immune responses. Front Endocrinol (Lausanne). (2018) 9:320. doi: 10.3389/fendo.2018.00320
61. Fei S, Liu M, Shanshan H, Xie R, Danni W, Ningying Z. Association between weight-adjusted-waist index and depression: a cross-sectional study. Endocr Connect. (2024) 13(6):e230450. doi: 10.1530/EC-23-0450
Keywords: sKlotho, WWI, SII, aging, mediating effect, NHANES
Citation: Yang M, Xu Q, Huang L and Zhu L (2025) Associations of weight-adjusted-waist index and systemic immune-inflammatory index with sKlotho: evidence from the NHANES 2007-2016. Front. Endocrinol. 16:1544796. doi: 10.3389/fendo.2025.1544796
Received: 13 December 2024; Accepted: 11 April 2025;
Published: 01 May 2025.
Edited by:
James Harper, Sam Houston State University, United StatesReviewed by:
Dimitrios Kehagias, University of Patras, GreeceSedat Arslan, Bandirma Onyedi Eylül University, Türkiye
Copyright © 2025 Yang, Xu, Huang and Zhu. This is an open-access article distributed under the terms of the Creative Commons Attribution License (CC BY). The use, distribution or reproduction in other forums is permitted, provided the original author(s) and the copyright owner(s) are credited and that the original publication in this journal is cited, in accordance with accepted academic practice. No use, distribution or reproduction is permitted which does not comply with these terms.
*Correspondence: Qiong Xu, NDI1MDAwMTMwQHFxLmNvbQ==