- 1Department of Cardiology, Shanghai Tenth People’s Hospital, Tongji University School of Medicine, Shanghai, China
- 2Department of Geriatrics, Shanghai Putuo People’s Hospital, School of Medicine, Tongji University, Shanghai, China
- 3Department of Cardiology, Central Laboratory of Lishui Hospital of Wenzhou Medical University, The First Affiliated Hospital of Lishui University, Lishui People’s Hospital, Lishui, Zhejiang, China
- 4Department of pharmacy, Central Laboratory of The Lishui Hospital of Wenzhou Medical University, The First Affiliated Hospital of Lishui University, Lishui, Zhejiang, China
- 5Department of Vascular Surgery, Lishui People’s Hospital, Lishui Hospital of Wenzhou Medical University, The First Affiliated Hospital of Lishui University, Lishui, Zhejiang, China
Background: Cardiovascular disease (CVD) remains a leading cause of morbidity and mortality globally, with metabolic syndrome (MS) being a significant contributor to major adverse cardiovascular events (MACE). While the relationship between MS and CVD is well established, limited studies have focused on elderly populations, particularly in the context of long-term cardiovascular outcomes. This study aims to fill this gap by investigating the impact of MS and its components on MACE in an elderly population.
Methods: We conducted a prospective cohort study involving 3,352 elderly residents from the Northern Shanghai Study (NSS), followed for an average of 5.6 years. MS was defined based on modified NCEP ATP III criteria. The primary outcome was MACE, including non-fatal myocardial infarction (MI), non-fatal stroke, cardiovascular death, and all-cause mortality. Kaplan-Meier survival analysis and multivariate logistic regression were used to examine the association between MS and MACE.
Results: MS was present in 44.2% of the cohort. Participants with MS had a significantly higher incidence of MACE compared to those without MS (12.69% vs. 9.30%, p=0.02). MS was confirmed as an independent risk factor for MACE (HR 1.471, 95% CI 1.195–1.181, p=0.001). Among the individual components of MS, the combination of central obesity, hypertension, and hyperglycemia (ABD+BP+GLU) showed the strongest predictive value for MACE (HR 3.001, 95% CI 1.640–5.492, p<0.001).
Conclusions: MS is an independent predictor of MACE in elderly populations, with central obesity, hypertension, and hyperglycemia being the most critical risk factors. These findings underscore the importance of early identification and management of MS to reduce cardiovascular risk and improve long-term outcomes in elderly individuals.
1 Introduction
Cardiovascular disease (CVD) remains a leading cause of morbidity and mortality worldwide, with its prevalence and associated disability increasing significantly over recent decades (1–3). The Global Burden of Disease study highlights a dramatic rise in CVD cases, reaching 525 million by 2019, nearly doubling from 1990 (1). As populations continue to age globally, CVD is expected to remain a major health burden, particularly in elderly populations (4). Major adverse cardiovascular events (MACE), including non-fatal myocardial infarction (MI), non-fatal stroke, and cardiovascular-related death, are key outcomes used to assess the severity of CVD and its impact on health (5, 6). Preventing these outcomes is crucial in managing cardiovascular risk, particularly among elderly individuals who are more susceptible to MACE due to the accumulation of risk factors with age.
Metabolic syndrome (MS) is defined by a cluster of metabolic abnormalities, including central obesity, hypertension, dyslipidemia, and impaired glucose metabolism (7, 8). Rising rates of MS, driven by increasing obesity and sedentary lifestyles, contribute significantly to adverse cardiovascular outcomes and mortality (9–11). Studies have consistently shown that MS is a major risk factor for CVD, significantly increasing the risk of MACE (12–14). Meta-analyses and large cohort studies have demonstrated that MS nearly doubles the likelihood of cardiovascular events, including MI and stroke (15–19). Given this elevated risk, MS is considered a pre-disease state, emphasizing the need for early intervention to prevent the progression to overt cardiovascular disease (20). Elderly individuals are particularly vulnerable to the effects of MS, as multiple cardiovascular risk factors tend to cluster and intensify with age, amplifying the risk of MACE. However, most studies have focused on general populations or younger individuals, with few research exploring the specific impact of MS on MACE in elderly populations particularly in rapidly urbanizing regions where the burden of CVD and MS is increasing. Understanding the relationship between MS and MACE in elderly is crucial for improving early identification of high-risk individuals and improving preventive care strategies.
The current study aims to address the research gap by assessing the association between MS and MACE in an elderly, community-dwelling population in northern Shanghai. Additionally, we examine the contribution of individual components of MS—such as central obesity, hypertension, dyslipidemia, and impaired glucose metabolism—to the risk of MACE. These insights will inform clinicians and public health policymakers to better target preventive measures and reduce cardiovascular risk in the elderly.
2 Materials and methods
2.1 Research subjects
The Northern Shanghai Study (NSS, ClinicalTrials.gov Identifier: NCT02368938) is a prospective study aimed at establishing cardiovascular risk scores based on a community-dwelling elderly Chinese population. The study seeks to identify relevant cardiovascular risk factors and target organ damage profiles to guide future interventions. From June 2014 to July 2019, a total of 3,590 residents from the northern Shanghai community were invited to participate in the study, with 3,363 individuals (93.7%) participating in the initial screening process. Among them, 3,352 participants (99.7%) completed an average follow-up of 2,048 days. This study was approved by the Ethics Committee of the Tenth People’s Hospital affiliated with Tongji University, and all participants provided written informed consent.
2.1.1 Inclusion criteria
1. Age ≥ 65 years;
2. Community residents from northern Shanghai;
3. Ability to participate in long-term follow-up.
2.1.2 Exclusion criteria
1. Severe heart disease (New York Heart Association Class IV) or end-stage renal disease (Chronic Kidney Disease Stage ≥ 4);
2. Malignant tumors with an expected survival of < 5 years;
3. History of stroke within the past 3 months.
All participants in this study were surveyed using a standard questionnaire, which included basic information such as gender, age, and family history of cardiovascular disease, as well as smoking history and status. During the interviews, previous diagnoses of diabetes, hypertension, coronary artery disease, stroke, and stroke types were also collected.
2.2 Research methodology
2.2.1 Human body parameter measurement
Body weight is measured using a calibrated electronic scale with an accuracy of 0.1 kg, while height is measured using a tape measure. Body Mass Index (BMI) is calculated as follows: BMI = Weight (kg)/Height² (m²). Waist Circumference (WC) is measured as the horizontal circumference at the midpoint between the lower edge of the rib cage and the iliac crest along the midaxillary line (21–23). Blood Pressure (BP) measurement follows the recommendations of the European Society of Hypertension: the subject should sit quietly for more than 10 minutes. BP is measured 3 times using a conventional cuff mercury sphygmomanometer, with a 3-minute interval between each measurement, and the average value is taken.
2.2.2 Laboratory tests
Blood samples were collected from study participants after a 10-hour overnight fasting period and analyzed by the Department of Laboratory Medicine at Shanghai Tenth People’s Hospital using standardized protocols and certified equipment. Fasting plasma glucose (FPG) was measured using the hexokinase method on an automated biochemical analyzer (Hitachi 7600), with intra- and inter-assay coefficients of variation (CVs) of 1.5% and 2.0%, respectively. Lipid profiles, including total cholesterol (TC), triglycerides (TG), high-density lipoprotein cholesterol (HDL-c), and low-density lipoprotein cholesterol (LDL-c), were determined using enzymatic colorimetric assays. TC and TG measurements had intra-assay CVs of 1.2% and 1.8% and inter-assay CVs of 1.5% and 2.1%, respectively. HDL-c was measured using a homogeneous enzymatic assay (intra-assay CV: 1.3%; inter-assay CV: 1.7%), while LDL-c was calculated using the Friedewald formula (for TG < 4.5 mmol/L) or directly measured (intra-assay CV: 1.4%; inter-assay CV: 1.9%). Internal quality control samples were analyzed daily, and the laboratory participated in external quality assurance programs to ensure accuracy and precision.
2.2.3 Definition of metabolic syndrome
According to the Third Report of the National Cholesterol Education Program (NCEP) Adult Treatment Panel III (ATP III) in 2005 (24), MS is defined as the presence of at least three of the following metabolic disorders, with modifications made for the Asian population: Waist Circumference (WC) ≥90 cm for men and ≥80 cm for women; Blood Pressure (BP) ≥130/85 mmHg and/or treatment for previously diagnosed hypertension; Fasting Plasma Glucose (FPG) ≥5.6 mmol/L and/or previously diagnosed type 2 diabetes; Serum Triglycerides (TG) ≥1.7 mmol/L and/or specific treatment for lipid abnormalities; High-Density Lipoprotein Cholesterol (HDL-c) <1.03 mmol/L for men and <1.29 mmol/L for women and/or specific treatment for lipid abnormalities.
2.3 Follow-up
All participants were followed up through telephone interviews or on-site visits. The follow-up period ranged from 3 to 8 years, with an average follow-up time of 5.6 years. During this period, new diagnoses of diseases were tracked through self-reports, including non-fatal myocardial infarction, non-fatal stroke, cardiovascular death, and all-cause mortality. The exact time of these events was recorded. In the case of multiple clinical events occurring in the same patient, the first event was considered the endpoint event. Patients who were lost to follow-up or without MACE were regarded as censored patients. The follow-up endpoint event data is shown in Figure 1.
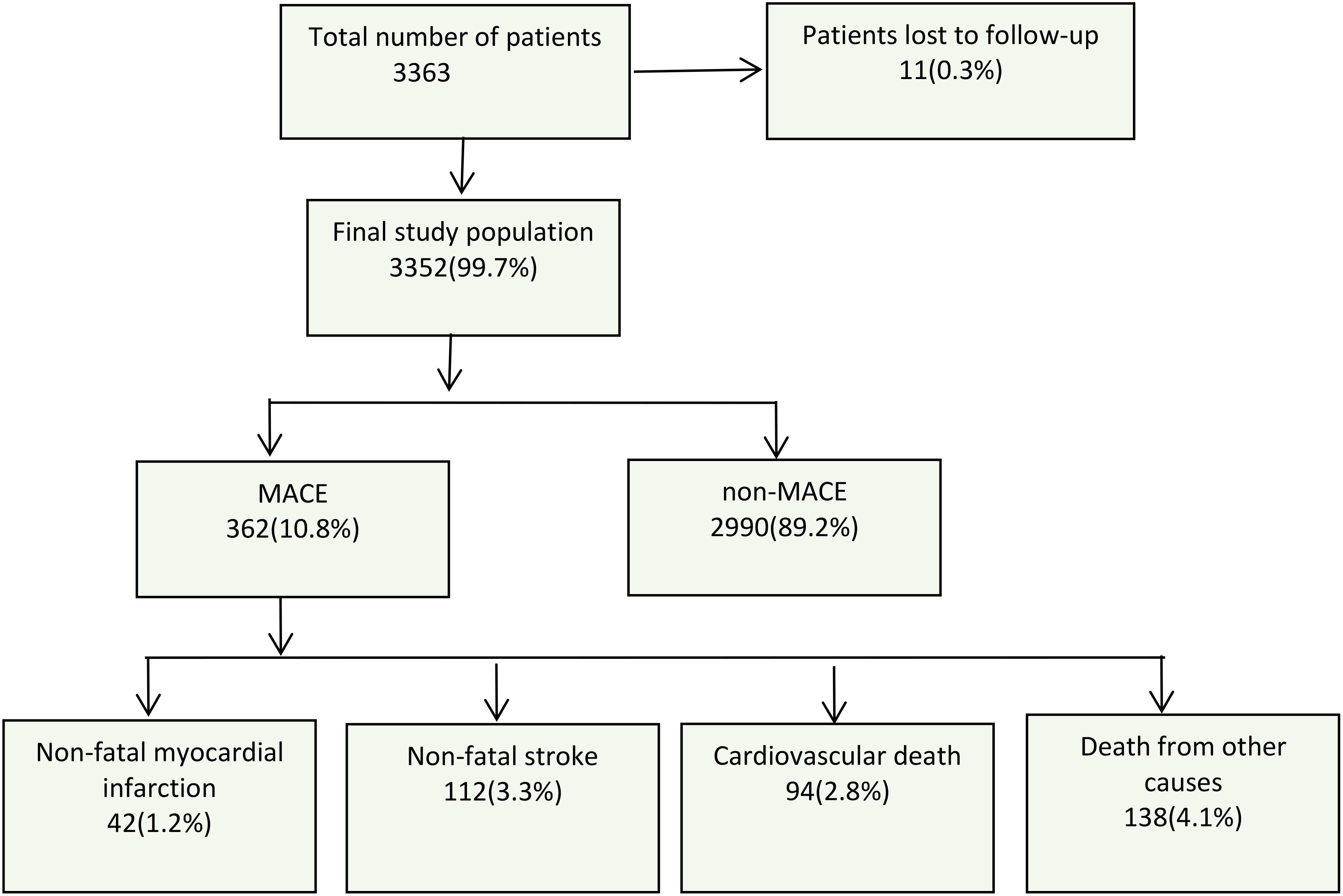
Figure 1. Flow chart of the study process and follow-up conditions. MACE, major adverse cardiovascular events.
2.4 Statistical analysis
Data were analyzed using SPSS 13.0, with p < 0.05 considered significant. Normality was assessed via the Kolmogorov-Smirnov test: normally distributed variables were reported as mean ± SD (compared via t-test), while non-normal data used median (IQR) (compared via Wilcoxon test). Categorical variables were expressed as n (%) (compared via χ² test). Univariate analysis screened risk factors for MACE, followed by Kaplan-Meier analysis (log-rank test) to assess MS-MACE associations over time. Logistic regression calculated ORs (95% CI) for MACE risk, and Cox proportional hazards models estimated adjusted HRs (95% CI) for MS and its components, with checks for proportional hazards assumptions.
3 Results
3.1 Clinical characteristics of study subjects
This study followed up on 3352 subjects, categorized into the MS group (1481 subjects, 44.2%) and the non-MS group (1871 subjects, 55.8%) according to NCEPIII. As shown in Table 1, there were no significant differences between the two groups in terms of age, systolic BP, TC, LDL-c, history of diabetes, and early onset of CVD. However, the MS group had higher waist circumference, hip circumference, waist-to-hip ratio (WHR), diastolic BP, TG, HDL-c, and FBG levels compared to the non-MS group (all P values <0.01). The MS group also had a higher prevalence of hypertension, stroke, MI, and a family history of hypertension. Interestingly, the proportion of smokers was higher in the non-MS group (all P values <0.01).
Analysis of the 1481 subjects with metabolic syndrome revealed that the most common combination of metabolic syndrome components was central obesity + hypertension + high blood glucose (ABD+BP+GLU) (299 cases, 20.2%), followed by central obesity + hypertension + high blood glucose + high triglycerides + low high-density lipoprotein cholesterol (ABD+BP+GLU+TRI+HDL-c) (191 cases, 12.9%), central obesity + hypertension + high blood glucose + high triglycerides (ABD+BP+GLU+TRI) (162 cases, 10.9%), and central obesity + hypertension + high triglycerides (ABD+BP+TRI) (151 cases, 10.2%). There were gender differences in the combination’s ABD+BP+GLU+TRI+HDL-c (12.9%), ABD+BP+TRI+HDL-c (10.0%), ABD+GLU+TRI+HDL-c (14.7%), BP+GLU+TRI+HDL-c (2.8%), and BP+GLU+TRI (4.4%) (see Table 2).
3.2 Analysis of endpoint events
The endpoint was MACE, including non-fatal MI, non-fatal stroke, cardiovascular death, and death from other causes. After an average follow-up of 5.6 years, there were a total of 362 (10.8%) endpoint events, including 41 (1.2%) cases of non-fatal MI, 112 (3.3%) cases of non-fatal stroke, 94 (2.8%) cases of cardiovascular death, and 138 (4.1%) cases of death from other causes (Figure 1).
At the end of the follow-up, the overall probability of endpoint events was higher in the MS group compared to the non-MS group, with a statistically significant difference between the two groups (188 cases, 12.69% vs. 174 cases, 9.30%, p=0.02). Regarding specific types of MACE, the MS group had higher rates than the non-MS group for non-fatal MI, non-fatal stroke, and overall mortality (2.02% vs. 1.64%, p<0.001; 4.04% vs. 2.77%, p=0.052; 7.94% vs. 6.13%, p<0.001). However, the difference in cardiovascular death between the two groups was not significant (3.43% vs. 2.34%, p=0.060) (Table 3).
3.3 Regression analysis of endpoint events
As shown in Table 4, we analyzed the relationship between MS and various endpoint events. According to the NCEP III (2005) criteria for identifying study subjects with MS, the unadjusted logistic regression analysis showed the following MACE risks: overall MACE (OR = 1.416, 95% CI: 1.138–1.762, P = 0.02), non-fatal myocardial infarction (OR = 3.200, 95% CI: 1.633–6.172, P = 0.01), non-fatal stroke (OR = 1.475, 95% CI: 1.011–2.125, P = 0.044), cardiovascular death (OR = 1.582, 95% CI: 0.938–2.861, P = 0.088), and all-cause mortality (OR = 1.320, 95% CI: 1.011–1.722, P = 0.041). After adjusting for age, gender, smoking history, family history of hypertension, and family history of early-onset CVD, the results remained similar. MS was significantly associated with overall endpoint events, non-fatal myocardial infarction, non-fatal stroke, and all-cause mortality (all P < 0.05).
The COX regression analysis indicated that MS is an independent risk factor for MACE [HR 1.471, 95% CI 1.195-1.181, p=0.001], primarily due to the increased risk of all-cause mortality, non-fatal stroke, and non-fatal MI (HR 1.422, 95% CI 1.097-1.843, p=0.008; HR 1.506, 95% CI 1.039-2.184, p=0.031; HR 3.484, 95% CI 1.780-6.811, p<0.001) (Table 5). There was a significant difference in the Kaplan-Meier curves between the two groups (p=0.001), showing similar results that the high MS group had a higher risk of MACE compared to the non-MS group (Figure 2).
Within the MS group, multivariate COX regression analysis showed that a BMI ≥ 28 mg/m² is an independent risk factor for MACE (HR 1.377, 95% CI 1.017–1.863, p=0.038). FBG was an independent risk factor for MACE in both the MS group and the non-MS group (HR 1.414, 95% CI 1.084–1.201, p<0.001; HR 1.165, 95% CI 1.092–1.204, p<0.001), while other components of MS did not show an increased risk for MACE (Figure 3).
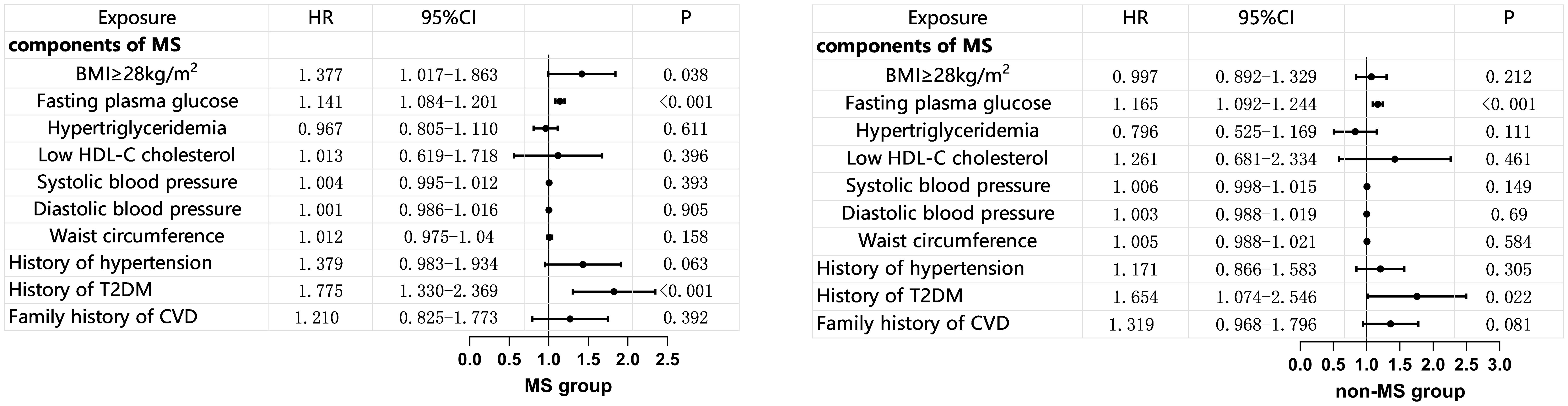
Figure 3. Multivariate COX Regression Analysis of MS Components and MACE (adjusted for age, gender, and smoking status). BMI, body mass index; HDL-C, high density lipoprotein-C; T2DM, Type 2 Diabetes Mellitus; CVD, cardiovascular disease; MS, metabolic syndrome.
Multivariate COX regression analysis of four common MS components with MACE showed that ABD+BP+GLU had a higher predictive value for MACE, with statistical significance compared to the control group (HR 3.001, 95% CI 1.640-5.492, p<0.001) (Table 6).
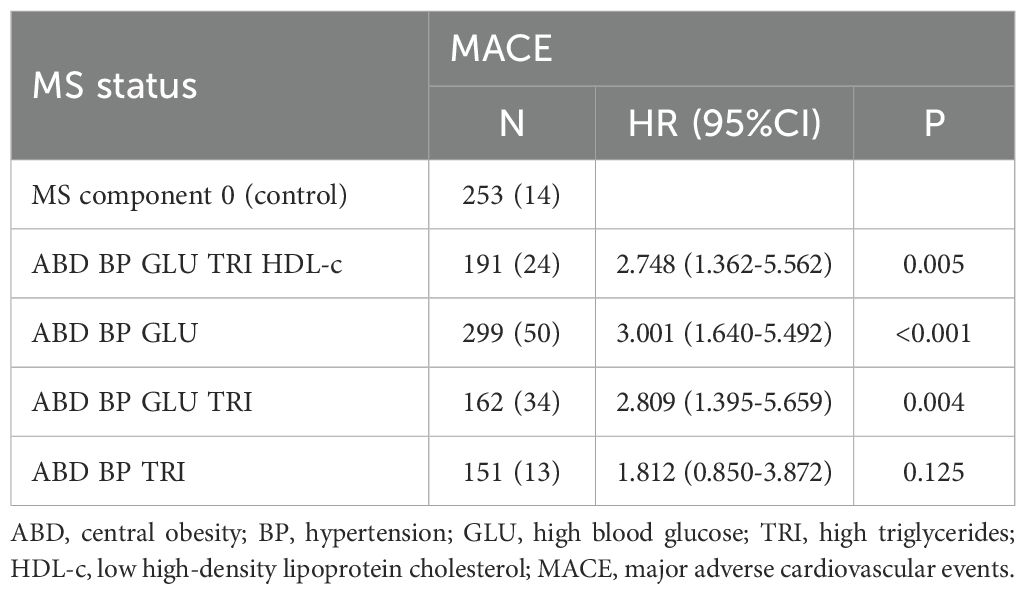
Table 6. COX regression analysis of different metabolic syndrome states and MACE (adjusted for age, gender, and smoking history).
4 Discussion
The present study aimed to explore the clinical characteristics and the relationship between MS and MACE in an elderly population from the Northern Shanghai Study, a prospective cohort that followed 3,352 subjects over an average of 5.6 years. The findings from this cohort indicate that MS significantly increases the risk of MACE, including non-fatal MI, non-fatal stroke, cardiovascular death, and all-cause mortality, confirming MS as a critical determinant of cardiovascular health in elderly individuals.
The analysis revealed that subjects with MS had notably higher waist circumference, hip circumference, waist-to-hip ratio, diastolic BP, TG, HDL-c, and FBG levels compared to their non-MS counterparts. This is consistent with previous studies that associate MS with metabolic abnormalities, further emphasizing that MS represents a cluster of conditions driving cardiovascular risk. Additionally, the prevalence of hypertension, stroke, MI, and a family history of hypertension were significantly higher in the MS group, further highlighting the burden of MS on cardiovascular health. Interestingly, the non-MS group had a higher proportion of smokers, which might indicate that factors other than smoking contribute significantly to the cardiovascular risk in the MS group.
Our findings emphasize that central obesity is a crucial component of MS among the elderly population. Specifically, four common combinations of MS components centered around central obesity were identified: ABD+BP+GLU, ABD+BP+GLU+TRI+HDL-c, ABD+BP+GLU+TRI, ABD+BP+TRI. These combinations accounted for 54% of the total MS cases. Previous research supports our findings regarding the prevalence and impact of central obesity (25–27). The Amsterdam Longitudinal Study on Aging (LASA) demonstrated that over a three-year follow-up period, BMI served as an independent predictor of metabolic syndrome in community-dwelling adults aged 55-85 years (28, 29). Additionally, Chu-Sheng Lin’s retrospective cohort study utilizing the New Taipei City Elderly Health Examination Database (NTCHD) from 2014 to 2016 observed an increase in the prevalence of metabolic syndrome and central obesity, alongside a decrease in BMI after a two-year follow-up period (30). Thus, aligns with previous studies, our findings also show that central obesity is a predominant feature of metabolic syndrome in the elderly population. However, while central obesity and hypertension were highlighted as key drivers of cardiovascular risk in our study, the role of dyslipidemia, particularly low HDL-c and elevated triglycerides, warrants further exploration. Although hypertriglyceridemia was not identified as an independent risk factor for MACE in our cohort, its interplay with other MS components, such as central obesity and insulin resistance, may still contribute to cardiovascular risk. For example, low HDL-c levels, which are a hallmark of dyslipidemia in MS, have been linked to oxidative stress and inflammation, as evidenced by the negative correlation between total antioxidant status and HDL-c in elderly individuals with MS (31). This suggests that oxidative stress may mediate the relationship between dyslipidemia and cardiovascular risk. In our study, HDL-c levels were significantly lower in the MS group compared to the non-MS group, consistent with the metabolic abnormalities associated with MS. Future studies should investigate the mechanisms underlying these associations, including the role of oxidative stress and inflammatory markers, to better understand their implications for cardiovascular risk.
The data from our cohort shows that the incidence of MACE was higher in individuals with MS compared to those without MS. Specifically, the MS group exhibited higher rates of non-fatal MI, non-fatal stroke, and overall mortality. These results are consistent with previous research demonstrating that individuals with MS are at a significantly higher risk of experiencing cardiovascular events. For example, a meta-analysis by Kazlauskiene et al. found that individuals with MS had a twofold increased risk of cardiovascular events, such as MI and stroke, compared to individuals without MS (32). Notably, non-fatal MI emerged as a major outcome, with individuals with MS facing a threefold increased risk. This aligns with prior studies that have demonstrated a strong link between MS and coronary artery disease (33–35). In a study by Sedaghat et al. MS is associated with a higher risk of MI, particularly in individuals with excess body weight. A meta-analysis found that individuals with obesity and MS had a HR of 1.68 for MI, indicating a significant positive association (36). The elevated risk of non-fatal MI in our study suggests that the combination of metabolic abnormalities in MS, particularly central obesity and dyslipidemia, may contribute to the development of atherosclerosis and coronary artery disease, ultimately leading to myocardial infarction. Similarly, the increased risk of non-fatal stroke in our study population supports previous research linking MS to cerebrovascular events (37–39). A meta-analysis of prospective cohort studies conducted by Li et al. found that individuals with MS have a 70% higher risk of incident stroke compared to those without MS, with RR of 1.70 (40). Our study found a similar relationship, with MS significantly increasing the likelihood of non-fatal stroke. This relationship may be driven by the hypertensive and atherogenic effects of MS components, such as elevated blood pressure and dyslipidemia, which are well-known risk factors for stroke. The increased risk of stroke in individuals with MS highlights the importance of managing these metabolic abnormalities to prevent cerebrovascular events, particularly in elderly individuals who are more vulnerable to stroke.
Interestingly, while cardiovascular death did not reach statistical significance between the MS and non-MS groups in our study, the trend toward higher cardiovascular mortality in the MS group is consistent with prior research. Several studies have reported a modest but significant association between MS and cardiovascular death (14, 41, 42). For instance, a study by Dekker et al. reported that MS increases the risk of cardiovascular death by 2-fold (43). The lack of statistical significance in our study may be due to the relatively small number of cardiovascular deaths during the follow-up period, but the observed trend is in line with the broader literature. One of the more striking outcomes in our study was the significantly higher all-cause mortality rate in individuals with MS compared to those without. This aligns with the results of previous studies, such as a study involving a large cohort from the LIPIDOGRAM studies found that MS significantly increased mortality risk in both obese and non-obese individuals, with HR ranging from 1.7 to 2.11 depending on the criteria used for MS diagnosis (44, 45). The elevated mortality risk in individuals with MS can be attributed to the combined effect of multiple metabolic abnormalities, which contribute not only to cardiovascular diseases but also to other chronic conditions, such as type 2 diabetes and cancer, further increasing overall mortality.
The logistic regression analysis identified MS as a significant predictor of various MACEs, including non-fatal MI, non-fatal stroke, and all-cause mortality. These associations persisted even after adjusting for potential confounders such as age, gender, smoking history, and family history of hypertension and early-onset CVD. Further supporting these findings, the Cox regression analysis indicated that MS is an independent risk factor for MACE, primarily driven by an increased risk of all-cause mortality, non-fatal stroke, and non-fatal MI. Notably, within the MS group, BMI of ≥28 mg/m² emerged as an independent risk factor for MACE, underscoring the significant role of obesity in cardiovascular risk. The association between higher BMI and increased cardiovascular risk observed in our study is supported by previous research, including the INTERHEART study, which identified abdominal obesity as a key contributor to MI risk (46, 47). Our findings emphasize the importance of weight management in elderly individuals with MS to reduce cardiovascular risk. In contrast, among those without metabolic syndrome, a BMI of ≥28 mg/m² did not predict the risk of MACE effectively. This discrepancy may be attributed to the lower average BMI of the non-MS group in this study (mean BMI of 23.3 mg/m²). Therefore, when considering whether BMI can predict MACE, the characteristics of the population should be considered. Another set of data from this study showed that participants with a high middle-upper arm circumference (MUAC) were associated with higher body weight, BMI, WC, and WHR, indicating more obesity and central obesity. However, among participants with a high MUAC, there was a cardiovascular risk benefit (48). This phenomenon might be related to the pattern of fat accumulation, which warrants further investigation. Additionally, among the individual components of MS, FBG was found to be an independent risk factor for MACE in both the MS and non-MS groups. This finding is consistent with numerous studies showing that hyperglycemia, even in the prediabetic range, is strongly associated with increased cardiovascular risk (49, 50). The role of hyperglycemia in promoting endothelial dysfunction, inflammation, and atherosclerosis likely explains its contribution to cardiovascular events in our cohort, underscoring the critical impact of glycemic control on cardiovascular outcomes.
The multivariate Cox regression analysis of common MS components revealed that the combination of central obesity, hypertension, and high blood glucose (ABD+BP+GLU) had a higher predictive value for MACE. This combination significantly increased the risk of MACE compared to the control group, highlighting the importance of targeting these specific components in the management of MS and underscores the necessity of targeting central obesity and its associated metabolic abnormalities in the prevention and management of cardiovascular risk among elderly individuals with MS. Interestingly, hypertriglyceridemia was not identified as a risk factor for cardiovascular risk; in fact, higher triglyceride levels in the non-MS group appeared to be a protective factor for cardiovascular health. There has been ongoing debate about whether lowering triglycerides can provide cardiovascular benefits (51, 52). Some research has confirmed that triglycerides are an independent risk factor for cardiovascular disease (53–55). However, the results of the PROMINENT study (a multicenter, double-blind, randomized controlled trial) released at the 2022 American Heart Association (AHA) annual meeting were similar to our findings. This study showed that patients with mild to moderate hypertriglyceridemia who received fibrate therapy did not have a lower incidence of cardiovascular events compared to the placebo group (56). This phenomenon may be related to the increased efficiency of converting triglyceride-rich lipoprotein remnants into low-density lipoprotein rather than an increase in clearance efficiency by the liver. This suggests that the relationship between triglycerides and cardiovascular risk may be more complex than previously understood, potentially influenced by factors like lipid metabolism efficiency and further research is needed to elucidate the mechanisms involved.
This study has several limitations that should be acknowledged. First, the cohort was composed of elderly individuals from a specific community in northern Shanghai, which may limit the generalizability of the findings to other geographic regions, ethnic groups, or younger populations. The unique demographic, cultural, and environmental characteristics of this population may not be representative of other settings, potentially influencing the applicability of the results. Second, the absence of repeated measurements of anthropometric and biological parameters throughout the follow-up period restricts our ability to capture changes in participants’ health status that could impact cardiovascular outcomes. For example, fluctuations in blood pressure, lipid levels, or glucose metabolism over time may contribute to cardiovascular risk, but these dynamics could not be assessed due to the lack of longitudinal data. Future studies should aim to include more diverse populations and incorporate repeated measurements at regular intervals to better understand the temporal relationships between risk factors and cardiovascular outcomes. Third, although our analysis adjusted for several confounders, such as age, gender, and smoking history, unmeasured variables like lifestyle factors (e.g., physical activity, dietary habits) and medication use (e.g., statins, antihypertensives) may influence the results. While some baseline data on the use of antihypertensive, lipid-lowering, and glucose-lowering agents were available, we lacked comprehensive information on changes in medication regimens, adherence, or dosages throughout the follow-up period. These pharmacological interventions can significantly impact metabolic parameters and cardiovascular outcomes, potentially modifying the relationship between metabolic syndrome and MACE. The absence of detailed, longitudinal data on these variables may have introduced residual confounding. Future studies should aim to incorporate more granular tracking of lifestyle and medication-related factors to enhance the accuracy and interpretability of findings. Fourth, the use of self-reported data for MACE may introduce recall bias, as participants may inaccurately remember or report events such as non-fatal MI or stroke. Although we cross-verified self-reported events with medical records whenever possible, the potential for misclassification remains. Fifth, the relatively small number of cardiovascular deaths in our cohort may limit the robustness of some conclusions, particularly regarding the association between MS and cardiovascular mortality. While the trend toward higher cardiovascular mortality in the MS group aligns with prior research, the lack of statistical significance may reflect insufficient power due to the low event rate. Finally, while our study highlights the individual contributions of MS components to cardiovascular outcomes, it does not fully explore the potential interactions and synergies among these components, such as how central obesity, insulin resistance, hypertension, and dyslipidemia may collectively amplify cardiovascular risk. Future studies should aim to include more diverse populations, incorporate repeated measurements, investigate interactions among MS components, and use more objective measures, such as hospital records or adjudicated endpoints, to reduce bias and improve the robustness of the findings. Despite these limitations, our study provides valuable insights into the cardiovascular health of elderly individuals in northern Shanghai, using standardized and rigorous laboratory methods to ensure the reliability of the findings.
In conclusion, this study confirms the strong association between MS and MACE in the elderly community of northern Shanghai. Our findings highlight that MS, particularly the combination of central obesity, hypertension, and high blood glucose, plays a pivotal role in predicting cardiovascular outcomes, including non-fatal MI, non-fatal stroke, and all-cause mortality. Given the increasing prevalence of MS in aging populations, these results underscore the urgency of targeted interventions to mitigate cardiovascular risk in elderly individuals.
Future research should focus on elucidating the mechanisms linking MS components to cardiovascular outcomes, particularly the role of oxidative stress, inflammation, and metabolic dysregulation. Longitudinal studies with repeated biochemical and anthropometric measurements are necessary to assess the temporal progression of MS and its impact on cardiovascular health. Additionally, exploring the effects of lifestyle modifications, such as diet and physical activity, as well as pharmacological interventions in MS management, will provide valuable insights into potential strategies for reducing MACE risk.
From a clinical perspective, our findings emphasize the need for early identification and comprehensive management of MS components, particularly central obesity and dysglycemia, to improve cardiovascular outcomes in elderly populations. Integrating routine MS screening into primary care settings, alongside personalized lifestyle and medical interventions, may help prevent the development of severe cardiovascular complications. Future studies should also evaluate the efficacy of targeted prevention programs in high-risk populations to optimize cardiovascular risk management in aging communities.
Data availability statement
The raw data supporting the conclusions of this article will be made available by the authors, without undue reservation.
Ethics statement
This study was conducted in compliance with the principles of the Helsinki Declaration and received approval from the ethical review board of Shanghai Tenth People’s Hospital. Written informed consent was obtained from each participant involved in the study.
Author contributions
SK: Writing – original draft. FZ: Writing – review & editing. YX: Writing – review & editing. YZ: Writing – review & editing. CZ: Writing – review & editing. IS: Writing – review & editing. SS: Writing – original draft, Writing – review & editing. YF: Writing – original draft, Writing – review & editing.
Funding
The author(s) declare that financial support was received for the research and/or publication of this article. This study was partially supported by the funds of Municipal Public Welfare Self-Financing Technology Application Research Project of Lishui (2022SJZC074&2022SJZC079) and Post-Doctoral Research Start-Up Fund of Lishui People’s Hospital (2024bsh001).
Acknowledgments
We are deeply grateful to all the members who contributed to this work.
Conflict of interest
The authors declare that the research was conducted in the absence of any commercial or financial relationships that could be construed as a potential conflict of interest.
Generative AI statement
The author(s) declare that no Generative AI was used in the creation of this manuscript.
Publisher’s note
All claims expressed in this article are solely those of the authors and do not necessarily represent those of their affiliated organizations, or those of the publisher, the editors and the reviewers. Any product that may be evaluated in this article, or claim that may be made by its manufacturer, is not guaranteed or endorsed by the publisher.
Supplementary material
The Supplementary Material for this article can be found online at: https://www.frontiersin.org/articles/10.3389/fendo.2025.1570191/full#supplementary-material
Abbreviations
CVD, Cardiovascular disease; MS, Metabolic syndrome; MACE, Major adverse cardiovascular events; NSS, Northern Shanghai Study; MI, Myocardial infarction; NCEP, National Cholesterol Education Program; BMI, Body Mass Index; WC, Waist Circumference; BP, Blood Pressure; FPG, Fasting plasma glucose; TG, Triglycerides; HDL-c, High-density lipoprotein cholesterol; LDL-c, Low-density lipoprotein cholesterol; OR, odds ratio; CI, confidence interval; HR, hazard ratio; RR, relative risk; WHR, waist-hip ratio; MUAC, High middle-upper arm circumference; AHA, American Heart Association.
References
1. Roth GA, Mensah GA, Johnson CO, Addolorato G, Ammirati E, Baddour LM, et al. Global burden of cardiovascular diseases and risk factors, 1990-2019: update from the GBD 2019 study. J Am Coll Cardiol. (2020) 76(25):2982–3021.
2. McAloon CJ, Osman F, Glennon P, Lim PB, and Hayat SA. Global epidemiology and incidence of cardiovascular disease. Cardiovasc Dis: Genet Susceptibility Environ Factors Their Interaction. (2016) p:57–96. Elsevier Inc. doi: 10.1016/B978-0-12-803312-8.00004-5
3. Benjamin EJ, Muntner P, Alonso A, Bittencourt MS, Callaway CW, Carson AP, et al. Heart disease and stroke statistics-2019 update: A report from the american heart association. Circulation. (2019) 139:e56–528. doi: 10.1161/CIR.0000000000000659
4. Li Z, Lin L, Wu H, Yan L, Wang H, Yang H, et al. Global, regional, and national death, and disability-adjusted life-years (DALYs) for cardiovascular disease in 2017 and trends and risk analysis from 1990 to 2017 using the global burden of disease study and implications for prevention. Front Public Health. (2021) 9. doi: 10.3389/fpubh.2021.559751
5. Neumann JT, Thao LTP, Callander E, Chowdhury E, Williamson JD, Nelson MR, et al. Cardiovascular risk prediction in healthy older people. Geroscience. (2022) 44:403–13. doi: 10.1007/s11357-021-00486-z
6. Bosco E, Hsueh L, McConeghy KW, Gravenstein S, and Saade E. Major adverse cardiovascular event definitions used in observational analysis of administrative databases: a systematic review. BMC Med Res Methodol. (2021) 21(1). doi: 10.1186/s12874-021-01440-5
7. Alberti KGMM, Zimmet P, and Shaw J. Metabolic syndrome - A new world-wide definition. A consensus statement from the International Diabetes Federation. Diabetic Med. (2006) p:469–80.
8. Jamali Z, Ayoobi F, Jalali Z, Bidaki R, Lotfi MA, Esmaeili-Nadimi A, et al. Metabolic syndrome: a population-based study of prevalence and risk factors. Sci Rep. (2024) 14:1–11. doi: 10.1038/s41598-024-54367-4
9. Galassi A, Reynolds K, and He J. Metabolic syndrome and risk of cardiovascular disease: A meta-analysis. Am J Med. (2006) 119:812–9. doi: 10.1016/j.amjmed.2006.02.031
10. Mottillo S, Filion KB, Genest J, Joseph L, Pilote L, Poirier P, et al. The metabolic syndrome and cardiovascular risk: A systematic review and meta-analysis. J Am Coll Cardiol. (2010) 56:1113–32. doi: 10.1016/j.jacc.2010.05.034
11. Earl. Risks for all-cause mortality, cardiovascular disease, and diabetes. Diabetes Care. (2005) 28(7).
12. Kokubo Y, Okamura T, Yoshimasa Y, Miyamoto Y, Kawanishi K, Kotani Y, et al. Impact of metabolic syndrome components on the incidence of cardiovascular disease in a general urban Japanese population: The suita study. Hypertension Res. (2008) 31:2027–35. doi: 10.1291/hypres.31.2027
13. Gami AS, Witt BJ, Howard DE, Erwin PJ, Gami LA, Somers VK, et al. Metabolic syndrome and risk of incident cardiovascular events and death. A systematic review and meta-analysis of longitudinal studies. J Am Coll Cardiol. (2007) 49:403–14. doi: 10.1016/j.jacc.2006.09.032
14. Lakka HM, Laaksonen DE, Lakka TA, Niskanen LK, Kumpusalo E, Tuomilehto J, et al. The metabolic syndrome and total and cardiovascular disease mortality in middle-aged men. J Am Med Assoc. (2002) 288:2709–16. doi: 10.1001/jama.288.21.2709
15. Simmons RK, Alberti KGMM, Gale EAM, Colagiuri S, Tuomilehto J, Qiao Q, et al. The metabolic syndrome: Useful concept or clinical tool? Report of a WHO expert consultation. Diabetologia. (2010) 53:600–5. doi: 10.1007/s00125-009-1620-4
16. Zhao LH, Liu Y, Xiao JY, Wang JX, Li XW, Cui Z, et al. Prognostic value of metabolic syndrome in patients with non-ST elevated myocardial infarction undergoing percutaneous coronary intervention. Front Cardiovasc Med. (2022) 9. doi: 10.3389/fcvm.2022.912999
17. Hosseini K, Khalaji A, Behnoush AH, Soleimani H, Mehrban S, Amirsardari Z, et al. The association between metabolic syndrome and major adverse cardiac and cerebrovascular events in patients with acute coronary syndrome undergoing percutaneous coronary intervention. Sci Rep. (2024) 14(1). doi: 10.1038/s41598-024-51157-w
18. Kammerlander AA, Mayrhofer T, Ferencik M, Pagidipati NJ, Karady J, Ginsburg GS, et al. Association of metabolic phenotypes with coronary artery disease and cardiovascular events in patients with stable chest pain. Diabetes Care. (2021) 44:1038–45. doi: 10.2337/dc20-1760
19. Park S, Lee S, Kim Y, Lee Y, Kang MW, Han K, et al. Altered risk for cardiovascular events with changes in the metabolic syndrome status a nationwide population-based study of approximately 10 million persons. Ann Intern Med. (2019) 171:875–84. doi: 10.7326/M19-0563
20. Huang PL. A comprehensive definition for metabolic syndrome. DMM Dis Models Mechanisms. (2009) 2:231–7. doi: 10.1242/dmm.001180
21. Haam JH, Kim BT, Kim EM, Kwon H, Kang JH, Park JH, et al. Diagnosis of obesity: 2022 update of clinical practice guidelines for obesity by the korean society for the study of obesity. J Obes Metab Syndr. (2023) p:121–9. doi: 10.7570/jomes23031
22. Tondt J, Freshwater M, Christensen S, Iliakova M, Weaver E, Benson-Davies S, et al. Obesity Algorithm eBook. Obesity Medicine Association (2023).
23. Organization WH. (2011). Waist Circumference and Waist-hip Ratio: Report of a WHO Expert Consultation, 8-11 December 2008. Geneva: World Health Organization (2011).
24. Grundy SM, Cleeman JI, Daniels SR, Donato KA, Eckel RH, Franklin BA, et al. Diagnosis and management of the metabolic syndrome: An American Heart Association/National Heart, Lung, and Blood Institute scientific statement. Circulation. (2005) p:2735–52. doi: 10.1161/CIRCULATIONAHA.105.169404
25. Engin AB and Engin A. Obesity and lipotoxicity. Cham: Springer (2017) 960. doi: 10.1007/978-3-319-48382-5
26. Han TS and Lean ME. A clinical perspective of obesity, metabolic syndrome and cardiovascular disease. JRSM Cardiovasc Dis. (2016) 5:204800401663337. doi: 10.1177/2048004016633371
27. Mata AJ and Jasul G. Prevalence of metabolic syndrome and its individual features across different (Normal, overweight, pre-obese and obese) body mass index (BMI) categories in a tertiary hospital in the Philippines. J ASEAN Fed Endocr Soc. (2017) 32:117–22. doi: 10.15605/jafes.032.02.04
28. Mbbs RS, Rn AN, Mbbs PK, and Mbbs AT. Influence of BMI on short and long-term outcomes in patients with STEMI and LV dysfunction. Heart Lung Circ. (2019), 1–7.
29. Ancum JMV, Jonkman NH, Schoor NMV, Tressel E, Meskers GM, Pijnappels M, et al. Predictors of metabolic syndrome in community-dwelling older adults. PloS One. (2018) 10:1–12, 13. doi: 10.1371/journal.pone.0206424
30. Lin C, Lee W, Lin S, Lin H, Chen R, Lin C, et al. Subtypes of premorbid metabolic syndrome and associated clinical outcomes in older adults. Front Med. (2022) 8:698728. doi: 10.3389/fmed.2021.698728
31. Dzięgielewska-Gęsiak S, Wyszomirska K, Fatyga E, Wysocka E, and Muc-Wierzgoń M. The role of oxidant-antioxidant markers and resistin in metabolic syndrome elderly individuals. Sci Prog. (2021) 104(2). doi: 10.1177/00368504211006510
32. Kazlauskiene L, Butnoriene Jr, and Norkus A. Metabolic syndrome related to cardiovascular events in a 10-year prospective study. Diabetol Metab Syndr. (2015) 7(1). doi: 10.1186/s13098-015-0096-2
33. Alshammary AF, Alharbi KK, Alshehri NJ, Vennu V, and Khan IA. Metabolic syndrome and coronary artery disease risk: A meta-analysis of observational studies. Int J Environ Res Public Health. (2021) 18:1–17. doi: 10.3390/ijerph18041773
34. Mohammad J, Sarbast S, and Ahmed M. The prevalence of metabolic syndrome in coronary artery disease and the association of metabolic syndrome with severity of coronary artery disease. J Univ Duhok. (2022) 25:81–7. doi: 10.26682/sjuod.2022.25.1.11
35. Montazerifar F, Bolouri A, Mozaffar MM, and Karajibani M. The prevalence of metabolic syndrome in coronary artery disease patients. Cardiol Res. (2016) 7:202–8. doi: 10.14740/cr507w
36. Sedaghat Z, Khodakarim S, Nejadghaderi SA, and Sabour S. Association between metabolic syndrome and myocardial infarction among patients with excess body weight: a systematic review and meta-analysis. BMC Public Health. (2024) 24(1). doi: 10.1186/s12889-024-17707-7
37. Boden-Albala B, Sacco RL, Lee HS, Grahame-Clarke C, Rundek T, Elkind MV, et al. Metabolic syndrome and ischemic stroke risk: Northern Manhattan Study. Stroke. (2008) 39:30–5. doi: 10.1161/STROKEAHA.107.496588
38. Liang Y, Yan Z, Hao Y, Wang Q, Zhang Z, She R, et al. Metabolic syndrome in patients with first-ever ischemic stroke: prevalence and association with coronary heart disease. Sci Rep. (2022) 12(1). doi: 10.1038/s41598-022-17369-8
39. Moghadam-Ahmadi A, Soltani N, Ayoobi F, Jamali Z, Sadeghi T, Jalali N, et al. Association between metabolic syndrome and stroke: a population based cohort study. BMC Endocr Disord. (2023) 23(1). doi: 10.1186/s12902-023-01383-6
40. Li X, Li X, Lin H, Fu X, Lin W, Li M, et al. Metabolic syndrome and stroke: A meta-analysis of prospective cohort studies. J Clin Neurosci Churchill Livingstone. (2017) p:34–8. doi: 10.1016/j.jocn.2017.01.018
41. Li W, Chen D, Peng Y, Lu Z, Kwan MP, and Tse LA. Association between metabolic syndrome and mortality: prospective cohort study. JMIR Public Health Surveill. (2023) 9(1). doi: 10.2196/44073
42. Andreadis EA, Tsourous GI, Tzavara CK, Georgiopoulos DX, Katsanou PM, Marakomichelakis GE, et al. Metabolic syndrome and incident cardiovascular morbidity and mortality in a mediterranean hypertensive population. Am J Hypertens. (2007) 20:558–64. doi: 10.1016/j.amjhyper.2006.12.001
43. Dekker JM, Girman C, Rhodes T, Nijpels G, Stehouwer CDA, Bouter LM, et al. Metabolic syndrome and 10-year cardiovascular disease risk in the Hoorn Study. Circulation. (2005) 112:666–73. doi: 10.1161/CIRCULATIONAHA.104.516948
44. Osadnik K, Osadnik T, Gierlotka M, Windak A, Tomasik T, Mastej M, et al. Metabolic syndrome is associated with similar long-term prognosis in those living with and without obesity: an analysis of 45 615 patients from the nationwide LIPIDOGRAM 2004-2015 studies. Eur J Prev Cardiol. (2023) 30:1195–204. doi: 10.1093/eurjpc/zwad101
45. De Geest B and Mishra M. The metabolic syndrome in obese and non-obese subjects: a reappraisal of the syndrome X of Reaven. Eur J Prev Cardiol. (2023) p:1193–4. Oxford University Press. doi: 10.1093/eurjpc/zwad144
46. Teo KK, Liu L, Chow CK, Wang X, Islam S, Jiang L, et al. Potentially modifiable risk factors associated with myocardial infarction in China: The INTERHEART China study. Heart. (2009) 95:1857–64. doi: 10.1136/hrt.2008.155796
47. Yusuf PS, Hawken S, Ôunpuu S, Dans T, Avezum A, Lanas F, et al. Effect of potentially modifiable risk factors associated with myocardial infarction in 52 countries (the INTERHEART study): Case-control study. Lancet. (2004) 364:937–52. doi: 10.1016/S0140-6736(04)17018-9
48. Zheng Y, Zhang J, Ren Z, Meng W, Tang J, Zhao S, et al. Prognostic value of arm circumference for cardiac damage and major adverse cardiovascular events: A friend or a foe? A 2-year follow-up in the northern shanghai study. Front Cardiovasc Med. (2022) 9. doi: 10.3389/fcvm.2022.816011
49. Wei W, Liang Y, Guo D, Xu X, Xu Q, Li S, et al. Hyperglycemia newly detected by glycated hemoglobin affects all-cause mortality in coronary artery disease patients: a retrospective cohort study. Diabetes Res Clin Pract. (2022) 191. doi: 10.1016/j.diabres.2022.110053
50. Meshref TS, Ashry MA, El-Aal RFA, Imam HM, and Hamad DA. Unique role of admission hyperglycemia on myocardial infarction size and area at risk following an acute ST-elevation myocardial infarction. Egypt J Intern Med. (2020) 32(1). doi: 10.1186/s43162-020-00015-y
51. Reiner Ž. Hypertriglyceridaemia and risk of coronary artery disease. Nat Rev Cardiol. (2017) 14:401–11. doi: 10.1038/nrcardio.2017.31
52. Miller M, Stone NJ, Ballantyne C, Bittner V, Criqui MH, Ginsberg HN, et al. Triglycerides and cardiovascular disease: A scientific statement from the American Heart Association. Circulation. (2011) 123:2292–333. doi: 10.1161/CIR.0b013e3182160726
53. Toth PP. Triglyceride-rich lipoproteins as a causal factor for cardiovascular disease. Vasc Health Risk Manage. (2016) 12:171–83. doi: 10.2147/VHRM.S104369
54. Patel A. Serum triglycerides as a risk factor for cardiovascular diseases in the Asia-Pacific region. Circulation. (2004) 110:2678–86. doi: 10.1161/01.CIR.0000145615.33955.83
55. Koo BK, Park S, Han KD, and Moon MK. Hypertriglyceridemia is an independent risk factor for cardiovascular diseases in Korean adults aged 30–49 years: A nationwide population-based study. J Lipid Atheroscler. (2021) 10:88–98. doi: 10.12997/jla.2021.10.1.88
Keywords: metabolic syndrome, cardiovascular disease, elderly, major adverse cardiovascular events, mortality
Citation: Kumar Karn S, Zhao F, Xu Y, Zhang Y, Zeng C, Shaikh II, Singh S and Feng Y (2025) Metabolic syndrome with mortality and major adverse cardiovascular events in an elderly population. Front. Endocrinol. 16:1570191. doi: 10.3389/fendo.2025.1570191
Received: 03 February 2025; Accepted: 28 April 2025;
Published: 22 May 2025.
Edited by:
Carsten Grötzinger, Charité University Medicine Berlin, GermanyReviewed by:
Sylwia Dziegielewska-Gesiak, Medical University of Silesia, PolandHiroya Ohta, Hokkaido University of Science, Japan
Hitesh Singh Chaouhan, National Institute of Neurological Disorders and Stroke (NIH), United States
Copyright © 2025 Kumar Karn, Zhao, Xu, Zhang, Zeng, Shaikh, Singh and Feng. This is an open-access article distributed under the terms of the Creative Commons Attribution License (CC BY). The use, distribution or reproduction in other forums is permitted, provided the original author(s) and the copyright owner(s) are credited and that the original publication in this journal is cited, in accordance with accepted academic practice. No use, distribution or reproduction is permitted which does not comply with these terms.
*Correspondence: Imran Ibrahim Shaikh, c2hhaWtoaW1yYW44OEB3bXUuZWR1LmNu; Shekhar Singh, ZHJzaGVraGFyc2luZ2hAaWNsb3VkLmNvbQ==; Yuling Feng, eXVsaW5nZmVuZy5kckBob3RtYWlsLmNvbQ==