- 1Institute of Dairy Science, College of Animal Sciences, Zhejiang University, Hangzhou, China
- 2Qinghai-Tibetan Plateau Animal Genetic Resource Reservation and Utilization Key Laboratory of Sichuan Province, Chengdu, China
- 3College of Animal Science and Technology, Henan University of Animal Husbandry and Economy, Zhengzhou, China
- 4Institute of Nanjiang Yellow Goat Sciences, Bazhong, Sichuan, China
- 5Key Laboratory of Molecular Animal Nutrition, Ministry of Education, Zhejiang University, Hangzhou, China
The balance of the microbiome, which is sensitive to temperature changes, plays a crucial role in maintaining overall health and reducing the risk of diseases. However, the specific mechanisms by which immunity and microbiota interact to adapt to cold stress have yet to be addressed. In this study, Nanjiang Yellow goats were chosen as a model and sampled during the cold (winter, cold stress) and warm (spring) seasons, respectively. Analyses of serum immune factors, as well as the composition of rumen and fecal microbial communities, were conducted to explore the crosstalk between microbiota and innate immunity under cold stress. Significantly increased levels of IgA (P < 0.01) were observed in the cold season compared to the warm season. Conversely, the levels of IL-2 (P = 0.02) and IL-6 (P < 0.01) diminished under cold stress. However, no significant differences were observed in IgG (P = 0.89), IgM (P = 0.42), and IL-4 (P = 0.56). While there were no significant changes in the diversity of bacterial communities between the warm and cold seasons, positive correlations between serum IgA, IL-2, IL-6 concentrations and several genera were observed. Furthermore, the weighted gene co-expression network analysis indicated that the microbiota enriched in the MEbrown module positively correlated with IgA, while the microbiota enriched in the MEblue module positively correlated with IL-2 and IL-6. The strong correlation between certain probiotics, including Alistipes, Bacteroides, Blautia, and Prevotellaceae_UCG.004, and the concentration of IL-2, and IL-6 suggests their potential role in immunomodulatory properties. This study provides valuable insights into the crosstalk between microbial communities and immune responses under the challenge of cold stress. Further studies on the immunomodulatory properties of these probiotics would contribute to the development of strategies to enhance the stress resistance of animals for improved overall health and survival.
Introduction
Emerging evidence suggests that cold stress induces inflammation, adversely affecting animal growth and development (1, 2). For instance, cold exposure impacts mammary blood flow and lactose synthesis in dairy livestock (3, 4), thereby reducing milk secretion and diminishing birth weight and survival of young animals (5). In rodents, cold stress reduces the diversity of the gut microbiota and increases the abundance of potentially pathogenic bacteria (6), suggesting a crosstalk between immunity and microbiota under cold stress. However, the mechanisms by which immunity and microbiota interact to adapt to cold exposure remain unaddressed.
There has been a growing interest in unraveling the intricate relationship between innate immunity and the profile of microbiota (7–9). These microbiota serve multifaceted functions, including defending against pathogens (10), facilitating exogenous metabolism (11), and supporting the maturation of the immune system (12), vital for their sustained survival (13). In the ruminants, maintaining a healthy-balanced microbial community is particularly vital for the reconstruction of rumen and intestinal function, exerting enduring effects on overall health (14–17). These microorganisms possess the capability to generate short-chain fatty acids by breaking down plant polysaccharides such as starch, cellulose, and hemicellulose (18, 19). These short-chain fatty acids contribute to thermogenesis and immune function (20, 21). Comprising bacteria, protozoa, fungi, and viruses, the balance of microbiota between commensal and pathogenic microorganisms is essential for maintaining animal health (22). Disruptions in the microbial community have been linked to the onset of various diseases (23–25).
The survival strategies of goats, as adaptable and widespread ruminants, are intricately linked to seasonal changes (26). Dynamic changes occur in bacterial communities in the rumen and the feces of ruminants as they experience different seasons (27). However, limited studies have investigated whether changes in microbiota influenced by ambient temperature are associated with immune responses. Since immunity increases concomitantly with changes in rumen and fecal microbiota, it may be assumed that the microbiota mediates the alterations in host immunity. Exploring the compositional differences between the bacterial communities of animals during the cold season (winter, cold stress) and warm season (spring, warm weather) may aid in identifying potential probiotics with immunomodulatory properties. Administering these potential probiotics to animals under stress could enhance their adaptation to cold stress, therefore, promoting production efficiency in extreme weather conditions. Nanjiang Yellow goats, a typical mountain-bred breed, exhibit robust adaptability to endure cold stress in Sichuan Province, China. In the current study, Nanjiang Yellow goats were selected as a model and sampled during the cold season and the warm season. We conducted analyses of serum immune factors, as well as the composition of rumen and fecal microbial communities, to explore the crosstalk between microbiota and innate immunity under cold stress.
Materials and methods
Animals and experiment design
This study was carried out following the regulations of Instructive Notions with Respect to Caring for Experimental Animals and following review and approval of the protocol by the Experimental Animal Management Committee of the Zhejiang University.
The goats were sourced from the Nanjiang Yellow Goat Original Breeding Farm located in Bazhong, China. They were housed in dedicated pens that were equipped with provisions for feeding with unrestricted access to drinking water, and all goats were in one block in the feeding room. Commercial concentrate was provided twice daily at 08:00 and 18:00 throughout the trial. The detailed nutrient composition of the commercial concentrate is available in the Supplementary Table 1. Ten healthy and well-conditioned Nanjiang Yellow goats (aged 6 months, similar body weight, male) were selected for sampling Blood, rumen, and fecal samples were collected from the goats in the cold season (at the beginning of January, average temperature: 0°C) and warm season (at end of March, average temperature:18°C). Goats with histories of disease were not included in the experiment.
Sampling and analysis
The ground and walls of the building were treated with insect repellent. Disinfection was carried out using Bromo Germaine (CAS: 7281-04-1, China Pharmaceutical Group Co., Ltd., Beijing, China). The clinical condition of the animals was assessed and recorded. All animals were found to be in good health at both samplings with no signs of diarrhea.
The collection of rumen contents and fecal followed the methods described previously (28, 29). Approximately, 25 mL of rumen contents were collected from each goat. Rumen contents were collected from each goat using oral stomach tubes, 3 hours after morning feeding. The initial 5 mL of rumen contents from each sampling was discarded to eliminate potential saliva contamination. The remaining rumen contents were filtered through four layers of cheesecloth. Fecal samples were collected by rectal stimulation. Both rumen and fecal samples were rapidly frozen in liquid nitrogen and stored until DNA isolation and analysis. The collected samples were divided into four groups: rumen contents of the cold season (CR), rumen contents of the warm season (WR), fecal of the cold season (CF), and fecal of the warm season (WF).
3 mL of blood was collected from the jugular vein of each goat, 3 hours after morning feeding and before collecting rumen contents and fecal samples. The serum was separated from the collected blood by centrifugation at 1500 × g for 15 minutes. The separated serum was transferred to microcentrifuge tubes. The serum samples were stored at -80°C until analysis. Serum immunoglobulin A (IgA, H108-1-1), immunoglobulin G (IgG, H106-1-1), immunoglobulin M (IgM, H109-1-1), interleukin-2 (IL-2, H003-1-1), interleukin-4 (IL-4, H005-1-1), and interleukin-6 (IL-6, H007-1-1) were measured. Measurements were conducted at a wavelength of 450 nm using commercial ELISA kits (Nanjing Jiancheng Biotech, Jiangsu, China). The analysis followed the manufacturer’s protocol, with readings performed using a microtiter plate reader (BioTek, USA).
16S rRNA gene sequencing
Total genomic DNA from fecal samples and rumen contents was extracted. A commercial kit (Tiangen Biotech, Beijing, China) was used for DNA extraction. Bacteria were enzymatically lysed during the DNA extraction process. DNA concentration and purity were assessed using a 1% agarose gel. Amplification of DNA was performed using the primer set 341F/806R (341F: 5’-CCTATYGGGRBGCASCAG-3’, 806R: 5’-GGACTACNNGGGTATCTAAT-3’). The primers targeted the V3-V4 region of the bacterial 16S rRNA gene. Paired-ended sequencing (2×300 bp) was conducted on the Illumina MiSeq platform. The sequencing was carried out by standard procedures (Novogene Technology Co. Ltd., Tianjin, China) (28, 30).
Processing of sequencing data
Following the methods previously described (31), raw sequencing reads from different samples were subjected to demultiplexing and quality filtering to obtain high-quality and valid data. Using QIIME2 (http://qiime.org) was utilized for bioinformatics analysis. Sequencing data from CR and WR groups were compared. The objective was to analyze differences in the rumen microbiota of goats under warm and cold-temperature conditions. Sequencing data from CF and WF groups were compared to analyze differences in the fecal microbiota among goats under warm and cold-temperature conditions. Microbiota analyses involve assessing microbial diversity, composition, and abundance in the rumen samples. Linear discriminant analysis Effect Size (LEfSe) was applied to determine differential abundance of bacterial taxa between different samples. The principal coordinate analysis (PCoA) and LEfSe analysis were performed using the Novomagic (https://magic.novogene.com).
Statistical analysis
Paired t-tests were used for the statistical analyses of serum immune factors and microbial diversity. Data were presented as mean ± SEM. Spearman correlation analysis was conducted using SPSS software (version 19, SPSS Inc., Chicago, IL, USA) to explore the relationships between immune factors and bacterial taxa. Correlation heatmaps were generated using the R program and the pheatmap package. A significance level of P < 0.05 was considered statistically significant. The weighted gene co-expression network analysis (WGCNA) was carried out using the WGCNA package in R (version 4.0.2) to investigate the relationship between immune indices and microbiota profiling with the power at 4. The different colors were used to identify different modules. The relationship of the microbial composition of positive correlation modules in WGCNA results was further explored, and the network was drawn by Cytoscape (Version 3.8.0).
Results
Higher lgA and lower IL-2 and IL-6 in goats during the cold season
Temperature changes significantly influenced the concentration of serum IgA (P < 0.01) with levels higher when goats were exposed to cold stress (Figure 1A). In comparison to the warm season, cold stress significantly reduced the serum concentrations of IL-2 (P = 0.02) and IL-6 (P < 0.01, Figures 1D, F). No significant differences in serum concentrations of IgG (P = 0.89), IgM (P = 0.42), and IL-4 (P = 0.56) were observed between the cold and warm seasons (Figures 1B, C, E).
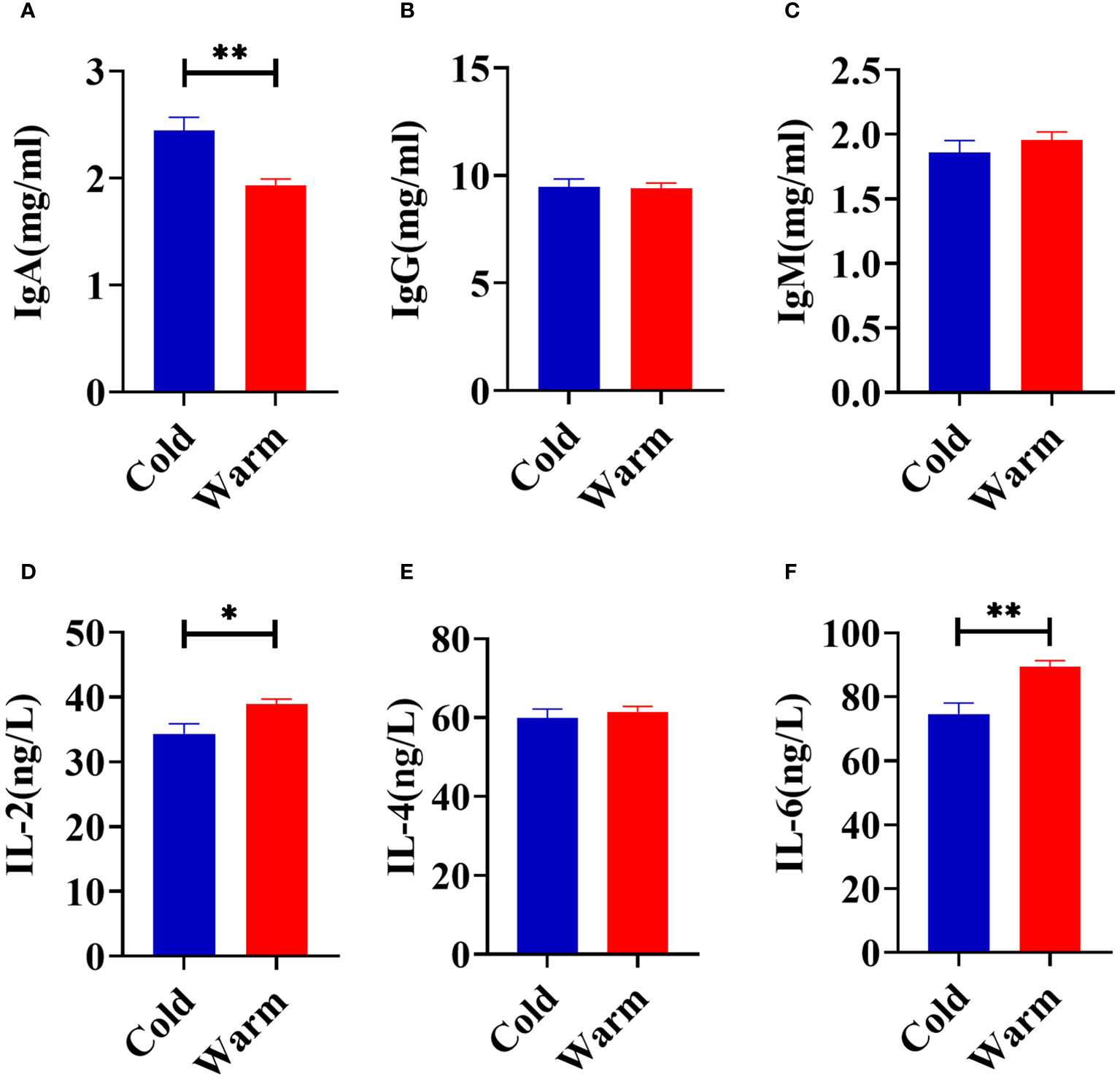
Figure 1 Comparison of serum immune factors concentrations of goats between cold and warm seasons. (A) The concentration of IgA. (B) The concentration of IgG. (C) The concentration of IgM. (D) The concentration of IL-2. (E) The concentration of IL-4. (F) The concentration of IL-6. The data were analyzed with paired t-tests, and the data were expressed as mean ± SEM. *P < 0.05 was statistically significance. **P < 0.01 was extremely significance.
Comparison of rumen microbiota analysis between cold and warm seasons
Amplification sequencing of the 16S rRNA gene in rumen samples identified 5103 operational taxonomic units (OTUs, Supplementary Table 2). After removing OTUs annotated as Archaea and unannotated OTUs, there were 5081 OTUs annotated as Bacteria. The rarefaction curves (Supplementary Figure 1A) gradually flattened out, indicating an even distribution of species and a reasonable amount of sequencing data for subsequent analysis. The Venn analysis showed that the CR and WR groups shared 2441 OTUs, and 1392 and 1114 OTUs were uniquely detected, respectively (Figure 2A). PCoA of OTUs showed a clear clustering of samples by temperature variation (PCoA1 = 51.54%, PCoA2 = 9.85%, Figure 2B, Supplementary Figure 1B). However, there were no significant differences in Chao1, Simpson, and Shannon indices between the two groups, indicating that temperature changes did not significantly affect microbial species diversity in the rumen (Supplementary Figure 1C).
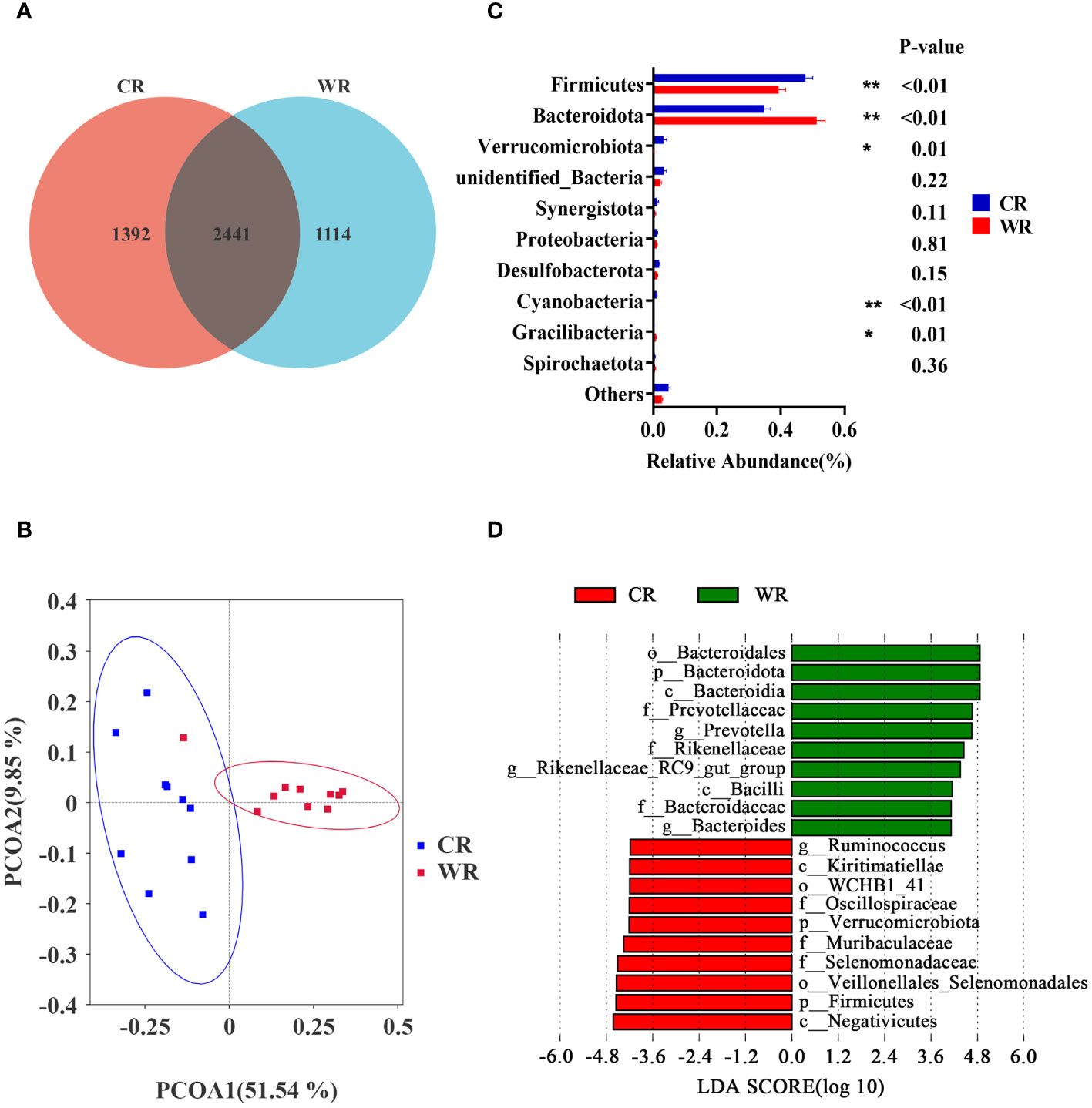
Figure 2 Comparison of rumen microbiota analysis between cold and warm seasons. (A) OTU Venn Diagram. (B) Principal coordinate analysis (PCoA) of microbial-based on weighted UniFrac. (C) Relative abundance of top10 phyla, *P<0.05, **P<0.01. (D) The bar chart shows the LDA scores of goats. LDA score > 4. CR: rumen of goats of the cold season. WR: rumen of goats of the warm season.
Based on the data at the phylum level, the dominant phyla in the CR and WR groups were Firmicutes and Bacteroidota. As shown in Figure 2C, the CR group exhibited higher levels of Firmicutes (P< 0.01), Verrucomicrobiota (P = 0.01), and Cyanobacteria (P < 0.01) compared with the WR group. In contrast, the CR group had lower levels of Bacteroidota (P < 0.01) and Gracilibacteria (P = 0.01) compared with the WR group. To determine the differences in abundance of bacterial taxa between CR and WR, LEfSe were employed with Linear Discriminant Analysis (LDA) score > 4 (Figure 2D). The WR group displayed a higher abundance of g_Prevotella, g_Rikenellaceae_RC9_gut_group, and g_Bacteroides, while the CR group exhibited a higher abundance of g_Ruminococcus.
Comparison of fecal microbiota analysis between cold and warm seasons
Amplification sequencing of the 16S rRNA gene in fecal samples identified 5005 operational taxonomic units (OTUs, Supplementary Table 3). After removing OTUs annotated as Archaea and unannotated OTUs, there were 4980 operational taxonomic units annotated as Bacteria. The rarefaction curves gradually leveled off (Supplementary Figure 1D). The Venn analysis showed that the CF and WF groups shared 2723 OTUs, and 1367 and 749 OTUs were uniquely detected, respectively (Figure 3A). PCoA analysis of OTUs showed a clear clustering of samples by temperature variation (PCoA1 = 52.24%, PCoA2 = 25.18%, Figure 3B, and Supplementary Figure 1E). However, there was no significant difference in the Chao1, Simpson, and Shannon indices between the two groups (Supplementary Figure 1F).
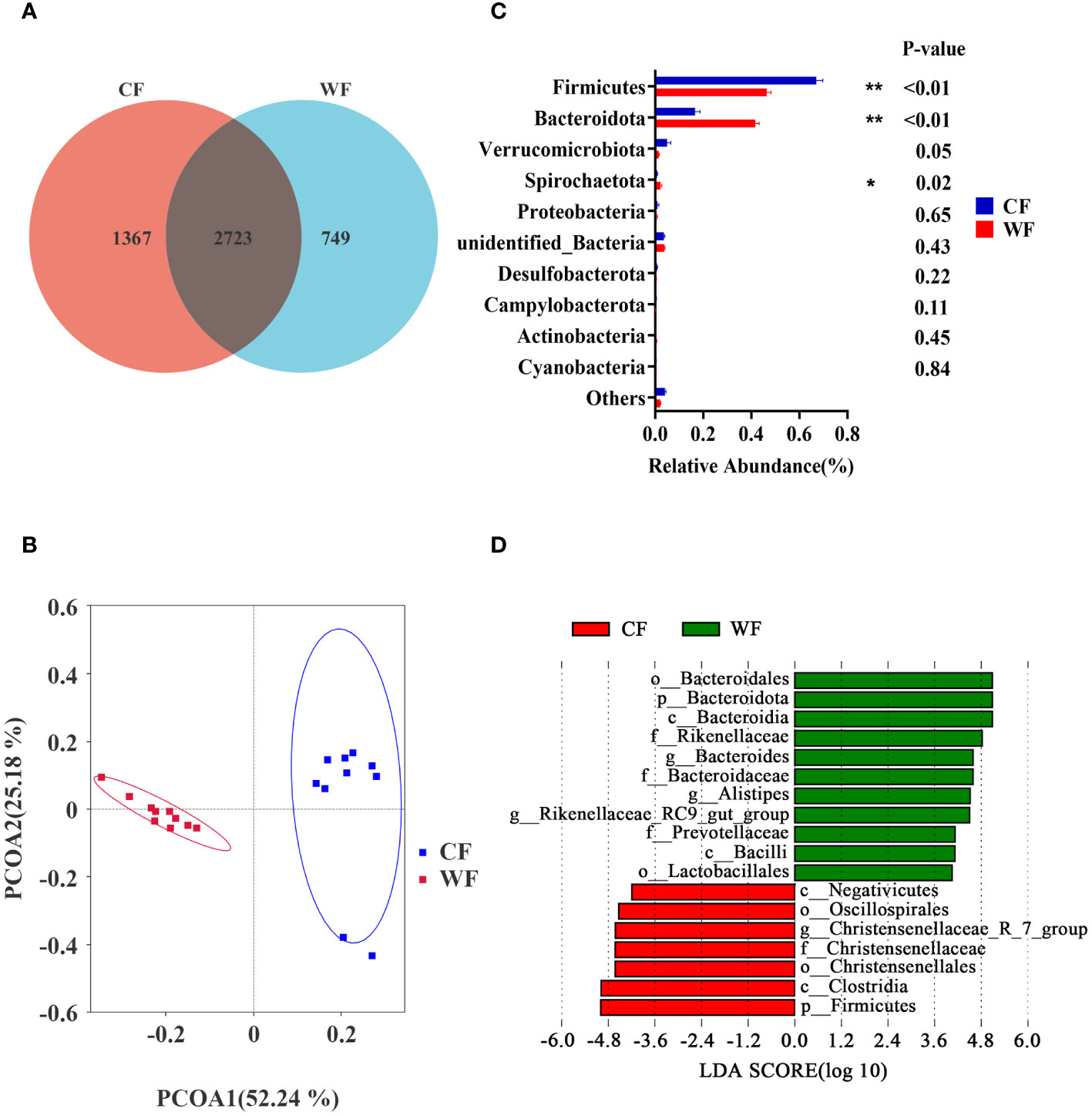
Figure 3 Comparison of fecal microbiota analysis between cold and warm seasons. (A) OTU Venn Diagram. (B) Principal coordinate analysis (PCoA) of microbial-based on weighted UniFrac. (C) Relative abundance of top10 phyla, *P<0.05, **P<0.01. (D) The bar chart shows the LDA scores of goats. LDA score > 4. CF: fecal of goats of the cold season. WF: fecal of goats of the warm season.
The Firmicutes and Bacteroidota are the main phyla shared by CF and WF groups. As shown in Figure 3C, the CF group had a higher abundance of Firmicutes (P < 0.01) compared to the WF group. Additionally, the CF group had lower levels of Bacteroidota (P < 0.01) and Spirochaetota (P = 0.02) compared with the MF group. The LEfSe analysis, with LDA score > 4, showed that g_Bacteroides, g_Alistipes, and g_Rikenellaceae_RC9_gut_group were more abundant in the WF, while g_ Christensenellaceae_R_7_group was more abundant in the CF group (Figure 3D).
Alterations of rumen-fecal microbiota under cold stress are associated with concentrations of immune factors
To determine the association between feces-rumen bacteria and concentration of IgA, IgG, IgM, IL-2, IL-4, and IL-6, spearman correlation analyses were conducted at the genera level. In the rumen, correlation analysis revealed a positive correlation between IgA and Lachnospiraceae_NK3A20_group (P = 0.02, R = 0.53). It was also observed that IL-2 showed a positive correlation with four bacterial genera, including UCG.001 (P = 0.04, R = 0.47), Alistipes (P = 0.03, R = 0.49), Blautia (P = 0.01, R = 0.55), and Bacteroides (P < 0.01, R = 0.60). It was found that eight bacterial genera were positively associated with IL-6, including Rikenellaceae_RC9_gut_group (P = 0.03, R = 0.48), Anaeroplasma (P = 0.02, R = 0.50), Romboutsia (P = 0.04, R = 0.47), UCG.001 (P < 0.01, R = 0.58), Alistipes (P = 0.05, R = 0.45), Listeria (P = 0.01, R = 0.55), Bacteroides (P < 0.01, R = 0.59), and Lactococcus (P < 0.01, R = 0.63). Five genera in the rumen showed a negative correlation with serum IgA, including Anaeroplasma (P = 0.02, R = -0.53), Romboutsia (P = 0.04, R = -0.46), UCG.001 (P < 0.01, R = -0.58), Alistipes (P = 0.02, R = -0.52), and Bacteroides (P = 0.05, R = -0.45). Four genera in the rumen were negatively correlated with IL-2, including Ruminococcus (P = 0.02, R = -0.51), V9D2013_group (P = 0.02, R = -0.53), Alloprevotella (P = 0.02, R = -0.52), and Candidatus_Saccharimonas (P = 0.02, R = -0.52). Ruminococcus (P = 0.03, R = -0.49), V9D2013_group (P < 0.01, R = -0.57), and Alloprevotella (P < 0.01, R = -0.71) were negatively correlated with IL-6 (Figure 4A).
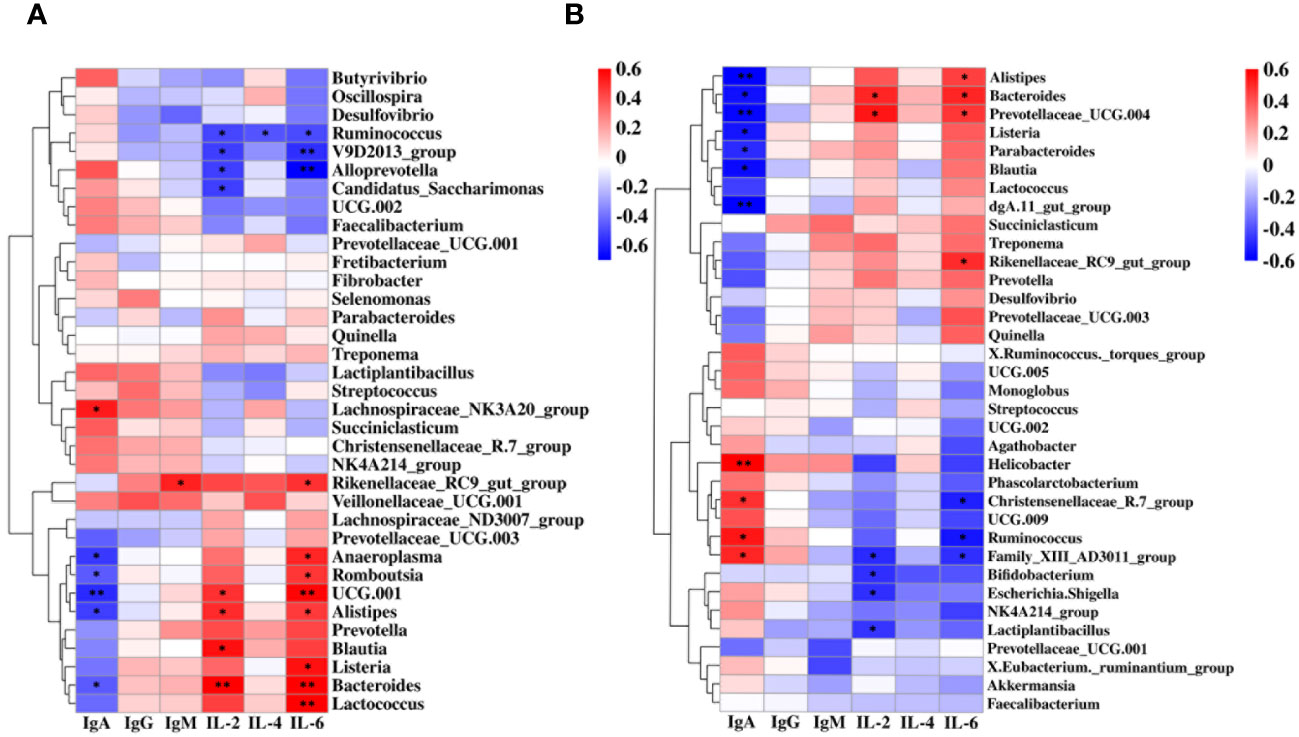
Figure 4 Correlation between the immune factors and the rumen and fecal bacteria at the genus level. (A) Spearman correlation between rumen samples (CR, WR) and serum immune factors. (B) Spearman correlation between fecal samples (CF, WF) and serum immune factors. *P < 0.05, **P < 0.01.
Four genera were also positively associated with IgA in feces, including Helicobacter (P < 0.01, R = 0.63), Christensenellaceae_R.7_group (P = 0.03, R = 0.49), Ruminococcus (P = 0.01, R = 0.54), and Family_XIII_AD3011_group (P = 0.02, R = 0.51). Bacteroides (P = 0.02, R = 0.51) and Prevotellaceae_UCG.004 (P = 0.01, R = 0.54) were positively correlated with IL-2. While Alistipes (P = 0.05, R = 0.45), Bacteroides (P = 0.02, R = 0.51), Prevotellaceae_UCG.004 (P = 0.03, R = 0.48), and Rikenellaceae_RC9_gut_group (P = 0.03, R = 0.50) were positively correlated with IL-6. In addition, seven genera were negatively associated with IgA in feces, including Alistipes (P < 0.01, R = -0.62), Bacteroides (P = 0.01, R = -0.54), Prevotellaceae_UCG.004 (P < 0.01, R = -0.57), Listeria (P = 0.02, R = -0.53), Parabacteroides (P = 0.03, R = -0.49), Blautia (P = 0.01, R = -0.55), and dgA.11_gut_group (P < 0.01, R = -0.58). Four genera were negatively correlated with IL-2, including Family_XIII_ AD3011_group (P = 0.03, R = -0.48), Bifidobacterium (P = 0.04, R = -0.47), Escherichia.Shigella (P = 0.03, R = -0.48), and Lactiplantibacillus (P = 0.04, R = -0.45). Christensenellaceae_R.7_group (P = 0.02, R = -0.50), Ruminococcus (P = 0.01, R = -0.54), and Family_XIII_AD3011_group (P = 0.03, R = -0.48) were negatively correlated with IL-6 (Figure 4B).
Core microbiota is associated with the alterations of immune factors under cold stress
To identify the core microbiota altering the concentrations of immune factors, we further performed the WGCNA analysis. A total of 11 relevant microbiota modules were identified (Figure 5A). Among them, the MEbrown module was significantly associated with IgA (P = 0.01, R = 0.39). While MEBlue module was significantly correlated with IgA (P < 0.01, R = -0.56), IL-2 (P < 0.01, R = 0.46), and IL-6 (P < 0.01, R = 0.60). The network exported from the MEbrown module showed that Succiniclasticum, Lachnospiraceae_NK3A20_group, Anaerovorax, Pyramidobacter, and unidentified_Lachnospiraceae_2 was the core microbiota in the MEbrown module (Figure 5B). Networks derived from the MEblue module showed that UCG-005, Monoglobus, UCG-009, Lachnospiraceae_NK4A136_group, and Mailhella were the central microbiota in the MEblue module (Figure 5C).
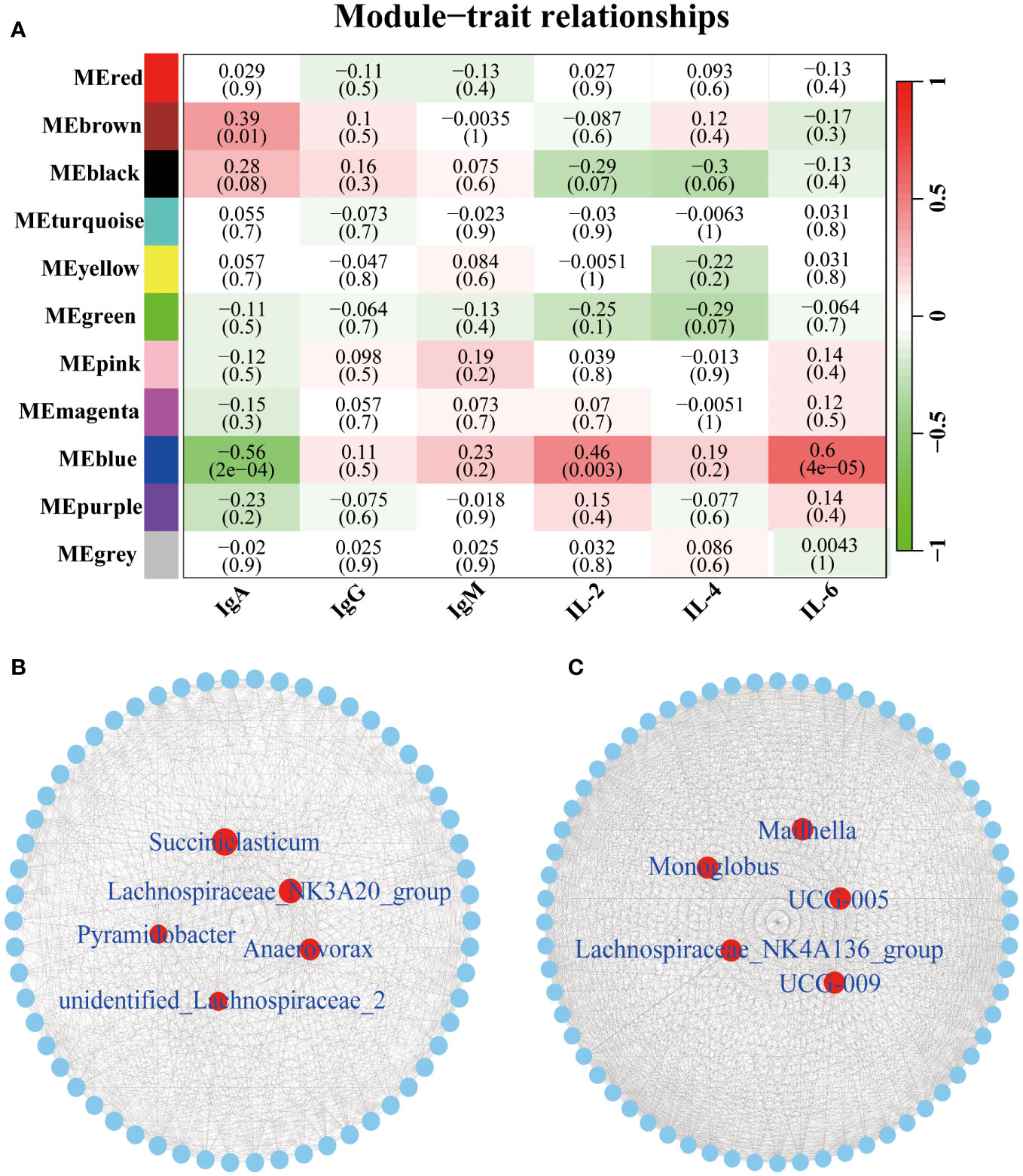
Figure 5 Correlation between the serum immune factors and abundance of the microbiome in rumen and fecal at the genus level. The correlated module was analyzed using a weighted gene co-expression network analysis (WGCNA). Correlation networks were generated using Spearman’s rank correlation coefficients and visualized using the Cytoscape. (A) The heatmap of the WGCNA module. (B) Microbiome interaction network and core microbes in the MEbrown module. Microbiota that make up the network are listed in the Supplementary Table 4. (C) Microbiome interaction network and core microbes in the MEblue module. Microbiota that make up the network are listed in the Supplementary Table 5.
Discussion
The stress resistance of organisms is of great significance to their survival. Young animals, characterized by their immature immune systems, often display heightened susceptibility to challenges posed by cold stress. This susceptibility can lead to microbiome imbalances, making young animals an ideal model to study the crosstalk between microbial profiles and host immune responses (32, 33). In the current study, we measured the profiles of rumen and feces microbiota to investigate their relationship with innate immunity in goats. Notable shifts in microbial distribution and concentrations of IgA were observed during the transition from cold to warm seasons. These findings underscore a crosstalk between microbiota compositions and innate immunity under cold stress in goats.
In order to maintain overall health, the immune system of ruminants actively combats pathogenic microbiota by secreting immunoglobulins (34, 35). The concentrations of these immunoglobulins in serum serve as direct indicators of resistance to external pathogenic microbiota (36). IgA is crucial for mucosal immunity and helps protect the body from infections (37). In the current study, the elevated concentrations of IgA in goats under cold stress suggest an active immune response. IL-2 is essential for the activation and growth of T cells, while IL-6 is involved in regulating the immune response (38, 39). The lower levels of IL-2 and IL-6 observed during the cold season suggest a compromised immunity. These observations are consistent with the increased susceptibility of goats to diseases during colder seasons (40). Understanding these immune responses is crucial for implementing strategies to support the health and well-being of ruminants, particularly during challenging environmental conditions.
The study revealed that the diversity of the rumen microbial community showed no significant difference between the cold and warm seasons, which could be attributed to the ongoing maturation of the rumen in young goats. Despite this, there were notable alterations in the compositions of the rumen microbiota. Consistent with previous findings, the dominant phyla in the rumen microbial community during both seasons were Firmicutes and Bacteroidota (29). Interestingly, there was a heightened abundance of Verrucomicrobiota in the cold season, aligning with its role in polysaccharide degradation, crucial for meeting the energy requirements of the host (41, 42). The study also observed variations in the abundance of disease-protection-associated genera, such as Prevotella (43) and Bacteroides (44), with higher prevalence in the rumen during the warm season, implying heightened immunity. This was confirmed by positive correlations between the abundance of four genera in the rumen and IL-2 concentrations, as well as the positive correlations between the abundance of eight genera and IL-6 concentrations. Among these genera, Alistipes (45), Bacteroides (44), and Blautia (46), at least, have associations with inflammation and disease protection. This suggests that the modulation of these microbiota could potentially enhance host immunity under cold stress.
The diversity of gut microbes has emerged as a novel marker for evaluating gut health and metabolic capacity (47). Interestingly, we found no significant differences in gut microbiome diversity between the cold and warm seasons, suggesting a certain resilience of fecal microbes to variations in ambient temperature. Similar to the data of rumen, the dominant phyla in the feces during both seasons were Firmicutes and Bacteroidota, known for their pivotal roles in carbohydrate and protein metabolism (48). Notably, there was a lower abundance of Alistipes and Bacteroides in the feces under cold stress, implying a diminishing immune response at this stage. Ruminococcus, positive correlation with IgA concentrations, plays a role in the degradation of complex polysaccharides, converting them into nutrients for the host (49). Among the six genera positively correlated with IL-2 and IL-6 concentrations, Alistipes, Bacteroides, and Prevotellaceae_UCG.004 were associated with increasing antioxidant performance in sheep (50). These findings highlight a crosstalk between rumen and feces microbiota and the immune response in goats under cold stress.
The development of additives with immunomodulatory properties is proposed as a valuable strategy to enhance the production efficiency of animals under cold stress (51). positive correlation between the MEbrown module and IgA is supported by the dominance of Succiniclasticum (52), Lachnospiraceae_NK3A20_group (53), and Pyramidobacter (54), which are associated with nutrient metabolism. This aligns with the concept of increased energy metabolism and nutrient demands in goats during cold stress. Additionally, the MEblue module, particularly the presence of Lachnospiraceae_NK4A136_group, is linked to the production of short-chain fatty acids, known to improve the intestinal epithelial barrier and inhibit inflammation (55). This group exhibits a positive correlation with IL-2 and IL-6. Collectively, these findings suggest that microbiota associated with immunity plays a pivotal role in maintaining the health of goats under cold stress. However, it is emphasized that further studies are needed to comprehensively evaluate the immune-related bacteria identified in this study. The potential development of additives with immunomodulatory properties could prove beneficial to fortify the immunity of animals and enhance their ability to withstand the challenges posed by cold stress.
Conclusion
The study comprehensively investigated the correlation between rumen-fecal microbiota and the immune response in goats under cold stress. Notably, we observed a decrease in IL-2 and IL-6 and an increase in IgA during cold stress. While acknowledging that measuring specific antibodies against bacteria would provide a more nuanced understanding of the host immune response, our data identified eight genera in the rumen and four genera in the feces that positively correlated with changes in immune factors. The positive correlations between certain probiotics, including Alistipes, Bacteroides, Blautia, and Prevotellaceae_UCG.004, and IL-2, and IL-6 suggest their potential role in immunomodulatory properties. However, further experiments are necessary to elucidate the mechanisms that enhance tolerance to cold stress. Collectively, our study underscores the crosstalk between rumen-fecal microbiota and innate immune responses under cold stress in goats. Identifying these microbiotas with immunomodulatory properties is crucial for developing strategies to enhance the production efficiency of animals facing cold stress.
Data availability statement
The datasets presented in this study can be found in online repositories. The names of the repository/repositories and accession number(s) can be found below: https://www.ncbi.nlm.nih.gov/, PRJNA1050866.
Ethics statement
The animal study was approved by The Experimental Animal Management Committee of the Zhejiang University. The study was conducted in accordance with the local legislation and institutional requirements.
Author contributions
TL: Data curation, Project administration, Software, Writing – original draft. JZ: Project administration, Writing – review & editing. KL: Data curation, Software, Writing – review & editing. YL: Project administration, Software, Writing – review & editing. JL: Project administration, Software, Writing – review & editing. YC: Project administration, Software, Writing – review & editing. HS: Funding acquisition, Writing – review & editing, Conceptualization, Project administration, Supervision.
Funding
The author(s) declare financial support was received for the research, authorship, and/or publication of this article. This study was jointly supported by Key R&D program of Zhejiang Province (2022C04017) and Scientific Research Fund of Zhejiang University (XY2022002).
Acknowledgments
The authors thank the owners and staff of Nanjiang Yellow Goat Original Breeding Farm (Bazhong, China) for allowing the use of their goats in this experiment and their kind help for the sample collection.
Conflict of interest
The authors declare that the research was conducted in the absence of any commercial or financial relationships that could be construed as a potential conflict of interest.
Publisher’s note
All claims expressed in this article are solely those of the authors and do not necessarily represent those of their affiliated organizations, or those of the publisher, the editors and the reviewers. Any product that may be evaluated in this article, or claim that may be made by its manufacturer, is not guaranteed or endorsed by the publisher.
Supplementary material
The Supplementary Material for this article can be found online at: https://www.frontiersin.org/articles/10.3389/fimmu.2024.1363664/full#supplementary-material
Supplementary Table | Nutrient compositions of the commercial concentrate for goats.
Supplementary File 1 | OTUs of rumen samples.
Supplementary File 2 | OTUs of fecal samples.
Supplementary File 3 | The remaining microbiota that makes up the network of the MEbrown module.
Supplementary File 4 | The remaining microbiota that makes up the network of the MEblue module.
Supplementary Figure 1 | Rarefaction curves (A), PCoA based on unweighted UniFrac distances (B), and alpha index (Chao1, Simpson, and Shannon) (C) of CR and WR. Rarefaction curves (D), PCoA based on unweighted UniFrac distances (E), and alpha index (Chao1, Simpson, and Shannon) (F) of CF and WF. CR: rumen of goats of the cold season. WR: rumen of goats of the warm season. CF: fecal of goats of the cold season. WF: fecal of goats of the warm season.
References
1. Toghiani S, Hay E, Roberts A, Rekaya R. Impact of cold stress on birth and weaning weight in a composite beef cattle breed. Livest Sci. (2020) 236:104053. doi: 10.1016/j.livsci.2020.104053
2. White RR, Miller PS, Hanigan MD. Evaluating equations estimating change in swine feed intake during heat and cold stress. J Anim Sci. (2015) 93:5395–410. doi: 10.2527/jas.2015-9220
3. Thompson GE, Thomson EM. Effect of cold-exposure on mammary circulation, oxygen-consumption and milk secretion in goat. J Physiol. (1977) 272:187–96. doi: 10.1113/jphysiol.1977.sp012040
4. Faulkner A, Thomson EM, Bassett JM, Thomson GE. Cold-exposure and mammary glucose-metabolism in the lactating goat. Brit J Nutr. (1980) 43:163–70. doi: 10.1079/Bjn19800075
5. Luo NJ, Wang J, Hu Y, Zhao ZQ, Zhao YJ, Chen XC. Cold and heat climatic variations reduce indigenous goat birth weight and enhance pre-weaning mortality in subtropical monsoon region of China. Trop Anim Health Pro. (2020) 52:1385–94. doi: 10.1007/s11250-019-02142-3
6. Wang B, Liu JT, Lei RY, Xue BD, Li YL, Tian XY, et al. Cold exposure, gut microbiota, and hypertension: a mechanistic study. Sci Total Environ. (2022) 833:155199. doi: 10.1016/j.scitotenv.2022.155199
7. Nicholson JK, Holmes E, Kinross J, Burcelin R, Gibson G, Jia W, et al. Host-gut microbiota metabolic interactions. Science. (2012) 336:1262–7. doi: 10.1126/science.1223813
8. Fan Y, Pedersen O. Gut microbiota in human metabolic health and disease. Nat Rev Microbiol. (2021) 19:55–71. doi: 10.1038/s41579-020-0433-9
9. Lin LM, Xie F, Sun DM, Liu JH, Zhu WY, Mao SY. Ruminal microbiome-host crosstalk stimulates the development of the ruminal epithelium in a lamb model. Microbiome. (2019) 7:83. doi: 10.1186/s40168-019-0701-y
10. Buffie CG, Bucci V, Stein RR, McKenney PT, Ling LL, Gobourne A, et al. Precision microbiome reconstitution restores bile acid mediated resistance to clostridium difficile. Nature. (2015) 517:205–U7. doi: 10.1038/nature13828
11. Clarke G, Sandhu KV, Griffin BT, Dinan TG, Cryan JF, Hyland NP. Gut reactions: breaking down xenobiotic-microbiome interactions. Pharmacol Rev. (2019) 71:198–224. doi: 10.1124/pr.118.015768
12. Atarashi K, Tanoue T, Oshima K, Suda W, Nagano Y, Nishikawa H, et al. Treg induction by a rationally selected mixture of clostridia strains from the human microbiota. Nature. (2013) 500:232–6. doi: 10.1038/nature12331
13. Liu X, Sha YZ, Dingkao R, Zhang W, Lv WB, Wei H, et al. Interactions between rumen microbes, vfas, and host genes regulate nutrient absorption and epithelial barrier function during cold season nutritional stress in tibetan sheep. Front Microbiol. (2020) 11:593062. doi: 10.3389/fmicb.2020.593062
14. Zeineldin M, Barakat R, Elolimy A, Salem AZM, Elghandour MMY, Monroy JC. Synergetic action between the rumen microbiota and bovine health. Microb Pathog. (2018) 124:106–15. doi: 10.1016/j.micpath.2018.08.038
15. Zhuang YM, Chai JM, Cui K, Bi YL, Diao QY, Huang WQ, et al. Longitudinal investigation of the gut microbiota in goat kids from birth to postweaning. Microorganisms. (2020) 8:1111. doi: 10.3390/microorganisms8081111
16. Yáñez-Ruiz DR, Abecia L, Newbold CJ. Manipulating rumen microbiome and fermentation through interventions during early life: a review. Front Microbiol. (2015) 6:1133. doi: 10.3389/fmicb.2015.01133
17. Palma-Hidalgo JM, Jiménez E, Popova M, Morgavi DP, Martín-García AI, Yáñez-Ruiz DR, et al. Inoculation with rumen fluid in early life accelerates the rumen microbial development and favours the weaning process in goats. Anim Microbiome. (2021) 3:11. doi: 10.1186/s42523-021-00073-9
18. Chen L, Qin Q, Jiang Y, Wang K, Lin ZS, Li ZP, et al. Large-scale ruminant genome sequencing provides insights into their evolution and distinct traits. Science. (2019) 364:eaav6202. doi: 10.1126/science.aav6202
19. Gharechahi J, Vahidi MF, Sharifi G, Ariaeenejad S, Ding XZ, Han JL, et al. Lignocellulose degradation by rumen bacterial communities: new insights from metagenome analyses. Environ Res. (2023) 229:115925. doi: 10.1016/j.envres.2023.115925
20. Canfora EE, Jocken JW, Blaak EE. Short-chain fatty acids in control of body weight and insulin sensitivity. Nat Rev Endocrinol. (2015) 11:577–91. doi: 10.1038/nrendo.2015.128
21. Gonçalves P, Araújo JR, Di Santo JP. A cross-talk between microbiota-derived short-chain fatty acids and the host mucosal immune system regulates intestinal homeostasis and inflammatory bowel disease. Inflamm Bowel Dis. (2018) 24:558–72. doi: 10.1093/ibd/izx029
22. Dominguez-Bello MG, Godoy-Vitorino F, Knight R, Blaser MJ. Role of the microbiome in human development. Gut. (2019) 68:1108–14. doi: 10.1136/gutjnl-2018-317503
23. Shi N, Li N, Duan XW, Niu HT. Interaction between the gut microbiome and mucosal immune system. Mil Med Res. (2017) 4:14. doi: 10.1186/s40779-017-0122-9
24. Han ZQ, Li K, Shahzad M, Zhang H, Luo HQ, Qiu G, et al. Analysis of the intestinal microbial community in healthy and diarrheal perinatal yaks by high-throughput sequencing. Microb Pathog. (2017) 111:60–70. doi: 10.1016/j.micpath.2017.08.025
25. Lavelle A, Sokol H. Gut microbiota-derived metabolites as key actors in inflammatory bowel disease. Nat Rev Gastroenterol Hepatol. (2020) 17:223–37. doi: 10.1038/s41575-019-0258-z
26. Nair MRR, Sejian V, Silpa M, Fonsêca VFC, Costa CCD, Devaraj C, et al. Goat as the ideal climate-resilient animal model in tropical environment: revisiting advantages over other livestock species. Int J Biometeorol. (2021) 65:2229–40. doi: 10.1007/s00484-021-02179-w
27. Huang XD, Mi JD, Denman SE, Basangwangdui, Pingcuozhandui, Zhang Q, et al. Changes in rumen microbial community composition in yak in response to seasonal variations. J Appl Microbiol. (2022) 132:1652–65. doi: 10.1111/jam.15322
28. Liao RR, Xie XH, Lv YH, Dai JJ, Lin YX, Zhu LH. Ages of weaning influence the gut microbiota diversity and function in chongming white goats. Appl Microbiol Biot. (2021) 105:3649–58. doi: 10.1007/s00253-021-11301-2
29. Wang LZ, Xu Q, Kong FL, Yang YD, Wu D, Mishra S, et al. Exploring the goat rumen microbiome from seven days to two years. PloS One. (2016) 11:e0154354. doi: 10.1371/journal.pone.0154354
30. Fan QS, Wanapat M, Hou FJ. Chemical composition of milk and rumen microbiome diversity of yak, impacting by herbage grown at different phenological periods on the qinghai-tibet plateau. Animals-Basel. (2020) 10:1030. doi: 10.3390/ani10061030
31. Zhong YF, Xue MY, Sun HZ, Valencak TG, Guan LL, Liu JX. Rumen and hindgut bacteria are potential indicators for mastitis of mid-lactating holstein dairy cows. Microorganisms. (2020) 8:2042. doi: 10.3390/microorganisms8122042
32. Zhang XY, Sukhchuluun G, Bo TB, Chi QS, Yang JJ, Chen B, et al. Huddling remodels gut microbiota to reduce energy requirements in a small mammal species during cold exposure. Microbiome. (2018) 6:103. doi: 10.1186/s40168-018-0473-9
33. Yoo JY, Groer M, Dutra SVO, Sarkar A, McSkimming DI. Gut microbiota and immune system interactions. Microorganisms. (2020) 8:1587. doi: 10.3390/microorganisms8101587
34. Schroeder HW, Cavacini L. Structure and function of immunoglobulins. J Allergy Clin Immunol. (2010) 125:S41–52. doi: 10.1016/j.jaci.2009.09.046
35. Corthésy B. Multi-faceted functions of secretory iga at mucosal surfaces. Front Immunol. (2013) 4:185. doi: 10.3389/fimmu.2013.00185
36. McKee MD, Fichera A, Nishimura MI. T cell immunotherapy. Front Biosci. (2007) 12:919–32. doi: 10.2741/2114
37. Bunker JJ, Bendelac A. Iga responses to microbiota. Immunity. (2018) 49:211–24. doi: 10.1016/j.immuni.2018.08.011
38. Hagenstein J, Melderis S, Nosko A, Warkotsch MT, Richter JV, Ramcke T, et al. A novel role for il-6 receptor classic signaling: induction of rorγt+foxp3+ tregs with enhanced suppressive capacity. J Am Soc Nephrol. (2019) 30:1438–52. doi: 10.1681/Asn.2019020118
39. Niederlova V, Tsyklauri O, Kovar M, Stepanek O. Il-2-driven cd8+t cell phenotypes: implications for immunotherapy. Trends Immunol. (2023) 44:890–901. doi: 10.1016/j.it.2023.09.003
40. Doubek J, Slosárková S, Fleischer P, Malá G, Skrivánek M. Metabolic and hormonal profiles of potentiated cold stress in lambs during early postnatal period. Czech J Anim Sci. (2003) 48:403–11. doi: 10.1080/00039420310001607743
41. Chevalier C, Stojanovic O, Colin DJ, Suarez-Zamorano N, Tarallo V, Veyrat-Durebex C, et al. Gut microbiota orchestrates energy homeostasis during cold. Cell. (2015) 163:1360–74. doi: 10.1016/j.cell.2015.11.004
42. Becken B, Davey L, Middleton DR, Mueller KD, Sharma A, Holmes ZC, et al. Genotypic and phenotypic diversity among human isolates of Akkermansia muciniphila. mBio. (2021) 12:e00478–21. doi: 10.1128/mBio.00478-21
43. Larsen JM. The immune response to prevotella bacteria in chronic inflammatory disease. Immunology. (2017) 151:363–74. doi: 10.1111/imm.12760
44. Zafar H, Saier MH. Gut bacteroides species in health and disease. Gut Microbes. (2021) 13:1–20. doi: 10.1080/19490976.2020.1848158
45. Parker BJ, Wearsch PA, Veloo ACM, Rodriguez-Palacios A. The genus alistipes: gut bacteria with emerging implications to inflammation, cancer, and mental health. Front Immunol. (2020) 11:906. doi: 10.3389/fimmu.2020.00906
46. Liu XM, Mao BY, Gu JY, Wu JY, Cui SM, Wang G, et al. Blautia-a new functional genus with potential probiotic properties? Gut Microbes. (2021) 13:1–21. doi: 10.1080/19490976.2021.1875796
47. Clarke SF, Murphy EF, O'Sullivan O, Lucey AJ, Humphreys M, Hogan A, et al. Exercise and associated dietary extremes impact on gut microbial diversity. Gut. (2014) 63:1913–20. doi: 10.1136/gutjnl-2013-306541
48. Guo JZ, Li PF, Zhang K, Zhang L, Wang XL, Li L, et al. Distinct stage changes in early-life colonization and acquisition of the gut microbiota and its correlations with volatile fatty acids in goat kids. Front Microbiol. (2020) 11:584742. doi: 10.3389/fmicb.2020.584742
49. La Reau AJ, Suen G. The ruminococci: key symbionts of the gut ecosystem. J Microbiol. (2018) 56:199–208. doi: 10.1007/s12275-018-8024-4
50. Li C, Chen N, Zhang XX, Shahzad K, Qi RX, Zhang ZB, et al. Mixed silage with chinese cabbage waste enhances antioxidant ability by increasing ascorbate and aldarate metabolism through rumen prevotellaceae ucg-004 in hu sheep. Front Microbiol. (2022) 13:978940. doi: 10.3389/fmicb.2022.978940
51. Lavoy ECP, McFarlin BK, Simpson RJ. Immune responses to exercising in a cold environment. Wilderness Environ Med. (2011) 22:343–51. doi: 10.1016/j.wem.2011.08.005
52. Chang HM, Wang XL, Zeng HF, Zhai YF, Huang N, Wang CJ, et al. Comparison of ruminal microbiota, metabolomics, and milk performance between montbeliardexholstein and holstein cattle. Front Vet Sci. (2023) 10:1178093. doi: 10.3389/fvets.2023.1178093
53. Conte G, Dimauro C, Daghio M, Serra A, Mannelli F, McAmmond BM, et al. Exploring the relationship between bacterial genera and lipid metabolism in bovine rumen. Animal. (2022) 16:100520. doi: 10.1016/j.animal.2022.100520
54. Pan XH, Xue FG, Nan XM, Tang ZW, Wang K, Beckers Y, et al. Illumina sequencing approach to characterize thiamine metabolism related bacteria and the impacts of thiamine supplementation on ruminal microbiota in dairy cows fed high-grain diets. Front Microbiol. (2017) 8:1818. doi: 10.3389/fmicb.2017.01818
Keywords: immunity, probiotics, cold stress, microbiota, rumen, feces
Citation: Luo T, Zhu J, Li K, Li Y, Li J, Chen Y and Shi H (2024) Crosstalk between innate immunity and rumen-fecal microbiota under the cold stress in goats. Front. Immunol. 15:1363664. doi: 10.3389/fimmu.2024.1363664
Received: 03 January 2024; Accepted: 08 February 2024;
Published: 26 February 2024.
Edited by:
Yangchun Cao, Northwest A&F University, ChinaReviewed by:
Qingbiao Xu, Huazhong Agricultural University, ChinaJunshi Shen, Nanjing Agricultural University, China
Copyright © 2024 Luo, Zhu, Li, Li, Li, Chen and Shi. This is an open-access article distributed under the terms of the Creative Commons Attribution License (CC BY). The use, distribution or reproduction in other forums is permitted, provided the original author(s) and the copyright owner(s) are credited and that the original publication in this journal is cited, in accordance with accepted academic practice. No use, distribution or reproduction is permitted which does not comply with these terms.
*Correspondence: Hengbo Shi, c2hpaGVuZ2JvQHpqdS5lZHUuY24=
†These authors have contributed equally to this work