- Vaccine Research Center, National Institute of Allergy and Infectious Diseases, NIH, Bethesda, MD, United States
The evolution of antibodies in an individual during an immune response by somatic hypermutation (SHM) is essential for the ability of the immune system to recognize and remove the diverse spectrum of antigens that may be encountered. These mutations are not produced at random; nucleotide motifs that result in increased or decreased rates of mutation were first reported in 1992. Newer models that estimate the propensity for mutation for every possible 5- or 7-nucleotide motif have emphasized the complexity of SHM targeting and suggested possible new hot spot motifs. Even with these fine-grained approaches, however, non-local context matters, and the mutations observed at a specific nucleotide motif varies between species and even by locus, gene segment, and position along the gene segment within a single species. An alternative method has been provided to further abstract away the molecular mechanisms underpinning SHM, prompted by evidence that certain stereotypical amino acid substitutions are favored at each position of a particular V gene. These “substitution profiles,” whether obtained from a single B cell lineage or an entire repertoire, offer a simplified approach to predict which substitutions will be well-tolerated and which will be disfavored, without the need to consider path-dependent effects from neighboring positions. However, this comes at the cost of merging the effects of two distinct biological processes, the generation of mutations, and the selection acting on those mutations. Since selection is contingent on the particular antigens an individual has been exposed to, this suggests that SHM may have evolved to prefer mutations that are most likely to be useful against pathogens that have co-evolved with us. Alternatively, the ability to select favorable mutations may be strongly limited by the biases of SHM targeting. In either scenario, the sequence space explored by SHM is significantly limited and this consequently has profound implications for the rational design of vaccine strategies.
Introduction
In order to combat an arbitrarily large number of unknown pathogens, the humoral immune system relies on three mechanisms to generate diversity in antibody variable domains. In the primary repertoire, combinatorial diversity is created by the random joining of germline-encoded V, D, and J heavy chain or V and J light chain gene segments. During this process, junctional diversity is also introduced through the action of exonucleases and terminal deoxynucleotidyl transferase. This results in an estimated 1015–1018 possible unique naive B cell (1, 2). Furthermore, upon encountering cognate antigen, a naive B cell can enter a germinal center and begin to undergo somatic hypermutation (SHM), increasing the number of realizable antibodies by several additional orders of magnitude. However, the total number of circulating B cells in a human is only ~109 (3, 4), meaning that if all possible antibodies were equally likely to be made, the odds of correctly producing one capable of binding to and clearing a particular antigen would be minuscule. In fact, precisely such arguments were initially used to argue against the “somatic” theory of antibody diversity predicting the existence of SHM (5). Hood and Talmage even pointed out that potential number of wasted mutations alone (i.e., those leading to non-functional antibodies and cell death) would far exceed the total number of cells thought to be produced over a human lifetime (6).
Nonetheless, the immune system has also evolved mechanisms for biasing the generation of diversity in ways, which presumably optimize the search for effective antibodies. For instance, different V gene segments are used at different frequencies (7, 8) and certain D genes may be more often recombined with specific J genes (9, 10). Many studies have shown that the parameters governing recombination vary dramatically from a uniform distribution and are generally reproducible between individuals (2, 11–14). Indeed, they appear to be optimized to produce B cells that can pass tolerance checkpoints and mature into naive B cells (2).
The SHM process is similarly biased. Soon after the first experimental confirmations of SHM (15, 16), it was quickly noted that mutations are more clustered together than random expectation (17) and fall into intrinsic hot spots (18, 19). Since the discovery of activation-induced cytidine deaminase (AID), the enzyme that initiates SHM by deaminating cytidine to uridine (20–22), much progress has been made in understanding the molecular origins of these biases. Many factors have been described that participate in targeting AID activity to the Ig loci by associating it with enhancer transcription and polymerase stalling [reviewed in Ref. (23–25)]. Studies of the specificity loop of AID (26–28) have elucidated the basis for the preferential deaminations of cytidines within specific microsequence motifs. Finally, investigations of uracil-DNA glycosylase, MutSα, DNA polymerase η, and many other components of the base excision and mismatch repair pathways have revealed some of the mechanisms behind patterns of mutations other than the C→T transitions generated directly by AID [reviewed in Ref. (25, 29, 30)].
The study of AID and other molecular components of the SHM machinery has always been complemented and even driven by computational approaches. For instance, the two-phase model of SHM (deamination by AID, followed by removal of the resulting uracil and error-prone repair) was first proposed in response to the observation that SHM is more focused on RGYW (where R is A or G; Y is C or T; and W is A or T) hot spots in MSH2-deficient mice (31). Similarly, the role of DNA polymerase η was deduced in part by comparing the motifs mutated by that enzyme to the WA hot spot motifs observed in SHM (32).
In addition, computational analysis can be clarifying, abstracting away molecular details to reveal higher level patterns such as the canonical RGYW hot spot motif itself. Recent work has suggested that the repertoire of nucleotide mutations generated by SHM can be further abstracted to amino acid substitution profiles (33, 34). These profiles point toward a new, simpler avenue for predictive analyses of the immune system, such as understanding potential responses to a specific vaccine immunogen. Here, we review the history, use, and limitations of microsequence motifs for predicting the targeting of SHM; the evidence that evolution has focused the SHM machinery toward producing specific types of amino acid changes at specific positions; the emerging use of substitution profiles and other similar predictive frameworks (FWR) for amino acid usages, along with their potential challenges and limitations; and how substitution profiles might find use in rational vaccine design.
Microsequence Motifs
The idea that the diversity of antibody specificities could be attributed to ongoing accumulation of genetic mutations in proliferating lymphocytes was first proposed by Lederberg (35). Brenner and Milstein then suggested a mechanism based on DNA cleavage targeted to specific genetic loci, followed by exonuclease activity and error-prone repair (36). After the emergence of experimental support for this hypothesis (17, 37), analogy to the action of known mutagenic agents led Rogozin and Kolchanov to examine the possible influence of neighboring bases on the occurrences of mutations in antibodies. This resulted in the discovery of the now-canonical RGYW/WRCY hot spot motif (where the underline indicates the mutated base), as well as the apparently equally mutable TAA motif (38). Later, a disfavored cold spot motif of SYC (where S is C or G) was also reported (39).
However, despite the usefulness of the WRCY and TAA motifs, only about 30% of observed SHMs fall into such hot spots (38). Moreover, it quickly became clear that not all 8 WRCY sequences were equally “hot,” with AGCT being favored (19, 40–42) and AGCC or TGCG being disfavored (43, 44). At various times, WRCH (where H is A, C, or T) (45), WRCR (46), and WRCW (47) have been suggested as more accurate motifs, with the WRC thought to be the core motif (39, 46), with the last base possibly influencing the choice of repair pathways (45). Similarly, the originally proposed TAA motif was later refined to WA (32). In addition, other potential hot spot motifs have been suggested, such as CRCY and ATCT (48).
Another approach has been to explicitly calculate mutation rates for each possible nucleotide sequence of a given length. In the first such study, Smith estimated the relative mutability for all possible di- and trinucleotide motifs using downstream JK sequences from mouse hybridoma lines, concluding that the dinucleotides explained most of the variation in mutational targeting (40). They later extended this analysis to mouse and human heavy chains (49) and human kappa chains (50), using non-productive rearrangements instead of intronic sequences to calculate mutabilities in humans (49, 50). They found broad similarity between species and between heavy and kappa (49, 50), while a later analysis of non-productive human IgL sequences with higher mutation levels suggested substantial differences from IgH (51). Ohm-Laursen used non-productive rearrangements of VH3-23 with JH4 or JH6 to derive a quartet model and showed that the frequency of mutation at specific motifs in the D and J genes correlated well with those in the V gene (43). A different quartet model used the V gene region of all publicly available antibody sequences and modeled the effects of the flanking nucleotides as independent from the position of the mutation itself (52). These authors found a high correlation of observed quartet mutation frequencies (~0.7) between heavy and light chains and between human and mouse antibodies. However, the full model could only explain around half of the variation in mutation frequencies in the real data (52).
More recently, with the advent of high-throughput sequencing technologies, attempts have been made to build out more finely discriminatory models. Yaari constructed a 5 nucleotide motif model using only synonymous mutations from functional sequences (44). The frequency at which each motif was targeted was highly correlated between individuals (~0.9), but the correlation between expected and observed mutations was only 0.67. Moreover, 46% of possible 5-mer motifs were not observed directly and had to be estimated from other similar motifs (44). The same group also immunized mice transgenic for the B1-8 heavy chain with (4-hydroxy-3-nitrophenyl)acetyl, which produces a response heavily biased toward λ chain usage (53). They sequenced the non-productive kappa chains from these animals and confirmed that the 5-mer mutation frequencies from functional and non-functional sequences correlated well with each other (48). They also built 5-mer models for mouse heavy chains and human light chains, finding an overall correlation of only 0.63 between the species. Specifically, C:G base pairs were observed to be more likely to mutate in mice and also to have a higher probability to result in a transition substitution than in humans (48).
To overcome the limitation of motifs that do not appear in the repertoire of germline Ig sequences, Elhanati et al. constructed a 7-nucleotide position weight matrix (PWM) that treats each position independently, finding a correlation of 0.8 between predicted and observed mutations frequencies (2). A later refinement of this model also calculated 7-mer PWMs for D and J gene-derived nucleotides, finding that those differed sharply from the PWMs learned for V genes (54). Another new approach, termed “samm,” uses a proportional hazards model with a lasso penalty and a flexible motif dictionary to extract the most important features and construct motifs accordingly (55). When used to build a 5-mer motif model and compared directly to Cui et al. (48), the results are similar, but samm tended to discount the effect of the final nucleotide, inferring only 382 unique mutability values instead of 1,015 (55).
In addition to calculating the frequency of mutations at each motif, many groups have investigated the resulting mutation spectrums, or the relative rates of mutation to each possible destination nucleotide. Although a preference for transitions over transversions was first reported in the early 1990s (19, 56), Cowell and Kepler were the first to report a dependency on neighboring bases for mutations spectrums (57). They found that both nucleotides in a homodimer have an increased propensity for transitions, while AT and TA dinucleotides have a preference to mutate to AA or TT homodimers (57). Ohm-Laursen calculated mutation spectrums for all 4-nucleotide motifs (43), but did not specifically analyze the effects of context. The quartet model of Cohen calculated mutation frequencies independently for each possible destination nucleotide, finding that the particular substitution had as much impact on the variability of mutation frequencies as did the microsequence context (52). Several other groups have calculated mutation spectrums, as well (2, 44, 48, 54) though those authors all deemphasized mutation spectrums compared to mutation frequencies or general properties of the antibody repertoire. This is due to the fact that mutation spectrums are considered less computationally tractable, as the underlying molecular machinery is significantly more complex and less well understood. In addition, they have been thought to be less useful, as the observed substitutions are presumed to be heavily influenced by selection for antigen binding, which acts on the amino acid sequence. One attempt has been made to parameterize an amino acid substitution matrix for antibodies (58), which does not compare favorably to real data when used to simulate SHM (33).
Even extended to 5- and 7-nucleotide motifs, microsequence context can only account for 70–80% of variability in mutation frequencies (2, 44, 52, 54). Much of the residual variation appears to be due to positional effects within the antibody sequence. Differences between FWR and complementarity determining regions (CDR) have been reported (49, 50, 52, 59), and regional variation can be observed even in non-Ig transgenes (59). In addition, mutation frequencies for the same sequence decay exponentially with distance from the transcription start site (60). In addition, differences between the heavy, kappa, and lambda chain loci are consistently observed (48, 49, 51, 52). The complex interdependence among all of these factors suggests that an evolutionary balancing has optimized the types and distributions of mutations produced by SHM.
Evolutionary Optimization of SHM
One of the primary selective pressures driving antibody gene evolution is the need for functional diversity. Antibody genes were originally thought to be subject to “coincidental” or “concerted” evolution, as seen for other multigene families like ribosomal RNA and histone genes, with diversity generated by unequal crossing over and/or gene conversion (61, 62). However, an early study of the phylogenetic relationships between mouse and human VH genes suggested that the rate of VH gene duplication would have to be over 100-fold lower than for other multigene families (63). Later, studies with access to more sequences from more species were able to show that V gene evolution is instead governed by a “birth-and-death” process, which results in a more dynamic and diverse repertoire between species (64, 65). Within VH genes, moreover, the germline sequences of the CDRs, but not FWRs, are under diversifying selection (63, 66). In addition, SHM is itself an evolutionarily ancient diversification mechanism, preceding the emergence of combinatorial V(D)J joining and the full diversification of VH genes (67). SHM has been observed in vivo in the horn shark (67), and AID orthologs with in vitro deaminase activity have been isolated from cartilaginous fish (68) and even jawless vertebrates (69). Although all of the AID orthologs tested retained a general preference for WRC motifs over non-WRC substrates, the exact microsequence specificity varied substantially (68), suggesting co-evolution of the SHM machinery with antibody gene sequences to optimize the humoral immune response.
The interplay between evolution of the primary sequences of the germline repertoire and the biased mechanisms of SHM can also be seen in the fact that the codon composition of CDRs make them more prone to replacement mutations, while the structurally important FWRs use codons that are biased toward silent mutations (70–72). Similarly, Wagner et al. found that highly mutable AGY codons are preferentially used to encode serines in CDRs, while less mutable TCN codons tend to appear in FWRs (73). Kepler reported a general difference in codon usage between CDRs and FWRs, which was strongly correlated with differential mutability (74). Moreover, both the specific serine bias (75–77) and the general codon bias (78) appear to be phylogenetically conserved, emphasizing the importance of plasticity in the CDRs. In fact, recent work has demonstrated that AGC hot spot triplets in the CDRs are specifically conserved in the serine reading frame (79). These codons are exceptionally plastic, and mutated AGY serine codons are disproportionately involved in antigen contacts seen in crystal structures (79).
Shaping of the action of SHM extends beyond differences between CDRs and FWRs. For instance, Zheng et al. showed that C→T transitions are predominantly silent, and that those which would lead to replacement mutations are found primarily in cold spots (80). A similar, though less strict, distribution was reported for G→A transitions. Those authors speculate that this pattern might have evolved to keep mutations created directly by AID from overwhelming those caused by error-prone repair in phase II (80).
Somatic hypermutation is also targeted to be able to introduce gross structural changes to antibodies in a favorable way. For instance, mutations are frequently observed in human Vκ1-derived antibodies at two FWR positions, which affect interdomain dynamics and enhance thermostability (81). Similarly, sequences that can give rise to an NxS/T glycosylation motif with only one nucleotide change are concentrated in the antigen-proximal loops of the variable domains (82).
Evolution appears to shape the naive repertoire, as well. Recent work has demonstrated that observed biases in the usages of various V gene segments correlates with the predisposition of each gene to focus SHM toward its CDRs (72). More generally, the likelihood that the antibody encoded by an immature B cell can survive central tolerance and get selected into the naive repertoire correlates with the likelihood of that sequence being generated by the recombination machinery in the first place (2). In a similar vein, mouse antibodies have substantially less DH gene variation and junctional diversity than humans, which has been hypothesized to overcome the limitations of a numerically small B cell population by focusing the naive repertoire on the most critical specificities (83).
Even in humans, these biases allow the development of stereotyped antibodies, specific recombinations using particular genetic elements that can be reproducibly elicited by a particular antigen (12). These stereotyped antibodies can even target complex antigens such as influenza HA (84–86) and HIV Env (87, 88). In addition to stereotyped genes, the antibody response can reproducibly make use of specific amino acid substitutions generated by SHM. This had been observed in both mice (18, 89) and humans (84, 85, 90), and even when the mutation in question occurs in a cold spot of AID activity (91). Moreover, substitutions that appeared in VH1-46-derived antibodies targeting the CD4-binding site of HIV Env from multiple donors were also observed in VH1-46-derived antibodies from HIV-uninfected donors (92). This demonstrates that shared substitutions can occur in the selected functional repertoire even without a common antigen and may reflect the way that the SHM machinery has evolved to sample the mutations that are most likely to be useful.
Substitution Profiles
It seems counterintuitive that a priori predictions can be made about the state of the selected functional repertoire without reference to the antigens that have driven that selection. However, the number of unique clones in which a particular position has been substituted is correlated with the diversity of germline amino acids available at that position, in both CDRs and FWRs (93). Strikingly, the diversity of substitutions at changed positions is also correlated with germline diversity, though the diversity of the germline amino acids is less than that of the substitutions (93). While this at least partially reflects the structural constraints of the antibody domain, the physicochemical properties of the observed substitutions did not generally parallel those of the germline residues at the same positions (93).
In fact, the diversity of the observed substitutions is constrained not only by the diversity of all germline genes at a position but specifically by the particular gene from which the antibody was derived (33, 34) (Figure 1). As seen in studies of microsequence motifs (44) and V(D)J recombination (2, 11, 13, 91, 94), these substitution profiles are stable between individuals and across time (33). Similar findings have been reported both for sequences isolated from peripheral memory B cells (33) and from bone marrow cells (34). Both substitution frequency and the diversity of observed substitutions are generally higher in CDRs than in FWRs, though several FWR positions have substitution profiles similar to those characteristic of CDRs (33, 34). In addition, assorted VH genes accumulate substitutions in CDRH1 versus CDRH2 at different rates, and similar variations appear in the preferred locations of insertions and deletions (34).
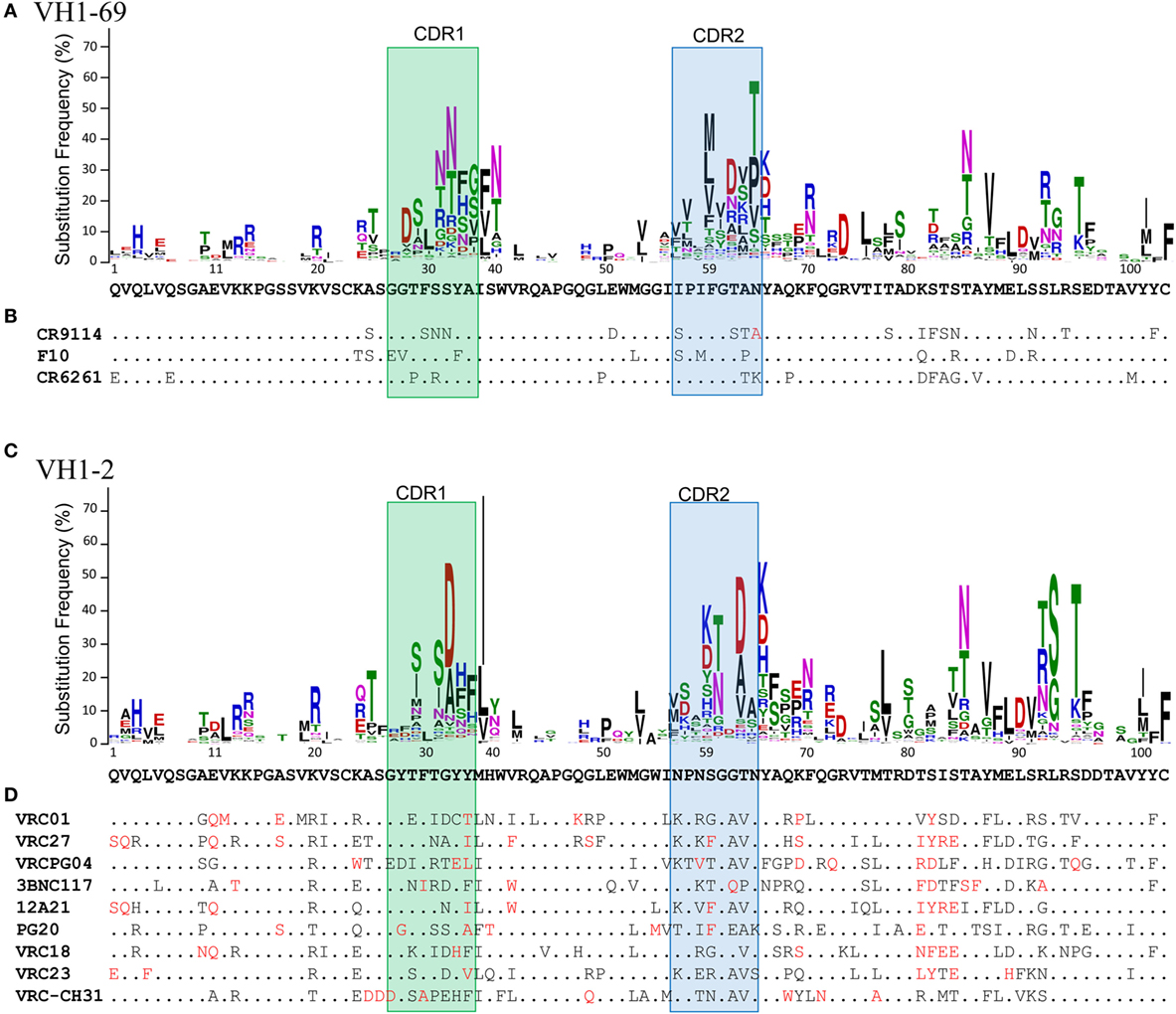
Figure 1. Substitution profiles and the accumulation of rare mutations. (A) Substitution profile of VH1-69 as calculated by Sheng et al. (33). The germline amino acid sequence is shown below the logo plot. (B) Sequence alignment of VH1-69-derived influenza antibodies capable of neutralizing multiple subtypes. Dots indicate the germline amino acid and extremely rare substitutions [as defined in Ref. (33)] are shown in red. (C) Substitution profile of VH1-2. (D) Sequence alignment of VH1-2-derived broadly neutralizing HIV antibodies targeting the CD4 binding site. Each contains greater than ~20% or more extremely rare substitutions.
Many expected factors contribute to the observed substitution profiles. For instance, the frequencies of substitution are generally lower at structurally important residues such as the charge cluster (95), though individual genes may display higher rates, as for R95 of VH1-8 (34) [residue numberings are reported using the IMGT convention (96)]. Similarly, when a particular gene carries a residue that is distinct from other genes in its VH family (e.g., L71 in VH1-18 and T46 of VH1-8), substitutions at that positions are frequently biased toward the germline residue(s) encoded by the other members of the gene’s family (in this case, F and P, respectively) (34). The presence or absence of a microsequence hot spot also clearly impacts the observed differential substitution rates at some positions, such as S29 in VH5-51, which forms an AGCT hot spot and diversifies extensively, while the same serine in VH4 genes is encoded by a TCC codon and mutates only rarely (34). However, it cannot account for all such differences; for instance, R80 in VH1-8 diversifies extensively despite the absence of a canonical hot spot, while the equivalent arginine in VH3 genes is almost complete conserved, without the presence of an AID cold spot (34). Simulations indicate that microsequence motifs can account for about 70% of the variation in substitution frequencies, similar to previous reports (44), but only about 50% of the variation when the identity of the substitutions is included (33). Another contributing factor to substitution profiles is the fact that observed substitutions are typically those that can be reached by a single nucleotide change. However, the same codon can have substantially different profiles even in a highly similar sequence context. Thus, the TCC codon encoding S83 of VH1-2 is most likely to be substituted to A, followed by T and P, while the most likely substitutions for S83 of VH1-46 are P and F, followed by A (33). Furthermore, while biases in substitutions are somewhat correlated with the physicochemical similarity between the germline amino acid and the observed substitution, many commonly seen substitutions are non-conservative, and even conservative substitutions are frequently asymmetric (e.g., E→D and K→R substitutions are more likely to occur than D→E and R→K substitutions, respectively) (33, 97).
In addition to highly significant similarities in substitution profiles between individuals with presumably distinct antigen-exposure histories, substitution profiles observed in the selected functional repertoire are also correlated to those derived from non-functional passenger alleles (33). This convergence of the selected and unselected repertoires is quite surprising and implies stricter limitations on the action of SHM than had previously been understood. One possibility is that the evolutionary optimizations described above are fine-tuned enough to strongly bias the production of mutations toward those that are most likely to be selected for by the suite of antigens that has been most commonly encountered over the evolutionary history of a species (33). In this vein, recent work has shown that relatively low-affinity antibody lineages can persist in germinal centers responding to complex protein antigens (98–100). This results in a memory response with increased clonal diversity compared to that generated by haptens, and it has been hypothesized that this diversity enhances the capacity of the immune system to respond to future challenges from novel but structurally related antigens (101). It may be that the characteristic substitutions observed in substitution profiles serve to optimize the structure to this diversity. An additional, perhaps complementary, alternative is that the biases in the mutations produced by the SHM machinery are strong enough that most mutations are not produced frequently enough to be acted upon by selection. In either case, there would appear to be drastic implications for rational vaccine design efforts, as certain substitutions may not be reliably available in a typical repertoire, even with an optimal antigen.
More generally, the existence of substitution profiles indicates that there are preferred pathways for antibody affinity maturation that depend powerfully on the germline gene used. This, in turn, suggests that germline-based substitution profiles contain useful information about which substitutions are likely to be tolerated at each position, which can be leveraged for antibody engineering. As most engineering efforts begin from a known monoclonal antibody, a narrower substitution profile, encompassing a single antibody lineage, may be of particular use (102). These lineage-specific substitution profiles are expected to be different from gene-specific substitution profiles (33), but may better reflect the constraints of binding to a specific antigen. They also provide an opportunity to extract information about which substitutions can be tolerated at positions in CDR3 and FWR4, which are absent in V gene-specific profiles. Frequently, however, the antibody that is being engineered is the only known member of its lineage; even when deep repertoire sampling is done with high-throughput sequencing, most lineages are represented by only one or a few members (103).
A new program named SPURF attempts to overcome that limitation by combining several types of substitution profiles derived from a large public data set to predict the substitution profile of an antibody lineage from the sequence of a single member (102). In training the SPURF model, the authors found that the most important sources of information are the V gene-specific substitution profile and the inferred naive sequence, in addition to the input sequence itself. They also use a gene-family substitution profile (i.e., derived from all VH1 genes, etc.) and a substitution profile calculated from simulations of neutral mutation of the inferred naive sequence using the S5F model from reference (44, 102). In particular, the inclusion of the inferred naive sequence allows the prediction of a substitution profile for CDR3 and FWR4, which are not encoded by the V gene and, therefore, missed by a V gene-specific profile alone.
Open Questions
While SPURF performs well predicting the lineage-specific substitution profiles of an out-of-sample validation set (102) and is designed to be used for antibody engineering and improvement, it has not yet been tested in that context. Similarly, it remains to be seen if substitution profiles can be successfully incorporated into a predictive model of SHM. And while rare substitutions can be functionally important (104), systematic comparisons of the structural and biophysical effects of common versus rare substitutions are ongoing. In addition, substitution profiles treat the mutations observed at each position as being independent. However, recent work suggests that affinity-enhancing mutations may be co-selected with structurally stabilizing ones (105), and the possibility of correlations between the substitution profiles of different positions should be investigated.
Another open question involves the effects of allelic variants on substitution profiles. Even silent polymorphisms could theoretically change the pattern of mutations generated by SHM by the introduction or removal of a microsequence hot spot. More importantly, allelic variants are sometimes distinguished by replacement mutations (e.g., G55 versus R55 in VH1-69). Since the germline residue remains the most commonly observed amino acid at most positions, these variants will have a large impact on the resulting substitution profile. So far, this has been handled in an ad hoc manner, by either excluding genes from donors who have previously been determined to be heterozygous for such variants (34) or by collectively excluding all possible germline residues at each position from the substitution profile, irrespective of individual genotype (33). Since the germline residues at homologous positions in closely related genes are frequently observed substitutions (34), a more systematic way of investigating the effects of allelic variants is necessary. This is especially true as it has recently become clear that many such variants remain to be discovered (106–109).
Finally, one of the most striking findings about substitution profiles is the similarity of the selected and unselected repertoires. Yet, this observation rests on mere 650 non-productive rearrangements derived from a single VH–JH gene pair (33, 110). Although the strong correlations between substitution profiles from different individuals also support the idea that SHM is capable of generating only a limited set of mutations, more data are needed to test this. Meanwhile, it is clear that mutation and selection are distinct biological processes. In order to avoid possible confounding effects of selection, studies of microsequence motifs have typically used sequences derived from introns, transgenes, or non-productive rearrangements; or, if using sequences from functional antibodies subject to selection, have included only silent mutations extracted from those data sets.
Separately, many efforts have been made to detect and quantify the action of selection on affinity maturation. Initially, these evaluated the frequency of replacement mutations observed in CDRs versus FWRs using a binomial (111) or multinomial (112) distribution. The binomial model has also been extended to account for codon biases that lead to a higher neutral rate of replacement mutations CDRs (70) and to account for general differences in mutability driven by microsequence context (113). However, determining the appropriate null distribution of replacement versus silent mutations in antibodies has proven challenging, as the intrinsic biases of SHM can give the appearance of selection (114) even when microsequence motifs are accounted for (113). One strategy for addressing this difficulty has been to use a focused binomial test examining the replacement mutations from only a single CDR or FWR at time, while using the silent mutations from all regions (115, 116). Another strategy exploited a large data set of non-productive rearrangements to normalize the ratio of replacement to silent mutations on a germline- and position-specific basis (94). Other recent advancements include the use of a log-odds ratio of the posterior distribution of the replacement mutation frequency compared to the expected distribution for the germline sequence, to quantify the strength of selection (117); the integration of phylogenetic information (118, 119); and estimation of the null distribution for the number of replacement mutations so that selection effects can be calculated for a single sequence (120).
While there is general agreement that purifying selection typically acts on FWRs, reports have been inconsistent as to whether diversifying selection acting on CDRs can (94, 115, 117) or cannot (114, 121) be detected at the repertoire level. Meanwhile, a review of available structural data found no relation between hot spot motifs and observed substitutions; the latter were instead strongly correlated with antigen contacts and contributions to calculated binding energy (122). In addition, a recent study found that the need to distinguish between closely related foreign and self antigens can drive the expansion of higher affinity clonal variants that remain subdominant in the absence of self antigen (123), demonstrating another way in which selection can influence the observed substitutions in a repertiore. On the other hand, an in-depth analysis of an antibody against influenza hemagglutinin found that mutability and selection synergized, such that replacement mutations expected to occur more frequently under a neutral model were also more like to be selected once generated (124). It is, therefore, clear that more work is needed to resolve when the effects of selection must be explicitly accounted for and when they can be implicitly included by the use of substitution profiles or other similar abstractions. Structural and biophysical characterizations of common versus rare substitutions should help resolve this question and will also be important for understanding the underlying biological mechanisms.
Vaccine Implications
Reverse vaccinology 2.0 (125, 126) is a strategy for rational vaccine design that starts by characterizing the epitope targeted by an effective natural antibody and selecting or designing an immunogen that can elicit a similar antibody in other individuals. One particular implementation is lineage-based vaccine design, which attempts to find a series of immunogens that can together induce a vaccine-elicited antibody to recapitulate the ontogeny of a known lineage (127–130). Both strategies rest on the assumption that antibody elicitation is fundamentally reproducible. Thus, lineage-based vaccine design for HIV has focused on “classes” of antibodies (128) with similar genetic characteristics that have been observed in multiple donors. Despite genetic and structural similarity, however, several obstacles to the successful design of a vaccine capable of eliciting protective classes of antibodies remain to be overcome.
In particular, antibodies capable of broad neutralization of HIV have particularly high levels of SHM (128, 131, 132) and tend to be enriched for rare substitutions (104, 133, 134) (Figure 1). Extraordinary levels of SHM (15–35% nucleotide mutations) are characteristic of antibodies targeting HIV (135), and elevated levels of SHM have also been observed in other types of chronic infection and in systemic autoimmune disorders (136). By contrast, the maximum level of SHM that has been observed in vaccine-responsive antibodies is 8–10% nucleotide mutations, even after multiple doses (137, 138).
Fortunately, however, many mutations found in broadly neutralizing antibodies (bnAbs) against HIV appear to be unnecessary for full function (139, 140). In fact, two HIV bnAbs have recently been reported with at least 50% breadth and less than 10% nucleotide mutation in VH: CAP256-VRC26.25 (141) and DH270.1 (142). Importantly, though, both contain other unusual features. CAP256-VRC26.25 has an extraordinarily long heavy chain CDR3 of 38 amino acids, including a 1 amino acid insertion relative to the inferred naive ancestor (141), while the neutralization activity of DH270.1 depends on a critical Gly64Arg (IMGT numbering) mutation in a canonical SYC cold spot (142). As noted above, such rare mutations are generally enriched in HIV bnAbs compared to flu bnAbs (Figure 1) and antibodies from normal repertoires or induced by a vaccine (104). While accumulation of some rare substitutions may be incidental to the overall level of SHM (33, 104), a recent report demonstrated that half of the HIV bnAbs studied have accumulated significantly more rare mutations than expected under a neutral evolutionary model of SHM (104). Similarly, several positions with low intrinsic mutation rates were determined to be significantly enriched in a class of VH1-2-derived HIV bnAbs, based on their recurrence in members of that class (133). These observations suggest that, as for the DH270 lineage, at least some rare mutations may be functionally important. Indeed, this has recently been confirmed for three additional HIV bnAbs (104). The identification of critical rare mutations and strategies to reproduce them will be central to the success of lineage-based vaccine design.
One possible approach is to design immunogens capable of exerting strong selection on rare mutations as soon as they occur (104). However, even mutations that increase the affinity of an antibody 10-fold take much longer to dominate a germinal center reaction than would be expected from a simple model of SHM (91, 143). Moreover, recent work has shown that lower affinity subclones can persist in germinal centers (98–100), which may prevent antibodies with the desired rare substitution from reaching protective levels, even with an optimal immunogen. Indeed, while several recent studies in transgenic mice have elicited B cells enriched for substitutions present in the targeted mature antibody (144–146), none have yet specifically elicited critical rare substitutions or fully recapitulated the neturalization activity of the target antibodies. Notably, however, the most successful example focuses on PGT121 (146), which contains fewer rare substitutions than many other HIV antibodies (104, 134). It may, therefore, be more prudent to choose lineage-based vaccine design targets by avoiding those with functionally important rare substitutions (33, 134).
Conclusion
The mechanisms of antibody diversification have evolved to achieve a balance between the plasticity needed to successfully bind to unknown novel antigens and the robustness needed to do so in a biologically feasible manner. This results in a series of patterns and variations that can be studied computationally both to illuminate the underlying cellular processes and to predict the response to specific manipulations. As advances in technology have made it possible to collect ever larger datasets, our ability to detect and understand these patterns has grown, as well. The insights provided thus far by substitution profiles and related concepts have already begun to be applied to antibody engineering and vaccine design. Concurrently, work is ongoing to understand the biology behind these patterns and to develop them into predictive models of immune function.
Author Contributions
CS wrote the paper. All authors reviewed, commented on, and approved the manuscript.
Conflict of Interest Statement
The authors declare that the research was conducted in the absence of any commercial or financial relationships that could be construed as a potential conflict of interest.
Acknowledgments
We thank Dr. Zizhang Sheng for helpful comments and assistance with the figure.
Funding
Funding was provided by the intramural program of the Vaccine Research Center, National Institute of Allergy and Infectious Disease, National Institutes of Health.
References
1. Schroeder HW. Similarity and divergence in the development and expression of the mouse and human antibody repertoires. Dev Comp Immunol (2006) 30(1):119–35. doi:10.1016/j.dci.2005.06.006
2. Elhanati Y, Sethna Z, Marcou Q, Callan CG, Mora T, Walczak AM. Inferring processes underlying B-cell repertoire diversity. Philos Trans R Soc Lond B Biol Sci (2015) 5(1676):370. doi:10.1098/rstb.2014.0243
3. Morbach H, Eichhorn EM, Liese JG, Girschick HJ. Reference values for B cell subpopulations from infancy to adulthood. Clin Exp Immunol (2010) 162(2):271–9. doi:10.1111/j.1365-2249.2010.04206.x
4. Perez-Andres M, Paiva B, Nieto WG, Caraux A, Schmitz A, Almeida J, et al. Human peripheral blood B-cell compartments: a crossroad in B-cell traffic. Cytometry B Clin Cytom (2010) 78B(S1):S47–60. doi:10.1002/cyto.b.20547
5. Gearhart PJ. Antibody wars: extreme diversity. J Immunol (2006) 177(7):4235–6. doi:10.4049/jimmunol.177.7.4235
6. Hood L, Talmage DW. Mechanism of antibody diversity: germ line basis for variability. Science (1970) 168(3929):325–34. doi:10.1126/science.168.3929.325
7. Schroeder HW, Hillson JL, Perlmutter RM. Early restriction of the human antibody repertoire. Science (1987) 238(4828):791–3. doi:10.1126/science.3118465
8. Suzuki I, Pfister L, Glas A, Nottenburg C, Milner EC. Representation of rearranged VH gene segments in the human adult antibody repertoire. J Immunol (1995) 154(8):3902–11.
9. Volpe JM, Kepler TB. Large-scale analysis of human heavy chain V(D)J recombination patterns. Immunome Res (2008) 4:3. doi:10.1186/1745-7580-4-3
10. Hansen TØ, Lange AB, Barington T. Sterile DJH rearrangements reveal that distance between gene segments on the human Ig H chain locus influences their ability to rearrange. J Immunol (2015) 194(3):973–82. doi:10.4049/jimmunol.1401443
11. Briney BS, Willis JR, Hicar MD, Thomas JW, Crowe JE. Frequency and genetic characterization of V(DD)J recombinants in the human peripheral blood antibody repertoire. Immunology (2012) 137(1):56–64. doi:10.1111/j.1365-2567.2012.03605.x
12. Henry Dunand CJ, Wilson PC. Restricted, canonical, stereotyped and convergent immunoglobulin responses. Philos Trans R Soc Lond B Biol Sci (2015) 370. doi:10.1098/rstb.2014.0238
13. Ralph DK, Matsen FA. Consistency of VDJ rearrangement and substitution parameters enables accurate B cell receptor sequence annotation. PLoS Comput Biol (2016) 12(1):e1004409. doi:10.1371/journal.pcbi.1004409
14. Briney BS, Willis JR, McKinney BA, Crowe JE. High-throughput antibody sequencing reveals genetic evidence of global regulation of the naïve and memory repertoires that extends across individuals. Genes Immun (2012) 13(6):469–73. doi:10.1038/gene.2012.20
15. Weigert MG, Cesari IM, Yonkovich SJ, Cohn M. Variability in the lambda light chain sequences of mouse antibody. Nature (1970) 228(5276):1045–7. doi:10.1038/2281045a0
16. Bernard O, Hozumi N, Tonegawa S. Sequences of mouse immunoglobulin light chain genes before and after somatic changes. Cell (1978) 15(4):1133–44. doi:10.1016/0092-8674(78)90041-7
17. Gearhart PJ, Bogenhagen DF. Clusters of point mutations are found exclusively around rearranged antibody variable genes. Proc Natl Acad Sci U S A (1983) 80(11):3439–43. doi:10.1073/pnas.80.11.3439
18. Berek C, Milstein C. Mutation drift and repertoire shift in the maturation of the immune response. Immunol Rev (1987) 96:23–41. doi:10.1111/j.1600-065X.1987.tb00507.x
19. Betz AG, Rada C, Pannell R, Milstein C, Neuberger MS. Passenger transgenes reveal intrinsic specificity of the antibody hypermutation mechanism: clustering, polarity, and specific hot spots. Proc Natl Acad Sci U S A (1993) 90(6):2385–8. doi:10.1073/pnas.90.6.2385
20. Muramatsu M, Sankaranand VS, Anant S, Sugai M, Kinoshita K, Davidson NO, et al. Specific expression of activation-induced cytidine deaminase (AID), a novel member of the RNA-editing deaminase family in germinal center B cells. J Biol Chem (1999) 274(26):18470–6. doi:10.1074/jbc.274.26.18470
21. Muramatsu M, Kinoshita K, Fagarasan S, Yamada S, Shinkai Y, Honjo T. Class switch recombination and hypermutation require activation-induced cytidine deaminase (AID), a potential RNA editing enzyme. Cell (2000) 102(5):553–63. doi:10.1016/S0092-8674(00)00078-7
22. Revy P, Muto T, Levy Y, Geissmann F, Plebani A, Sanal O, et al. Activation-induced cytidine deaminase (AID) deficiency causes the autosomal recessive form of the hyper-IgM syndrome (HIGM2). Cell (2000) 102(5):565–75. doi:10.1016/S0092-8674(00)00079-9
23. Chandra V, Bortnick A, Murre C. AID targeting: old mysteries and new challenges. Trends Immunol (2015) 36(9):527–35. doi:10.1016/j.it.2015.07.003
24. Casellas R, Basu U, Yewdell WT, Chaudhuri J, Robbiani DF, Noia JMD. Mutations, kataegis and translocations in B cells: understanding AID promiscuous activity. Nat Rev Immunol (2016) 16(3):164–76. doi:10.1038/nri.2016.2
25. Methot SP, Di Noia JM. Molecular mechanisms of somatic hypermutation and class switch recombination. Adv Immunol (2017) 133:37–87. doi:10.1016/bs.ai.2016.11.002
26. Kohli RM, Abrams SR, Gajula KS, Maul RW, Gearhart PJ, Stivers JT. A portable hot spot recognition loop transfers sequence preferences from APOBEC family members to activation-induced cytidine deaminase. J Biol Chem (2009) 284(34):22898–904. doi:10.1074/jbc.M109.025536
27. Wang M, Rada C, Neuberger MS. Altering the spectrum of immunoglobulin V gene somatic hypermutation by modifying the active site of AID. J Exp Med (2010) 207(1):141–53. doi:10.1084/jem.20092238
28. Pham P, Afif SA, Shimoda M, Maeda K, Sakaguchi N, Pedersen LC, et al. Structural analysis of the activation-induced deoxycytidine deaminase required in immunoglobulin diversification. DNA Repair (2016) 43:48–56. doi:10.1016/j.dnarep.2016.05.029
29. Halemano K, Guo K, Heilman KJ, Barrett BS, Smith DS, Hasenkrug KJ, et al. Immunoglobulin somatic hypermutation by APOBEC3/Rfv3 during retroviral infection. Proc Natl Acad Sci U S A (2014) 111(21):7759–64. doi:10.1073/pnas.1403361111
30. Zanotti KJ, Gearhart PJ. Antibody diversification caused by disrupted mismatch repair and promiscuous DNA polymerases. DNA Repair (2016) 38:110–6. doi:10.1016/j.dnarep.2015.11.011
31. Rada C, Ehrenstein MR, Neuberger MS, Milstein C. Hot spot focusing of somatic hypermutation in MSH2-deficient mice suggests two stages of mutational targeting. Immunity (1998) 9(1):135–41. doi:10.1016/S1074-7613(00)80595-6
32. Rogozin IB, Pavlov YI, Bebenek K, Matsuda T, Kunkel TA. Somatic mutation hotspots correlate with DNA polymerase eta error spectrum. Nat Immunol (2001) 2(6):530–6. doi:10.1038/88732
33. Sheng Z, Schramm CA, Kong R; NISC Comparative Sequencing Program, Mullikin JC, Mascola JR, et al. Gene-specific substitution profiles describe the types and frequencies of amino acid changes during antibody somatic hypermutation. Front Immunol (2017) 8:537. doi:10.3389/fimmu.2017.00537
34. Kirik U, Persson H, Levander F, Greiff L, Ohlin M. Antibody heavy chain variable domains of different germline gene origins diversify through different paths. Front Immunol (2017) 8:1433. doi:10.3389/fimmu.2017.01433
35. Lederberg J. Genes and antibodies. Science (1959) 129(3364):1649–53. doi:10.1126/science.129.3364.1649
36. Brenner S, Milstein C. Origin of antibody variation. Nature (1966) 211(5046):242–3. doi:10.1038/211242a0
37. Selsing E, Storb U. Somatic mutation of immunoglobulin light-chain variable-region genes. Cell (1981) 25(1):47–58. doi:10.1016/0092-8674(81)90230-0
38. Rogozin IB, Kolchanov NA. Somatic hypermutagenesis in immunoglobulin genes: II. Influence of neighbouring base sequences on mutagenesis. Biochim Biophys Acta (1992) 1171(1):11–8. doi:10.1016/0167-4781(92)90134-L
39. Pham P, Bransteitter R, Petruska J, Goodman MF. Processive AID-catalysed cytosine deamination on single-stranded DNA simulates somatic hypermutation. Nature (2003) 424(6944):103–7. doi:10.1038/nature01760
40. Smith DS, Creadon G, Jena PK, Portanova JP, Kotzin BL, Wysocki LJ. Di- and trinucleotide target preferences of somatic mutagenesis in normal and autoreactive B cells. J Immunol (1996) 156(7):2642–52.
41. Jolly CJ, Wagner SD, Rada C, Klix N, Milstein C, Neuberger MS. The targeting of somatic hypermutation. Semin Immunol (1996) 8(3):159–68. doi:10.1006/smim.1996.0020
42. Dörner T, Foster SJ, Farner NL, Lipsky PE. Somatic hypermutation of human immunoglobulin heavy chain genes: targeting of RGYW motifs on both DNA strands. Eur J Immunol (1998) 28(10):3384–96. doi:10.1002/(SICI)1521-4141(199810)28:10<3384::AID-IMMU3384>3.0.CO;2-T
43. Ohm-Laursen L, Barington T. Analysis of 6912 unselected somatic hypermutations in human VDJ rearrangements reveals lack of strand specificity and correlation between phase II substitution rates and distance to the nearest 3’ activation-induced cytidine deaminase target. J Immunol (2007) 178(7):4322–34. doi:10.4049/jimmunol.178.7.4322
44. Yaari G, Vander Heiden JA, Uduman M, Gadala-Maria D, Gupta N, Stern JNH, et al. Models of somatic hypermutation targeting and substitution based on synonymous mutations from high-throughput immunoglobulin sequencing data. Front Immunol (2013) 4:358. doi:10.3389/fimmu.2013.00358
45. Rogozin IB, Diaz M. Cutting edge: DGYW/WRCH is a better predictor of mutability at G:C bases in Ig hypermutation than the widely accepted RGYW/WRCY motif and probably reflects a two-step activation-induced cytidine deaminase-triggered process. J Immunol (2004) 172(6):3382–4. doi:10.4049/jimmunol.172.6.3382
46. Yu K, Huang F-T, Lieber MR. DNA substrate length and surrounding sequence affect the activation-induced deaminase activity at cytidine. J Biol Chem (2004) 279(8):6496–500. doi:10.1074/jbc.M311616200
47. Wei L, Chahwan R, Wang S, Wang X, Pham PT, Goodman MF, et al. Overlapping hotspots in CDRs are critical sites for V region diversification. Proc Natl Acad Sci U S A (2015) 112(7):E728–37. doi:10.1073/pnas.1500788112
48. Cui A, Niro RD, Heiden JAV, Briggs AW, Adams K, Gilbert T, et al. A model of somatic hypermutation targeting in mice based on high-throughput Ig sequencing data. J Immunol (2016) 197(9):3566–74. doi:10.4049/jimmunol.1502263
49. Shapiro GS, Aviszus K, Ikle D, Wysocki LJ. Predicting regional mutability in antibody V genes based solely on di- and trinucleotide sequence composition. J Immunol (1999) 163(1):259–68.
50. Shapiro GS, Aviszus K, Murphy J, Wysocki LJ. Evolution of Ig DNA sequence to target specific base positions within codons for somatic hypermutation. J Immunol (2002) 168(5):2302–6. doi:10.4049/jimmunol.168.5.2302
51. Boursier L, Su W, Spencer J. Imprint of somatic hypermutation differs in human immunoglobulin heavy and lambda chain variable gene segments. Mol Immunol (2003) 39(16):1025–34. doi:10.1016/S0161-5890(03)00033-6
52. Cohen RM, Kleinstein SH, Louzoun Y. Somatic hypermutation targeting is influenced by location within the immunoglobulin V region. Mol Immunol (2011) 48(12–13):1477–83. doi:10.1016/j.molimm.2011.04.002
53. Chan OT, Hannum LG, Haberman AM, Madaio MP, Shlomchik MJ. A novel mouse with B cells but lacking serum antibody reveals an antibody-independent role for B cells in murine lupus. J Exp Med (1999) 189(10):1639–48. doi:10.1084/jem.189.10.1639
54. Marcou Q, Mora T, Walczak AM. High-throughput immune repertoire analysis with IGoR. Nat Commun (2018) 9(1):561. doi:10.1038/s41467-018-02832-w
55. Feng J, Shaw DA, Minin VN, Simon N, Matsen FA IV. Survival analysis of DNA mutation motifs with penalized proportional hazards. arXiv (2017) arXiv:1711.04057.
56. Lebecque SG, Gearhart PJ. Boundaries of somatic mutation in rearranged immunoglobulin genes: 5’ boundary is near the promoter, and 3’ boundary is approximately 1 kb from V(D)J gene. J Exp Med (1990) 172(6):1717–27. doi:10.1084/jem.172.6.1717
57. Cowell LG, Kepler TB. The nucleotide-replacement spectrum under somatic hypermutation exhibits microsequence dependence that is strand-symmetric and distinct from that under germline mutation. J Immunol (2000) 164(4):1971–6. doi:10.4049/jimmunol.164.4.1971
58. Mirsky A, Kazandjian L, Anisimova M. Antibody-specific model of amino acid substitution for immunological inferences from alignments of antibody sequences. Mol Biol Evol (2015) 32:806–819. doi:10.1093/molbev/msu340
59. Yeap L-S, Hwang JK, Du Z, Meyers RM, Meng F-L, Jakubauskaitė A, et al. Sequence-intrinsic mechanisms that target AID mutational outcomes on antibody genes. Cell (2015) 163(5):1124–37. doi:10.1016/j.cell.2015.10.042
60. Rada C, Milstein C. The intrinsic hypermutability of antibody heavy and light chain genes decays exponentially. EMBO J (2001) 20(16):4570–6. doi:10.1093/emboj/20.16.4570
61. Hood L, Campbell JH, Elgin SC. The organization, expression, and evolution of antibody genes and other multigene families. Annu Rev Genet (1975) 9:305–53. doi:10.1146/annurev.ge.09.120175.001513
62. Ohta T. Evolution and Variation of Multigene Families. Berlin, Heidelberg: Springer-Verlag (1980).
63. Gojobori T, Nei M. Concerted evolution of the immunoglobulin VH gene family. Mol Biol Evol (1984) 1(2):195–212.
64. Ota T, Nei M. Divergent evolution and evolution by the birth-and-death process in the immunoglobulin VH gene family. Mol Biol Evol (1994) 11(3):469–82.
65. Nei M, Gu X, Sitnikova T. Evolution by the birth-and-death process in multigene families of the vertebrate immune system. Proc Natl Acad Sci U S A (1997) 94(15):7799–806. doi:10.1073/pnas.94.15.7799
66. Tanaka T, Nei M. Positive Darwinian selection observed at the variable-region genes of immunoglobulins. Mol Biol Evol (1989) 6(5):447–59.
67. Hinds-Frey KR, Nishikata H, Litman RT, Litman GW. Somatic variation precedes extensive diversification of germline sequences and combinatorial joining in the evolution of immunoglobulin heavy chain diversity. J Exp Med (1993) 178(3):815–24. doi:10.1084/jem.178.3.815
68. Quinlan EM, King JJ, Amemiya CT, Hsu E, Larijani M. Biochemical regulatory features of activation-induced cytidine deaminase remain conserved from lampreys to humans. Mol Cell Biol (2017) 37(20). doi:10.1128/MCB.00077-17
69. Rogozin IB, Iyer LM, Liang L, Glazko GV, Liston VG, Pavlov YI, et al. Evolution and diversification of lamprey antigen receptors: evidence for involvement of an AID-APOBEC family cytosine deaminase. Nat Immunol (2007) 8(6):647–56. doi:10.1038/ni1463
70. Chang B, Casali P. The CDR1 sequences of a major proportion of human germline Ig VH genes are inherently susceptible to amino acid replacement. Immunol Today (1994) 15(8):367–73. doi:10.1016/0167-5699(94)90175-9
71. Hershberg U, Shlomchik MJ. Differences in potential for amino acid change after mutation reveals distinct strategies for kappa and lambda light-chain variation. Proc Natl Acad Sci U S A (2006) 103(43):15963–8. doi:10.1073/pnas.0607581103
72. Saini J, Hershberg U. B cell variable genes have evolved their codon usage to focus the targeted patterns of somatic mutation on the complementarity determining regions. Mol Immunol (2015) 65(1):157–67. doi:10.1016/j.molimm.2015.01.001
73. Wagner SD, Milstein C, Neuberger MS. Codon bias targets mutation. Nature (1995) 376(6543):732. doi:10.1038/376732a0
74. Kepler TB. Codon bias and plasticity in immunoglobulins. Mol Biol Evol (1997) 14(6):637–43. doi:10.1093/oxfordjournals.molbev.a025803
75. Golub R, Charlemagne J. Structure, diversity, and repertoire of VH families in the Mexican axolotl. J Immunol (1998) 160(3):1233–9.
76. Oreste U, Coscia M. Specific features of immunoglobulin VH genes of the Antarctic teleost Trematomus bernacchii. Gene (2002) 295(2):199–204. doi:10.1016/S0378-1119(02)00686-8
77. Conticello SG, Thomas CJF, Petersen-Mahrt SK, Neuberger MS. Evolution of the AID/APOBEC family of polynucleotide (deoxy)cytidine deaminases. Mol Biol Evol (2005) 22(2):367–77. doi:10.1093/molbev/msi026
78. Oprea M, Kepler TB. Genetic plasticity of V genes under somatic hypermutation: statistical analyses using a new resampling-based methodology. Genome Res (1999) 9(12):1294–304. doi:10.1101/gr.9.12.1294
79. Detanico T, Phillips M, Wysocki LJ. Functional versatility of AGY serine codons in immunoglobulin variable region genes. Front Immunol (2016) 7:525. doi:10.3389/fimmu.2016.00525
80. Zheng N-Y, Wilson K, Jared M, Wilson PC. Intricate targeting of immunoglobulin somatic hypermutation maximizes the efficiency of affinity maturation. J Exp Med (2005) 201(9):1467–78. doi:10.1084/jem.20042483
81. Koenig P, Lee CV, Walters BT, Janakiraman V, Stinson J, Patapoff TW, et al. Mutational landscape of antibody variable domains reveals a switch modulating the interdomain conformational dynamics and antigen binding. Proc Natl Acad Sci U S A (2017) 114(4):E486-E495. doi:10.1073/pnas.1613231114
82. van de Bovenkamp FS, Derksen NIL, Ooijevaar-de Heer P, van Schie KA, Kruithof S, Berkowska MA, et al. Adaptive antibody diversification through N-linked glycosylation of the immunoglobulin variable region. Proc Natl Acad Sci U S A (2018) 115(8):1901–6. doi:10.1073/pnas.1711720115
83. Collins AM, Jackson KJL. On being the right size: antibody repertoire formation in the mouse and human. Immunogenetics (2018) 70(3):143–58. doi:10.1007/s00251-017-1049-8
84. Krause JC, Tsibane T, Tumpey TM, Huffman CJ, Briney BS, Smith SA, et al. Epitope-specific human influenza antibody repertoires diversify by B cell intraclonal sequence divergence and interclonal convergence. J Immunol (2011) 187(7):3704–11. doi:10.4049/jimmunol.1101823
85. Jackson KJL, Liu Y, Roskin KM, Glanville J, Hoh RA, Seo K, et al. Human responses to influenza vaccination show seroconversion signatures and convergent antibody rearrangements. Cell Host Microbe (2014) 16(1):105–14. doi:10.1016/j.chom.2014.05.013
86. Joyce MG, Wheatley AK, Thomas PV, Chuang G-Y, Soto C, Bailer RT, et al. Vaccine-induced antibodies that neutralize group 1 and group 2 influenza A viruses. Cell (2016) 166(3):609–23. doi:10.1016/j.cell.2016.06.043
87. Zhou T, Zhu J, Wu X, Moquin S, Zhang B, Acharya P, et al. Multidonor analysis reveals structural elements, genetic determinants, and maturation pathway for HIV-1 neutralization by VRC01-class antibodies. Immunity (2013) 39(2):245–58. doi:10.1016/j.immuni.2013.04.012
88. Zhou T, Lynch RM, Chen L, Acharya P, Wu X, Doria-Rose NA, et al. Structural repertoire of HIV-1-neutralizing antibodies targeting the CD4 supersite in 14 donors. Cell (2015) 161(6):1280–92. doi:10.1016/j.cell.2015.05.007
89. Allen D, Cumano A, Dildrop R, Kocks C, Rajewsky K, Rajewsky N, et al. Timing, genetic requirements and functional consequences of somatic hypermutation during B-cell development. Immunol Rev (1987) 96:5–22. doi:10.1111/j.1600-065X.1987.tb00506.x
90. Pappas L, Foglierini M, Piccoli L, Kallewaard NL, Turrini F, Silacci C, et al. Rapid development of broadly influenza neutralizing antibodies through redundant mutations. Nature (2014) 516(7531):418–22. doi:10.1038/ature13764
91. Radmacher MD, Kelsoe G, Kepler TB. Predicted and inferred waiting times for key mutations in the germinal centre reaction: evidence for stochasticity in selection. Immunol Cell Biol (1998) 76(4):373–81. doi:10.1046/j.1440-1711.1998.00753.x
92. Bonsignori M, Zhou T, Sheng Z, Chen L, Gao F, Joyce MG, et al. Maturation pathway from germline to broad HIV-1 neutralizer of a CD4-mimic antibody. Cell (2016) 165(2):449–63. doi:10.1016/j.cell.2016.02.022
93. Schwartz GW, Hershberg U. Germline amino acid diversity in B cell receptors is a good predictor of somatic selection pressures. Front Immunol (2013) 4:357. doi:10.3389/fimmu.2013.00357
94. McCoy CO, Bedford T, Minin VN, Bradley P, Robins H, Matsen FA. Quantifying evolutionary constraints on B-cell affinity maturation. Philos Trans R Soc Lond B Biol Sci (2015) 5:370. doi:10.1098/rstb.2014.0244
95. Honegger A, Malebranche AD, Röthlisberger D, Plückthun A. The influence of the framework core residues on the biophysical properties of immunoglobulin heavy chain variable domains. Protein Eng Des Sel PEDS (2009) 22(3):121–34. doi:10.1093/protein/gzn077
96. Lefranc MP. Unique database numbering system for immunogenetic analysis. Immunol Today (1997) 18(11):509. doi:10.1016/S0167-5699(97)01163-8
97. Clark LA, Ganesan S, Papp S, van Vlijmen HWT. Trends in antibody sequence changes during the somatic hypermutation process. J Immunol (2006) 177(1):333–40. doi:10.4049/jimmunol.177.1.333
98. Tas JMJ, Mesin L, Pasqual G, Targ S, Jacobsen JT, Mano YM, et al. Visualizing antibody affinity maturation in germinal centers. Science (2016) 351:1048–54. doi:10.1126/science.aad3439
99. Kuraoka M, Schmidt AG, Nojima T, Feng F, Watanabe A, Kitamura D, et al. Complex antigens drive permissive clonal selection in germinal centers. Immunity (2016) 44(3):542–52. doi:10.1016/j.immuni.2016.02.010
100. Reshetova P, van Schaik BD, Klarenbeek PL, Doorenspleet ME, Esveldt RE, et al. Computational model reveals limited correlation between germinal center B-cell subclone abundancy and affinity: implications for repertoire sequencing. Front Immunol (2017) 8:221. doi:10.3389/fimmu.2017.00221
101. Baumgarth N. How specific is too specific? B-cell responses to viral infections reveal the importance of breadth over depth. Immunol Rev (2013) 255(1):82–94. doi:10.1111/imr.12094
102. Dhar A, Davidsen K, Matsen IVFA, Minin VN. Predicting B cell receptor substitution profiles using public repertoire data. arXiv (2018). arXiv:1802.06406
103. Ralph DK, Matsen FA. Likelihood-based inference of B cell clonal families. PLoS Comput Biol (2016) 12(10):e1005086. doi:10.1371/journal.pcbi.1005086
104. Wiehe K, Bradley T, Meyerhoff RR, Hart C, Williams WB, Easterhoff D, et al. Functional relevance of improbable antibody mutations for HIV broadly neutralizing antibody development. Cell Host Microbe (2018) 23(6):759–65.e6. doi:10.1016/j.chom.2018.04.018
105. Julian MC, Li L, Garde S, Wilen R, Tessier PM. Efficient affinity maturation of antibody variable domains requires co-selection of compensatory mutations to maintain thermodynamic stability. Sci Rep (2017) 7. doi:10.1038/srep45259
106. Scheepers C, Shrestha RK, Lambson BE, Jackson KJL, Wright IA, Naicker D, et al. Ability to develop broadly neutralizing HIV-1 antibodies is not restricted by the germline Ig gene repertoire. J Immunol (2015) 194(9):4371–8. doi:10.4049/jimmunol.1500118
107. Corcoran MM, Phad GE, Vázquez Bernat N, Stahl-Hennig C, Sumida N, Persson MAA, et al. Production of individualized V gene databases reveals high levels of immunoglobulin genetic diversity. Nat Commun (2016) 20(7):13642. doi:10.1038/ncomms13642
108. Luo S, Yu JA, Song YS. Estimating copy number and allelic variation at the immunoglobulin heavy chain locus using short reads. PLoS Comput Biol (2016) 12(9):e1005117. doi:10.1371/journal.pcbi.1005117
109. Yu Y, Ceredig R, Seoighe C. A database of human immune receptor alleles recovered from population sequencing data. J Immunol (2017) 198(5):2202–10. doi:10.4049/jimmunol.1601710
110. Ohm-Laursen L, Nielsen M, Larsen SR, Barington T. No evidence for the use of DIR, D-D fusions, chromosome 15 open reading frames or VH replacement in the peripheral repertoire was found on application of an improved algorithm, JointML, to 6329 human immunoglobulin H rearrangements. Immunology (2006) 119(2):265–77. doi:10.1111/j.1365-2567.2006.02431.x
111. Shlomchik MJ, Marshak-Rothstein A, Wolfowicz CB, Rothstein TL, Weigert MG. The role of clonal selection and somatic mutation in autoimmunity. Nature (1987) 328(6133):805–11. doi:10.1038/328805a0
112. Lossos IS, Tibshirani R, Narasimhan B, Levy R. The inference of antigen selection on Ig genes. J Immunol (2000) 165(9):5122–6. doi:10.4049/jimmunol.165.9.5122
113. Bose B, Sinha S. Problems in using statistical analysis of replacement and silent mutations in antibody genes for determining antigen-driven affinity selection. Immunology (2005) 116(2):172–83. doi:10.1111/j.1365-2567.2005.02208.x
114. Dunn-Walters DK, Spencer J. Strong intrinsic biases towards mutation and conservation of bases in human IgVH genes during somatic hypermutation prevent statistical analysis of antigen selection. Immunology (1998) 95(3):339–45. doi:10.1046/j.1365-2567.1998.00607.x
115. Hershberg U, Uduman M, Shlomchik MJ, Kleinstein SH. Improved methods for detecting selection by mutation analysis of Ig V region sequences. Int Immunol (2008) 20(5):683–94. doi:10.1093/intimm/dxn026
116. Uduman M, Yaari G, Hershberg U, Stern JA, Shlomchik MJ, Kleinstein SH. Detecting selection in immunoglobulin sequences. Nucleic Acids Res (2011) 39(Web Server issue):W499–504. doi:10.1093/nar/gkr413
117. Yaari G, Uduman M, Kleinstein SH. Quantifying selection in high-throughput immunoglobulin sequencing data sets. Nucleic Acids Res (2012) 40(17):e134. doi:10.1093/nar/gks457
118. Shahaf G, Barak M, Zuckerman NS, Swerdlin N, Gorfine M, Mehr R. Antigen-driven selection in germinal centers as reflected by the shape characteristics of immunoglobulin gene lineage trees: a large-scale simulation study. J Theor Biol (2008) 255(2):210–22. doi:10.1016/j.jtbi.2008.08.005
119. Uduman M, Shlomchik MJ, Vigneault F, Church GM, Kleinstein SH. Integrating B cell lineage information into statistical tests for detecting selection in Ig sequences. J Immunol (2014) 192(3):867–74. doi:10.4049/jimmunol.1301551
120. Yaari G, Benichou JIC, Vander Heiden JA, Kleinstein SH, Louzoun Y. The mutation patterns in B-cell immunoglobulin receptors reflect the influence of selection acting at multiple time-scales. Philos Trans R Soc Lond B Biol Sci (2015):370. doi:10.1098/rstb.2014.0242
121. MacDonald CM, Boursier L, D’Cruz DP, Dunn-Walters DK, Spencer J. Mathematical analysis of antigen selection in somatically mutated immunoglobulin genes associated with autoimmunity. Lupus (2010) 19(10):1161–70. doi:10.1177/0961203310367657
122. Burkovitz A, Sela-Culang I, Ofran Y. Large-scale analysis of somatic hypermutations in antibodies reveals which structural regions, positions and amino acids are modified to improve affinity. FEBS J (2014) 281(1):306–19. doi:10.1111/febs.12597
123. Burnett DL, Langley DB, Schofield P, Hermes JR, Chan TD, Jackson J, et al. Germinal center antibody mutation trajectories are determined by rapid self/foreign discrimination. Science (2018) 360(6385):223–6. doi:10.1126/science.aao3859
124. Kepler TB, Munshaw S, Wiehe K, Zhang R, Yu J-S, Woods CW, et al. Reconstructing a B-cell clonal lineage. II. Mutation, selection, and affinity maturation. Front Immunol (2014) 5:170. doi:10.3389/fimmu.2014.00170
125. Rappuoli R, Bottomley MJ, D’Oro U, Finco O, Gregorio ED. Reverse vaccinology 2.0: human immunology instructs vaccine antigen design. J Exp Med (2016) 213(4):469–81. doi:10.1084/jem.20151960
126. Burton DR. What are the most powerful immunogen design vaccine strategies? Reverse vaccinology 2.0 shows great promise. Cold Spring Harb Perspect Biol (2017) 9(11):a030262. doi:10.1101/cshperspect.a030262
127. Haynes BF, Kelsoe G, Harrison SC, Kepler TB. B-cell-lineage immunogen design in vaccine development with HIV-1 as a case study. Nat Biotechnol (2012) 30(5):423–33. doi:10.1038/nbt.2197
128. Kwong PD, Mascola JR. Human antibodies that neutralize HIV-1: identification, structures, and B cell ontogenies. Immunity (2012) 37(3):412–25. doi:10.1016/j.immuni.2012.08.012
129. Kwong PD, Mascola JR. HIV-1 vaccines based on antibody identification, B cell ontogeny, and epitope structure. Immunity (2018) 48(5):855–71. doi:10.1016/j.immuni.2018.04.029
130. Doria-Rose NA, Joyce MG. Strategies to guide the antibody affinity maturation process. Curr Opin Virol (2015) 11:137–47. doi:10.1016/j.coviro.2015.04.002
131. Wu X, Zhou T, Zhu J, Zhang B, Georgiev I, Wang C, et al. Focused evolution of HIV-1 neutralizing antibodies revealed by structures and deep sequencing. Science (2011) 333(6049):1593–602. doi:10.1126/science.1207532
132. Scheid JF, Mouquet H, Ueberheide B, Diskin R, Klein F, Oliveira TYK, et al. Sequence and structural convergence of broad and potent HIV antibodies that mimic CD4 binding. Science (2011) 333(6049):1633–7. doi:10.1126/science.1207227
133. Hwang JK, Wang C, Du Z, Meyers RM, Kepler TB, Neuberg D, et al. Sequence intrinsic somatic mutation mechanisms contribute to affinity maturation of VRC01-class HIV-1 broadly neutralizing antibodies. Proc Natl Acad Sci U S A (2017) 114(32):8614–9. doi:10.1073/pnas.1709203114
134. Chuang G-Y, Zhou J, Rawi R, Shen C-H, Sheng Z, West AP, et al. Structural survey of HIV-1-neutralizing antibodies targeting Env trimer delineates epitope categories and suggests vaccine templates. bioRxiv (2018). doi:10.1101/312579
135. Scheid JF, Mouquet H, Feldhahn N, Seaman MS, Velinzon K, Pietzsch J, et al. Broad diversity of neutralizing antibodies isolated from memory B cells in HIV-infected individuals. Nature (2009) 458(7238):636–40. doi:10.1038/nature07930
136. Breden F, Lepik C, Longo NS, Montero M, Lipsky PE, Scott JK. Comparison of antibody repertoires produced by HIV-1 infection, other chronic and acute infections, and systemic autoimmune disease. PLoS One (2011) 6(3):e16857. doi:10.1371/journal.pone.0016857
137. Moody MA, Yates NL, Amos JD, Drinker MS, Eudailey JA, Gurley TC, et al. HIV-1 gp120 vaccine induces affinity maturation in both new and persistent antibody clonal lineages. J Virol (2012) 86(14):7496–507. doi:10.1128/JVI.00426-12
138. Scherer EM, Smith RA, Simonich CA, Niyonzima N, Carter JJ, Galloway DA. Characteristics of memory B cells elicited by a highly efficacious HPV vaccine in subjects with no pre-existing immunity. PLoS Pathog (2014) 10(10):e1004461. doi:10.1371/journal.ppat.1004461
139. Georgiev IS, Rudicell RS, Saunders KO, Shi W, Kirys T, McKee K, et al. Antibodies VRC01 and 10E8 neutralize HIV-1 with high breadth and potency even with Ig-framework regions substantially reverted to germline. J Immunol (2014) 192(3):1100–6. doi:10.4049/jimmunol.1302515
140. Jardine JG, Sok D, Julien J-P, Briney B, Sarkar A, Liang C-H, et al. Minimally mutated HIV-1 broadly neutralizing antibodies to guide reductionist vaccine design. PLoS Pathog (2016) 12(8):e1005815. doi:10.1371/journal.ppat.1005815
141. Doria-Rose NA, Bhiman JN, Roark RS, Schramm CA, Gorman J, Chuang G-Y, et al. New member of the V1V2-directed CAP256-VRC26 lineage that shows increased breadth and exceptional potency. J Virol (2016) 90(1):76–91. doi:10.1128/JVI.01791-15
142. Bonsignori M, Kreider EF, Fera D, Meyerhoff RR, Bradley T, Wiehe K, et al. Staged induction of HIV-1 glycan-dependent broadly neutralizing antibodies. Sci Transl Med (2017) 9(381):eaai7514. doi:10.1126/scitranslmed.aai7514
143. Kleinstein SH, Singh JP. Why are there so few key mutant clones? The influence of stochastic selection and blocking on affinity maturation in the germinal center. Int Immunol (2003) 15(7):871–84. doi:10.1093/intimm/dxg085.sgm
144. Briney B, Sok D, Jardine JG, Kulp DW, Skog P, Menis S, et al. Tailored immunogens direct affinity maturation toward HIV neutralizing antibodies. Cell (2016) 166(6):1459–70.e11. doi:10.1016/j.cell.2016.08.005
145. Tian M, Cheng C, Chen X, Duan H, Cheng H-L, Dao M, et al. Induction of HIV neutralizing antibody lineages in mice with diverse precursor repertoires. Cell (2016) 166(6):1471–84.e18. doi:10.1016/j.cell.2016.07.029
Keywords: somatic hypermutation, hot spot motifs, affinity maturation, substitution profiles, vaccine design
Citation: Schramm CA and Douek DC (2018) Beyond Hot Spots: Biases in Antibody Somatic Hypermutation and Implications for Vaccine Design. Front. Immunol. 9:1876. doi: 10.3389/fimmu.2018.01876
Received: 31 May 2018; Accepted: 30 July 2018;
Published: 14 August 2018
Edited by:
Gur Yaari, Bar-Ilan University, IsraelReviewed by:
Andrew M. Collins, University of New South Wales, AustraliaMasaki Hikida, Akita University, Japan
Copyright: © 2018 Schramm and Douek. This is an open-access article distributed under the terms of the Creative Commons Attribution License (CC BY). The use, distribution or reproduction in other forums is permitted, provided the original author(s) and the copyright owner(s) are credited and that the original publication in this journal is cited, in accordance with accepted academic practice. No use, distribution or reproduction is permitted which does not comply with these terms.
*Correspondence: Chaim A. Schramm, Y2hhaW0uc2NocmFtbUBuaWguZ292;
Daniel C. Douek, ZGRvdWVrQG1haWwubmloLmdvdg==