- 1Tuberculosis Prevention and Control Key Laboratory/Beijing Key Laboratory of New Techniques of Tuberculosis Diagnosis and Treatment, Senior Department of Tuberculosis, The 8th Medical Center of PLA General Hospital, Beijing, China
- 2State Key Laboratory of Pathogen and Biosecurity, Beijing Institute of Biotechnology, Beijing, China
- 3 Hebei North University, Zhangjiakou City, China
- 4State Key Laboratory of Pathogen and Biosecurity, Beijing Institute of Microbiology and Epidemiology, Beijing, China
Tuberculosis (TB) is an infectious disease caused by Mycobacterium tuberculosis. As a result of the coronavirus disease 2019 (COVID-19) pandemic, the global TB mortality rate in 2020 is rising, making TB prevention and control more challenging. Vaccination has been considered the best approach to reduce the TB burden. Unfortunately, BCG, the only TB vaccine currently approved for use, offers some protection against childhood TB but is less effective in adults. Therefore, it is urgent to develop new TB vaccines that are more effective than BCG. Accumulating data indicated that peptides or epitopes play essential roles in bridging innate and adaptive immunity and triggering adaptive immunity. Furthermore, innovations in bioinformatics, immunoinformatics, synthetic technologies, new materials, and transgenic animal models have put wings on the research of peptide-based vaccines for TB. Hence, this review seeks to give an overview of current tools that can be used to design a peptide-based vaccine, the research status of peptide-based vaccines for TB, protein-based bacterial vaccine delivery systems, and animal models for the peptide-based vaccines. These explorations will provide approaches and strategies for developing safer and more effective peptide-based vaccines and contribute to achieving the WHO’s End TB Strategy.
1 Introduction
As an ancient infectious disease, tuberculosis (TB) has followed the footsteps of humanity for thousands of years (1, 2). Mycobacterium tuberculosis is the pathogen that causes TB. The war between humans and M. tuberculosis has never stopped from ancient times to modern society. Even today, TB remains a serious health threat. It has been reported that there were almost 1.3 million TB deaths among the human immunodeficiency virus (HIV) negative population in 2020 globally, up from 1.2 million in 2019 (3). These data indicated that the coronavirus disease 2019 (COVID-19) pandemic had disturbed years of global progress in reducing TB deaths, pushing the total number of TB deaths in 2020 back to the 2017 level (3). Furthermore, the emergence of drug-resistant and multidrug-resistant TB (MDR-TB) and the lack of effective methods for differential diagnosis of latent TB infection (LTBI) pose many challenges to TB prevention and treatment (4, 5).
Vaccination is the most effective way to control TB. The only licensed TB vaccine is Bacille Calmette-Guérin (BCG), which has been used for more than 100 years (6, 7). Previous studies have reported that BCG can protect children from severe TB and miliary TB. Therefore, BCG has been recommended by the World Health Organization (WHO) for widespread use in childhood immunization programmes in 154 countries in 2020 (3). However, a growing number of studies have reported that BCG is protective for only 10 - 20 years (8). This may be the reason why the defensive efficiency of BCG in adult pulmonary TB ranges from 0% to 80% (1, 9). According to the report released by WHO, there are 14 TB vaccine candidates in clinical trials, including AEC/BC02, Ad5 Ag85A, and ChAdOx185A-MVA85A vaccines in Phase I, MTBVAC, ID93 + GLA-SE, TB/FLU-04L, and GamTBvac vaccines in Phase IIa, DAR-901 booster, H56: IC31, M72/AS01E, BCG revaccination, and RUTI® vaccines in Phase IIb, VPM1002 and MIP/Immuvac vaccines in Phase III (10). These TB novel vaccines can be divided into four categories: Viral vector vaccines, subunit vaccines, attenuated live mycobacterial vaccines, and inactivated vaccines (10). The most promising of these vaccines is M72/AS01E. It has been reported that the M72/AS01E vaccine had good protection in healthy adults (11, 12), HIV infected adults in Switzerland (13), and healthy infants in Gambia (14). Consequently, M72 has been selected for further vaccine development. In 2018, a Phase IIb controlled trial of the M72/AS01E vaccine showed that the protective efficacy of the vaccine against active pulmonary TB in adults was 54.0%, and there was no obvious safety problem (15). One year later, after three years of follow-up, the New England journal of medicine (NEJM) published the final results of this Phase IIb clinical trial of the M72/AS01E vaccine. It was found that the total effectiveness was 49.7% after 36 months of follow-up, and the evaluation of vaccine efficacy increased throughout the study period, with vaccine effectiveness of 27.4% in the first year, 55.2% in the second year and 60.2% in the third year (16). However, it needs to be recognized that even if M72/AS01E vaccine is proven to be reliable in larger populations, TB control cannot be based on the M72/AS01E vaccine alone. We should develop more effective and safer vaccines to prevent and control TB.
The biggest obstacle to developing a TB vaccine is the lack of understanding of the pathogenesis of M. tuberculosis and host immune protective mechanism. The innate and adaptive immunity of the host plays a vital role in the elimination or killing of M. tuberculosis (Figure 1). Innate immune cells, such as macrophages, dendritic cells (DCs), and natural killer (NK) cells, are the front-line to resist M. tuberculosis invasion. As the most important antigen presenting cells (APCs), macrophages and DCs play an essential role in phagocytosing M. tuberculosis. DCs activated by M. tuberculosis migrate to lymph nodes to display peptides of mycobacteria on their surface, which will be recognized by CD4+ T cells and CD8+ T cells through major histocompatibility complex (MHC) II and MHC I molecules, respectively (5, 17). Interestingly, the recognition between T cells and APCs is based on peptides rather than full-length protein (Figure 1). Therefore, the selection of vaccine candidate antigens and the prediction and screening of these immunodominant peptides are the key to designing a new generation of TB vaccine, known as peptide-based vaccine. The most significant advantage of peptide-based vaccines is the aggregation of immunodominant epitopes, which improves the immunogenicity of the vaccine and reduces its side effects (2).
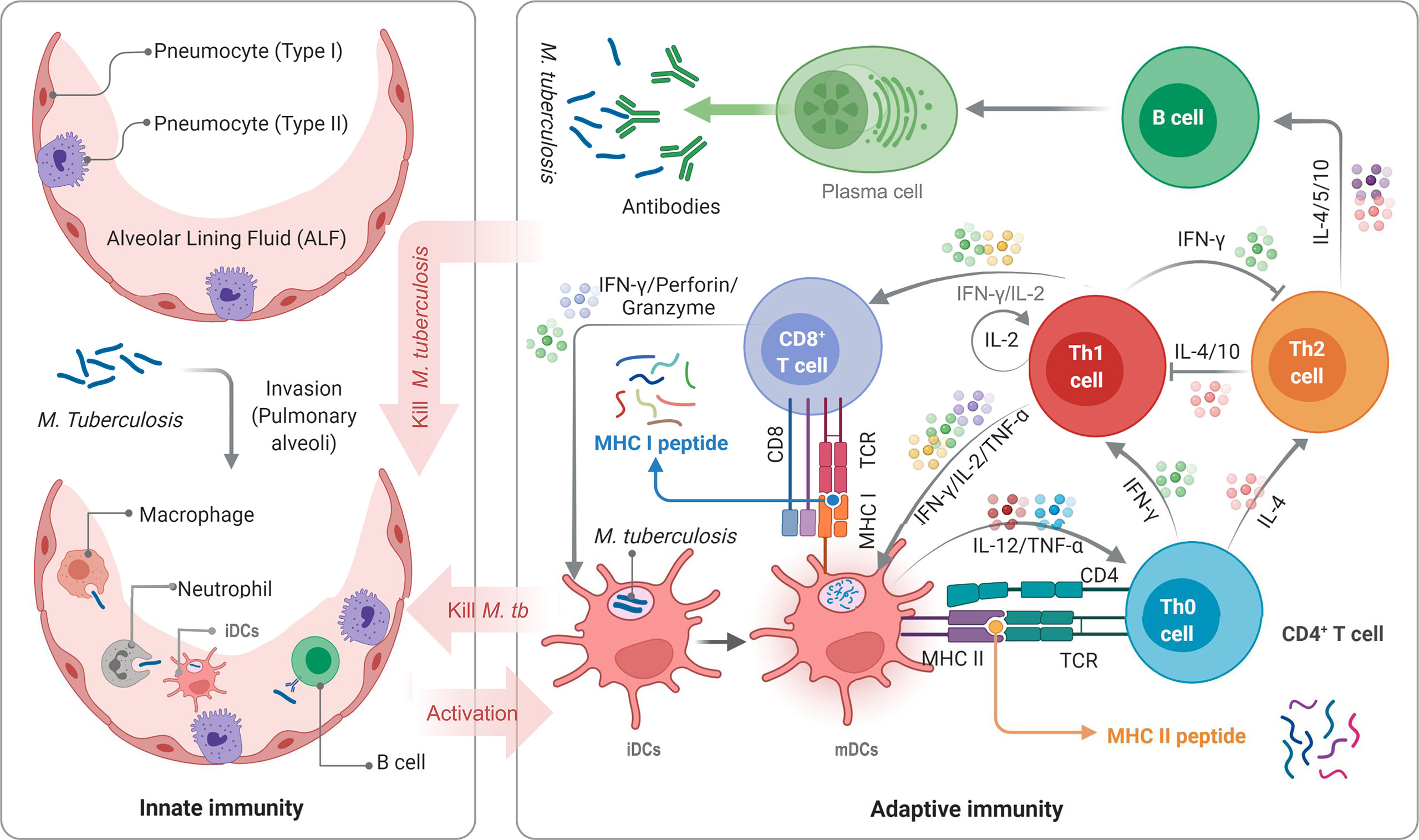
Figure 1 Schematic diagram of TB infection and anti-TB immunity of the host. APCs, such as DCs, macrophages, neutrophils, and even B cells, not only play the role of innate immune cells but also serve as a bridge between innate and adaptive immunity. DCs are the most important APCs, and their antigen presenting ability in vitro is 10-100 times that of other APCs. M. tuberculosis bacilli invading host’s pulmonary alveoli are first recognized and swallowed by APCs. Then, immature dendritic cells (iDCs) take up M. tuberculosis antigens and migrate to lymph nodes. During this process, the expression of MHC molecules on the surface is up-regulated, the antigen presentation function and the ability to activate T cells are also enhanced, and iDCs are transformed into mature dendritic cells (mDCs). mDCs can secrete interleukin-12 (IL-12), tumor necrosis factor-α (TNF-α), and interferon-α (IFN-α) to act on native CD4+ T cells (Th0 cells) to differentiate into Th1 cells. IFN-γ, IL-2, and TNF-α produced by Th1 cells can effectively activate CD8+ T cells and macrophages to eliminate intracellular M. tuberculosis by perforin, granzyme, reactive oxygen, and reactive nitrogen. Furthermore, mDCs produce IL-4, making Th0 cells differentiate into Th2 cells. The function of the Th2 response and lL-4 in the anti-tuberculosis immune response remains unclear. It is generally believed that Th2 cells will affect B lymphocytes by secreting IL-4, IL-5, and IL-10, mediating humoral immune response.
This study reviewed the latest bioinformatics tools, linkers, and adjuvants used in designing peptide-based vaccines, the research status of peptide-based vaccines for TB, the protein-based bacterial vaccine delivery system, and animal models for peptide-based vaccines. To our knowledge, this is the first detailed and comprehensive review to report peptide-based vaccines for TB. We hope that through this review, we can provide practical tools and methods for designing peptide-based vaccines and contribute new ideas to developing novel TB vaccines.
2 The Development of Bioinformatics Technology Has Laid the Foundation for the Rise of Peptide-Based Vaccines for TB
The rapid development of bioinformatics technology makes it possible to predict and design a peptide-based vaccine with computers in advance. Compared with conditional vaccines, peptide-based vaccines have many advantages: more straightforward and faster production, more stable and convenient transportation and storage, lower cost, and decreased side effects (18). Despite these benefits of peptide-based vaccines, the following tasks must be done to design a peptide-based vaccine successfully: identification of potential antigens, prediction of T cell and B cell epitopes, analysis of epitope immunogenicity, antigenicity, allergenicity, and toxicity, selection of linkers and adjuvant peptides, construction and optimization of final vaccine, and analysis of the characteristics of the final vaccines. A list of databases or servers used to construct a peptide-based vaccine has been shown in Table 1.
2.1 Epitope Prediction
2.1.1 Determination of Protective Antigens and the Coverage of MHC Alleles
Before predicting T cell or B cell epitopes, it is necessary to determine the protective antigens for vaccine construction and the coverage of MHC alleles in specific populations. Protective antigens should be selected from previous studies, and these antigens must have been proven to have significantly higher protective efficacy in animal models or clinical trials. Amino acid sequences of protective antigens can be obtained from National Center of Biotechnology Information (NCBI, https://www.ncbi.nlm.nih.gov/protein), Target-Pathogen database (http://target.sbg.qb.fcen.uba.ar/patho), Uniprot protein database (https://beta.uniprot.org/), and GeneDB database (http://www.genedb.org/Homepage) (19, 21–23).
Fortunately, with the rapid development of bioinformatics technology, a growing number of TB specific databases or servers have been developed for TB vaccine construction, such as Mycobrowser (20), MtbVeb (24), MycobacRV (26), and PeMtb (25). These novel tools provide powerful support for designing peptide-based vaccines for TB. Mycobrowser (https://mycobrowser.epfl.ch/) is a comprehensive genomic and proteomic database for ten pathogenic mycobacteria species, including M. tuberculosis H37Rv, M. tuberculosis 18b, M. smegmatis MC2-155, M. orygis 51145, M. marinum M, M. lepromatosis Mx1-22A, M. leprae TN, M. haemophilum DSM_44634/ATCC_29548, M. bovis AF2122/97, and M. abscessus ATCC_19977. The Mycobrowser knowledge base provides general annotation, gene or protein summary information, orthologues, and cross-references to the UniProt, Gene Ontology, SWISS-MODEL, and TB database (20). MtbVeb (http://crdd.osdd.net/raghava/mtbveb/) is a database developed by scientists from Institute of Microbial Technology in India for designing TB vaccines by using three approaches, such as strain, antigen, and epitope based vaccines (24). This database maintains integrated genomic information of 59 mycobacterium strains and provides comprehensive information for the antigenicity of potential vaccine candidates (24). MycobacRV database (http://mycobacteriarv.igib.res.in), developed by scientists from CSIR-Institute of Genomics and Integrative Biology in India, includes whole-genome sequences of 22 pathogenic mycobacterium species and one non-pathogenic mycobacterium H37Ra strain, and a set of 233 most probable vaccine candidates (26). Recently, a database of MHC antigenic peptide of M. tuberculosis named PeMtb has been developed to assist scientists in more efficient selection of epitopes that can be used for peptide-based vaccine construction (25). PeMtb is a free platform for predicting potential antigenic peptides of M. tuberculosis, which has unique advantages in epitopes prediction for TB vaccines development.
Furthermore, the most significant difference between peptide-based vaccines and traditional vaccines is that the former has MHC restriction. Human MHC molecules are also known as human leukocyte antigen (HLA). The HLA gene is located at the end of the short arm of human chromosome 6 and is divided into three regions: HLA Class I, HLA Class II, and HLA Class III (101). HLA genes with antigen presentation function are classic HLA genes located in HLA class I and HLA class II regions. The classic HLA I genes are divided into three categories: HLA-A, HLA-B, and HLA-C, and the classic HLA II genes are divided into three categories: HLA-DP, HLA-DQ, and HLA-DR (102). Therefore, selecting peptides with different MHC binding specificities will increase the coverage of the target population of peptide-based vaccines. However, due to the polymorphism of MHC molecules in other races, the design of peptide-based vaccines has become more complicated. Therefore, the coverage of MHC alleles in the vaccinated population must be considered when constructing a peptide-based vaccine. To overcome this issue, some databases and resources have been developed, including the Allele Frequency Net Database (http://www.allelefrequencies.net/pops.asp) and population coverage submodule of Immune Epitope Database and Analysis Resource (IEDB, www.iedb.org) (103). The Allele Frequency database provides allele frequencies for 115 countries and 21 different ethnicities grouped into 16 other geographical areas (28). IEDB is a popular database for providing information on immune epitopes. There are two components in the IEDB database, including the home page search and Analysis Resource. The home page search is designed to simplify the search process for many commonly queries such as Epitope (Linear peptide, discontinuous peptide, non-peptidic, and Any), Assay (T cell, B cell, and MHC ligand), Epitope Source (Organism and Antigen), MHC Restriction (Class I, Class II, Non-classical, and Any), hosts (humans, non-human primates, and other animal species), and Disease. The Analysis Resource component provides a set of tools for predicting and analyzing immune epitopes, which can be divided into three categories: (1) T Cell Epitope Prediction Tools: Peptide binding to MHC class I or II molecules (29, 37), peptide processing predictions and immunogenicity predictions (67, 104–106), TCRmatch (107), and structure tools such as LYRA (Lymphocyte Receptor Automated Modelling) (108), SCEptRe (Structural Complexes of Epitope Receptor) (109), and Docktope (109); (2) B Cell Epitope Prediction Tools: Prediction of linear epitopes from protein sequence including Chou & Fasman Beta-Turn Prediction, Emini Surface Accessibility Prediction, Karplus & Schulz Flexibility Prediction, Kolaskar & Tongaonkar Antigenicity, Parker Hydrophilicity Prediction, Bepipred Linear Epitope Prediction, and Bepipred Linear Epitope Prediction 2.0 (46–52); Discotope (110), ElliPro (59), methods for modeling and docking of antibody and protein 3D structures (111), LYRA server (108), and SCEptRe (109); (3) Analysis tools: Population Coverage (28), Epitope Conservancy Analysis (112), Epitope Cluster Analysis (80), Computational Methods for Mapping Mimotopes to Protein Antigens (http://tools.iedb.org/main/analysis-tools/mapping-mimotopes/), RATE (Restrictor Analysis Tool for Epitopes) (113), and ImmunomeBrowser (114). The components of the IEDB database related to peptide-based vaccine development are described in detail below.
2.1.2 T Cell Epitope Prediction Tools
MHC molecules, expressed on the surface of APCs, are responsible for presenting antigenic peptides to T cells, making them irreplaceable in activating adaptive immunity (34). MHC molecules can be divided into two sets, MHC Class I (MHC I) and MHC Class II (MHC II), which primarily presents intracellular and extracellular peptides, respectively. Hence, identifying peptides binding to MHC I and II molecules is crucial for activating CD8+ and CD4+ T lymphocytes. Furthermore, recent studies have reported that engaging both helper T lymphocytes (HTL) epitopes binding to MHC II molecule and cytotoxic T lymphocytes (CTL) epitopes binding to MHC I molecule is desirable for inducing a robust immune response against M. tuberculosis (115, 116). Therefore, accurate computational prediction of HTL and CTL epitopes is the cornerstone for successfully constructing a peptide-based vaccine.
Currently, a growing number of bioinformatics technologies are available for HTL and CTL epitopes prediction, such as the IEDB database (29), RANKPEP server (30), MetaMHCIIpan (31), ProPred (32, 33), NetMHCIIpan-4.0 (34, 35), NetMHCIIpan 3.2 (36), NetCTL-1.2 (38), RANKPEP server (30), ProPred1 (39), NetMHCpan-4.1 (34), MetaMHCIpan (31), NetMHC4.0 (40), MHCpred 2.0 (41), EpiJen (42), and CTLPred (43) (Table 1). Three of these servers or databases can predict both HTL and CTL epitopes, including RANKPEP server, MetaMHCpan, and MHCPred. RANKPEP server predicts HTL and CTL epitopes from protein sequence using Position Specific Scoring Matrices (PSSMs) (30). MetaMHCpan has two parts: MetaMHCIpan and MetaMHCIIpan, for predicting CTL and HTL epitopes, respectively. MetaMHCIpan is based on two existing predictors (MHC2SKpan and LApan), while MetaMHCIIpan is based on four existing predictors (TEPITOPEpan, MHC2SKpan, LApan, and MHC2MIL) (31). MHCPred was developed to predict both HTL and CTL epitopes based on an Allele specific Quantitative Structure Activity Relationship (QSAR) model generated using partial least squares (PLS). MHCPred 2.0 covers 11 HLA class I, three human HLA class II, and three mouse MHC class I models (41). Furthermore, MHCPred 2.0 has multiple significant merits, such as incorporating a binding model for human transporter associated with antigen processing (TAP) that offers additional evidence, comprising a tool for designing heteroclitic peptides, and providing a confidence percentage for every peptide prediction (41).
As the most popular epitope prediction database, IEDB has unique advantages in HTL and CTL epitopes prediction. For MHC II epitopes prediction, nine methods are implemented, including IEDB recommended, Consensus method (117), Combinatorial library, NN-align-2.3 (netMHCII-2.3) (36), NN-align-2.2 (netMHCII-2.2) (118), SMM-align (netMHCII-1.1) (119), Sturniolo (120), NetMHCIIpan-3.1 (121), and NetMHCIIpan-3.2 (36). IEDB Recommended, selected as the default method, uses the best possible scenario for a given MHC molecule based on the following rules: the Consensus approach (a combination of any three of NN-align, SMM-align, CombLib, and Sturniolo) will be used if any corresponding predictor is available for the antigen. Otherwise, NetMHCIIpan is used. The performance of the MHC class II binding predictions has been evaluated in two studies based on over 10,000 binding affinities (117) and 40,000 binding affinities (29), and one study comparing pan-specific methods (122). For MHC I epitopes prediction, ten methods are implemented, including IEDB recommended 2020.09 (NetMHCpan EL4.1), Artificial neural network (ANN) (40), Stabilized matrix method (SMM) (123), SMM with a Peptide: MHC Binding Energy Covariance matrix (SMMPMBEC), Scoring Matrices derived from Combinatorial Peptide Libraries (Comblib_Sidney2008) (124), Consensus (125), NetMHCpan (126), NetMHCcons (127), PickPocket (128) and NetMHCstabpan (129). Similarly, IEDB recommended NetMHCpan EL 4.1 is selected as the default method and used across all alleles.
2.1.3 B Cell Epitope Prediction Tools
More and more attention has been paid to the role of B cells in M. tuberculosis infection. B cells secrete antigen-specific antibodies to fight against M. tuberculosis invasion. Antigenic peptides are critical triggers for B cell antibody recognition. Therefore, the prediction of B cell epitopes is helpful to study the mechanism of the host’s self-protection system and design a peptide-based vaccine. Unlike T cell epitopes, B cell epitopes have both continuous (also known as linear epitopes) and discontinuous (also known as conceptual epitopes). A linear epitope is a continuous fragment from an antigen sequence. In contrast, a conformational epitope comprises several fragments distributed in an antigen sequence that form a structural domain-like interface in three dimensions.
At present, most of the available methods for predicting B cell epitopes are focused on continuous epitopes, such as ABCpred (44, 45), IEDB B-cell epitope tools (46–52), BCPred (53, 54; a), BepiPred 2.0 (52), APCpred (55), and SVMTriP (56). These methods are based on antigen amino acid sequence, and the operation method is simple and easy to study. Overall, the sensitivity and specificity of these methods for predicting linear B cell epitopes ranged from 60% to 70%, and the area under the curve (AUC) ranged from 0.6 to 0.8 (Table 1). Compared with other methods, IEDB Antibody Epitope Prediction is a collection of seven methods to predict continuous B cell epitopes based on antigen sequence using amino acid scales and machine learning algorithm Hidden Markov Model (HMM), including Bepipred Linear Epitope Prediction 2.0, Bepipred Linear Epitope Prediction, Chou & Fasman Beta-Turn Prediction, Emini Surface Accessibility Prediction, Karplus & Schulz Flexibility Prediction, Kolaskar & Tongaonkar Antigenicity, and Parker Hydrophilicity Prediction (46–52). These seven methods of IEDB predict linear B cell epitopes based on specific characteristics of an antigen sequence, such as hydrophilicity, accessibility, flexibility, turns, polarity, exposed surface, and antigenic propensity.
Previous studies have shown that up to 90% of B cell epitopes are discontinuous in nature, but most predictions have focused on linear epitopes, which may be related to the tertiary structure of proteins required for B cell conformational epitopes prediction. Despite the rapid development of single-crystal X-ray diffraction (SCXRD), nuclear magnetic resonance (NMR) spectroscopy, and X-ray crystallography, many tertiary structures of biological macromolecules have been elucidated, but accurate prediction of B cell epitopes remains challenging (58). Currently, several methods have been used for predicting conformational B cell epitopes, including DiscoTope-2.0 (57), BEpro (formerly known as PEPITO) (58), ElliPro (59), SEPPA (60), Epitopia (61), EPCES (62), EPSVR (63), EPMeta (63). A previous study compared the performance of DiscoTope-2.0 to the PEPITO, ElliPro, SEPPA, Epitopia, EPCES and EPSVR methods. The results indicated that the AUC value of DiscoTope-2.0 was observably higher than that of ElliPro but comparable to PEPITO. Furthermore, DiscoTope-2.0 revealed an enhanced AUC value compared to that of SEPPA (0.720 vs 0.711), EPCES (0.733 vs 0.695), Epitopia (0.727 vs 0.652) and EPSVR (0.746 vs 0.588) based on benchmark dataset (57).
2.1.4 Peptide Analysis and Screening Tools
As shown in Figure 1, the IFN-γ and IL-4 cytokines secreted by APCs play an essential role in promoting the differentiation of native CD4+ T cells into Th1 and Th2 cells, which is the principal arm for controlling and killing intracellular M. tuberculosis (130). Three methods have been developed to predict the IFN-γ, IL-4, and IL-10 inducers by bioinformatics technologies, including IFNepitope (64), IL4pred (65), and IL-10pred (66). IFNepitope is an online prediction server that can predict the epitopes that can induce CD4+ T cells to secrete IFN-γ based on the protein sequence. It can help immunologists select and design IFN-γ-induced MHC Class II binding epitopes from proteins of interest, which is essential for designing better and more effective peptide-based vaccines (64). IL4pred and IL-10pred were developed to predict IL-4 and IL-10 inducing MHC II binding epitopes, respectively. The algorithm of the three servers relies on the following three models: Motif based model, Support Vector Machine (SVM) based model, and/or Hybrid approach (a combination of Motif and SVM). The maximum prediction accuracy of the three servers is 82.10%, 75.76%, and 81.24%, and the Matthew’s correlation coefficient (MCC) is 0.62, 0.51, and 0.59, respectively (64–66).
In the design of peptide-based vaccines, in addition to considering those mentioned cytokine-induced epitopes, it is also necessary to assess the immunogenicity, antigenicity, allergenicity, and toxicity of the epitopes. Previous studies have shown that these characteristics vary significantly among epitopes (67, 72, 74, 78). Therefore, how to choose epitopes with strong immunogenicity and antigenicity but low toxicity and allergenicity is a challenge for peptide-based vaccine design. To overcome these obstacles, some new algorithms, models or servers have been developed, including IEDB MHC I immunogenicity, IEDB CD4 T cell immunogenicity prediction, MARIA, BciPep, and PopCover-2.0 for immunogenicity (67–71), VaxiJen 2.0 and ANTIGENpro for antigenicity (72, 73), AllerTOP v 2.0, AllergenFP v.1.0, AlgPred 2.0, and Allermatch™ for allergenicity (74–77), ToxinPred and T3DB for toxicity (78, 79). In addition, other useful tools have been developed to help scientists design more effective peptide-based vaccines, such as IEDB Clusters with Similar Sequences for epitope cluster analysis (80), PIP-EL for proinflammatory peptides prediction (81), and PreAIP for anti-inflammatory peptides prediction (82).
2.2 Construction of Peptide-Based Vaccines
The most potential immunodominant epitopes are short peptides composed of dozens of amino acid residues and quickly degrade at the inoculation site. In order to overcome this shortcoming, it is necessary to use appropriate linkers and/or additional helper peptides (adjuvant peptides and agonists used in constructing a peptide-base vaccine) to fuse these dispersed epitopes to improve the efficiency of the vaccine.
2.2.1 Linkers
Linkers are short amino acid sequences of natural origin that separate multiple domains in proteins (131). The selection of suitable linkers to link protein domains together is always complicated, but it is often overlooked in the design of peptide-based vaccines. If there are no linkers, a direct fusion of immunodominant epitopes may lead to many undesirable results, including misfolding of peptide-based vaccine (132), low vaccine yields (133), and impaired biological activity (134). Therefore, the selection and rational design of linkers connecting dominant epitopes is a crucial but undeveloped field in developing peptide-based vaccines.
According to the characteristics of linkers, they can be divided into three categories: flexible linkers, rigid linkers, and cleavable linkers. (1) Flexible linkers are usually used to connect protein domains that need mobility and interaction. They contain some polar or nonpolar amino acids with small molecular weight, which provides flexibility for the movement and interaction between protein domains (135). The commonly used flexible linkers include (GGGGS)n (135), (Gly)8 (136), (Gly)6 (137), GSAGSAAGSGEF (138), KESGSVSSEQLAQFRSLD and EGKSSGSGSESKST (139). The GSAGSAAGSGEF linker is better than the (GGGGS)4 linker due to its better hydrophilicity and no-repeats (140). Providing flexibility for the movement and interaction between protein domains is the advantage of flexible linkers, but the lack of rigidity may lead to inefficient expression of recombinant proteins or loss of biological activity (133, 141). (2) Rigid linkers are usually used to maintain the distance between protein domains, effectively separating different protein domains and reducing the interaction and influence between the domains. Common rigid linkers are (EAAAK)n, A(EAAAK)nA (n = 2-5), PAPAP, (Ala-Pro)n (132, 140, 142, 143). The rigid linker exhibits a relatively rigid structure by adopting an α-helical structure, and they separate protein domains more effectively than flexible linkers. Furthermore, the length of the rigid linker can be easily adjusted by changing the number of copies to achieve the best distance between domains. Therefore, rigid linkers are chosen when the spatial separation of domains is essential to maintain the stability or biological activity of the fusion protein. (3) Cleavage linkers are usually used to separate domains or peptides from protein or vaccine to achieve the biological functions of a single domain or peptide. These linkers can reduce steric hindrance, improve biological activity, and realize the independent function/metabolism of a single domain of the recombinant fusion protein after the linker is cut. However, the design of cleavable linkers for recombinant fusion proteins in vivo is a very challenging subject. Hence, cleavage linkers are rarely used in the design of peptide-based vaccines. Linkers used in peptide-based vaccine construction can be found in Table 2.
2.2.2 TLR Agonists and Helper Peptides
Like other subunit vaccines, weak immunogenicity is one of the disadvantages of peptide-based vaccines. Covalent conjugation of helper peptides to peptide-based vaccines appears to be a powerful strategy for improving the immunogenicity and protective efficiency of peptide-based vaccines. Currently, two kinds of adjuvants have been used in peptide-based vaccine construction to enhance its protective efficacy: Toll-like receptor (TLR) agonists and helper peptides. TLRs are important protein molecules involved in innate immunity and serve as a bridge between innate and adaptive immunity (168). There are ten common TLRs, of which, TLR1, TLR2 (a heterodimer of TLR1 and TLR6), TLR4, TLR5, TLR6 and TLR10 are located on the cell membrane, while TLR3, TLR7, TLR8, and TLR9 are located on the membrane of the endosome (Figure 2). Among these TLRs, TLR10 is an orphan receptor whose ligand, signaling pathway and biological function are still unknown (Figure 2).
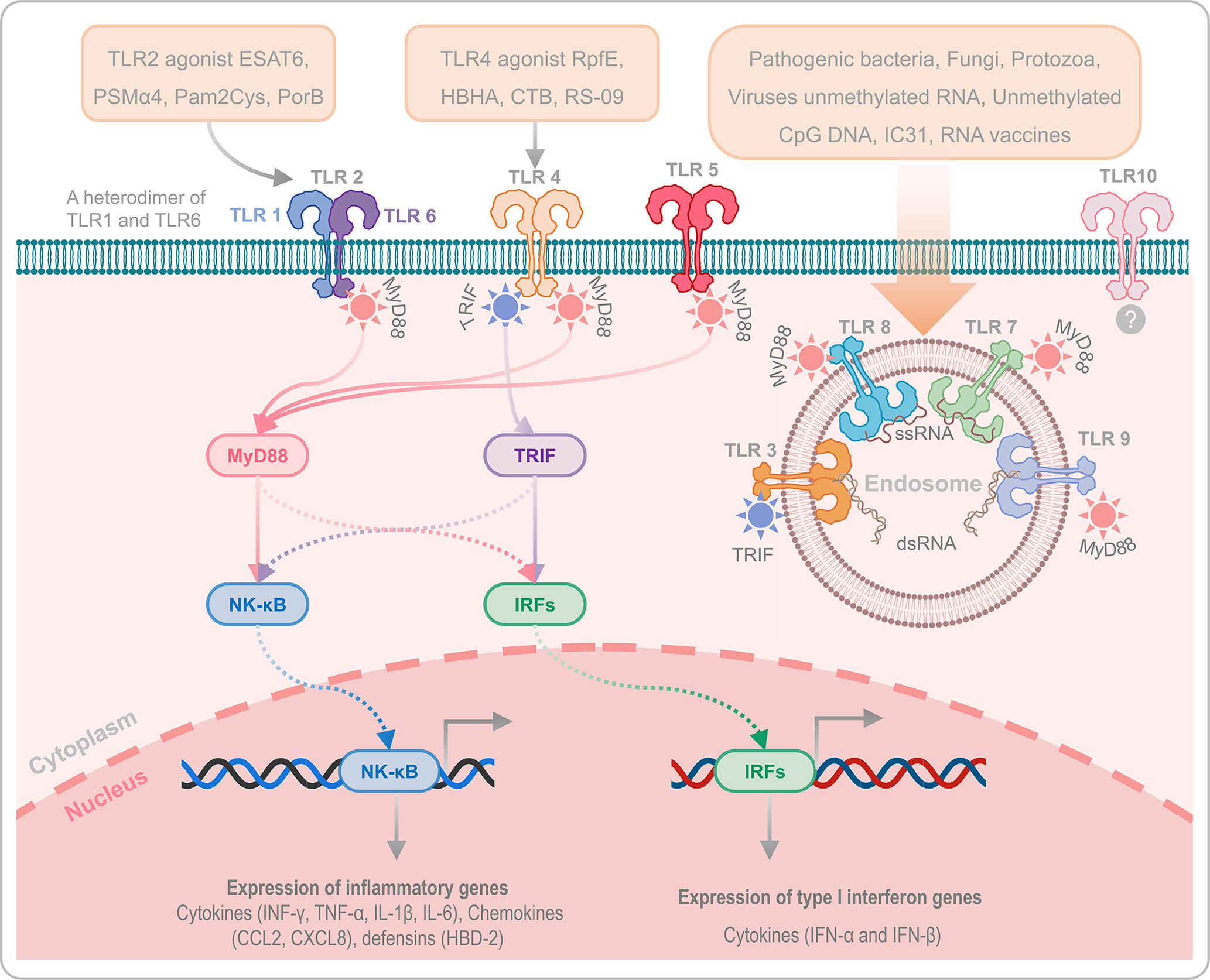
Figure 2 Toll-like receptors and their agonists. TLRs play an essential role in innate immunity and serve as a bridge between innate and adaptive immunity. TLR1, TLR2 (a heterodimer of TLR1 and TLR6), TLR4, TLR5, TLR6, and TLR10 are located on the cell membrane, while TLR3, TLR7, TLR8, and TLR9 are located on the membrane of the endosome. It has been reported that TLR2, TLR4, and TLR9 are critical for host defense against M. tuberculosis infection. In addition, the agonists of TLR2, TLR4, and TLR9 can enhance the immunogenicity and protective efficacy of peptide-based vaccines via TLR2- MyD88-NK-κB/IRFs-IFN-I/γ signaling pathway and TLR4-MyD88/TRIF-NK-κB/IRFs-IFN-I/γ signaling pathway. TLR10 is an orphan receptor without confirmed ligands, signalling pathway and biological function.
Previous studies have reported that TLR2-, TLR4-, and TLR9-mediated immune responses are critical for host defense against M. tuberculosis infection (169, 170). TLR9, expressed by human B cells and DCs, play an essential role in recognizing the CpG DNA in bacterial rather than in not mammalian, which induces the differentiation, maturation, and proliferation of macrophages, NK cells, monocytes, T cells, and B cells, and enhances the production of Th1 type cytokines, such as IFN-γ, TNF-α, and IL-12 (171). More currently, there are three kinds of CpG oligonucleotides (ODN) that have been used as TLR-9 agonists, 1) Type A CpG ODN is consist of a phosphodiester/phosphothioate backbone, a palindrome sequence containing CpG dinucleotide, and poly G tail at the 3’ and 5’ terminals, which can activate the plasmacytoid dendritic cells (pDCs) to produce IFN-α (172); Type B CpG ODN is composed of multiple CpG motifs, which has solid immunostimulatory activity against B cells, but cannot activate pDCs (173); Type C CpG ODN is composed of whole phosphorothioate and palindromic CpG motifs, has the activity of both type A and type B CpG-ODNs, and can activate B cells and pDCs (174). TLR2 and TLR4 activate DCs by recognizing with the pathogen associated molecular patterns (PAMPs), and the activated DCs will produce kinds of cytokines to kill M. tuberculosis via TLR2- MyD88-NK-κB/IRFs-IFN-I/γ signaling pathway and TLR4-MyD88/TRIF-NK-κB/IRFs-IFN-I/γ signaling pathway (Figure 2) (175). Therefore, enhancing host immune responses with TLR2 and TLR4 agonists may be the option for constructing an ideal peptide-based vaccine in future. At present, some TLR2 and TLR4 agonists have been used in peptide-based vaccines against infectious diseases, including TB, such as TLR2 agonists ESAT6 (144), phenol-soluble modulin α4 (PSMα4) (145), dipalmitoyl-S-glyceryl cysteine (Pam2Cys) (115, 146), and PorB (147, 148), TLR4 agonists RpfE (Rv2450c) (149), 50S ribosomal protein L7/L12 (RplL) (22, 150–155), heparin binding hemagglutinin (HBHA) (156), cholera toxin subunit B (CTB) (157–159), and RS-09 (160, 161). In addition, helper peptides and antimicrobial peptides are also used to construct peptide-based vaccines to enhance their immune effects, such as PADRE (148) (151) (156), Hsp70 (161), TR-433 (161), human β-defensin 1 (HBD-1) (162), HBD-2 (163), HBD-3 (19, 164–167), and Griselimycin (84). The amino acid sequences of the mentioned helper peptides can be found in Table 2.
2.2.3 Codon Optimization and Prediction of Structure and Function of Peptide-Based Vaccines
After the prediction and screening of dominant epitopes and the use of linkers and helper peptides (or adjuvants), a preliminary peptide-based vaccine was constructed. However, this native vaccine needs further optimization to become a mature peptide vaccine, including codon optimization, cloning and expression evaluation, and solubility prediction. Codon optimization is essential because the degeneracy of the genetic code allows one amino acid to be encoded by multiple codons (84). Java Codon Adaptation Tool (JCat) is the most popular tool for codon adaptation (Table 1). Compared with previous tools, JCat has superiorities in avoiding unnecessary cleavage sites for restriction endonuclease and Rho-independent transcription terminators and defining highly expressed genes as more intelligent, faster, and more accessible (83). Codon Adaptation Index (CAI) values are used to evaluate codon optimization. The best CAI value is 1.0, while CAI > 0.8 is regarded as a good score (83). Then, the sequence of the final vaccine optimized with JCat should be inserted into an appropriate plasmid vector using SnapGene software (84). Finally, the solubility of the final vaccine should be predicted by bioinformatics methods such as Protein-Sol server (85).
TCR and MHC are the bridges connecting APCs, T lymphocytes and peptide-based vaccines. Accurate recognition of TCR and major histocompatibility complex presented antigenic peptides (pMHC) triggers adoptive immune responses to kill M. tuberculosis. In the past, the crystallization and structural resolution of TCR-pMHC complexes were expensive and took a lot of time. However, with the development of computational technology, some valuable models or algorithms have been developed to study the TCR-pMHC interaction at the molecular level, such as PAComplex (86), ZDOCK (90), LightDock (89), ClusPro (88), HADDOCK (87) and iMOD (91). Furthermore, a recent study compared the ability of four standard tools (including ZDOCK, LightDock, ClusPro, and HADDOCK) to perform accurate molecular docking of the TCR-pMHC based on an expanded benchmark set of 44 TCR-pMHC docking cases (176). It was suggested that achieved success rates of HADDOCK, ClusPro, ZDOCK, and LightDock are 34.1%, 27.3%, 15.9% and 6.8%, respectively, indicating that HADDOCK is the best performer. At present, HADDOCK has been updated to version 2.2, which provides some new characteristics such as additional experimental restraints, support for mixed molecule types, improved protocols, and a more friendly interface (87).
The epitope prediction is based on the amino acid sequence of the protein. However, the immunological function of the peptide-based vaccine depends not only on the amino acid sequence but also on the secondary structure and tertiary structure of the vaccine candidate (177). Recently, some bioinformatics approaches and immunoinformatics technologies have been widely used in predicting the secondary structure and tertiary structure of peptide-based vaccines, including PDBsum (92), SSpro8 (93), GOR V server (94), and SOPMA (95) for secondary structure prediction, GalaxyWEB (96), CABS-Flex 2.0 (97), 3Dpro (98), Phyre2 (99), and SWISS-MODEL (100) for tertiary structure prediction.
3 Research Status of TB Peptide-Based Vaccines
Peptide-based vaccines are subunit vaccines and are new vaccines with unique advantages. Compared with traditional subunit vaccines, peptide-based vaccines are more precise and accurate in design (178). As mentioned above, the construction of peptide-based vaccines involves the identification of potential antigens, prediction and screening of dominant epitopes, comparison of MHC affinity, the addition of adjuvants or helper peptides, codon optimization, and prediction of structure and function. These tedious but indispensable processes enable peptide-based vaccines to efficiently cluster dominant epitopes together to induce a more robust immune response in the recipient, improving the efficiency and reducing side effects by excluding unwanted material from a full-length protein or whole pathogen (179).
The first peptide-based vaccine was reported and developed to fight against Plasmodium falciparum by Etlinger HM et al. in 1988 (180). This peptide-based vaccine consisted of a synthetic peptide [Ac-Cys-(NANP)3] and a tetanus toxoid protein. The immunological parameters of this vaccine were evaluated in a mouse model. To determine the research process of peptide-based vaccine for TB, we searched the PubMed database with terms of “peptide, epitope, and tuberculosis” (Figure 3A). Analyzing these results showed that the research of peptide-based vaccines for TB can be traced back to around 1990, but due to the lack of bioinformatics technology, the research progress is slow. Around 2010, with the rapid development of bioinformatics technology, the research of peptide-based vaccines for TB began to enter the fast lane. Especially in 2020, with the rise of COVID-19 peptide-based vaccines, the investigation of peptide-based vaccines for TB has also been extensively developed (red bubbles in Figure 3A).
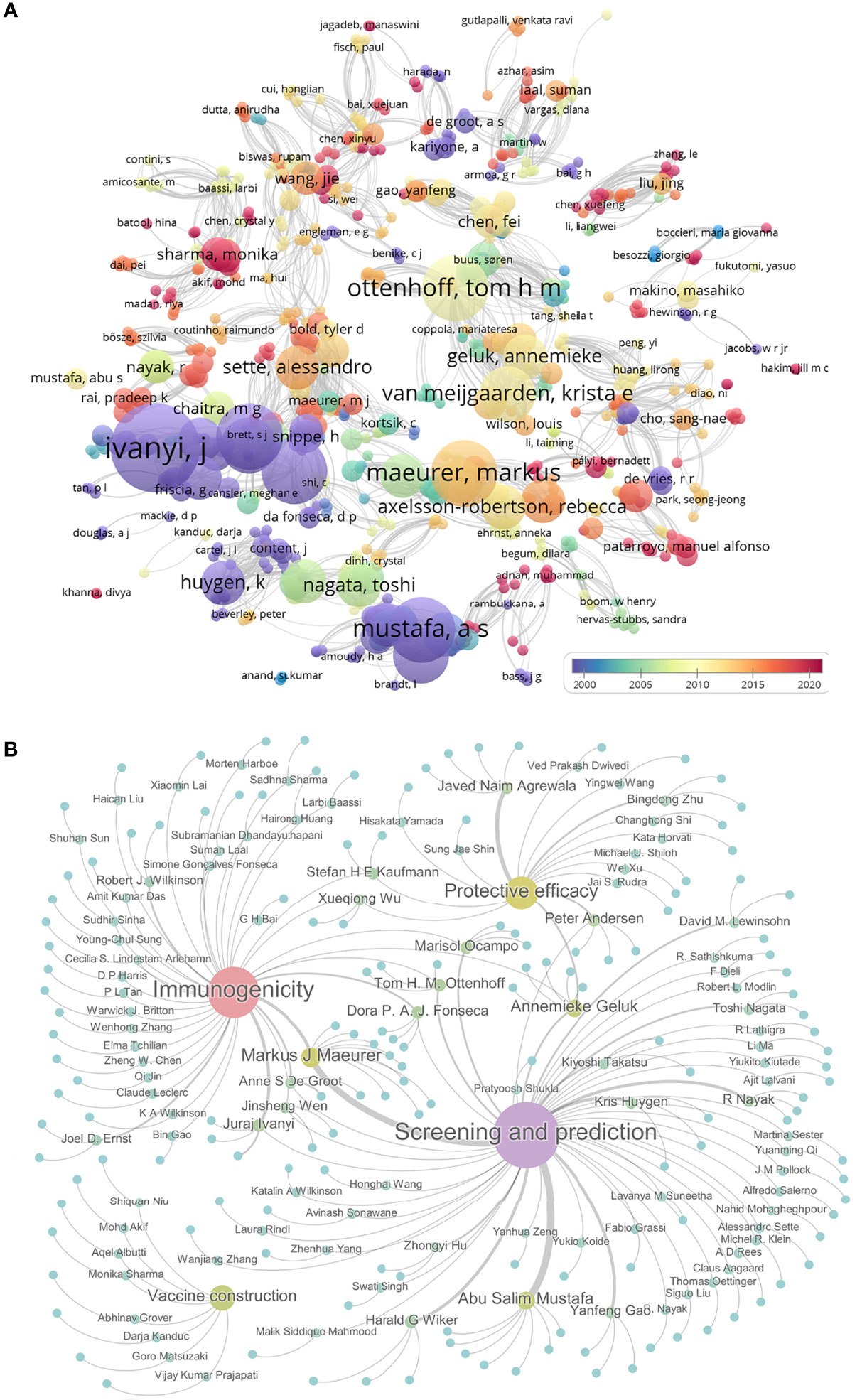
Figure 3 The progress in peptide-based vaccines for TB. The PubMed database was used to search the literature related to the peptide-based vaccine for TB using “peptide, epitope, and tuberculosis”. Their relationships were shown as bubble charts based on publication year and association strength by using VOSviewer software (A). Furthermore, the relationships among 150 pieces of literature related to peptides or epitopes for TB were shown by using Gephi software. These literatures were divided into four categories: epitope screening and prediction, vaccine construction, immunogenicity, and protective efficacy. Gephi software was used to show 150 articles under corresponding authors. Each bubble represents a study, the size of which is proportional to the number of papers the author has published, and the color from blue to purple indicates the level of activity (B).
Further analysis indicated that 150 studies involved in the peptide or epitope for TB, including 76 studies in epitope screening and prediction, 45 studies in evaluating immunogenicity, 8 studies in peptide-based vaccine construction, and 21 studies in assessing vaccine efficacy in animal models (Figure 3B and Table S1). Among these 150 articles, we found that 14 teams had published at least 3 articles in these four areas of TB epitopes or peptides, including six studies on epitope screening and prediction (181–186) and three studies on immunogenicity (187–189) from Pro. Markus J Maeurer’s team; seven studies on epitope screening and prediction from Pro. Abu Salim Mustafa’s team (190–196); one study on epitope screening and prediction (197) and two studies on immunogenicity (197, 198) from Pro. Anne S De Groot’s team; two studies on epitope screening and prediction (199, 200), one study on immunogenicity (201), and two studies on protective efficacy (202, 203) from Pro. Annemieke Geluk’s team; one study on epitope screening and prediction (204) and two studies on immunogenicity (205, 206) from Pro. Dora P. A. J. Fonseca’s team; three studies on epitope screening and prediction from Pro. Harald G Wiker’s team (207–209); four studies on protective efficacy from Pro. Javed Naim Agrewala’s team (115, 146, 210, 211); one study on epitope screening and prediction (212) and three studies on immunogenicity (212–214) from Pro. Juraj Ivanyi’s team; three studies on epitope screening and prediction from Pro. Kris Huygen’s team (215–217); two studies on epitope screening and prediction (218, 219) and one study on immunogenicity (220) from Pro. Marisol Ocampo’s team; one study on epitope screening and prediction (221) and two studies on protective efficacy (222, 223) from Pro. Peter Andersen’s team; four studies on epitope screening and prediction from Pro. R Nayak’s team (224–227); two studies on epitope screening and prediction (228, 229) and one study on protective efficacy (230) from Pro. Tom H. M. Ottenhoff’s team; three studies on epitope screening and prediction from Pro. Yanfeng Gao’s team (231–233). The works from these teams and the efforts of other scientists have laid the foundation for the development of peptide-based TB vaccines.
The detailed information of TB peptide-based vaccines in the stage of prediction, construction, and immunogenicity can be found in Tables 2, S1. Therefore, the following sections will focus on TB peptide-based vaccines in the stage of efficacy evaluation (Table 3).

Table 3 A list of TB peptide-based vaccines evaluated for immunogenicity and protection in animal models.
3.1 Peptide-Based TB Vaccines Evaluated for Immunogenicity
As early as 2004, Mollenkopf HJ et al. identified 36 DNA vaccine candidates preselected by comparative proteomic and found that BCG prime-Rv3407 encoding DNA vaccine boost vaccination induced significantly higher protection compared to BCG alone (234). Then, the putative MHC I epitopes of Rv3407 were predicted by computational method and determined by enzyme-linked immunospot assay (ELISPOT). It was found that Rv3407 64-72 (IPARRPQNL) and Rv3407 68-76 (RPQNLLDVT) peptides stimulated splenocytes collected from BALB/c mice immunized with Rv3407 DNA vaccine secreting significantly higher IFN-γ (234). Similarly, a year later, McMurry J et al. also identified 15 MHC II binding epitopes by bioinformatics tools and ELISPOT, these peptides from 15 antigens of M. tuberculosis could stimulate the PBMCs obtained from healthy or asymptomatic tuberculin skin test-positive donors producing high levels of IFN-γ (197). Although both studies simply validated potential peptides in vitro, they provide new insights into the development of peptide-based vaccines.
With the deepening of the understanding of epitopes, studies on evaluating the immunogenicity of peptides began to shift from in vitro experiments to in vivo experiments. Wang QM et al. constructed a pcDNA3-M-38 vaccine consisting of a pcDNA3 vector and two MHC II binding peptides MPT64 190-198 (FAVTNDGVI) and 38 kDa proteins 166-175 (IAALNPGVNL). The results showed that immunization with the p3-M-38 vaccine could induce epitope-specific CD8+ CTL response in C57BL/6 (H-2b) mice (235). Recently, a study constructed a new peptide-based vaccine, PstS1p, which consists of PstS1 169-405 and PstS1 802-1119 peptides (237). The immunity and immunogenicity of the PstS1p vaccine and PstS1 (Rv0934) protein were evaluated in BALB/c mice. The results showed that both vaccines elicited remarkably higher levels of IgG antibodies and IFN-γ as well as IL-2 Th1-type cytokines (237). Interestingly, the PstS1p peptide-based vaccine showed more potent immunogenicity than the PstS1 vaccine, indicating that the peptide-based vaccine has better prospects than the traditional subunit vaccine.
These studies indicate an excellent method to construct peptide-based vaccines using bioinformatics technology to predict the dominant epitopes and ELSPOT technology for in vitro validation and screening. However, the immunogenicity of peptide-based vaccines containing only dominant epitopes is not ideal, and the addition of adjuvants or helper peptides can significantly improve the immunogenicity of peptide-based vaccines. Garnica O et al. used RVG peptide (YTIWMPENPRPGTPCDIFTNSR) to enhance the immunogenicity of Ag85B 96–111 (QDAYNAAGGHNAVFN) and Ag85B 241–256 (PAFEWYYQSGLSIVMP) peptides (236). They observed that stimulation with RVG peptide fused Ag85B 96–111 or Ag85B 241–256 peptide can improve the antigen presentation ability of mouse bone marrow derived DCs (BMDCs) and human THP-1 macrophages. Furthermore, the number of IFN-γ, IL-2, and TNF-α producing cells were significantly higher in mice immunized with RVG peptide fused Ag85B 241–256 peptide than in mice immunized with Ag85B 241–256 peptide only (236). These results reveal that helper peptide RVG may be a promising adjuvant to developing effective peptide-based TB vaccines. The limitation of these studies is that the animal models used were wild-type mice. Still, the MHC molecules of mice and humans are significantly different, which may result in the failure of a peptide-based vaccine in clinical trials, which has been proved to have an excellent protective effect in the mouse model (2). To overcome this disadvantage, De Groot AS et al. developed HLA-DR B*0101 transgenic mice to evaluate the immunogenicity of a DNA multi-epitope vaccine that contains 24 epitopes derived from Antigen 85 complex, MPT 64, MPB/MPT 70, MPT 63, the 38 kDa, 14-kDa, 16-kDa, 19-kDa, and 32-kDa Mtb proteins (198). The results found that 8 of the 24 epitopes induced immune responses in these HLA-DR B*0101 transgenic mice.
3.2 Peptide-Based TB Vaccines Evaluated for Protective Efficacy in Animal Models
Previous studies on TB subunit vaccines have provided a large number of vaccine candidate antigens for peptide-based vaccines development, such as Ag85A (Rv3804c) (241), Ag85B (Rv1886c) (116, 202, 239, 240), 6-kDa early secretory antigenic target (ESAT-6, Rv3875) (222, 223, 238, 239), heat shock protein HspX (also known as Hsp16.3, Acr, and 14 kDa antigen, Rv2031c) (115, 146, 202, 210, 211, 242), TB10.4 (Rv0288) (2, 115, 211, 240), Rv0476 (211), Hsp65 (202), 19-kDa lipoprotein (Rv3763) (202), Rv1733c (202, 203), PE/PPE proteins (2, 243, 244, 248), MPT64 (Rv1980c) (246, 247), Mtb8.4 (2), and resuscitation-promoting factors (Rpfs) (2, 228). These antigens have been reported to be attractive vaccine candidates for preventing and controlling TB. Herein, we will summarize the peptide-based vaccines developed from these protective antigens.
3.2.1 Peptide-Based Vaccines Derived From ESAT-6 Family Proteins
ESAT-6 family antigens are low-mass fractions of culture filtrates of M. tuberculosis (249). Previous studies reported that ESAT-6, CFP10, and TB10.4 antigens belong to ESAT-6 family members, they play an essential role in TB pathogenesis and induced significantly enhanced humoral and cellular responses in animal models or clinical trials (250–256). This evidence lay the foundation for constructing peptide-based vaccines based on ESAT-6 family antigens. Aagaard CS et al. identified an immunodominant peptide ESAT-6 (Rv3875) 1–15 (MTEQQWNFAGIEAAA) that can be recognized by the splenocytes of CB6F1 mice and triggered a significant release of IFN-γ (223). To further investigate the role of ESAT-6 1–15 epitope in ESAT-6 full-length antigen, they compared the protective efficacy of a full-length ESAT-6 vaccine and a Δ15ESAT-6 vaccine with the absence of ESAT-6 1–15 epitope. Surprisingly, although both vaccines decreased bacterial numbers of the lung at the early time point, only the Δ15ESAT-6 vaccine revealed significant protection at the long time point (223). These data suggest that the ESAT-6 1–15 immunodominant epitope may negatively affect the full-length ESAT-6 vaccine, reminding that excluding some epitopes may be a potential approach to construct a more protective vaccine. Besides ESAT-6 1–15 epitope, other immunodominant epitopes of ESAT-6 antigen were also determined by different studies. Olsen AW et al. investigated the vaccine potential of two peptides, ESAT-6 1–20 (MTEQQWNFAGIEAAASAIQG) and ESAT-6 51–70 (YQGVQQKWDATATELNNALQ), in B6CBAF1 (H-2b,k) mice. The results showed that both peptides were recognized by CD4+ T cells and induced a significantly higher IFN-γ release, but only the vaccine based on the ESAT-6 51–70 peptide promoted significant protection against M. tuberculosis infection (222).
More recently, Jiang Q et al. constructed a recombinant DNA vaccine (pIRES-epitope-peptide-FL) encoding three T cell peptides of ESAT-6 antigen, including ESAT-6 4-18 (QQWNFAGIEAAASAI), ESAT-6 22-36 (VTSIHSLLDEGKQSL), and ESAT-6 56-70 (QKWDATATELNNALQ) peptides (238). Results revealed that pIRES-epitope-peptide-FL vaccination increased the proliferation of IFN-γ+ T cells, induced significantly higher levels of IFN-γ and IL-12 but relatively lower levels of IL-4 and IL-10, and enhanced protection from M. tuberculosis challenge in C57BL/6 mice (238). The results of the above studies suggest that there is competition between ESAT-6 1–15 or ESAT-6 1–20 epitope and other epitopes of ESAT-6 antigen, and ESAT-6 1–15 and ESAT-6 1–20 epitopes may weaken the recognition ability of other epitopes to MHC molecule (222). It may explain why the Δ15ESAT-6 vaccine or ESAT-6 51–70 peptide vaccine is more protective than the ESAT-6 vaccine or ESAT-6 1–20 peptide vaccine. These results provide a new strategy that synthetic long peptides (SLPs) in peptide-based vaccine design may attenuate the adverse effects of some epitopes and improve the immunogenicity and vaccine efficacy (116). Furthermore, ESAT-6 is a virulence factor secreted by M. tuberculosis, the safety of this protein and their peptides should be considered in designing a peptide-based vaccine.
3.2.2 Peptide-Based Vaccines Derived From Ag85A and Ag85B Proteins
M. tuberculosis Ag85 complex consists of three homologous proteins, including Ag85A (Rv3804c), Ag85B (Rv1886c), and Ag85C (Rv0129c), which induce strong humoral and cellular immune responses (257). They play critical roles in virulence, preventing the formation of phagolysosomes and drug-resistant TB of the pathogen (258). Therefore, Ag85 complex proteins have been utilized by scientists to construct TB vaccines, such asrBCG30 (259), AERAS-422 (rBCG::Ag85A-Ag85B-Rv3407) (260), MVA85A (AERAS-485) (261), Ad35/AERAS-402 (262), Ad5Ag85A (262), ChAdOx1.85A (263), and AEC/BC02 (264). Previous studies have shown that Ag85A and Ag85B proteins are rich in epitope resources, giving them a distinct advantage in constructing peptide-based vaccines (257, 265). Kumar S et al. generated a vaccine mixture (peptide-TLR agonist-liposomes, abbreviation for PTL), consisting of three Ag85B peptides, four ESAT-6 peptides, TLR2 agonist Pam3Cys-SK-4, and TLR9 agonist CpG ODN (Table 3) (239). Interestingly, the BCG-PTL coimmunization enhanced the proportion of vaccine-induced Tcm cells and polyfunctional cytokine responses and increased the defensive efficiency against TB compared with BCG vaccination (239). Linear T cell epitopes are usually short and therefore less immunogenic and stable in vivo. It is an effective strategy to enhance the immunogenicity and presentation of epitopes by using conjugation and palmitoylation approaches. To investigate this strategy, Horváti K et al. developed three peptide-based vaccines termed A/P/I mix, A(P)I, and Pal-A(P)I, respectively (116). The similarity of the three vaccines is that they consist of Ag85B 239-247 (KLVANNTRL), isoniazid inductible gene protein IniB (Rv0341) 33-45 (GLIDIAPHQISSV), and PPE68 (Rv3873) 127-136 (FFGINTIPIA) peptides. The difference is that A/P/I mix is a mixture of three peptides, A(P)I is a conjugation of three peptides separated with a tuftsin sequence (TKPKG), and Pal-A(P)I is the palmitoylated A(P)I. As expected, the internalization rates of A(P)I and Pal-A(P)I vaccines were higher than these of A/P/I mix in human murine bone marrow-derived macrophages (BMDMs) or MonoMac6 human monocytes (MM6), especially the Pal-A(P)I vaccine. Immunization with Pal-A(P)I vaccine induced significantly higher levels of splenocytes proliferation and Th1-type cytokines, and lower numbers of bacteria in the lung or spleen of mice. This exploration suggests that conjugation and palmitoylation are a promising route to enhance the immunogenicity and protective efficacy of peptide-based vaccine.
In addition to the conjugation and palmitoylation routes described above, other novel vaccination strategies have been developed to design peptide-based vaccines. Recently, Chesson CB et al. reported a vaccination strategy based on self-assembling peptide-nanofibers to present TB10.4 (Rv0288) 4–11 (IMYNYPAM) and Ag85B (Rv1886c) 280–294 (FQDAYNAAGGHNAVF) peptides (240). It was found that intranasal immunization with self-assembling peptide-nanofibers induced an 8-fold expansion in multifunctional CD8+ T cell populations and bacterial loads in the lungs of mice primed with BCG and boosted intranasally with co-assembled nanofibers of TB10.4 4–11 peptide and Pam2Cys adjuvant showed a 1.3 log10 CFUs reduction compared to naïve mice (240). Thus, the utilization of new materials or adjuvants can significantly improve the immunogenicity and protection efficiency of peptide-based vaccines. Furthermore, the BCG prime-boost strategy can also considerably enhance the protection efficiency of peptide-based vaccines. Sugawara I et al. confirmed the prime-boost strategy by developing a recombinant (Ag85A) BCG vaccine, an Ag85A DNA vaccine, and an Ag85A (Rv3804c) 141-160 peptide (241). The results presented that the recombinant BCG Tokyo (Ag85A) or Ag85A DNA vaccination boosted with Ag85A 141-160 peptide could remarkably reduce pathological lesions and CFUs burden in the lung of guinea pigs.
3.2.3 Peptide-Based Vaccines Derived From 16-kDa Alpha-Crystallin (Acr, Rv2031c) Protein
Acr, also known as HspX and Hsp16.3, is a heat shock protein localized in the inner membrane. This protein plays an essential role in maintaining the long-term survival during latent or asymptomatic infection and slowing the growth of M. tuberculosis in the early stage of active disease (266, 267). Previous study has suggested it as a subunit vaccine candidate (268). Currently, three peptides from Acr protein have been used to develop peptide-based vaccines, including Acr 31–50 (LRPTFDTRLMRLEDEMKEGR), Acr 91–104 (SEFAYGSFVRTVSL), and Acr 91–110 (SEFAYGSFVRTVSLPVGADE) peptides (Table 3). Shi C et al. compared the immune responses and protection of Acr protein vaccine and its peptide Acr 91–104 mixed with TLR4 agonist DDA-MPLA (N, N’-dimethyl-N, N’-dioctadecylammonium bromide-Monophosphoryl lipid A) on BALB/c mice. They observed that, compared to the BCG vaccine, both vaccines induced significantly higher levels of antibodies, splenolymphocyte proliferation, lower levels of IFN-γ, and equivalent protection (242). Two previous studies constructed a peptide-based vaccine termed L91 by linking Acr 91–110 (SEFAYGSFVRTVSLPVGADE) peptide to TLR-2 agonist Pam2Cys. Both studies found similar results that L91 vaccination stimulated significantly higher levels of Th1 and Th17 immune responses and induced significantly lower CFUs in the lungs of BALB/c mice or Duncan-Hartley guinea pigs than BCG vaccine (146, 210). The possible immune protective mechanism of the L91 vaccine is to reduce the inhibitory effect of M. tuberculosis on APCs by enhancing the expression of NF-κB and iNOS (146, 269). Interestingly, to achieve better protection, Rai, PK et al. improved the L91 vaccine by incorporating a CD8 T cell epitope TB10.4 20-28 (GYAGTLQSL), and the new peptide-based vaccine was named as L4.8 (115). The results showed that the L4.8 vaccination elicited significantly higher levels of CD4+ and CD8+ T cells immunity, and the BCG-L4.8 prime-boost strategy resulted in better protection against M. tuberculosis infection than L91 and BCG vaccines. It can be seen from the above studies that peptide-based vaccines using both HTL and CTL peptides as well as agonists or helper peptides can induce stronger CD4+ and CD8+ T cell immunity to improve their protective effect (115, 270).
Furthermore, two additional studies were performed to improve the immunogenicity and protection of Acr 91-110 peptide by adding other peptides, such as TB10.4 1-13 (MSQIMYNYPAMLG), TB10.4 78-94 (ANTMAMMARDTAEAAKW), Rv0476 1-19 (MLVLLVAVL VTAVYAFVHA), CFP10 (Rv3874) 71-90 (EISTNIRQAGVQ YSRADEEQ), Acr 21-40 (LFAAFPSFAGLRPTFDTRLM), Hsp65 (Rv0440) 3–13 (KTIAYDEEARR), Ag85B (Rv1886c) 56–64 (PSMGRDIKV), 19 kDa (Rv3763) 51–61 (KVVIDGKDQNV), Acr 31–50 (LRPTFDTRLMRLEDEMKEGR) and Rv1733c 63–77 (AGTAVQDSRSHVYAH) (202, 203). As expected, the results revealed that peptide-based vaccine with multi-peptides could induce significantly higher levels of IgG antibodies, IFN-γ, TNF, and IL-2 cytokines, and lower CFUs in lungs of C57BL/6 mice (211) or HLA-DR3 transgenic mice (202).
3.2.4 Peptide-Based Vaccines Derived From PE/PPE Family Proteins
In M. tuberculosis, PE/PPE family has up to 167 members, most of which are located on the surface of M. tuberculosis or secreted out of the bacteria and can be well recognized by the host immune system. Despite the function of most PE/PPE family members is still unknown, accumulating studies indicate that PE/PPE family members related to the ESAT6 family are considered as “immunogenicity islands” due to their high immunogenicity and immunopathogenic (271; 248). This evidence suggests that PE/PPE proteins may be promising candidates for the design of peptide-based vaccines. Wu M et al. designed a multi-epitope DNA vaccine termed as pPES by grafting four Th1 epitopes ESAT-6 1–20 (MTEQQWNFAGIEAAASAIQG), Ag85B 241–255 (VANNTRL WVYCGNGT), PE19 (Rv1791) 4–18 (VTTQPEALAAAAANL), PPE25 (Rv1787) 241–255 (AQFFASIAQQLTFGP), and a CTL epitope MTB10.4 (Rv0288) 3–11 (QIMYNYPAM) into Hsp65 (Rv0440) scaffold protein (243). pPES vaccination generated HSP65-specific antibodies, induced higher levels of IFN-γ+CD4+ T cell response, multi-functional CD4+ T cell response, cytotoxic CD8+ T cell response, and lower bacterial loads in lungs and spleens of mice (243). These data indicated that epitope grafting did not reduce the immunogenicity of HSP65 protein, and epitope grafting strategy may be a potential method to construct peptide-based vaccines. A study identified 21 immunodominant peptides from 167 proteins of the PE/PPE family and constructed three peptide-based vaccines by fusing these peptides to ESAT-6 protein, including PE peptides + ESAT-6 (shorten as PE-ESAT-6), PPE peptides + ESAT-6 (PPE-ESAT-6), and PE + PPE peptides + ESAT-6 (PE/PPE-ESAT-6) (244). The results showed that, compared to control, PE/PPE-ESAT-6 immunization induced significantly higher levels of IFN-γ, multifunctional CD4+CD44+CD62L- T cells, and lower CFUs loads in lungs and/or spleens of C57BL/6J mice.
We recently developed a novel peptide-based vaccine termed as MP3RT (2). This vaccine is made up of six immunogenicity HTL peptides, including Mtb8.4 (Rv1174c) 69-83 (LRNFLAAPPPQRAAM), PPE18 115-129 (RAELMILIATNLLGQ), PPE18 (Rv1196) 149-163 (AAAMFGYAAATATAT), PPE68 (Rv3873) 138-152 (DYFIRMWNQAALAME), RpfA (Rv0867c) 377-391 (AYTKKLWQAIRAQDV), and TB10.4 (Rv0288) 21-35 (YAGTLQSLGAEIAVE). To evaluate the effect of epitope MHC restriction on the immunogenicity and protective efficiency of the MP3RT vaccine, humanized C57BL/6 mice and wild- C57BL/6 mice were used. Our results showed that MP3RT induced significantly higher levels of IFN-γ and CD3+IFN-γ+ T lymphocytes and lower CFUs in the lungs and spleens of humanized mice rather than wild-type mice (2). The same method was used to evaluate other peptide-based vaccine named as ACP that only contains three peptides Ag85B (Rv1886c) 12-26 (GRRLMIGTAAAVVLP), CFP21 (Rv1984c) 12-26 (VVVATT LALVSAPAG), and PPE18 (Rv1196) 149-163 (AAAMFGYAAATA TAT) (9). We found that although ACP induced significant humoral and cellular immune responses in humanized mice, its protective efficiency was not significantly better than that of the phosphate buffer solution (PBS) control. Taken together, these data once again demonstrated that grafting or fusion of multiple immunodominant epitopes on the protective antigen skeleton could significantly improve the immunogenicity and protection efficiency of the antigen, and these findings provide new ideas for the construction of peptide-based vaccines for TB.
3.2.5 Peptide-Based Vaccines Derived From MPT64 (Rv1980c) Protein
The MPT64 protein is an immunogenic protein initially isolated from the culture filtrate of the BCG Tokyo strain (272). Previous studies have shown that MPT64 protein contains T or B cell epitopes, inducing strong humoral or cellular immune responses (273). Therefore, MPT64 protein is a promising candidate for constructing a peptide-based vaccine. Peptide MPT64 190-198 (FAVTNDGVI) has received more attention in recent years. Professor Zhu BD et al. developed a peptide-based vaccine Ag85B-MPT64(190-198)-Mtb8.4 (named as AMM). They investigated its immunogenicity and capacity to boost BCG-primed immunity in a DDA-BCG PSN adjuvant (dimethyl-dioctyldecyl ammonium bromide and BCG polysaccharide nucleic acid). They found that BCG-AMM prime-boost vaccination induced significantly higher levels of immune responses and better protection than BCG or AMM vaccination alone (245). Subsequently, they further confirmed this vaccine and developed a novel vaccine named AMH that consists of Ag85B-MPT64(190-198)- HspX (246). Compared with the mice receiving BCG only, the mice boosted with AMH, AMM, or their combination (AMH+AMM) showed significantly higher levels of specific antibodies and IFN-γ+ T cells. In addition, the mice boosted with the combination of AMM and AMH had substantially lower bacterial counts in the lungs, whereas mice boosted with AMH or AMM did not. Heterogeneity of protective effect of AMM vaccine in both studies may be related to vaccine dose. Analysis of the two studies showed that all parameters were identical except the vaccine dose. In their first study, they used 20 µg of the AMM vaccine, but in the second study, the vaccine dose was reduced to 10 µg. It indicates that vaccine dose significantly affects its protection efficiency, suggesting that future studies should select an appropriate vaccine dose to immunize mice to avoid vaccine failure due to this factor.
More recently, Zhu’s team modified and upgraded the AMM vaccine and constructed a new peptide-based vaccine called LT70, which consists of ESAT6-Ag85B-MPT64(190-198)-Mtb8.4-Rv2626c (247). They observed that LT70 was well recognized by T cells obtained from TB patients and LTBI volunteers and induced dramatically higher levels of cellular and humoral immunity as well as protective efficacy compared to BCG vaccine or PBS control in C57BL/6 mice. There were significant differences in experimental design between this study and the previous two studies. For example, the vaccine dose was adjusted to 13 μg, BCG-PSN adjuvant was replaced by Poly (I:C) adjuvant, and the route of the challenge was changed from intravenous injection to respiratory aerosol inhalation. These optimizations and improvements have contributed to the improved immune protection efficiency of the LT70 vaccine.
3.2.6 Peptide-Based Vaccines Derived From Rv1733c Protein
Rv1733c is a probably conserved transmembrane protein of M. tuberculosis and belongs to dormancy survival regulon antigens (DosRs) related to LTBI (5). Rv1733c protein has been considered an immunopotent T cell candidate of the 45 top-ranking antigens (274). Black GF et al. compared the immunogenicity of 51 DosR regulon-encoded M. tuberculosis recombinant proteins among 131 individuals from Uganda, Gambia, and South Africa. They found that of the 51 DosRs, Rv1733c is one of the most frequently recognized DosRs in all three population groups (275). Furthermore, it has been shown that Rv1733c also induces strong IFN-γ response in T cells collected from tuberculin skin test positive (TST+) individuals (276) and a Rv1733c DNA prime followed by boosting with Rv1733c protein increased T cell proliferation and IFN-γ secretion in mice (277).
Thus, it can be seen that Rv1733c has good immunogenicity and is expected to be a new vaccine candidate for fighting against LTBI. Coppola M et al. investigated an SLP Rv1733c 57–84 (IPFAAAAGTAVQDSRSHVYAHQAQTRHP) derived from Rv1733c protein and assessed its immunogenicity and protective capacity in HLA-DRB1*0301/DRA transgenic mice (203). After three times’ immunization, the mice vaccinated with Rv1733c SLP and TLR9 agonist CpG ODN1826 showed significantly higher levels of IFN-γ+ TNF+ and IFN-γ+ CD4+ T cells and Rv1733c protein-specific antibodies. Interestingly, compared with mice vaccinated with BCG only, the mice primed with BCG and boosted with Rv1733c SLP revealed the highest reduction in CFUs burdens in lungs (203). Furthermore, Geluk A et al. also evaluated the immunogenicity of another peptide Rv1733c 63–77 (AGTAVQDSRSHVYAH) in HLA-DRB1*03:01/DRA transgenic mice. It was found that Rv1733c 63–77 stimulated significantly higher levels of IFN-γ in splenocytes harvested from HLA-DR3 mice infected with M. tuberculosis and showed higher levels of IFN-γ+, TNF+, or IL-2+ CD4+ T cells (202). These data suggest that Rv1733c SLP may be a potential booster vaccine for TB.
4 Protein-Based Bacterial Vaccine Delivery System
In recent years, subunit vaccines, especially peptide-based vaccines with more single and safer components, have gradually become new vaccine forms. However, their weak immunogenicity makes it difficult to induce an adequate immune response and thus often need adding additional adjuvants. With the development of immunology, the delivery system, which aims to enhance antigens targeting secondary lymphoid organs and the activation of APCs, is continuously developed and applied in the vaccine design. Although many potential delivery systems have been widely explored in therapeutic vaccines, there have not been thoroughly studied in prophylactic vaccines because of the higher requirements for the safety of the materials usually applied in healthy people, even the elderly and children. With that in mind, more compatible and safer protein-based delivery vectors have great potential in prophylactic vaccine research (Table 4).
4.1 Self-Assembled Proteinaceous Nanoparticles
Some proteins can assemble into particles of a specific size under natural conditions and have been developed as great delivery systems. One kind is virus-like particles (VLPs) which are artificial nanostructures that self-assembled after the expression of viral capsid protein. It has been reported that poly (ribosylribitolphosphate) (PRP) polysaccharide of Hib was connected to Hepatitis B virus surface antigen (HBsAg) VLP via an adipic acid dihydrazide (ADH) spacer, and stronger IgG antibodies to both the PRP were induced than a commercial conjugate vaccine in mice (279). Similar, VLPs (e.g., Qβ and HBsAg) could also chemically load S. pneumonia capsular polysaccharide. The VLP vaccines could induce serotype-specific IgG antibodies. With synthetic biology and protein glycosylation system development, a new and simpler coupling method between polysaccharide antigens and protein has been explored. Li X. et al. successfully prepared the Shigella conjugate vaccine by using bacterial in vivo protein glycosylation reaction to couple the complete pathogenic bacterial polysaccharide to VLP (AP205) for the first time (281, 364). This VLP based conjugate vaccine showed better immune and protective effects in mice than the conventional vector. Besides, flock house virus VLP and bacteriophage T4 nanoparticle vaccine were explored and exhibited good protection against the challenge (282, 283). Designable self-assembled nanoparticle is another kind of self-assembled proteinaceous delivery vector used in pathogenic bacterial vaccines. Due to its modular design, it is a promising protein vector, which has shown good development potential in the development of vaccines such as viruses, but it seems to have just begun in the field of bacteria. Pan et al. developed a Nano-B5 system to produce self-assembled nano vaccines by fusion expression of bacterial B5 toxin and trimeric peptide and connected polysaccharide antigen through glycosylation in the pathogenic host (284). This particle was about 25 nm, which prolonged retention in draining lymph nodes and could stimulate strong cellular and humoral immune responses. Further, the system could be introduced into a modified E. coli host to prepare exogenous pathogenic bacteria, such as Klebsiella pneumoniae, nano-scaled conjugate vaccine and protect mice from systemic and pulmonary infection (285). Polysaccharide conjugate vaccine is considered the most successful bacterial vaccine at present. Although immunogenicity of carbohydrate antigen itself is very weak, it could be significantly improved by conjugating them (either synthetic short sugar chain or natural polysaccharide) with proteinaceous nanoparticles. Thus, self-assembled proteinaceous has excellent potential to enhance weak antigen immunogenicity and be used in the bacterial vaccine.
4.2 Viral Vector
Vaccines consist of a non-replicating virus that contains certain genetic material from the pathogen that needs to be immunized. It seems to be an ideal vaccine delivery system because of its natural viral structure, which can be well recognized by the immune system (365). The adenovirus vector is widely used (366) to develop a bacterial vaccine. Other viral vectors (e.g. influenza viral and semliki forest virus) have also been explored. McConnell M J et al. described a replication-incompetent adenovirus expressing domain 4 (D4) of B. anthracis protective antigen (PA) (Ad.D4), which could induce a more robust humoral and cellular immune responses than anthrax vaccine absorbed (AVA) (the only one FDA-approved anthrax vaccine which needs to be vaccinated six times within 18 months and enhanced once each year) (367) and provide complete protection against lethal spore challenge in single immunized mice (286). However, pre-existing immunity to Ad in humans may inhibit subsequent immunization-induced antibody responses. Influenza viral vectors may be another promising one for human use because of the lack of pre-existing immunity, safety and immunogenicity, which have been demonstrated in various models (chickens, ferrets and rhesus macaques and humans) (368–370). Tabynov K et al. developed recombinant influenza A viruses of the subtypes Н5N1 and H1N1 expressing Brucella protective antigen (ribosomal protein L7/L12 or Omp16) and strong cellular immune response and protection effect were induced (291). Moreover, these vaccines with adjuvant could provide long term protection for cattle and induced good cross-protection against B. melitensis infection in pregnant heifers and even sheep and goats (371–375). However, influenza viruses expressing B. anthracis PA unable to induced in vitro anthrax toxin neutralization activity antibodies, although the titers against PA were high (287, 376). Interesting, this situation could be solved by heterologous prime/boost immunization strategy, which may be attributed to the B-cell affinity maturation and Ig gene high-frequency mutation in germinal centres by combining different heterologous vectors. Moreover, antigen epitopes (PA232–247 and PA628–637) also could be expressed in a plant-virus Tobacco Mosaic Virus (TMV). However, immunized mice showed almost no protection (377). Possible reasons may be the too weak immunogenicity of short epitopes or plant virus self, and a prime/boost immunization strategy can be tried.
4.3 Lactic Acid Bacteria Vector
Lactic acid bacteria are commonly used as delivery vehicles because they are safe and human friendliness and can stimulate mucosal and systemic immune responses through mucosal pathways. Many kinds of lactic acid bacteria (e.g. L. reuteri, L. casei, L. plantarum and L. plantarum) have been used as delivery vectors, of which L. casei is the most studied (378). Lactic acid bacteria are generally applied for the vaccine design against intestinal and respiratory infection, and there are main two strategies for antigen delivery. One is to express antigen in vivo directly. Studies have shown that an oral vaccine expressing E.coli F41 in L. casei can stimulate strong systemic and local mucosal immune responses simultaneously and protect mice from the lethal challenge, even still achieving more than 80% protection nine weeks after the last immunization (303, 304). In addition, Yu M et al. found that when the antigen was co-expressed with B5 toxins (such as LTB), it induced a more robust mucosal immune response and provided 100% protection (311). Moreover, even some capsular polysaccharides, such as type 3 and type 14 of S. pneumonia, have been successfully expressed in L. lactis, and the immune response of type 3 vaccine was detected, showing that L. lactis is a potential host for capsule vaccine antigens (379). Another strategy is using a non-genetically modified gram-positive enhancer matrix (GEM) particle for antigen delivery. The particles were prepared from living bacteria and had no nucleic acid and cytoplasmic components while maintaining the size and cell wall components of the bacteria. Multiple S. pneumonia protein antigens (e.g., PppA, PpmA, SlrA and IgA1p) have been anchored on the particles by a lactococcal peptidoglycan binding domain and shown to be efficacious against pneumococci in animal models (299, 328, 380). In addition, GEM loading epitope antigens also showed a significant effect. For example, when the H. pylori multi-epitope vaccine (CUE) (based on CTB fusing with T and B cell epitopes from H. pylori urease A and B subunits) was displayed on the surface of GEM, these prophylactic and therapeutic effects in orally immunized mice could further enhanced by inducing mucosal specific antibody responses and local Th1/Th17 cell-mediated immune response (330, 331), which was an optimal immunity type against H. pylori infection (381, 382).
4.4 Outer Membrane Vesicles (OMVs)
A large number of gram-negative bacteria naturally could produce extracellular OMVs, which is from 50 to 250 nm in diameter, suitable for targeting and being phagocytized by APCs (383). OMV contains many components, such as outer membrane proteins (OMPs) and lipoproteins, which are conducive to immune response and various immunogenic antigens. At present, the use of OMVs has become a very promising vaccination strategy. OMVs from many pathogenic bacteria (e.g. Klebsiella pneumoniae, B. pertussis, E. coli, Shigella, Vibrio cholerae, Salmonella, Helicobacter pylori and Neisseria meningitidis group B) has been proved to have the ability to stimulate humoral and cellular immune responses and provide good protective effect after immunization (337, 384–386).
Because natural OMVs often contains toxic components like LPS, which could induce host inflammatory responses, many studies have focused on reducing the toxicity of OMVs by deleting the lipid A related genes (e.g. msbB, htrB, pagP, lpxL, or pagL) (355, 387–390) or toxin genes (391, 392). For example, Kim S H et al. generated OMVs from E. coli O157:H7 with the mutation of msbB (encoding an acyltransferase catalyzing the final myristoylation step during lipid A biosynthesis) and Shiga toxin A StxA. The reduced toxicity OMVs were immunized by eyedrop in BALB/c mice and showed that it was safe and could induce both humoral and mucosal immune (tear, saliva, and fecal) responses, which is enough to protect the vaccinated animal from the challenge of the lethal HUS-causative agent (wtOMVs) (341). Another example was that the knocking out of lpxL, which is involved in lipid A biosynthesis, in N. meningitidis could result in at least a 200-fold decrease in pyrogenicity than wild-type OMV. The protective effect can be largely restored by adding adjuvants used in humans (393). In addition, Sinha R et al. reported that the OMV-mediated toxicity could be significantly reduced by being pre-treated with all-trans retinoic acid (ATRA), active metabolites of vitamin A, which are anti-inflammatory and mucosal adjuvant properties, and the immunity was enhanced (394). Fredriksen J H et al. produced a group B N. meningitidis OMV vaccines by including an additional step of detergent extraction (395). The detergent extracted outer membrane vesicles contain much fewer LPS (5–8%) and have been helpful in several countries (396). Moreover, a hydroquinone non-pathogenic OMV from E. coli was developed as delivery vehicles by expressing group B glycan antigens (361). However, most bacterial capsular polysaccharides in gram-positive bacteria are difficult to express in gram-negative bacteria E. coli efficiently. The difference of membrane structure and the polysaccharide gene cluster is relatively large (mostly more than 10 kbp), making it difficult for cloning.
OMVs were usually combined with other delivery systems to meet some specific requirements. For example, to solve the problems of the poor size uniformity and low stability of OMV, Wu, G. et al. produced a 70-90 nm sized OMV (from K. pneumoniae) based nanovaccine by depositing the hollow-structured OMVs onto bovine serum albumin nanoparticles. As a result, the OMV could be reinforced from the core-shell structure. The protecting effect against carbapenem-resistant K. pneumoniae (CRKP) was significantly improved after vaccination (354). Camacho A I et al. found that when Shigella OMVs were encapsulated in polyanhydride nanoparticles, a stronger Th1 immune response, which was more needed against intracellular bacteria, was induced (357, 397). OMVs also be encapsulated in biopolymer chitosan, which was used to prepare nanogel particles by ionotropic gelation with tripolyphosphate. After being coated with an enteric polymer, mice were administrated orally and showed better protection against infection after 78 days of immunization, whereas free OMVs have no protection (359). Therefore, although OMV as a delivery carrier faces some problems, it can achieve the expected effect through further optimization and transformation.
4.5 Liposome
The liposome is a kind of phospholipid bilayer sphere formed via self-assembly in water and proved to be a safe and effective delivery system. Although liposomes do not belong to protein delivery carriers, we will still review their application in vaccine design, especially for peptide-based vaccines, because of their wide use (398). The versatility and plasticity characteristics of liposomes make them designable according to different parameter requirements, such as lipid composition, charge, size, entrapment and location (399). Besides loading various antigens in a liposome, adjuvants and/or functional molecules could also be loaded quickly to enhance further the immune effect (400). For peptide antigens, they were usually coupled to liposomes via lipid core peptide technology, which consists of an oligomeric polylysine core conjugated to a series of lipoamino acids for anchoring of the antigen (401, 402), and this strategy has been frequently utilized in Group A Streptococcal (GAS) vaccine studies (403–405). Ghaffar et al. developed a cationic liposome through the film hydration method with dimethyldioctadecylammonium bromide (DDAB). Lipopeptides antigens, entrapped by the liposome, could induce both mucosal and systemic response for a long time in intranasally immunized mice (403). In addition, the high-level antibody reaction was further confirmed in various sizes (70 nm to 1000 nm) of the carriers (406). In addition, some polymer, such as polyethylenimine (PEI), which could attach to the cells’ surface and deliver cargo into endosomal and cytosolic compartments, was introduced in the lipopeptide-based vaccine design. PEI incorporated in liposome peptide vaccine could induce significant specific mucosal and systemic antibodies, which effectively opsonize multiple isolates of clinically isolated GAS (407). Further, they found that the ratio of PEI, rather than molecular weight, present in the liposome vaccines impact immune response (408). Besides, the addition of some functional elements can realize the immune enhancement of vaccines. For example, Yang et al. designed a cell-penetrating peptides (CPPs)-liposome delivery system on the liposomal nanoparticles, in which CPP could enhance both cellular and humoral immune responses through direct delivery of antigen into the cytoplasm and from the endocytic pathway (409–411). Nasal immunization of the vaccine in mice could induce antibodies that showed high opsonic activity against clinically isolated GAS strains (412). Liposomes have also been used in the study of TB vaccines. Dimethyldioctadecylammonium (DDA) could self-assemble into closed vesicular bilayers in water similar to liposomes and was known as an effective adjuvant for eliciting cellular and humoral responses (413, 414). However, the physical instability of the DDA liposomes limits its application. To solve this problem, Davidsen et al. incorporated a glycolipid trehalose 6,6′-dibehenate (TDB), comprising a 6,6′-diester of α,α′-trehalose with two long 22-carbon acyl chains, into the DDA liposome bilayers. By loading tuberculosis vaccine antigen Ag85B–ESAT-6 fusion protein and immunization of mice, a robust specific Th1 type immune response was induced (415). The post-challenge bacterial growth of M. bovis BCG was reduced in adult or neonatal murine (416), suggesting the increase of adjuvant efficacy of DDA liposomes. In conclusion, the characteristics of liposomes endow them with more designability, and some deficiencies can be solved by further transformation and optimization so that liposomes have great potential in peptide vaccine delivery design.
5 Animal Models for Peptide-Based Vaccines
Many animal models have been utilized to develop TB vaccines, ranging from expensive none-human primates (NHPs) to small non-mammals such as zebrafish. In comparison, NHP, which can well reflect the human immune response and susceptibility to TB, has been used in many preclinical experiments. At the same time, smaller animal models such as mice, rats, guinea pigs, rabbits and zebrafish are generally more suitable for studying narrower aspects of the immune response to Mycobacterium tuberculosis, such as granuloma formation, susceptibility to different strains, or immunogenicity of vaccine candidates.
NHP represents one of the most frequently used and most important models when testing vaccines against M. tuberculosis infection. This model has significant similarities in human physiology, genome, and immune response (417, 418). Rhesus macaques and cynomolgus are the most commonly used NHPs in TB infections for vaccine evaluation (417, 419–421). Today, the NHP models have become indispensable for the preclinical evaluation of vaccine effects. However, some of the difficulties in using NHP models to evaluate anti-tuberculosis vaccines are the considerable investment requirements for the maintenance and use of BLS-3 biosafety facilities, the lack of commercial molecular and immune reagents, and the timely availability of sufficient animals. Compared with the NHP model, the advantages of using the mouse model include the availability of genetically modified strains, more common molecular and immunological reagents on the market, and lower cost of feeding and specialized containment facilities. These characteristics ensure that the mouse model is always the first choice for TB vaccine research, especially for exploratory studies on vaccines before preclinical evaluation using NHP models. Although common mouse strains exampled as C57BL/6 and BALB/c are often not susceptible to tuberculosis infection, they are still widely used in vaccine research. To date, most M. tuberculosis peptides are discovered in the C57BL/6 model (Table 3).
The use of mice is ubiquitous in scientific research. Still, the experimental results obtained on rodents, and primarily murine, in reality, are often very different from the clinical immune response of humans. Moreover, this difference in immune response has a certain relationship with the species differences between animals and humans, causing the development of many novel vaccines and drugs to stagnate or even fail to continue when they reach clinical trial phase I/II. Therefore, the development of small animal models that can more accurately reflect the characteristics of human immune response is a problem that deserves special attention.
MHC is one of the gene groups with the most polymorphism in humans and mammals. It induces and regulates innate immunity and adaptive immune response and participates in the development and maturation of T lymphocytes, the presentation of exogenous/endogenous antigens and immune signals, as well as the establishment of central immune tolerance (422). At the same time, MHC is closely related to the occurrence and progression of many autoimmune diseases and chronic diseases and has essential biological functions and significance. For example, the HLA-A11 subtype is closely associated with the occurrence of many infectious diseases, such as familial otosclerosis (423, 424), TB (425), leprosy (426), epilepsy and cytomegalovirus infection (427), etc. In addition, increased specific expression of the HLA-A11 gene was found in patients with upper laryngeal cancer (428) and osteosarcoma (429). Furthermore, HLA-A11, DR3 and DR4 subtypes played a synergistic role in the occurrence of autoimmune hepatitis (430). In addition to HLA-A11, other individual MHC subtypes also play a significant role in the disease process after HIV-1 infection (431–435). In recent years, how to use and exert the biological functions of HLA through animal models has gradually become a research hotspot in animal models, and the first is the development of new vaccines based on MHC-restricted CTL and HTL epitopes.
Mice and humans share more than 95% of genes and about 80% of genetic material. As small animal models, mice are widely used in vaccines and drugs preclinical trials (436). Although the MHC of the mouse (H-2) and human (HLA) are very similar in structure and function, there are still significant differences in the presentation of antigens (437), and the dominant antigen peptides presented also have different structural characteristics. Therefore, the MHC humanized mouse has become an essential model for epitope research and the development and evaluation of epitope vaccines.
In recent years, MHC humanized mouse models have played a vital role in developing and evaluating disease immune pathogenic mechanisms, vaccines, and drugs. This mouse model has also undergone a continuous development and progress stage. In the first stage, the whole HLA molecule is usually directly transferred into the mouse genome, such as HLA-B27 mice (438), HLA-B7 mice (439), HLA-A2 mice (440), HLA-Cw3 mice (441) and other early developed models. However, the binding force between the α3 functional region in the human HLA molecule and the mouse CD8 is weak (442–444). At the same time, the presence of mouse MHC (H-2) leads to competitive inhibition of mouse H-2-I restricted immune response to human HLA-I restricted immune response (445). In this stage, the mouse immune response is still dominant in the model, which cannot reflect the function and role of human HLA molecules alone in the immune response. Studies have shown that when H-2 is present in mice, the expression of HLA transgenic molecules on the surface of mouse lymphocytes is significantly reduced (446). In the second stage, the scientists improved the MHC humanized mouse model through two methods. One is to transfer the gene fragment encoding human CD4+ or CD8+ into the mouse genome so that the mouse can express both murine and human CD4+ or CD8+ molecules (447), which can effectively improve the binding efficiency of HLA and CD4 or CD8 accessory molecules, and efficiently start the second signal of antigen presentation. Another method is to optimize the structure of HLA transgenic molecules, replace the transmembrane α3 functional region with murine α3 functional region, and construct a chimeric HLA molecule (HHM). The murine α3 structure can promote HLA and mouse CD4 or mouse CD8. Representative mouse models include the HLA-B27 (HHM) mice model (448, 449). After optimization of the above two methods, the mouse model can produce a certain HLA-restriction-specific response. However, most cellular immune response is still regulated by mouse H-2 molecules. In the third stage, the researchers adopted a new construction strategy to design transgenic vectors and constructed a chimeric transgenic vector (HHD) (450) of HLA and H-2, including the promoter of HLA-I, light chain β2m, and heavy chain. The α1 and α2 functional regions, the α3 functional region and the transmembrane region of H-2-Db, and the important components β2m and IAβ of mouse H-2 are also knocked out. For example, in the HLA-A11 humanized mouse model (451), the α3 functional area of mouse H-2 is used to replace the α3 functional area of human HLA-A11, which effectively enhances the binding force between the human HLA-A11 molecule and the mouse CD8 and avoids the complexity of transferring human CD8 into the mouse genome. At the same time, knock out the important components β2m and IAβ (the β chain of the IA molecule) of the mouse-derived H-2 I and II molecules, and replace them with fragments larger than the originals. Such pseudogene fragments of the gene achieving the purpose of silencing the expression of mouse H-2 molecules. Therefore, in this type of HLA-I humanized mice, the competitive inhibition of the H-2-I restricted reaction is eliminated, and only the HLA-I restricted immune response can be produced. The humanized mouse model of HLA-II was also constructed using the same strategy. The resulting HLA-I/II humanized mouse model was replaced with human HLA molecules at the level of MHC-I and II molecules. HLA exerts a restrictive function in antigen presentation, regulates the immune response in mice, and enables MHC mice to be “humanized” to a greater extent, which can more effectively simulate human immune response at the HLA level. The representative HLA-A2/DR1 and HLA-A11/DR1 transgenic mouse models have been well applied in HIV、EBOV and SARS-CoV-2 epitope screenings (451–453). Encouragingly, the MHC humanized mouse model has been well used for TB peptide-based vaccine research (2, 9, 198, 202, 203).
Although the MHC humanized mouse model can effectively simulate the human immune restriction and affect the immune function. However, as a better model for epitope vaccine research and evaluation, MHC humanized mouse models have two problems that need to be optimized in future development. On the one hand, it makes MHC humanized mouse models sensitive to pathogens. On the other hand, mice are not susceptible to many human pathogens or cannot be infected due to differences in receptors. For example, regular mice are not sensitive to SARS-CoV-2, SARS-CoV, MERS-CoV, and many subtypes of influenza viruses. Therefore, it requires changing the virus (constructing a mouse-adapted strain) or changing the animal (pathogen-receptor humanized mouse) to obtain a sensitive mouse model. Another aspect is to combine MHC humanized mice with immunodeficient mice for better humanized immune reconstitution. At present, although NOD/SCID mice have played a critical role in the research fields of immune transplantation and tumor immunity, the transplanted human-derived cells have undergone development and differentiation in the mouse thymus. However, the MHC obtained restriction is still restricted by mouse H-2, and it is impossible to carry out research on specific HLA-restricted CTL and Th immune responses. Therefore, through the combination of MHC humanized mice and immunodeficient mice, immune reconstitution can be humanized, and a more real humanized immune system can be realized.
6 Conclusions and Future Perspectives
Vaccination has been considered as the most effective strategy to eliminate TB infection. Accumulating studies have showed that peptide-based vaccines are promising vaccine candidates for preventing and controlling TB due to their advantages, such as aggregation of immunodominant epitopes, preservation of peptide structure, good stability, easy to store and transport, lower cost, and decreased side effects. Furthermore, the rapid development of bioinformatics technology provides a tool for predicting and constructing peptide-based vaccines, which dramatically saves time and reduces the cost of peptide-based vaccine research. Herein, we give a detailed description of how to design a peptide vaccine using an immunoinformatics approach, including determination of protective antigens, T and B cell epitope prediction, screening of immunodominant epitopes, and selection of selection linkers, adjuvant or helper peptides, codon optimization, and in silico analysis. We further reviewed the peptide-based vaccine candidates worldwide based on this basic knowledge. We found that 150 previous articles related to peptide-based vaccines for TB are being investigated in pre-clinical studies, including 76 studies in epitope screening and prediction, 45 studies in evaluating immunogenicity, 8 studies in peptide-based vaccine construction, and 21 in assessing vaccine efficacy in animal models. However, some drawbacks of peptide-based vaccines should not be ignored, such as weak immunogenicity for a single peptide, MHC restriction, and high requirements for animal models.
In the future, these disadvantages can be solved by the following strategies: (1) a detailed understanding of the potential cellular and molecular mechanisms involved in peptide-based vaccine immunity is the key to improving its immunogenicity and protective efficiency (454); (2) improving the vaccine construction techniques, including broad antigen repertoire, SLPs, conjugation and palmitoylation of peptides, grafting epitopes into a protective antigen; (3) using appropriate linkers, helper peptides, TLR agonists, adjuvants, and potential delivery systems to enhance the immunogenicity; (4) primed with BCG and boosted with peptide-based vaccines; (5) employing transgenic animal models with human HLA molecules to evaluate peptide-based vaccines.
Author Contributions
Conceptualization: XW and WG. Data curation: WG, CP, PC, JW, and GZ. Formal analysis: WG. Funding acquisition: WG. Methodology: WG, CP, PC, JW, and GZ. Software: WG. Writing - original draft: WG, CP, and GZ. Writing - review & editing: WG and XW. All authors contributed to the article and approved the submitted version.
Funding
This study was funded by the National Natural Science Foundation of China (Grant No. 81801643), Beijing Municipal Science & Technology Commission (Grant No. 19L2152), and Chinese PLA General Hospital (Grant No. QNC19047).
Conflict of Interest
The authors declare that the research was conducted in the absence of any commercial or financial relationships that could be construed as a potential conflict of interest.
Publisher’s Note
All claims expressed in this article are solely those of the authors and do not necessarily represent those of their affiliated organizations, or those of the publisher, the editors and the reviewers. Any product that may be evaluated in this article, or claim that may be made by its manufacturer, is not guaranteed or endorsed by the publisher.
Supplementary Material
The Supplementary Material for this article can be found online at: https://www.frontiersin.org/articles/10.3389/fimmu.2022.830497/full#supplementary-material
Supplementary Table S1 | Summary of studies related with peptide or epitope candidates against Mycobacterium tuberculosis.
Abbreviations
ADH, adipic acid dihydrazide; ATRA, all-trans retinoic acid; ANN, Artificial neural network; APCs, antigen presenting cells; AUCs, areas under the curve; BCG, Bacillus Calmette-Guérin; BMDCs, bone marrow derived DCs; BMDMs, bone marrow-derived macrophages; CAI, Codon Adaptation Index; CFP-10, culture filtrate protein 10; CRKP, carbapenem-resistant K. pneumoniae; CTB, cholera toxin subunit B; CTL, cytotoxic T-lymphocytes; COVID-19, coronavirus disease 2019; DCs, dendritic cells; DosRs, dormancy survival regulon antigens; ELISpot, enzyme-linked immunospot; ESAT-6, early secreted antigenic target 6; GEM, gram-positive enhancer matrix; HBD, human β-defensin;, HBHA, heparin binding hemagglutinin; HBsAg, Hepatitis B virus surface antigen; HTL, helper T lymphocytes; HIV, human immunodeficiency virus; HLA, human leukocyte antigen; HMM, Hidden Markov Model; IEDB, Immune Epitope Database and Analysis Resource; IFN-γ, interferon-γ; IL, Interleukin; IP-10, interferon gamma inducible protein 10; LTBI, latent TB infection; MCC, Matthew’s correlation coefficient; MDR-TB, drug-resistant and multidrug-resistant TB; MM6, MonoMac6 human monocytes; MHC, major histocompatibility complex; NCBI, National Center of Biotechnology Information; NMR, nuclear magnetic resonance; NK, natural killer; ODN, oligonucleotides; OMVs, Outer membrane vesicles; Pam2Cys, dipalmitoyl-S-glyceryl cysteine; PA, protective antigen; PAMPs, pathogen associated molecular patterns; PBS, phosphate buffer solution; pDCs, plasmacytoid dendritic cells; PLS, partial least squares; pMHC, major histocompatibility complex presented antigenic peptides; PRP, ribosylribitolphosphate; PSMα4, phenol-soluble modulin α4; QSAR, Quantitative Structure Activity Relationship; RD, region of difference; Rpfs, resuscitation-promoting factors; RplL, 50S ribosomal protein L7/L12; SCXRD, single-crystal X-ray diffraction; SLPs, synthetic long peptides; SMM, Stabilized matrix method; SVM, Support Vector Machine; TB, tuberculosis; Th, helper T-lymphocytes; TLR, toll-like receptor; TMV, Tobacco Mosaic Virus; TNF-α, tumor necrosis factor-α; TST, tuberculin skin test; VLPs, virus-like particles; WHO, World Health Organization.
Glossary
References
1. Gong W, Liang Y, Wu X. The Current Status, Challenges, and Future Developments of New Tuberculosis Vaccines. Hum Vaccin Immunother (2018) 14(7):1697–716. doi: 10.1080/21645515.2018.1458806
2. Gong W, Liang Y, Mi J, Jia Z, Xue Y, Wang J, et al. Peptides-Based Vaccine MP3RT Induced Protective Immunity Against Mycobacterium Tuberculosis Infection in a Humanized Mouse Model. Front Immunol (2021) 12:666290(1393). doi: 10.3389/fimmu.2021.666290
4. Allué-Guardia A, García JI, Torrelles JB. Evolution of Drug-Resistant Mycobacterium Tuberculosis Strains and Their Adaptation to the Human Lung Environment. Front Microbiol (2021) 12:612675. doi: 10.3389/fmicb.2021.612675
5. Gong W, Wu X. Differential Diagnosis of Latent Tuberculosis Infection and Active Tuberculosis: A Key to a Successful Tuberculosis Control Strategy. Front Microbiol (2021) 12:745592(3126). doi: 10.3389/fmicb.2021.745592
6. Aspatwar A, Gong W, Wang S, Wu X, Parkkila S. Tuberculosis Vaccine BCG: The Magical Effect of the Old Vaccine in the Fight Against the COVID-19 Pandemic. Int Rev Immunol (2021) 2021:1–14. doi: 10.1080/08830185.2021.1922685 Published online first.
7. Gong W, Aspatwar A, Wang S, Parkkila S, Wu X. COVID-19 Pandemic: SARS-CoV-2 Specific Vaccines and Challenges, Protection via BCG Trained Immunity, and Clinical Trials. Expert Rev Vaccines (2021) 20(7):857–80. doi: 10.1080/14760584.2021.1938550
8. Andersen P, Doherty TM. The Success and Failure of BCG - Implications for a Novel Tuberculosis Vaccine. Nat Rev Microbiol (2005) 3(8):656–62. doi: 10.1038/nrmicro1211
9. Gong W, Liang Y, Mi J, Xue Y, Wang J, Wang L, et al. A Peptide-Based Vaccine ACP Derived From Antigens of Mycobacterium Tuberculosis Induced Th1 Response But Failed to Enhance the Protective Efficacy of BCG in Mice. Indian J Tuberculosis (2021). doi: 10.1016/j.ijtb.2021.08.016 In Press.
11. Day CL, Tameris M, Mansoor N, van Rooyen M, de Kock M, Geldenhuys H, et al. Induction and Regulation of T-Cell Immunity by the Novel Tuberculosis Vaccine M72/AS01 in South African Adults. Am J Respir Crit Care Med (2013) 188(4):492–502. doi: 10.1164/rccm.201208-1385OC
12. Montoya J, Solon JA, Cunanan SR, Acosta L, Bollaerts A, Moris P, et al. A Randomized, Controlled Dose-Finding Phase II Study of the M72/AS01 Candidate Tuberculosis Vaccine in Healthy PPD-Positive Adults. J Clin Immunol (2013) 33(8):1360–75. doi: 10.1007/s10875-013-9949-3
13. Thacher EG, Cavassini M, Audran R, Thierry AC, Bollaerts A, Cohen J, et al. Safety and Immunogenicity of the M72/AS01 Candidate Tuberculosis Vaccine in HIV-Infected Adults on Combination Antiretroviral Therapy: A Phase I/II, Randomized Trial. Aids (2014) 28(12):1769–81. doi: 10.1097/qad.0000000000000343
14. Idoko OT, Owolabi OA, Owiafe PK, Moris P, Odutola A, Bollaerts A, et al. Safety and Immunogenicity of the M72/AS01 Candidate Tuberculosis Vaccine When Given as a Booster to BCG in Gambian Infants: An Open-Label Randomized Controlled Trial. Tuberculosis (Edinb) (2014) 94(6):564–78. doi: 10.1016/j.tube.2014.07.001
15. Van Der Meeren O, Hatherill M, Nduba V, Wilkinson RJ, Muyoyeta M, Van Brakel E, et al. Phase 2b Controlled Trial of M72/AS01(E) Vaccine to Prevent Tuberculosis. N Engl J Med (2018) 379(17):1621–34. doi: 10.1056/NEJMoa1803484
16. Tait DR, Hatherill M, van der Meeren O, Ginsberg AM, Van Brakel E, Salaun B, et al. Final Analysis of a Trial of M72/AS01(E) Vaccine to Prevent Tuberculosis. N Engl J Med (2019) 381(25):2429–39. doi: 10.1056/NEJMoa1909953
17. Ernst JD. Mechanisms of M. Tuberculosis Immune Evasion as Challenges to TB Vaccine Design. Cell Host Microbe (2018) 24(1):34–42. doi: 10.1016/j.chom.2018.06.004
18. Bellini C, Horváti K. Recent Advances in the Development of Protein- and Peptide-Based Subunit Vaccines Against Tuberculosis. Cells (2020) 9(12):2673. doi: 10.3390/cells9122673
19. Dong R, Chu Z, Yu F, Zha Y. Contriving Multi-Epitope Subunit of Vaccine for COVID-19: Immunoinformatics Approaches. Front Immunol (2020) 11:1784. doi: 10.3389/fimmu.2020.01784
20. Kapopoulou A, Lew JM, Cole ST. The MycoBrowser Portal: A Comprehensive and Manually Annotated Resource for Mycobacterial Genomes. Tuberculosis (Edinb) (2011) 91(1):8–13. doi: 10.1016/j.tube.2010.09.006
21. Consortium U. UniProt: A Worldwide Hub of Protein Knowledge. Nucleic Acids Res (2019) 47(D1):D506–15. doi: 10.1093/nar/gky1049
22. Sanches RCO, Tiwari S, Ferreira LCG, Oliveira FM, Lopes MD, Passos MJF, et al. Immunoinformatics Design of Multi-Epitope Peptide-Based Vaccine Against Schistosoma Mansoni Using Transmembrane Proteins as a Target. Front Immunol (2021) 12:621706. doi: 10.3389/fimmu.2021.621706
23. Sosa EJ, Burguener G, Lanzarotti E, Defelipe L, Radusky L, Pardo AM, et al. Target-Pathogen: A Structural Bioinformatic Approach to Prioritize Drug Targets in Pathogens. Nucleic Acids Res (2018) 46(D1):D413–d418. doi: 10.1093/nar/gkx1015
24. Dhanda SK, Vir P, Singla D, Gupta S, Kumar S, Raghava GP. A Web-Based Platform for Designing Vaccines Against Existing and Emerging Strains of Mycobacterium Tuberculosis. PloS One (2016) 11(4):e0153771. doi: 10.1371/journal.pone.0153771
25. Zia Q, Azhar A, Ahmad S, Afsar M, Hasan Z, Owais M, et al. PeMtb: A Database of MHC Antigenic Peptide of Mycobacterium Tuberculosis. Curr Pharm Biotechnol (2017) 18(8):648–52. doi: 10.2174/1389201018666170914150115
26. Chaudhuri R, Kulshreshtha D, Raghunandanan MV, Ramachandran S. Integrative Immunoinformatics for Mycobacterial Diseases in R Platform. Syst Synth Biol (2014) 8(1):27–39. doi: 10.1007/s11693-014-9135-9
27. Gonzalez-Galarza FF, McCabe A, Santos E, Jones J, Takeshita L, Ortega-Rivera ND, et al. Allele Frequency Net Database (AFND) 2020 Update: Gold-Standard Data Classification, Open Access Genotype Data and New Query Tools. Nucleic Acids Res (2020) 48(D1):D783–8. doi: 10.1093/nar/gkz1029
28. Bui HH, Sidney J, Dinh K, Southwood S, Newman MJ, Sette A. Predicting Population Coverage of T-Cell Epitope-Based Diagnostics and Vaccines. BMC Bioinf (2006) 7:153. doi: 10.1186/1471-2105-7-153
29. Wang P, Sidney J, Kim Y, Sette A, Lund O, Nielsen M, et al. Peptide Binding Predictions for HLA DR, DP and DQ Molecules. BMC Bioinf (2010) 11:568. doi: 10.1186/1471-2105-11-568
30. Reche PA, Glutting JP, Zhang H, Reinherz EL. Enhancement to the RANKPEP Resource for the Prediction of Peptide Binding to MHC Molecules Using Profiles. Immunogenetics (2004) 56(6):405–19. doi: 10.1007/s00251-004-0709-7
31. Xu Y, Luo C, Mamitsuka H, Zhu S. MetaMHCpan, A Meta Approach for Pan-Specific MHC Peptide Binding Prediction. Methods Mol Biol (2016) 1404:753–60. doi: 10.1007/978-1-4939-3389-1_49
32. Singh H, Raghava GP. ProPred: Prediction of HLA-DR Binding Sites. Bioinformatics (2001) 17(12):1236–7. doi: 10.1093/bioinformatics/17.12.1236
33. Mustafa AS, Shaban FA. ProPred Analysis and Experimental Evaluation of Promiscuous T-Cell Epitopes of Three Major Secreted Antigens of Mycobacterium Tuberculosis. Tuberculosis (Edinb) (2006) 86(2):115–24. doi: 10.1016/j.tube.2005.05.001
34. Reynisson B, Alvarez B, Paul S, Peters B, Nielsen M. NetMHCpan-4.1 and NetMHCIIpan-4.0: Improved Predictions of MHC Antigen Presentation by Concurrent Motif Deconvolution and Integration of MS MHC Eluted Ligand Data. Nucleic Acids Res (2020) 48(W1):W449–w454. doi: 10.1093/nar/gkaa379
35. Reynisson B, Barra C, Kaabinejadian S, Hildebrand WH, Peters B, Nielsen M. Improved Prediction of MHC II Antigen Presentation Through Integration and Motif Deconvolution of Mass Spectrometry MHC Eluted Ligand Data. J Proteome Res (2020) 19(6):2304–15. doi: 10.1021/acs.jproteome.9b00874
36. Jensen KK, Andreatta M, Marcatili P, Buus S, Greenbaum JA, Yan Z, et al. Improved Methods for Predicting Peptide Binding Affinity to MHC Class II Molecules. Immunology (2018) 154(3):394–406. doi: 10.1111/imm.12889
37. Andreatta M, Nielsen M. Gapped Sequence Alignment Using Artificial Neural Networks: Application to the MHC Class I System. Bioinformatics (2016) 32(4):511–7. doi: 10.1093/bioinformatics/btv639
38. Larsen MV, Lundegaard C, Lamberth K, Buus S, Lund O, Nielsen M. Large-Scale Validation of Methods for Cytotoxic T-Lymphocyte Epitope Prediction. BMC Bioinf (2007) 8:424. doi: 10.1186/1471-2105-8-424
39. Singh H, Raghava GP. ProPred1: Prediction of Promiscuous MHC Class-I Binding Sites. Bioinformatics (2003) 19(8):1009–14. doi: 10.1093/bioinformatics/btg108
40. Nielsen M, Lundegaard C, Worning P, Lauemøller SL, Lamberth K, Buus S, et al. Reliable Prediction of T-Cell Epitopes Using Neural Networks With Novel Sequence Representations. Protein Sci (2003) 12(5):1007–17. doi: 10.1110/ps.0239403
41. Guan P, Hattotuwagama CK, Doytchinova IA, Flower DR. MHCPred 2.0: An Updated Quantitative T-Cell Epitope Prediction Server. Appl Bioinf (2006) 5(1):55–61. doi: 10.2165/00822942-200605010-00008
42. Doytchinova IA, Guan P, Flower DR. EpiJen: A Server for Multistep T Cell Epitope Prediction. BMC Bioinf (2006) 7:131. doi: 10.1186/1471-2105-7-131
43. Srivastava VK, Kaushik S, Bhargava G, Jain A, Saxena J, Jyoti A. A Bioinformatics Approach for the Prediction of Immunogenic Properties and Structure of the SARS-COV-2 B.1.617.1 Variant Spike Protein. BioMed Res Int (2021) 2021:7251119. doi: 10.1155/2021/7251119
44. Saha S, Raghava GP. Prediction of Continuous B-Cell Epitopes in an Antigen Using Recurrent Neural Network. Proteins (2006) 65(1):40–8. doi: 10.1002/prot.21078
45. Khanna D, Rana PS. Improvement in Prediction of Antigenic Epitopes Using Stacked Generalisation: An Ensemble Approach. IET Syst Biol (2020) 14(1):1–7. doi: 10.1049/iet-syb.2018.5083
46. Chou PY, Fasman GD. Prediction of the Secondary Structure of Proteins From Their Amino Acid Sequence. Adv Enzymol Relat Areas Mol Biol (1978) 47:45–148. doi: 10.1002/9780470122921.ch2
47. Emini EA, Hughes JV, Perlow DS, Boger J. Induction of Hepatitis A Virus-Neutralizing Antibody by a Virus-Specific Synthetic Peptide. J Virol (1985) 55(3):836–9. doi: 10.1128/jvi.55.3.836-839.1985
48. PA K, GE S. Prediction of Chain Flexibility in Proteins - A Tool for the Selection of Peptide Antigens. Naturwissenschafren (1985) 72:212–3. doi: 10.1007/BF01195768
49. Parker JM, Guo D, Hodges RS. New Hydrophilicity Scale Derived From High-Performance Liquid Chromatography Peptide Retention Data: Correlation of Predicted Surface Residues With Antigenicity and X-Ray-Derived Accessible Sites. Biochemistry (1986) 25(19):5425–32. doi: 10.1021/bi00367a013
50. Kolaskar AS, Tongaonkar PC. A Semi-Empirical Method for Prediction of Antigenic Determinants on Protein Antigens. FEBS Lett (1990) 276(1-2):172–4. doi: 10.1016/0014-5793(90)80535-q
51. Larsen JE, Lund O, Nielsen M. Improved Method for Predicting Linear B-Cell Epitopes. Immunome Res (2006) 2:2. doi: 10.1186/1745-7580-2-2
52. Jespersen MC, Peters B, Nielsen M, Marcatili P. BepiPred-2.0: Improving Sequence-Based B-Cell Epitope Prediction Using Conformational Epitopes. Nucleic Acids Res (2017) 45(W1):W24–9. doi: 10.1093/nar/gkx346
53. Chen J, Liu H, Yang J, Chou KC. Prediction of Linear B-Cell Epitopes Using Amino Acid Pair Antigenicity Scale. Amino Acids (2007) 33(3):423–8. doi: 10.1007/s00726-006-0485-9
54. El-Manzalawy Y, Dobbs D, Honavar V. Predicting Linear B-Cell Epitopes Using String Kernels. J Mol Recognit (2008) 21(4):243–55. doi: 10.1002/jmr.893
55. Shen W, Cao Y, Cha L, Zhang X, Ying X, Zhang W, et al. Predicting Linear B-Cell Epitopes Using Amino Acid Anchoring Pair Composition. BioData Min (2015) 8:14. doi: 10.1186/s13040-015-0047-3
56. Yao B, Zhang L, Liang S, Zhang C. SVMTriP: A Method to Predict Antigenic Epitopes Using Support Vector Machine to Integrate Tri-Peptide Similarity and Propensity. PloS One (2012) 7(9):e45152. doi: 10.1371/journal.pone.0045152
57. Kringelum JV, Lundegaard C, Lund O, Nielsen M. Reliable B Cell Epitope Predictions: Impacts of Method Development and Improved Benchmarking. PloS Comput Biol (2012) 8(12):e1002829. doi: 10.1371/journal.pcbi.1002829
58. Sweredoski MJ, Baldi P. PEPITO: Improved Discontinuous B-Cell Epitope Prediction Using Multiple Distance Thresholds and Half Sphere Exposure. Bioinformatics (2008) 24(12):1459–60. doi: 10.1093/bioinformatics/btn199
59. Ponomarenko J, Bui HH, Li W, Fusseder N, Bourne PE, Sette A, et al. ElliPro: A New Structure-Based Tool for the Prediction of Antibody Epitopes. BMC Bioinf (2008) 9:514. doi: 10.1186/1471-2105-9-514
60. Zhou C, Chen Z, Zhang L, Yan D, Mao T, Tang K, et al. SEPPA 3.0-Enhanced Spatial Epitope Prediction Enabling Glycoprotein Antigens. Nucleic Acids Res (2019) 47(W1):W388–w394. doi: 10.1093/nar/gkz413
61. Rubinstein ND, Mayrose I, Martz E, Pupko T. Epitopia: A Web-Server for Predicting B-Cell Epitopes. BMC Bioinf (2009) 10:287. doi: 10.1186/1471-2105-10-287
62. Liang S, Zheng D, Zhang C, Zacharias M. Prediction of Antigenic Epitopes on Protein Surfaces by Consensus Scoring. BMC Bioinf (2009) 10:302. doi: 10.1186/1471-2105-10-302
63. Liang S, Zheng D, Standley DM, Yao B, Zacharias M, Zhang C. EPSVR and EPMeta: Prediction of Antigenic Epitopes Using Support Vector Regression and Multiple Server Results. BMC Bioinf (2010) 11:381. doi: 10.1186/1471-2105-11-381
64. Dhanda SK, Vir P, Raghava GP. Designing of Interferon-Gamma Inducing MHC Class-II Binders. Biol Direct (2013) 8:30. doi: 10.1186/1745-6150-8-30
65. Dhanda SK, Gupta S, Vir P, Raghava GP. Prediction of IL4 Inducing Peptides. Clin Dev Immunol (2013) 2013:263952. doi: 10.1155/2013/263952
66. Nagpal G, Usmani SS, Dhanda SK, Kaur H, Singh S, Sharma M, et al. Computer-Aided Designing of Immunosuppressive Peptides Based on IL-10 Inducing Potential. Sci Rep (2017) 7:42851. doi: 10.1038/srep42851
67. Calis JJ, Maybeno M, Greenbaum JA, Weiskopf D, De Silva AD, Sette A, et al. Properties of MHC Class I Presented Peptides That Enhance Immunogenicity. PloS Comput Biol (2013) 9(10):e1003266. doi: 10.1371/journal.pcbi.1003266
68. Dhanda SK, Karosiene E, Edwards L, Grifoni A, Paul S, Andreatta M, et al. Predicting HLA CD4 Immunogenicity in Human Populations. Front Immunol (2018) 9:1369. doi: 10.3389/fimmu.2018.01369
69. Chen B, Khodadoust MS, Olsson N, Wagar LE, Fast E, Liu CL, et al. Predicting HLA Class II Antigen Presentation Through Integrated Deep Learning. Nat Biotechnol (2019) 37(11):1332–43. doi: 10.1038/s41587-019-0280-2
70. Nilsson JB, Grifoni A, Tarke A, Sette A, Nielsen M. PopCover-2.0. Improved Selection of Peptide Sets With Optimal HLA and Pathogen Diversity Coverage. Front Immunol (2021) 12:728936. doi: 10.3389/fimmu.2021.728936
71. Saha S, Raghava GP. Searching and Mapping of B-Cell Epitopes in Bcipep Database. Methods Mol Biol (2007) 409:113–24. doi: 10.1007/978-1-60327-118-9_7
72. Doytchinova IA, Flower DR. VaxiJen: A Server for Prediction of Protective Antigens, Tumour Antigens and Subunit Vaccines. BMC Bioinf (2007) 8(1):4. doi: 10.1186/1471-2105-8-4
73. Magnan CN, Zeller M, Kayala MA, Vigil A, Randall A, Felgner PL, et al. High-Throughput Prediction of Protein Antigenicity Using Protein Microarray Data. Bioinformatics (2010) 26(23):2936–43. doi: 10.1093/bioinformatics/btq551
74. Anand R, Biswal S, Bhatt R, Tiwary BN. Computational Perspectives Revealed Prospective Vaccine Candidates From Five Structural Proteins of Novel SARS Corona Virus 2019 (SARS-CoV-2). PeerJ (2020) 8:e9855. doi: 10.7717/peerj.9855
75. Dimitrov I, Naneva L, Doytchinova I, Bangov I. AllergenFP: Allergenicity Prediction by Descriptor Fingerprints. Bioinformatics (2014) 30(6):846–51. doi: 10.1093/bioinformatics/btt619
76. Sharma N, Patiyal S, Dhall A, Pande A, Arora C, Raghava GPS. AlgPred 2.0: An Improved Method for Predicting Allergenic Proteins and Mapping of IgE Epitopes. Brief Bioinform (2021) 22(4):bbaa294. doi: 10.1093/bib/bbaa294
77. Fiers MW, Kleter GA, Nijland H, Peijnenburg AA, Nap JP, van Ham RC. Allermatch, a Webtool for the Prediction of Potential Allergenicity According to Current FAO/WHO Codex Alimentarius Guidelines. BMC Bioinf (2004) 5:133. doi: 10.1186/1471-2105-5-133
78. Gupta S, Kapoor P, Chaudhary K, Gautam A, Kumar R, Raghava GP. In Silico Approach for Predicting Toxicity of Peptides and Proteins. PloS One (2013) 8(9):e73957. doi: 10.1371/journal.pone.0073957
80. Dhanda SK, Vaughan K, Schulten V, Grifoni A, Weiskopf D, Sidney J, et al. Development of a Novel Clustering Tool for Linear Peptide Sequences. Immunology (2018) 155(3):331–45. doi: 10.1111/imm.12984
81. Manavalan B, Shin TH, Kim MO, Lee G. PIP-EL: A New Ensemble Learning Method for Improved Proinflammatory Peptide Predictions. Front Immunol (2018) 9:1783. doi: 10.3389/fimmu.2018.01783
82. Khatun MS, Hasan MM, Kurata H. PreAIP: Computational Prediction of Anti-Inflammatory Peptides by Integrating Multiple Complementary Features. Front Genet (2019) 10:129. doi: 10.3389/fgene.2019.00129
83. Grote A, Hiller K, Scheer M, Münch R, Nörtemann B, Hempel DC, et al. JCat: A Novel Tool to Adapt Codon Usage of a Target Gene to its Potential Expression Host. Nucleic Acids Res (2005) 33(Web Server issue):W526–531. doi: 10.1093/nar/gki376
84. Bibi S, Ullah I, Zhu B, Adnan M, Liaqat R, Kong WB, et al. In Silico Analysis of Epitope-Based Vaccine Candidate Against Tuberculosis Using Reverse Vaccinology. Sci Rep (2021) 11(1):1249. doi: 10.1038/s41598-020-80899-6
85. Hebditch M, Carballo-Amador MA, Charonis S, Curtis R, Warwicker J. Protein-Sol: A Web Tool for Predicting Protein Solubility From Sequence. Bioinformatics (2017) 33(19):3098–100. doi: 10.1093/bioinformatics/btx345
86. Liu IH, Lo YS, Yang JM. PAComplex: A Web Server to Infer Peptide Antigen Families and Binding Models From TCR-pMHC Complexes. Nucleic Acids Res (2011) 39(Web Server issue):W254–260. doi: 10.1093/nar/gkr434
87. van Zundert GCP, Rodrigues J, Trellet M, Schmitz C, Kastritis PL, Karaca E, et al. The HADDOCK2.2 Web Server: User-Friendly Integrative Modeling of Biomolecular Complexes. J Mol Biol (2016) 428(4):720–5. doi: 10.1016/j.jmb.2015.09.014
88. Kozakov D, Hall DR, Xia B, Porter KA, Padhorny D, Yueh C, et al. The ClusPro Web Server for Protein-Protein Docking. Nat Protoc (2017) 12(2):255–78. doi: 10.1038/nprot.2016.169
89. Jiménez-García B, Roel-Touris J, Romero-Durana M, Vidal M, Jiménez-González D, Fernández-Recio J. LightDock: A New Multi-Scale Approach to Protein-Protein Docking. Bioinformatics (2018) 34(1):49–55. doi: 10.1093/bioinformatics/btx555
90. Chen R, Li L, Weng Z. ZDOCK: An Initial-Stage Protein-Docking Algorithm. Proteins (2003) 52(1):80–7. doi: 10.1002/prot.10389
91. Kremer JR, Mastronarde DN, McIntosh JR. Computer Visualization of Three-Dimensional Image Data Using IMOD. J Struct Biol (1996) 116(1):71–6. doi: 10.1006/jsbi.1996.0013
92. Laskowski RA, Jabłońska J, Pravda L, Vařeková RS, Thornton JM. PDBsum: Structural Summaries of PDB Entries. Protein Sci (2018) 27(1):129–34. doi: 10.1002/pro.3289
93. Pollastri G, Przybylski D, Rost B, Baldi P. Improving the Prediction of Protein Secondary Structure in Three and Eight Classes Using Recurrent Neural Networks and Profiles. Proteins (2002) 47(2):228–35. doi: 10.1002/prot.10082
94. Sen TZ, Jernigan RL, Garnier J, Kloczkowski A. GOR V Server for Protein Secondary Structure Prediction. Bioinformatics (2005) 21(11):2787–8. doi: 10.1093/bioinformatics/bti408
95. Geourjon C, Deléage G. SOPMA: Significant Improvements in Protein Secondary Structure Prediction by Consensus Prediction From Multiple Alignments. Comput Appl Biosci (1995) 11(6):681–4. doi: 10.1093/bioinformatics/11.6.681
96. Ko J, Park H, Heo L, Seok C. GalaxyWEB Server for Protein Structure Prediction and Refinement. Nucleic Acids Res (2012) 40(Web Server issue):W294–297. doi: 10.1093/nar/gks493
97. Kuriata A, Gierut AM, Oleniecki T, Ciemny MP, Kolinski A, Kurcinski M, et al. CABS-Flex 2.0: A Web Server for Fast Simulations of Flexibility of Protein Structures. Nucleic Acids Res (2018) 46(W1):W338–w343. doi: 10.1093/nar/gky356
98. Cheng J, Randall AZ, Sweredoski MJ, Baldi P. SCRATCH: A Protein Structure and Structural Feature Prediction Server. Nucleic Acids Res (2005) 33(Web Server issue):W72–76. doi: 10.1093/nar/gki396
99. Kelley LA, Mezulis S, Yates CM, Wass MN, Sternberg MJ. The Phyre2 Web Portal for Protein Modeling, Prediction and Analysis. Nat Protoc (2015) 10(6):845–58. doi: 10.1038/nprot.2015.053
100. Waterhouse A, Bertoni M, Bienert S, Studer G, Tauriello G, Gumienny R, et al. SWISS-MODEL: Homology Modelling of Protein Structures and Complexes. Nucleic Acids Res (2018) 46(W1):W296–w303. doi: 10.1093/nar/gky427
101. Seedat F, James I, Loubser S, Waja Z, Mallal SA, Hoffmann C, et al. Human Leukocyte Antigen Associations With Protection Against Tuberculosis Infection and Disease in Human Immunodeficiency Virus-1 Infected Individuals, Despite Household Tuberculosis Exposure and Immune Suppression. Tuberculosis (Edinb) (2021) 126:102023. doi: 10.1016/j.tube.2020.102023
102. Malkova A, Starshinova A, Zinchenko Y, Basantsova N, Mayevskaya V, Yablonskiy P, et al. The Opposite Effect of Human Leukocyte Antigen Genotypes in Sarcoidosis and Tuberculosis: A Narrative Review of the Literature. ERJ Open Res (2020) 6(3):00155–2020. doi: 10.1183/23120541.00155-2020
103. Vita R, Mahajan S, Overton JA, Dhanda SK, Martini S, Cantrell JR, et al. The Immune Epitope Database (IEDB): 2018 Update. Nucleic Acids Res (2019) 47(D1):D339–d343. doi: 10.1093/nar/gky1006
104. Nielsen M, Lundegaard C, Lund O, Keşmir C. The Role of the Proteasome in Generating Cytotoxic T-Cell Epitopes: Insights Obtained From Improved Predictions of Proteasomal Cleavage. Immunogenetics (2005) 57(1-2):33–41. doi: 10.1007/s00251-005-0781-7
105. Tenzer S, Peters B, Bulik S, Schoor O, Lemmel C, Schatz MM, et al. Modeling the MHC Class I Pathway by Combining Predictions of Proteasomal Cleavage, TAP Transport and MHC Class I Binding. Cell Mol Life Sci (2005) 62(9):1025–37. doi: 10.1007/s00018-005-4528-2
106. Giguère S, Drouin A, Lacoste A, Marchand M, Corbeil J, Laviolette F. MHC-NP: Predicting Peptides Naturally Processed by the MHC. J Immunol Methods (2013)400–1:307–36. doi: 10.1016/j.jim.2013.10.003 30-36.
107. Chronister WD, Crinklaw A, Mahajan S, Vita R, Koşaloğlu-Yalçın Z, Yan Z, et al. TCRMatch: Predicting T-Cell Receptor Specificity Based on Sequence Similarity to Previously Characterized Receptors. Front Immunol (2021) 12:640725. doi: 10.3389/fimmu.2021.640725
108. Klausen MS, Anderson MV, Jespersen MC, Nielsen M, Marcatili P. LYRA, a Webserver for Lymphocyte Receptor Structural Modeling. Nucleic Acids Res (2015) 43(W1):W349–355. doi: 10.1093/nar/gkv535
109. Mahajan S, Yan Z, Jespersen MC, Jensen KK, Marcatili P, Nielsen M, et al. Benchmark Datasets of Immune Receptor-Epitope Structural Complexes. BMC Bioinf (2019) 20(1):490. doi: 10.1186/s12859-019-3109-6
110. Haste Andersen P, Nielsen M, Lund O. Prediction of Residues in Discontinuous B-Cell Epitopes Using Protein 3D Structures. Protein Sci (2006) 15(11):2558–67. doi: 10.1110/ps.062405906
111. Kuroda D, Shirai H, Jacobson MP, Nakamura H. Computer-Aided Antibody Design. Protein Eng Des Sel (2012) 25(10):507–21. doi: 10.1093/protein/gzs024
112. Bui HH, Sidney J, Li W, Fusseder N, Sette A. Development of an Epitope Conservancy Analysis Tool to Facilitate the Design of Epitope-Based Diagnostics and Vaccines. BMC Bioinf (2007) 8:361. doi: 10.1186/1471-2105-8-361
113. Paul S, Arlehamn CSL, Schulten V, Westernberg L, Sidney J, Peters B, et al. Experimental Validation of the RATE Tool for Inferring HLA Restrictions of T Cell Epitopes. BMC Immunol (2017) 18(Suppl 1):20. doi: 10.1186/s12865-017-0204-1
114. Dhanda SK, Vita R, Ha B, Grifoni A, Peters B, Sette A. ImmunomeBrowser: A Tool to Aggregate and Visualize Complex and Heterogeneous Epitopes in Reference Proteins. Bioinformatics (2018) 34(22):3931–3. doi: 10.1093/bioinformatics/bty463
115. Rai PK, Chodisetti SB, Maurya SK, Nadeem S, Zeng W, Janmeja AK, et al. A Lipidated Bi-Epitope Vaccine Comprising of MHC-I and MHC-II Binder Peptides Elicits Protective CD4 T Cell and CD8 T Cell Immunity Against Mycobacterium Tuberculosis. J Transl Med (2018) 16(1):279. doi: 10.1186/s12967-018-1653-x
116. Horváti K, Pályi B, Henczkó J, Balka G, Szabó E, Farkas V, et al. A Convenient Synthetic Method to Improve Immunogenicity of Mycobacterium Tuberculosis Related T-Cell Epitope Peptides. Vaccines (Basel) (2019) 7(3):101. doi: 10.3390/vaccines7030101
117. Wang P, Sidney J, Dow C, Mothé B, Sette A, Peters B. A Systematic Assessment of MHC Class II Peptide Binding Predictions and Evaluation of a Consensus Approach. PloS Comput Biol (2008) 4(4):e1000048. doi: 10.1371/journal.pcbi.1000048
118. Nielsen M, Lund O. NN-Align. An Artificial Neural Network-Based Alignment Algorithm for MHC Class II Peptide Binding Prediction. BMC Bioinf (2009) 10:296. doi: 10.1186/1471-2105-10-296
119. Nielsen M, Lundegaard C, Lund O. Prediction of MHC Class II Binding Affinity Using SMM-Align, a Novel Stabilization Matrix Alignment Method. BMC Bioinf (2007) 8:238. doi: 10.1186/1471-2105-8-238
120. Sturniolo T, Bono E, Ding J, Raddrizzani L, Tuereci O, Sahin U, et al. Generation of Tissue-Specific and Promiscuous HLA Ligand Databases Using DNA Microarrays and Virtual HLA Class II Matrices. Nat Biotechnol (1999) 17(6):555–61. doi: 10.1038/9858
121. Nielsen M, Lundegaard C, Blicher T, Peters B, Sette A, Justesen S, et al. Quantitative Predictions of Peptide Binding to Any HLA-DR Molecule of Known Sequence: NetMHCIIpan. PloS Comput Biol (2008) 4(7):e1000107. doi: 10.1371/journal.pcbi.1000107
122. Zhang L, Udaka K, Mamitsuka H, Zhu S. Toward More Accurate Pan-Specific MHC-Peptide Binding Prediction: A Review of Current Methods and Tools. Brief Bioinform (2012) 13(3):350–64. doi: 10.1093/bib/bbr060
123. Peters B, Sette A. Generating Quantitative Models Describing the Sequence Specificity of Biological Processes With the Stabilized Matrix Method. BMC Bioinf (2005) 6:132. doi: 10.1186/1471-2105-6-132
124. Sidney J, Assarsson E, Moore C, Ngo S, Pinilla C, Sette A, et al. Quantitative Peptide Binding Motifs for 19 Human and Mouse MHC Class I Molecules Derived Using Positional Scanning Combinatorial Peptide Libraries. Immunome Res (2008) 4:2. doi: 10.1186/1745-7580-4-2
125. Moutaftsi M, Peters B, Pasquetto V, Tscharke DC, Sidney J, Bui HH, et al. A Consensus Epitope Prediction Approach Identifies the Breadth of Murine T(CD8+)-Cell Responses to Vaccinia Virus. Nat Biotechnol (2006) 24(7):817–9. doi: 10.1038/nbt1215
126. Hoof I, Peters B, Sidney J, Pedersen LE, Sette A, Lund O, et al. NetMHCpan, a Method for MHC Class I Binding Prediction Beyond Humans. Immunogenetics (2009) 61(1):1–13. doi: 10.1007/s00251-008-0341-z
127. Karosiene E, Lundegaard C, Lund O, Nielsen M. NetMHCcons: A Consensus Method for the Major Histocompatibility Complex Class I Predictions. Immunogenetics (2012) 64(3):177–86. doi: 10.1007/s00251-011-0579-8
128. Zhang H, Lund O, Nielsen M. The PickPocket Method for Predicting Binding Specificities for Receptors Based on Receptor Pocket Similarities: Application to MHC-Peptide Binding. Bioinformatics (2009) 25(10):1293–9. doi: 10.1093/bioinformatics/btp137
129. Rasmussen M, Fenoy E, Harndahl M, Kristensen AB, Nielsen IK, Nielsen M, et al. Pan-Specific Prediction of Peptide-MHC Class I Complex Stability, a Correlate of T Cell Immunogenicity. J Immunol (2016) 197(4):1517–24. doi: 10.4049/jimmunol.1600582
130. Abebe F. Synergy Between Th1 and Th2 Responses During Mycobacterium Tuberculosis Infection: A Review of Current Understanding. Int Rev Immunol (2019) 38(4):172–9. doi: 10.1080/08830185.2019.1632842
131. Reddy Chichili VP, Kumar V, Sivaraman J. Linkers in the Structural Biology of Protein-Protein Interactions. Protein Sci (2013) 22(2):153–67. doi: 10.1002/pro.2206
132. Zhao HL, Yao XQ, Xue C, Wang Y, Xiong XH, Liu ZM. Increasing the Homogeneity, Stability and Activity of Human Serum Albumin and Interferon-Alpha2b Fusion Protein by Linker Engineering. Protein Expr Purif (2008) 61(1):73–7. doi: 10.1016/j.pep.2008.04.013
133. Amet N, Lee HF, Shen WC. Insertion of the Designed Helical Linker Led to Increased Expression of Tf-Based Fusion Proteins. Pharm Res (2009) 26(3):523–8. doi: 10.1007/s11095-008-9767-0
134. Bai Y, Shen WC. Improving the Oral Efficacy of Recombinant Granulocyte Colony-Stimulating Factor and Transferrin Fusion Protein by Spacer Optimization. Pharm Res (2006) 23(9):2116–21. doi: 10.1007/s11095-006-9059-5
135. Argos P. An Investigation of Oligopeptides Linking Domains in Protein Tertiary Structures and Possible Candidates for General Gene Fusion. J Mol Biol (1990) 211(4):943–58. doi: 10.1016/0022-2836(90)90085-z
136. Sabourin M, Tuzon CT, Fisher TS, Zakian VA. A Flexible Protein Linker Improves the Function of Epitope-Tagged Proteins in Saccharomyces Cerevisiae. Yeast (2007) 24(1):39–45. doi: 10.1002/yea.1431
137. de Bold MK, Sheffield WP, Martinuk A, Bhakta V, Eltringham-Smith L, de Bold AJ. Characterization of a Long-Acting Recombinant Human Serum Albumin-Atrial Natriuretic Factor (ANF) Expressed in Pichia Pastoris. Regul Pept (2012) 175(1-3):7–10. doi: 10.1016/j.regpep.2012.01.005
138. Waldo GS, Standish BM, Berendzen J, Terwilliger TC. Rapid Protein-Folding Assay Using Green Fluorescent Protein. Nat Biotechnol (1999) 17(7):691–5. doi: 10.1038/10904
139. Bird RE, Hardman KD, Jacobson JW, Johnson S, Kaufman BM, Lee SM, et al. Single-Chain Antigen-Binding Proteins. Science (1988) 242(4877):423–6. doi: 10.1126/science.3140379
140. Chen X, Zaro JL, Shen WC. Fusion Protein Linkers: Property, Design and Functionality. Adv Drug Delivery Rev (2013) 65(10):1357–69. doi: 10.1016/j.addr.2012.09.039
141. Maeda Y, Ueda H, Kazami J, Kawano G, Suzuki E, Nagamune T. Engineering of Functional Chimeric Protein G-Vargula Luciferase. Anal Biochem (1997) 249(2):147–52. doi: 10.1006/abio.1997.2181
142. Ulrich JT, Cieplak W, Paczkowski NJ, Taylor SM, Sanderson SD. Induction of an Antigen-Specific CTL Response by a Conformationally Biased Agonist of Human C5a Anaphylatoxin as a Molecular Adjuvant. J Immunol (2000) 164(10):5492–8. doi: 10.4049/jimmunol.164.10.5492
143. McCormick AL, Thomas MS, Heath AW. Immunization With an Interferon-Gamma-Gp120 Fusion Protein Induces Enhanced Immune Responses to Human Immunodeficiency Virus Gp120. J Infect Dis (2001) 184(11):1423–30. doi: 10.1086/324371
144. Medha P, Sharma S, Sharma M. Design of a Peptide-Based Vaccine From Late Stage Specific Immunogenic Cross-Reactive Antigens of PE/PPE Proteins of Mycobacterium Tuberculosis. Eur J Pharm Sci (2021) 168:106051. doi: 10.1016/j.ejps.2021.106051
145. Shiraz M, Lata S, Kumar P, Shankar UN, Akif M. Immunoinformatics Analysis of Antigenic Epitopes and Designing of a Multi-Epitope Peptide Vaccine From Putative Nitro-Reductases of Mycobacterium Tuberculosis DosR. Infect Genet Evol (2021) 94:105017. doi: 10.1016/j.meegid.2021.105017
146. Rai PK, Chodisetti SB, Zeng W, Nadeem S, Maurya SK, Pahari S, et al. A Lipidated Peptide of Mycobacterium Tuberculosis Resuscitates the Protective Efficacy of BCG Vaccine by Evoking Memory T Cell Immunity. J Transl Med (2017) 15(1):201. doi: 10.1186/s12967-017-1301-x
147. Dorosti H, Eslami M, Negahdaripour M, Ghoshoon MB, Gholami A, Heidari R, et al. Vaccinomics Approach for Developing Multi-Epitope Peptide Pneumococcal Vaccine. J Biomol Struct Dyn (2019) 37(13):3524–35. doi: 10.1080/07391102.2018.1519460
148. Rostamtabar M, Rahmani A, Baee M, Karkhah A, Prajapati VK, Ebrahimpour S, et al. Development a Multi-Epitope Driven Subunit Vaccine for Immune Response Reinforcement Against Serogroup B of Neisseria Meningitidis Using Comprehensive Immunoinformatics Approaches. Infect Genet Evol (2019) 75:103992. doi: 10.1016/j.meegid.2019.103992
149. Sharma R, Rajput VS, Jamal S, Grover A, Grover S. An Immunoinformatics Approach to Design a Multi-Epitope Vaccine Against Mycobacterium Tuberculosis Exploiting Secreted Exosome Proteins. Sci Rep (2021) 11(1):13836. doi: 10.1038/s41598-021-93266-w
150. Chatterjee N, Ojha R, Khatoon N, Prajapati VK. Scrutinizing Mycobacterium Tuberculosis Membrane and Secretory Proteins to Formulate Multiepitope Subunit Vaccine Against Pulmonary Tuberculosis by Utilizing Immunoinformatic Approaches. Int J Biol Macromol (2018) 118(Pt A):180–8. doi: 10.1016/j.ijbiomac.2018.06.080
151. Rahmani A, Baee M, Rostamtabar M, Karkhah A, Alizadeh S, Tourani M, et al. Development of a Conserved Chimeric Vaccine Based on Helper T-Cell and CTL Epitopes for Induction of Strong Immune Response Against Schistosoma Mansoni Using Immunoinformatics Approaches. Int J Biol Macromol (2019) 141:125–36. doi: 10.1016/j.ijbiomac.2019.08.259
152. Tahir Ul Qamar M, Ahmad S, Fatima I, Ahmad F, Shahid F, Naz A, et al. Designing Multi-Epitope Vaccine Against Staphylococcus Aureus by Employing Subtractive Proteomics, Reverse Vaccinology and Immuno-Informatics Approaches. Comput Biol Med (2021) 132:104389. doi: 10.1016/j.compbiomed.2021.104389
153. Ghosh P, Bhakta S, Bhattacharya M, Sharma AR, Sharma G, Lee SS, et al. A Novel Multi-Epitopic Peptide Vaccine Candidate Against Helicobacter Pylori: In-Silico Identification, Design, Cloning and Validation Through Molecular Dynamics. Int J Pept Res Ther (2021) 27:1–18. doi: 10.1007/s10989-020-10157-w
154. Yadav S, Prakash J, Shukla H, Das KC, Tripathi T, Dubey VK. Design of a Multi-Epitope Subunit Vaccine for Immune-Protection Against Leishmania Parasite. Pathog Glob Health (2020) 114(8):471–81. doi: 10.1080/20477724.2020.1842976
155. Shey RA, Ghogomu SM, Esoh KK, Nebangwa ND, Shintouo CM, Nongley NF, et al. In-Silico Design of a Multi-Epitope Vaccine Candidate Against Onchocerciasis and Related Filarial Diseases. Sci Rep (2019) 9(1):4409. doi: 10.1038/s41598-019-40833-x
156. Yazdani Z, Rafiei A, Irannejad H, Yazdani M, Valadan R. Designing a Novel Multiepitope Peptide Vaccine Against Melanoma Using Immunoinformatics Approach. J Biomol Struct Dyn (2020) 2020:1–13. doi: 10.1080/07391102.2020.1846625
157. Saadi M, Karkhah A, Nouri HR. Development of a Multi-Epitope Peptide Vaccine Inducing Robust T Cell Responses Against Brucellosis Using Immunoinformatics Based Approaches. Infect Genet Evol (2017) 51:227–34. doi: 10.1016/j.meegid.2017.04.009
158. Nezafat N, Karimi Z, Eslami M, Mohkam M, Zandian S, Ghasemi Y. Designing an Efficient Multi-Epitope Peptide Vaccine Against Vibrio Cholerae via Combined Immunoinformatics and Protein Interaction Based Approaches. Comput Biol Chem (2016) 62:82–95. doi: 10.1016/j.compbiolchem.2016.04.006
159. Meza B, Ascencio F, Sierra-Beltrán AP, Torres J, Angulo C. A Novel Design of a Multi-Antigenic, Multistage and Multi-Epitope Vaccine Against Helicobacter Pylori: An in Silico Approach. Infect Genet Evol (2017) 49:309–17. doi: 10.1016/j.meegid.2017.02.007
160. Albutti A. An Integrated Computational Framework to Design a Multi-Epitopes Vaccine Against Mycobacterium Tuberculosis. Sci Rep (2021) 11(1):21929. doi: 10.1038/s41598-021-01283-6
161. Mitra D, Pandey J, Jain A, Swaroop S. In Silico Design of Multi-Epitope-Based Peptide Vaccine Against SARS-CoV-2 Using its Spike Protein. J Biomol Struct Dyn (2021) 2020:1–14. doi: 10.1080/07391102.2020.1869092
162. Kalita P, Padhi AK, Zhang KYJ, Tripathi T. Design of a Peptide-Based Subunit Vaccine Against Novel Coronavirus SARS-CoV-2. Microb Pathog (2020) 145:104236. doi: 10.1016/j.micpath.2020.104236
163. Abdulla F, Adhikari UK, Uddin MK. Exploring T & B-Cell Epitopes and Designing Multi-Epitope Subunit Vaccine Targeting Integration Step of HIV-1 Lifecycle Using Immunoinformatics Approach. Microb Pathog (2019) 137:103791. doi: 10.1016/j.micpath.2019.103791
164. Shahid F, Ashfaq UA, Javaid A, Khalid H. Immunoinformatics Guided Rational Design of a Next Generation Multi Epitope Based Peptide (MEBP) Vaccine by Exploring Zika Virus Proteome. Infect Genet Evol (2020) 80:104199. doi: 10.1016/j.meegid.2020.104199
165. Pavitrakar DV, Atre NM, Tripathy AS, Shil P. Design of a Multi-Epitope Peptide Vaccine Candidate Against Chandipura Virus: An Immuno-Informatics Study. J Biomol Struct Dyn (2020) 2020:1–12. doi: 10.1080/07391102.2020.1816493
166. Saha R, Ghosh P, Burra V. Designing a Next Generation Multi-Epitope Based Peptide Vaccine Candidate Against SARS-CoV-2 Using Computational Approaches. 3 Biotech (2021) 11(2):47. doi: 10.1007/s13205-020-02574-x
167. Jakhar R, Kaushik S, Gakhar SK. 3CL Hydrolase-Based Multiepitope Peptide Vaccine Against SARS-CoV-2 Using Immunoinformatics. J Med Virol (2020) 92(10):2114–23. doi: 10.1002/jmv.25993
168. Schurz H, Daya M, Möller M, Hoal EG, Salie M. TLR1, 2, 4, 6 and 9 Variants Associated With Tuberculosis Susceptibility: A Systematic Review and Meta-Analysis. PloS One (2015) 10(10):e0139711. doi: 10.1371/journal.pone.0139711
169. Gopalakrishnan A, Dietzold J, Salgame P. Vaccine-Mediated Immunity to Experimental Mycobacterium Tuberculosis is Not Impaired in the Absence of Toll-Like Receptor 9. Cell Immunol (2016) 302:11–8. doi: 10.1016/j.cellimm.2015.12.009
170. Wani BA, Shehjar F, Shah S, Koul A, Yusuf A, Farooq M, et al. Role of Genetic Variants of Vitamin D Receptor, Toll-Like Receptor 2 and Toll-Like Receptor 4 in Extrapulmonary Tuberculosis. Microb Pathog (2021) 156:104911. doi: 10.1016/j.micpath.2021.104911
171. Hemmi H, Takeuchi O, Kawai T, Kaisho T, Sato S, Sanjo H, et al. A Toll-Like Receptor Recognizes Bacterial DNA. Nature (2000) 408(6813):740–5. doi: 10.1038/35047123
172. Krug A, Rothenfusser S, Hornung V, Jahrsdörfer B, Blackwell S, Ballas ZK, et al. Identification of CpG Oligonucleotide Sequences With High Induction of IFN-Alpha/Beta in Plasmacytoid Dendritic Cells. Eur J Immunol (2001) 31(7):2154–63. doi: 10.1002/1521-4141(200107)31:7<2154::aid-immu2154>3.0.co;2-u
173. Steinhagen F, Kinjo T, Bode C, Klinman DM. TLR-Based Immune Adjuvants. Vaccine (2011) 29(17):3341–55. doi: 10.1016/j.vaccine.2010.08.002
174. Vollmer J, Weeratna R, Payette P, Jurk M, Schetter C, Laucht M, et al. Characterization of Three CpG Oligodeoxynucleotide Classes With Distinct Immunostimulatory Activities. Eur J Immunol (2004) 34(1):251–62. doi: 10.1002/eji.200324032
175. Akira S, Uematsu S, Takeuchi O. Pathogen Recognition and Innate Immunity. Cell (2006) 124(4):783–801. doi: 10.1016/j.cell.2006.02.015
176. Peacock T, Chain B. Information-Driven Docking for TCR-pMHC Complex Prediction. Front Immunol (2021) 12:686127. doi: 10.3389/fimmu.2021.686127
177. Akıl M, Aykur M, Karakavuk M, Can H, Döşkaya M. Construction of a Multiepitope Vaccine Candidate Against Fasciola Hepatica: An in Silico Design Using Various Immunogenic Excretory/Secretory Antigens. Expert Rev Vaccines (2021) 2021:1–14. doi: 10.1080/14760584.2022.1996233
178. Malonis RJ, Lai JR, Vergnolle O. Peptide-Based Vaccines: Current Progress and Future Challenges. Chem Rev (2020) 120(6):3210–29. doi: 10.1021/acs.chemrev.9b00472
179. Andersen P, Scriba TJ. Moving Tuberculosis Vaccines From Theory to Practice. Nat Rev Immunol (2019) 19(9):550–62. doi: 10.1038/s41577-019-0174-z
180. Etlinger HM, Heimer EP, Trzeciak A, Felix AM, Gillessen D. Assessment in Mice of a Synthetic Peptide-Based Vaccine Against the Sporozoite Stage of the Human Malaria Parasite, P. Falciparum. Immunology (1988) 64(3):551–8.
181. Weichold FF, Mueller S, Kortsik C, Hitzler WE, Wulf MJ, Hone DM, et al. Impact of MHC Class I Alleles on the M. Tuberculosis Antigen-Specific CD8+ T-Cell Response in Patients With Pulmonary Tuberculosis. Genes Immun (2007) 8(4):334–43. doi: 10.1038/sj.gene.6364392
182. Gaseitsiwe S, Valentini D, Mahdavifar S, Magalhaes I, Hoft DF, Zerweck J, et al. Pattern Recognition in Pulmonary Tuberculosis Defined by High Content Peptide Microarray Chip Analysis Representing 61 Proteins From M. Tuberculosis. PloS One (2008) 3(12):e3840. doi: 10.1371/journal.pone.0003840
183. Axelsson-Robertson R, Weichold F, Sizemore D, Wulf M, Skeiky YA, Sadoff J, et al. Extensive Major Histocompatibility Complex Class I Binding Promiscuity for Mycobacterium Tuberculosis TB10.4 Peptides and Immune Dominance of Human Leucocyte Antigen (HLA)-B*0702 and HLA-B*0801 Alleles in TB10.4 CD8 T-Cell Responses. Immunology (2010) 129(4):496–505. doi: 10.1111/j.1365-2567.2009.03201.x
184. Gaseitsiwe S, Valentini D, Mahdavifar S, Reilly M, Ehrnst A, Maeurer M. Peptide Microarray-Based Identification of Mycobacterium Tuberculosis Epitope Binding to HLA-DRB1*0101, DRB1*1501, and DRB1*0401. Clin Vaccine Immunol (2010) 17(1):168–75. doi: 10.1128/cvi.00208-09
185. Axelsson-Robertson R, Ahmed RK, Weichold FF, Ehlers MM, Kock MM, Sizemore D, et al. Human Leukocyte Antigens A*3001 and A*3002 Show Distinct Peptide-Binding Patterns of the Mycobacterium Tuberculosis Protein TB10.4: Consequences for Immune Recognition. Clin Vaccine Immunol (2011) 18(1):125–34. doi: 10.1128/cvi.00302-10
186. Valentini D, Rao M, Ferrara G, Perkins M, Dodoo E, Zumla A, et al. Immune Recognition Surface Construction of Mycobacterium Tuberculosis Epitope-Specific Antibody Responses in Tuberculosis Patients Identified by Peptide Microarrays. Int J Infect Dis (2017) 56:155–66. doi: 10.1016/j.ijid.2017.01.015
187. Höhn H, Kortsik C, Nilges K, Necker A, Freitag K, Tully G, et al. Human Leucocyte Antigen-A2 Restricted and Mycobacterium Tuberculosis 19-kDa Antigen-Specific CD8+ T-Cell Responses are Oligoclonal and Exhibit a T-Cell Cytotoxic Type 2 Response Cytokine-Secretion Pattern. Immunology (2001) 104(3):278–88. doi: 10.1046/j.1365-2567.2001.01307.x
188. Höhn H, Kortsik C, Tully G, Nilges K, Necker A, Freitag K, et al. Longitudinal Analysis of Mycobacterium Tuberculosis 19-kDa Antigen-Specific T Cells in Patients With Pulmonary Tuberculosis: Association With Disease Activity and Cross-Reactivity to a Peptide From HIVenv Gp120. Eur J Immunol (2003) 33(6):1613–23. doi: 10.1002/eji.200323480
189. Tully G, Kortsik C, Höhn H, Zehbe I, Hitzler WE, Neukirch C, et al. Highly Focused T Cell Responses in Latent Human Pulmonary Mycobacterium Tuberculosis Infection. J Immunol (2005) 174(4):2174–84. doi: 10.4049/jimmunol.174.4.2174
190. Oftung F, Mustafa AS, Shinnick TM, Houghten RA, Kvalheim G, Degre M, et al. Epitopes of the Mycobacterium Tuberculosis 65-Kilodalton Protein Antigen as Recognized by Human T Cells. J Immunol (1988) 141(8):2749–54.
191. Mustafa AS, Oftung F, Amoudy HA, Madi NM, Abal AT, Shaban F, et al. Multiple Epitopes From the Mycobacterium Tuberculosis ESAT-6 Antigen are Recognized by Antigen-Specific Human T Cell Lines. Clin Infect Dis (2000) 30 Suppl 3:S201–205. doi: 10.1086/313862
192. Mustafa AS, Shaban FA, Abal AT, Al-Attiyah R, Wiker HG, Lundin KE, et al. Identification and HLA Restriction of Naturally Derived Th1-Cell Epitopes From the Secreted Mycobacterium Tuberculosis Antigen 85B Recognized by Antigen-Specific Human CD4(+) T-Cell Lines. Infect Immun (2000) 68(7):3933–40. doi: 10.1128/iai.68.7.3933-3940.2000
193. Mustafa AS, Shaban FA, Al-Attiyah R, Abal AT, El-Shamy AM, Andersen P, et al. Human Th1 Cell Lines Recognize the Mycobacterium Tuberculosis ESAT-6 Antigen and its Peptides in Association With Frequently Expressed HLA Class II Molecules. Scand J Immunol (2003) 57(2):125–34. doi: 10.1046/j.1365-3083.2003.01204.x
194. Mustafa AS, Al-Attiyah R, Hanif SN, Shaban FA. Efficient Testing of Large Pools of Mycobacterium Tuberculosis RD1 Peptides and Identification of Major Antigens and Immunodominant Peptides Recognized by Human Th1 Cells. Clin Vaccine Immunol (2008) 15(6):916–24. doi: 10.1128/cvi.00056-08
195. Mustafa AS, Shaban F. Mapping of Th1-Cell Epitope Regions of Mycobacterium Tuberculosis Protein MPT64 (Rv1980c) Using Synthetic Peptides and T-Cell Lines From M. Tuberculosis-Infected Healthy Humans. Med Princ Pract (2010) 19(2):122–8. doi: 10.1159/000273073
196. Mustafa AS. Characterization of a Cross-Reactive, Immunodominant and HLA-Promiscuous Epitope of Mycobacterium Tuberculosis-Specific Major Antigenic Protein PPE68. PloS One (2014) 9(8):e103679. doi: 10.1371/journal.pone.0103679
197. McMurry J, Sbai H, Gennaro ML, Carter EJ, Martin W, De Groot AS. Analyzing Mycobacterium Tuberculosis Proteomes for Candidate Vaccine Epitopes. Tuberculosis (Edinb) (2005) 85(1-2):95–105. doi: 10.1016/j.tube.2004.09.005
198. De Groot AS, McMurry J, Marcon L, Franco J, Rivera D, Kutzler M, et al. Developing an Epitope-Driven Tuberculosis (TB) Vaccine. Vaccine (2005) 23(17-18):2121–31. doi: 10.1016/j.vaccine.2005.01.059
199. Geluk A, van Meijgaarden KE, de Vries RR, Sette A, Ottenhoff TH. A DR17-Restricted T Cell Epitope From a Secreted Mycobacterium Tuberculosis Antigen Only Binds to DR17 Molecules at Neutral pH. Eur J Immunol (1997) 27(4):842–7. doi: 10.1002/eji.1830270406
200. Geluk A, Taneja V, van Meijgaarden KE, Zanelli E, Abou-Zeid C, Thole JE, et al. Identification of HLA Class II-Restricted Determinants of Mycobacterium Tuberculosis-Derived Proteins by Using HLA-Transgenic, Class II-Deficient Mice. Proc Natl Acad Sci USA (1998) 95(18):10797–802. doi: 10.1073/pnas.95.18.10797
201. Commandeur S, van den Eeden SJ, Dijkman K, Clark SO, van Meijgaarden KE, Wilson L, et al. The In Vivo Expressed Mycobacterium Tuberculosis (IVE-TB) Antigen Rv2034 Induces CD4⁺ T-Cells That Protect Against Pulmonary Infection in HLA-DR Transgenic Mice and Guinea Pigs. Vaccine (2014) 32(29):3580–8. doi: 10.1016/j.vaccine.2014.05.005
202. Geluk A, van den Eeden SJ, van Meijgaarden KE, Dijkman K, Franken KL, Ottenhoff TH. A Multistage-Polyepitope Vaccine Protects Against Mycobacterium Tuberculosis Infection in HLA-DR3 Transgenic Mice. Vaccine (2012) 30(52):7513–21. doi: 10.1016/j.vaccine.2012.10.045
203. Coppola M, van den Eeden SJ, Wilson L, Franken KL, Ottenhoff TH, Geluk A. Synthetic Long Peptide Derived From Mycobacterium Tuberculosis Latency Antigen Rv1733c Protects Against Tuberculosis. Clin Vaccine Immunol (2015) 22(9):1060–9. doi: 10.1128/CVI.00271-15
204. da Fonseca DP, Joosten D, van der Zee R, Jue DL, Singh M, Vordermeier HM, et al. Identification of New Cytotoxic T-Cell Epitopes on the 38-Kilodalton Lipoglycoprotein of Mycobacterium Tuberculosis by Using Lipopeptides. Infect Immun (1998) 66(7):3190–7. doi: 10.1128/iai.66.7.3190-3197.1998
205. da Fonseca DP, Frerichs J, Singh M, Snippe H, Verheul AF. Induction of Antibody and T-Cell Responses by Immunization With ISCOMS Containing the 38-Kilodalton Protein of Mycobacterium Tuberculosis. Vaccine (2000) 19(1):122–31. doi: 10.1016/s0264-410x(00)00102-x
206. Fonseca DP, Benaissa-Trouw B, van Engelen M, Kraaijeveld CA, Snippe H, Verheul AF. Induction of Cell-Mediated Immunity Against Mycobacterium Tuberculosis Using DNA Vaccines Encoding Cytotoxic and Helper T-Cell Epitopes of the 38-Kilodalton Protein. Infect Immun (2001) 69(8):4839–45. doi: 10.1128/iai.69.8.4839-4845.2001
207. Harboe M, Christensen A, Ahmad S, Ulvund G, Harkness RE, Mustafa AS, et al. Cross-Reaction Between Mammalian Cell Entry (Mce) Proteins of Mycobacterium Tuberculosis. Scand J Immunol (2002) 56(6):580–7. doi: 10.1046/j.1365-3083.2002.01172.x
208. Das AK, Mitra D, Harboe M, Nandi B, Harkness RE, Das D, et al. Predicted Molecular Structure of the Mammalian Cell Entry Protein Mce1A of Mycobacterium Tuberculosis. Biochem Biophys Res Commun (2003) 302(3):442–7. doi: 10.1016/s0006-291x(03)00116-5
209. Harboe M, Das AK, Mitra D, Ulvund G, Ahmad S, Harkness RE, et al. Immunodominant B-Cell Epitope in the Mce1A Mammalian Cell Entry Protein of Mycobacterium Tuberculosis Cross-Reacting With Glutathione S-Transferase. Scand J Immunol (2004) 59(2):190–7. doi: 10.1111/j.0300-9475.2004.01383.x
210. Gowthaman U, Singh V, Zeng W, Jain S, Siddiqui KF, Chodisetti SB, et al. Promiscuous Peptide of 16 kDa Antigen Linked to Pam2Cys Protects Against Mycobacterium Tuberculosis by Evoking Enduring Memory T-Cell Response. J Infect Dis (2011) 204(9):1328–38. doi: 10.1093/infdis/jir548
211. Maurya SK, Aqdas M, Das DK, Singh S, Nadeem S, Kaur G, et al. A Multiple T Cell Epitope Comprising DNA Vaccine Boosts the Protective Efficacy of Bacillus Calmette-Guérin (BCG) Against Mycobacterium Tuberculosis. BMC Infect Dis (2020) 20(1):677. doi: 10.1186/s12879-020-05372-1
212. Harris DP, Vordermeier HM, Roman E, Lathigra R, Brett SJ, Moreno C, et al. Murine T Cell-Stimulatory Peptides From the 19-kDa Antigen of Mycobacterium Tuberculosis. Epitope-Restricted Homology With the 28-kDa Protein of Mycobacterium Leprae. J Immunol (1991) 147(8):2706–12.
213. Vordermeier HM, Harris DP, Friscia G, Román E, Surcel HM, Moreno C, et al. T Cell Repertoire in Tuberculosis: Selective Anergy to an Immunodominant Epitope of the 38-kDa Antigen in Patients With Active Disease. Eur J Immunol (1992) 22(10):2631–7. doi: 10.1002/eji.1830221024
214. Vordermeier HM, Harris DP, Mehrotra PK, Roman E, Elsaghier A, Moreno C, et al. M. Tuberculosis-Complex Specific T-Cell Stimulation and DTH Reactions Induced With a Peptide From the 38-kDa Protein. Scand J Immunol (1992) 35(6):711–8. doi: 10.1111/j.1365-3083.1992.tb02979.x
215. Launois P, DeLeys R, Niang MN, Drowart A, Andrien M, Dierckx P, et al. T-Cell-Epitope Mapping of the Major Secreted Mycobacterial Antigen Ag85A in Tuberculosis and Leprosy. Infect Immun (1994) 62(9):3679–87. doi: 10.1128/iai.62.9.3679-3687.1994
216. Denis O, Tanghe A, Palfliet K, Jurion F, van den Berg TP, Vanonckelen A, et al. Vaccination With Plasmid DNA Encoding Mycobacterial Antigen 85A Stimulates a CD4+ and CD8+ T-Cell Epitopic Repertoire Broader Than That Stimulated by Mycobacterium Tuberculosis H37Rv Infection. Infect Immun (1998) 66(4):1527–33. doi: 10.1128/iai.66.4.1527-1533.1998
217. Romano M, Denis O, D’Souza S, Wang XM, Ottenhoff TH, Brulet JM, et al. Induction of In Vivo Functional Db-Restricted Cytolytic T Cell Activity Against a Putative Phosphate Transport Receptor of Mycobacterium Tuberculosis. J Immunol (2004) 172(11):6913–21. doi: 10.4049/jimmunol.172.11.6913
218. Sánchez-Barinas CD, Ocampo M, Vanegas M, Castañeda-Ramirez JJ, Patarroyo MA, Patarroyo ME. Mycobacterium Tuberculosis H37Rv LpqG Protein Peptides Can Inhibit Mycobacterial Entry Through Specific Interactions. Molecules (2018) 23(3):526. doi: 10.3390/molecules23030526
219. Sánchez-Barinas CD, Ocampo M, Tabares L, Bermúdez M, Patarroyo MA, Patarroyo ME. Specific Binding Peptides From Rv3632: A Strategy for Blocking Mycobacterium Tuberculosis Entry to Target Cells? BioMed Res Int (2019) 2019:8680935. doi: 10.1155/2019/8680935
220. Ocampo M, Curtidor H, Vanegas M, Patarroyo MA, Patarroyo ME. Specific Interaction Between Mycobacterium Tuberculosis Lipoprotein-Derived Peptides and Target Cells Inhibits Mycobacterial Entry In Vitro. Chem Biol Drug Des (2014) 84(6):626–41. doi: 10.1111/cbdd.12365
221. Brandt L, Oettinger T, Holm A, Andersen AB, Andersen P. Key Epitopes on the ESAT-6 Antigen Recognized in Mice During the Recall of Protective Immunity to Mycobacterium Tuberculosis. J Immunol (1996) 157(8):3527–33. doi: 10.1016/S0165-2478(97)86823-X
222. Olsen AW, Hansen PR, Holm A, Andersen P. Efficient Protection Against Mycobacterium Tuberculosis by Vaccination With a Single Subdominant Epitope From the ESAT-6 Antigen. Eur J Immunol (2000) 30(6):1724–32. doi: 10.1002/1521-4141(200006)30:6<1724::aid-immu1724>3.0.co;2-a
223. Aagaard CS, Hoang TT, Vingsbo-Lundberg C, Dietrich J, Andersen P. Quality and Vaccine Efficacy of CD4+ T Cell Responses Directed to Dominant and Subdominant Epitopes in ESAT-6 From Mycobacterium Tuberculosis. J Immunol (2009) 183(4):2659–68. doi: 10.4049/jimmunol.0900947
224. Chaitra MG, Hariharaputran S, Chandra NR, Shaila MS, Nayak R. Defining Putative T Cell Epitopes From PE and PPE Families of Proteins of Mycobacterium Tuberculosis With Vaccine Potential. Vaccine (2005) 23(10):1265–72. doi: 10.1016/j.vaccine.2004.08.046
225. Chaitra MG, Shaila MS, Nayak R. Evaluation of T-Cell Responses to Peptides With MHC Class I-Binding Motifs Derived From PE_PGRS 33 Protein of Mycobacterium Tuberculosis. J Med Microbiol (2007) 56(Pt 4):466–74. doi: 10.1099/jmm.0.46928-0
226. Chaitra MG, Shaila MS, Chandra NR, Nayak R. HLA-A*0201-Restricted Cytotoxic T-Cell Epitopes in Three PE/PPE Family Proteins of Mycobacterium Tuberculosis. Scand J Immunol (2008) 67(4):411–7. doi: 10.1111/j.1365-3083.2008.02078.x
227. Chaitra MG, Shaila MS, Nayak R. Characterization of T-Cell Immunogenicity of Two PE/PPE Proteins of Mycobacterium Tuberculosis. J Med Microbiol (2008) 57(Pt 9):1079–86. doi: 10.1099/jmm.0.47565-0
228. Commandeur S, van Meijgaarden KE, Lin MY, Franken KL, Friggen AH, Drijfhout JW, et al. Identification of Human T-Cell Responses to Mycobacterium Tuberculosis Resuscitation-Promoting Factors in Long-Term Latently Infected Individuals. Clin Vaccine Immunol (2011) 18(4):676–83. doi: 10.1128/cvi.00492-10
229. Tang ST, van Meijgaarden KE, Caccamo N, Guggino G, Klein MR, van Weeren P, et al. Genome-Based in Silico Identification of New Mycobacterium Tuberculosis Antigens Activating Polyfunctional CD8+ T Cells in Human Tuberculosis. J Immunol (2011) 186(2):1068–80. doi: 10.4049/jimmunol.1002212
230. Commandeur S, Coppola M, Dijkman K, Friggen AH, van Meijgaarden KE, van den Eeden SJ, et al. Clonal Analysis of the T-Cell Response to In Vivo Expressed Mycobacterium Tuberculosis Protein Rv2034, Using a CD154 Expression Based T-Cell Cloning Method. PloS One (2014) 9(6):e99203. doi: 10.1371/journal.pone.0099203
231. Zhu YH, Gao YF, Chen F, Liu W, Zhai MX, Zhai WJ, et al. Identification of Novel T Cell Epitopes From Efflux Pumps of Mycobacterium Tuberculosis. Immunol Lett (2011) 140(1-2):68–73. doi: 10.1016/j.imlet.2011.06.009
232. Chen F, Zhai MX, Zhu YH, Qi YM, Zhai WJ, Gao YF. In Vitro and In Vivo Identification of a Novel Cytotoxic T Lymphocyte Epitope From Rv3425 of Mycobacterium Tuberculosis. Microbiol Immunol (2012) 56(8):548–53. doi: 10.1111/j.1348-0421.2012.00470.x
233. Li D, Dou Z, Wu Y, Qi Y, Chen J, Gao Y. Identification of Novel Cytotoxic T Lymphocyte Epitopes of Drug- Resistance Related Protein InhA From Mycobacterium Tuberculosis. Protein Pept Lett (2020) 27(11):1141–50. doi: 10.2174/0929866527666200505215346
234. Mollenkopf HJ, Grode L, Mattow J, Stein M, Mann P, Knapp B, et al. Application of Mycobacterial Proteomics to Vaccine Design: Improved Protection by Mycobacterium Bovis BCG Prime-Rv3407 DNA Boost Vaccination Against Tuberculosis. Infect Immun (2004) 72(11):6471–9. doi: 10.1128/iai.72.11.6471-6479.2004
235. Wang QM, Sun SH, Hu ZL, Zhou FJ, Yin M, Xiao CJ, et al. Epitope DNA Vaccines Against Tuberculosis: Spacers and Ubiquitin Modulates Cellular Immune Responses Elicited by Epitope DNA Vaccine. Scand J Immunol (2004) 60(3):219–25. doi: 10.1111/j.0300-9475.2004.01442.x
236. Garnica O, Das K, Devasundaram S, Dhandayuthapani S. Enhanced Delivery of Mycobacterium Tuberculosis Antigens to Antigen Presenting Cells Using RVG Peptide. Tuberculosis (Edinb) (2019) 116s:S34–s41. doi: 10.1016/j.tube.2019.04.009
237. Fan X, Li X, Wan K, Zhao X, Deng Y, Chen Z, et al. Construction and Immunogenicity of a T Cell Epitope-Based Subunit Vaccine Candidate Against Mycobacterium Tuberculosis. Vaccine (2021) 39(47):6860–5. doi: 10.1016/j.vaccine.2021.10.034
238. Jiang Q, Zhang J, Chen X, Xia M, Lu Y, Qiu W, et al. A Novel Recombinant DNA Vaccine Encoding Mycobacterium Tuberculosis ESAT-6 and FL Protects Against Mycobacterium Tuberculosis Challenge in Mice. J BioMed Res (2013) 27(5):406–20. doi: 10.7555/jbr.27.20120114
239. Kumar S, Bhaskar A, Patnaik G, Sharma C, Singh DK, Kaushik SR, et al. Intranasal Immunization With Peptide-Based Immunogenic Complex Enhances BCG Vaccine Efficacy in a Murine Model of Tuberculosis. JCI Insight (2021) 6(4):e145228. doi: 10.1172/jci.insight.145228
240. Chesson CB, Huante M, Nusbaum RJ, Walker AG, Clover TM, Chinnaswamy J, et al. Nanoscale Peptide Self-Assemblies Boost BCG-Primed Cellular Immunity Against Mycobacterium Tuberculosis. Sci Rep (2018) 8(1):12519. doi: 10.1038/s41598-018-31089-y
241. Sugawara I, Udagawa T, Taniyama T. Protective Efficacy of Recombinant (Ag85A) BCG Tokyo With Ag85A Peptide Boosting Against Mycobacterium Tuberculosis-Infected Guinea Pigs in Comparison With That of DNA Vaccine Encoding Ag85A. Tuberculosis (Edinb) (2007) 87(2):94–101. doi: 10.1016/j.tube.2006.05.001
242. Shi C, Zhang H, Zhang T, Wang X, Bai B, Zhao Y, et al. New Alternative Vaccine Component Against Mycobacterium Tuberculosis–Heat Shock Protein 16.3 or its T-Cell Epitope. Scand J Immunol (2009) 70(5):465–74. doi: 10.1111/j.1365-3083.2009.02325.x
243. Wu M, Li M, Yue Y, Xu W. DNA Vaccine With Discontinuous T-Cell Epitope Insertions Into HSP65 Scaffold as a Potential Means to Improve Immunogenicity of Multi-Epitope Mycobacterium Tuberculosis Vaccine. Microbiol Immunol (2016) 60(9):634–45. doi: 10.1111/1348-0421.12410
244. Choi SY, Kwon KW, Kim H, Choi HH, Shin SJ. Vaccine Potential of ESAT-6 Protein Fused With Consensus CD4(+) T-Cell Epitopes of PE/PPE Proteins Against Highly Pathogenic Mycobacterium Tuberculosis Strain HN878. Biochem Biophys Res Commun (2018) 503(4):2195–201. doi: 10.1016/j.bbrc.2018.06.017
245. Luo Y, Wang B, Hu L, Yu H, Da Z, Jiang W, et al. Fusion Protein Ag85B-MPT64(190-198)-Mtb8.4 has Higher Immunogenicity Than Ag85B With Capacity to Boost BCG-Primed Immunity Against Mycobacterium Tuberculosis in Mice. Vaccine (2009) 27(44):6179–85. doi: 10.1016/j.vaccine.2009.08.018
246. Li Q, Yu H, Zhang Y, Wang B, Jiang W, Da Z, et al. Immunogenicity and Protective Efficacy of a Fusion Protein Vaccine Consisting of Antigen Ag85B and HspX Against Mycobacterium Tuberculosis Infection in Mice. Scand J Immunol (2011) 73(6):568–76. doi: 10.1111/j.1365-3083.2011.02531.x
247. Liu X, Peng J, Hu L, Luo Y, Niu H, Bai C, et al. A Multistage Mycobacterium Tuberculosis Subunit Vaccine LT70 Including Latency Antigen Rv2626c Induces Long-Term Protection Against Tuberculosis. Hum Vaccin Immunother (2016) 12(7):1670–7. doi: 10.1080/21645515.2016.1141159
248. Qian J, Chen R, Wang H, Zhang X. Role of the PE/PPE Family in Host-Pathogen Interactions and Prospects for Anti-Tuberculosis Vaccine and Diagnostic Tool Design. Front Cell Infect Microbiol (2020) 10:594288. doi: 10.3389/fcimb.2020.594288
249. Sørensen AL, Nagai S, Houen G, Andersen P, Andersen AB. Purification and Characterization of a Low-Molecular-Mass T-Cell Antigen Secreted by Mycobacterium Tuberculosis. Infect Immun (1995) 63(5):1710–7. doi: 10.1128/iai.63.5.1710-1717.1995
250. Skjøt RL, Oettinger T, Rosenkrands I, Ravn P, Brock I, Jacobsen S, et al. Comparative Evaluation of Low-Molecular-Mass Proteins From Mycobacterium Tuberculosis Identifies Members of the ESAT-6 Family as Immunodominant T-Cell Antigens. Infect Immun (2000) 68(1):214–20. doi: 10.1128/iai.68.1.214-220.2000
251. Hoang T, Aagaard C, Dietrich J, Cassidy JP, Dolganov G, Schoolnik GK, et al. ESAT-6 (EsxA) and TB10.4 (EsxH) Based Vaccines for Pre- and Post-Exposure Tuberculosis Vaccination. PloS One (2013) 8(12):e80579. doi: 10.1371/journal.pone.0080579
252. Yang E, Lu Y, Xu Y, Liang Q, Wang C, Wang H, et al. Recombinant BCG Coexpressing Ag85B, ESAT-6 and Rv3620c Elicits Specific Th1 Immune Responses in C57BL/6 Mice. Microb Pathog (2014) 69-70:53–9. doi: 10.1016/j.micpath.2014.03.011
253. Li W, Li M, Deng G, Zhao L, Liu X, Wang Y. Prime-Boost Vaccination With Bacillus Calmette Guerin and a Recombinant Adenovirus Co-Expressing CFP10, ESAT6, Ag85A and Ag85B of Mycobacterium Tuberculosis Induces Robust Antigen-Specific Immune Responses in Mice. Mol Med Rep (2015) 12(2):3073–80. doi: 10.3892/mmr.2015.3770
254. Liang Y, Bai X, Zhang J, Song J, Yang Y, Yu Q, et al. Ag85A/ESAT-6 Chimeric DNA Vaccine Induces an Adverse Response in Tuberculosis-Infected Mice. Mol Med Rep (2016) 14(2):1146–52. doi: 10.3892/mmr.2016.5364
255. Yu W, Hu T. Conjugation With an Inulin-Chitosan Adjuvant Markedly Improves the Immunogenicity of Mycobacterium Tuberculosis CFP10-TB10.4 Fusion Protein. Mol Pharm (2016) 13(11):3626–35. doi: 10.1021/acs.molpharmaceut.6b00138
256. Wang C, Lu J, Du W, Wang G, Li X, Shen X, et al. Ag85b/ESAT6-CFP10 Adjuvanted With Aluminum/Poly-IC Effectively Protects Guinea Pigs From Latent Mycobacterium Tuberculosis Infection. Vaccine (2019) 37(32):4477–84. doi: 10.1016/j.vaccine.2019.06.078
257. Karbalaei Zadeh Babaki M, Soleimanpour S, Rezaee SA. Antigen 85 Complex as a Powerful Mycobacterium Tuberculosis Immunogene: Biology, Immune-Pathogenicity, Applications in Diagnosis, and Vaccine Design. Microb Pathog (2017) 112:20–9. doi: 10.1016/j.micpath.2017.08.040
258. Adewumi AT, Elrashedy A, Soremekun OS, Ajadi MB, Soliman MES. Weak Spots Inhibition in the Mycobacterium Tuberculosis Antigen 85C Target for Antitubercular Drug Design Through Selective Irreversible Covalent Inhibitor-SER124. J Biomol Struct Dyn (2020) 2020:1–21. doi: 10.1080/07391102.2020.1844061
259. Hoft DF, Blazevic A, Abate G, Hanekom WA, Kaplan G, Soler JH, et al. A New Recombinant Bacille Calmette-Guerin Vaccine Safely Induces Significantly Enhanced Tuberculosis-Specific Immunity in Human Volunteers. J Infect Dis (2008) 198(10):1491–501. doi: 10.1086/592450
260. Hoft DF, Blazevic A, Selimovic A, Turan A, Tennant J, Abate G, et al. Safety and Immunogenicity of the Recombinant BCG Vaccine AERAS-422 in Healthy BCG-Naive Adults: A Randomized, Active-Controlled, First-In-Human Phase 1 Trial. EBioMedicine (2016) 7(C):278–86. doi: 10.1016/j.ebiom.2016.04.010
261. Hawkridge T, Scriba TJ, Gelderbloem S, Smit E, Tameris M, Moyo S, et al. Safety and Immunogenicity of a New Tuberculosis Vaccine, MVA85A, in Healthy Adults in South Africa. J Infect Dis (2008) 198(4):544–52. doi: 10.1086/590185
262. Kaufmann SH, Weiner J, von Reyn CF. Novel Approaches to Tuberculosis Vaccine Development. Int J Infect Dis (2017) 56(C):263–7. doi: 10.1016/j.ijid.2016.10.018
263. Stylianou E, Griffiths KL, Poyntz HC, Harrington-Kandt R, Dicks MD, Stockdale L, et al. Improvement of BCG Protective Efficacy With a Novel Chimpanzee Adenovirus and a Modified Vaccinia Ankara Virus Both Expressing Ag85A. Vaccine (2015) 33(48):6800–8. doi: 10.1016/j.vaccine.2015.10.017
264. Lu JB, Chen BW, Wang GZ, Fu LL, Shen XB, Su C, et al. Recombinant Tuberculosis Vaccine AEC/BC02 Induces Antigen-Specific Cellular Responses in Mice and Protects Guinea Pigs in a Model of Latent Infection. J Microbiol Immunol Infect (2015) 48(6):597–603. doi: 10.1016/j.jmii.2014.03.005
265. Viljoen A, Alsteens D, Dufrêne Y. Mechanical Forces Between Mycobacterial Antigen 85 Complex and Fibronectin. Cells (2020) 9(3):716. doi: 10.3390/cells9030716
266. Yuan Y, Crane DD, Simpson RM, Zhu YQ, Hickey MJ, Sherman DR, et al. The 16-kDa Alpha-Crystallin (Acr) Protein of Mycobacterium Tuberculosis Is Required for Growth in Macrophages. Proc Natl Acad Sci USA (1998) 95(16):9578–83. doi: 10.1073/pnas.95.16.9578
267. Hu Y, Movahedzadeh F, Stoker NG, Coates AR. Deletion of the Mycobacterium Tuberculosis Alpha-Crystallin-Like hspX Gene Causes Increased Bacterial Growth In Vivo. Infect Immun (2006) 74(2):861–8. doi: 10.1128/iai.74.2.861-868.2006
268. Wieczorek AE, Troudt JL, Knabenbauer P, Taylor J, Pavlicek RL, Karls R, et al. HspX Vaccination and Role in Virulence in the Guinea Pig Model of Tuberculosis. Pathog Dis (2014) 71(3):315–25. doi: 10.1111/2049-632x.12147
269. Siddiqui KF, Amir M, Gurram RK, Khan N, Arora A, Rajagopal K, et al. Latency-Associated Protein Acr1 Impairs Dendritic Cell Maturation and Functionality: A Possible Mechanism of Immune Evasion by Mycobacterium Tuberculosis. J Infect Dis (2014) 209(9):1436–45. doi: 10.1093/infdis/jit595
270. Woodworth JS, Behar SM. Mycobacterium Tuberculosis-Specific CD8+ T Cells and Their Role in Immunity. Crit Rev Immunol (2006) 26(4):317–52. doi: 10.1615/critrevimmunol.v26.i4.30
271. Vordermeier HM, Hewinson RG, Wilkinson RJ, Wilkinson KA, Gideon HP, Young DB, et al. Conserved Immune Recognition Hierarchy of Mycobacterial PE/PPE Proteins During Infection in Natural Hosts. PloS One (2012) 7(8):e40890. doi: 10.1371/journal.pone.0040890
272. Geisbrecht BV, Nikonenko B, Samala R, Nakamura R, Nacy CA, Sacksteder KA. Design and Optimization of a Recombinant System for Large-Scale Production of the MPT64 Antigen From Mycobacterium Tuberculosis. Protein Expr Purif (2006) 46(1):64–72. doi: 10.1016/j.pep.2005.08.011
273. Goyal B, Kumar K, Gupta D, Agarwal R, Latawa R, Sheikh JA, et al. Utility of B-Cell Epitopes Based Peptides of RD1 and RD2 Antigens for Immunodiagnosis of Pulmonary Tuberculosis. Diagn Microbiol Infect Dis (2014) 78(4):391–7. doi: 10.1016/j.diagmicrobio.2013.12.018
274. Zvi A, Ariel N, Fulkerson J, Sadoff JC, Shafferman A. Whole Genome Identification of Mycobacterium Tuberculosis Vaccine Candidates by Comprehensive Data Mining and Bioinformatic Analyses. BMC Med Genomics (2008) 1:18. doi: 10.1186/1755-8794-1-18
275. Black GF, Thiel BA, Ota MO, Parida SK, Adegbola R, Boom WH, et al. Immunogenicity of Novel DosR Regulon-Encoded Candidate Antigens of Mycobacterium Tuberculosis in Three High-Burden Populations in Africa. Clin Vaccine Immunol (2009) 16(8):1203–12. doi: 10.1128/cvi.00111-09
276. Leyten EM, Lin MY, Franken KL, Friggen AH, Prins C, van Meijgaarden KE, et al. Human T-Cell Responses to 25 Novel Antigens Encoded by Genes of the Dormancy Regulon of Mycobacterium Tuberculosis. Microbes Infect (2006) 8(8):2052–60. doi: 10.1016/j.micinf.2006.03.018
277. Bivas-Benita M, Lin MY, Bal SM, van Meijgaarden KE, Franken KL, Friggen AH, et al. Pulmonary Delivery of DNA Encoding Mycobacterium Tuberculosis Latency Antigen Rv1733c Associated to PLGA-PEI Nanoparticles Enhances T Cell Responses in a DNA Prime/Protein Boost Vaccination Regimen in Mice. Vaccine (2009) 27(30):4010–7. doi: 10.1016/j.vaccine.2009.04.033
278. Qian W, Huang Z, Chen Y, Yang J, Wang L, Wu K, et al. Elicitation of Integrated Immunity in Mice by a Novel Pneumococcal Polysaccharide Vaccine Conjugated With HBV Surface Antigen. Sci Rep (2020) 10(1):6470. doi: 10.1038/s41598-020-62185-7
279. An SJ, Woo JS, Chae MH, Kothari S, Carbis R. Preparation and Testing of a Haemophilus Influenzae Type B/Hepatitis B Surface Antigen Conjugate Vaccine. Vaccine (2015) 33(13):1614–9. doi: 10.1016/j.vaccine.2015.01.061
280. Polonskaya Z, Deng S, Sarkar A, Kain L, Comellas-Aragones M, McKay CS, et al. T Cells Control the Generation of Nanomolar-Affinity Anti-Glycan Antibodies. J Clin Invest (2017) 127(4):1491–504. doi: 10.1172/JCI91192
281. Li X, Pan C, Sun P, Peng Z, Feng E, Wu J, et al. Orthogonal Modular Biosynthesis of Nanoscale Conjugate Vaccines for Vaccination Against Infection. Nano Res (2021) 2021:1–9. doi: 10.1007/s12274-021-3713-4
282. Manayani DJ, Thomas D, Dryden KA, Reddy V, Siladi ME, Marlett JM, et al. A Viral Nanoparticle With Dual Function as an Anthrax Antitoxin and Vaccine. PloS Pathog (2007) 3(10):1422–31. doi: 10.1371/journal.ppat.0030142
283. Tao P, Mahalingam M, Zhu J, Moayeri M, Sha J, Lawrence WS, et al. A Bacteriophage T4 Nanoparticle-Based Dual Vaccine Against Anthrax and Plague. mBio (2018) 9(5):e01926–18. doi: 10.1128/mBio.01926-18
284. Pan C, Wu J, Qing S, Zhang X, Zhang L, Yue H, et al. Biosynthesis of Self-Assembled Proteinaceous Nanoparticles for Vaccination. Adv Mater (2020) 32(42):e2002940. doi: 10.1002/adma.202002940
285. Peng Z, Wu J, Wang K, Li X, Sun P, Zhang L, et al. Production of a Promising Biosynthetic Self-Assembled Nanoconjugate Vaccine Against Klebsiella Pneumoniae Serotype O2 in a General Escherichia Coli Host. Adv Sci (Weinh) (2021) 8(14):e2100549. doi: 10.1002/advs.202100549
286. McConnell MJ, Hanna PC, Imperiale MJ. Adenovirus-Based Prime-Boost Immunization for Rapid Vaccination Against Anthrax. Mol Ther (2007) 15(1):203–10. doi: 10.1038/sj.mt.6300034
287. Langley WA, Bradley KC, Li ZN, Smith ME, Schnell MJ, Steinhauer DA. Induction of Neutralizing Antibody Responses to Anthrax Protective Antigen by Using Influenza Virus Vectors: Implications for Disparate Immune System Priming Pathways. J Virol (2010) 84(16):8300–7. doi: 10.1128/JVI.00183-10
288. Wang HC, An HJ, Yu YZ, Xu Q. Potentiation of Anthrax Vaccines Using Protective Antigen-Expressing Viral Replicon Vectors. Immunol Lett (2015) 163(2):206–13. doi: 10.1016/j.imlet.2014.07.012
289. Onate AA, Donoso G, Moraga-Cid G, Folch H, Cespedes S, Andrews E. An RNA Vaccine Based on Recombinant Semliki Forest Virus Particles Expressing the Cu,Zn Superoxide Dismutase Protein of Brucella Abortus Induces Protective Immunity in BALB/c Mice. Infect Immun (2005) 73(6):3294–300. doi: 10.1128/IAI.73.6.3294-3300.2005
290. Cabrera A, Saez D, Cespedes S, Andrews E, Onate A. Vaccination With Recombinant Semliki Forest Virus Particles Expressing Translation Initiation Factor 3 of Brucella Abortus Induces Protective Immunity in BALB/c Mice. Immunobiology (2009) 214(6):467–74. doi: 10.1016/j.imbio.2008.11.016
291. Tabynov K, Sansyzbay A, Kydyrbayev Z, Yespembetov B, Ryskeldinova S, Zinina N, et al. Influenza Viral Vectors Expressing the Brucella OMP16 or L7/L12 Proteins as Vaccines Against B. Abortus Infection. Virol J (2014) 11:69. doi: 10.1186/1743-422X-11-69
292. Bugybayeva D, Kydyrbayev Z, Zinina N, Assanzhanova N, Yespembetov B, Kozhamkulov Y, et al. A New Candidate Vaccine for Human Brucellosis Based on Influenza Viral Vectors: A Preliminary Investigation for the Development of an Immunization Schedule in a Guinea Pig Model. Infect Dis Poverty (2021) 10(1):13. doi: 10.1186/s40249-021-00801-y
293. Brennan FR, Bellaby T, Helliwell SM, Jones TD, Kamstrup S, Dalsgaard K, et al. Chimeric Plant Virus Particles Administered Nasally or Orally Induce Systemic and Mucosal Immune Responses in Mice. J Virol (1999) 73(2):930–8. doi: 10.1128/JVI.73.2.930-938.1999
294. Oliveira ML, Areas AP, Campos IB, Monedero V, Perez-Martinez G, Miyaji EN, et al. Induction of Systemic and Mucosal Immune Response and Decrease in Streptococcus Pneumoniae Colonization by Nasal Inoculation of Mice With Recombinant Lactic Acid Bacteria Expressing Pneumococcal Surface Antigen A. Microbes Infect (2006) 8(4):1016–24. doi: 10.1016/j.micinf.2005.10.020
295. Hanniffy SB, Carter AT, Hitchin E, Wells JM. Mucosal Delivery of a Pneumococcal Vaccine Using Lactococcus Lactis Affords Protection Against Respiratory Infection. J Infect Dis (2007) 195(2):185–93. doi: 10.1086/509807
296. Campos IB, Darrieux M, Ferreira DM, Miyaji EN, Silva DA, Areas AP, et al. Nasal Immunization of Mice With Lactobacillus Casei Expressing the Pneumococcal Surface Protein A: Induction of Antibodies, Complement Deposition and Partial Protection Against Streptococcus Pneumoniae Challenge. Microbes Infect (2008) 10(5):481–8. doi: 10.1016/j.micinf.2008.01.007
297. Medina M, Villena J, Vintini E, Hebert EM, Raya R, Alvarez S. Nasal Immunization With Lactococcus Lactis Expressing the Pneumococcal Protective Protein A Induces Protective Immunity in Mice. Infect Immun (2008) 76(6):2696–705. doi: 10.1128/IAI.00119-08
298. Ferreira DM, Darrieux M, Silva DA, Leite LC, Ferreira JM Jr, Ho PL, et al. Characterization of Protective Mucosal and Systemic Immune Responses Elicited by Pneumococcal Surface Protein PspA and PspC Nasal Vaccines Against a Respiratory Pneumococcal Challenge in Mice. Clin Vaccine Immunol (2009) 16(5):636–45. doi: 10.1128/CVI.00395-08
299. Vintini E, Villena J, Alvarez S, Medina M. Administration of a Probiotic Associated With Nasal Vaccination With Inactivated Lactococcus Lactis-PppA Induces Effective Protection Against Pneumoccocal Infection in Young Mice. Clin Exp Immunol (2010) 159(3):351–62. doi: 10.1111/j.1365-2249.2009.04056.x
300. Hernani Mde L, Ferreira PC, Ferreira DM, Miyaji EN, Ho PL, Oliveira ML. Nasal Immunization of Mice With Lactobacillus Casei Expressing the Pneumococcal Surface Protein C Primes the Immune System and Decreases Pneumococcal Nasopharyngeal Colonization in Mice. FEMS Immunol Med Microbiol (2011) 62(3):263–72. doi: 10.1111/j.1574-695X.2011.00809.x
301. Chu H, Kang S, Ha S, Cho K, Park SM, Han KH, et al. Lactobacillus Acidophilus Expressing Recombinant K99 Adhesive Fimbriae has an Inhibitory Effect on Adhesion of Enterotoxigenic Escherichia Coli. Microbiol Immunol (2005) 49(11):941–8. doi: 10.1111/j.1348-0421.2005.tb03687.x
302. Wu CM, Chung TC. Mice Protected by Oral Immunization With Lactobacillus Reuteri Secreting Fusion Protein of Escherichia Coli Enterotoxin Subunit Protein. FEMS Immunol Med Microbiol (2007) 50(3):354–65. doi: 10.1111/j.1574-695X.2007.00255.x
303. Liu JK, Hou XL, Wei CH, Yu LY, He XJ, Wang GH, et al. Induction of Immune Responses in Mice After Oral Immunization With Recombinant Lactobacillus Casei Strains Expressing Enterotoxigenic Escherichia Coli F41 Fimbrial Protein. Appl Environ Microbiol (2009) 75(13):4491–7. doi: 10.1128/AEM.02672-08
304. Wei CH, Liu JK, Hou XL, Yu LY, Lee JS, Kim CJ. Immunogenicity and Protective Efficacy of Orally or Intranasally Administered Recombinant Lactobacillus Casei Expressing ETEC K99. Vaccine (2010) 28(24):4113–8. doi: 10.1016/j.vaccine.2009.05.088
305. Ferreira PC, da Silva JB, Piazza RM, Eckmann L, Ho PL, Oliveira ML. Immunization of Mice With Lactobacillus Casei Expressing a Beta-Intimin Fragment Reduces Intestinal Colonization by Citrobacter Rodentium. Clin Vaccine Immunol (2011) 18(11):1823–33. doi: 10.1128/CVI.05262-11
306. Wen LJ, Hou XL, Wang GH, Yu LY, Wei XM, Liu JK, et al. Immunization With Recombinant Lactobacillus Casei Strains Producing K99, K88 Fimbrial Protein Protects Mice Against Enterotoxigenic Escherichia Coli. Vaccine (2012) 30(22):3339–49. doi: 10.1016/j.vaccine.2011.08.036
307. Liu JK, Wei CH, Hou XL, Yu LY. Passive Protection of Mice Pups Through Oral or Intranasal Immunization of Dams With Recombinant Lactobacillus Casei Vaccine Against ETEC F41. Res Vet Sci (2014) 96(2):283–7. doi: 10.1016/j.rvsc.2014.01.010
308. Ashrafi F, Fallah Mehrabadi J, Siadat SD, Aghasadeghi MR. Expression and Purification of the Uropathogenic Escherichia Coli PapG Protein and its Surface Absorption on Lactobacillus Reuteri: Implications for Surface Display System Vaccines. Jundishapur J Microbiol (2015) 8(9):e25595. doi: 10.5812/jjm.25595
309. Lin R, Zhang Y, Long B, Li Y, Wu Y, Duan S, et al. Oral Immunization With Recombinant Lactobacillus Acidophilus Expressing espA-Tir-M Confers Protection Against Enterohemorrhagic Escherichia Coli O157:H7 Challenge in Mice. Front Microbiol (2017) 8:417. doi: 10.3389/fmicb.2017.00417
310. Yang G, Jiang Y, Tong P, Li C, Yang W, Hu J, et al. Alleviation of Enterotoxigenic Escherichia Coli Challenge by Recombinant Lactobacillus Plantarum Expressing a FaeG- and DC-Targeting Peptide Fusion Protein. Benef Microbes (2017) 8(3):379–91. doi: 10.3920/BM2016.0116
311. Yu M, Qi R, Chen C, Yin J, Ma S, Shi W, et al. Immunogenicity of Recombinant Lactobacillus Casei-Expressing F4 (K88) Fimbrial Adhesin FaeG in Conjunction With a Heat-Labile Enterotoxin A (LTAK63) and Heat-Labile Enterotoxin B (LTB) of Enterotoxigenic Escherichia Coli as an Oral Adjuvant in Mice. J Appl Microbiol (2017) 122(2):506–15. doi: 10.1111/jam.13352
312. Okuno T, Kashige N, Satho T, Irie K, Hiramatsu Y, Sharmin T, et al. Expression and Secretion of Cholera Toxin B Subunit in Lactobacilli. Biol Pharm Bull (2013) 36(6):952–8. doi: 10.1248/bpb.b12-01021
313. Kajikawa A, Satoh E, Leer RJ, Yamamoto S, Igimi S. Intragastric Immunization With Recombinant Lactobacillus Casei Expressing Flagellar Antigen Confers Antibody-Independent Protective Immunity Against Salmonella Enterica Serovar Enteritidis. Vaccine (2007) 25(18):3599–605. doi: 10.1016/j.vaccine.2007.01.055
314. Kajikawa A, Igimi S. Innate and Acquired Immune Responses Induced by Recombinant Lactobacillus Casei Displaying Flagellin-Fusion Antigen on the Cell-Surface. Vaccine (2010) 28(19):3409–15. doi: 10.1016/j.vaccine.2010.02.077
315. Zegers ND, Kluter E, van der Stap H, van Dura E, van Dalen P, Shaw M, et al. Expression of the Protective Antigen of Bacillus Anthracis by Lactobacillus Casei: Towards the Development of an Oral Vaccine Against Anthrax. J Appl Microbiol (1999) 87(2):309–14. doi: 10.1046/j.1365-2672.1999.00900.x
316. Mohamadzadeh M, Duong T, Sandwick SJ, Hoover T, Klaenhammer TR. Dendritic Cell Targeting of Bacillus Anthracis Protective Antigen Expressed by Lactobacillus Acidophilus Protects Mice From Lethal Challenge. Proc Natl Acad Sci USA (2009) 106(11):4331–6. doi: 10.1073/pnas.0900029106
317. Mohamadzadeh M, Durmaz E, Zadeh M, Pakanati KC, Gramarossa M, Cohran V, et al. Targeted Expression of Anthrax Protective Antigen by Lactobacillus Gasseri as an Anthrax Vaccine. Future Microbiol (2010) 5(8):1289–96. doi: 10.2217/fmb.10.78
318. Kathania M, Zadeh M, Lightfoot YL, Roman RM, Sahay B, Abbott JR, et al. Colonic Immune Stimulation by Targeted Oral Vaccine. PloS One (2013) 8(1):e55143. doi: 10.1371/journal.pone.0055143
319. O’Flaherty S, Klaenhammer TR. Multivalent Chromosomal Expression of the Clostridium Botulinum Serotype A Neurotoxin Heavy-Chain Antigen and the Bacillus Anthracis Protective Antigen in Lactobacillus Acidophilus. Appl Environ Microbiol (2016) 82(20):6091–101. doi: 10.1128/AEM.01533-16
320. Pontes DS, Dorella FA, Ribeiro LA, Miyoshi A, Le Loir Y, Gruss A, et al. Induction of Partial Protection in Mice After Oral Administration of Lactococcus Lactis Producing Brucella Abortus L7/L12 Antigen. J Drug Target (2003) 11(8-10):489–93. doi: 10.1080/10611860410001670035
321. Saez D, Fernandez P, Rivera A, Andrews E, Onate A. Oral Immunization of Mice With Recombinant Lactococcus Lactis Expressing Cu,Zn Superoxide Dismutase of Brucella Abortus Triggers Protective Immunity. Vaccine (2012) 30(7):1283–90. doi: 10.1016/j.vaccine.2011.12.088
322. Shirdast H, Ebrahimzadeh F, Taromchi AH, Mortazavi Y, Esmaeilzadeh A, Sekhavati MH, et al. Recombinant Lactococcus Lactis Displaying Omp31 Antigen of Brucella Melitensis Can Induce an Immunogenic Response in BALB/c Mice. Probiotics Antimicrob Proteins (2021) 13(1):80–9. doi: 10.1007/s12602-020-09684-1
323. Veloso TR, Mancini S, Giddey M, Vouillamoz J, Que YA, Moreillon P, et al. Vaccination Against Staphylococcus Aureus Experimental Endocarditis Using Recombinant Lactococcus Lactis Expressing ClfA or FnbpA. Vaccine (2015) 33(30):3512–7. doi: 10.1016/j.vaccine.2015.05.060
324. Clow F, Peterken K, Pearson V, Proft T, Radcliff FJ. PilVax, a Novel Lactococcus Lactis-Based Mucosal Vaccine Platform, Stimulates Systemic and Mucosal Immune Responses to Staphylococcus Aureus. Immunol Cell Biol (2020) 98(5):369–81. doi: 10.1111/imcb.12325
325. Corthesy B, Boris S, Isler P, Grangette C, Mercenier A. Oral Immunization of Mice With Lactic Acid Bacteria Producing Helicobacter Pylori Urease B Subunit Partially Protects Against Challenge With Helicobacter Felis. J Infect Dis (2005) 192(8):1441–9. doi: 10.1086/444425
326. Hongying F, Xianbo W, Fang Y, Yang B, Beiguo L. Oral Immunization With Recombinant Lactobacillus Acidophilus Expressing the Adhesin Hp0410 of Helicobacter Pylori Induces Mucosal and Systemic Immune Responses. Clin Vaccine Immunol (2014) 21(2):126–32. doi: 10.1128/CVI.00434-13
327. del Rio B, Fuente JL, Neves V, Dattwyler R, Seegers JF, Gomes-Solecki M. Platform Technology to Deliver Prophylactic Molecules Orally: An Example Using the Class A Select Agent Yersinia Pestis. Vaccine (2010) 28(41):6714–22. doi: 10.1016/j.vaccine.2010.07.084
328. Audouy SA, van Selm S, van Roosmalen ML, Post E, Kanninga R, Neef J, et al. Development of Lactococcal GEM-Based Pneumococcal Vaccines. Vaccine (2007) 25(13):2497–506. doi: 10.1016/j.vaccine.2006.09.026
329. Shi W, Kou Y, Jiang H, Gao F, Kong W, Su W, et al. Novel Intranasal Pertussis Vaccine Based on Bacterium-Like Particles as a Mucosal Adjuvant. Immunol Lett (2018) 198:26–32. doi: 10.1016/j.imlet.2018.03.012
330. Liu W, Tan Z, Xue J, Luo W, Song H, Lv X, et al. Therapeutic Efficacy of Oral Immunization With a non-Genetically Modified Lactococcus Lactis-Based Vaccine CUE-GEM Induces Local Immunity Against Helicobacter Pylori Infection. Appl Microbiol Biotechnol (2016) 100(14):6219–29. doi: 10.1007/s00253-016-7333-y
331. Liu W, Tan Z, Liu H, Zeng Z, Luo S, Yang H, et al. Nongenetically Modified Lactococcus Lactis-Adjuvanted Vaccination Enhanced Innate Immunity Against Helicobacter Pylori. Helicobacter (2017) 22(5):e12426. doi: 10.1111/hel.12426
332. Stokes MG, Titball RW, Neeson BN, Galen JE, Walker NJ, Stagg AJ, et al. Oral Administration of a Salmonella Enterica-Based Vaccine Expressing Bacillus Anthracis Protective Antigen Confers Protection Against Aerosolized B. Anthracis. Infect Immun (2007) 75(4):1827–34. doi: 10.1128/IAI.01242-06
333. Zhao Z, Li M, Luo D, Xing L, Wu S, Duan Y, et al. Protection of Mice From Brucella Infection by Immunization With Attenuated Salmonella Enterica Serovar Typhimurium Expressing A L7/L12 and BLS Fusion Antigen of Brucella. Vaccine (2009) 27(38):5214–9. doi: 10.1016/j.vaccine.2009.06.075
334. Senevirathne A, Hewawaduge C, Lee JH. Live Vaccine Consisting of Attenuated Salmonella Secreting and Delivering Brucella Ribosomal Protein L7/L12 Induces Humoral and Cellular Immune Responses and Protects Mice Against Virulent Brucella Abortus 544 Challenge. Vet Res (2020) 51(1):6. doi: 10.1186/s13567-020-0735-y
335. Xu C, Zhang BZ, Lin Q, Deng J, Yu B, Arya S, et al. Live Attenuated Salmonella Typhimurium Vaccines Delivering SaEsxA and SaEsxB via Type III Secretion System Confer Protection Against Staphylococcus Aureus Infection. BMC Infect Dis (2018) 18(1):195. doi: 10.1186/s12879-018-3104-y
336. He Y, Vemulapalli R, Schurig GG. Recombinant Ochrobactrum Anthropi Expressing Brucella Abortus Cu,Zn Superoxide Dismutase Protects Mice Against B. Abortus Infection Only After Switching of Immune Responses to Th1 Type. Infect Immun (2002) 70(5):2535–43. doi: 10.1128/iai.70.5.2535-2543.2002
337. Lee WH, Choi HI, Hong SW, Kim KS, Gho YS, Jeon SG. Vaccination With Klebsiella Pneumoniae-Derived Extracellular Vesicles Protects Against Bacteria-Induced Lethality via Both Humoral and Cellular Immunity. Exp Mol Med (2015) 47:e183. doi: 10.1038/emm.2015.59
338. Roberts R, Moreno G, Bottero D, Gaillard ME, Fingermann M, Graieb A, et al. Outer Membrane Vesicles as Acellular Vaccine Against Pertussis. Vaccine (2008) 26(36):4639–46. doi: 10.1016/j.vaccine.2008.07.004
339. Bottero D, Gaillard ME, Errea A, Moreno G, Zurita E, Pianciola L, et al. Outer Membrane Vesicles Derived From Bordetella Parapertussis as an Acellular Vaccine Against Bordetella Parapertussis and Bordetella Pertussis Infection. Vaccine (2013) 31(45):5262–8. doi: 10.1016/j.vaccine.2013.08.059
340. Raeven RHM, Brummelman J, Pennings JLA, van der Maas L, Helm K, Tilstra W, et al. Molecular and Cellular Signatures Underlying Superior Immunity Against Bordetella Pertussis Upon Pulmonary Vaccination. Mucosal Immunol (2018) 11(3):1009. doi: 10.1038/mi.2017.110
341. Choi KS, Kim SH, Kim ED, Lee SH, Han SJ, Yoon S, et al. Protection From Hemolytic Uremic Syndrome by Eyedrop Vaccination With Modified Enterohemorrhagic E. Coli Outer Membrane Vesicles. PloS One (2014) 9(7):e100229. doi: 10.1371/journal.pone.0100229
342. Mitra S, Chakrabarti MK, Koley H. Multi-Serotype Outer Membrane Vesicles of Shigellae Confer Passive Protection to the Neonatal Mice Against Shigellosis. Vaccine (2013) 31(31):3163–73. doi: 10.1016/j.vaccine.2013.05.001
343. Gerke C, Colucci AM, Giannelli C, Sanzone S, Vitali CG, Sollai L, et al. Production of a Shigella Sonnei Vaccine Based on Generalized Modules for Membrane Antigens (GMMA), 1790gahb. PloS One (2015) 10(8):e0134478. doi: 10.1371/journal.pone.0134478
344. Obiero CW, Ndiaye AGW, Scire AS, Kaunyangi BM, Marchetti E, Gone AM, et al. A Phase 2a Randomized Study to Evaluate the Safety and Immunogenicity of the 1790GAHB Generalized Modules for Membrane Antigen Vaccine Against Shigella Sonnei Administered Intramuscularly to Adults From a Shigellosis-Endemic Country. Front Immunol (2017) 8:1884. doi: 10.3389/fimmu.2017.01884
345. Raso MM, Gasperini G, Alfini R, Schiavo F, Aruta MG, Carducci M, et al. GMMA and Glycoconjugate Approaches Compared in Mice for the Development of a Vaccine Against Shigella Flexneri Serotype 6. Vaccines (Basel) (2020) 8(2):160. doi: 10.3390/vaccines8020160
346. Schild S, Nelson EJ, Camilli A. Immunization With Vibrio Cholerae Outer Membrane Vesicles Induces Protective Immunity in Mice. Infect Immun (2008) 76(10):4554–63. doi: 10.1128/IAI.00532-08
347. Roy N, Barman S, Ghosh A, Pal A, Chakraborty K, Das SS, et al. Immunogenicity and Protective Efficacy of Vibrio Cholerae Outer Membrane Vesicles in Rabbit Model. FEMS Immunol Med Microbiol (2010) 60(1):18–27. doi: 10.1111/j.1574-695X.2010.00692.x
348. Alaniz RC, Deatherage BL, Lara JC, Cookson BT. Membrane Vesicles are Immunogenic Facsimiles of Salmonella Typhimurium That Potently Activate Dendritic Cells, Prime B and T Cell Responses, and Stimulate Protective Immunity In Vivo. J Immunol (2007) 179(11):7692–701. doi: 10.4049/jimmunol.179.11.7692
349. Howlader DR, Koley H, Sinha R, Maiti S, Bhaumik U, Mukherjee P, et al. Development of a Novel S. Typhi and Paratyphi A Outer Membrane Vesicles Based Bivalent Vaccine Against Enteric Fever. PloS One (2018) 13(9):e0203631. doi: 10.1371/journal.pone.0203631
350. Wang X, Thompson CD, Weidenmaier C, Lee JC. Release of Staphylococcus Aureus Extracellular Vesicles and Their Application as a Vaccine Platform. Nat Commun (2018) 9(1):1379. doi: 10.1038/s41467-018-03847-z
351. Fan X, Wang F, Zhou X, Chen B, Chen G. Size-Dependent Antibacterial Immunity of Staphylococcus Aureus Protoplast-Derived Particulate Vaccines. Int J Nanomed (2020) 15:10321–30. doi: 10.2147/IJN.S285895
352. Liu Q, Li X, Zhang Y, Song Z, Li R, Ruan H, et al. Orally-Administered Outer-Membrane Vesicles From Helicobacter Pylori Reduce H. Pylori Infection via Th2-Biased Immune Responses in Mice. Pathog Dis (2019) 77(5):ftz050. doi: 10.1093/femspd/ftz050
353. Wang X, Singh AK, Zhang X, Sun W. Induction of Protective Antiplague Immune Responses by Self-Adjuvanting Bionanoparticles Derived From Engineered Yersinia Pestis. Infect Immun (2020) 88(5):e00081–20. doi: 10.1128/IAI.00081-20
354. Wu G, Ji H, Guo X, Li Y, Ren T, Dong H, et al. Nanoparticle Reinforced Bacterial Outer-Membrane Vesicles Effectively Prevent Fatal Infection of Carbapenem-Resistant Klebsiella Pneumoniae. Nanomedicine (2020) 24:102148. doi: 10.1016/j.nano.2019.102148
355. Asensio CJ, Gaillard ME, Moreno G, Bottero D, Zurita E, Rumbo M, et al. Outer Membrane Vesicles Obtained From Bordetella Pertussis Tohama Expressing the Lipid A Deacylase PagL as a Novel Acellular Vaccine Candidate. Vaccine (2011) 29(8):1649–56. doi: 10.1016/j.vaccine.2010.12.068
356. Noroozi N, Gargari SLM, Nazarian S, Sarvary S, Adriani RR. Immunogenicity of Enterotoxigenic Escherichia Coli Outer Membrane Vesicles Encapsulated in Chitosan Nanoparticles. Iran J Basic Med Sci (2018) 21(3):284–91. doi: 10.22038/ijbms.2018.25886.6371
357. Camacho AI, de Souza J, Sanchez-Gomez S, Pardo-Ros M, Irache JM, Gamazo C. Mucosal Immunization With Shigella Flexneri Outer Membrane Vesicles Induced Protection in Mice. Vaccine (2011) 29(46):8222–9. doi: 10.1016/j.vaccine.2011.08.121
358. Camacho AI, Irache JM, de Souza J, Sanchez-Gomez S, Gamazo C. Nanoparticle-Based Vaccine for Mucosal Protection Against Shigella Flexneri in Mice. Vaccine (2013) 31(32):3288–94. doi: 10.1016/j.vaccine.2013.05.020
359. Sarvary S, Gargari SLM, Nazarian S, Adriani RR, Noroozi N. Immunogenicity of Shigella Sonnei Outer Membrane Vesicles Extracted in Different Environmental Conditions. Biologia (2021) 76):721–8. doi: 10.2478/s11756-020-00606-8
360. Chen G, Bai Y, Li Z, Wang F, Fan X, Zhou X. Bacterial Extracellular Vesicle-Coated Multi-Antigenic Nanovaccines Protect Against Drug-Resistant Staphylococcus Aureus Infection by Modulating Antigen Processing and Presentation Pathways. Theranostics (2020) 10(16):7131–49. doi: 10.7150/thno.44564
361. Valentine JL, Chen L, Perregaux EC, Weyant KB, Rosenthal JA, Heiss C, et al. Immunization With Outer Membrane Vesicles Displaying Designer Glycotopes Yields Class-Switched, Glycan-Specific Antibodies. Cell Chem Biol (2016) 23(6):655–65. doi: 10.1016/j.chembiol.2016.05.014
362. Kim OY, Choi SJ, Jang SC, Park KS, Kim SR, Choi JP, et al. Bacterial Protoplast-Derived Nanovesicles as Vaccine Delivery System Against Bacterial Infection. Nano Lett (2015) 15(1):266–74. doi: 10.1021/nl503508h
363. Irene C, Fantappie L, Caproni E, Zerbini F, Anesi A, Tomasi M, et al. Bacterial Outer Membrane Vesicles Engineered With Lipidated Antigens as a Platform for Staphylococcus Aureus Vaccine. Proc Natl Acad Sci USA (2019) 116(43):21780–8. doi: 10.1073/pnas.1905112116
364. Pan C, Sun P, Liu B, Liang H, Peng Z, Dong Y, et al. Biosynthesis of Conjugate Vaccines Using an O-Linked Glycosylation System. MBio (2016) 7(2):e00443–00416. doi: 10.1128/mBio.00443-16
365. Peek LJ, Middaugh CR, Berkland C. Nanotechnology in Vaccine Delivery. Adv Drug Delivery Rev (2008) 60(8):915–28. doi: 10.1016/j.addr.2007.05.017
366. Zhang J, Tarbet EB, Toro H, Tang DC. Adenovirus-Vectored Drug-Vaccine Duo as a Potential Driver for Conferring Mass Protection Against Infectious Diseases. Expert Rev Vaccines (2011) 10(11):1539–52. doi: 10.1586/erv.11.141
367. Shi Z, Zeng M, Yang G, Siegel F, Cain LJ, van Kampen KR, et al. Protection Against Tetanus by Needle-Free Inoculation of Adenovirus-Vectored Nasal and Epicutaneous Vaccines. J Virol (2001) 75(23):11474–82. doi: 10.1128/JVI.75.23.11474-11482.2001
368. Hien TT, de Jong M, Farrar J. Avian Influenza–a Challenge to Global Health Care Structures. N Engl J Med (2004) 351(23):2363–5. doi: 10.1056/NEJMp048267
369. Romanova J, Krenn BM, Wolschek M, Ferko B, Romanovskaja-Romanko E, Morokutti A, et al. Preclinical Evaluation of a Replication-Deficient Intranasal DeltaNS1 H5N1 Influenza Vaccine. PloS One (2009) 4(6):e5984. doi: 10.1371/journal.pone.0005984
370. Wacheck V, Egorov A, Groiss F, Pfeiffer A, Fuereder T, Hoeflmayer D, et al. A Novel Type of Influenza Vaccine: Safety and Immunogenicity of Replication-Deficient Influenza Virus Created by Deletion of the Interferon Antagonist NS1. J Infect Dis (2010) 201(3):354–62. doi: 10.1086/649428
371. Tabynov K, Ryskeldinova S, Sansyzbay A. An Influenza Viral Vector Brucella Abortus Vaccine Induces Good Cross-Protection Against Brucella Melitensis Infection in Pregnant Heifers. Vaccine (2015) 33(31):3619–23. doi: 10.1016/j.vaccine.2015.06.045
372. Tabynov K, Yespembetov B, Matikhan N, Ryskeldinova S, Zinina N, Kydyrbayev Z, et al. First Evaluation of an Influenza Viral Vector Based Brucella Abortus Vaccine in Sheep and Goats: Assessment of Safety, Immunogenicity and Protective Efficacy Against Brucella Melitensis Infection. Vet Microbiol (2016) 197:15–20. doi: 10.1016/j.vetmic.2016.11.001
373. Tabynov K, Yespembetov B, Ryskeldinova S, Zinina N, Kydyrbayev Z, Kozhamkulov Y, et al. Prime-Booster Vaccination of Cattle With an Influenza Viral Vector Brucella Abortus Vaccine Induces a Long-Term Protective Immune Response Against Brucella Abortus Infection. Vaccine (2016) 34(4):438–44. doi: 10.1016/j.vaccine.2015.12.028
374. Mailybayeva A, Yespembetov B, Ryskeldinova S, Zinina N, Sansyzbay A, Renukaradhya GJ, et al. Improved Influenza Viral Vector Based Brucella Abortus Vaccine Induces Robust B and T-Cell Responses and Protection Against Brucella Melitensis Infection in Pregnant Sheep and Goats. PloS One (2017) 12(10):e0186484. doi: 10.1371/journal.pone.0186484
375. Mailybayeva A, Ryskeldinova S, Zinina N, Zhou EM, Renukaradhya GJ, Tabynov K. Evaluation of Duration of Immunogenicity and Protective Efficacy of Improved Influenza Viral Vector-Based Brucella Abortus Vaccine Against Brucella Melitensis Infection in Sheep and Goats. Front Vet Sci (2020) 7:58. doi: 10.3389/fvets.2020.00058
376. Smith ME, Koser M, Xiao S, Siler C, McGettigan JP, Calkins C, et al. Rabies Virus Glycoprotein as a Carrier for Anthrax Protective Antigen. Virology (2006) 353(2):344–56. doi: 10.1016/j.virol.2006.05.010
377. McComb RC, Ho CL, Bradley KA, Grill LK, Martchenko M. Presentation of Peptides From Bacillus Anthracis Protective Antigen on Tobacco Mosaic Virus as an Epitope Targeted Anthrax Vaccine. Vaccine (2015) 33(48):6745–51. doi: 10.1016/j.vaccine.2015.10.075
378. LeCureux JS, Dean GA. Lactobacillus Mucosal Vaccine Vectors: Immune Responses Against Bacterial and Viral Antigens. mSphere (2018) 3(3):e00061–18. doi: 10.1128/mSphere.00061-18
379. Gilbert C, Robinson K, Le Page RW, Wells JM. Heterologous Expression of an Immunogenic Pneumococcal Type 3 Capsular Polysaccharide in Lactococcus Lactis. Infect Immun (2000) 68(6):3251–60. doi: 10.1128/iai.68.6.3251-3260.2000
380. Audouy SA, van Roosmalen ML, Neef J, Kanninga R, Post E, van Deemter M, et al. Lactococcus Lactis GEM Particles Displaying Pneumococcal Antigens Induce Local and Systemic Immune Responses Following Intranasal Immunization. Vaccine (2006) 24(26):5434–41. doi: 10.1016/j.vaccine.2006.03.054
381. Ermak TH, Giannasca PJ, Nichols R, Myers GA, Nedrud J, Weltzin R, et al. Immunization of Mice With Urease Vaccine Affords Protection Against Helicobacter Pylori Infection in the Absence of Antibodies and is Mediated by MHC Class II-Restricted Responses. J Exp Med (1998) 188(12):2277–88. doi: 10.1084/jem.188.12.2277
382. Kao JY, Zhang M, Miller MJ, Mills JC, Wang B, Liu M, et al. Helicobacter Pylori Immune Escape is Mediated by Dendritic Cell-Induced Treg Skewing and Th17 Suppression in Mice. Gastroenterology (2010) 138(3):1046–54. doi: 10.1053/j.gastro.2009.11.043
383. Mashburn-Warren LM, Whiteley M. Special Delivery: Vesicle Trafficking in Prokaryotes. Mol Microbiol (2006) 61(4):839–46. doi: 10.1111/j.1365-2958.2006.05272.x
384. Lee JC, Lee EJ, Lee JH, Jun SH, Choi CW, Kim SI, et al. Klebsiella Pneumoniae Secretes Outer Membrane Vesicles That Induce the Innate Immune Response. FEMS Microbiol Lett (2012) 331(1):17–24. doi: 10.1111/j.1574-6968.2012.02549.x
385. Raeven RH, Brummelman J, Pennings JL, van der Maas L, Tilstra W, Helm K, et al. Bordetella Pertussis Outer Membrane Vesicle Vaccine Confers Equal Efficacy in Mice With Milder Inflammatory Responses Compared to a Whole-Cell Vaccine. Sci Rep (2016) 6:38240. doi: 10.1038/srep38240
386. Song Z, Li B, Zhang Y, Li R, Ruan H, Wu J, et al. Outer Membrane Vesicles of Helicobacter Pylori 7.13 as Adjuvants Promote Protective Efficacy Against Helicobacter Pylori Infection. Front Microbiol (2020) 11:1340. doi: 10.3389/fmicb.2020.01340
387. Lee SR, Kim SH, Jeong KJ, Kim KS, Kim YH, Kim SJ, et al. Multi-Immunogenic Outer Membrane Vesicles Derived From an MsbB-Deficient Salmonella Enterica Serovar Typhimurium Mutant. J Microbiol Biotechnol (2009) 19(10):1271–9. doi: 10.4014/jmb.0901.0055
388. Leitner DR, Feichter S, Schild-Prufert K, Rechberger GN, Reidl J, Schild S. Lipopolysaccharide Modifications of a Cholera Vaccine Candidate Based on Outer Membrane Vesicles Reduce Endotoxicity and Reveal the Major Protective Antigen. Infect Immun (2013) 81(7):2379–93. doi: 10.1128/IAI.01382-12
389. Rossi O, Caboni M, Negrea A, Necchi F, Alfini R, Micoli F, et al. Toll-Like Receptor Activation by Generalized Modules for Membrane Antigens From Lipid A Mutants of Salmonella Enterica Serovars Typhimurium and Enteritidis. Clin Vaccine Immunol (2016) 23(4):304–14. doi: 10.1128/CVI.00023-16
390. De Benedetto G, Alfini R, Cescutti P, Caboni M, Lanzilao L, Necchi F, et al. Characterization of O-Antigen Delivered by Generalized Modules for Membrane Antigens (GMMA) Vaccine Candidates Against Nontyphoidal Salmonella. Vaccine (2017) 35(3):419–26. doi: 10.1016/j.vaccine.2016.11.089
391. Kim SH, Kim KS, Lee SR, Kim E, Kim MS, Lee EY, et al. Structural Modifications of Outer Membrane Vesicles to Refine Them as Vaccine Delivery Vehicles. Biochim Biophys Acta (2009) 1788(10):2150–9. doi: 10.1016/j.bbamem.2009.08.001
392. Kim SH, Lee SR, Kim KS, Ko A, Kim E, Kim YH, et al. Shiga Toxin A Subunit Mutant of Escherichia Coli O157:H7 Releases Outer Membrane Vesicles Containing the B-Pentameric Complex. FEMS Immunol Med Microbiol (2010) 58(3):412–20. doi: 10.1111/j.1574-695X.2010.00654.x
393. Fisseha M, Chen P, Brandt B, Kijek T, Moran E, Zollinger W. Characterization of Native Outer Membrane Vesicles From lpxL Mutant Strains of Neisseria Meningitidis for Use in Parenteral Vaccination. Infect Immun (2005) 73(7):4070–80. doi: 10.1128/IAI.73.7.4070-4080.2005
394. Sinha R, Howlader DR, Ta A, Mitra S, Das S, Koley H. Retinoic Acid Pre-Treatment Down Regulates V. Cholerae Outer Membrane Vesicles Induced Acute Inflammation and Enhances Mucosal Immunity. Vaccine (2017) 35(28):3534–47. doi: 10.1016/j.vaccine.2017.05.036
395. Fredriksen JH, Rosenqvist E, Wedege E, Bryn K, Bjune G, Froholm LO, et al. Production, Characterization and Control of MenB-Vaccine “Folkehelsa”: An Outer Membrane Vesicle Vaccine Against Group B Meningococcal Disease. NIPH Ann (1991) 14(2):67–79. discussion 79-80.
396. Borrow R, Balmer P, Miller E. Meningococcal Surrogates of Protection–Serum Bactericidal Antibody Activity. Vaccine (2005) 23(17-18):2222–7. doi: 10.1016/j.vaccine.2005.01.051
397. Tamayo I, Irache JM, Mansilla C, Ochoa-Reparaz J, Lasarte JJ, Gamazo C. Poly(anhydride) Nanoparticles Act as Active Th1 Adjuvants Through Toll-Like Receptor Exploitation. Clin Vaccine Immunol (2010) 17(9):1356–62. doi: 10.1128/CVI.00164-10
398. Pan C, Yue H, Zhu L, Ma GH, Wang HL. Prophylactic Vaccine Delivery Systems Against Epidemic Infectious Diseases. Adv Drug Delivery Rev (2021) 176:113867. doi: 10.1016/j.addr.2021.113867
399. Schwendener RA. Liposomes as Vaccine Delivery Systems: A Review of the Recent Advances. Ther Adv Vaccines (2014) 2(6):159–82. doi: 10.1177/2051013614541440
400. Wang N, Chen M, Wang T. Liposomes Used as a Vaccine Adjuvant-Delivery System: From Basics to Clinical Immunization. J Control Release (2019) 303:130–50. doi: 10.1016/j.jconrel.2019.04.025
401. Hayman WA, Toth I, Flinn N, Scanlon M, Good MF. Enhancing the Immunogenicity and Modulating the Fine Epitope Recognition of Antisera to a Helical Group A Streptococcal Peptide Vaccine Candidate From the M Protein Using Lipid-Core Peptide Technology. Immunol Cell Biol (2002) 80(2):178–87. doi: 10.1046/j.1440-1711.2002.01067.x
402. Olive C, Batzloff MR, Toth I. Lipid Core Peptide Technology and Group A Streptococcal Vaccine Delivery. Expert Rev Vaccines (2004) 3(1):43–58. doi: 10.1586/14760584.3.1.43
403. Ghaffar KA, Marasini N, Giddam AK, Batzloff MR, Good MF, Skwarczynski M, et al. Liposome-Based Intranasal Delivery of Lipopeptide Vaccine Candidates Against Group A Streptococcus. Acta Biomater (2016) 41:161–8. doi: 10.1016/j.actbio.2016.04.012
404. Marasini N, Khalil ZG, Giddam AK, Ghaffar KA, Hussein WM, Capon RJ, et al. Lipid Core Peptide/Poly(Lactic-Co-Glycolic Acid) as a Highly Potent Intranasal Vaccine Delivery System Against Group A Streptococcus. Int J Pharm (2016) 513(1-2):410–20. doi: 10.1016/j.ijpharm.2016.09.057
405. Bartlett S, Skwarczynski M, Toth I. Lipids as Activators of Innate Immunity in Peptide Vaccine Delivery. Curr Med Chem (2020) 27(17):2887–901. doi: 10.2174/0929867325666181026100849
406. Ghaffar KA, Marasini N, Giddam AK, Batzloff MR, Good MF, Skwarczynski M, et al. The Role of Size in Development of Mucosal Liposome-Lipopeptide Vaccine Candidates Against Group A Streptococcus. Med Chem (2016) 13(1):22–7. doi: 10.2174/1573406412666160720093138
407. Dai CC, Yang J, Hussein WM, Zhao L, Wang X, Khalil ZG, et al. Polyethylenimine: An Intranasal Adjuvant for Liposomal Peptide-Based Subunit Vaccine Against Group A Streptococcus. ACS Infect Dis (2020) 6(9):2502–12. doi: 10.1021/acsinfecdis.0c00452
408. Dai CC, Huang W, Yang J, Hussein WM, Wang J, Khalil ZG, et al. Polyethylenimine Quantity and Molecular Weight Influence its Adjuvanting Properties in Liposomal Peptide Vaccines. Bioorg Med Chem Lett (2021) 40:127920. doi: 10.1016/j.bmcl.2021.127920
409. Muto K, Kamei N, Yoshida M, Takayama K, Takeda-Morishita M. Cell-Penetrating Peptide Penetratin as a Potential Tool for Developing Effective Nasal Vaccination Systems. J Pharm Sci (2016) 105(6):2014–7. doi: 10.1016/j.xphs.2016.03.026
410. Bahadoran A, Ebrahimi M, Yeap SK, Safi N, Moeini H, Hair-Bejo M, et al. Induction of a Robust Immune Response Against Avian Influenza Virus Following Transdermal Inoculation With H5-DNA Vaccine Formulated in Modified Dendrimer-Based Delivery System in Mouse Model. Int J Nanomed (2017) 12:8573–85. doi: 10.2147/IJN.S139126
411. Huang HC, Lu HF, Lai YH, Lee CP, Liu HK, Huang C. Tat-Enhanced Delivery of the C Terminus of HDAg-L Inhibits Assembly and Secretion of Hepatitis D Virus. Antiviral Res (2018) 150:69–78. doi: 10.1016/j.antiviral.2017.12.009
412. Yang J, Firdaus F, Azuar A, Khalil ZG, Marasini N, Capon RJ, et al. Cell-Penetrating Peptides-Based Liposomal Delivery System Enhanced Immunogenicity of Peptide-Based Vaccine Against Group A Streptococcus. Vaccines (Basel) (2021) 9(5):499. doi: 10.3390/vaccines9050499
413. Ribeiro AM, Chaimovich H. Preparation and Characterization of Large Dioctadecyldimethylammonium Chloride Liposomes and Comparison With Small Sonicated Vesicles. Biochim Biophys Acta (1983) 733(1):172–9. doi: 10.1016/0005-2736(83)90103-7
414. Carmona-Ribeiro AM, Chaimovich H. Salt-Induced Aggregation and Fusion of Dioctadecyldimethylammonium Chloride and Sodium Dihexadecylphosphate Vesicles. Biophys J (1986) 50(4):621–8. doi: 10.1016/S0006-3495(86)83501-9
415. Davidsen J, Rosenkrands I, Christensen D, Vangala A, Kirby D, Perrie Y, et al. Characterization of Cationic Liposomes Based on Dimethyldioctadecylammonium and Synthetic Cord Factor From M. Tuberculosis (Trehalose 6,6’-Dibehenate)-a Novel Adjuvant Inducing Both Strong CMI and Antibody Responses. Biochim Biophys Acta (2005) 1718(1-2):22–31. doi: 10.1016/j.bbamem.2005.10.011
416. Kamath AT, Rochat AF, Christensen D, Agger EM, Andersen P, Lambert PH, et al. A Liposome-Based Mycobacterial Vaccine Induces Potent Adult and Neonatal Multifunctional T Cells Through the Exquisite Targeting of Dendritic Cells. PloS One (2009) 4(6):e5771. doi: 10.1371/journal.pone.0005771
417. Kaushal D, Mehra S, Didier PJ, Lackner AA. The non-Human Primate Model of Tuberculosis. J Med Primatol (2012) 41(3):191–201. doi: 10.1111/j.1600-0684.2012.00536.x
418. Pena JC, Ho WZ. Non-Human Primate Models of Tuberculosis. Microbiol Spectr (2016) 4(4). doi: 10.1128/microbiolspec.TBTB2-0007-2016
419. McMurray DN. A Nonhuman Primate Model for Preclinical Testing of New Tuberculosis Vaccines. Clin Infect Dis (2000) 30:S210–212. doi: 10.1086/313885
420. Kaushal D, Foreman TW, Gautam US, Alvarez X, Adekambi T, Rangel-Moreno J, et al. Mucosal Vaccination With Attenuated Mycobacterium Tuberculosis Induces Strong Central Memory Responses and Protects Against Tuberculosis. Nat Commun (2015) 6:8533. doi: 10.1038/ncomms9533
421. Foreman TW, Veatch AV, LoBato DN, Didier PJ, Doyle-Meyers LA, Russell-Lodrigue KE, et al. Nonpathologic Infection of Macaques by an Attenuated Mycobacterial Vaccine Is Not Reactivated in the Setting of HIV Co-Infection. Am J Pathol (2017) 187(12):2811–20. doi: 10.1016/j.ajpath.2017.08.014
422. Goldberg AC, Rizzo LV. MHC Structure and Function - Antigen Presentation. Part 1. Einstein (Sao Paulo Brazil) (2015) 13(1):153–6. doi: 10.1590/s1679-45082015rb3122
423. Gregoriadis S, Zervas J, Varletzidis E, Toubis M, Pantazopoulos P, Fessas P. HLA Antigens and Otosclerosis. A Possible New Genetic Factor. Arch Otolaryngol (Chicago Ill: 1960) (1982) 108(12):769–71. doi: 10.1001/archotol.1982.00790600013004
424. Singhal SK, Mann SB, Datta U, Panda NK, Gupta AK. Genetic Correlation in Otosclerosis. Am J Otolaryngol (1999) 20(2):102–5. doi: 10.1016/s0196-0709(99)90019-4
425. Xu XP, Li SB, Wang CY, Li QH. Study on the Association of HLA With Pulmonary Tuberculosis. Immunol Investigat (1986) 15(4):327–32. doi: 10.3109/08820138609052951
426. Kim SJ, Choi IH, Dahlberg S, Nisperos B, Kim JD, Hansen JA. HLA and Leprosy in Koreans. Tissue Antigens (1987) 29(3):146–53. doi: 10.1111/j.1399-0039.1987.tb01567.x
427. Iannetti P, Morellini M, Raucci U, Cappellacci S. HLA Antigens, Epilepsy and Cytomegalovirus Infection. Brain Dev (1988) 10(4):256–8. doi: 10.1016/s0387-7604(88)80008-1
428. Konieczna A, Turowski G. HLA-ABC Antigens in Supraglottic Cancer Patients and Their Relationship With Incidence and Survival. Materia Medica Polona. Polish J Med Pharm (1993) 25(2):73–9.
429. Barona P, Sierrasesumaga L, Antillon F, Villa-Elizaga I. Study of HLA Antigens in Patients With Osteosarcoma. Hum Hered (1993) 43(5):311–4. doi: 10.1159/000154149
430. Marcos Y, Fainboim HA, Capucchio M, Findor J, Daruich J, Reyes B, et al. Two-Locus Involvement in the Association of Human Leukocyte Antigen With the Extrahepatic Manifestations of Autoimmune Chronic Active Hepatitis. Hepatol (Baltimore Md) (1994) 19(6):1371–4. doi: 10.1016/0270-9139(94)90230-5
431. Kaslow RA, Carrington M, Apple R, Park L, Munoz A, Saah AJ, et al. Influence of Combinations of Human Major Histocompatibility Complex Genes on the Course of HIV-1 Infection. Nat Med (1996) 2(4):405–11. doi: 10.1038/nm0496-405
432. Carrington M, O’Brien SJ. The Influence of HLA Genotype on AIDS. Annu Rev Med (2003) 54:535–51. doi: 10.1146/annurev.med.54.101601.152346
433. Zhang YH, Peng YC, Yan HP, Xu KY, Saito M, Wu H, et al. Multilayered Defense in HLA-B51-Associated HIV Viral Control. J Immunol (2011) 187(2):684–91. doi: 10.4049/jimmunol.1100316
434. Apps R, Qi Y, Carlson JM, Chen HY, Gao XJ, Thomas R, et al. Influence of HLA-C Expression Level on HIV Control. Science (2013) 340(6128):87–91. doi: 10.1126/science.1232685
435. Zhang H, Zhao B, Han XX, Wang Z, Liu BG, Lu CM, et al. Associations of HLA Class I Antigen Specificities and Haplotypes With Disease Progression in HIV-1-Infected Hans in Northern China. Hum Immunol (2013) 74(12):1636–42. doi: 10.1016/j.humimm.2013.08.287
436. Taneja V, David CS. HLA Transgenic Mice as Humanized Mouse Models of Disease and Immunity. J Clin Invest (1998) 101(5):921–6. doi: 10.1172/jci2860
437. Pascolo S. HLA Class I Transgenic Mice: Development, Utilisation and Improvement. Expert Opin Biol Ther (2005) 5(7):919–38. doi: 10.1517/14712598.5.7.919
438. Kievits F, Ivanyi P, Krimpenfort P, Berns A, Ploegh HL. HLA-Restricted Recognition of Viral Antigens in HLA Transgenic Mice. Nature (1987) 329(6138):447–9. doi: 10.1038/329447a0
439. Chamberlain JW, Nolan JA, Gromkowski SH, Kelley KA, Eisenstadt JM, Herrup K, et al. Cell Surface Expression and Alloantigenic Function of a Human Class I MHC Heavy Chain Gene (HLA-B7) in Transgenic Mice. J Immunol (Baltimore Md: 1950) (1988) 140(4):1285–92. doi: 10.0000/PMID3125253
440. Bernhard EJ, Le AX, Barbosa JA, Lacy E, Engelhard VH. Cytotoxic T Lymphocytes From HLA-A2 Transgenic Mice Specific for HLA-A2 Expressed on Human Cells. J Exp Med (1988) 168(3):1157–62. doi: 10.1084/jem.168.3.1157
441. Dill O, Kievits F, Koch S, Ivanyi P, Hammerling GJ. Immunological Function of HLA-C Antigens in HLA-Cw3 Transgenic Mice. Proc Natl Acad Sci USA (1988) 85(15):5664–8. doi: 10.1073/pnas.85.15.5664
442. Connolly JM, Potter TA, Wormstall EM, Hansen TH. The Lyt-2 Molecule Recognizes Residues in the Class I Alpha 3 Domain in Allogeneic Cytotoxic T Cell Responses. J Exp Med (1988) 168(1):325–41. doi: 10.1084/jem.168.1.325
443. Irwin MJ, Heath WR, Sherman LA. Species-Restricted Interactions Between CD8 and the Alpha 3 Domain of Class I Influence the Magnitude of the Xenogeneic Response. J Exp Med (1989) 170(4):1091–101. doi: 10.1084/jem.170.4.1091
444. Engelhard VH, Lacy E, Ridge JP. Influenza A-Specific, HLA-A2.1-Restricted Cytotoxic T Lymphocytes From HLA-A2.1 Transgenic Mice Recognize Fragments of the M1 Protein. J Immunol (Baltimore Md (1991) 1950) 146(4):1226–32.
445. Barra C, Gournier H, Garcia Z, Marche PN, Jouvin-Marche E, Briand P, et al. Abrogation of H-2-Restricted CTL Responses and Efficient Recognition of HLA-A3 Molecules in DBA/2 HLA/A24 Responder Mice. J Immunol (Baltimore Md: 1950) (1993) 150(9):3681–9.
446. Boucherma R, Kridane-Miledi H, Bouziat R, Rasmussen M, Gatard T, Langa-Vives F, et al. HLA-A*01:03, HLA-A*24:02, HLA-B*08:01, HLA-B*27:05, HLA-B*35:01, HLA-B*44:02, and HLA-C*07:01 Monochain Transgenic/H-2 Class I Null Mice: Novel Versatile Preclinical Models of Human T Cell Responses. J Immunol (2013) 191(2):583–93. doi: 10.4049/jimmunol.1300483
447. LaFace DM, Vestberg M, Yang Y, Srivastava R, DiSanto J, Flomenberg N, et al. Human CD8 Transgene Regulation of HLA Recognition by Murine T Cells. J Exp Med (1995) 182(5):1315–25. doi: 10.1084/jem.182.5.1315
448. Kalinke U, Arnold B, Hammerling GJ. Strong Xenogeneic HLA Response in Transgenic Mice After Introducing an Alpha 3 Domain Into HLA B27. Nature (1990) 348(6302):642–4. doi: 10.1038/348642a0
449. Vitiello A, Marchesini D, Furze J, Sherman LA, Chesnut RW. Analysis of the HLA-Restricted Influenza-Specific Cytotoxic T Lymphocyte Response in Transgenic Mice Carrying a Chimeric Human-Mouse Class I Major Histocompatibility Complex. J Exp Med (1991) 173(4):1007–15. doi: 10.1084/jem.173.4.1007
450. Pascolo S, Bervas N, Ure JM, Smith AG, Lemonnier FA, Perarnau B. HLA-A2.1-Restricted Education and Cytolytic Activity of CD8(+) T Lymphocytes From Beta2 Microglobulin (Beta2m) HLA-A2.1 Monochain Transgenic H-2Db Beta2m Double Knockout Mice. J Exp Med (1997) 185(12):2043–51. doi: 10.1084/jem.185.12.2043
451. Zeng Y, Gao TT, Zhao GY, Jiang YT, Yang Y, Yu H, et al. Generation of Human MHC (HLA-A11/DR1) Transgenic Mice for Vaccine Evaluation. Hum Vaccines Immunother (2016) 12(3):829–36. doi: 10.1080/21645515.2015.1103405
452. Li D, Li P, Song NP, Jiang YT, Zeng Y, Zhao GY, et al. Identification of Novel HLA-A11-Restricted T-Cell Epitopes in the Ebola Virus Nucleoprotein. Microbes Infection (2019) 21(1):56–62. doi: 10.1016/j.micinf.2018.04.005
453. Jin XX, Ding Y, Sun SH, Wang XY, Zhou ZN, Liu XT, et al. Screening HLA-A-Restricted T Cell Epitopes of SARS-CoV-2 and the Induction of CD8(+) T Cell Responses in HLA-A Transgenic Mice. Cell Mol Immunol (2021) 18(12):2588–608. doi: 10.1038/s41423-021-00784-8
Keywords: tuberculosis (TB), peptide-based vaccine, epitope, bioinformatics, immunity, adjuvants, animal models
Citation: Gong W, Pan C, Cheng P, Wang J, Zhao G and Wu X (2022) Peptide-Based Vaccines for Tuberculosis. Front. Immunol. 13:830497. doi: 10.3389/fimmu.2022.830497
Received: 07 December 2021; Accepted: 10 January 2022;
Published: 31 January 2022.
Edited by:
Stephanie Yanow, University of Alberta, CanadaReviewed by:
Ved Prakash Dwivedi, International Centre for Genetic Engineering and Biotechnology, IndiaSandeep Kumar Dhanda, St. Jude Children’s Research Hospital, United States
Copyright © 2022 Gong, Pan, Cheng, Wang, Zhao and Wu. This is an open-access article distributed under the terms of the Creative Commons Attribution License (CC BY). The use, distribution or reproduction in other forums is permitted, provided the original author(s) and the copyright owner(s) are credited and that the original publication in this journal is cited, in accordance with accepted academic practice. No use, distribution or reproduction is permitted which does not comply with these terms.
*Correspondence: Xueqiong Wu, eHVlcWlvbmd3dUAxMzkuY29t; Guangyu Zhao, Z3Vhbmd5dTA1MjVAMTYzLmNvbQ==
†These authors have contributed equally to this work