- 1Department of Urology, The People’s Hospital of Zhongshan, Zhongshan, China
- 2Department of Urology, Weifang People’s Hospital, Weifang, China
- 3Department of Urology, The First Affiliated Hospital of Harbin Medical University, Harbin, China
- 4Department of Urology, The First Affiliated Hospital of Sun Yat-sen University, Guangzhou, China
- 5Department of Oncology, The People’s Hospital of Zhongshan, Zhongshan, China
Opa interacting protein 5 (OIP5), overexpressed in some types of human cancers, has been reported to be associated with the carcinogenesis of human cancer. However, its contribution to cancer immunity remains unknown. Furthermore, the relationship between OIP5 and cancer immunity remains uncertain. In our research, we explored the different expression of OIP5 between 539 ccRCC and 72 normal renal tissues base on TCGA data set. We analyzed the associations between OIP5 expression with ccRCC progression and survival. Next, we compared immune cell profiles in cancer tissues and normal tissues in the Cancer Genome Atlas (TCGA) ccRCC cohort. We found that the level of immune cell infiltration was correlated with the copy number of OIP5 gene in ccRCC. The effect of OIP5 on immune activity was verified by Gene Set Enrichment Analysis of RNA-seq data from 32 ccRCC cell lines in the public database. Moreover, a pathway enrichment analysis of 49 OIP5-associated immunomodulators demonstrated the involvement of the T cell receptor signaling pathway, the JAK-STAT signaling pathway, the NF-kappa B signaling pathway and the primary immunodeficiency pathway. In addition, using OIP5-associated immunomodulators, we constructed multiple-gene risk prediction signatures using the Cox regression model. Our results provided insights into the role of OIP5 in tumor immunity and revealed that OIP5 may be a potential immunotherapeutic target for ccRCC. Designated immune signature is a promising prognostic biomarker in ccRCC.
Introduction
Renal cell carcinoma accounts for 4.2% of all new cancer cases and is one of the most common urinary tumors in the world. In 2019, it was estimated that there were 73,750 new diagnoses of renal cancer and 14,830 will die of this disease in the United States (1). Clear cell renal cell carcinoma (ccRCC) accounts for 85% of all renal cancers and is the most common type of renal cancer (2). In most cases, radiotherapy and chemotherapy are less effective in the treatment of ccRCC. Radical nephrectomy is the first choice for early localized renal cancer, although it still does not solve the problem of distant metastasis or tumor recurrence in more than 20% of patients after surgery (3). Moreover, the prognosis of ccRCC with metastasis is relatively poor, with a median survival time of only about 10 months (4).
As a new treatment for ccRCC, immunotherapy is complementary to surgery, chemotherapy, and targeted therapies. In the past few decades, the treatment of renal cancer has undergone a transition from a nonspecific immune approach to vascular endothelial growth factor (VEGF) targeting therapy, and now immunotherapy is one of the newest treatment methods for renal cancer (5). In recent years, immunotherapy has made significant progress in the treatment of metastatic renal cell carcinoma. Compared to non-specific immunotherapies for IL-2 or IL-6, immune checkpoint blockade (ICB) therapy has performed very well in the treatment of advanced RCC since it was approved as second-line therapy in 2015. A clinical trial demonstrated that the PD-L1 inhibitor avelumab and the PD-1 inhibitor pembrolizumab plus axinib with that of sunitinib had superior advantages contrasted to sunitinib in RCC (6). However, it is estimated that only about 20% of patients will benefit from immunotherapy (7). Therefore, it is necessary to use predictive biomarkers to initially assess a patient’s potential response to ICB treatment.
OIP5 was first identified as an Opa (Neisseria gonorrhoeae opacity-associated) interacting protein by yeast two-hybrid analysis (8). OIP5 encodes a 25 kDa protein with a coiled domain, also named MIS18Beta and Lint-25. Notably, abnormal regulation of OIP5 is commonly seen in a variety of tumors, such as glioblastoma (9), bladder cancer (10), breast cancer (11) and gastric cancer (12). Currently, it has been proved that the up-regulation of OIP5 plays a crucial role in development of tumors, which has been confirmed in vivo and in vitro. We have reported that OIP5 was overexpression in ccRCC in 2013 (13). However, up to now, the specific mechanism of OIP5 in ccRCC has not been studied, and the relationship between OIP5 and immunity has not been explored. In this study, using the TCGA database, we further proved that OIP5 was highly expressed in ccRCC, and that OIP5 was significantly correlated with the grade of ccRCC and the prognosis of patients. Then, we systematically assessed the status of lymphocytes and elucidate the relationship between OIP5 and ccRCC immunity, as well as the signaling pathways that regulate OIP5-mediated immune response. Finally, we used OIP5-associated immunomodulators to generate prognostic immune signatures.
Materials and Methods
Acquirement of ccRCC Expression Profiles From TCGA Datasets and Bioinformatics Analysis
We obtained ccRCC dataset from the TCGA project (https://cancergenome.nih.gov/). The ccRCC dataset contained 539 cancerous and 72 normal tissues, accompanied by clinical information. RNA expression data and clinic data were further processed using R software.
Detection of Tumor Infiltrating Immune Cells in TCGA Renal Clear Cell Carcinoma
CIBERSORT (http://cibersort.stanford.edu/) is a kind of analysis tool, which provides an estimation of the abundances of immune cells in mixed cell populations, using gene expression data (14). We identified and quantified 22 immune cells in tissues, including seven T cell types, naïve and memory B cells, plasma cells, NK cells, and myeloid subsets, by Estimating Relative Subsets Of RNA Transcripts (CIBERSORT) (14, 15). LM22 is adopted, which is a leukocyte gene signature matrix. This document contains 547 genes that distinguish 22 human hematopoietic cell phenotypes (14). Using CIBERSORT L22 as a reference, we investigated the mRNA expression matrix with the CIBERSORT R script obtained from the CIBERSORT website。
Relationship Between OIP5 and Tumor Immune Cell Infiltration
Tumor Immune Estimation Resource furnished a detailed data of tumor immune cells of pan-cancer (cistrome.dfci.harvard.edu/TIMER/) (16). This website provides several modules, including Survival, Gene, Mutations, Diff Exp, SCNA, Estimation and Correlation. We investigated the relationship between immune cell infiltration and OIP5 copy numbers.
Gene Set Enrichment Analysis
Gene Set Enrichment Analysis (GSEA) is a method to determine whether predefined gene sets are differentially expressed in different phenotypes. The Cancer Cell Line Encyclopedia (CCLE) dataset is sponsored and maintained by the Broad Institute, the Novartis Biomedical Research Institute and the Genomics Institute of the Novartis Research Foundation. This database contains most of the common cancer types, including kidney cancer, breast cancer, liver cancer, stomach cancer and lung cancer. The RNAseq data of 32 ccRCC cell lines was downloaded from the CCLE website. The expression level of OIP5 in various renal clear cell carcinomas was extracted for further analysis. Taking the average expression of OIP5 gene as a cutoff value, all ccRCC cell lines were divided into OIP5high group and OIP5low group. In this study, GSEA was used to explore the significant signal pathways associated with the OIP5 gene.
Immunomodulators
TISIDB database (http://cis.hku.hk/TISIDB) was adopted to further research the relationships between the expression of OIP5 and immunomodulators. The TISIDB database covers the interaction between tumors and the immune system, including high-throughput screening techniques, 988 reported immune-related anti-tumor genes, molecular maps and paracancerous data sets, as well as a variety of immune data resources obtained from seven public databases (17). We recruited immunoinhibitors and immunostimulators which were significantly associated with OIP5 regarding gene expression (Spearman correlation test, P < 0.05). Then, we analyzed the OIP5-associated immunomodulators using the cBioPortal for Cancer Genomics (www.cbioportal.org). Using two WEB-based GEne SeT AnaLysis Toolkit (http://www.webgestalt.org/) and web-based tools (https://string-db.org/) (18, 19), the resulting protein network was subjected to Kyoto Encyclopedia of Genes and Genomes (KEGG) pathway enrichment analysis and GO annotation (Figure 1).
Survival Analysis
Next, we developed a prognostic multiple immune gene signature from OIP5-related immunomodulators. Akaike Information Criterion was used to select stepwise variables in Cox model (20). After identifying the immune genes, the prognostic index, namely risk score, was obtained: risk score =β1x1 + β2x2 +… + βixi. In this algorithm, xi is the expression level of each gene, and βi is the risk coefficient of each gene in the Cox model. Then, Kaplan–Meier survival curve was performed to analysis the relationship between risk score and patient survival (Figure 1).
Statistical Analysis
All statistical analysis were conducted by R software 3.6.2. (R Foundation for Statistical Computing, Vienna, Austria) and IBM SPSS Statistics 26.0 (IBM, Inc., Armonk, NY, USA). Comparisons for continuous variables among groups ≥3 were performed by One-way ANOVA. P < 0.05 was considered to be statistically significant.
Result
Increased Expression of OIP5 in ccRCC Tissues
The gene expressions data of 539 ccRCC and 72 normal renal tissues were downloaded from TCGA. Our results revealed the OIP5 gene was highly expressed in ccRCC compared to normal renal tissues (P < 0.001) (Figure 2A). Furthermore, the paired test also confirmed that OIP5 was highly expressed in ccRCC (N=72, P < 0.001) (Figure 2B).
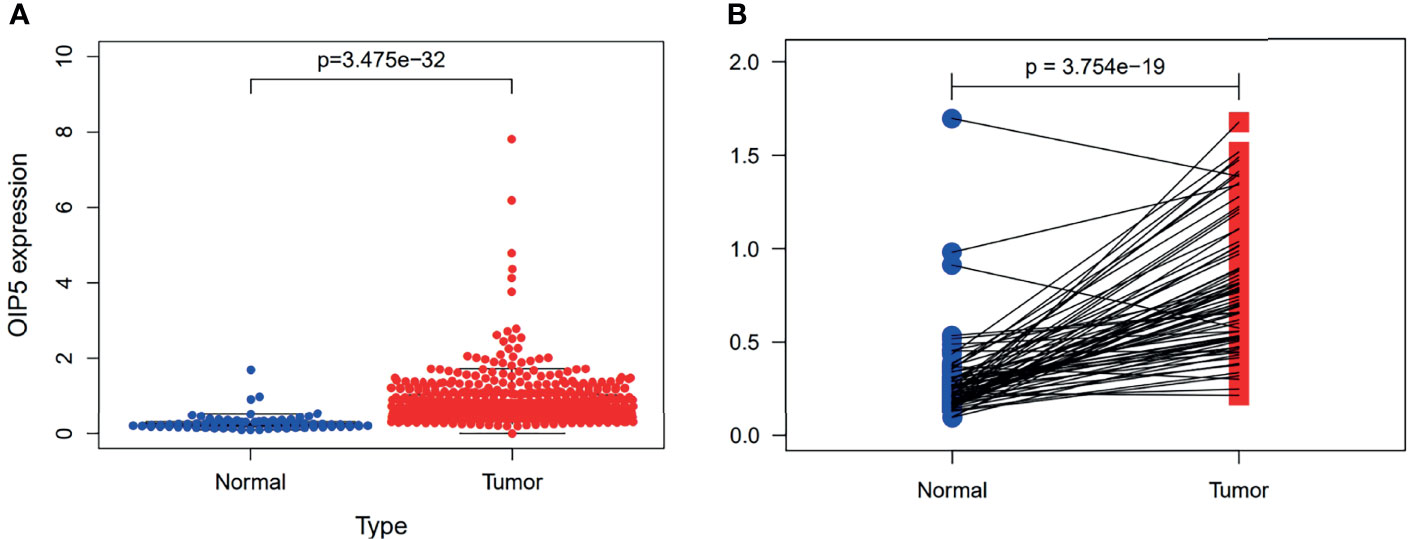
Figure 2 Expression of OIP5 in 539 ccRCC and 72 normal renal tissues. (A) The different expression of OIP5 in 539 ccRCC and 72 normal renal tissues. (B) The differential expression of OIP5 in 72 pairs of ccRCC tissues and adjacent non-tumor tissues.
Relationship Between OIP5 Expression and the Clinicopathological Features of ccRCC
A total of 539 ccRCC tissues with OIP5 expression data across all patient characteristics were analyzed from TCGA. As shown in Figure 3, high expression of OIP5 gene correlated significantly with the patient’s age (P =0.026), the tumor histological grade (P <0.001), T classification (P <0.001), N classification (P <0.001), M classification (P <0.001) and clinical stage (P <0.001).
Impact of OIP5 Overexpression on Overall Survival in ccRCC
Kaplan-Meier survival analysis demonstrated that ccRCC with OIP5-high expression had a worse prognosis than that with OIP5-low expression (Figure 4, P = 0.008). To explore the impact of OIP5 overexpression and other clinicopathological parameters in ccRCC patients, we performed univariate and multivariate analyses. The univariate analysis revealed that OIP5 overexpression (P < 0.001), age (P < 0.001), grade (P < 0.001), stage (P < 0.001), T classification (P < 0.001) and M classification (P < 0.001) were all positively associated with a poor prognosis (Table 1). Besides, multivariate analyses showed that OIP5 overexpression (P = 0.037), age (P < 0.001), grade (P = 0.001) and stage (P = 0.046) were all independent predictors of an unfavorable prognosis (Table 1).
The Landscape of Infiltrating Immune Cells in ccRCC and Normal Renal Tissues
We first extracted and processed signature gene expression profiles using the CIBERSORT method to systematically describe the patterns of immune cells. After excluding samples with P ≥ 0.05, the landscape of immune cells infiltration in ccRCC and normal renal tissue were shown in Figure 5A. Compared to normal renal tissues, the proportions of T cells CD8, T cells follicular helper, T cells regulatory (Tregs), Macrophages M0 and Macrophages M1 were significantly overexpressed, while B cells naïve, Plasma cells, T cells CD4 naïve, T cells CD4 memory resting, Monocytes, Dendritic cells resting and Mast cells resting decreased in ccRCC (Figure 5B). Figure 5C showed different patterns of infiltrating immune cells in ccRCC compared with normal tissue. Further, different correlation patterns among immune cells were detected in ccRCC (Figure 5D).
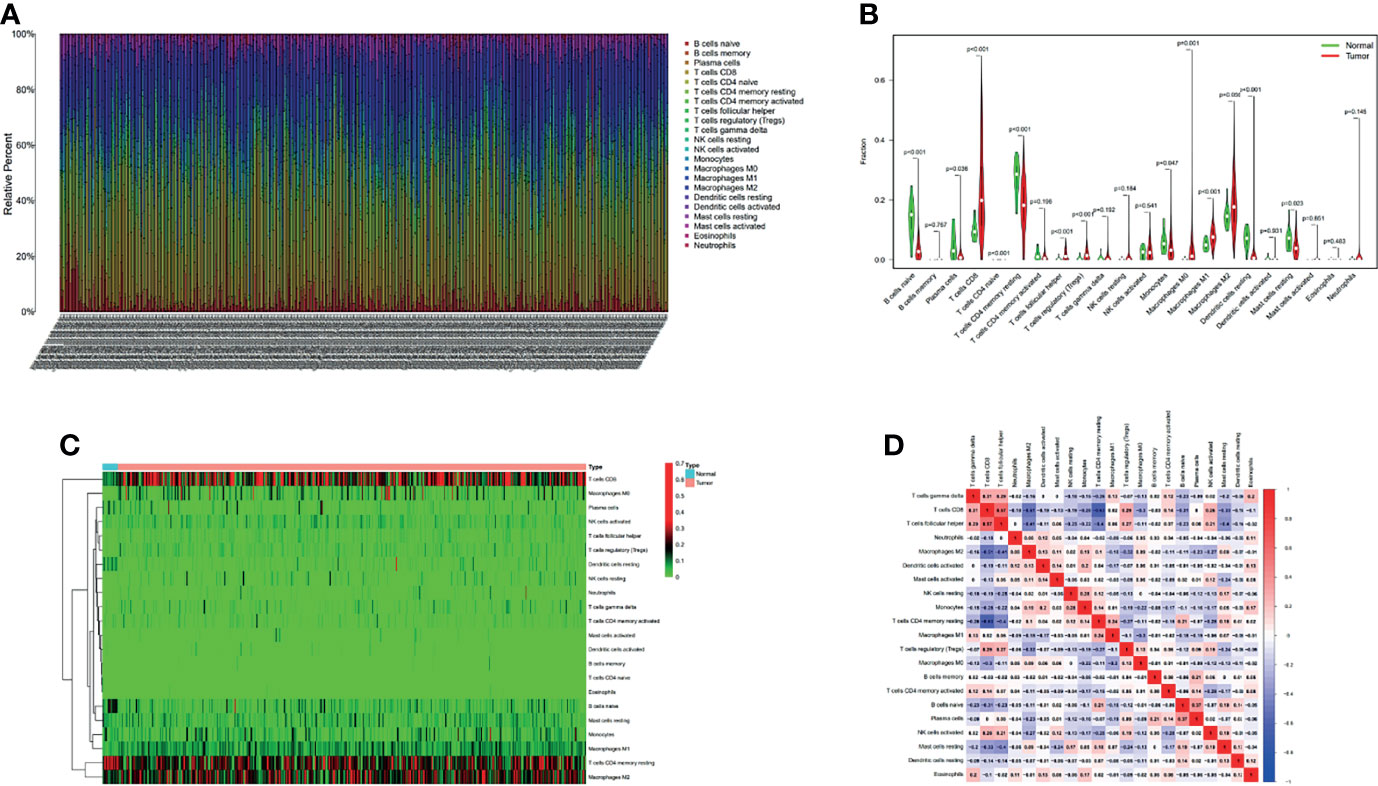
Figure 5 The proportion of 22 immune cell infiltrates was assessed using Cell Type Identification By Estimating Relative Subsets Of RNA Transcripts method in ccRCC cohort of TCGA (A). Heatmaps and violin plots showed the differences in the immune cell distribution between malignant (red) and normal (blue) tissues in ccRCC cohorts (B, C). Different correlation patterns among immune cell subsets in ccRCC cohorts (D).
Relationship Between OIP5 and Immunity
We explored the Relationship between OIP5 and the immune system. We found that the expression level of OIP5 was positively correlated with T cells CD8 (P < 0.001), T cells CD4 memory activated (P = 0.006), T cells follicular helper (P < 0.001), T cells regulatory (Tregs) (P < 0.001), T cells gamma delta (P = 0.001) and Macrophages M1 (P = 0.016). While, the expression level of OIP5 was negatively correlated with B cells naïve (P < 0.001), Plasma cells (P = 0.014), T cells CD4 memory resting (P = 0.001), NK cells resting (P = 0.001), Dendritic cells activated (P = 0.01) and Mast cells resting (P < 0.001) (Figure 6). At the same time, we found that the levels of several immune cell infiltration appeared to correlate with altered copy number of OIP5 gene, including B cell, CD4+T cell, CD8+T cell, NK cell, T cell regulatory (Tregs), Neutrophil, Monocyte, Mast cell activated and Endothelial cell in ccRCC (Figure 7).
Then we analyzed the RNAseq data of 32 ccRCC cell lines downloaded from the CCLE website. The KEGG results suggested that OIP5 may regulate immunity through Homologous recombination, Human T−cell leukemia virus 1 infection, Fanconi anemia pathway and Nucleotide excision repair signaling pathway (Figure 8). Further, GSEA analysis showed that OIP5 was associated with several immune-related signaling pathways, including the homologous recombination signaling pathway (NES = 1.75, P =0.004), autoimmune thyroid disease signaling pathway (NES = -1.68, P = 0.004), primary immunodeficiency signaling pathway (NES = -1.58, P = 0.021), and intestinal immune network for IgA production (NES = -1.54, P = 0.03) (Figure 9).
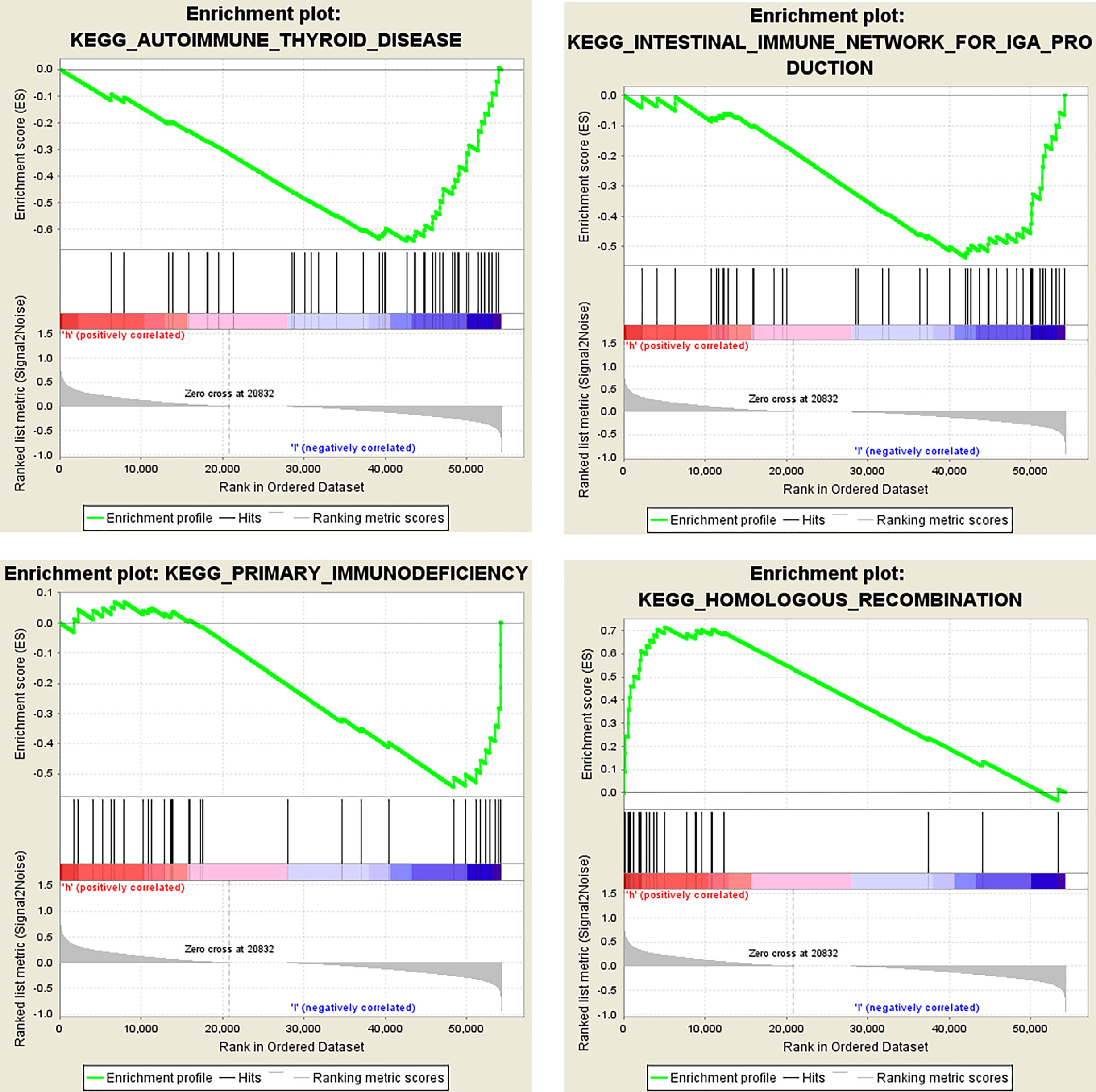
Figure 9 Dissection of OIP5-associated immune signaling pathways by Gene Set Enrichment Analysis of 32 ccRCC cell lines from the Cancer Cell Line Encyclopedia database.
We further investigated the signaling pathways that OIP5 may be involved in regulating the immune response to ccRCC. We identified 34 immunostimulators (CD27, CD28, CD40, CD40LG, CD48, CD70, CD80, CD86, CD276, CXCR4, ENTPD1, ICOS, IL2RA, IL6, IL6R, KLRC1, KLRK1, LTA, MICB, NT5E, RAET1E, TMIGD2, TNFRSF4, TNFRSF9, TNFRSF17, TNFRSF18, TNFSF4, TNFSF9, TNFSF13, TNFSF13B, TNFSF14, TNFSF15, ULBP1 and TNFRSF8) (Figure 10A) and 15 immunoinhibitors (BTLA, CD96, CD244, CSF1R, CTLA4, HAVCR2, IL10, IL10RB, KDR, LAG3, LGALS9, PDCD1, PDCD1LG2, TGFB1 and TIGIT) (Figure 10B) significantly associated with OIP5 in ccRCC. We also screened the protein-protein interaction (PPI) network in the String database using these immunomodulators (Figure 10C). Next, we investigated the enriched GO terms and KEGG pathways using these immunomodulators (Figures 10D, E). The results of KEGG pathways showed that T cell receptor signaling pathway, primary immunodeficiency, Natural killer cell mediated cytotoxicity, NF-kappa B signaling pathway, JAK-STAT signaling pathway and Cytokine-cytokine receptor interaction were related to OIP5-mediated immune events (Figure 10E).
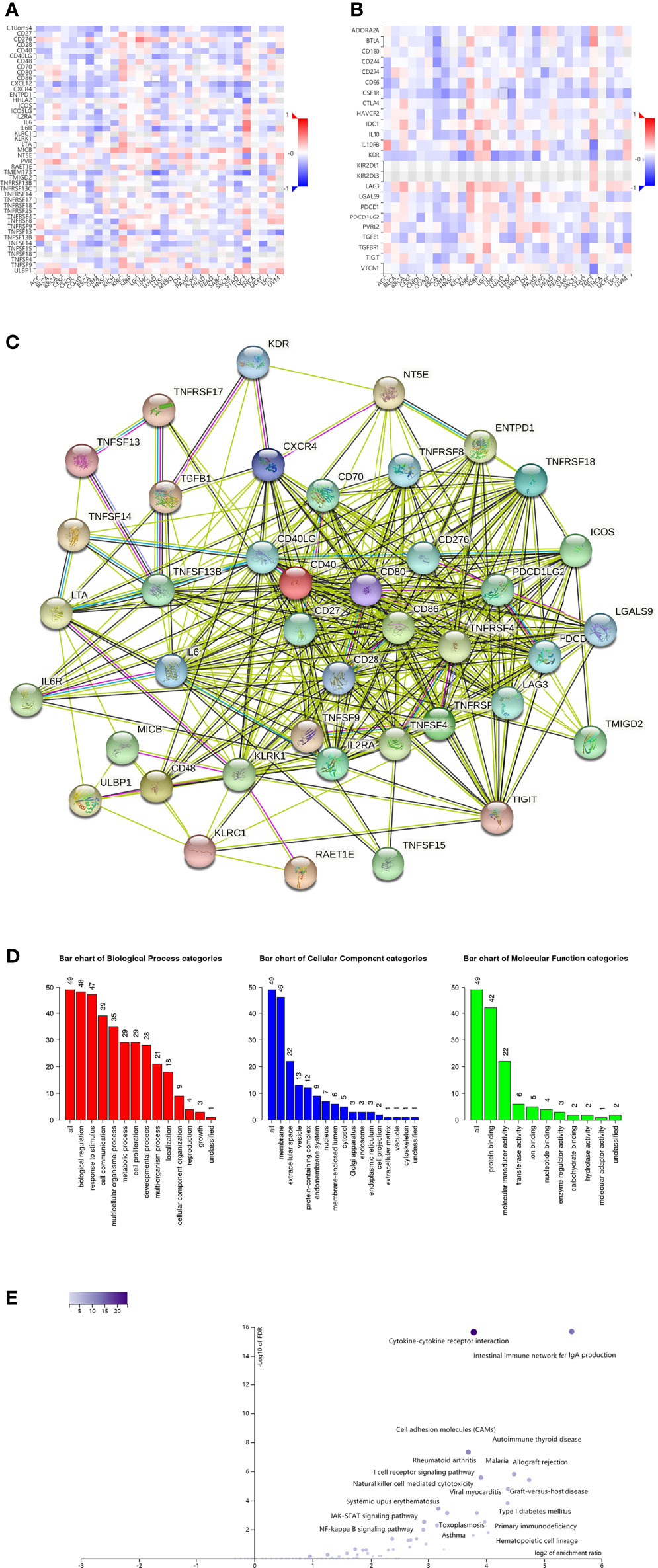
Figure 10 Identification and analysis of immunomodulators associated with the OIP5 gene. (A) The heatmap of relationship between the immunostimulators and the OIP5 gene in ccRCC. (B) The heatmap of relationship between the immunoinhibitors and the OIP5 gene in ccRCC. (C) Protein–protein network of 49 OIP5-associated immunomodulators in ccRCC, plotted by the STRING online tool. (D) Gene Ontology annotation of 49 OIP5-associated immunomodulators in ccRCC. (E) Kyoto Encyclopedia of Genes and Genomes pathway analysis of 49 OIP5-associated immunomodulators in ccRCC.
The Effect of OIP5-Related Immunomodulators on Prognosis of ccRCC
In order to investigate the prognostic value of OIP5-related immunomodulators in ccRCC, the univariate Cox regression analysis was performed on these genes. We identified 14 genes that were significantly associated with the prognosis of ccRCC patients (Figure 11A). Then, multivariate stepwise Cox regression analysis was performed for these variables. This method produced an optimal 7-gene prognostic signature in ccRCC (Figure 11B). The risk scores were obtained by adding up the product of expression value and coefficient of each gene. The Kaplan-Meier survival curve demonstrated that patients with high-risk scores had significantly shorter survival than those with low-risk scores (log-rank test, P < 0.001) (Figure 11C). We also investigated the distributions of signature gene expression profiles, risk scores and survival statuses for ccRCC (Figures 11D–F).
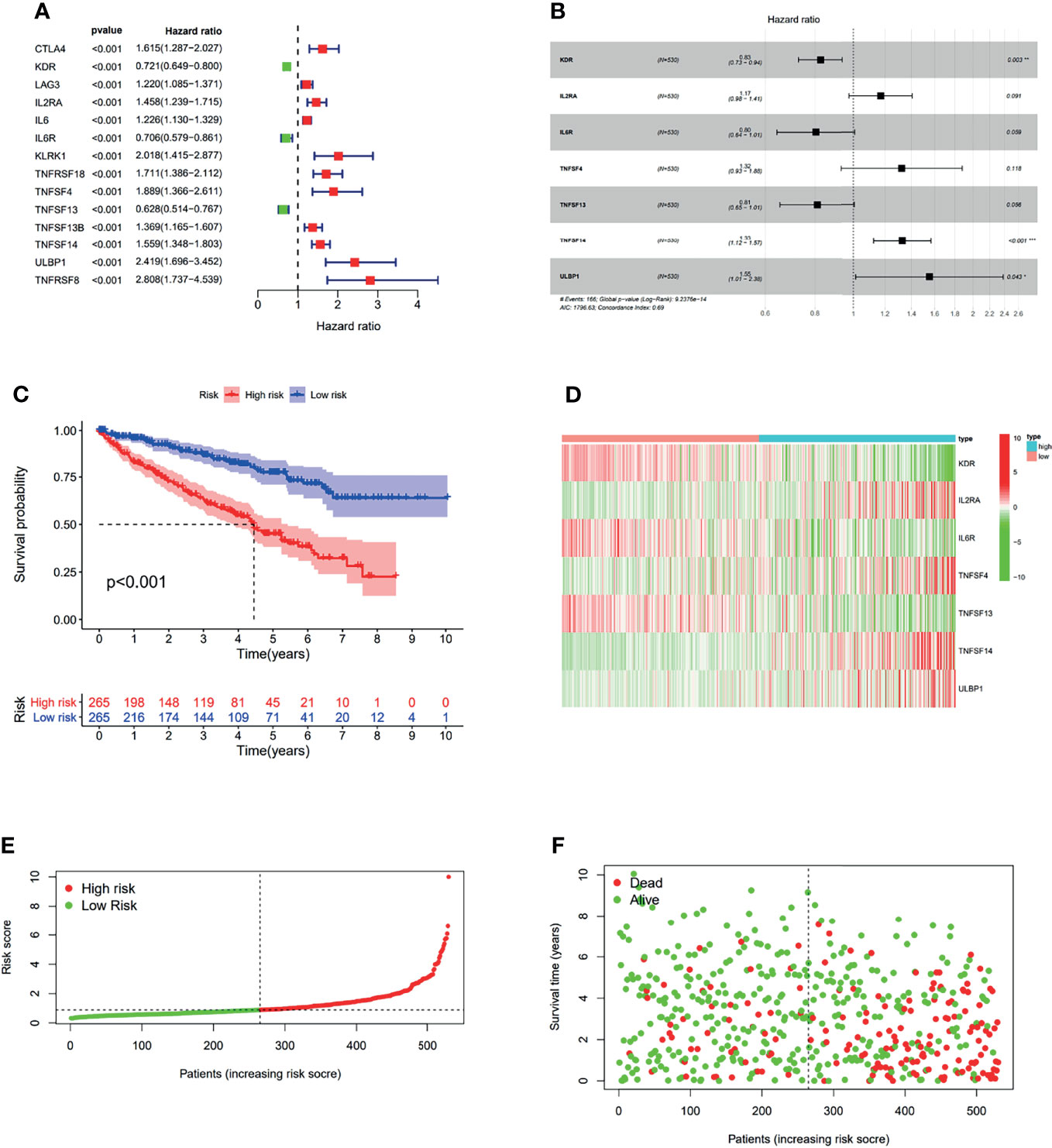
Figure 11 The development of prognostic gene signatures based on 49 OIP5-associated immunomodulators. The hazard ratios of genes integrated into the prognostic signatures are shown in the forest plots for ccRCC (A, B). Kaplan–Meier curves for ccRCC regarding the risk scores (C). Distribution of risk scores, along with survival statuses, and gene expression profiles for ccRCC (D–F). *P < 0.05, **P < 0.005, ***P < 0.001.
Discussion
In the United States, there are about 64,000 new cases and nearly 14,000 deaths due to renal cell cancer each year (1). Therefore, there is an urgent need to find an effective diagnosis and treatment for renal cancer. In the past decade, tumor microenvironment (TME) has rapidly attracted the attention of the oncology community, and is considered to be a key factor affecting the development, treatment resistance and prognosis of tumors (21–23). Previous studies have demonstrated that tumor-infiltrating immune cells (TICS) in TME can be used as a valid indicator of prognosis and therapeutic efficacy (24). In this research, we found that OIP5 was closely related to the immunity of ccRCC. The expression of OIP5 gene was related to the level of immune cell infiltration and immunomodulators. Using stepwise Cox regression model, we successfully obtained multi-gene risk prediction signals from OIP5-related immunomodulators.
Our results showed that OIP5 was highly expressed in ccRCC and high expression of OIP5 gene was correlated significantly with the patient’s age, the tumor histological grade, T classification, N classification, M classification and clinical stage, which was consistent with our previous findings (13). The results further confirmed the role of the OIP5 as an oncogene in ccRCC. However, the specific mechanism of OIP5 in ccRCC and the relationship between OIP5 and immunity are still unclear. We evaluated the composition of intratumoral immune subsets in individual patients using CIBERSORT analysis, because some immunotherapies have been developed to modulate these cells. Our results showed that the composition of 22 immune subsets in the tumor microenvironment of ccRCC were significantly altered compared to normal kidney tissue. These results suggested that the pattern of intratumoral immune cell infiltration is related to the prognosis of ccRCC.
Importantly, we discovered that OIP5, a putative oncogene, was associated with immune cell infiltration in ccRCC. To our knowledge, this research first demonstrated an association between OIP5 and ccRCC immunity. We found that OIP5 gene copy number was correlated with infiltration levels of B cell, CD4+T cell, CD8+T cell, NK cell, T cell regulatory (Tregs), Neutrophil, Monocyte, Mast cell activated and Endothelial cell in ccRCC.
A deeper analysis of the complexity of TME may discover the advanced biomarkers that can help identify patient populations which respond to current immune checkpoint therapy and help identify new adjuvant therapy targets. The combination with immunotherapy drugs will be an important supplement to reduce the toxic side effects and improve the treatment effect of advanced ccRCC (25). The relationship between tumor cells and the immune system is determined by a complex network of cell–cell interactions. In addition, infiltrating stromal cells and immune cells are the main components of normal cells in tumor tissue. So, the specific immune cell composition of the tumor was closely related to patient’s prognosis. Our research demonstrated that OIP5 could change the components of immune cells of ccRCC.
Further, a KEGG pathway analysis of OIP5-associated immunomodulators showed that the primary immunodeficiency pathway, the JAK-STAT signaling pathway, the T cell receptor signaling pathway and the NF-kappa B signaling pathway might be involved in OIP5-mediated immune response. Previous research revealed that OIP5 could promote metastasis of nasopharyngeal carcinoma cells by promoting EMT via modulation of JAK2/STAT3 signal (26). Although several mechanisms have been identified that prevent the generation of anti-tumor immune responses, the one that has attracted the most attention is the expression of key receptors on the surface of T cells, which prevent full activation of T cells (27–31). In conclusion, it is speculated that OIP5 inhibitors may also enhance tumor immunity from a biological perspective, thus contributing to the anti-tumor efficacy of immune checkpoint blockers.
Consistently, we constructed immune gene signatures for ccRCC with OIP5-associated immunomodulators. The risk scores derived from the gene signatures were dramatically associated with the survival of ccRCC patients. Our research showed that the risk scores derived from OIP5-associated immunomodulators could distinguish risk groups identified by a differential expression of a set of signature genes. The results of our study may improve the development of well-verified signatures for the prognosis of ccRCC.
Despite some merits of our study, there still exists several shortcomings. First, the analysis results from TCGA have not been detected in GEO database. Second, all the analyses were conducted with public datasets. Thus, if possible, further experiments should be conducted in vivo and in vitro to certificate these results.
In general, this study revealed an important relationship between OIP5 gene and tumor immune microenvironments. The prognostic signatures derived from OIP5-associated immunomodulators were independently predictors of overall survival in ccRCC. More studies are needed to verify the oncogenic role and potential mechanism of OIP5 in ccRCC, and more cohorts are needed to improve the predictive accuracy then help the personalized management of ccRCC patients.
Data Availability Statement
The datasets presented in this study can be found in online repositories. The names of the repository/repositories and accession number(s) can be found in the article/supplementary material.
Author Contributions
All authors listed have made a substantial, direct, and intellectual contribution to the work, and approved it for publication.
Funding
Supported by Science and Technology Plan Project of Zhongshan City 2019B1064, Science and Technology Plan Project of Zhongshan City 2018B1031 and Major Science and Technology Plan project of Zhongshan City 2016B1003.
Conflict of Interest
The authors declare that the research was conducted in the absence of any commercial or financial relationships that could be construed as a potential conflict of interest.
Publisher’s Note
All claims expressed in this article are solely those of the authors and do not necessarily represent those of their affiliated organizations, or those of the publisher, the editors and the reviewers. Any product that may be evaluated in this article, or claim that may be made by its manufacturer, is not guaranteed or endorsed by the publisher.
References
1. Siegel RL, Miller KD, Jemal A. Cancer Statistics, 2020. CA Cancer J Clin (2020) 70(1):7–30. doi: 10.3322/caac.21590
2. Choueiri TK, Motzer RJ. Systemic Therapy for Metastatic Renal-Cell Carcinoma. N Engl J Med (2017) 376(4):354–66. doi: 10.1056/NEJMra1601333
3. Ljungberg B, Albiges L, Abu-Ghanem Y, Bensalah K, Dabestani S, Fernández-Pello S, et al. European Association of Urology Guidelines on Renal Cell Carcinoma: The 2019 Update. Eur Urol (2019) 75:799–810. doi: 10.1016/j.eururo.2019.02.011
4. Kasenda B, Larkin J, Gore M. Immunotherapies in Early and Advanced Renal Cell Cancer. Prog Tumor Res (2015) 42:1–10. doi: 10.1159/000436988
5. Barata PC, Rini BI. Treatment of Renal Cell Carcinoma: Current Status and Future Directions. CA Cancer J Clin (2017) 67(6):507–24. doi: 10.3322/caac.21411
6. Motzer RJ, Penkov K, Haanen J, Rini B, Albiges L, Campbell MT, et al. Avelumab Plus Axitinib Versus Sunitinib for Advanced Renal-Cell Carcinoma. N Engl J Med (2019) 380:1103–15. doi: 10.1056/NEJMoa1816047
7. Braun DA, Burke KP, Van Allen EM. Genomic Approaches to Understanding Response and Resistance to Immunotherapy. Clin Cancer Res (2016) 22:5642–50. doi: 10.1158/1078-0432.CCR-16-0066
8. Williams JM, Chen GC, Zhu L, Rest RF. Using the Yeast Two-Hybrid System to Identify Human Epithelial Cell Proteins That Bind Gonococcal Opa Proteins: Intracellular Gonococci Bind Pyruvate Kinase via Their Opa Proteins and Require Host Pyruvate for Growth. Mol Microbiol (1998) 27:171–86. doi: 10.1046/j.1365-2958.1998.00670.x
9. He J, Zhao Y, Zhao E, Wang X, Dong Z, Chen Y, et al. Cancer-Testis Specific Gene OIP5: A Down-Stream Gene of E2F1 That Promotes Tumorigenesis and Metastasis in Glioblastoma by Stabilizing E2F1 Signaling. Neuro Oncol (2018) 20:1173–84. doi: 10.1093/neuonc/noy037
10. He X, Hou J, Ping J, Wen D, He J. Opa Interacting Protein 5 Acts as an Oncogene in Bladder Cancer. J Cancer Res Clin Oncol (2017) 143:2221–33. doi: 10.1007/s00432-017-2485-4
11. Mobasheri MB, Shirkoohi R, Modarressi MH. Cancer/Testis OIP5 and TAF7L Genes Are Up-Regulated in Breast Cancer. Asian Pac J Cancer Prev (2015) 16:4623–8. doi: 10.7314/apjcp.2015.16.11.4623
12. Chun HK, Chung KS, Kim HC, Kang JE, Kang MA, Kim JT, et al. OIP5 Is a Highly Expressed Potential Therapeutic Target for Colorectal and Gastric Cancers. BMB Rep (2010) 43:349–54. doi: 10.5483/bmbrep.2010.43.5.349
13. Gong M, Xu Y, Dong W, Guo G, Ni W, Wang Y, et al. Expression of Opa Interacting Protein 5 (OIP5) Is Associated With Tumor Stage and Prognosis of Clear Cell Renal Cell Carcinoma. Acta Histochem (2013) 115(8):810–5. doi: 10.1016/j.acthis.2013.03.008
14. Newman AM, Liu CL, Green MR, Gentles AJ, Feng W, Xu Y, et al. Robust Enumeration of Cell Subsets From Tissue Expression Profiles. Nat Methods (2015) 12:453–7. doi: 10.1038/nmeth.3337
15. Chen B, Khodadoust MS, Liu CL, Newman AM, Alizadeh AA. Profiling Tumor Infiltrating Immune Cells With CIBERSORT. Methods Mol Biol (2018) 1711:243–59. doi: 10.1007/978-1-4939-7493-1_12
16. Li T, Fan J, Wang B, Traugh N, Chen Q, Liu JS, et al. TIMER: A Web Server for Comprehensive Analysis of Tumor-Infiltrating Immune Cells. Cancer Res (2017) 77:e108–10. doi: 10.1158/0008-5472.CAN-17-0307
17. Ru B, Wong CN, Tong Y, Zhong JY, Zhong SSW, Wu WC, et al. TISIDB: An Integrated Repository Portal for Tumor–Immune System Interactions. Bioinformatics (2019) 35:4200–2. doi: 10.1093/bioinformatics/btz210
18. Zhang B, Kirov S, Snoddy J. WebGestalt: An Integrated System for Exploring Gene Sets in Various Biological Contexts. Nucleic Acids Res (2005) 33:W741–8. doi: 10.1093/nar/gki475
19. Liao Y, Wang J, Jaehnig EJ, Shi Z, Zhang B. WebGestalt 2019: Gene Set Analysis Toolkit With Revamped UIs and APIs. Nucleic Acids Res (2019) 47:W199–205. doi: 10.1093/nar/gkz401
20. Choi I, Wells BJ, Yu C, Kattan MW. An Empirical Approach to Model Selection Through Validation for Censored Survival Data. J BioMed Inform (2011) 44:595–606. doi: 10.1016/j.jbi.2011.02.005
21. Chen F, Zhuang X, Lin L, Yu P, Wang Y, Shi Y, et al. New Horizons in Tumor Microenvironment Biology: Challenges and Opportunities. BMC Med (2015) 13:45. doi: 10.1186/s12916-015-0278-7
22. Wu T, Dai Y. Tumor Microenvironment and Therapeutic Response. Cancer Lett (2017) 387:61–8. doi: 10.1016/j.canlet.2016.01.043
23. Liu Y, Guo J, Huang L. Modulation of Tumor Microenvironment for Immunotherapy: Focus on Nanomaterial-Based Strategies. Theranostics (2020) 10:3099–117. doi: 10.7150/thno.42998
24. Xiong Y, Wang Z, Zhou Q, Zeng H, Zhang H, Liu Z, et al. Identification and Validation of Dichotomous Immune Subtypes Based on Intratumoral Immune Cells Infiltration in Clear Cell Renal Cell Carcinoma Patients. J Immunother Cancer (2020) 8:e000447. doi: 10.1136/jitc-2019-000447
25. George S, Rini BI, Hammers HJ. Emerging Role of Combination Immunotherapy in the First-Line Treatment of Advanced Renal Cell Carcinoma: A Review. JAMA Oncol (2019) 5(3):411–21. doi: 10.1001/jamaoncol.2018.4604
26. Merhi Z, Buyuk E, Berger DS, Zapantis A, Israel DD, Chua S Jr, et al. Leptin Suppresses Anti-Mullerian Hormone Gene Expression Through the JAK2/STAT3 Pathway in Luteinized Granulosa Cells of Women Undergoing IVF. Hum Reprod (2013) 28:1661–9. doi: 10.1093/humrep/det072
27. Sharma P, Hu-Lieskovan S, Wargo JA, Ribas A. Primary, Adaptive, and Acquired Resistance to Cancer Immunotherapy. Cell (2017) 168:707–23. doi: 10.1016/j.cell.2017.01.017
28. Sharma P, Allison JP. The Future of Immune Checkpoint Therapy. Science (2015) 348:56–61. doi: 10.1126/science.aaa8172
29. Topalian SL, Drake CG, Pardoll DM. Immune Checkpoint Blockade: A Common Denominator Approach to Cancer Therapy. Cancer Cell (2015) 27:450–61. doi: 10.1016/j.ccell.2015.03.001
30. Pardoll DM. The Blockade of Immune Checkpoints in Cancer Immunotherapy. Nat Rev Cancer (2012) 12:252–64. doi: 10.1038/nrc3239
Keywords: OIP5, ccRCC, immune cells, prognosis, survival
Citation: Gong M, Li Y, Song E, Li M, Qiu S, Dong W and Yuan R (2022) OIP5 Is a Novel Prognostic Biomarker in Clear Cell Renal Cell Cancer Correlating With Immune Infiltrates. Front. Immunol. 13:805552. doi: 10.3389/fimmu.2022.805552
Received: 30 October 2021; Accepted: 27 January 2022;
Published: 15 February 2022.
Edited by:
Zhangqun Ye, Huazhong University of Science and Technology, ChinaReviewed by:
Haoran Liu, Stanford University, United StatesLuyao Chen, The First Affiliated Hospital of Nanchang University, China
Liangyou Gu, People’s Liberation Army General Hospital, China
Copyright © 2022 Gong, Li, Song, Li, Qiu, Dong and Yuan. This is an open-access article distributed under the terms of the Creative Commons Attribution License (CC BY). The use, distribution or reproduction in other forums is permitted, provided the original author(s) and the copyright owner(s) are credited and that the original publication in this journal is cited, in accordance with accepted academic practice. No use, distribution or reproduction is permitted which does not comply with these terms.
*Correspondence: Wenjing Dong, dmFsZXJpZTAwNjdAMTYzLmNvbQ==; Runqiang Yuan, eXVhbnJ1bnFpYW5nMjAxM0AxNjMuY29t