- 1School of Life Sciences, Faculty of Science, University of Technology Sydney, Sydney, NSW, Australia
- 2Department of Computer Science, University of Tabriz, Tabriz, Iran
- 3Institute of Biochemistry and Biophysics (IBB), University of Tehran, Tehran, Iran
- 4Department of Quantitative Health Sciences, John A. Burns School of Medicine, University of Hawaii at Manoa, Honolulu, HI, United States
- 5Department of Biology and Research Center for Animal Development Applied Biology, Mashhad Branch, Islamic Azad University, Mashhad, Iran
- 6Infectious Diseases and Tropical Medicine Research Center, Isfahan University of Medical Sciences, Isfahan, Iran
- 7Department of Biology, Faculty of Sciences, Shahid Bahonar University of Kerman, Kerman, Iran
- 8Department of Biology, Science and Research Branch, Islamic Azad University, Tehran, Iran
- 9Department of Neurology, The Second Affiliated Hospital, Zhengzhou University, Zhengzhou, Henan, China
- 10Respiratory Cellular and Molecular Biology, Woolcock Institute of Medical Research, Sydney, NSW, Australia
In line with encountering the world with the emergence of vaccine-resistance variants of SARS-CoV-2, 15,669,529 samples that received COVID-19 vaccines until April 2023 were investigated as two doses in the first phase and booster vaccinations in the second phase. The analysis shows that D614G and P681 mutations occurred in both phases. The E484 and Y655 mutations significantly emerged during the second phase. The 762-889 and 254-381 regions are revealed as conserved parts and could be considered in vaccine design. The Kruskal–Wallis test revealed a significant reduction in single mutations between populations with 20%–50% and those with 70%–100% vaccination coverage (p=0.017). The Mann–Whitney U test proposes a link between vaccination and suppression of viral mutation rates. Dynamic modeling suggests that key mutations have facilitated the virus’ evolution and immune escape. The study’s findings are crucial for understanding virus genome mutations, especially E614 and P681 in Delta and E484 and H655 in Omicron. This highlights the need to adjust strategies and strengthen global efforts in combating the pandemic.
Introduction
The World Health Organization (WHO) declared a pandemic due to the novel severe acute respiratory coronavirus 2 (SARS-CoV-2) infection in early 2020 and named it coronavirus infection disease 19 (COVID-19). The virus, similar to SARS-COV, belongs to the beta-lineage coronaviruses. The encapsulated single-stranded RNA of the virus encodes several non-structural proteins and approximately 30 functional proteins (1–3). The spike is critically essential in infection and immunogenicity mediating virus entry into cells (4). Immediately after binding the spike to the cell receptor, two proteases, furin and TMPRSS2, cleavage the spike’s S1/S2 and S2 subunits, respectively (5). The first cleavage exposes the receptor-binding domain (RBD) and/or the S2 subunit after disassociation and shedding of S1. The proteolytic activity of TMPRSS2 results in the S2 subunit cleavage, the binding of virus capsid to the cell membrane, and the infusion of virus RNA to the host cell (6). Neutralizing antibodies (nAbs) induced by vaccines are the cornerstone of COVID-19 treatment (7). Various vaccines were developed, many based on the spike protein (8), particularly the S1 subunit, which contains the neutralizing antibody epitopes mainly on the RBD (located on the C-terminus of S1) and the recognition sites (9). However, the fast transmission of the virus and the emergence of various mutations due to amino acid (A.A) changes in the spike region obligate monitoring to improve the vaccine design. For instance, the mortality rate of SARS-CoV-2 in some countries was associated with the dominance of D614G mutation in the spike protein and various variants such as delta and omicron, even with the administration of booster vaccinations (10, 11). On the other hand, the P681 mutation has a key role in the replacement of the Alpha-to-Delta variant (12). These mutations still exist in samples despite the vaccination’s upward trend. This issue emphasizes the critical role of these spike mutations in spreading the disease globally and subsequently affecting the efficacy of developed vaccines (13, 14). Furthermore, tracking these mutations in the spike protein during various phases of vaccine administration across countries could provide insights into the evolution of SARS-CoV-2 mutations and vaccine efficacy.
In this study, we aim to monitor the spike protein’s amino acid mutation pattern using the GISAID and Sars2Mutant databases (15, 16). We will compare the rate and type of mutations between the two vaccine doses and booster vaccinations in countries where more than 50% of the population has received booster shots. As a result, a big data approach by the Sars2Mutant database (17) is used to look for variants, as well as these A.A mutation patterns of spike protein in these time points. The findings provide valuable information supporting the design of more effective treatment, primarily vaccines for COVID-19 (Figure 1).
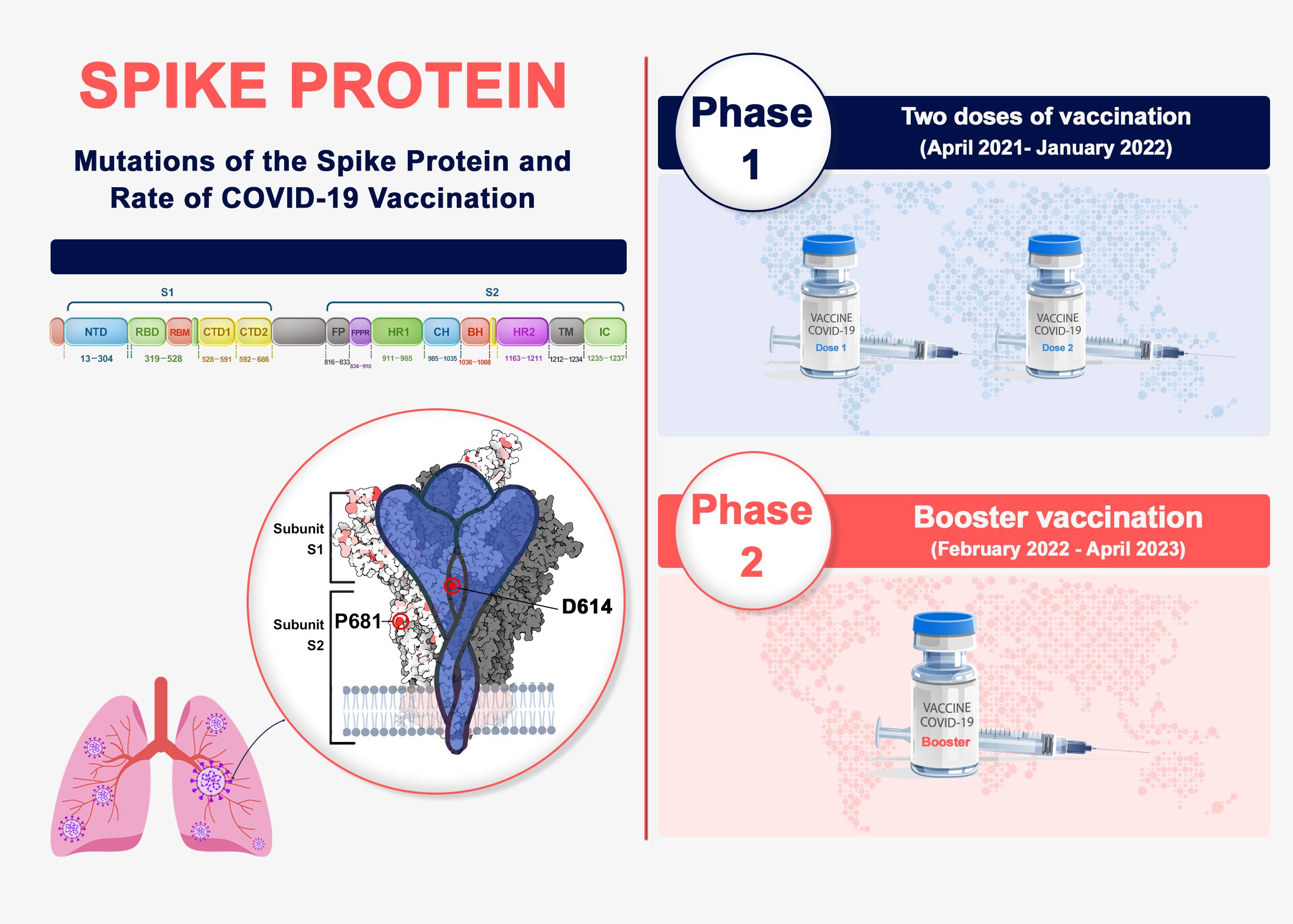
Figure 1. Spike protein genome and phases of vaccination in COVID-19. Spike genome organization includes two S1 and S2 subunits. The top mutation occurrences in spike were analyzed in two phases of vaccination progress.
Methods
Data repositories and spike variant calling
The present study was designed to address the mutations as a reflection of genome sequence in the spike protein of SARS-CoV-2 compromising 1,273 A.As during the different time points of vaccinations. The top A.A changes were chosen from countries that got 50% and more vaccination. These mutations in the spike region were investigated in specific eligible vaccinated countries categorized into two phases of vaccinated populations, which are presented as follows by exact periods:
• Phase 1 (first two doses of vaccination): At least 50% of the population had two doses of vaccination, as shown in Supplementary Tables 1, 2 (April 2021-January 2022)
○ Before vaccination (March 2020-December 2020)
○ Initial vaccination (January 2021-March 2021)
○ Middle of vaccination (April 2021-Jun 2021)
○ End of vaccination (July 2021-January 2022)
• Phase 2 (booster): More than 50% of people had booster vaccination, as shown in Supplementary Table 3 (February 2022-April 2023)
Data analysis was carried out by trimming the outputs in a specific pattern. Within each phase, mutations were ranked based on their frequency across eligible countries, those meeting the vaccination coverage threshold. From this ranking list, the top four highest mutation rates in multiple countries were selected. These four mutations exceeded the ≥50% prevalence threshold across all or the majority of eligible countries in that phase. Eventually, these top mutations were considered significant and frequent by rate of substitution to report as phase 1 and 2 results and then compare them.
Further metadata analysis was carried out by Python 3.8.0 to isolate the spike sequence. Furthermore, the FASTA sequences of the SARS-CoV-2 spike protein (S1 and S2 subunits) were aligned to the reference sequence, and the variants were called. Among the obtained records of spike protein sequences, trimming was carried out on the non-human samples, and sequences with less than 1,273 A.As and non-specified A.As were selected. Finally, 15,669,529 sequences were included in the current study. The “Numpy” and “Pandas” library approaches were adopted to improve the efficiency of all stages. The applied algorithm for identifying the mutations is described as follows. Since all sequences have equal lengths, the following algorithm used “Refseq” and “seq” to refer to the reference sequence sample sequence, respectively.
For refitem, seqitem in zipping (RefSeq, seq)
If (refitem! =seqitem)
Report a new mutant
Subsequently, the determined mutations of SARS-CoV-2 locations were classified based on the continent, country names, and countries’ global coordinates using country-convert 0.5.8 software and the “Titlecase” library in Python.
The gathered data on vaccination based on the reports from the New York Times (https://www.nytimes.com/interactive/2021/world/covid-vaccinations-tracker), the economists (https://www.economist.com/graphic-detail/tracking-coronavirus-across-the-world), and our world in data (https://ourworldindata.org/covid-vaccinations). Furthermore, the countries underwent the trimming with the following criteria:
1. The recipient of booster vaccination was considered fully vaccinated and included in the study.
2. Countries have vaccinated their populations with similar vaccines in different groups (only FDA-approved vaccines, namely: Pfizer/BioNTech, Moderna, Johnson & Johnson, AstraZeneca, Sinovac, Sinopharm/BIBP, Covishield, Covaxin, and so on).
3. The registered sequences are normal and are analyzable.
4. Samples exclusion of: divergent, short, or lengthy sequences, gaps, unspecified amino acids (indicated by X), and genomes from non-human hosts.
5. Each country has equal input NGS data regarding the number of next generation sequence (NGS) data.
The initial strain of the COVID-19 virus, designated as “EPI_ISL_402124,” served as the benchmark reference sequence for aligning all subsequent samples. To explore and call variants, the GISAID (www.gisaid.org) data source was utilized to collect the data from July 2021 to April 2023 with 15,669,529 sequences (16, 18, 19). It is worth mentioning that trimming was applied based on the nonsense changes and drawing out the sequences with deletions, which resulted in a shorter sequence of 1,273 A.As. Access to GISAID was provided by permission of John A. Burns School of Medicine Department of Quantitative Health Sciences.
Statistical analysis of mutations and vaccination stages
The quantitative counts of SARS-CoV-2 mutations were standardized, and statistical analyses, including the Kruskal–Wallis test and Mann–Whitney U test, were applied to ascertain differences in mutation counts across vaccination rate groups. Correlation coefficients were computed for pairwise phase comparisons, and two heatmaps were constructed to synthesize the findings. The study provided a detailed explanation for the selection of the statistical tests, highlighting their suitability for the non-parametric nature of the data, ordinal data, unequal sample sizes, and specific hypothesis testing. Ethical considerations were addressed, and institutional review board approval was not required as the study involved the analysis of publicly available data without direct human or animal subjects, and all methods were performed following relevant guidelines and regulations.
Secondary protein structure and dynamic prediction
The study analyzed the mutational structure and molecular flexibility of the spike protein modeling on the D614G, E484K, and H655Y mutations, which are the most frequent mutations in COVID-19. The DynaMut web server (http://biosig.unimelb.edu.au/dynamut/) was used to perform the analysis, and the PDB ID of protein (7QUS) was taken from the Protein Data Bank (https://www.rcsb.org/).
Results
To investigate the mutation dynamics in spike protein during the vaccinations, we carried out the mutational analysis. All the reported mutations were the identified mutations at the end of vaccination (July 2021-January 2022), highlighting their dominance and stability in the viral population.
These mutations were investigated during two distinctive phases (phase 1 receiving two doses of vaccination and phase 2 being the booster). The occurrence threshold was at least 50% in all countries (equal to more than 10 repeats). In this regard, the mutual mutations in each separated group are reported and compared. Figure 2 illustrates the workflow from data gathering, processing, and analysis.
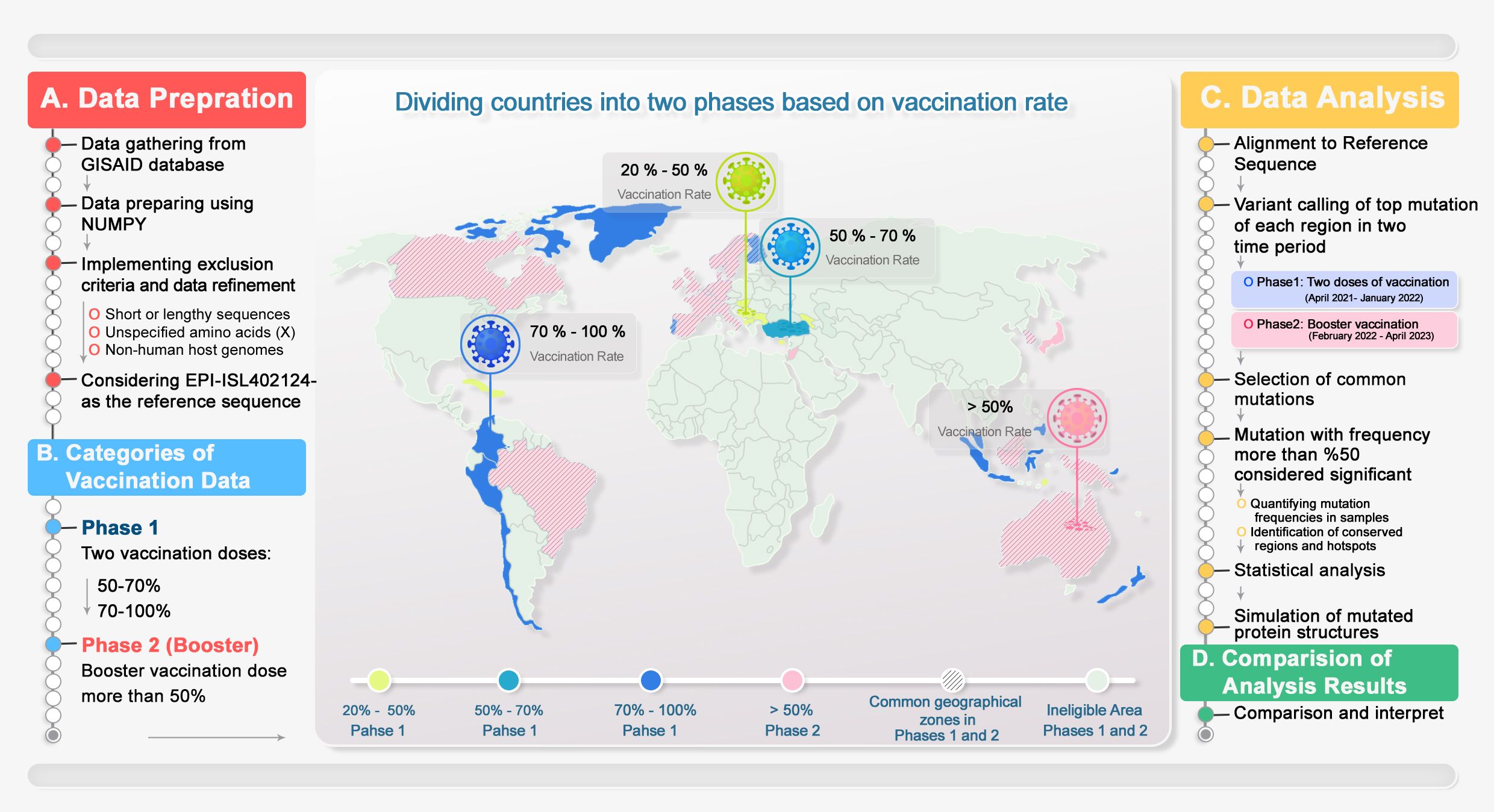
Figure 2. Methodology overview. This is a schematic representation of the data mining process, categorized into two distinct phases: data refining and analysis, followed by data reporting. Countries were categorized into two phases based on number of received doses of COVID-19 vaccines. Overlapping areas between groups were hatched. 1) Data from GISAID were extracted and analyzed, and the NGS data criteria for each country and group were applied. 2) Geographical data categorized based on doses of vaccination with a chance of 50% occurrence in each phase of vaccination were reported. 3) The Wuhan genome was considered the reference genome. Next, the obtained mutations were processed. The common mutations with more than 50% at the different vaccination phases were concluded. 4) The top mutations of each period of vaccination were compared, and common or unique mutations were presented to help the vaccine design industry.
Common mutation patterns across countries in two distinct phases
The top detected mutations in each phase at specified vaccination periods were selected, and then the mutual mutations were highlighted. Only the samples with more than 50% repetitive mutations in each phase were reported in the next step. All the common mutations in different phases are presented in Table 1.
Phase 1 two doses of vaccination and mutation patterns
Countries with 20%–50% vaccination coverage
The 20%–50% group in phase 1 (population receiving two doses of vaccine) was selected to investigate the initiation of mutation dynamics compared with the Wuhan genome. Furthermore, this phase of vaccination is considered as the baseline for before, initial, middle, and end periods presented in Supplementary Table 4.
This group covers the countries where only 20%–50% of their population have received two doses of their vaccines. P681H/R/- mutation was observed in the initial, middle, and end of the designated time points for a vaccination with (64.1%, 94.43%, and 99.23%) respectively. P681 mutation with A.A conversion to histidine was dominant during the initial (58.07%) and middle (88.1%); however, the deletion of the proline at the 681 positions was the prevalent substitution seen at the end of the selected vaccination time point with 78.73%. The mutations E156- (86.52%), F157- (86.3%), L452R (86.42%), T19R (85.53%), T478K (86.4%), D950N (87.87%), G142D (82.05%), and R158G (88.28%) were the other mutations detected only at the end of the selected time points for vaccination (Table 1).
Countries with 50%–70% vaccination coverage
In the countries that had 50%–70% of the population vaccinated, the most frequent mutation was D614G detected at the end of the phase 1 period of vaccination with a high rate of up to 99.96%. Furthermore, P681 is another mutation detected in all the stages of the phase 1. The P681 substitution mutation shows with a gradual increase toward the end of phase 1, reaching a prevalence of 92.02%. Considerably, we found D950N (90.63%), E156- (91.66%), F157- (91.7%), L452R (92.28%), R158G (91.71%), T19R (92.25%), T478K (92.41%), and G142D (76.28%) mutations at the end of the selected phase 1 vaccination period (Table 1).
Countries with 70%–100% vaccination coverage
In countries with coverage of 70%–100% vaccinated population in phase 1, the D614G mutation is the most common mutation among all of the top mutations that maintained itself as long as two doses of vaccination were ongoing at a high rate (99.95%). On the other hand, P681 was another common mutation during all the assigned time points of investigation, with a significant increase among the nations with 97.06% at the end of the first phase (Supplementary Table 5). Some of the mutations were noticed to be significant at the end of the phase 1 period of vaccination in these countries, such as E156- (93%), F157- (93.03%), L452R (94.46%), T19R (93.97%), T478K (94.39%), D950N (94.15%), R158G (95.03%), and G142D (82.27%) (Table 1).
Comparative analysis between mutations in 50%–70% vs. 70%–100% vaccinated populations in phase 1
The performed analysis presented notable results about the maintenance of mutations in the spike protein. All frequent mutations in 50%–70% of the dose-vaccinated group were also detected in 70%–100% of the vaccinated population in phase 1. Despite continuous process of vaccination, the rate of each mutation has increased, which could be a useful sign in vaccine design industry.
Phase 2 insights: similarities and differences in countries with over 50% booster vaccination coverage
In countries with coverage of more than 50% booster, D614G was the most frequent mutation with a significant rate among the nations (95.92%). Also, it is notable that P681, E484, and H655 mutations were observed in all eligible countries along the booster vaccination dose with 94.69%, 93.88%, and 93.27%, respectively (Table 1).
Mutations across phases 1 and 2
After investigating separately into two phases of vaccination, we found the two common mutations in both two doses of vaccination and booster periods. D614G and P681 also appeared and were conserved in the second phase despite booster vaccination. However, we also discovered that the E484 and H655 mutations in the booster dosage are distinct variants that were not found in phase 1, which is a noteworthy finding that should be taken into account when designing vaccines.
Variant calling rate in investigated samples in each group in the first phase of immunization and booster dose
Countries with 20%–50% two-dose vaccination
This study group showed that, before the initiation of immunization, a single, dominant mutation existed, with a frequency of 46.62%. The majority of the investigated spikes (54.63%) had four or more alterations at the start of vaccination. With the progress of the vaccination, the four and more mutations in spike emerged and 52.62% and 31.63% of the sequences showed no mutation (Figure 3A; Supplementary Table 4).
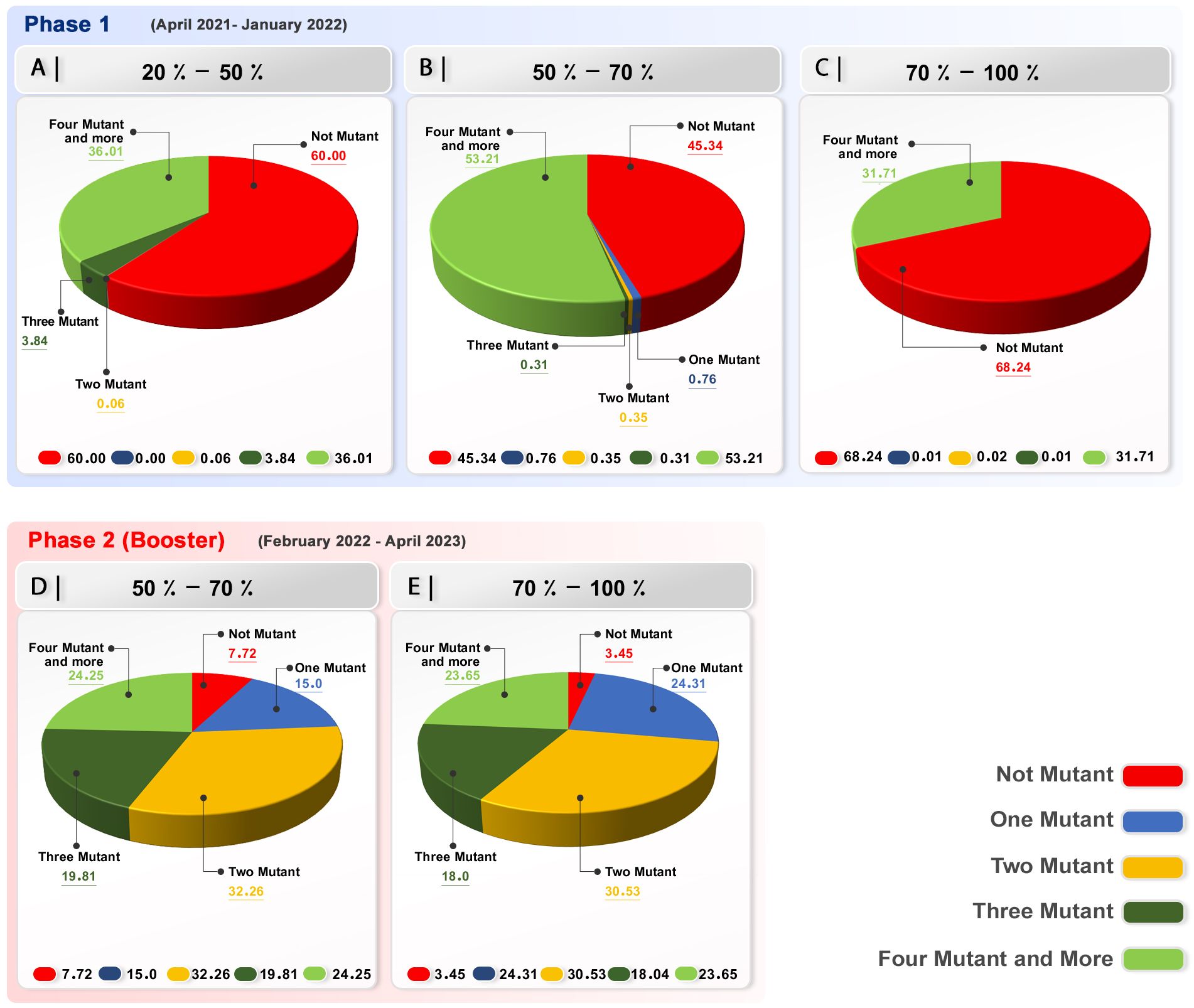
Figure 3. Variant calling rate in investigated samples in each group in the first phase of immunization and booster dose. Phase 1 (A) Group with 20-50% receiving two doses of vaccine; a significant number of sequences demonstrated no mutation in their spike. (B) Group with 50-70% receiving two vaccine doses; a significant number of sequences demonstrated no mutation in their spike. However, four mutations in the investigated samples were nearly equal to no mutation. (C) In the group with 70-100% two-dose-vaccinated population in phase 1, with the progress of the vaccination, a significant number of sequences demonstrated no mutation in their spike. Phase 2 (Booster) (D, E) Booster group, 50% of the population receiving booster dose; a significant number of sequences demonstrated more than one mutation in their spike.
Countries with 50%–70% two-dose
The number of mutations in this group can be categorized into two distinct sections. The first section shows that 51.87% of the spike sequences had no mutations, whereas the second section, comprising 46.12% of the sequences, had four or more mutations, indicating a significant increase after vaccination (Figure 3B; Supplementary Table 1).
Countries with 70%–100% two-dose
This group’s data, with a focus on the number of mutations in different vaccination stages, demonstrated that the population in phase 1 had a higher percentage of four or more mutations at the beginning of vaccination with 47.83%. Interestingly, at the end of vaccination, 70% or more of the population had received two doses of vaccine, and 63.7 of the samples showed no mutation in their spike. These findings highlight the efficiency and inhibitory effect of the vaccination when 70% or more of the population has been immunized (Figure 3C; Supplementary Table 2).
Phase 2 (booster dose)
The booster immunization phase in our study demonstrated that the occurrence of two mutations was dominant (31.23%). There were four or more mutations in the sequences, and one mutation was detected at 22.93% and another at 22.44%. Three mutations are present in the sequences of 18.08% of the samples (Supplementary Table 3). Nonetheless, a low percentage of the sequences (5.30%) displayed no mutation in their spike.
The result highlights the effect of vaccination on the mutation dynamic of the spike, and by increasing the number of vaccinated population and, more importantly, the fast rate of vaccination, the occurrence of mutation diminishes (Figures 3D–E).
Vaccination impact: heatmap analysis of hotspots and conserved spike regions
We then categorized the spike sequence into 10 regions (1-127, 127-254, 254-381, 381-508, 508-635, 635-762, 762-889, 889-1016, 1,016-1,143, and 1,143-1,270), and we evaluated the mutation dynamics during the different periods of immunization to evaluate the susceptible parts of the spike to the mutations (Figure 4).
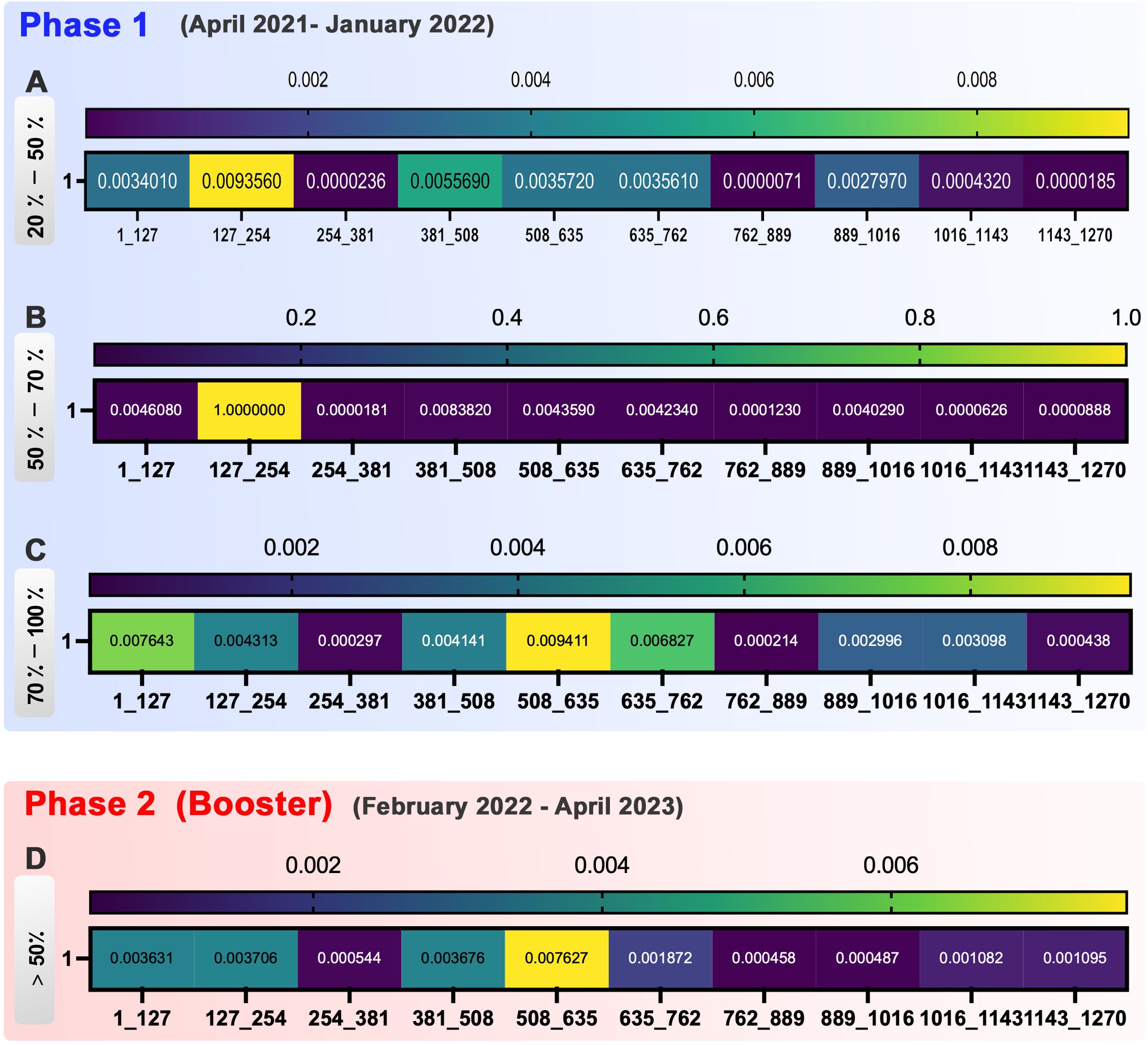
Figure 4. Heatmap represents the occurrence of spike mutations at various phases of vaccination. Phase 1, (A) In the 20-50% group of phase 1, 254-381, 762-889, and 1,016-1,270 were shown as conserved regions. (B) In the 50%-70% group of phase 1, the hot spots of mutation incidence are 127-254 regions in spike. Furthermore, 254-1,270 were detected as the conserved site. (C) In the 70%-100% group of phase 1, sequences 254-381, 762-889, and 1,143-1,270 are conserved to the mutation incidence, whereas 1-127, 381-508, 635-762, and 889-1,143 were more susceptible to mutations. Phase 2 (Booster), (D) In phase 2 of vaccination, the hot spots of mutation incidence are next to each other in this group as 381-762 regions. Besides them, 254-381 and 762-1,016 are shown as conserved regions.
In group 20%-50% of phase 1, the dynamic of mutations showed high variation at the different time points. Sequences 254-381, 762-889, and 1,143-1,270 were the most conservatory sequences with minor mutation occurrence in this group (Figure 4A).
In the 50%-70% group of phase 1, sequence 127-254 demonstrated to be the hot spot for mutation occurrence by vaccination progress. The other hot spots of mutation detected in spike during the vaccination were the 381-508, 635-762, and 889-1,016 sequences. The conserved site was 254-381, with the lowest mutation incidence (Figure 4B).
In the 70-100% group of phase 1, the sequences 1-127, 381-508, 635-762, and 889-1,143 were more susceptible to mutations during the vaccination process. On the other hand, sequences 254-381, 762-889, and 1,143-1,270 simultaneously were conserved for mutation incidence (Figure 4C).
Despite the variation in mutation frequency in the 1,016-1,143 and 1,143-1,270 sequences, the mutation rate decreased significantly at the end of the two doses of vaccination (Figure 4).
In phase 2 (booster) of vaccination progress, we had four common mutations. E484 and P681 were mutants in 381-508 and 635-762, respectively. D614G and H655 were shown in the 508-635 region. Therefore, by considering the effect of booster vaccination on spike sequence, these three regions that are rated as top high-rate mutations could present as hot spot regions of spike in the second phase of vaccination. Taken together, between the three groups, sequence 762-889 was the most conserved part of spike mutual among groups (Figures 4C, D).
Statistical significance of mutation counts across vaccination rates
The Kruskal–Wallis test revealed differentiated patterns in the distribution of SARS-CoV-2 mutations in response to varying vaccination rates during the initial phase of vaccine rollout. A statistically significant decrease in the incidence of one mutant was identified when comparing populations with a vaccination rate of 20%-50% against those with 70%-100% coverage, yielding a p-value of 0.017. This implies that a decrease in the incidence of single mutations may be linked to increased vaccination coverage, possibly demonstrating the impact of vaccination on reducing the rates of viral mutation.
In a similar vein, the frequency of more than four mutants and more profiles showed significant variation between the 20%-50% and 50%-70% groups, with a p-value of 0.05. This highlights a substantial relationship between intermediate levels of vaccination coverage and the emergence of complex mutations.
The 20%–50% and 50%–70% vaccination rate groups in the “Two Mutant” category, on the other hand, showed a non-significant trend in “Phase 1 (last),” with a p-value of 0.13. Even though this does not reach the threshold for statistical significance, it suggests a potential relationship that needs more research and may become significant with a larger dataset.
It is noteworthy that the “Not Mutant” category across all phases consistently showed non-significant p-values, indicating no substantial difference in the absence of mutations among the various vaccination rate groups. This consistency implies that vaccination rates may not influence the overall rate of no mutation (Figure 5). These results highlight the fact that high vaccination rates exert strong immune selection pressure on SARS-CoV-2, reducing the prevalence of single, random mutations. Instead, the virus is driven to evolve clusters of mutations (e.g., in variants of concern like Delta and Omicron) that enhance immune evasion or transmission efficiency.
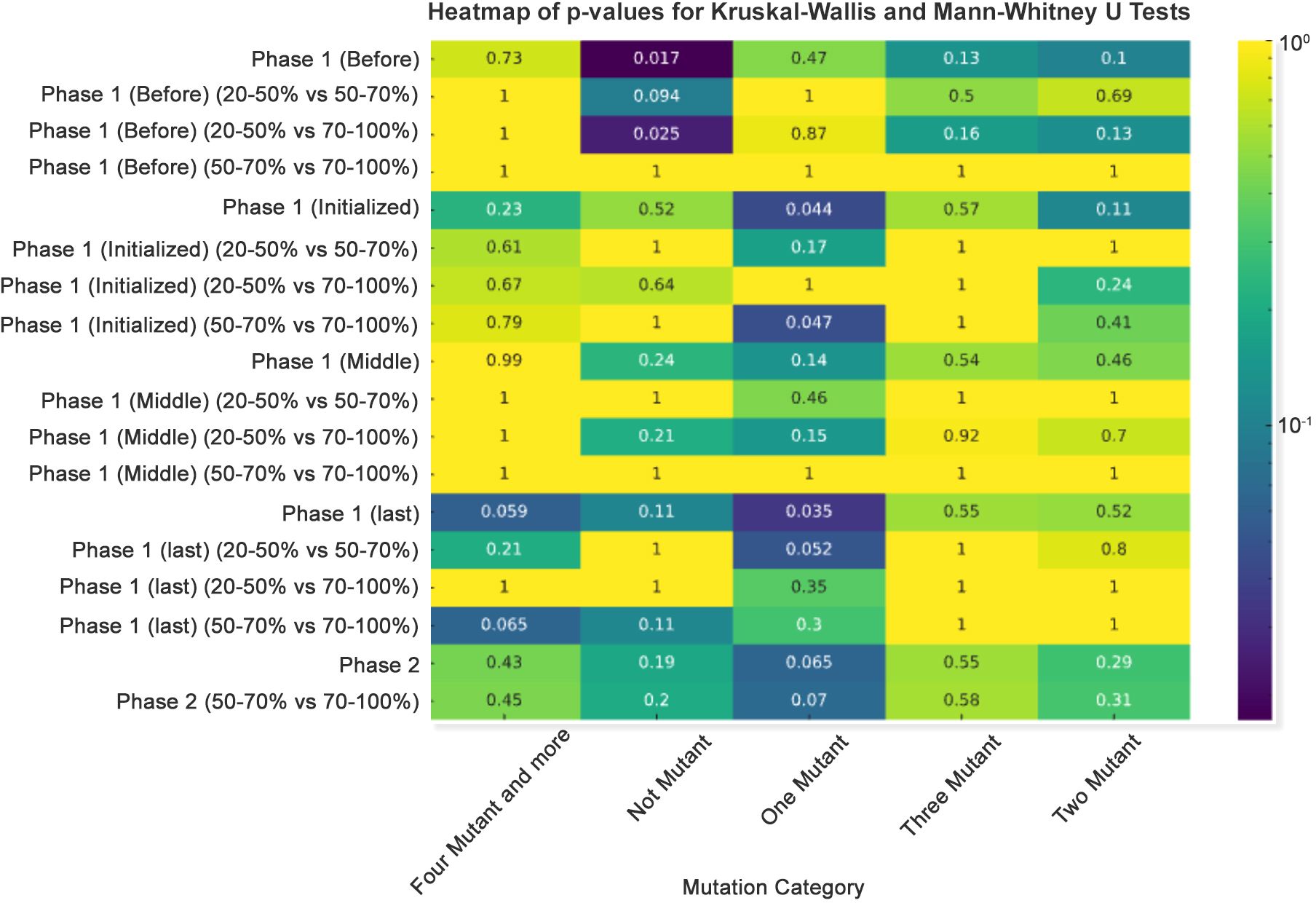
Figure 5. Heatmap of p-values for Kruskal–Wallis and Mann–Whitney U tests. A p-value heatmap was created to illustrate the significance of differences in mutation counts, employing a sequential color scheme to signify p-value gradations, with darker hues indicating lower p-values.
Correlational analysis across phases demonstrated significant linear relationships in several mutation categories. The correlation coefficient for the “Three Mutant” category between Phase 1 (Before) and Phase 1 (Initialized) was 0.76, denoting a strong positive correlation. Conversely, the correlation between Phase 1 (Middle) and Phase 2 (Booster) for the “Four Mutant and more” category was −0.73, reflecting a strong negative correlation, suggesting an inverse relationship between these phases as the booster campaign unfolded (Figure 6).
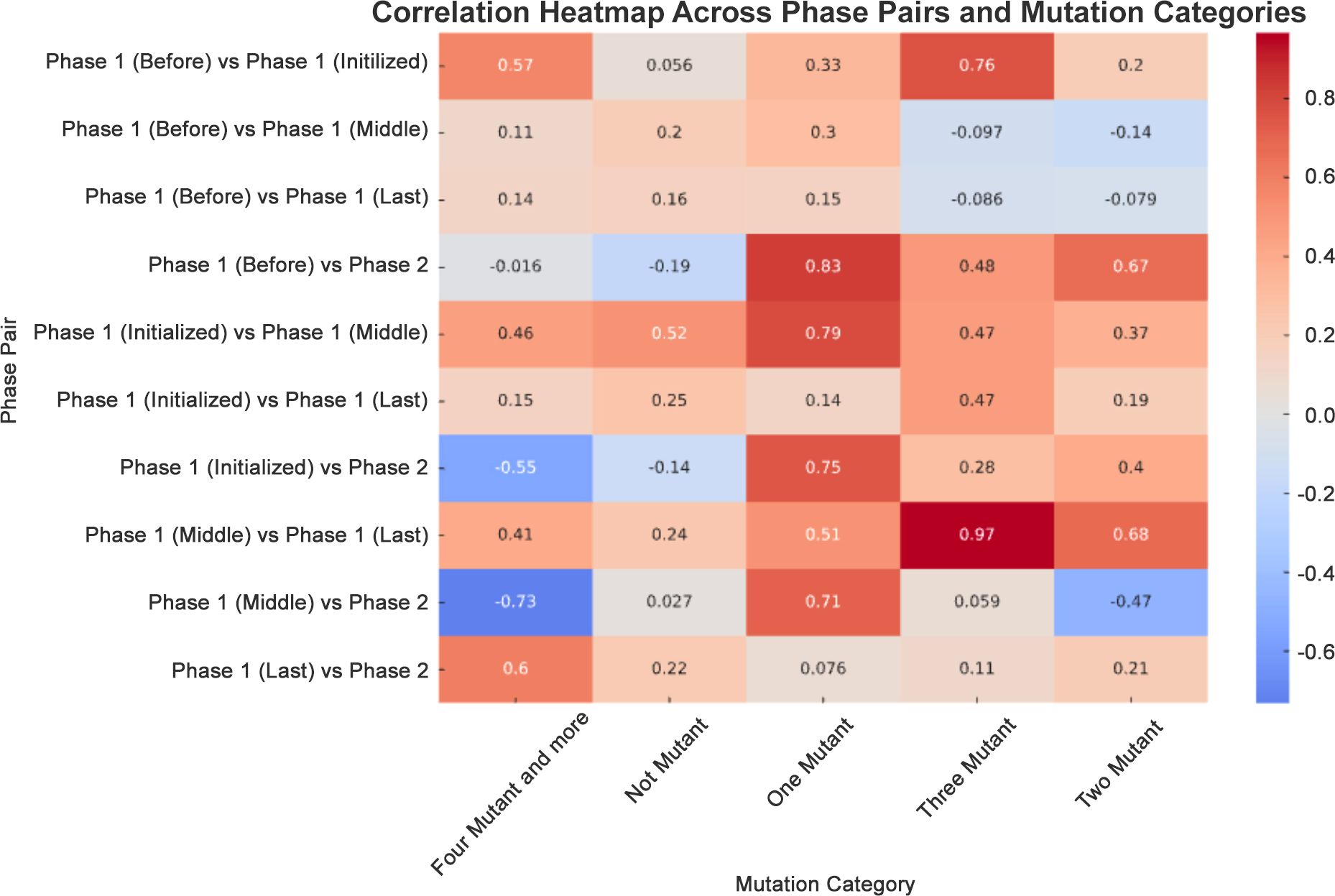
Figure 6. Correlation heatmap across phase pairs and mutation categories. A correlation heatmap was generated to depict the relationship between mutation counts across different phases. This used a diverging color palette to distinguish between positive (blue) and negative (red) correlations.
Assessment of D614G, E484K, and H655Y mutations on dynamicity and flexibility of spike
To assess how the top mutations examined in this study impact the spike protein’s structure, we utilized the DynaMut website for protein modeling. We determined the variation in vibrational entropy energy (ΔΔSvibENCoM) between the wild-type and mutant forms. The results indicate that the mutation at position D614G increased molecular flexibility within the spike structure by 0.768 kcal·mol−1·K−1, whereas the mutation at position E484K resulted in a flexibility increase of 0.309 kcal·mol−1·K−1.
Conversely, alterations from aspartate to glycine at position 614 and from glutamic acid to lysine at position 484 were found to destabilize the spike protein structure, leading to a binding affinity change of −0.323 and −0.312 kcal/mol, respectively. Among these structural destabilizer mutations, there was also a noteworthy H655Y mutation that offered a slight increase in molecule stabilization with a ΔΔG value of 0.013 kcal/mol. However, this mutation simultaneously contributed to an increase in molecular flexibility with a value of 0.117 kcal·mol−1·K−1. By studying how these amino acid alterations impact intramolecular interactions, we can gain insights into why protein structure destabilization occurs following mutations and vaccination processes (Figure 7).
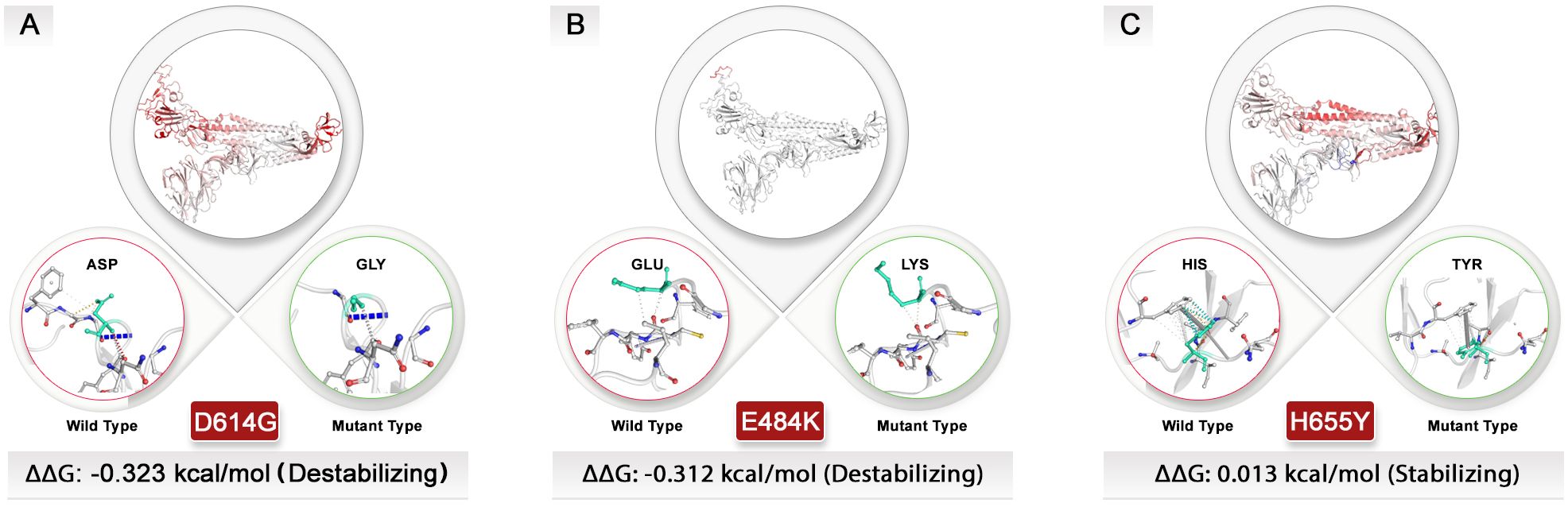
Figure 7. Exploring the structural dynamics of spike protein: unraveling the influence of D614G, E484K, and H655Y mutations. Vibrational entropy changes upon mutation are visually depicted through a color-coded scheme, with amino acids taking on different hues. Notably, the vibrant red color signifies an increase in flexibility within the spike protein. To provide a comprehensive view, wild-type and mutant residues are displayed as light-green sticks, juxtaposed with the neighboring residues engaged in various types of interactions. (A) The D614G and (B) E484K mutations with negative ΔΔG values could destabilize spike structure. (C) In contrast, the H655Y mutation had a different effect on the protein’s structure and was stabilized, unlike other top mutations. All dynamic modeling used the 7QUS PDB ID. Due to the unavailability of a PDB ID that presents mutations in the P681 site, modeling for this mutation was not possible.
Discussion
The vaccination strategy is the most effective public health measure to control and alleviate the SARS-CoV-2 pandemic (20, 21). Even after the start of global vaccination, the emergence of new strains, including those resistant to vaccines, remains a major concern. So far, established databases for the SARS-CoV-2 are limited, and their classifications are based on viruses at the genome mutations and evolutionary levels (22, 23). The Sars2Mutant database has facilitated these limitations by providing features such as gene classification, and detection of mutations at protein levels in the various continents, countries, and timelines. Furthermore, this database provides insights into the exact loci of the mutations regarding the frequency in each gene, hotspot, and highly conserved region of SARS-CoV-2 (17). The 762-889 region, as the most conserved part of SARS-CoV-2 in the current study, was shown as part of 16 relatively conservative epitopes (747‐763, 749‐771, 754‐770) including vaccine candidate epitopes (24).
In this regard, the findings of our study showed that with the progress of vaccination, the occurrence of mutations decreases, and at the end of the second-dose vaccination time point, the majority of the sequences showed no mutations. In support of our findings, a SIR-derived model-based study found that the rapid vaccination rate reduces the emergence of vaccine-resistant strains (25). Nevertheless, the percentage of the vaccinated population is not the only factor in the occurrence of mutations and the rise of new strains. The transmission of the virus is another critical factor influencing the emergence of new strains. This could be a plausible explanation for shifting all the study groups to no mutation in their sequences. The study indicates that during the booster vaccination in phase 2, the mutated sequences increased due to increased virus transmission power. This finding is significant because it highlights the need to revise vaccine design to adapt to the evolving genomic variations.
A variety of mutations have been detected in the two phases. However, some of these mutations were abundantly and consistently seen in all groups, meaning that the virus is more prone to keep these mutations. The most dominant reported spike protein mutation detected in all groups is D614G, which enhanced virus replication via increased infectivity and stability (26, 27).
The D614G in Alpha, Delta, Beta, and Omicron variants modulates the binding to the angiotensin-converting enzyme 2 (ACE2) receptor, which could be important in determining virus entry, immune escape, transmissibility increment, and reinfection (28–31). Also, P681 is the other dominant mutation in the spike that could display highly increased fusogenic activity and syncytia formation capability (32). P681 was a mutation common in all groups that increased gradually in the process of vaccination. P681 became one of the dominant mutations in both vaccination phases. P681 mutation cooperates with the furin cleavage site. However, Lubinski et al.’s study demonstrated that this mutation does not affect the proteolytic feature of spike protein and, thereby, the viral entry or cell–cell spread (33). P681 was the mutation only reported in the Delta variant that seems to augment the spike processing and spike fitness compared with the alpha variant (12, 34). Interestingly, this mutation has not been appropriately investigated and detected in isolated human B.1.1.7 samples from USA and India (35).
The data analysis illustrated that E484 was among the common mutations in both two phases of vaccination; however, it had a meaningful increase in the second phase. The E484 site has a functional role in RBD that significantly affects the binding affinity with the ACE2 receptor. Subsequently, a mutation in the E484 site could have a significant impact on the immunogenicity of the RBD protein, which could affect the pathogenicity and transmissibility of the mutant virus (36–38). Aligning the RBD sequence confirmed both Beta and Omicron harboring mutation at residue E484 that were replaced to Lysine for Beta and to Alanine for Omicron variants (39, 40). The bivalent Original/Omicron BA.1 vaccine contains mRNA that carries instructions for cells in the body to make the spike protein that is also on the virus. The cells then make antibodies against the spike protein to help fight off the virus. The bivalent vaccine is effective in producing high levels of neutralizing antibodies against Omicron subvariants (41). Recent research suggests that incorporating more than one strain in vaccine development, booster shots, and other vaccine changes is crucially important (42). Following booster vaccination, the H655Y mutation appeared at a high rate in samples, resulting in increased viral endpoint yields in human respiratory cells, and was associated with enhanced spike processing in the Gamma and Omicron variants. Furthermore, Y655 is found close to the fusion site, enhancing virus–cell fusion. In this regard, vaccination and neutralizing antibodies are the major obstacles in this process. Therefore, this mutation will enable the virus to overcome this problem, potentially giving it an advantage in spreading among populations (43, 44). H655Y, as a notable mutation in the second phase with stabilizing feature for spike protein could be an important for the vaccine industry (45, 46).
An overview of these four prevalent mutations may help us identify key spike structure components, particularly CTD2 and RBD. Given that E484 in RBD, and D614G, H655, and P681 are located in CTD2, these regions of the spike could be considered as the highlighted regions in the COVID-19 vaccine design (47). Vaccination flow alongside appearance and increasing rate of E484 and H655 in the second phase of vaccination by booster dose could explain why these two mutations are resistant to booster dose. The explanation for the outbreak of these variants, which is consistent with earlier research, was provided by the synchronization of the spread of Alpha, Gamma, and significantly Omicron variants with the appearance of these mutations (48).
Higher vaccination rates are associated with a decrease in the incidence of one mutant, which may indicate that immunization limits viral evolution. Conversely, the appearance of more intricate mutation profiles at intermediate vaccination rates—four mutants or higher—may point to a complex interaction between vaccination coverage and virus evolution. The marginal trends observed in some categories, such as the “two mutant” group, highlight areas for further investigation, suggesting that expanding the dataset could unveil additional significant relationships (49, 50).
Our study suggests that mutations in COVID-19 are growing due to the increase in virus transmission capacity, especially after booster vaccination. The study identified four top mutations, two of which were shown in each of the two phases, whereas two high-rate mutations were only reported after booster vaccination in the population. The results highlight the need to revise vaccine design to adapt to the evolving genomic variations by considering conserved spike regions into multi-epitope or universal vaccines. Moreover, vaccine developers can create polyvalent mRNA or protein-subunit vaccines that maintain efficacy even as SARS-CoV-2 continues to evolve. Such a strategy would be helpful to ensure that future vaccine formulations keep pace with the emergence of immune escape mutations while reducing the need for frequent vaccine reformulation.
Data availability statement
The original contributions presented in the study are included in the article/Supplementary Material. Further inquiries can be directed to the corresponding authors.
Author contributions
ST: Conceptualization, Investigation, Writing – original draft. SS: Investigation, Writing – original draft, Validation. KR: Validation, Writing – original draft, Data curation, Formal analysis, Methodology, Resources, Software. MM: Methodology, Software, Validation, Conceptualization, Supervision, Writing – review & editing. SB: Visualization, Writing – original draft. SA: Data curation, Investigation, Writing – original draft. BM: Data curation, Investigation, Writing – original draft. RM: Formal analysis, Validation, Writing – original draft. YW: Project administration, Writing – review & editing. BO: Project administration, Writing – review & editing. YD: Conceptualization, Funding acquisition, Project administration, Writing – review & editing.
Funding
The author(s) declare financial support was received for the research, authorship, and/or publication of this article. This work was supported by the NIH Grants; (U54CA143727, P30GM114737, P20GM103466, U54HG013243, T32DK137523, U54MD007601, P30CA071789, P20GM139753, and U54GM138062).
Acknowledgments
The authors thank all researchers who have shared genome data openly via the Global Initiative on Sharing All Influenza Data (GISAID).
Conflict of interest
The authors declare that the research was conducted in the absence of any commercial or financial relationships that could be construed as a potential conflict of interest.
The author(s) declared that they were an editorial board member of Frontiers, at the time of submission. This had no impact on the peer review process and the final decision.
Publisher’s note
All claims expressed in this article are solely those of the authors and do not necessarily represent those of their affiliated organizations, or those of the publisher, the editors and the reviewers. Any product that may be evaluated in this article, or claim that may be made by its manufacturer, is not guaranteed or endorsed by the publisher.
Supplementary material
The Supplementary Material for this article can be found online at: https://www.frontiersin.org/articles/10.3389/fimmu.2025.1435873/full#supplementary-material
References
1. Abavisani M, Rahimian K, Mahdavi B, Tokhanbigli S, Mollapour Siasakht M, Farhadi A, et al. Mutations in SARS-CoV-2 structural proteins: a global analysis. Virol J. (2022) 19:1–19. doi: 10.1186/s12985-022-01951-7
2. Fooladinezhad H, Shahidi M, Mahmanzar M, Mahdavi B, Tokhanbigli S, Sisakht MM, et al. SARS-CoV-2 NSP3, NSP4 and NSP6 mutations and Epistasis during the pandemic in the world: evolutionary trends and natural selections in six continents. medRxiv. (2022). doi: 10.1101/2022.05.22.22275422
3. Ghaleh SS, Rahimian K, Mahmanzar M, Mahdavi B, Tokhanbigli S, Sisakht MM, et al. SARS-CoV-2 Non-structural protein 1 (NSP1) mutation virulence and natural selection: Evolutionary trends in the six continents. Virus Res. (2023) 323:199016. doi: 10.1016/j.virusres.2022.199016
4. Walls AC, Park Y-J, Tortorici MA, Wall A, Mcguire AT, Veesler D. Structure, function, and antigenicity of the SARS-CoV-2 spike glycoprotein. Cell. (2020) 183(6):1735. doi: 10.1016/j.cell.2020.11.032
5. Zhang J, Xiao T, Cai Y, Chen B. Structure of SARS-CoV-2 spike protein. Curr Opin Virol. (2021) 50:173–82. doi: 10.1016/j.coviro.2021.08.010
6. Shang J, Wan Y, Luo C, Ye G, Geng Q, Auerbach A, et al. Cell entry mechanisms of SARS-CoV-2. Proc Natl Acad Sci. (2020) 117:11727–34. doi: 10.1073/pnas.2003138117
7. Hurt AC, Wheatley AK. Neutralizing antibody therapeutics for COVID-19. Viruses. (2021) 13:628. doi: 10.3390/v13040628
8. Martinez-Flores D, Zepeda-Cervantes J, Cruz-Resendiz A, Aguirre-Sampieri S, Sampieri A, Vaca L. SARS-CoV-2 vaccines based on the spike glycoprotein and implications of new viral variants. Front Immunol. (2021) 12:701501. doi: 10.3389/fimmu.2021.701501
9. Liu L, Wang P, Nair MS, Yu J, Rapp M, Wang Q, et al. Potent neutralizing antibodies against multiple epitopes on SARS-CoV-2 spike. Nature. (2020) 584:450–6. doi: 10.1038/s41586-020-2571-7
10. Martin-Orozco N, Vale N, Mihic A, Amor T, Reiter L, Arita Y, et al. Phase I randomized, observer-blinded, placebo-controlled study of a SARS-CoV-2 mRNA vaccine PTX-COVID19-B. Sci Rep. (2023) 13:8557. doi: 10.1038/s41598-023-35662-y
11. Abbasian MH, Rahimian K, Mahmanzar M, Bayat S, Kuehu DL, Sisakht MM, et al. Comparative atlas of SARS-CoV-2 substitution mutations: A focus on Iranian strains amidst global trends. Viruses. (2024) 16:1331. doi: 10.3390/v16081331
12. Liu Y, Liu J, Johnson BA, Xia H, Ku Z, Schindewolf C, et al. Delta spike P681R mutation enhances SARS-CoV-2 fitness over Alpha variant. Cell Rep. (2022) 39(7):110829. doi: 10.1016/j.celrep.2022.110829
13. Hodcroft EB, Zuber M, Nadeau S, Vaughan TG, Crawford KH, Althaus CL, et al. Spread of a SARS-CoV-2 variant through Europe in the summer of 2020. Nature. (2021) 595:707–12. doi: 10.1038/s41586-021-03677-y
14. Kannan SR, Spratt AN, Cohen AR, Naqvi SH, Chand HS, Quinn TP, et al. Evolutionary analysis of the Delta and Delta Plus variants of the SARS-CoV-2 viruses. J Autoimmun. (2021) 124:102715. doi: 10.1016/j.jaut.2021.102715
15. Shu Y, McCauley J. GISAID: Global initiative on sharing all influenza data–from vision to reality. Eurosurveillance. (2017) 22:30494. doi: 10.2807/1560-7917.ES.2017.22.13.30494
16. Khare S, Gurry C, Freitas L, Schultz MB, Bach G, Diallo A, et al. GISAID’s role in pandemic response. China CDC weekly. (2021) 3:1049. doi: 10.46234/ccdcw2021.255
17. Rahimian K, Arefian E, Mahdavi B, Mahmanzar M, Kuehu DL, Deng Y. SARS2Mutant: SARS-CoV-2 amino-acid mutation atlas database. NAR Genomics Bioinf. (2023) 5:lqad037. doi: 10.1093/nargab/lqad037
18. Elbe S, Buckland-Merrett G. Data, disease and diplomacy: GISAID’s innovative contribution to global health. Global challenges. (2017) 1:33–46. doi: 10.1002/gch2.1018
19. Singanayagam A, Patel M, Charlett A, Bernal JL, Saliba V, Ellis J, et al. Duration of infectiousness and correlation with RT-PCR cycle threshold values in cases of COVID-19, England, January to May 2020. Eurosurveillance. (2020) 25:2001483. doi: 10.2807/1560-7917.ES.2020.25.32.2001483
20. Anderson RM, Vegvari C, Truscott J, Collyer BS. Challenges in creating herd immunity to SARS-CoV-2 infection by mass vaccination. Lancet. (2020) 396:1614–6. doi: 10.1016/S0140-6736(20)32318-7
21. Shahzamani K, Mahmoudian F, Ahangarzadeh S, Ranjbar MM, Beikmohammadi L, Bahrami S, et al. Vaccine design and delivery approaches for COVID-19. Int Immunopharmacol. (2021) 100:108086. doi: 10.1016/j.intimp.2021.108086
22. Alam I, Radovanovic A, Incitti R, Kamau AA, Alarawi M, Azhar EI, et al. CovMT: an interactive SARS-CoV-2 mutation tracker, with a focus on critical variants. Lancet Infect Dis. (2021) 21:602. doi: 10.1016/S1473-3099(21)00078-5
23. Bernasconi A, Gulino A, Alfonsi T, Canakoglu A, Pinoli P, Sandionigi A, et al. VirusViz: comparative analysis and effective visualization of viral nucleotide and amino acid variants. Nucleic Acids Res. (2021) 49:e90–0. doi: 10.1093/nar/gkab478
24. He J, Huang F, Zhang J, Chen Q, Zheng Z, Zhou Q, et al. Vaccine design based on 16 epitopes of SARS-CoV-2 spike protein. J Med Virol. (2021) 93:2115–31. doi: 10.1002/jmv.26596
25. Rella SA, Kulikova YA, Dermitzakis ET, Kondrashov FA. Rates of SARS-CoV-2 transmission and vaccination impact the fate of vaccine-resistant strains. Sci Rep. (2021) 11:15729. doi: 10.1038/s41598-021-95025-3
26. Lauring AS, Hodcroft EB. Genetic variants of SARS-CoV-2—what do they mean? JAMA. (2021) 325(6):529–31. doi: 10.1001/jama.2020.27124
27. Zhang W, Davis BD, Chen SS, Martinez JMS, Plummer JT, Vail E. Emergence of a novel SARS-CoV-2 variant in Southern California. JAMA. (2021) 325:1324–6. doi: 10.1001/jama.2021.1612
28. Bhattacharya M, Chatterjee S, Sharma AR, Agoramoorthy G, Chakraborty C. D614G mutation and SARS-CoV-2: impact on S-protein structure, function, infectivity, and immunity. Appl Microbiol Biotechnol. (2021) 105(24):9035–9045. doi: 10.1007/s00253-021-11676-2
29. Rajah MM, Hubert M, Bishop E, Saunders N, Robinot R, Grzelak L, et al. SARS-CoV-2 Alpha, Beta, and Delta variants display enhanced Spike-mediated syncytia formation. EMBO J. (2021) 40:e108944. doi: 10.15252/embj.2021108944
30. Tian D, Sun Y, Zhou J, Ye Q. The global epidemic of the SARS-CoV-2 delta variant, key spike mutations and immune escape. Front Immunol. (2021) 12:751778. doi: 10.3389/fimmu.2021.751778
31. Santra D, Banerjee A, Maiti S. Better binding informatics of delta variants (B. 1.617. 2) with ACE2 than wild, D614G or N501Y CoV-2 is fully blocked by 84 amino-acid cut of wild spike. Inf Med Unlocked. (2022) 29:100900. doi: 10.1016/j.imu.2022.100900
32. Kuzmina A, Ottolenghi A, Korovin D, Cohen-Lass I, Atari N, Hu P, et al. Changes within the P681 spike residue of the polybasic motif dictate fusogenicity and syncytia formation of delta and omicron variants of SARS-CoV-2 with no effects on neutralization or infectivity. Heliyon. (2023) 9(6):e16750. doi: 10.1016/j.heliyon.2023.e16750
33. Lubinski B, Fernandes MH, Frazier L, Tang T, Daniel S, Diel DG, et al. Functional evaluation of the P681H mutation on the proteolytic activation of the SARS-CoV-2 variant B. 1.1. 7 (Alpha) spike. Iscience. (2022) 25(1):103589. doi: 10.1016/j.isci.2021.103589
34. Ou J, Lan W, Wu X, Zhao T, Duan B, Yang P, et al. Tracking SARS-CoV-2 Omicron diverse spike gene mutations identifies multiple inter-variant recombination events. Signal Transduction Targeted Ther. (2022) 7:138. doi: 10.1038/s41392-022-00992-2
35. Follis KE, York J, Nunberg JH. Furin cleavage of the SARS coronavirus spike glycoprotein enhances cell–cell fusion but does not affect virion entry. Virology. (2006) 350:358–69. doi: 10.1016/j.virol.2006.02.003
36. Harvey WT, Carabelli AM, Jackson B, Gupta RK, Thomson EC, Harrison EM, et al. SARS-CoV-2 variants, spike mutations and immune escape. Nat Rev Microbiol. (2021) 19:409–24. doi: 10.1038/s41579-021-00573-0
37. Wang WB, Liang Y, Jin YQ, Zhang J, Su JG, Li QM. E484K mutation in SARS-CoV-2 RBD enhances binding affinity with hACE2 but reduces interactions with neutralizing antibodies and nanobodies: Binding free energy calculation studies. J Mol Graphics Model. (2021) 109:108035. doi: 10.1016/j.jmgm.2021.108035
38. Zhang Z, Wan X, Li X, Cai S, Wan C. Enhancing the immunogenicity of RBD protein variants through amino acid E484 mutation in SARS-CoV-2. Viruses. (2022) 14:2020. doi: 10.3390/v14092020
39. Chang MR, Ke H, Coherd CD, Wang Y, Mashima K, Kastrunes GM, et al. Analysis of a SARS-CoV-2 convalescent cohort identified a common strategy for escape of vaccine-induced anti-RBD antibodies by Beta and Omicron variants. EBioMedicine. (2022) 80:104025. doi: 10.1016/j.ebiom.2022.104025
40. Sun Y, Lin W, Dong W, Xu J. Origin and evolutionary analysis of the SARS-CoV-2 Omicron variant. J Biosafety Biosecur. (2022) 4:33–7. doi: 10.1016/j.jobb.2021.12.001
41. Wagenhäuser I, Reusch J, Gabel A, Krone LB, Kurzai O, Petri N, et al. Bivalent BNT162b2 mRNA original/omicron BA.4-5 booster vaccination: adverse reactions and inability to work compared with the monovalent COVID-19 booster. Clin Microbiol infection. (2023) 29(4):554–6. doi: 10.1016/j.cmi.2023.01.008
42. Chalkias S, Harper C, Vrbicky K, Walsh SR, Essink B, Brosz A, et al. A bivalent Omicron-containing booster vaccine against Covid-19. New Engl J Med. (2022) 387:1279–91. doi: 10.1056/NEJMoa2208343
43. Wu L, Peng C, Yang Y, Shi Y, Zhou L, Xu Z, et al. Exploring the immune evasion of SARS-CoV-2 variant harboring E484K by molecular dynamics simulations. Briefings Bioinf. (2022) 23:bbab383. doi: 10.1093/bib/bbab383
44. Halfmann PJ, Minor NR, Haddock Iii LA, Maddox R, Moreno GK, Braun KM, et al. Evolution of a globally unique SARS-CoV-2 Spike E484T monoclonal antibody escape mutation in a persistently infected, immunocompromised individual. Virus Evol. (2023) 9:veac104. doi: 10.1093/ve/veac104
45. Yamamoto M, Tomita K, Hirayama Y, Inoue J-I, Kawaguchi Y, Gohda J. SARS-CoV-2 Omicron spike H655Y mutation is responsible for enhancement of the endosomal entry pathway and reduction of cell surface entry pathway. BioRxiv. (2022). doi: 10.1101/2022.03.21.485084
46. Vu MN, Alvarado RE, Morris DR, Lokugamage KG, Zhou Y, Morgan AL, et al. Loss-of-function mutation in Omicron variants reduces spike protein expression and attenuates SARS-CoV-2 infection. bioRxiv. (2023). doi: 10.1101/2023.04.17.536926
47. Barton MI, Macgowan SA, Kutuzov MA, Dushek O, Barton GJ, van der Merwe PA. Effects of common mutations in the SARS-CoV-2 Spike RBD and its ligand, the human ACE2 receptor on binding affinity and kinetics. Elife. (2021) 10:e70658. doi: 10.7554/eLife.70658
48. Ballesteros-Sanabria L, Pelaez-Prestel HF, Ras-Carmona A, Reche PA. Resilience of spike-specific immunity induced by COVID-19 vaccines against SARS-CoV-2 variants. Biomedicines. (2022) 10:996. doi: 10.3390/biomedicines10050996
49. Fibke CD, Joffres Y, Tyson JR, Colijn C, Janjua NZ, Fjell C, et al. Spike mutation profiles associated with SARS-CoV-2 breakthrough infections in delta emerging and predominant time periods in British Columbia, Canada. Front Public Health. (2022) 10:915363. doi: 10.3389/fpubh.2022.915363
Keywords: D614G, P681, E484, Y655, mutation, SARS-CoV-2, spike, vaccination
Citation: Tokhanbigli S, Salami Ghaleh S, Rahimian K, Mahmanzar M, Bayat S, Ahangarzadeh S, Moradi B, Mahmanzar R, Wang Y, Oliver BGG and Deng Y (2025) Intersecting SARS-CoV-2 spike mutations and global vaccine efficacy against COVID-19. Front. Immunol. 16:1435873. doi: 10.3389/fimmu.2025.1435873
Received: 21 May 2024; Accepted: 12 February 2025;
Published: 07 March 2025.
Edited by:
Romina Salpini, University of Rome Tor Vergata, ItalyReviewed by:
Peng Wang, Coriell Institute for Medical Research, United StatesStefano D’Anna, University of Rome Tor Vergata, Italy
Copyright © 2025 Tokhanbigli, Salami Ghaleh, Rahimian, Mahmanzar, Bayat, Ahangarzadeh, Moradi, Mahmanzar, Wang, Oliver and Deng. This is an open-access article distributed under the terms of the Creative Commons Attribution License (CC BY). The use, distribution or reproduction in other forums is permitted, provided the original author(s) and the copyright owner(s) are credited and that the original publication in this journal is cited, in accordance with accepted academic practice. No use, distribution or reproduction is permitted which does not comply with these terms.
*Correspondence: Youping Deng, ZGVuZ3lAaGF3YWlpLmVkdQ==; Brian Gregory George Oliver, QnJpYW4uT2xpdmVyQHV0cy5lZHUuYXU=; Yunliang Wang, d2FuZ3l1bmxpYW5nODFAMTYzLmNvbQ==
†These authors have contributed equally to this work