- 1College of Health and Life Sciences, Hamad Bin Khalifa University, Doha, Qatar
- 2William Harvey Research Institute, Queen Mary University of London, London, United Kingdom
- 3Pharmacology and Therapeutics, Institute of Systems, Molecular and Integrative Biology, University of Liverpool, Liverpool, United Kingdom
Tumor necrosis factor alpha inhibitors (TNFi) are biologic drugs that target TNFα, a key pro-inflammatory cytokine, to suppress disease activity and alleviate symptoms of various autoimmune diseases, including inflammatory bowel disease. This review focuses on the five US FDA-approved TNFi including the monoclonal antibodies Infliximab, Adalimumab, Golimumab, Certolizumab pegol and the soluble TNFα receptor fusion protein Etanercept, with a brief mention of other available biosimilars to TNFi. The review aims to summarize the recent evidence on the pharmacokinetics, pharmacodynamics, and pharmacogenomics of TNFi with a particular focus on Human Leukocyte Antigen (HLA) variants in terms of their genetic contribution to the response to TNFi. HLA variants have been linked to heterogeneity in the efficacy and safety of TNFi among patients. Building on the summarized evidence, the last part of the review discusses the potential clinical utility of testing for pharmacogenetic variants that are linked to the response to TNFi prior to the drug prescription, and it also addresses the future directions to achieve personalized treatment for TNFi users.
1 Introduction
Tumor Necrosis Factor alpha inhibitors (TNFi) are widely used in the clinical setting to treat severe autoimmune diseases as they have shown promising efficacy and safety (1). As the name suggests, TNFi are designed to block the action of Tumor Necrosis Factor alpha (TNFα), which is one of the most potent proinflammatory cytokines. TNFα has been associated with the pathogenesis of several autoimmune diseases (ADs) including rheumatoid arthritis (RA) (2–4), inflammatory bowel disease (IBD) (5–7), psoriasis (PS) (8, 9), and ankylosing spondylitis (AS) (10), serving as a driver of chronic inflammation (11). TNFα is a type II transmembrane protein produced mainly by macrophages and to a lesser extent by T and B lymphocytes, natural killer cells, and neutrophils (12, 13). It exists in a membrane-bound form (mTNFα) and a soluble form (sTNFα) (14). Both forms of TNFα bind to specific receptors known as TNFR1 (also known as TNFRSF1A or p55) and TNFR2 (also known as TNFFSRF1B or p75); however, sTNFα has less affinity to TNFR2 (15). The transmembrane form of TNF is the prime activating ligand of the 80 kDa tumor necrosis factor receptor (16). TNFR1 is universally present on the membranes of almost all nucleated cells while TNFR2 is primarily present on membranes of immune cells, endothelial cells, and some tumor cells (17, 18). Upon binding TNFR1 and TNFR2, TNFα initiates a complex cascade of molecular signals for various biological functions and different cellular responses including inflammation, cell death, cell proliferation, and differentiation (19, 20). The signaling cascade initiated upon the activation of TNFR1 by TNFα leads to the activation of the Nuclear factor kappa-light-chain-enhancer of activated B cells (NF-κB) (21) pathway and the mitogen-activated protein kinases (MAPK) pathway (22), which subsequently induce inflammation, immune response, tissue degeneration, and cell survival and proliferation. Furthermore, the binding of TNFα to TNFR1 can mediate cell death via apoptosis or necroptosis through activating caspase-8 or mixed lineage kinase domain-like protein (MLKL), respectively. In contrast to TNFR1, TNFR2 lacks a death domain and thus does not prompt cell death directly. However, similar to TNFR1, the activation of TNFR2 stimulates NF-κB, MAPKs, and protein kinase B (AKT) pathways, promoting cell proliferation, tissue regeneration, and inflammatory responses against pathogens (23). TNFα drives inflammation by stimulating the secretion of other inflammatory cytokines, activating immune cells, and amplifying its own expression through a positive feedback loop (24). The rationale behind TNFα being a suitable target in ADs lies in its central role in inflammation and that it is “at the apex” of the signaling cascade of the pro-inflammatory cytokines (25), along with its implication in the pathogenesis of several ADs. In addition, early experiments proving the efficacy of the first TNFi, infliximab, in AD patients (26), opened the door for developing and improving anti-TNF treatment as a therapeutic approach in several ADs gradually (Figure 1).
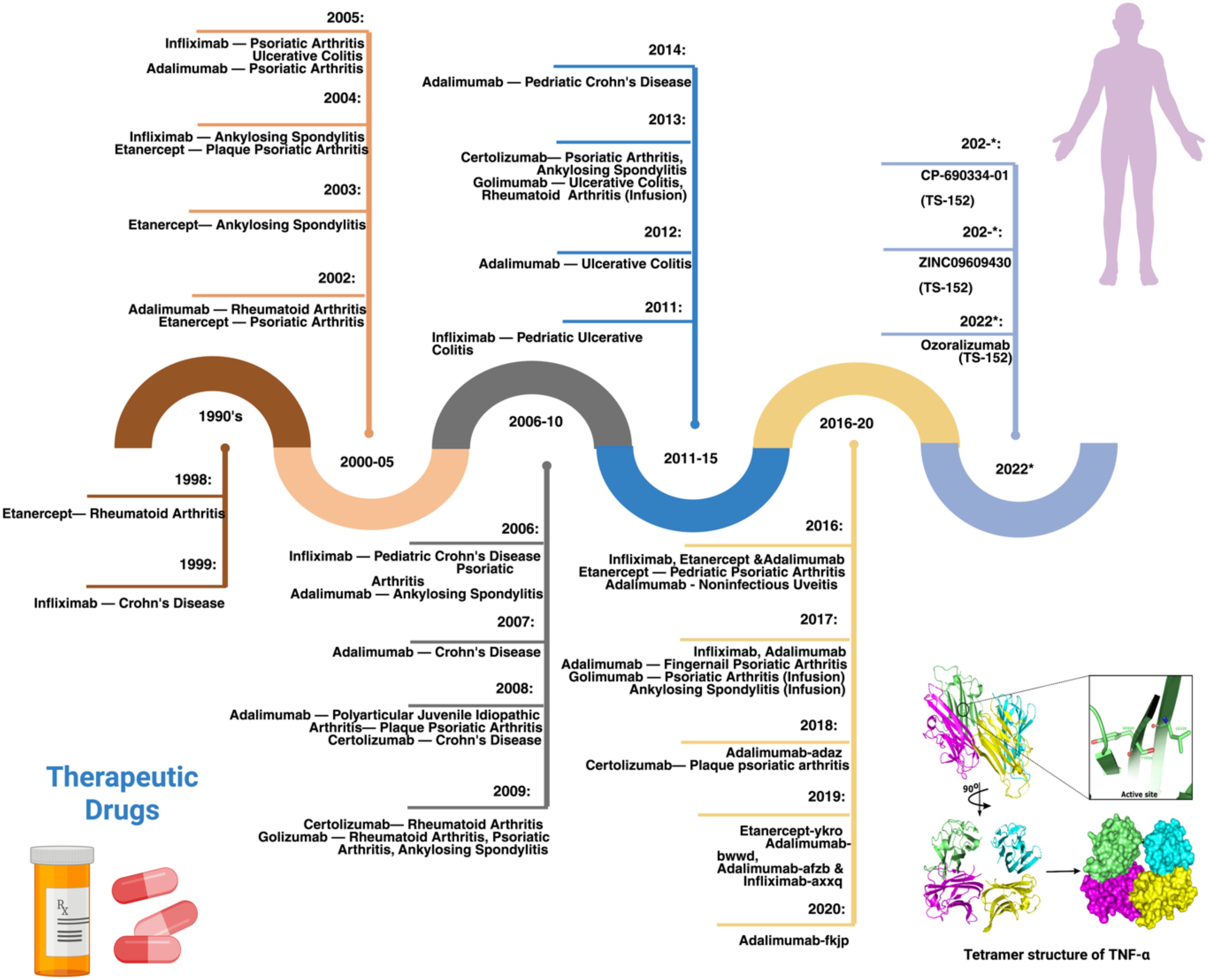
Figure 1. Timeline of the development and approval of TNF inhibitors along with the structure of TNFα. 1990s: In 1999, Infliximab was approved to treat Crohn’s disease (CD), later it was used for Rheumatoid Arthritis (RA), Ankylosing Spondylitis, and other autoimmune diseases. 2000-05: In 2002, Adalimumab was approved by the FDA to treat RA. Later, it was also used for CD, Psoriatic Arthritis, Juvenile Idiopathic and other diseases. 2006-10: In 2009, Golimumab was approved by the FDA to treat RA and used in combination with methotrexate, which was also later approved for Ankylosing Spondylitis, Ulcerative colitis, and Psoriatic arthritis treatment. 2011-15: In 2011, Infliximab was approved by the FDA for pediatric Ulcerative Colitis treatment, followed by Adalimumab and Golimumab; Adalimumab for CD. 2016-20: In 2018, Certolizumab pegol was used to treat RA, Psoriatic arthritis, CD, Ankylosing Spondylitis, and Psoriatic Arthritis. 2021-22: Ozoralizumab (TS-152) developed by Taisho research institute to treat RA.
Adalimumab, Infliximab, Certolizumab pegol, Golimumab, and Etanercept are the five TNFi approved by the US Food and Drug Administration (FDA) (27). The timeline for the development of TNFi has been comprehensively discussed in previous reviews (20, 28), from which we adapted and modified the figure presented here (Figure 1). Apart from Etanercept, which is a fusion protein that serves as a receptor for TNFα, the other four TNFi are monoclonal antibodies (mAbs). Each TNFi has two functional regions: the constant region (Fc) and the variable region (Fab), except for Certolizumab pegol, which lacks the constant region. When the Fab region of the mAb binds to TNFα, it prevents TNFα from interacting with its receptors, which is the therapeutic objective of this family of compounds (13). Although these inhibitors have been used since the past decade, the complete mechanism of action is still unclear. Previous studies have reported that TNFi neutralizes TNFα, induces direct and indirect apoptosis, modulates the immune system, induces Fc-dependent apoptosis, and promotes outside-to-inside signaling. The inflammation is reduced by the immediate neutralization reaction driven by the TNFi, which inactivates the proinflammatory cytokine TNFα (29). Nonetheless, these inhibitors appear to have more complex activities than simple blocking, owing to the complexity of the TNFα signaling. TNFi have substantially improved the treatment course of autoimmune diseases. However, response to TNFi is significantly variable, with up to 40% of patients not experiencing a positive clinical outcome. This lack of response can be classified as primary, where patients fail to respond from the outset, or secondary, where the response diminishes over time despite initial effectiveness (30).
Pharmacogenomic (PGx) variants influence how individuals respond to drugs. Variable responses to medications among ethnic groups have been attributed to the diversity of PGx variants. Previous studies highlighted that approximately 20-30% of the variability in drug efficacy and toxicity can be better elucidated by exploring PGx variants associated with drug response (31). Genetic variants have been identified in pharmacogenes that affect both pharmacokinetics and pharmacodynamics of specific drugs. Moreover, previous studies have identified that more than 90% of patients carry at least one variant in pharmacogenes that prompts a change in dosage or drug selection (32, 33). After identifying genetic loci associated with drug responses, it becomes imperative to analyze the distribution of these variants in populations, particularly because some variants, such as the human leukocyte antigen (HLA) variants, increase the risk of severe adverse effects in response to some medications. This review discusses the pharmacokinetics, pharmacodynamics, and pharmacogenomics aspects of TNFα inhibitors, with a particular focus on HLA variants.
2 Pharmacokinetics of TNF inhibitors
TNF inhibitors are protein compounds that target either membrane-bound or soluble TNF (34). These medications have complex pharmacokinetics and pharmacodynamics features due to the high affinity to their pharmacological target, compared to small-molecule medicines (35). Since TNFi are large protein molecules with low membrane permeability, they are poorly absorbed in the gastrointestinal tract. Therefore, they are parenterally administered and distributed via the lymphatic system before being metabolized and broken down into small molecules. Biologics that target soluble TNF, such as Etanercept, have a linear elimination profile, whereas biologics that target membrane-bound antigen have a non-linear elimination profile (36). The non-linear elimination of the latter category of biologics is related to target-mediated drug disposal (TMDD). One important pharmacokinetic property of TNF inhibitors is half-life (37). Etanercept has the lowest half-life of 3–5.5 days, while all other inhibitors have half-lives of ~ two weeks (38). The frequency of administration of TNFi is associated with its half-life, wherein a short half-life warrants more frequent administration. Infliximab is injected intravenously, while all other TNF inhibitors are used both intravenously and subcutaneously (39). The pharmacokinetics of TNFi is influenced by several variables as detailed in Figure 2.
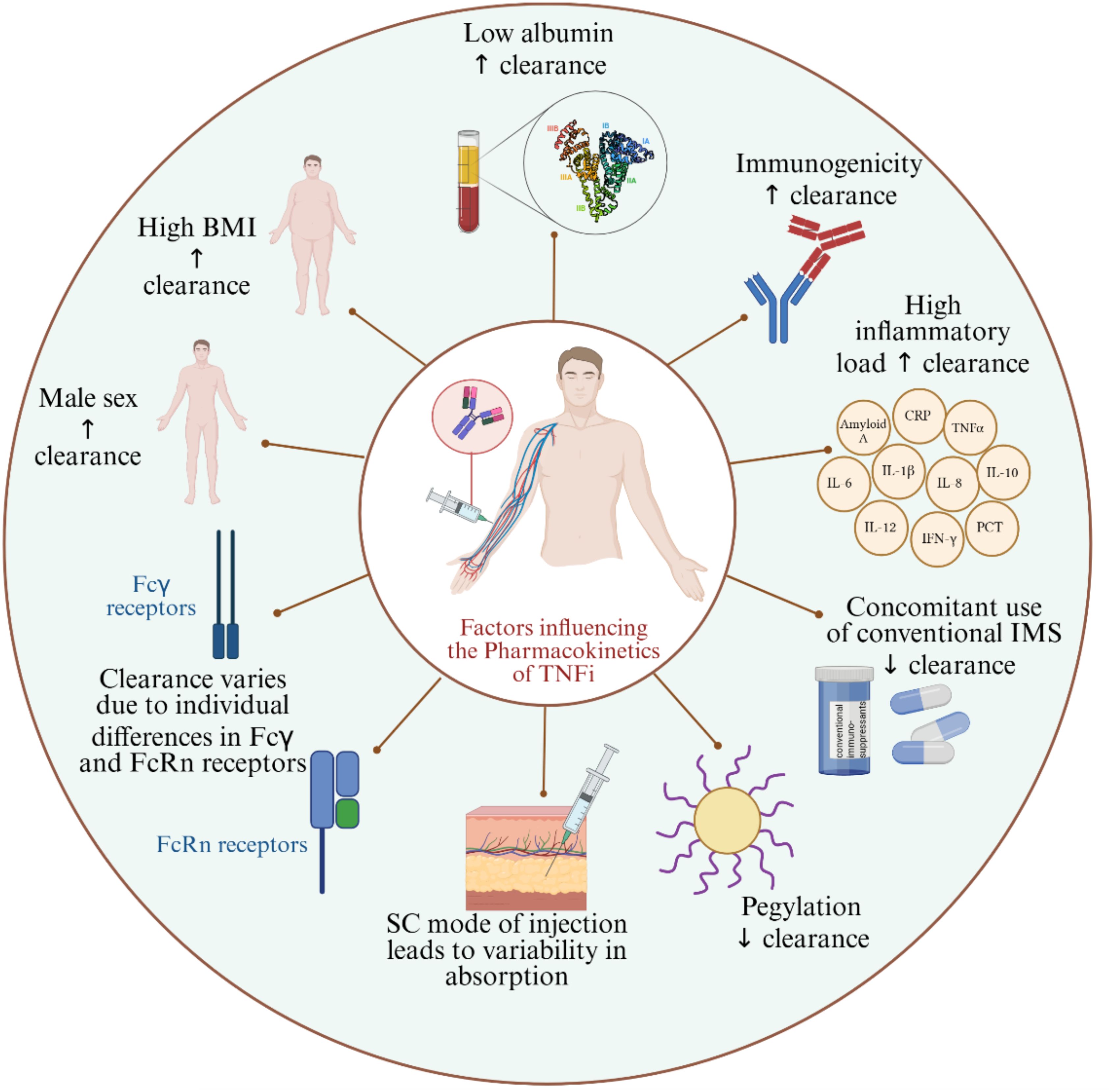
Figure 2. Factors influencing the pharmacokinetics of TNFi. BMI, Body Mass Index; SC, Subcutaneous; IMS, Immunosuppressants; FcRn, Neonatal Fc receptor; ↑: increases; ↓: decreases.
The response to TNFi is influenced by pharmacokinetic variables. Males appear to have higher clearance of TNFi compared to females (40). In general, the sex-related pharmacokinetic differences are attributed to molecular and physiological differences between males and females. To our knowledge, the available evidence on the TNFi pharmacokinetic discrepancies between males and females is observational rather than investigating the mechanisms in effect (41, 42). One plausible factor for higher drug clearance in males is that females naturally have more body fat (43); the logic behind it is that the blood circulation in fat tissues is poor, thus having more fat composition contributes to variations in drug kinetics (44). Another possible reason is the difference in innate and adaptive immune responses between males and females; for instance, the peripheral blood mononuclear cells and neutrophils (45) in men were shown to produce more TNFα than in women after stimulation by lipopolysaccharide; hence, when TNFi is bound to its target, it is cleared faster. On the contrary to normal levels of body fat, excess body fat is correlated with lower serum levels of TNFi, whereby studies have shown that people with high body mass index (BMI) have lower trough levels of TNFi compared to individuals with normal weight (46). Evidence on the mechanism behind the inverse association of obesity and serum TNFi levels is scarce; however, some studies attributed it to the amplified inflammatory status induced by obesity and to a phenomenon known as TNF sink or antigen sink (47, 48). Briefly, in an inflammatory state, the anti-inflammatory drug, in this case, TNFi, can bind to all TNF in the blood, and the unbound TNFi will be sequestered by the saturated TNF via a pattern described as a sink; studies found that this phenomenon leads to rapid clearance of TNFi (48). Furthermore, low albumin levels (49) and high levels of inflammatory biomarkers, such as C-reactive protein (CRP) (50), are associated with higher clearance of TNFi. To our knowledge, the link between hypoalbuminemia and the reduced clearance of TNFi is not clearly elaborated. It is well-established that albumin is the most abundant serum protein and that low albumin levels are associated with severe inflammatory status. One of its key roles is binding drugs and other molecules in the blood; although it does not bind large molecules, such as TNFi, it was shown to have a protective effect against the degradation of drugs, including TNFi (49, 51). This could explain why low albumin level is linked to higher clearance of TNFi. On the other hand, high CRP levels reflect the presence of inflammation, and its production is usually triggered by enhanced TNFα production as is the case in systemic inflammatory diseases; therefore, a high CRP level is linked to high serum TNFα levels, which consequently lead to accelerated clearance of TNFi (50). Another well-established factor implicated in TNFi kinetics is the development of anti-drug antibodies (ADAs), whereby lower trough levels of TNFi were reported in presence of ADAs (52). ADAs can neutralize TNFα by binding to the pharmacologically active site of the TNFi, or they can bind to TNFi without neutralizing it; both cases lead to enhanced clearance of TNFi (53–55). On the contrary, some studies found that the concomitant intake of immunomodulators (56) as well as the pegylation of TNFi (57) are linked to reduced clearance and higher drug levels. The use of concomitant immunosuppressives could prevent ADAs production and consequently lead to prolonged presence of TNFi in serum and slower clearance (58, 59). More studies are required for conclusive evidence on some of these variables, such as the concomitant use of immunomodulators (60). In terms of PEGylation, the hydrophilic nature of PEG molecules improves the solubility of therapeutic proteins, such as TNFi, and promotes higher accumulation at target sites through enhanced permeability (61, 62). In fact, PEGylation can also protect therapeutic proteins, such as TNFi, from proteolytic degradation, thereby reducing their clearance (63). Clearance of TNFi has also been shown to vary according to differences in the neonatal Fc receptor (FcRn) (64) and Fcγ receptors (65). For instance, two alleles out of five in a variable number of tandem repeats in FcRn, showed lower levels of TNFi upon initiating the treatment, whereby patients with VNTR2/VNTR3 genotype had lower levels of infliximab or adalimumab in their serum compared to patients with VNTR3/VNTR3 genotype (64). The Fcγ receptors might accelerate clearance through an increased binding of the Fc portions of therapeutic mAbs to high affinity FcgRs inducing their elimination and resulting in a rapid decrease in serum drug levels (66). Subcutaneous administration of TNFi was also found associated with variabilities in absorption and, consequently, the trough levels of the drug (67). The primary limitations of subcutaneous administration include the restriction on the volume that can be delivered (typically no more than 1 mL) compared to the intravenous route, and the heterogeneity in dosage absorption among patients, ranging from 50% to 100%, which result in greater pharmacokinetic variability between individuals and doses (60). The subcutaneous administration also slows down the absorption of the drug due to longer processing of foreign bodies in the skin (60). One study reported more stable drug levels upon subcutaneous infliximab administration compared to intravenous infliximab (68), while another study showed that intra-articular injection of TNFi was more effective than subcutaneous TNFi administration (69).
3 Pharmacodynamics of TNF inhibitors
Monoclonal TNFi antibodies interact with membrane bound TNFα, while the fusion proteins like Etanercept engage with free soluble TNF. These mechanisms prohibit TNFα from binding to its own receptors on the inflammatory cells. The interaction of TNFi with TNFα is known as TNFα neutralization, by which downstream inflammatory cytokines, such as IL-1 and IL-6, are subsequently suppressed, and the inflammatory processes subside (70). Although TNFi appear to have a selective and precise anti-inflammatory action, they can cause significant side effects such as infections and malignancies (70). This could be due to TNFα’s involvement in normal physiological processes, including tumoricidal activities and host defense. Because monoclonal antibodies can be directed against a wide range of soluble or membrane-bound targets, they can exert their pharmacological effects through several ways. Soluble TNFα exists as a circulating cytokine and is involved in systemic inflammation (71). mAbs such as infliximab, adalimumab, and golimumab primarily target soluble TNFα, neutralizing its pro-inflammatory effects by preventing its interaction with TNF receptors on immune cells. This leads to the inhibition of downstream signaling pathways, particularly the NF-κB and MAPK pathways, which are critical for the activation of immune responses, inflammation, and the recruitment of other immune cells to sites of infection or injury (72, 73). On the other hand, membrane-bound TNFα is expressed on the surface of certain immune cells, such as T-cells, macrophages, and dendritic cells (74). mAbs like etanercept, which acts as a soluble TNF receptor fusion protein, bind to both soluble and membrane bound TNFα (75). By blocking membrane-bound TNF, etanercept can modulate immune cell interactions differently than mAbs targeting only soluble TNFα. This may impact the activation of various immune responses, such as cell-mediated immunity, through the reverse signaling mechanism of membrane-bound TNF, which can influence the activation of both pro-inflammatory and anti-inflammatory pathways (29, 76). Targeting soluble TNFα can primarily dampen systemic inflammation, while blocking membrane-bound TNFα could alter immune cell functions more directly by affecting cell-cell communication and immune cell activation (77).
Moreover, the TNFi are administered parenterally and are absorbed into the bloodstream through mechanisms such as diffusion and facilitated transport at the injection site. Intravenous (IV) administration of TNFi offers 100% bioavailability since the drug is delivered directly into the bloodstream, leading to a quicker onset of action (78, 79) (Figure 3). In contrast, subcutaneous (SC) administration typically results in slower absorption and lower bioavailability, but it allows for more gradual drug delivery over time (80). Studies comparing the two routes, such as those involving infliximab (IV) and adalimumab (SC), have shown comparable therapeutic efficacy, although IV formulations are more commonly associated with infusion-related reactions (IRRs) including fever, chills, and shortness of breath (81). SC administration, on the other hand, is generally linked to local injection site reactions, such as pain or redness (82), but carries a lower risk of IRRs. Additionally, while both delivery methods activate the immune system similarly, the faster delivery associated with IV administration may lead to a more immediate immune response, whereas SC administration offers a more gradual immune modulation (83).
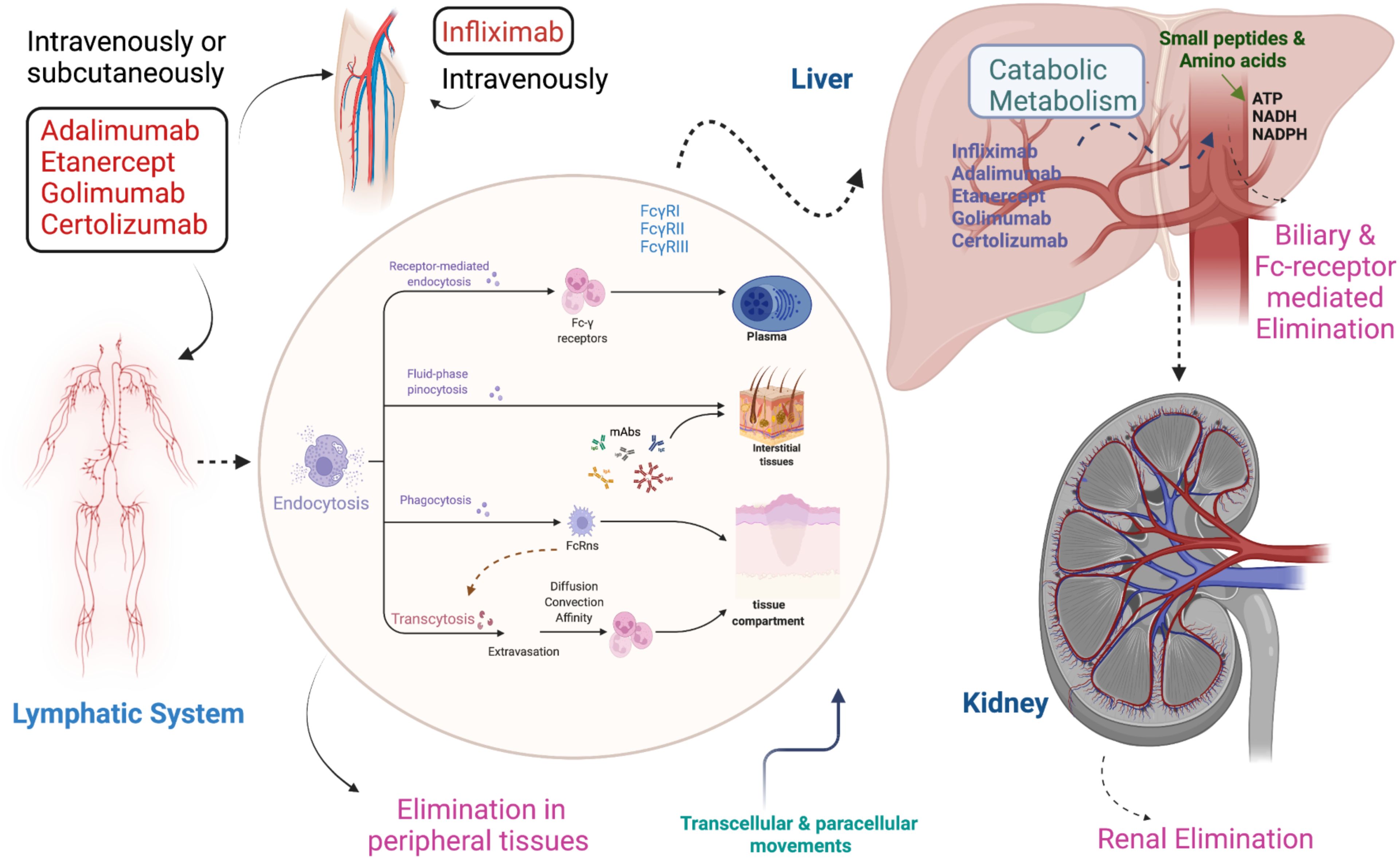
Figure 3. Pharmacokinetics and pharmacodynamics of TNF inhibitors. Infliximab is injected intravenously while other TNFi such as Adalimumab, Golimumab, Etanercept, and Certolizumab pegol are given intravenously and subcutaneously. All TNFi circulate via the lymphatic system and absorb into the body by endocytosis. Absorption occurs through different mechanisms, including receptor-mediated endocytosis, fluid phase pinocytosis, phagocytosis, and transcytosis. Metabolism occurs in the liver, while elimination takes place from the body via three different ways: 1) Peripheral tissues, 2) Biliary & Fc-receptor 3) Renal.
4 Safety and efficacy of TNF inhibitors
Some rare reports have been published that describe the adverse reactions of TNFi treatment, including tuberculosis (TB), heart failure, skin cancer, lymphoma, and the occurrence of demyelinating diseases (65). Some recent studies reported that treatment with TNFi does not increase the chance of cancer in RA patients. A study reported that all TNFi are effective; however, Etanercept is better in terms of safety compared to other TNF blockers as minimal side effects were reported (84). Another study unraveled the effectiveness and safety of anti-TNFα therapy for Juvenile Idiopathic Arthritis and reported that Adalimumab has good efficacy and safety compared to Infliximab. In contrast, Infliximab has higher efficacy as compared to Etanercept. However, Golimumab and Certolizumab have lower efficacy than these three drugs (1). A higher risk of serious infections was associated with Infliximab, Certolizumab, and Adalimumab, whereas Etanercept contributes to a lower risk of discontinuation due to adverse reactions (85). A study involving a cohort of over 42 spondyloarthritis (SpA) patients found that anti-TNF therapy demonstrated acceptable safety and a positive response rate in SpA patients (86). The safety profiles of TNFi vary among ankylosing spondylitis and rheumatoid arthritis patients. A meta-analysis conducted on ankylosing spondylitis patients found that Adalimumab and Infliximab have good clinical outcomes with Infliximab being more effective compared to Adalimumab (87). There is limited evidence on golimumab, but one study observed low infection rate during treatment with golimumab in a patient that had RA (88).
5 Heterogeneity in response to TNF inhibitors
About 30% of patients initially fail to respond to anti-TNFα therapy (primary non-responders) and up to 50% of patients lose their response during the course of treatment (secondary non-responders). The substantial proportions of patients experiencing failure of TNFi treatment whether upon initiation or at a later stage highlight the need for biomarkers that can predict response to these inhibitors. One possible clinical biomarker is serum calprotectin, which is also known as S100A8/A9 or MRP8/14 complex. A study conducted in three cohorts, one with Infliximab, one with Adalimumab, and one with Rituximab showed that TNFi responders had considerably greater baseline serum calprotectin levels than non-responders in rheumatoid arthritis patients. The study reported that the MRP8/14 (myeloid-related protein) levels were frequently higher in those who responded to targeted therapy, regardless of the mechanism of TNFi (89). Another plausible predictor could be the baseline TNFα levels; in a study conducted on 36 Crohn’s disease patients, it was found that primary non-response was related to higher TNF levels at baseline (56). Apart from clinical biomarkers, the links between genetic variants and response to TNFi were also assessed to identify genetic biomarkers. The genetic profiles of primary non-responders (PNR) have been investigated by previous studies by targeting the genes that are associated with cytokines and their receptors. For instance, associations were identified between non-response to TNFi and different genetic variants in TNFRI, IL13R2, VNTR2/VNTR3, IL23R, MAPK and FcYRIIIa (90). The secondary failure of response has been attributed in some cases to toxicity and immunogenicity. The risk of developing anti-drug antibodies is associated with several factors, including patient-related and treatment-related factors. The latter involves administration route, dosage, frequency, concomitant use of immunomodulators, and past TNFi treatment. While the individual-based factors that have been linked to immunogenicity include genetic susceptibility (91, 92), disease activity (93), BMI (56, 93, 94), and smoking status (56, 95),from a genetic perspective, the link between HLA markers in particular and immunogenicity is well-documented.
6 HLA variants and association with efficacy and toxicity to TNF inhibitor response
Adverse drug reactions (ADRs) are a major concern in healthcare. The presence of specific alleles in the human leukocyte antigen (HLA) system has been linked to the development of idiosyncratic ADRs. Understanding these connections is critical for prioritizing patient safety and customizing drug therapies. The HLA gene is the most extensively studied genetic biomarker for predicting therapeutic outcomes of rheumatoid arthritis (RA). The HLA-DRB1 gene, a disease susceptibility marker, accounts for 30-50% of the genetic risks in RA. A distinct sequence at positions 70-74, termed shared epitope, is associated with the onset and pathology of RA (96). Shared epitope positivity was found to be linked to progressive joint destruction, particularly when valine is present at position 11 of HLA-DRB1. In terms of TNFi efficacy, a study using a UK RA cohort found significant disease activity improvement with TNFi therapy when valine, lysine and alanine were at positions 11, 71, and 74 of HLA-DRB1, respectively (97). Moreover, the HLA-DQA1*05 allele has been recognized as a risk factor for the development of antibodies against anti-TNF agents. HLA-DQA1*05:01 and its extended haplotypes were associated with infliximab immunogenicity, but not adalimumab whereas HLA-DQA1*05:05 and its extended haplotypes were associated with immunogenicity to both adalimumab and infliximab, with a stronger effect for adalimumab (98). Similarly, the HLA-DRB9 g.32465390 G>T (rs2395185) G variant was also associated with IBD risk and primary non-response to Infliximab in a subset of patients with an age at diagnosis under 21 years (99). Moreover, other HLA variants such as HLA-DQA1 g.32622994 T>A/T>C (rs2097432) and HLA-DRB1 g.32465390 G>T (rs2395185) were associated with long-term anti-TNF drug response in children with IBD, highlighting their potential as predictive biomarkers for therapy outcomes (100). Previous studies recommend testing HLA-DRB1*3, HLA-DQA1*05:01, and HLA-DQA1*05:05 to predict immunogenicity to anti-TNF drugs; prior genetic testing for these variants could aid in therapy selection and preventive strategies (101). Moreover, another study reported that HLA-B rs41563412-GCA (p.Ser33LeufsTer9) homozygous carriers did not respond to adalimumab whereas HLA-DRB1 rs1071752-C (p.Gln125Gln) heterozygous and homozygous carriers showed response to infliximab (102). These two variants are situated in the exonic and intronic regions of the HLA-DRB1 and HLA-B genes, respectively; both are an integral part of the adaptive immune system. These genes encode pivotal proteins for presenting antigens to CD8+ (in MHC-1) and CD4+ T-cells (MHC-II) in a dependent manner, shaping the altered adaptive immune response observed in Crohn’s disease (CD) patients. While genetic factors influencing clinical CD phenotypes may diverge from those related to anti-TNF response, the HLA region stands as a prominent risk locus for CD. Numerous genetic association studies have underscored the significant association between variants in HLA-B and HLA-DRB1 with the development of CD. In contrast to CD4+ T-cells, antigen presentation to CD8+ T-cells follows MHC-I restrictions. Specifically, the HLA-B g. 31356966 (rs41563412) variant, located in the intronic region of the HLA-B gene, aligns with this MHC-I family. Notably, the sole homozygous carrier of the pathogenic HLA-B rs41563412-GCA variant, featuring a frameshift mutation (p. Ser33LeufsTer9), exhibited unresponsiveness to anti-TNF therapy. This suggests the potential involvement of this loss-of-function variant in modulating the CD8+ cytotoxic immune response, emphasizing its role in therapeutic outcomes (102).
Pharmacogenomics studies principally focusing on discovering variants in the HLA region and their associations with TNFi response, are reviewed in Table 1 (98, 100, 103–110, 115). The assessment of genetic profiles of autoimmune patients is essential for identifying such HLA variants to enhance clinical diagnosis and implement personalized treatment.
7 Other pharmacogenomic variants associated with TNF inhibitors
Several clinical and genome-wide association studies investigated the link between genetic variants and response to TNFi across various autoimmune diseases (Table 2). A meta-analysis, focused on spondyloarthropathy, psoriasis and Crohn’s disease, identified that the G allele in TNF -238G>A (rs361525), TNF -308G>A (rs1800629) and the C allele in TNF -857C>T(rs1799724) showed better response to TNFα blockers in the European population, but failed to show a significant impact in the Asian population (119, 144). Another meta-analysis conducted on individuals of European population assessed the association between SNPs and response to anti-TNF-α therapy across major autoimmune diseases, including psoriasis, rheumatoid arthritis, inflammatory bowel disease, and spondyloarthritis. They identified six SNPs in the FCGR2A (rs1801274), FCGR3A (rs396991), TNF (rs361525, rs1800629, rs1799724), and TNFRSF1B (rs1061622) genes that were significantly associated with treatment response, primarily within disease-specific subgroups. However, no single pharmacogenetic marker was consistently predictive across all conditions, highlighting the need for further research to identify robust biomarkers for guiding anti-TNF therapy (120).
In RA, a study conducted on 1752 patients treated with Infliximab in the UK found that the FTO g.54026293G>A (rs7195994) was related to Infliximab response (116). Another study examined variants in NKG2D in 280 RA patients undergoing anti-TNF therapy. NKG2D g.10379727A>G(rs2255336) and NKG2D g.10372766C>G (rs1049174) variants showed significant associations of CC or GG genotypes to poor response (p=0.003, p=0.004 respectively). Moreover, the GG genotype was associated with non-response in etanercept-treated patients after 12 weeks (124). The genetic link to the response to TNFi has also been investigated in IBD patients. A study of an Italian cohort reported that IBD patients possessing a variant in FCGR3A exhibited diminished clinical response by the end of the induction period. Moreover, they observed a remarkable correlation between the FCGR3A variant and median Infliximab levels during maintenance therapy, revealing that patients with the wild-type genotype demonstrated elevated Infliximab levels compared to those with the variant allele. Additionally, individuals with the variant allele displayed an increased likelihood of developing antidrug antibodies (145). Another longitudinal study conducted on 132 Crohn’s disease patients identified that SNPs including TLR2 g.153688371T>C(rs1816702) and TLR2 c.597T>C (rs3804099) were linked with long-term response to Infliximab, whereas IL6 c.6331T>C (rs10499563) correlated with supratherapeutic and infratherapeutic Infliximab levels (117). They also identified that genotypes AG and GG in TNFRSF1B has been associated with lower response to adalimumab and infliximab. In pediatric Crohn’s disease patients treated with Infliximab, studies reported that genotypes TNFRSF1B CC>CT (rs3397) was associated with the anti-Infliximab antibody production, suggesting its potential in identifying patients prone to immunogenicity (146). More variants were recently associated with long-term response to infliximab or adalimumab in IBD pediatric patients, including rs10508884 (CXCL12), rs2241880 (ATG16L1), and rs6100556 (PHACTR3); these three SNPs were linked to poor long-term response to TNFi (92). Certain other variants were associated with disease type or drug type; for instance, rs6908425 (CDKAL1) was associated with poor response in CD, while rs2188962 (IRF1-AS1), rs2241880 and rs6100556, were associated with worse outcomes in ulcerative colitis (UC). Moreover, rs2241880 was linked to poor response to both infliximab and adalimumab, whereas rs6100556 correlated with poor response to infliximab specifically (92). Furthermore, in a genetic association study cohort comprised of 474 IBD patients with European ancestry, two loci were found to be significantly associated with response to TNFi and were replicated with a p <1 x 10–03 in a validation cohort: rs116724455 in TNFSF4/18 and rs2228416 in PLIN2. Allele C of rs116724455, located on chromosome 1 near the TNFSF4 and TNFSF18 genes, was strongly associated with nonresponse to TNFi (OR = 19.9, p = 4.79×10−⁸). Likewise, allele T of rs2228416 on chromosome 9 near the PLIN2 and HAUS6 genes, was also linked to nonresponse (OR = 5.25, p = 5.24×10−⁶) (147). Four other SNPs showed suggestive association with the response to TNFi; those include rs762787 (allele T) near LTF, CCR5, and CCRL2, rs9572250 (allele G) near KLHL1, rs144256942 (allele G) near PROX1 and RPS6KC1 and rs523781 (allele G) near RORB and TRPM6 (147). In a very recent study, certain genetic variants were associated with the likelihood of achieving steroid-free remission (SFR) in a small cohort of European IBD patients (83 patients) treated with infliximab or adalimumab (148). Patients carrying the GG genotype of rs1800629 (p = 0.025) and the AA genotype of rs1061624 (p = 0.029) had significantly higher odds of achieving SFR compared to those who did not. In contrast, patients with the AA genotype of rs361525 or the CC genotype of rs767455 were significantly less likely to achieve SFR. Moreover, the allele A of rs1800629 was more frequent in patients who discontinued TNFi treatment and was linked to a higher risk of early therapy interruption (148).
Similar to RA and IBD patients, studies have identified genetic variants linked to variable response to TNFi in psoriasis patients; one example is the TNFRSF1B c.196T>G (rs1061622) SNP, which was associated with a higher risk of poor response to TNFi in psoriasis patients of European ancestry (139). Remarkably, the G allele of rs1061622 was found more frequent in patients carrying the allele HLA-CW6*0602 (149). In a more recent study, a candidate variant, rs1991820, was identified via a retrospective study approach involving 1849 psoriasis patients (149). Although it did not reach genome-wide significance, rs1991820 (an intronic variant of KLK7 gene) showed a promising association with positive response to TNFi (p = 1.30 × 10–6). The study found that rs1991820 is significantly correlated with elevated expression of KLK7 in the skin tissue of psoriatic patients and that TNFi treatment gradually reduced KLK7 expression levels (149). In a study involving 312 ankylosing spondylitis patients treated with Etanercept, patients with specific genotypes (CYP2C9*3, CYP2D6*10, and CYP3A5*3) showed lower joint swelling, erythrocyte sedimentation rate, and C-reactive protein levels after 24 weeks of Etanercept treatment. The CYP2D6*10/*10 and CYP3A5*3/*3 diplotypes were associated with higher efficacy scores, suggesting a potential correlation between genetic variations and Etanercept treatment response in these patients (142). In one study of 74 Italian Behçet syndrome patients receiving anti-TNFα therapy, the TNF -308G>A (rs1800629) variant was found to be associated with treatment response; GG genotype prevalence was significantly higher among responders (86.2%) compared to non-responders (56.3%) (150). Well-characterized cohorts with larger samples size are needed for further validation of TNF -308G>A (rs1800629) as a predictive biomarker for TNFi response. A study on an Italian cohort found a significant association between the TNF -308G>A (rs1800629) and clinical remission without steroids in pediatric patients receiving infliximab therapy. Additionally, the study identified a potential link between carriers of the HLA-DQA1*05 and a higher risk of developing anti-TNFα immunogenicity (151). A previous study conducted on Psoriasis patients from the European population reported that variants in the TNF -857C and TNFRSF1B 676T genes were associated with positive response when treated with Etanercept. Moreover, no significant associations were found between variants in these genes and treatment outcomes for infliximab or adalimumab (121). Another study conducted on European population reported that variants in IL1B (rs1143623, rs1143627), LY96 (rs11465996), TLR2 (rs11938228, rs4696480) and TLR9 (rs352139) were associated with response to Adalimumab, Infliximab and Etanercept (122). Furthermore, a recent study conducted on 738 European patients with IBD using a candidate gene approach identified 19 functional polymorphisms in genes involved in NFκB activation via TLR pathways, TNF-α signaling, and NFκB-regulated cytokines as significant predictors of response to anti-TNF therapy. The study highlighted that a genetically strong TNF-mediated inflammatory response was associated with favorable treatment outcomes, while also suggesting alternative cytokine targets such as IL-1β, IL-6, and IFN-γ for non-responders (136). These findings suggest that pharmacogenetic monitoring could become an essential tool in tailoring anti-TNF therapies for both pediatric and adult populations.
8 Clinical implications
Autoimmune disease treatment poses significant clinical and economic challenges, including decisions on when to start targeted therapy and which drug class to select. The decision of prescribing TNFi therapies is often driven by general guidelines (described below), pricing incentives, and habitual prescribing patterns (152). One study reported that 98.8% of participating rheumatologists (n= 248) expressed interest in a predictive test for inadequate TNFi response, noting that such a tool would influence their treatment decisions and patient management. The study also highlighted that 71% of rheumatologists were concerned that inadequate response to TNFi therapies result in patients paying for ineffective treatments. The current prescribing practices entail patients switching between multiple TNFi drugs before switching to drugs with different mechanisms of action (152, 153). This approach raises healthcare costs, extends high disease activity, and negatively impacts quality of life. According to the guidelines of the American College of Rheumatology, the physician’s decision to initiate or switch biologic DMARDs should consider a patient’s disease activity, prior DMARD use, and comorbidities (154). Studies investigating the predictors of biologic treatment in RA patients have shown variable findings (130). Some findings suggest that biologic initiation is influenced by previous glucocorticoid or non-biologic DMARD use (155). Other studies highlight the association between sociodemographic factors, such as lower income and older age, and accessibility to biologics. The differences in study design, population, and disease stage have led to inconsistent conclusions. While most research efforts in this area focused on initiating biologics, evidence on switching between biologics is scarce. When the optimal treatment is delayed, patients are subjected to a poor quality of life and several risks, including adverse events, disease progression, and chronic pain. The clinical applications of pharmacogenomics of TNFi entail personalized drug prescription and dosage based on the genetic profile of the patient, prediction of response prior to treatment initiation, and prediction of the risk of adverse events and immunogenicity before they occur, aiming to achieve personalized treatment and better health outcomes.
8.1 Individualized dosing
The relationship between the dosage and the serum concentration of biologics is not linear due to many implicated factors pertaining to the patient and the drug. Although a personalized approach based on genetic variation has not yet been developed for TNFi dosage, the association between genetic variation and drug dosage is well established. Genetic variations can affect the metabolism and clearance of drugs. By analyzing specific genes involved in drug metabolism, healthcare providers can tailor the dosage of TNFi to match the patient’s genetic profile. One of the promising examples of how personalized TNFi dosage can improve clinical outcomes is the Bayesian Dashboard approach, which is designed to consider pharmacokinetic factors that affect drug clearance (156). The model aims to provide individualized dosage plans tailored to maintain the treatment goal of drug concentration or trough levels as set by the physician. Due to the complex and multifactorial nature of the association between patient’s traits and the pharmacokinetics of TNFi, the Bayesian Dashboard approach models adjusting for these multi-factors. It achieves that by employing multiple functions to analyze new patient data and generating two predictions (157). Briefly, the model is first built using the pharmacokinetic parameters of the population, then it is refined by adding covariates data, such as the patient’s sex, BMI, disease activity, CRP level, albumin level, etc. A standard prediction is derived from the population parameters and patient traits. Afterwards, the model is updated with individual data to balance the population data and train the model on personalized data; the individual data includes information on drug concentration and the development of anti-drug antibodies (ADAs) during the course of the treatment. Then, the models can be averaged, or one model is selected to predict the dose and clearance of the drug in a personalized manner (individual prediction). For robust prediction, ADAs are included as a factor in the model because they can alter the pharmacokinetics of TNFi by binding to them and neutralizing them, or by increasing the drug clearance after forming a complex with TNFi (55). The standard prediction is beneficial for patients without prior drug concentration measurements, while the individual prediction aids in managing patients with existing drug concentration data (157). In regard to TNFi, several studies demonstrated the success of Bayesian Dashboard-based dosing in enhancing the clinical outcome of patients receiving Infliximab compared to standard dosing (158–160). For instance, 88% of patients with IBD maintained remission after precision dosing of Infliximab using a Bayesian-dashboard model compared to IBD patients following a standard therapeutic plan (64%) (160). In a real-life care situation involving 108 patients from a single center, a Bayesian Dashboard model was used to forecast personalized dose of infliximab. Based on the model, a higher dose was prescribed in 34 patients, a lower dose was prescribed in 9 patients, while infliximab administration was discontinued in 16 patients. As a result, the overall remission rate increased from 65.7% to 80.0% (158). Although the Bayesian-dashboard model does not involve pharmacogenomic variants, it serves as proof of the clinical utility and application of precision dosage on the outcomes of the disease.
The Bayesian Dashboard model has its advantages and disadvantages. As explained previously, the model includes multi-factors to accommodate the complexity of the link between these factors and drug dose and concentrations. Moreover, the model tends to be flexible, and this is reflected by the ability to refine it and update it with clinical values once available. Also, if a certain value was missing, the model can use the most recent value instead to perform the prediction. In other words, if CRP and albumin levels were not available, for example, they can be added at a later stage; and if CRP value was missing for a certain dose, the model will use the most recent one instead to predict the drug concentration during that period (157). Nevertheless, the Bayesian Dashboard model has some limitations. The model requires the values of 3 concentrations at different time points for the prediction to be maximally accurate. Furthermore, the robustness of the model is dependent on the size of the population data incorporated, and it was tested only with infliximab among the five TNFi. The cost-effectiveness of the model is also not assessed yet (157).
The clinical significance of pharmacogenomic variants on response to some drugs is solid and well-documented; however, the clinical significance of genetics variants associated with response to TNFi lacks validation before being implemented in clinical practice. Nonetheless, the genetic association to response to TNFi is extensively studied and reported. One example is the association reported by Salvadore-Martin et al, whereby SNP rs1816702 at TLR2g. 153688371(rs1816702) was identified as a potential biomarker for TNFi treatment plan in IBD pediatric patients (161). Four other SNPs, were also detected in association with subtherapeutic, supratherapeutic or absolute trough values of Adalimumab or Infliximab; the SNPs were TLR4g.117712004 G > A (rs5030728), LY96g.73989727C>G (rs11465996), TNFRSF1B g.12207235 C>T (rs3397), and CD14 g.140633331A> G (rs2569190) (161). Patients carrying these variants in this study exhibited different responses to the same TNFi; for instance, patients carrying allele T at TLR2g. 153688371(rs1816702) or allele G at CD14 g.140633331(rs2569190) had higher levels of serum adalimumab, while patients carrying allele C at TNFRSF1B g.12207235 C>T (rs3397) had lower serum levels of adalimumab. These associations became insignificant after removing patients with intensified drug dosage from the cohort; the authors referred this loss of association to low sample size (n=48), particularly because the impact of the first two SNPs was reported previously. The study also proposes that patients carrying genotype TT in TLR2 g. 153688371(rs1816702) could benefit from adalimumab treatment more than infliximab (161). Another example is the association between variants in NOD2 and response to TNFi; several studies evaluated this correlation, for example, Juanola et al, examined three SNPs in NOD2 gene, whereby patients carrying any of the three variants needed an intensified TNFi regime due to loss of response (162). While such studies are promising, the clinical significance and utility of genetic polymorphisms in terms of response to TNFi still lack validation in large sample sizes to prove their robustness.
8.2 Identification of responders and non-responders
Pharmacogenomic insights can help identify patients who are likely to respond well to a particular drug, allowing for more targeted and effective treatment strategies. In parallel, early identification of non-responders can prevent unnecessary exposure to potentially ineffective medications. A plethora of genetic variants have been linked to the response to TNFi, and the variability of TNFi efficacy among patients was associated with specific genotypic variation. The association between HLA-B27 genotype and the response to TNFi has been examined in that regards for the treatment of axial spondyloarthritis, whereby HLA-B27 positivity predicted the reduction of Bath Ankylosing Spondylitis Disease Activity Index (BASDAI) within 6 months [OR = 2.11, 95% CI: 1.25, 3.56) (P = 0.005)]. In addition, HLA-B27 positivity predicted improved drug survival (HR = 1.76, 95% CI: 1.07, 2.89) (P = 0.027) (163). Nonetheless, HLA-B27 genotype’s utility and impact on predicting response is not straightforward; it was reported to have less weight in the prediction power compared to other factors such as the sex, inflammatory status and severity of the disease (112). However, HLA-B27 can aid in predicting the success of TNFi therapy after taking into consideration other implicated factors such as the gender and CRP levels of the patients (112). The mechanism of how HLA-B27 genotype is linked to TNFi response is not elucidated, but previous studies reported that patients with positive HLA-B27 had lower TNFα production by the T cells, and the mechanism behind it is unknown (164).
The known genetic associations to TNFi response have not been utilized in clinical settings yet; however, promising findings on the clinical utility of genotype have emerged. One illustration is generating Genetic Risk Scores (GRSs) based on a combination of clinical and genetic factors to predict response to TNFi; Barber et al. demonstrated that a GRS composed of 31 distinct SNPs can be used to predict primary non-response and durable response with higher accuracy than prediction based on clinical factors solely (90). Similarly, a risk model and a preliminary “anti-TNF refractory score” designed by Wang et al. that included clinical and genetic predictors, highlighted that adding the genetic factors enhanced the predictability of the model. The genetic compartment of the model involved 18 novel genome-wide significant loci in the TNFSF4 gene, along with several other suggestive loci that correlated to the response to TNFi. The predictability of the model, represented by the area under the curve, raised from 0.72 to 0.89 upon adding the genetic markers to the model. The anti-TNF refractory score, which involved clinical factors along with the genotypes of the most significantly associated loci, differentiated between responders and non-responders to TNFi, with better accuracy metrics than when clinical data is used solely. After adding the genetic data, the specificity increased from 75% to 95%, the positive predictive value increased from 19% to 50%, the negative predictive value increased from 95% to 96%, while sensitivity exceptionally dropped from 61% to 56%. Hence, the study proposes the possible utility of such models as a precision medicine tool for predicting response to anti-TNF treatment, after validating the power of the model in an independent cohort (147). Furthermore, another research team proposed a model based on Gaussian Process Regression (GPR) (165). The model incorporates clinical, demographic, and genetic data of rheumatoid arthritis (RA) patients to anticipate variations in Disease Activity Score in 28 joints (DAS28). Due to heterogeneity among patients, the model matches the data of patients from the testing group to the data of patients in the training set to predict changes in DAS28 and classify patients as responders and non-responders. The model succeeded in predicting the response to TNFi of 78% of the patients (with an AUC of ~0.66). The GPR model works properly with heterogeneous datasets, commonly encountered in cross-sectional studies, in which disease variability and limited sample sizes are often the challenges. GPR addresses this by matching patients with similar conditions through its kernel function and predicts the response to treatment per subpopulations. Unlike complex machine learning algorithms that lack interpretability for clinical applications, GPR uses intuitive similarity-based modeling, and its results can be easily interpreted by the physicians. Moreover, the model’s additive design facilitates integrating new features with minimal parameter adjustment and provides confidence intervals, which are valuable for physicians. However, GPR lacks built-in feature selection available in linear methods. To overcome this disadvantage, the study used a preselected set of genetic features validated through cross-validation with clinical data. Although the clinical features had a higher prediction power than the genetic features, the genetic data undeniably enhanced the prediction power of the model when it was combined with the clinical data (165). One drawback of the GPR model is lack of generalization, as it was designed using data of European patients, so the model needs to be refined before it is used for other populations (165).
Apart from genetic markers, the clinical utility of transcriptomics data was also evaluated, in which a machine learning prediction model for response to TNFi was designed based on gene expression data (166). The study tested various machine learning models (linear, non-linear, and kernel-based) to predict response to TNFi based on clinical data, flow cytometry data, proteomic data, and transcriptomic data. The models showed high prediction power; particularly, the transcriptomics-based model had a higher power in classifying non-responders than the clinical-based and proteomics-based models (166). The findings of these models should be validated in a larger cohort as the sample size in this study was small, especially the non-responder cohort; also, only female patients were included in this study to reduce heterogeneity, thus the proposed models need to be tested using male samples to examine its generalizability (166). The strength of genetic markers over clinical features is also highlighted in another study in which clinical data and imputed gene expression profiles from genome-wide genotype data were utilized to predict non-durable (short-term) response to TNFi through machine learning models. The top three significant features were the genetic markers (DPY19L3, GSTT1, and NUCB1) and not the clinical features (167).
Moreover, the power of combining genetic and transcriptomic data was also assessed in terms of predicting response to TNFi, whereby gene expression and SNPs data derived from RNA sequencing were combined along with clinical data to design a multifaceted algorithm comprised of 70 features in total (168). Although SNPs information is typically derived from whole genome sequencing (WGS), RNAseq data can also offer valuable insights into functionally active SNPs. In other words, SNP identification using RNA-seq data of over-expressed genes offers advantages over WGS, as it focuses on transcribed regions, which enhances detecting functionally significant SNPs located in coding exons, untranslated regions (UTRs), and introns (169). Upon combining these SNPs with gene expression profiles, they have the potential to significantly improve the accuracy and effectiveness of molecular signature predictions. This study involved SNPs derived from RNA-seq data and that are linked to rheumatoid arthritis and are associated with differential gene expression in peripheral blood mononuclear cells. Out of the 70 features used to train the prediction model, it used 23 features to classify responders and non-responders, including 8 transcripts, 10 SNPs, CRP and anti-CCP levels, gender, BMI, and patient disease assessment. The study involved 58 American female RA patients in the discovery cohort, while the training and validation cohorts were comprised of 145 and 175 patients, respectively. The model differentiated patients who are likely to have a poor response to TNFi with a positive predictive value (PPV) of 89.7% (95% CI 79.0–95.7%), specificity of 86.8% (95% CI 72.4–94.1%), and sensitivity of 50.0% (95% CI 40.8–58.7%). The authors in this study have not addressed the low sensitivity of the model although it misses half of the true non-responders. The study highlights that 70% of the unstratified patients didn’t achieve a 50% improvement after receiving TNFi treatment and that the patients didn’t achieve a 50% improvement after receiving TNFi treatment, therefore, the model could have saved half of them from taking TNFi by predicting the inefficacy of the treatment in these patients (168). Despite the model’s strong PPV and specificity, its low sensitivity highlights the need to refine the model to better identify all true non-responders; increasing sensitivity could be achieved by integrating additional biomarkers or expanding the dataset to include larger cohort. Several other research groups attempted to design prediction tools/models to anticipate the response to anti-TNFα treatment in the realm of precision medicine; however, those models still need further testing, validation in large cohorts, and enhancements before they can generate optimal prediction and be used in clinics.
Multi-omic analyses have also provided key insights into treatment responses in psoriasis. For example, a previous study applied a multi-omics approach to characterize treatment response to the Etanercept in patients with severe psoriasis (170). By integrating transcriptomic, proteomic and systems biology analyses across blood and skin at multiple timepoints, they revealed molecular signatures linked to therapeutic response. Importantly, the expression of TNF-regulated genes in both lesional skin and peripheral blood showed significant correlation with clinical outcomes. Despite conventional skepticism toward blood-based biomarkers in dermatology, the study highlighted the predictive potential of baseline blood samples. These findings provide not only valuable candidate biomarkers but also designed a scalable analytical framework for larger biomarker discovery efforts such as the full-scale Psoriasis Stratification to Optimise Relevant Therapy (PSORT) study (170).
8.3 Predicting and preventing adverse events and/or immunogenicity
As highlighted Table 1, genetic variants, such as variants in the HLA region, have been identified to be associated with adverse reactions after TNFi therapy. Immunogenicity is defined as an immune response triggered by a biologic drug that manifests as production of anti-drug antibodies (ADAs). It is one of the mechanisms behind treatment failure, by which ADAs would either neutralize the binding site of the biologic drug or increase its clearance, leading to lower therapeutic levels of the drug. As discussed earlier, previous evidence shows that immunogenicity has a genetic component, and several genetic variants, such as HLA variants, were associated with the development of ADAs in response to TNFi. Variants in CXCL12, IL-10, and Fc gamma receptor (FCGR3A) genes were also linked to ADAs in patients receiving TNFi. Therefore, predicting immunogenicity in susceptible patients can assist physicians in providing a personalized treatment plan to minimize the risk. A recent study showed that Crohn’s disease patients who had free antibodies to Infliximab responded poorly to an intensified drug dose compared to patients without free antibodies to the drug. The findings proposed that free antibodies level, but not total antibodies, could be a plausible biomarker of response to Infliximab. Furthermore, ADAs developed in response to Infliximab or Adalimumab were previously used to predict the discontinuation of treatment within 24 months after initiating the therapy with sensitivity of 62% and 79%, and specificities and positive predictive values of 100% (171).
However, immunogenicity does not always lead to drug inefficacy, which makes the prediction of drug inefficacy based on ADAs titer challenging. Nevertheless, ADAs have been linked to several adverse reactions. For example, a higher incidence of reactions to infusion and in the injection sites were reported in patients who developed ADAs compared to their counterparts who didn’t have ADAs (172). Moreover, switching to another biologic drug has been found effective in some patients who developed ADAs to specific biologics, especially because biologics have different immunogenicity levels. The immunogenicity levels of different TNFi vary, with some agents showing higher rates of antibody formation compared to others (54). These differences can impact treatment efficacy and the risk of adverse reactions in patients with inflammatory diseases such as rheumatoid arthritis, psoriasis, and inflammatory bowel disease. For example, studies have shown that monoclonal antibody-based TNFi, such as Infliximab and Adalimumab, tend to have higher immunogenicity rates compared to soluble receptor-based TNFi like Etanercept (173). In addition to genetic variants, transcriptomic data, such as mucosal TNFα expression level, was also reported to be used as a possible biomarker of response to TNFi and generation of ADAs. The subdued expression of mucosal TNFα at diagnosis was correlated with better response to TNFi (174). Although all of this sounds promising, to our knowledge, no model has been designed yet to examine the utility of genetic variants in predicting immunogenicity or adverse events in response to TNFi, and the associated genetic variants are still lacking validation before genetic testing is implemented in the clinics for this purpose.
8.4 Key challenges that impede validation of biomarkers
Despite the compelling evidence of the impact of genetic variants on response to TNFi, genetic testing has not been integrated into the clinical practice of prescribing TNFi due to a lack of replication and validation of the identified genetic biomarkers. The challenges behind this could be several. A quite obvious reason is the heterogeneity among patients in terms of medical conditions, lifestyle, and treatment plans. The treatment plan varies based on the condition of each patient, and most patients end up taking concomitant drugs; this type of inter-individual variability limits the power of the study. Other study design-related challenges that face the discovery of robust genetic biomarkers are the sample size and lack of standardization. For instance, to our knowledge, none of the GWAS and meta-analyses that have been published on the response of TNFi in rheumatoid arthritis exceeded 3000 participants; the largest cohort that a meta-analysis assessed was 2706 patients (175). This limitation likely contributes to the failure to replicate earlier candidate gene findings. Furthermore, unstandardized handling procedures could also affect the validity and replication of identified biomarkers, such procedures include collection, transportation, processing, and storage of samples. Another factor could be the limitation of clinical biomarkers in reflecting the true response to TNFi; for example, the disease score DAS-28, which is used to assess the disease activity in rheumatoid arthritis, has been criticized with some limitations despite its practicality and validity in the practice of rheumatology clinics (176, 177). The limitation primarily arises from the “general health” component incorporated in DAS-28 calculations; the general health is a subjective assessment provided by the patient, which makes it more prone to bias and measurement errors. Studies have reported cases where the general health score was high while all other components of DAS-28 didn’t reflect an active disease (178). While it is important to account for patient-reported assessment of the disease severity, given that autoimmune diseases are multifactorial, other more reliable patient-reported measures should be explored to replace the general health score in DAS-28 assessment. Furthermore, discrepancies in swollen-joint count have been reported due to differences in the training and experience of the physician assessing it, a lack of standardized assessment techniques, obscure definitions of swelling, or variations in the severity of joint deformities (177). Moreover, an overlooked factor could be the lack of patients’ adherence to TNFi; a previous study found that around 27% of patients receiving TNFi reported that they did not follow their treatment regimen, ending up with failing to respond (179). While reasons of non-adherence were not known in that study, another study discussed that non-adherence to TNFi could be for practical reasons or for perceptions that patients have regarding the treatment; the practical reasons include the administration route of the treatment and the cost of the treatment; whereas, the perceptual reasons include worries of side effects and unawareness of the patient about the importance of adherence and the consequences of non-adherence (180).
9 Future directions
As discussed in the previous section, several limitations need to be addressed before achieving a valid personalized approach for patients on TNFi. Concisely, the main factors to be addressed are reliability, standardization, and sufficient statistical power to discern authentic and robust associations. Investigating a sole aspect is not enough to explain the variability in response to TNFi. Therefore, future study designs must develop models that integrate genetic, clinical, demographic, and environmental data to capture the intricate heterogeneity among patients and achieve robust accuracy, specificity, and sensitivity values. This comes hand-in-hand with increasing sample size and studying diverse populations. As a matter of fact, in 2020 the pharmacogenomics GWAS represented around 7% only of all published GWAS, and the majority of these studies predominantly focused on European populations (181). The GWAS on response to TNFi is even more scarce; hence, large-scale GWAS of response to TNFi are needed in diverse and less represented populations. Moreover, patient stratification is another area that needs improvement, whereby previous studies primarily aimed to distinguish between responders and non-responders whilst grouping patients of various response, such as optimal response and moderate response, in one category under “good responders”. This might have been due to limited sample size, which reiterates the necessity of larger cohorts to achieve significant results. Similarly, it is equally essential to predict primary or secondary non-response to TNFi. As a matter of fact, secondary non-response results in many cases from immunogenicity-related causes, and models that predict immunogenicity are still lacking. Hence, patients who will develop a secondary failure of TNFi might be faultily categorized as responders. Designing models that can predict immunogenicity and secondary non-response would be helpful for the physicians in prescribing a less immunogenic TNFi or other complementary treatments that can prevent the probability of developing antidrug antibodies. In fact, the temporal change in response to TNFi necessitates longitudinal study designs to assess the response to treatment, particularly because a significant proportion of patients tend to lose their response to TNFi almost a year after the initiation of the treatment. Whilst most studies would evaluate patients’ response within 3 to 6 months, more studies focusing on the long-term response are required to capture the variability in response spectrum among patients and achieve a tailored treatment plan. Furthermore, from a precision medicine approach, future investigation of the mechanisms behind the failure of response to TNFi is also crucial, especially in the absence of cure for autoimmune diseases, whereby non-responders to TNFi suffer from a poor quality of life.
Emerging technologies such as machine learning (ML), single-cell genomics, and multi-omics, hold great promise in revolutionizing the prediction of TNFi response in patients. Previous studies have employed machine learning models to predict TNF inhibitor use in rheumatologic conditions (165–167). These studies typically analyze baseline clinical and laboratory data to identify patients likely to benefit from early TNF inhibitor therapy. ML approaches such as artificial neural networks (ANN) (165), have demonstrated superior predictive performance compared to traditional methods like logistic regression and support vector machines. ML with its ability to process large, complex datasets, can uncover non-obvious patterns within genetic, clinical, and environmental data (182). By training models on patient-specific characteristics, ML algorithms can predict who is more likely to respond to TNFi treatment, allowing for more personalized and effective therapeutic strategies (166).
Moreover, single-cell genomics enables the study of individual cells within heterogeneous tissue samples, offering detailed insights into immune cell dynamics at the molecular level. This technology is especially valuable in identifying rare or previously undetected cell populations that may play critical roles in drug response or resistance (183). By isolating and analyzing immune cells from patients on TNFi therapy, single-cell genomics can pinpoint specific immune pathways or cell types contributing to treatment efficacy or adverse effects, ultimately guiding better-targeted therapies. Furthermore, multi-omics approaches, which integrate data from genomics, transcriptomics, proteomics, and metabolomics (184), provide a comprehensive view of the biological processes that underlie drug response. These approaches can help identify novel biomarkers that predict treatment outcomes and uncover complex interactions between genes, proteins, and metabolites that influence how patients respond to TNFi. By combining data across multiple biological layers, multi-omics can reveal the full complexity of TNFi treatment responses, helping to tailor therapies to individual patients based on their unique molecular profiles. Together, these technologies represent the future of TNFi response prediction, offering the potential for more accurate, personalized treatment plans that improve patient outcomes while minimizing adverse effects. Moreover, the successful integration of TNFi response prediction into clinical practice requires collaboration between regulatory agencies, clinicians, and patients. Regulatory agencies like the FDA and EMA must validate predictive biomarkers and diagnostic tools, ensuring they meet safety and efficacy standards. Clinicians need to incorporate these tools into daily practice, interpreting complex data to make informed decisions tailored to individual patients. Patients must be educated on how genetic testing, and predictive models can help in tailoring a personalized treatment plan and adhering to it is part of the therapy; this is important to ensure informed consent and active participation in the decision-making process. Ultimately, close collaboration among these stakeholders will ensure the effective and safe implementation of personalized TNFi therapies.
10 Conclusion
Although TNFα inhibitors have improved the quality of life of many patients with autoimmune diseases, a significant proportion of patients remain in a progressive disease state and poor quality of life due to the failure of anti-TNFα therapy. Previous evidence demonstrated that the variability in response to TNFi is associated with genetic variants. Therefore, integrating a pharmacogenomic therapeutic approach seems to be promising for personalized treatment plans. As scientific research advances in this area, identifying robust genetic biomarkers to predict the response and the risk of adverse reactions to TNFi would ultimately improve the quality of care for patients with autoimmune diseases.
Author contributions
ZJ: Formal analysis, Investigation, Methodology, Writing – original draft, Writing – review & editing. FE: Data curation, Formal analysis, Investigation, Writing – original draft, Writing – review & editing. DV: Project administration, Supervision, Validation, Writing – original draft, Writing – review & editing. BM: Funding acquisition, Supervision, Writing – original draft, Writing – review & editing. PJ: Conceptualization, Funding acquisition, Project administration, Supervision, Writing – original draft, Writing – review & editing.
Funding
The author(s) declare that financial support was received for the research and/or publication of this article. The authors thank the College of Health & Life Sciences, Hamad Bin Khalifa University as well as the QNRF (now the QRDI Council) and the QGP (now the QPHI) for funding (PPM 04-0312-200037). The funders had no role in the development of this manuscript.
Conflict of interest
The authors declare that the research was conducted in the absence of any commercial or financial relationships that could be construed as a potential conflict of interest.
Generative AI statement
The author(s) declare that no Generative AI was used in the creation of this manuscript.
Publisher’s note
All claims expressed in this article are solely those of the authors and do not necessarily represent those of their affiliated organizations, or those of the publisher, the editors and the reviewers. Any product that may be evaluated in this article, or claim that may be made by its manufacturer, is not guaranteed or endorsed by the publisher.
References
1. Li Y, Mao X, Tang X, and Mao H. Efficacy and safety of anti-TNFα Therapy for uveitis associated with juvenile idiopathic arthritis: A systematic review and meta-analysis. Rheumatol Ther. (2021) 8:711–27. doi: 10.1007/s40744-021-00296-x
2. Matsuno H, Yudoh K, Katayama R, Nakazawa F, Uzuki M, Sawai T, et al. The role of TNF-α in the pathogenesis of inflammation and joint destruction in rheumatoid arthritis (RA): a study using a human RA/SCID mouse chimera. Rheumatology. (2002) 41:329–37. doi: 10.1093/rheumatology/41.3.329
3. Vasanthi P, Nalini G, and Rajasekhar G. Role of tumor necrosis factor-alpha in rheumatoid arthritis: a review. APLAR J Rheumatol. (2007) 10:270–4. doi: 10.1111/j.1479-8077.2007.00305.x
4. Farrugia M and Baron B. The role of TNF-α in rheumatoid arthritis: a focus on regulatory T cells. J Clin Transl Res. (2016) 2:84–90. doi: 10.18053/jctres.02.201603.005
5. Leppkes M, Roulis M, Neurath MF, Kollias G, and Becker C. Pleiotropic functions of TNF-α in the regulation of the intestinal epithelial response to inflammation. Int Immunol. (2014) 26:509–15. doi: 10.1093/intimm/dxu051
6. Sands BE and Kaplan GG. The role of TNFα in ulcerative colitis. J Clin Pharma. (2007) 47:930–41. doi: 10.1177/0091270007301623
7. Breese EJ, Michie CA, Nicholls SW, Murch SH, Williams CB, Domizio P, et al. Tumor necrosis factor α-producing cells in the intestinal mucosa of children with inflammatory bowel disease. Gastroenterology. (1994) 106:1455–66. doi: 10.1016/0016-5085(94)90398-0
8. Kane D and FitzGerald O. Tumor necrosis factor-α in psoriasis and psoriatic arthritis: A clinical, genetic, and histopathologic perspective. Curr Rheumatol Rep. (2004) 6:292–8. doi: 10.1007/s11926-004-0041-0
9. Victor FC, Gottlieb AB, and Menter A. Changing paradigms in dermatology: tumor necrosis factor alpha (tnf-α) blockade in psoriasis and psoriatic arthritis. Clinics Dermatol. (2003) 21:392–7. doi: 10.1016/j.clindermatol.2003.08.015
10. Gorman JD, Sack KE, and Davis JC. Treatment of ankylosing spondylitis by inhibition of tumor necrosis factor α. N Engl J Med. (2002) 346:1349–56. doi: 10.1056/NEJMoa012664
11. Andretto V, Dusi S, Zilio S, Repellin M, Kryza D, Ugel S, et al. Tackling TNF-α in autoinflammatory disorders and autoimmune diseases: From conventional to cutting edge in biologics and RNA- based nanomedicines. Advanced Drug Delivery Rev. (2023) 201:115080. doi: 10.1016/j.addr.2023.115080
12. Pennica D, Nedwin GE, Hayflick JS, Seeburg PH, Derynck R, Palladino MA, et al. Human tumour necrosis factor: precursor structure, expression and homology to lymphotoxin. Nature. (1984) 312:724–9. doi: 10.1038/312724a0
13. Sedger LM and McDermott MF. TNF and TNF-receptors: From mediators of cell death and inflammation to therapeutic giants - past, present and future. Cytokine Growth Factor Rev. (2014) 25:453–72. doi: 10.1016/j.cytogfr.2014.07.016
14. Black RA, Rauch CT, Kozlosky CJ, Peschon JJ, Slack JL, Wolfson MF, et al. A metalloproteinase disintegrin that releases tumour-necrosis factor-α from cells. Nature. (1997) 385:729–33. doi: 10.1038/385729a0
15. Horiuchi T, Mitoma H, Harashima S-i, Tsukamoto H, and Shimoda T. Transmembrane TNF- : structure, function and interaction with anti-TNF agents. Rheumatology. (2010) 49:1215–28. doi: 10.1093/rheumatology/keq031
16. Yang S, Wang J, Brand DD, and Zheng SG. Role of TNF-TNF receptor 2 signal in regulatory T cells and its therapeutic implications. Front Immunol. (2018) 9:784. doi: 10.3389/fimmu.2018.00784
17. Chu W-M. Tumor necrosis factor. Cancer Lett. (2013) 328:222–5. doi: 10.1016/j.canlet.2012.10.014
18. Ye L-L, Wei X-S, Zhang M, Niu Y-R, and Zhou Q. The significance of tumor necrosis factor receptor type II in CD8+ Regulatory T cells and CD8+ Effector T cells. Front Immunol. (2018) 9:583. doi: 10.3389/fimmu.2018.00583
19. Gough P and Myles IA. Tumor necrosis factor receptors: pleiotropic signaling complexes and their differential effects. Front Immunol. (2020) 11:585880. doi: 10.3389/fimmu.2020.585880
20. Jang D-I, Lee A-H, Shin H-Y, Song H-R, Park J-H, Kang T-B, et al. The role of tumor necrosis factor alpha (TNF-α) in autoimmune disease and current TNF-α Inhibitors in therapeutics. Int J Mol Sci. (2021) 22:2719. doi: 10.3390/ijms22052719
21. Wajant H and Scheurich P. TNFR1-induced activation of the classical NF-κB pathway. FEBS J. (2011) 278:862–76. doi: 10.1111/j.1742-4658.2011.08015.x
22. Wajant H, Pfizenmaier K, and Scheurich P. Tumor necrosis factor signaling. Cell Death Differ. (2003) 10:45–65. doi: 10.1038/sj.cdd.4401189
23. Wajant H and Siegmund D. TNFR1 and TNFR2 in the control of the life and death balance of macrophages. Front Cell Dev Biol. (2019) 7:91. doi: 10.3389/fcell.2019.00091
24. Zhang T, Li H, Shi J, Li S, Li M, Zhang L, et al. p53 predominantly regulates IL-6 production and suppresses synovial inflammation in fibroblast-like synoviocytes and adjuvant-induced arthritis. Arthritis Res Ther. (2016) 18:271. doi: 10.1186/s13075-016-1161-4
25. Monaco C, Nanchahal J, Taylor P, and Feldmann M. Anti-TNF therapy: past, present and future. Int Immunol. (2015) 27:55–62. doi: 10.1093/intimm/dxu102
26. Feldmann M and Maini RN. TNF defined as a therapeutic target for rheumatoid arthritis and other autoimmune diseases. Nat Med. (2003) 9:1245–50. doi: 10.1038/nm939
27. Medler J and Wajant H. Tumor necrosis factor receptor-2 (TNFR2): an overview of an emerging drug target. Expert Opin Ther Targets. (2019) 23:295–307. doi: 10.1080/14728222.2019.1586886
28. Leone GM, Mangano K, Petralia MC, Nicoletti F, and Fagone P. Past, present and (Foreseeable) future of biological anti-TNF alpha therapy. JCM. (2023) 12:1630. doi: 10.3390/jcm12041630
29. Billmeier U, Dieterich W, Neurath MF, and Atreya R. Molecular mechanism of action of anti-tumor necrosis factor antibodies in inflammatory bowel diseases. World J Gastroenterol. (2016) 22:9300–13. doi: 10.3748/wjg.v22.i42.9300
30. Kalden JR and Schulze-Koops H. Immunogenicity and loss of response to TNF inhibitors: implications for rheumatoid arthritis treatment. Nat Rev Rheumatol. (2017) 13:707–18. doi: 10.1038/nrrheum.2017.187
31. Zhou Y and Lauschke VM. Population pharmacogenomics: an update on ethnogeographic differences and opportunities for precision public health. Hum Genet. (2021) 141:1113–36. doi: 10.1007/s00439-021-02385-x
32. Hicks JK, El Rouby N, Ong HH, Schildcrout JS, Ramsey LB, Shi Y, et al. Opportunity for genotype-guided prescribing among adult patients in 11 US health systems. Clin Pharma Ther. (2021) 110:179–88. doi: 10.1002/cpt.2161
33. Ramsey LB, Ong HH, Schildcrout JS, Shi Y, Tang LA, Hicks JK, et al. Prescribing prevalence of medications with potential genotype-guided dosing in pediatric patients. JAMA Netw Open. (2020) 3:e2029411. doi: 10.1001/jamanetworkopen.2020.29411
34. Siegmund D and Wajant H. TNF and TNF receptors as therapeutic targets for rheumatic diseases and beyond. Nat Rev Rheumatol. (2023) 19:576–91. doi: 10.1038/s41584-023-01002-7
35. Dömling A and Li X. TNF-α: The shape of small molecules to come? Drug Discov Today. (2022) 27:3–7. doi: 10.1016/j.drudis.2021.06.018
36. Berkhout LC, I’Ami MJ, Kruithof S, Vogelzang EH, Hooijberg F, Hart MHL, et al. Formation and clearance of TNF-TNF inhibitor complexes during TNF inhibitor treatment. Br J Pharmacol. (2023) 181(8):1165–81. doi: 10.1111/bph.16269
37. Kontermann RE. Half-life extended biotherapeutics. Expert Opin Biol Ther. (2016) 16:903–15. doi: 10.1517/14712598.2016.1165661
38. Campanati A, Paolinelli M, Diotallevi F, Martina E, Molinelli E, and Offidani A. Pharmacodynamics OF TNF α inhibitors for the treatment of psoriasis. Expert Opin Drug Metab Toxicol. (2019) 15:913–25. doi: 10.1080/17425255.2019.1681969
39. Jinesh S. Pharmaceutical aspects of anti-inflammatory TNF-blocking drugs. Inflammopharmacology. (2015) 23:71–7. doi: 10.1007/s10787-015-0229-0
40. Fasanmade AA, Adedokun OJ, Ford J, Hernandez D, Johanns J, Hu C, et al. Population pharmacokinetic analysis of infliximab in patients with ulcerative colitis. Eur J Clin Pharmacol. (2009) 65:1211–28. doi: 10.1007/s00228-009-0718-4
41. Klein SL and Morgan R. The impact of sex and gender on immunotherapy outcomes. Biol Sex Differ. (2020) 11:24. doi: 10.1186/s13293-020-00301-y
42. Younes S. The relationship between gender and pharmacology. Curr Res Pharmacol Drug Discov. (2024) 7:100192. doi: 10.1016/j.crphar.2024.100192
43. Zucker I and Prendergast BJ. Sex differences in pharmacokinetics predict adverse drug reactions in women. Biol Sex Differ. (2020) 11:32. doi: 10.1186/s13293-020-00308-5
44. Cheymol G. Effects of obesity on pharmacokinetics: implications for drug therapy. Clin Pharmacokinetics. (2000) 39:215–31. doi: 10.2165/00003088-200039030-00004
45. Aomatsu M, Kato T, Kasahara E, and Kitagawa S. Gender difference in tumor necrosis factor-α production in human neutrophils stimulated by lipopolysaccharide and interferon-γ. Biochem Biophys Res Commun. (2013) 441:220–5. doi: 10.1016/j.bbrc.2013.10.042
46. De Wolff L, Arends S, Brouwer E, Bootsma H, and Spoorenberg A. High BMI is associated with lower TNF-α inhibitor serum trough levels and higher disease activity in patients with axial spondyloarthritis. Arthritis Res Ther. (2023) 25:202. doi: 10.1186/s13075-023-03187-4
47. Singh S, Facciorusso A, Singh AG, Casteele NV, Zarrinpar A, Prokop LJ, et al. Obesity and response to anti-tumor necrosis factor-α agents in patients with select immune-mediated inflammatory diseases: A systematic review and meta-analysis. PloS One. (2018) 13:e0195123. doi: 10.1371/journal.pone.0195123
48. Keizer RJ, Huitema ADR, Schellens JHM, and Beijnen JH. Clinical pharmacokinetics of therapeutic monoclonal antibodies. Clin Pharmacokinetics. (2010) 49:493–507. doi: 10.2165/11531280-000000000-00000
49. Kim ES, Kwon Y, Choe YH, and Kim MJ. Albumin-to-Globulin Ratio at 1 Year after Anti-Tumor Necrosis Factor α Therapy Can Serve as a Prognostic Biomarker in Pediatric Crohn’s Disease Patients. Gut Liver. (2022) 16:71–80. doi: 10.5009/gnl20322
50. Ternant D, Ducourau E, Perdriger A, Corondan A, Le Goff B, Devauchelle-Pensec V, et al. Relationship between inflammation and infliximab pharmacokinetics in rheumatoid arthritis. Brit J Clin Pharma. (2014) 78:118–28. doi: 10.1111/bcp.12313
51. Schoenefuss F and Hoffmann P. Serum γ-globulin and albumin concentrations predict secondary loss of response to anti-TNFα in inflammatory bowel disease patients. Eur J Gastroenterology Hepatology. (2019) 31:1563–8. doi: 10.1097/MEG.0000000000001493
52. Wade JR, Parker G, Kosutic G, Feagen BG, Sandborn WJ, Laveille C, et al. Population pharmacokinetic analysis of certolizumab pegol in patients with Crohn’s disease. J Clin Pharmacol. (2015) 55:866–74. doi: 10.1002/jcph.491
53. Moots RJ, Xavier RM, Mok CC, Rahman MU, Tsai W-C, Al-Maini MH, et al. The impact of anti-drug antibodies on drug concentrations and clinical outcomes in rheumatoid arthritis patients treated with adalimumab, etanercept, or infliximab: Results from a multinational, real-world clinical practice, non-interventional study. PloS One. (2017) 12:e0175207. doi: 10.1371/journal.pone.0175207
54. Atiqi S, Hooijberg F, Loeff FC, Rispens T, and Wolbink GJ. Immunogenicity of TNF-inhibitors. Front Immunol. (2020) 11:312. doi: 10.3389/fimmu.2020.00312
55. Mehta P and Manson JJ. What is the clinical relevance of TNF inhibitor immunogenicity in the management of patients with rheumatoid arthritis? Front Immunol. (2020) 11:589. doi: 10.3389/fimmu.2020.00589
56. Kennedy NA, Heap GA, Green HD, Hamilton B, Bewshea C, Walker GJ, et al. Predictors of anti-TNF treatment failure in anti-TNF-naive patients with active luminal Crohn’s disease: a prospective, multicentre, cohort study. Lancet Gastroenterol Hepatol. (2019) 4:341–53. doi: 10.1016/S2468-1253(19)30012-3
57. McDonnell T, Ioannou Y, and Rahman A. PEGylated drugs in rheumatology–why develop them and do they work? Rheumatology. (2014) 53:391–6. doi: 10.1093/rheumatology/ket278
58. Van Schaik T, Maljaars JPW, Roopram RK, Verwey MH, Ipenburg N, Hardwick JCH, et al. Influence of combination therapy with immune modulators on anti-TNF trough levels and antibodies in patients with IBD. Inflammatory Bowel Dis. (2014) 20:2292–8. doi: 10.1097/MIB.0000000000000208
59. Xu Z, Davis HM, and Zhou H. Clinical impact of concomitant immunomodulators on biologic therapy: Pharmacokinetics, immunogenicity, efficacy and safety. J Clin Pharma. (2015) 55:S60–74. doi: 10.1002/jcph.380
60. Ordás I, Mould DR, Feagan BG, and Sandborn WJ. Anti-TNF monoclonal antibodies in inflammatory bowel disease: pharmacokinetics-based dosing paradigms. Clin Pharmacol Ther. (2012) 91:635–46. doi: 10.1038/clpt.2011.328
61. Weir ANC, Nesbitt A, Chapman AP, Antoniw AGPP, and Lawson ADG. Formatting antibody fragments to mediate specific therapeutic functions. Biochem Soc Trans. (2002) 30:512–6. doi: 10.1042/bst0300512
62. Tun GS and Lobo AJ. Evaluation of pharmacokinetics and pharmacodynamics and clinical efficacy of certolizumab pegol for Crohn’s disease. Expert Opin Drug Metab Toxicol. (2015) 11:317–27. doi: 10.1517/17425255.2015.995166
63. Vugmeyster Y, Entrican CA, Joyce AP, Lawrence-Henderson RF, Leary BA, Mahoney CS, et al. Pharmacokinetic, biodistribution, and biophysical profiles of TNF nanobodies conjugated to linear or branched poly(ethylene glycol). Bioconjugate Chem. (2012) 23:1452–62. doi: 10.1021/bc300066a
64. Billiet T, Dreesen E, Cleynen I, Wollants W-J, Ferrante M, Van Assche G, et al. A genetic variation in the neonatal fc-receptor affects anti-TNF drug concentrations in inflammatory bowel disease. Am J Gastroenterology. (2016) 111:1438–45. doi: 10.1038/ajg.2016.306
65. Pauline O, Robert M, Bernardeau C, Hlavaty A, Fusaroli M, Roustit M, et al. Assessment of reported adverse events after interchanging between TNF-α Inhibitor biosimilars in the WHO pharmacovigilance database. BioDrugs. (2023) 37:699–707. doi: 10.1007/s40259-023-00603-8
66. Mahmoud I, Moalla M, Ben Tekaya A, Charfi R, Rouached L, Bouden S, et al. Assessment of the influence of Fc-γ receptor polymorphisms on biologics’ pharmacokinetics in Tunisian rheumatoid arthritis patients. Brit J Clin Pharma. (2023) 89:1834–43. doi: 10.1111/bcp.15658
67. Turner MR and Balu-Iyer SV. Challenges and opportunities for the subcutaneous delivery of therapeutic proteins. J Pharmaceutical Sci. (2018) 107:1247–60. doi: 10.1016/j.xphs.2018.01.007
68. Little RD, Ward MG, Wright E, Jois AJ, Boussioutas A, Hold GL, et al. Therapeutic drug monitoring of subcutaneous infliximab in inflammatory bowel disease—Understanding pharmacokinetics and exposure response relationships in a new era of subcutaneous biologics. JCM. (2022) 11:6173. doi: 10.3390/jcm11206173
69. Zhang F and Ma C. Comparison of the effectiveness on intra-articular and subcutaneous TNF inhibitor in rheumatoid arthritis patients. Clin Rheumatol. (2018) 37:199–204. doi: 10.1007/s10067-017-3806-3
70. Kalliolias GD and Ivashkiv LB. TNF biology, pathogenic mechanisms and emerging therapeutic strategies. Nat Rev Rheumatol. (2016) 12:49–62. doi: 10.1038/nrrheum.2015.169
71. Zelová H and Hošek J. TNF-α signalling and inflammation: interactions between old acquaintances. Inflammation Res. (2013) 62:641–51. doi: 10.1007/s00011-013-0633-0
72. Fischer R, Kontermann RE, and Pfizenmaier K. Selective targeting of TNF receptors as a novel therapeutic approach. Front Cell Dev Biol. (2020) 8:401. doi: 10.3389/fcell.2020.00401
73. Wong M, Ziring D, Korin Y, Desai S, Kim S, Lin J, et al. TNFα blockade in human diseases: Mechanisms and future directions. Clin Immunol. (2008) 126:121–36. doi: 10.1016/j.clim.2007.08.013
74. Deora A, Hegde S, Lee J, Choi C-H, Chang Q, Lee C, et al. Transmembrane TNF-dependent uptake of anti-TNF antibodies. mAbs. (2017) 9:680–95. doi: 10.1080/19420862.2017.1304869
75. Marotte H and Cimaz R. Etanercept – TNF receptor and IgG1 Fc fusion protein: is it different from other TNF blockers? Expert Opin Biol Ther. (2014) 14:569–72. doi: 10.1517/14712598.2014.896334
76. Mitoma H, Horiuchi T, Tsukamoto H, and Ueda N. Molecular mechanisms of action of anti-TNF-α agents – Comparison among therapeutic TNF-α antagonists. Cytokine. (2018) 101:56–63. doi: 10.1016/j.cyto.2016.08.014
77. Stow JL and Murray RZ. Intracellular trafficking and secretion of inflammatory cytokines. Cytokine Growth Factor Rev. (2013) 24:227–39. doi: 10.1016/j.cytogfr.2013.04.001
78. Hedaya MA. Routes of drug administration. In: Pharmaceutics. Netherlands: Elsevier (2024), ISBN: ISBN:978-0-323-99796-6. p. 537–54. doi: 10.1016/B978-0-323-99796-6.00006-0
79. Adepu S and Ramakrishna S. Controlled drug delivery systems: current status and future directions. Molecules. (2021) 26:5905. doi: 10.3390/molecules26195905
80. Davis JD, Bravo Padros M, Conrado DJ, Ganguly S, Guan X, Hassan HE, et al. Subcutaneous administration of monoclonal antibodies: pharmacology, delivery, immunogenicity, and learnings from applications to clinical development. Clin Pharma Ther. (2024) 115:422–39. doi: 10.1002/cpt.3150
81. Roselló S, Blasco I, García Fabregat L, Cervantes A, and Jordan K. Management of infusion reactions to systemic anticancer therapy: ESMO Clinical Practice Guidelines. Ann Oncol. (2017) 28:iv100–18. doi: 10.1093/annonc/mdx216
82. Gidudu J, Kohl KS, Halperin S, Hammer SJ, Heath PT, Hennig R, et al. A local reaction at or near injection site: Case definition and guidelines for collection, analysis, and presentation of immunization safety data. Vaccine. (2008) 26:6800–13. doi: 10.1016/j.vaccine.2008.10.006
83. Jain KK. An overview of drug delivery systems. In: Jain KK, editor. Drug delivery systems. Springer New York, New York, NY (2020). p. 1–54. doi: 10.1007/978-1-4939-9798-5_1ISBN:978-1-4939-9797-8
84. Aaltonen KJ, Joensuu JT, Virkki L, Sokka T, Aronen P, Relas H, et al. Rates of serious infections and Malignancies among patients with rheumatoid arthritis receiving either tumor necrosis factor inhibitor or rituximab therapy. J Rheumatol. (2015) 42:372–8. doi: 10.3899/jrheum.140853
85. Michaud TL, Rho YH, Shamliyan T, Kuntz KM, and Choi HK. The comparative safety of tumor necrosis factor inhibitors in rheumatoid arthritis: a meta-analysis update of 44 trials. Am J Med. (2014) 127:1208–32. doi: 10.1016/j.amjmed.2014.06.012
86. Plasencia C, Pascual-Salcedo D, García-Carazo S, Lojo L, Nuño L, Villalba A, et al. The immunogenicity to the first anti-TNF therapy determines the outcome of switching to a second anti-TNF therapy in spondyloarthritis patients. Arthritis Res Ther. (2013) 15:R79. doi: 10.1186/ar4258
87. Liu W, Wu Y-H, Zhang L, Liu X-Y, Xue B, Liu B, et al. Efficacy and safety of TNF-α inhibitors for active ankylosing spondylitis patients: Multiple treatment comparisons in a network meta-analysis. Sci Rep. (2016) 6:32768. doi: 10.1038/srep32768
88. Bessette L, Rahman P, Kelsall J, Purvis J, Rampakakis E, Lehman AJ, et al. Real-world incidence and determinants of infection in patients with rheumatoid arthritis treated with golimumab after a median follow-up time of 27 months. J Rheumatol. (2023) 50:1121–6. doi: 10.3899/jrheum.2022-1283
89. Choi IY, Gerlag DM, Herenius MJ, Thurlings RM, Wijbrandts CA, Foell D, et al. MRP8/14 serum levels as a strong predictor of response to biological treatments in patients with rheumatoid arthritis. Ann Rheum Dis. (2015) 74:499–505. doi: 10.1136/annrheumdis-2013-203923
90. Barber GE, Yajnik V, Khalili H, Giallourakis C, Garber J, Xavier R, et al. Genetic markers predict primary non-response and durable response to anti-TNF biologic therapies in crohn’s disease. Am J Gastroenterol. (2016) 111:1816–22. doi: 10.1038/ajg.2016.408
91. Solitano V, Facciorusso A, McGovern DPB, Nguyen T, Colman RJ, Zou L, et al. HLA-DQA1∗05 genotype and immunogenicity to tumor necrosis factor-α Antagonists: A systematic review and meta-analysis. Clin Gastroenterology Hepatology. (2023) 21:3019–3029.e5. doi: 10.1016/j.cgh.2023.03.044
92. Zapata-Cobo P, Salvador-Martín S, Velasco M, Palomino LM, Clemente S, Segarra O, et al. Polymorphisms indicating risk of inflammatory bowel disease or antigenicity to anti-TNF drugs as biomarkers of response in children. Pharmacol Res. (2023) 194:106859. doi: 10.1016/j.phrs.2023.106859
93. Hiltunen J, Parmanne P, Sokka T, Lamberg T, Isomäki P, Kaipiainen-Seppänen O, et al. Immunogenicity of subcutaneous TNF inhibitors and its clinical significance in real-life setting in patients with spondyloarthritis. Rheumatol Int. (2022) 42:1015–25. doi: 10.1007/s00296-021-04955-8
94. Mahmoud R, Schultheiss J, Louwers J, van der Kaaij M, Van Hellemondt B, Mahmmod N, et al. P414 Obesity is associated with a higher risk of immunogenicity to adalimumab, but not to infliximab in patients with Inflammatory Bowel Disease. J Crohn’s Colitis. (2021) 15:S420–2. doi: 10.1093/ecco-jcc/jjab076.538
95. Michelsen B, Berget KT, Kavanaugh A, and Haugeberg G. Association between TNFi anti-drug antibodies, smoking, and disease activity in patients with inflammatory arthritis: Results from a Norwegian cross-sectional observational study. Rheumatol Ther. (2022) 9:1171–9. doi: 10.1007/s40744-022-00464-7
96. Dedmon LE. The genetics of rheumatoid arthritis. Rheumatol (Oxford). (2020) 59:2661–70. doi: 10.1093/rheumatology/keaa232
97. Viatte S, Plant D, Han B, Fu B, Yarwood A, Thomson W, et al. Association of HLA-DRB1 haplotypes with rheumatoid arthritis severity, mortality, and treatment response. JAMA. (2015) 313:1645–56. doi: 10.1001/jama.2015.3435
98. Sazonovs A, Kennedy NA, Moutsianas L, Heap GA, Rice DL, Reppell M, et al. HLA-DQA1*05 carriage associated with development of anti-drug antibodies to infliximab and adalimumab in patients with crohn’s disease. Gastroenterology. (2020) 158:189–99. doi: 10.1053/j.gastro.2019.09.041
99. Dubinsky MC, Mei L, Friedman M, Dhere T, Haritunians T, Hakonarson H, et al. Genome wide association (GWA) predictors of anti-TNFalpha therapeutic responsiveness in pediatric inflammatory bowel disease. Inflammation Bowel Dis. (2010) 16:1357–66. doi: 10.1002/ibd.21174
100. Salvador-Martín S, Zapata-Cobo P, Velasco M, Palomino LM, Clemente S, Segarra O, et al. Association between HLA DNA variants and long-term response to anti-TNF drugs in a spanish pediatric inflammatory bowel disease cohort. IJMS. (2023) 24:1797. doi: 10.3390/ijms24021797
101. Labarile N, Ghosh S, Ng SC, Walters J, and Iacucci M. Tests that now deserve to be more widely adopted in IBD clinical practice. Therap Adv Gastroenterol. (2020) 13:1756284820944088. doi: 10.1177/1756284820944088
102. Chaparro M, Aterido A, Guerra I, Iborra M, Cabriada JL, Bujanda L, et al. Functional rare variants influence the clinical response to anti-TNF therapy in Crohn’s disease. Therap Adv Gastroenterol. (2019) 12:1756284819867848. doi: 10.1177/1756284819867848
103. Bruno CD, Fremd B, Church RJ, Daly AK, Aithal GP, Björnsson ES, et al. HLA associations with infliximab-induced liver injury. Pharmacogenomics J. (2020) 20:681–6. doi: 10.1038/s41397-020-0159-0
104. Iwaszko M, Świerkot J, Kolossa K, Jeka S, Wiland P, and Bogunia-Kubik K. Polymorphisms within the human leucocyte antigen-E gene and their associations with susceptibility to rheumatoid arthritis as well as clinical outcome of anti-tumour necrosis factor therapy. Clin Exp Immunol. (2015) 182:270–7. doi: 10.1111/cei.12696
105. Iwaszko M, Świerkot J, Dratwa M, Wysoczańska B, Korman L, Bugaj B, et al. Association of MICA-129Met/Val polymorphism with clinical outcome of anti-TNF therapy and MICA serum levels in patients with rheumatoid arthritis. Pharmacogenomics J. (2020) 20:760–9. doi: 10.1038/s41397-020-0164-3
106. Shimoda F, Naito T, Kakuta Y, Kawai Y, Tokunaga K, Consortium NCBNCWGS, et al. HLA-DQA1*05 and upstream variants of PPARGC1B are associated with infliximab persistence in Japanese Crohn’s disease patients. Pharmacogenomics J. (2023) 23:141–8. doi: 10.1038/s41397-023-00312-z
107. Liu M, Degner J, Davis JW, Idler KB, Nader A, Mostafa NM, et al. Identification of HLA-DRB1 association to adalimumab immunogenicity. PloS One. (2018) 13:e0195325. doi: 10.1371/journal.pone.0195325
108. Fuentes-Valenzuela E, García-Alonso FJ, Maroto-Martín C, Juan Casamayor L, Garrote JA, Almendros Muñoz R, et al. Influence of HLADQA1*05 genotype in adults with inflammatory bowel disease and anti-TNF treatment with proactive therapeutic drug monitoring: A retrospective cohort study. Inflammation Bowel Dis. (2023) 29:1586–93. doi: 10.1093/ibd/izac259
109. Gibson DS, McGeough CM, Watterson S, Blayney J, Wright GD, Pendleton A, et al. Anti-tumour necrosis factor-alpha response associated with combined CD226 and HLA-DRB1[*]0404 haplotype in rheumatoid arthritis. Clin Exp Rheumatol. (2021) 39:385–92. doi: 10.55563/clinexprheumatol/u1mi30
110. Wilson A, Peel C, Wang Q, Pananos AD, and Kim RB. HLADQA1*05 genotype predicts anti-drug antibody formation and loss of response during infliximab therapy for inflammatory bowel disease. Aliment Pharmacol Ther. (2020) 51:356–63. doi: 10.1111/apt.15563
111. Glintborg B, Sørensen IJ, Østergaard M, Dreyer L, Mohamoud AA, Krogh NS, et al. Ankylosing spondylitis versus nonradiographic axial spondyloarthritis: comparison of tumor necrosis factor inhibitor effectiveness and effect of HLA-B27 status. Observational Cohort Study Nationwide DANBIO Registry. J Rheumatol. (2017) 44:59–69. doi: 10.3899/jrheum.160958
112. Fröhlich F, Micheroli R, Hebeisen M, Kissling S, Bürki K, Exer P, et al. HLA-B27 as a predictor of effectiveness of treatment with TNF inhibitors in axial spondyloarthritis: data from the Swiss Clinical Quality Management Registry. Clin Rheumatol. (2023) 42:1267–74. doi: 10.1007/s10067-022-06490-8
113. Gallo E, Cabaleiro T, Román M, Solano-López G, Abad-Santos F, García-Díez A, et al. The relationship between tumour necrosis factor (TNF)-α promoter and IL12B/IL-23R genes polymorphisms and the efficacy of anti-TNF-α therapy in psoriasis: a case-control study. Br J Dermatol. (2013) 169:819–29. doi: 10.1111/bjd.2013.169.issue-4
114. Reppell M, Zheng X, Dreher I, Blaes J, Regan E, Haslberger T, et al. HLA-DQA1*05 associates with anti-tumor necrosis factor immunogenicity and low adalimumab trough concentrations in inflammatory bowel disease patients from the SERENE ulcerative colitis and crohn’s disease studies. J Crohn’s Colitis. (2025) 19:jjae129. doi: 10.1093/ecco-jcc/jjae129
115. Prieto-Pérez R, Solano-López G, Cabaleiro T, Román M, Ochoa D, Talegón M, et al. New polymorphisms associated with response to anti-TNF drugs in patients with moderate-to-severe plaque psoriasis. Pharmacogenomics J. (2018) 18:70–5. doi: 10.1038/tpj.2016.64
116. Massey J, Plant D, Hyrich K, Morgan AW, Wilson AG, Spiliopoulou A, et al. Genome-wide association study of response to tumour necrosis factor inhibitor therapy in rheumatoid arthritis. Pharmacogenomics J. (2018) 18:657–64. doi: 10.1038/s41397-018-0040-6
117. Salvador-Martín S, López-Cauce B, Nuñez O, Laserna-Mendieta EJ, García MI, Lobato E, et al. Genetic predictors of long-term response and trough levels of infliximab in crohn’s disease. Pharmacol Res. (2019) 149:104478. doi: 10.1016/j.phrs.2019.104478
118. Kim HJ, Trinh NT, Choi Y, Kim W, Min KH, Kang SO, et al. ADAM17 genetic variants and the response of TNF-α Inhibitor in rheumatoid arthritis patients. PGPM. (2020) 13:81–8. doi: 10.2147/PGPM.S235035
119. Song GG, Seo YH, Kim J-H, Choi SJ, Ji JD, and Lee YH. Association between TNF-α (-308 A/G, -238 A/G, -857 C/T) polymorphisms and responsiveness to TNF-α blockers in spondyloarthropathy, psoriasis and Crohn’s disease: a meta-analysis. Pharmacogenomics. (2015) 16:1427–37. doi: 10.2217/pgs.15.90
120. Antonatos C, Stavrou EF, Evangelou E, and Vasilopoulos Y. Exploring pharmacogenetic variants for predicting response to anti-TNF therapy in autoimmune diseases: a meta-analysis. Pharmacogenomics. (2021) 22:435–45. doi: 10.2217/pgs-2021-0019
121. Vasilopoulos Y, Manolika M, Zafiriou E, Sarafidou T, Bagiatis V, Krüger-Krasagaki S, et al. Pharmacogenetic analysis of TNF, TNFRSF1A, and TNFRSF1B gene polymorphisms and prediction of response to anti-TNF therapy in psoriasis patients in the greek population. Mol Diagn Ther. (2012) 16:29–34. doi: 10.1007/BF03256427
122. Loft ND, Skov L, Iversen L, Gniadecki R, Dam TN, Brandslund I, et al. Associations between functional polymorphisms and response to biological treatment in Danish patients with psoriasis. Pharmacogenomics J. (2018) 18:494–500. doi: 10.1038/tpj.2017.31
123. Bank S, Andersen PS, Burisch J, Pedersen N, Roug S, Galsgaard J, et al. Associations between functional polymorphisms in the NFκB signaling pathway and response to anti-TNF treatment in Danish patients with inflammatory bowel disease. Pharmacogenomics J. (2014) 14:526–34. doi: 10.1038/tpj.2014.19
124. Iwaszko M, Świerkot J, Kolossa K, Jeka S, Wiland P, and Bogunia-Kubik K. Influence of NKG2D genetic variants on response to anti-TNF agents in patients with rheumatoid arthritis. Genes. (2018) 9:64. doi: 10.3390/genes9020064
125. Koder S, Repnik K, Ferkolj I, Pernat C, Skok P, Weersma RK, et al. Genetic polymorphism in ATG16L1 gene influences the response to adalimumab in Crohn’s disease patients. Pharmacogenomics. (2015) 16:191–204. doi: 10.2217/pgs.14.172
126. Aita A, Basso D, Ramonda R, Moz S, Lorenzin M, Navaglia F, et al. Genetics in TNF-TNFR pathway: A complex network causing spondyloarthritis and conditioning response to anti-TNFα therapy. PloS One. (2018) 13:e0194693. doi: 10.1371/journal.pone.0194693
127. Morales-Lara MJ, Cañete JD, Torres-Moreno D, Hernández MV, Pedrero F, Celis R, et al. Effects of polymorphisms in TRAILR1 and TNFR1A on the response to anti-TNF therapies in patients with rheumatoid and psoriatic arthritis. Joint Bone Spine. (2012) 79:591–6. doi: 10.1016/j.jbspin.2012.02.003
128. Lee YH and Bae S-C. Associations between PTPRC rs10919563 A/G and FCGR2A R131H polymorphisms and responsiveness to TNF blockers in rheumatoid arthritis: a meta-analysis. Rheumatol Int. (2016) 36:837–44. doi: 10.1007/s00296-016-3476-5
129. Sode J, Vogel U, Bank S, Andersen PS, Hetland ML, Locht H, et al. Genetic variations in pattern recognition receptor loci are associated with anti-TNF response in patients with rheumatoid arthritis. PloS One. (2015) 10:e0139781. doi: 10.1371/journal.pone.0139781
130. Matsuoka K, Hamada S, Shimizu M, Nanki K, Mizuno S, Kiyohara H, et al. Factors predicting the therapeutic response to infliximab during maintenance therapy in Japanese patients with Crohn’s disease. PloS One. (2018) 13:e0204632. doi: 10.1371/journal.pone.0204632
131. López-Hernández R, Valdés M, Campillo JA, Martínez-Garcia P, Salama H, Salgado G, et al. Genetic polymorphisms of tumour necrosis factor alpha (TNF-α) promoter gene and response to TNF-α inhibitors in Spanish patients with inflammatory bowel disease. Int J Immunogenet. (2014) 41:63–8. doi: 10.1111/iji.12059
132. Qasem A, Ramesh S, and Naser SA. Genetic polymorphisms in tumour necrosis factor receptors (TNFRSF1A/1B) illustrate differential treatment response to TNFα inhibitors in patients with Crohn’s disease. BMJ Open Gastroenterol. (2019) 6:e000246. doi: 10.1136/bmjgast-2018-000246
133. Canet LM, Filipescu I, Cáliz R, Lupiañez CB, Canhão H, Escudero A, et al. Genetic variants within the TNFRSF1B gene and susceptibility to rheumatoid arthritis and response to anti-TNF drugs: a multicenter study. Pharmacogenetics Genomics. (2015) 25:323–33. doi: 10.1097/FPC.0000000000000140
134. Maxwell JR, Potter C, Hyrich KL, BRAGGSS, Barton A, Worthington J, et al. Association of the tumour necrosis factor-308 variant with differential response to anti-TNF agents in the treatment of rheumatoid arthritis. Hum Mol Genet. (2008) 17:3532–8. doi: 10.1093/hmg/ddn245
135. Ovejero-Benito MC, Prieto-Pérez R, Llamas-Velasco M, Muñoz-Aceituno E, Reolid A, Saiz-Rodríguez M, et al. Polymorphisms associated with adalimumab and infliximab response in moderate-to-severe plaque psoriasis. Pharmacogenomics. (2018) 19:7–16. doi: 10.2217/pgs-2017-0143
136. Bank S, Julsgaard M, Abed OK, Burisch J, Broder Brodersen J, Pedersen NK, et al. Polymorphisms in the NFkB, TNF-alpha, IL-1beta, and IL-18 pathways are associated with response to anti-TNF therapy in Danish patients with inflammatory bowel disease. Aliment Pharmacol Ther. (2019) 49:890–903. doi: 10.1111/apt.2019.49.issue-7
137. Conigliaro P, Ciccacci C, Politi C, Triggianese P, Rufini S, Kroegler B, et al. Polymorphisms in STAT4, PTPN2, PSORS1C1 and TRAF3IP2 genes are associated with the response to TNF inhibitors in patients with rheumatoid arthritis. PloS One. (2017) 12:e0169956. doi: 10.1371/journal.pone.0169956
138. De T, Zhang H, Alarcon C, Lec B, Avitia J, Smithberger E, et al. Genetic association of primary nonresponse to anti-TNFα therapy in patients with inflammatory bowel disease. Pharmacogenet Genomics. (2022) 32:1–9. doi: 10.1097/FPC.0000000000000445
139. González-Lara L, Batalla A, Coto E, Gómez J, Eiris N, Santos-Juanes J, et al. The TNFRSF1B rs1061622 polymorphism (p.M196R) is associated with biological drug outcome in Psoriasis patients. Arch Dermatol Res. (2015) 307:405–12. doi: 10.1007/s00403-014-1533-z
140. Lacruz-Guzmán D, Torres-Moreno D, Pedrero F, Romero-Cara P, García-Tercero I, Trujillo-Santos J, et al. Influence of polymorphisms and TNF and IL1β serum concentration on the infliximab response in Crohn’s disease and ulcerative colitis. Eur J Clin Pharmacol. (2013) 69:431–8. doi: 10.1007/s00228-012-1389-0
141. Jezernik G, Gorenjak M, and Potočnik U. MIF variant rs755622 is associated with severe crohn’s disease and better response to anti-TNF adalimumab therapy. Genes. (2023) 14:452. doi: 10.3390/genes14020452
142. Chen Y-Y. Correlations of CYP2C9*3/CYP2D6*10/CYP3A5*3 gene polymorphisms with efficacy of etanercept treatment for patients with ankylosing spondylitis: A case-control study. Med (Baltimore). (2017) 96:e5993. doi: 10.1097/MD.0000000000005993
143. Cabaleiro T, Prieto-Pérez R, Navarro R, Solano G, Román M, Ochoa D, et al. Paradoxical psoriasiform reactions to anti-TNFα drugs are associated with genetic polymorphisms in patients with psoriasis. Pharmacogenomics J. (2016) 16:336–40. doi: 10.1038/tpj.2015.53
144. Membrive Jiménez C, Pérez Ramírez C, Sánchez Martín A, Vieira Maroun S, Arias Santiago SA, Ramírez Tortosa MDC, et al. Influence of genetic polymorphisms on response to biologics in moderate-to-severe psoriasis. J Pers Med. (2021) 11:293. doi: 10.3390/jpm11040293
145. Curci D, Lucafò M, Cifù A, Fabris M, Bramuzzo M, Martelossi S, et al. Pharmacogenetic variants of infliximab response in young patients with inflammatory bowel disease. Clin Transl Sci. (2021) 14:2184–92. doi: 10.1111/cts.13075
146. Hu W, Feng Y, Ye Z, Tang Z, Qian L, Wang Y, et al. The association between genetic variants, pharmacokinetics, and infliximab efficacy in pediatric patients with crohn’s disease in China. Front Pediatr. (2021) 9:744599. doi: 10.3389/fped.2021.744599
147. Wang M-H, Friton JJ, Raffals LE, Leighton JA, Pasha SF, Picco MF, et al. Novel genetic risk variants can predict anti-TNF agent response in patients with inflammatory bowel disease. J Crohn’s Colitis. (2019) 13:1036–43. doi: 10.1093/ecco-jcc/jjz017
148. Rottura M, Pirrotta I, Giorgi DA, Irrera N, Arcoraci V, Mannino F, et al. Genetic polymorphisms on TNFA, TNFRSF1A, and TNFRSF1B genes predict the effectiveness of anti-TNF-α Treatment in inflammatory bowel disease patients. Biomedicines. (2025) 13:669. doi: 10.3390/biomedicines13030669
149. Zhang H, Patrick MT, Tejasvi T, Sarkar MK, Wasikowski R, Stuart PE, et al. Retrospective pharmacogenetic study of psoriasis highlights the role of KLK7 in tumour necrosis factor signalling. Br J Dermatol. (2023) 190:70–9. doi: 10.1093/bjd/ljad332
150. Padula MC, Padula AA, D’Angelo S, Lascaro N, Radice RP, Martelli G, et al. TNFα rs1800629 polymorphism and response to anti-TNFα Treatment in behçet syndrome: data from an italian cohort study. JPM. (2023) 13:1347. doi: 10.3390/jpm13091347
151. Cheli S, Savino D, De Silvestri A, Norsa L, Sansotta N, Penagini F, et al. Correction to: One year of experience with combined pharmacokinetic/pharmacogenetic monitoring of anti-TNF alpha agents: a retrospective study. Pharmacogenomics J. (2023) 23:140–0. doi: 10.1038/s41397-023-00311-0
152. Pappas DA, Brittle C, Mossell JE, Withers JB, Lim-Harashima J, and Kremer JM. Perceived clinical utility of a test for predicting inadequate response to TNF inhibitor therapies in rheumatoid arthritis: results from a decision impact study. Rheumatol Int. (2021) 41:585–93. doi: 10.1007/s00296-020-04746-7
153. Chastek B, Chen C-I, Proudfoot C, Shinde S, Kuznik A, and Wei W. Treatment persistence and healthcare costs among patients with rheumatoid arthritis changing biologics in the USA. Adv Ther. (2017) 34:2422–35. doi: 10.1007/s12325-017-0617-5
154. Singh JA, Furst DE, Bharat A, Curtis JR, Kavanaugh AF, Kremer JM, et al. 2012 Update of the 2008 American College of Rheumatology recommendations for the use of disease-modifying antirheumatic drugs and biologic agents in the treatment of rheumatoid arthritis. Arthritis Care Res. (2012) 64:625–39. doi: 10.1002/acr.21641
155. Kim G, Barner JC, Rascati K, and Richards K. Factors associated with the initiation of biologic disease-modifying antirheumatic drugs in texas medicaid patients with rheumatoid arthritis. JMCP. (2015) 21:401–7. doi: 10.18553/jmcp.2015.21.5.401
156. Drennan P, Doogue M, Van Hal SJ, and Chin P. Bayesian therapeutic drug monitoring software: past, present and future. Int J Pharmacokinetics. (2018) 3:109–14. doi: 10.4155/ipk-2018-0005
157. Desai DC, Dherai AJ, Strik A, and Mould DR. Personalized dosing of infliximab in patients with inflammatory bowel disease using a bayesian approach: A next step in therapeutic drug monitoring. J Clin Pharma. (2023) 63:480–9. doi: 10.1002/jcph.2189
158. Dubinsky MC, Mendiolaza ML, Phan BL, Moran HR, Tse SS, and Mould DR. Dashboard-driven accelerated infliximab induction dosing increases infliximab durability and reduces immunogenicity. Inflammatory Bowel Dis. (2022) 28:1375–85. doi: 10.1093/ibd/izab285
159. Dave MB, Dherai AJ, Desai DC, Mould DR, and Ashavaid TF. Optimization of infliximab therapy in inflammatory bowel disease using a dashboard approach—an Indian experience. Eur J Clin Pharmacol. (2021) 77:55–62. doi: 10.1007/s00228-020-02975-0
160. Strik AS, Löwenberg M, Mould DR, Berends SE, Ponsioen CI, Van Den Brande JMH, et al. Efficacy of dashboard driven dosing of infliximab in inflammatory bowel disease patients; a randomized controlled trial. Scandinavian J Gastroenterology. (2021) 56:145–54. doi: 10.1080/00365521.2020.1856405
161. Salvador-Martín S, Pujol-Muncunill G, Bossacoma F, Navas-López VM, Gallego-Fernández C, Segarra O, et al. Pharmacogenetics of trough serum anti-TNF levels in paediatric inflammatory bowel disease. Brit J Clin Pharma. (2021) 87:447–57. doi: 10.1111/bcp.14400
162. Juanola O, Moratalla A, Gutiérrez A, Sempere L, Zapater P, Giménez P, et al. Anti-TNF-alpha loss of response is associated with a decreased percentage of FoxP3+ T cells and a variant NOD2 genotype in patients with Crohn’s disease. J Gastroenterol. (2015) 50:758–68. doi: 10.1007/s00535-014-1020-5
163. Yahya F, Gaffney K, Hamilton L, Lonsdale E, Leeder J, Brooksby A, et al. Tumour necrosis factor inhibitor survival and predictors of response in axial spondyloarthritis—findings from a United Kingdom cohort. Rheumatology. (2018) 57:619–24. doi: 10.1093/rheumatology/kex457
164. Rudwaleit M, Siegert S, Yin Z, Eick J, Thiel A, Radbruch A, et al. Low T cell production of TNFα and IFNÎ3 in ankylosing spondylitis: its relation to HLA-B27 and influence of the TNF-308 gene polymorphism. Ann Rheumatic Dis. (2001) 60:36–42. doi: 10.1136/ard.60.1.36
165. Guan Y, Zhang H, Quang D, Wang Z, Parker SCJ, Pappas DA, et al. Machine learning to predict anti–tumor necrosis factor drug responses of rheumatoid arthritis patients by integrating clinical and genetic markers. Arthritis Rheumatol. (2019) 71:1987–96. doi: 10.1002/art.41056
166. Yoosuf N, Maciejewski M, Ziemek D, Jelinsky SA, Folkersen L, Müller M, et al. Early prediction of clinical response to anti-TNF treatment using multi-omics and machine learning in rheumatoid arthritis. Rheumatology. (2022) 61:1680–9. doi: 10.1093/rheumatology/keab521
167. Park SK, Kim YB, Kim S, Lee CW, Choi CH, Kang S-B, et al. Development of a machine learning model to predict non-durable response to anti-TNF therapy in crohn’s disease using transcriptome imputed from genotypes. JPM. (2022) 12:947. doi: 10.3390/jpm12060947
168. Mellors T, Withers JB, Ameli A, Jones A, Wang M, Zhang L, et al. Clinical validation of a blood-based predictive test for stratification of response to tumor necrosis factor inhibitor therapies in rheumatoid arthritis patients. Network Syst Med. (2020) 3:91–104. doi: 10.1089/nsm.2020.0007
169. Piskol R, Ramaswami G, and Li JB. Reliable identification of genomic variants from RNA-seq data. Am J Hum Genet. (2013) 93:641–51. doi: 10.1016/j.ajhg.2013.08.008
170. Foulkes AC, Watson DS, Carr DF, Kenny JG, Slidel T, Parslew R, et al. A framework for multi-omic prediction of treatment response to biologic therapy for psoriasis. J Invest Dermatol. (2019) 139:100–7. doi: 10.1016/j.jid.2018.04.041
171. Tournier Q, Paul S, Williet N, Berger A, Veyrard P, Boschetti G, et al. Early detection of anti-drug antibodies during initiation of anti-tumour necrosis factor therapy predicts treatment discontinuation in inflammatory bowel disease. Aliment Pharmacol Ther. (2021) 53:1190–200. doi: 10.1111/apt.16333
172. Thomas SS, Borazan N, Barroso N, Duan L, Taroumian S, Kretzmann B, et al. Comparative immunogenicity of TNF inhibitors: impact on clinical efficacy and tolerability in the management of autoimmune diseases. A systematic review and meta-analysis. BioDrugs. (2015) 29:241–58. doi: 10.1007/s40259-015-0134-5
173. Shah P, Siddique A, Thakkar A, Gharat S, Godad A, Kale P, et al. An update on novel therapeutic intervention in Rheumatoid arthritis. Int Immunopharmacol. (2022) 109:108794. doi: 10.1016/j.intimp.2022.108794
174. Olsen T, Cui G, Goll R, Husebekk A, and Florholmen J. Infliximab therapy decreases the levels of TNF-α and IFN-γ mRNA in colonic mucosa of ulcerative colitis. Scandinavian J Gastroenterology. (2009) 44:727–35. doi: 10.1080/00365520902803507
175. Cui J, Stahl EA, Saevarsdottir S, Miceli C, Diogo D, Trynka G, et al. Genome-wide association study and gene expression analysis identifies CD84 as a predictor of response to etanercept therapy in rheumatoid arthritis. PloS Genet. (2013) 9:e1003394. doi: 10.1371/journal.pgen.1003394
176. Ranzolin A, Brenol JCT, Bredemeier M, Guarienti J, Rizzatti M, Feldman D, et al. Association of concomitant fibromyalgia with worse disease activity score in 28 joints, health assessment questionnaire, and short form 36 scores in patients with rheumatoid arthritis. Arthritis Rheum. (2009) 61:794–800. doi: 10.1002/art.24430
177. Siemons L, Ten Klooster PM, Vonkeman HE, Van De Laar MAFJ, and Glas CAW. Further optimization of the reliability of the 28-joint disease activity score in patients with early rheumatoid arthritis. Zhang C editor. PloS One. (2014) 9:e100544. doi: 10.1371/journal.pone.0100544
178. Vermeer M, Kuper HH, van der Bijl AE, Baan H, Posthumus MD, Brus HLM, et al. The provisional ACR/EULAR definition of remission in RA: a comment on the patient global assessment criterion. Rheumatology. (2012) 51:1076–80. doi: 10.1093/rheumatology/ker425
179. Bluett J, Morgan C, Thurston L, Plant D, Hyrich KL, Morgan AW, et al. Impact of inadequate adherence on response to subcutaneously administered anti-tumour necrosis factor drugs: results from the Biologics in Rheumatoid Arthritis Genetics and Genomics Study Syndicate cohort. Rheumatology. (2015) 54:494–9. doi: 10.1093/rheumatology/keu358
180. Kane SV and Robinson A. Review article: understanding adherence to medication in ulcerative colitis – innovative thinking and evolving concepts. Aliment Pharmacol Ther. (2010) 32:1051–8. doi: 10.1111/j.1365-2036.2010.04445.x
181. McInnes G, Yee SW, Pershad Y, and Altman RB. Genomewide association studies in pharmacogenomics. Clin Pharma Ther. (2021) 110:637–48. doi: 10.1002/cpt.2349
182. Baronti F, Micheli A, Passaro A, and Starita A. Machine learning contribution to solve prognostic medical problems. In: Outcome prediction in cancer. Netherlands: Elsevier (2007), ISBN: ISBN:978-0-444-52855-1. p. 261–83. doi: 10.1016/B978-044452855-1/50012-X
183. Soni J and Pandey R. Single cell genomics based insights into the impact of cell-type specific microbial internalization on disease severity. Front Immunol. (2024) 15:1401320. doi: 10.3389/fimmu.2024.1401320
Keywords: pharmacogenomics, genetic variants, TNF inhibitors, human leukocyte antigen, drug efficacy and safety
Citation: Jan Z, El Assadi F, Velayutham D, Mifsud B and Jithesh PV (2025) Pharmacogenomics of TNF inhibitors. Front. Immunol. 16:1521794. doi: 10.3389/fimmu.2025.1521794
Received: 02 November 2024; Accepted: 30 April 2025;
Published: 21 May 2025.
Edited by:
Olga Maria Nardone, Federico II University Hospital, ItalyReviewed by:
Stefania Cheli, ASST Fatebenefratelli Sacco, ItalyYiannis Vasilopoulos, University of Patras, Greece
Copyright © 2025 Jan, El Assadi, Velayutham, Mifsud and Jithesh. This is an open-access article distributed under the terms of the Creative Commons Attribution License (CC BY). The use, distribution or reproduction in other forums is permitted, provided the original author(s) and the copyright owner(s) are credited and that the original publication in this journal is cited, in accordance with accepted academic practice. No use, distribution or reproduction is permitted which does not comply with these terms.
*Correspondence: Puthen Veettil Jithesh, anZlZXR0aWxAaGJrdS5lZHUucWE=
†These authors have contributed equally to this work