- 1Department of the Clinical Research Center, Henan Provincial People’s Hospital, People’s Hospital of Zhengzhou University, Zhengzhou, Henan, China
- 2Department of Endocrinology, Henan Provincial Key Medicine Laboratory of Intestinal Microecology and Diabetes, Henan Provincial People’s Hospital, People’s Hospital of Zhengzhou University, Zhengzhou, Henan, China
- 3Department of Clinical Microbiology, Henan Provincial People’s Hospital, People’s Hospital of Zhengzhou University, Zhengzhou, Henan, China
- 4Department of Blood Transfusion, Henan Provincial People’s Hospital, Department of Blood Transfusion of Central China Fuwai Hospital, Central China Fuwai Hospital of Zhengzhou University, Zhengzhou, Henan, China
The prevalence of autoimmune diseases worldwide has risen rapidly over the past few decades. Increasing evidence has linked gut dysbiosis to the onset of various autoimmune diseases. Thanks to the significant advancements in high-throughput sequencing technology, the number of gut microbiome studies has increased. However, they have primarily focused on bacteria, so our understanding of the role and significance of eukaryotic microbes in the human gut microbial ecosystem remains quite limited. Here, we selected Graves’ disease (GD) as an autoimmune disease model and investigated the gut multi-kingdom (bacteria, fungi, and protists) microbial communities from the health control, diseased, and medication-treated recovered patients. The results showed that physiological changes in GD increased homogenizing dispersal processes for bacterial community assembly and increased homogeneous selection processes for eukaryotic community assembly. The recovered patients vs. healthy controls had similar bacterial and protistan, but not fungal, community assembly processes. Additionally, eukaryotes (fungi and protists) may play a more significant role in gut ecosystem functions than bacteria. Overall, this study gives brief insights into the potential contributions of eukaryotes to gut and immune homeostasis in humans and their potential influence in relation to therapeutic interventions.
1 Introduction
The vital role of the gut microbiome in immune homeostasis and the maintenance of health in humans has been highlighted in numerous studies, revealing that the gut microbiome is inextricably linked to the development of various metabolic and autoimmune diseases. Graves’ disease (GD) is a common autoimmune disease and a major cause of hyperthyroidism. It occurs at all ages and is more common in women (1). Previous research mainly focused on the disruption of microbial taxa in GD patients, involving increased Prevotellaceae, Lactobacillales, and Bacilli and decreased Rikenellaceae, Alistipes, and Enterobacteriaceae (2, 3). However, previous studies only focused on the intestinal bacterial communities, while eukaryotes (such as fungi and protists) have rarely been studied. Nevertheless, the human gut microbiome is composed of multi-kingdom microbial communities that play indispensable but largely unrecognized roles. Turning our attention to the “microbial zoo” of the gut ecosystem will help to reveal the mechanisms underlying the link between the microbiome and immune disorders (4, 5).
The assembly and succession of complex gut microbiomes have attracted much attention in recent years. The assembly of ecological communities is normally influenced by both stochastic and deterministic processes (6). Deterministic processes indicate that the community diversity and structure are directional and predictable (7). For instance, diet (8), host genetics (9), medication use (10), and endocrine factors (11) can partially explain inter-individual microbiome variation via deterministic processes, while the external environmental factors seem impossible to influence communities through stochastic processes (also known as neutral processes), such as dispersal, specialization, and drift, even though they may also play a role in ecosystem shaping (12). In various health and disease states, the microbial load itself may be an identifier of a particular ecosystem configuration (13). However, the microbiome assembly processes in recovered patients after intervention, as well as the improvement of their gut dysbiosis, are generally overlooked. Furthermore, although interactions between certain physiological drivers and microbiome assembly have been studied (4, 14), our understanding of the ecological mechanisms underlying disordered and recovered gut microbiomes remains vague. Understanding these mechanisms is crucial for understanding overall health outcomes of GD.
In this study, we collected samples from 59 individuals, comprising 20 GD patients, 19 GD patients who had recovered after taking medication, and 20 healthy people, and assessed the ecological processes underlying their microbial kingdom (bacterial, fungal, and protistan) community assembly. In addition, we performed inter-kingdom analysis within each group to further explore the interactions among the microbial kingdoms and physiological indexes. The results extend our knowledge of GD in terms of the multi-kingdom microbial ecology, and provide a potential direction for the development of novel GD treatments based on gut fungi and protists as they may play a more significant role in gut ecosystem functions than bacteria.
2 Materials and methods
2.1 Participant recruitment and sample collection
From March 2020 to March 2021, 59 participants, comprising (1) 20 GD patients (Disease group), (2) 19 GD patients who recovered after medication treatment (Recovered group), and (3) 20 healthy controls (Healthy group), were recruited from the central plains of China. The diagnostic criteria for GD and the inclusion and exclusion criteria for the participants are described in detail in the Supplementary Materials.
All participants fasted overnight (≥8 h) before sample collection. Fecal samples were collected for DNA extraction, and serum samples were collected to assess thyroid hormones and inflammatory factors.
2.2 Measurement of physiological indexes
Participants’ demographic and clinical data were collected using questionnaires and electronic medical records. Chemiluminescence immunoassays were performed to assess free tetraiodothyronine (FT4), free triiodothyronine (FT3), thyroid-stimulating hormone (TSH), and thyrotropin receptor auto-antibodies (TRAb) using a Cobas e602 analyzer (Roche Diagnostics, Switzerland), and to assess thyroid peroxidase antibodies (TPOAb) and anti-thyroglobulin antibodies (TgAb) using a UniCel DxI 800 analyzer (Beckman Coulter, USA). Serum levels of inflammatory factors (tumor necrosis factor α, TNFα; interleukin 4, IL4; interleukin 6, IL-6; interleukin 10, IL10; interleukin 17, IL17) were assessed using human enzyme-linked immunosorbent assay (ELISA) kits (Cusabio Biotech, Wuhan, China). Detailed information is provided in Supplementary Table S1.
2.3 DNA extraction and Illumina MiSeq sequencing
The total DNA was extracted from the fecal samples using a QIAamp Fast DNA Stool Mini Kit (QIAGEN, Germany). DNA concentration and quality were assessed using a NanoDrop 2000 spectrophotometer (Thermo Fisher, USA). To amplify the V5–V7 region of the bacterial 16S rRNA gene, the ITS1 region of the fungal ITS gene, and the V4 region of the protistan 18S rRNA gene, the following primer pairs were used: 799F/1193R (799F: 5′-AAC MGG ATT AGA TAC CCK-3′; 1193R: 5′-ACG TCA TCC CCA CCT TCC-3′) (15), ITS1F/ITS2 (ITS1F: 5′-CTT GGT CAT TTA GAG GAA GTA A-3′; ITS2: 5′-GCT GCG TTC TTC ATC GAT GC-3′) (16, 17), and V4_1F/TAReukREV (5′-CCA GCA SCY GCG GTA ATW CC-3′; 5′-ACT TTC GTT CTT GAT YRA-3′) (18), respectively. Amplicon sequencing was conducted using an Illumina MiSeq platform by Magigen Biotechnology Co., Ltd. (Guangzhou, China).
Quality control of the sequence reads was performed using the UPARSE pipeline (19). Paired-end reads were assembled and trimmed (maximal expected errors of 0.25, reads length >300 bp for bacteria and protists, reads length >200 bp for fungi). Filtered sequences were clustered into 100% sequence similar zero-radius operational taxonomic unit (zOTU) using the UNOISE 3 algorithm implemented in USEARCH. For the bacterial communities, this step generated a 16S zOTU table of 59 samples × 3,370 zOTUs (3,275,695 reads). The number of high-quality sequences per sample was 31,660–95,736. For the fungal communities, this step generated an ITS zOTU table of 51 samples × 3,006 zOTUs (2,528,186 reads, the sample with high-quality sequences less than 3,000 was discarded). The number of high-quality sequences per sample was 3,615–133,846. Bacterial and fungal zOTUs were classified by the RDP classifier against the RDP 16 S rRNA gene database and the UNITE ITS database, respectively (20). Eukaryotic zOTUs were classified against the Protist Ribosomal Reference database (PR2) (21). We discarded zOTUs assigned as Rhodophyta, Streptophyta, Metazoa, Fungi, and unclassified Opisthokonta sequences to obtain the protistan zOTU table. For the protistan communities, we finally obtained a zOTUs table of 50 samples × 5,800 zOTUs (1,043,313 reads, the sample with high-quality sequences less than 3,000 was discarded). The number of high-quality sequences per sample was 3,062–61,354. To obtain an equivalent sequencing depth for further bioinformatics analysis, each sample was rarefied to 31,660 for bacterial communities, 3,615 sequences for fungal communities, and 3,062 for protistan communities in R through the package “GUniFrac” (Function: Rarefy).
2.4 Bioinformatics analysis of microbial sequencing results
Alpha diversity indicators, that is, richness (total number of observed species [Sobs]) and Shannon index, were determined for each sample using “vegan” (function: diversity) in R v4.2.2 for Windows. The differences among groups were determined based on the Wilcoxon signed-rank test using “ggpubr”.
The Bray–Curtis distance among different groups was determined using “vegan” based on zOTUs table, and the dissimilarities in microbial community composition were then visualized based on principal coordinate analysis (PCoA) plots using “ggplot2”. Subsequently, permutational multivariate analysis of variance (PERMANOVA) was conducted using “vegan” (function: adonis) with 9,999 permutations to assess the dissimilarities in community structure among different groups. The differences among groups were determined using Kruskal-Wallis test in IBM SPSS version 26.
The major ecological processes were determined in order to disentangle the characteristics of gut microbial community assembly for each kingdom (bacteria, fungi, and protists) in the Disease, Recovered, and Healthy groups. First, a neutral community model (NCM) was applied to predict the potential importance of stochastic processes in community assembly by determining the relationships between the microbial taxa detection frequency in a group of communities and their relative abundance across the metacommunity of all groups (22). Then, the dynamics of phylogenetic and taxonomic diversity were assessed using beta nearest taxon indices (βNTI) based on the null-model and Bray–Curtis-based Raup–Crick (RCBray) metrics through the R package “iCAMP”. The value of |βNTI| > 2 indicates that the deterministic processes, which can be divided into homogeneous selection (βNTI < −2, leading to similar community structures in similar environments) and variable selection (βNTI > 2, leading to dissimilar community structures in heterogeneous conditions), primarily govern community assembly. Conversely, |βNTI| < 2 suggests that stochastic processes govern the community compositions. Then, the RCBray was used to partition the stochastic processes. |RCbray| > 0.95 indicates homogenizing dispersal (RCbray < −0.95) or dispersal limitation (RCbray > 0.95) drives compositional turnover. When |βNTI| < 2 and |RCbray| < 0.95, this estimates the influence of “undominated” assembly, including weak dispersal, weak selection, diversification, and/or drift (23). The Linear regression model between physiological indexes and βNTI was calculated through the R package “ggpmisc”.
To evaluate the differences among all groups, an inter-kingdom network was separately constructed for each group. The zOTU tables were firstly calculated to the taxa table on a genus level. Then, the taxa tables for each dataset were limited to taxa present in at least half of the samples and comprised taxa with relative abundance ≥0.1% in each group. For each dataset, Spearman correlation scores were calculated in the MENA online pipeline (http://ieg4.rccc.ou.edu/mena/). The inter-kingdom co-occurrence networks were visualized in Gephi (version 0.9.2 for Windows).
3 Results
There were no significant differences in age or body mass index (BMI) among the three groups. Regarding the thyroid hormones, FT3 and FT4 were significantly lower in the Healthy and Recovered groups than the Disease group. TSH, TgAb, TPOAb, and TRAb were similar between the Disease and Recovered groups, but there were significant differences compared to the Healthy group. There were no significant differences in inflammatory factors among the three groups (Supplementary Figure S1).
The sequencing results of the bacterial, fungal, and protistan communities revealed that there were no significant differences in alpha diversity (Sobs or Shannon index) among the three groups (Supplementary Figure S2). There were differences among the three groups in the bacterial, fungal, and protistan communities based on Bray–Curtis distance, but the differences were only significant for the fungal communities (Figure 1A).
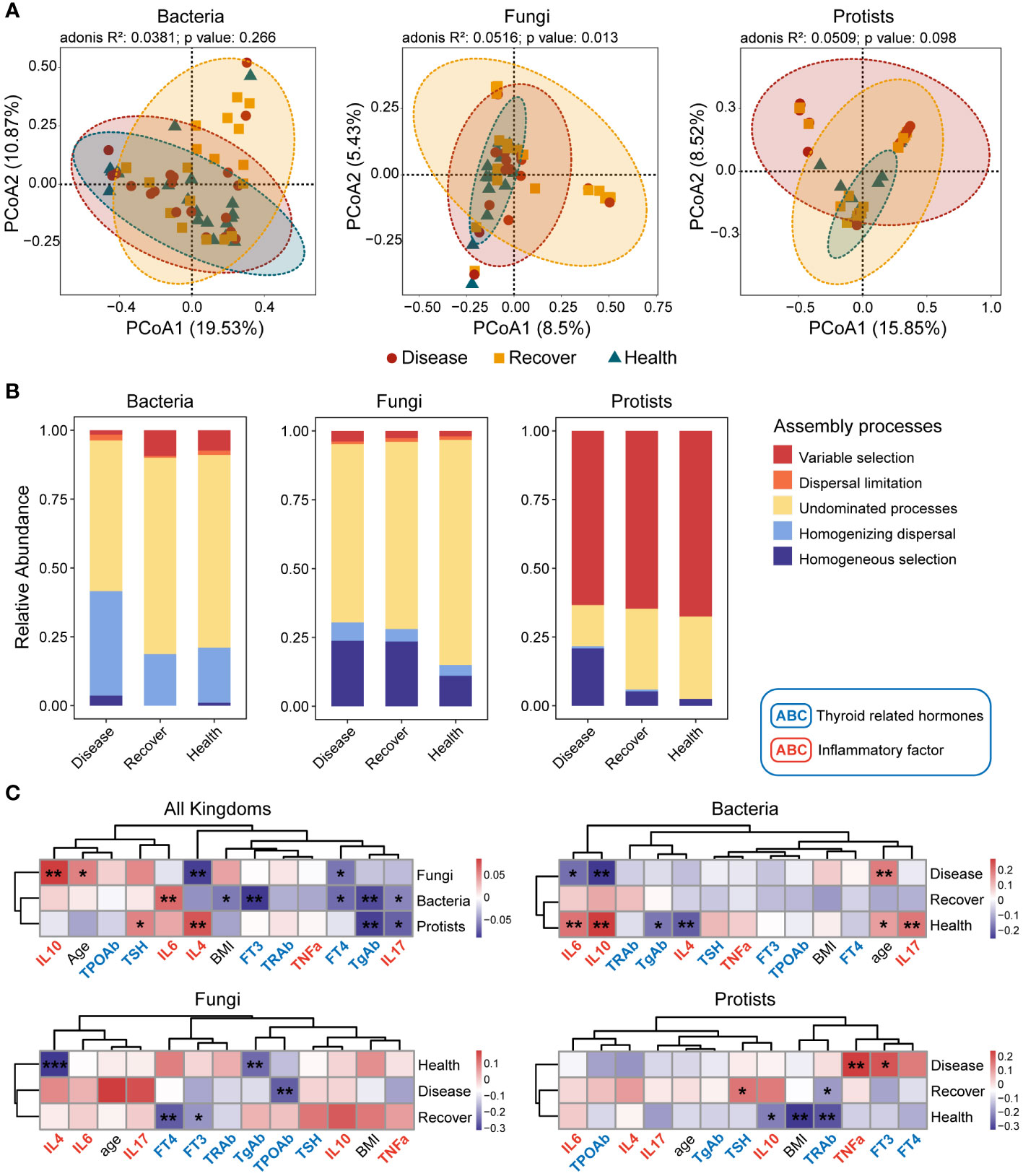
Figure 1 Bacterial, fungal, and protistan community compositions and their assembly processes. (A) PCoA plots based on Bray–Curtis distance for bacterial, fungal, and protistan communities. The p-values were calculated using PERMANOVA. (B) Relative influences of distinct processes on microbial community assembly. (C) Correlations between βNTI values and differences in physiological indexes. *p ≤ 0.05, **p ≤ 0.01 based on linear regression.
The three kingdoms all exhibited more convergence in the PCoA plots in the Healthy group. Therefore, we determined the assembly processes for each of the microbial communities. The NCM effectively predicted a significant portion of the associations between the frequency of occurrence of bacterial zOTUs and their changes in relative abundance, but it failed for fungal and protistan zOTUs. The NCM results indicated that stochastic processes were more important for bacterial community assembly in the Disease and Recovered groups than the Healthy group (Supplementary Figures S3–S5). We then evaluated the assembly processes based on the β-nearest taxon index (βNTI) and Bray–Curtis-based Raup–Crick (RCBray). Regarding bacterial community assembly, we found that homogenizing dispersal and undominated processes were the dominant processes, but there was more variable selection in the Healthy and Recovered groups than the Disease group. Regarding fungal community assembly, undominated processes dominated, but there was more homogeneous selection in the Disease and Recovered groups than the Healthy group. Lastly, regarding protistan community assembly, variable selection dominated, and there were more homogeneous selection and less undominated processes in the Disease group than the Recovered and Healthy groups (Supplementary Figure S6; Figure 1B). Based on family- and genus-level taxonomy analysis, the bacterial and protistan communities between the Healthy and Recovered groups were found to be similar. However, in terms of the fungal community, similarities were observed between the Disease and Recovered groups (Supplementary Figures S7, S8). Specifically, Lachnospiraceae and Eggerthellaceae were significantly higher in Disease than other bacterial groups. The abundance of Saccharomycetales incertae sedis was significantly lower in Healthy compared to other fungal groups, while Piptocephalidaceae and Spizellomycetaceae were significantly higher in Healthy. Blastocystis was higher in Healthy and Recovered than that in the protistan group of Disease (Supplementary Figure S7).
We also evaluated the relationships between βNTI values and physiological indexes (thyroid hormones and inflammatory factors) to further analyze the processes’ relative influences regarding mediating microbial community assembly. Many physiological indexes (such as TSH, FT3, FT4, TgAb, IL4, IL6, IL10, and IL17) influenced the microbial community assembly. There was a similar assembly process, influenced by thyroid hormones and inflammatory factors, for bacterial and protistan communities but not fungal communities (Figure 1C).
Moreover, we analyzed the assembly processes for each microbial kingdom in depth. The results showed that physiological indexes in the Recovered vs. Healthy groups similarly influenced bacterial and protistan community assembly, and physiological indexes in the Disease vs. Recovered groups similarly influenced fungal community assembly. The results indicated that the three microbial kingdoms in the Disease vs. Healthy groups were assembled via different processes, and the Recovered group fell between these two.
To further explore the interactions among the microbial kingdoms and physiological indexes, we constructed separate inter-kingdom networks for the Disease group (139 nodes and 635 edges), Recovered group (118 nodes and 379 edges), and Healthy group (121 nodes, 387 edges). The Disease network was the most complicated. Interestingly, the protists in the Disease network had 28.8% of nodes that were related to 60.8% of edges, which were much higher values than in the Recovered network (9.3% of nodes and 23.5% of edges) and Healthy network (10.7% of nodes and 21.7% of edges). There were also relatively fewer fungal nodes and edges in the Disease network (22.3% of nodes and 12.9% of edges) than the Recovered (33.0% of nodes and 54.9% of edges) and Healthy (37.2% of nodes and 50.9% of edges) networks. Moreover, we constructed three sub-networks that were based on all the nodes directly associated with physiological indexes, and we found that there were more protists and bacteria in the Disease network than the Recovered and Healthy networks, indicating the consequence of the ecological assembly under GD, which involves the physiological changes caused by GD, such as IL17, TSH, and TNFa, complexed with the gut microbial inter-kingdom interaction. Specifically, edges associated with bacterial taxonomy, such as Limosilactobacillus, Lacticaseibacillus, Bavariicoccus, and Roseburia, and Protistan taxonomy, such as Filamoeba, Paracercomonas, Pseudodendromonadales_XX, and Neoheteromita, were increased in Disease. The edges associated with fungal taxonomy, such as Torulaspora, Guehomyces, Saccharomyces, and Eurotium, were increased in Recovered or Healthy (Figure 2).
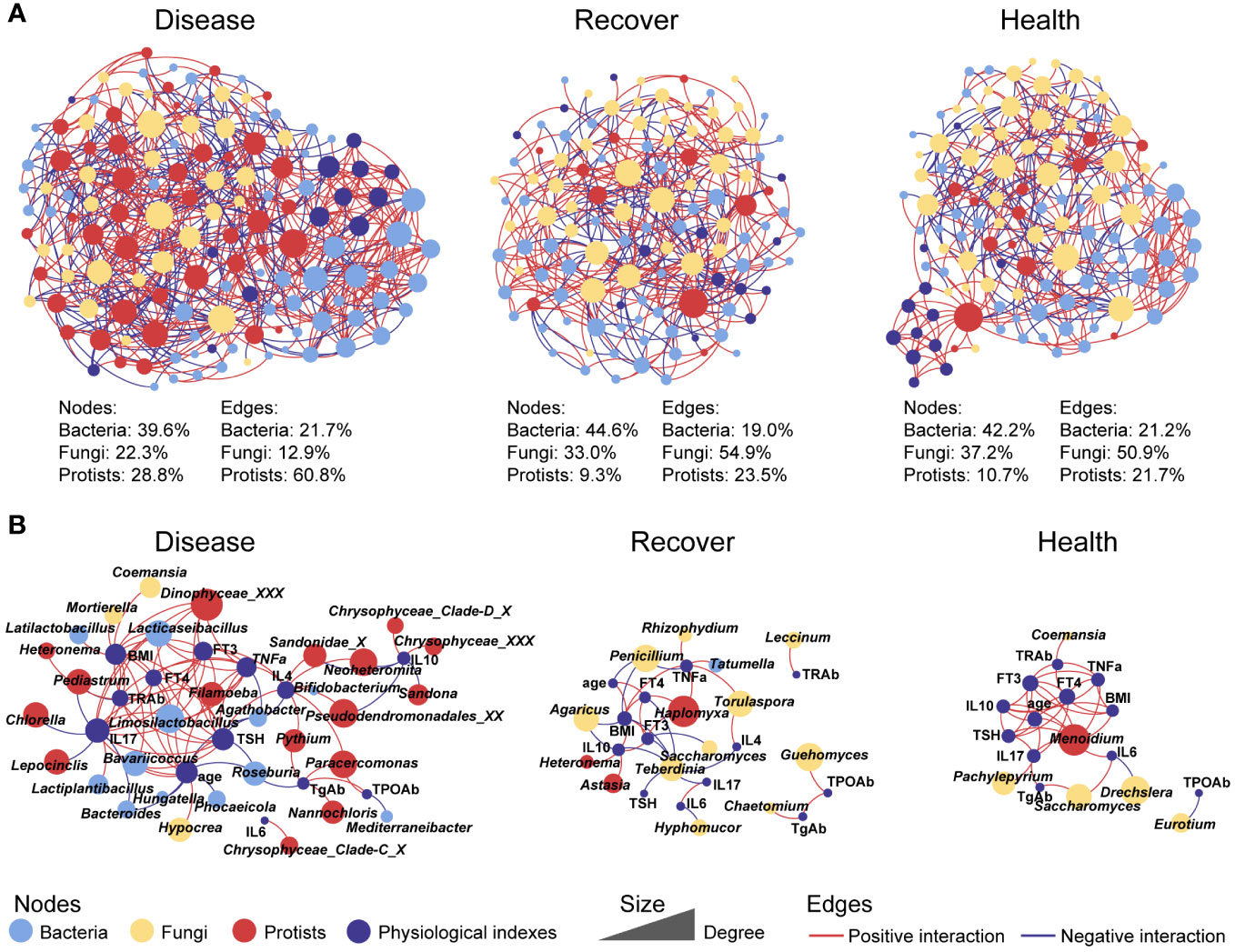
Figure 2 Co-occurrence networks between physiological indexes and all microbial kingdoms in each group. (A) Networks involving physiological indexes and all microbial taxa. (B) Networks involving physiological indexes and their associated microbial taxa.
4 Discussion
The thyroid gland influences the metabolic and immune processes in the body by producing thyroid hormones, which can lead to microbiome–thyroid homeostasis or disorder. This study explored the influence of physiological changes on gut microbial community assembly. We found that stochastic processes dominated the bacterial and fungal community assembly, while deterministic processes dominated the protistan community assembly. GD increased homogenizing dispersal processes for bacterial community assembly, and increased homogeneous selection processes for eukaryotic community assembly. This may be due to GD-induced changes in the gut environment that reduced the stability of the gut bacterial community and simultaneously altered the bacterial metabolism and reproduction rate, making it easier for fungi and protists to survive and reproduce under these specific environmental conditions (24–26). However, the medication-treated recovered patients vs. healthy controls had similar bacterial and protistan, but not fungal, community assembly processes. This concurs with the similarity of recovered patients vs. healthy controls in other disease models, although previous research mainly focused on bacterial communities (27, 28). The state of the fungal community in recovered patients may be related to the stability of this community (29). Gut bacteria are already regarded as specific diagnostic biomarkers for GD (30), and fungal and protistan biomarkers of disease progression should be identified in future research.
The functions of gut bacteria have been widely discussed in many studies (31, 32), while the functions of eukaryotes, which may explain many currently unexplained variables, have mostly been overlooked. In this study, fungi contributed more than half of the interactions among the multi-kingdom communities in the Healthy and Recovered groups. Disruption of the mycobiota can have detrimental impacts on the host immune system (33), and Leonardi et al. demonstrated that mucosal fungi in mice enhanced intestinal epithelial functions and protected against bacterial infection and intestinal injury (34). Specifically, Torulaspora and Saccharomyces, which belong to Saccharomycetales and Eurotium, contributed more interactions in Healthy and Recovered groups. Similarly, many reports have demonstrated that Saccharomyces could be used as a biotherapeutic agent owing to its anti-inflammatory, antibacterial, and immune modulatory properties (35, 36). Eurotium can also exhibit anti-colitis effects through regulating gut microbiota-dependent tryptophan metabolism (37). Therefore, fungi may play a vital role in maintaining health and avoiding GD in humans.
Additionally, protists dominated the interactions in multi-kingdom microbial communities of disease group. Predatory protozoa and algae contribute to these connections, which may be due to the disruption of the gut environment leading to an increase in bacteria, thereby enhancing the connection between protozoa and other microorganisms. Specific eukaryotic microbes in the gut ecosystem have been reported to have a role in modulating the host immune system (5). Over millions of years, eukaryotic microbes co-evolved with mammals; although there are fewer eukaryotic microbes than bacteria living in the gut, they are much larger in size and may have a disproportionate influence (5, 38). In this study, blastocystis was enriched in Healthy and Recovered groups. Blastocystis is a protistan parasite and also a common component of the healthy gut microbiome, and recent studies have reported that Blastocystis have a potentially beneficial effect on regulating host immune responses (39). Moreover, the latest report also emphasized the importance of commensal protists for regulating intestinal immunity and trans-kingdom competition (40). However, the role of protists in gut microbiome assembly in healthy humans remains mostly unrecognized. Thus, we argue that eukaryotic microbes, especially protists, represent an essential factor that should be taken into consideration when analyzing the gut microbiome.
Overall, this study highlights the intricate yet previously unexplored dynamics of gut multi-kingdom microbiome assembly in GD patients and medication-treated patients who recovered from GD. We conclude that, via physiological changes, GD increased homogenizing dispersal processes for bacterial community assembly and increased homogeneous selection processes for eukaryotic community assembly. The bacterial and protistan, but not fungal, assembly processes of the medication-treated recovered patients vs. healthy controls were similar. Furthermore, eukaryotic microbes potentially contributed more ecosystem functions than bacteria in the gut environment. This study gives brief insights into the potential contributions of eukaryotic microbes to gut and immune homeostasis in humans and their potential influence in relation to therapeutic interventions. Notably, more research into the potential benefits of eukaryotic microbes in humans will offer many exciting prospects for revealing the mechanisms of establishment and the complex traits of immune diseases.
Data availability statement
Raw sequences were deposited in the NCBI Sequence Read Archive database (https://www.ncbi.nlm.nih.gov/) with the accession number PRJNA1032235.
Ethics statement
The studies involving humans were approved by the Ethics Committee of Henan Provincial People’s Hospital. The studies were conducted in accordance with the local legislation and institutional requirements. Written informed consent for participation in this study was provided by the participants’ legal guardians/next of kin. Written informed consent was obtained from the individual(s), and minor(s)’ legal guardian/next of kin, for the publication of any potentially identifiable images or data included in this article.
Author contributions
XG: Methodology, Validation, Visualization, Writing – original draft. YL: Conceptualization, Funding acquisition, Methodology, Writing – review & editing. WX: Methodology, Visualization, Writing – review & editing. GL: Data curation, Visualization, Writing – review & editing. BX: Data curation, Investigation, Writing – review & editing. YF: Writing – review & editing. ST: Writing – review & editing. WW: Writing – review & editing. HY: Conceptualization, Funding acquisition, Writing – review & editing.
Funding
The author(s) declare financial support was received for the research, authorship, and/or publication of this article. This study was supported by the National Natural Science Foundation of China (grant numbers: 82000752, 81970705, 82270865).
Conflict of interest
The authors declare that the research was conducted in the absence of any commercial or financial relationships that could be construed as a potential conflict of interest.
Publisher’s note
All claims expressed in this article are solely those of the authors and do not necessarily represent those of their affiliated organizations, or those of the publisher, the editors and the reviewers. Any product that may be evaluated in this article, or claim that may be made by its manufacturer, is not guaranteed or endorsed by the publisher.
Supplementary material
The Supplementary Material for this article can be found online at: https://www.frontiersin.org/articles/10.3389/fimmu.2024.1334158/full#supplementary-material
References
1. Davies TF, Andersen S, Latif R, Nagayama Y, Barbesino G, Brito M, et al. Graves’ disease. Nat Rev Dis Primers. (2020) 6:1–23. doi: 10.1038/s41572-020-0184-y
2. Ishaq HM, Mohammad IS, Shahzad M, Ma C, Raza MA, Wu X, et al. Molecular alteration analysis of human gut microbial composition in graves’ disease patients. Int J Biol Sci. (2018) 14:1558–70. doi: 10.7150/IJBS.24151
3. Yan HX, An WC, Chen F, An B, Pan Y, Jin J, et al. Intestinal microbiota changes in Graves’ disease: a prospective clinical study. Biosci Rep. (2020) 40:BSR20191242. doi: 10.1042/BSR20191242
4. van Tilburg Bernardes E, Pettersen VK, Gutierrez MW, Laforest-Lapointe I, Jendzjowsky NG, Cavin JB, et al. Intestinal fungi are causally implicated in microbiome assembly and immune development in mice. Nat Commun. (2020) 11:1–16. doi: 10.1038/s41467-020-16431-1
5. Lukeš J, Stensvold CR, Jirků-Pomajbíková K, Wegener Parfrey L. Are human intestinal eukaryotes beneficial or commensals? PloS Pathog. (2015) 11:e1005039. doi: 10.1371/JOURNAL.PPAT.1005039
6. Stegen JC, Lin X, Konopka AE, Fredrickson JK. Stochastic and deterministic assembly processes in subsurface microbial communities. ISME J. (2012) 6:1653–64. doi: 10.1038/ismej.2012.22
7. Zhou J, Deng Y, Zhang P, Xue K, Liang Y, Van Nostrand JD, et al. Stochasticity, succession, and environmental perturbations in a fluidic ecosystem. Proc Natl Acad Sci U.S.A. (2014) 111:E836–45. doi: 10.1073/pnas.1324044111
8. David LA, Maurice CF, Carmody RN, Gootenberg DB, Button JE, Wolfe BE, et al. Diet rapidly and reproducibly alters the human gut microbiome. Nature. (2013) 505:559–63. doi: 10.1038/nature12820
9. Bonder MJ, Kurilshikov A, Tigchelaar EF, Mujagic Z, Imhann F, Vila AV, et al. The effect of host genetics on the gut microbiome. Nat Genet. (2016) 48:1407–12. doi: 10.1038/NG.3663
10. Forslund K, Hildebrand F, Nielsen T, Falony G, Le Chatelier E, Sunagawa S, et al. Disentangling type 2 diabetes and metformin treatment signatures in the human gut microbiota. Nature. (2015) 528:262–6. doi: 10.1038/NATURE15766
11. Mihajlovic J, Leutner M, Hausmann B, Kohl G, Schwarz J, Röver H, et al. Combined hormonal contraceptives are associated with minor changes in composition and diversity in gut microbiota of healthy women. Environ Microbiol. (2021) 23:3037–47. doi: 10.1111/1462-2920.15517
12. Furman O, Shenhav L, Sasson G, Kokou F, Honig H, Jacoby S, et al. Stochasticity constrained by deterministic effects of diet and age drive rumen microbiome assembly dynamics. Nat Commun. (2020) 11:1904. doi: 10.1038/S41467-020-15652-8
13. Lynch SV, Pedersen O. The human intestinal microbiome in health and disease. N Engl J Med. (2016) 375:2369–79. doi: 10.1056/NEJMRA1600266
14. Oliphant K, Parreira VR, Cochrane K, Allen-Vercoe E. Drivers of human gut microbial community assembly: coadaptation, determinism and stochasticity. ISME J. (2019) 13:3080–92. doi: 10.1038/s41396-019-0498-5
15. Chelius MK, Triplett EW. The diversity of archaea and bacteria in association with the roots of Zea mays L. Microb Ecol. (2001) 41:252–63. doi: 10.1007/s002480000087
16. Gardes M, Bruns TD. ITS primers with enhanced specificity for basidiomycetes - application to the identification of mycorrhizae and rusts. Mol Ecol. (1993) 2:113–8. doi: 10.1111/j.1365-294X.1993.tb00005.x
17. White T, Bruns T, Lee S, Taylor J, Innis M, Gelfand D. Amplification and direct sequencing of fungal ribosomal RNA genes for phylogenetics. PCR protocols: A guide to Methods Appl. (1990) 18:315–22. doi: 10.1016/B978-0-12-372180-8.50042-1.
18. Stoeck T, Bass D, Nebel M, Christen R, Jones MDM, Breiner HW, et al. Multiple marker parallel tag environmental DNA sequencing reveals a highly complex eukaryotic community in marine anoxic water. Mol Ecol. (2010) 19:21–31. doi: 10.1111/j.1365-294X.2009.04480.x
19. Edgar RC. UPARSE: highly accurate OTU sequences from microbial amplicon reads. Nat Methods. (2013) 10:996–8. doi: 10.1038/nmeth.2604
20. Wang Q, Garrity GM, Tiedje JM, Cole JR. Naive Bayesian classifier for rapid assignment of rRNA sequences into the new bacterial taxonomy. Appl Environ Microbiol. (2007) 73:5261–7. doi: 10.1128/AEM.00062-07
21. Guillou L, Bachar D, Audic S, Bass D, Berney C, Bittner L, et al. The Protist Ribosomal Reference database (PR2): a catalog of unicellular eukaryote Small Sub-Unit rRNA sequences with curated taxonomy. Nucleic Acids Res. (2013) 41:D597–604. doi: 10.1093/NAR/GKS1160
22. Sloan WT, Lunn M, Woodcock S, Head IM, Nee S, Curtis TP. Quantifying the roles of immigration and chance in shaping prokaryote community structure. Environ Microbiol. (2006) 8:732–40. doi: 10.1111/j.1462-2920.2005.00956.x
23. Stegen JC, Lin X, Fredrickson JK, Chen X, Kennedy DW, Murray CJ, et al. Quantifying community assembly processes and identifying features that impose them. ISME J. (2013) 7:2069–79. doi: 10.1038/ismej.2013.93
24. Lozupone CA, Stombaugh JI, Gordon JI, Jansson JK, Knight R. Diversity, stability and resilience of the human gut microbiota. Nature. (2012) 489:220–30. doi: 10.1038/nature11550
25. Fassarella M, Blaak EE, Penders J, Nauta A, Smidt H, Zoetendal EG. Gut microbiome stability and resilience: elucidating the response to perturbations in order to modulate gut health. Gut. (2021) 70:595–605. doi: 10.1136/GUTJNL-2020-321747
26. Guzzo GL, Mittinty MN, Llamas B, Andrews JM, Weyrich LS. Individuals with inflammatory bowel disease have an altered gut microbiome composition of fungi and protozoa. Microorganisms. (2022) 10:1910. doi: 10.3390/microorganisms10101910
27. Newsome RC, Gauthier J, Hernandez MC, Abraham GE, Robinson TO, Williams HB, et al. The gut microbiome of COVID-19 recovered patients returns to uninfected status in a minority-dominated United States cohort. Gut Microbes. (2021) 13:1–15. doi: 10.1080/19490976.2021.1926840
28. Cui GY, Rao BC, Zeng ZH, Wang XM, Ren T, Wang HY, et al. Characterization of oral and gut microbiome and plasma metabolomics in COVID-19 patients after 1-year follow-up. Mil Med Res. (2022) 9:1–13. doi: 10.1186/s40779-022-00387-y
29. Abstract G. Commensal fungi recapitulate the protective benefits of intestinal bacteria. Cell Host Microbe. (2017) 22:809–816.e4. doi: 10.1016/j.chom.2017.10.013
30. Zhu Q, Hou Q, Huang S, Ou Q, Huo D, Vázquez-Baeza Y, et al. Compositional and genetic alterations in Graves’ disease gut microbiome reveal specific diagnostic biomarkers. ISME J. (2021) 15:3399–411. doi: 10.1038/s41396-021-01016-7
31. Paik D, Yao L, Zhang Y, Bae S, D’Agostino GD, Zhang M, et al. Human gut bacteria produce TH17-modulating bile acid metabolites. Nature. (2022) 603:907–12. doi: 10.1038/s41586-022-04480-z
32. Su X, Zhao Y, Li Y, Ma S, Wang Z. Gut dysbiosis is associated with primary hypothyroidism with interaction on gut-thyroid axis. Clin Sci. (2020) 134:1521–35. doi: 10.1042/CS20200475
33. Iliev ID, Leonardi I. Fungal dysbiosis: immunity and interactions at mucosal barriers. Nat Rev Immunol. (2017) 17:635–46. doi: 10.1038/nri.2017.55
34. Leonardi I, Gao IH, Lin WY, Allen M, Li XV, Fiers WD, et al. Mucosal fungi promote gut barrier function and social behavior via Type 17 immunity. Cell. (2022) 185:831–846.e14. doi: 10.1016/J.CELL.2022.01.017
35. Stier H, Bischoff SC. Influence of saccharomyces boulardii CNCM I-745 on the gut-associated immune system. Clin Exp Gastroenterol. (2016) 9:269–79. doi: 10.2147/CEG.S111003
36. Abid R, Waseem H, Ali J, Ghazanfar S, Ali GM, Elasbali AM, et al. Probiotic yeast saccharomyces: back to nature to improve human health. J Fungi. (2022) 8:444. doi: 10.3390/JOF8050444
37. Zhang B, Ren D, Zhao A, Shao H, Li T, Niu P, et al. Eurotium cristatum Exhibited Anti-Colitis Effects via Modulating Gut Microbiota-Dependent Tryptophan Metabolism. J Agric Food Chem. (2022) 70:16164–75. doi: 10.1021/acs.jafc.2c05464
38. Chabé M, Lokmer A, Ségurel L. Gut protozoa: friends or foes of the human gut microbiota? Trends Parasitol. (2017) 33:925–34. doi: 10.1016/j.pt.2017.08.005
39. Deng L, Wojciech L, Gascoigne NRJ, Peng G, Tan KSW. New insights into the interactions between Blastocystis, the gut microbiota, and host immunity. PloS Pathog. (2021) 17:e1009253. doi: 10.1371/JOURNAL.PPAT.1009253
Keywords: gut microbiome, multi kingdom microbiome, community assembly, hyperthyroidism, inflammatory factors
Citation: Geng X, Liu Y, Xu W, Li G, Xue B, Feng Y, Tang S, Wei W and Yuan H (2024) Eukaryotes may play an important ecological role in the gut microbiome of Graves’ disease. Front. Immunol. 15:1334158. doi: 10.3389/fimmu.2024.1334158
Received: 06 November 2023; Accepted: 05 February 2024;
Published: 22 February 2024.
Edited by:
Maryam Dadar, Razi Vaccine and Serum Research Institute, IranReviewed by:
Jingjie Du, Cornell University, United StatesJulio Plaza-Diaz, Children’s Hospital of Eastern Ontario (CHEO), Canada
Copyright © 2024 Geng, Liu, Xu, Li, Xue, Feng, Tang, Wei and Yuan. This is an open-access article distributed under the terms of the Creative Commons Attribution License (CC BY). The use, distribution or reproduction in other forums is permitted, provided the original author(s) and the copyright owner(s) are credited and that the original publication in this journal is cited, in accordance with accepted academic practice. No use, distribution or reproduction is permitted which does not comply with these terms.
*Correspondence: Huijuan Yuan, aGp5dWFuQHp6dS5lZHUuY24=
†These authors have contributed equally to this work