- 1Integrative Genomics Lab, Center for Cooperative Research in Biosciences (CIC bioGUNE), Basque Research and Technology Alliance (BRTA), Bizkaia Technology Park, Derio, Spain
- 2Cancer Immunology and Immunotherapy Lab, Center for Cooperative Research in Biosciences (CIC bioGUNE), Basque Research and Technology Alliance (BRTA), Bizkaia Technology Park, Derio, Spain
- 3IKERBASQUE, Basque Foundation for Sciences, Bilbao, Spain
- 4Gastroenterology Department, Hospital Clínico Universitario de Santiago, Santiago de Compostela, Spain
- 5Gastroenterology Department, Hospital Universitario de Galdakao, Biobizkaia Health Research Institute, Galdakao, Spain
- 6Department of Medicine, Faculty of Health Sciences, University of Deusto, Bilbao, Spain
Despite the increasing incidence of Crohn’s disease (CD), its early immune disturbances have not all been described yet. We analysed single-cell RNASeq data from peripheral blood mononuclear cells of patients with incidentally-diagnosed CD, and compared their gene expression profile to healthy individuals. The main aim of our study was to perform an exploratory analysis of how the subclinical inflammatory process is modifying the individual’s immunologic environment while the patient is still in the preclinical period.
1 Introduction
Crohn’s disease (CD) and ulcerative colitis are chronic immune-mediated inflammatory diseases that consist the two primary forms of IBD. Due to the increasing worldwide incidence of IBD, the study of its preclinical phase is crucial since it will allow the understanding of the underlying immunological pathways that lead to the development of the disease prior to its clinical onset. Various initiatives have focused on providing insights into the transitional phase of the disease, such as the Genetics Environmental Microbial (GEM) project (1) and the Proteomic Evaluation and Discovery (PREDICTS) project (2). Moreover, a recent review by Rudbaek et al. (3) provided numerous studies that investigated the preclinical phase of IBD, either at a dedicated time-point or longitudinally, deriving insights from diverse omic samples such as serological proteomics and metabolomics as well as intestinal microbiome. Although these studies have demonstrated the possibility to identify processes that can be linked to the development of IBD well in advance of the clinical onset, still the transitional events that shift the asymptomatic patient towards the symptomatic phase remain unknown.
The EARLY study (NCT05698745) represents our prospective 10-years planned initiative, in collaboration with 25 hospitals in Spain, that will enrol asymptomatic IBD patients who have been incidentally diagnosed through general colorectal cancer screening programs (4). By implementing multi-omic integration and drawing comparisons between asymptomatic and symptomatic IBD patients, the goal of EARLY is to pinpoint the initial immunological alterations that modify the immunologic environment of patients during the preclinical phase of IBD and favor the transition towards the clinical onset of the disease. As part of EARLY, the current manuscript represents a pilot study of single-cell RNA sequencing (scRNASeq) from peripheral blood mononuclear cells (PBMC) of two preclinical CD patients, where their gene expression profile was compared to two non-IBD healthy individuals. Our aim was to explore the potential differences between the two groups, since characterization of the molecular landscape at the single-cell level is still lacking during the preclinical stage.
2 Methods
PBMC samples from two CD patients were collected within the first 2 months after diagnosis and without previous IBD-related therapy. These two patients included a 52-year-old female patient with ileocecal disease (L3) and inflammatory behaviour (B1), former smoker, and a 54-year-old male with ileal involvement (L1) and also inflammatory behaviour (B1), non-smoker. Neither of these patients had disease-related complications or extraintestinal manifestations. They were incidentally (i.e. asymptomatic) diagnosed with CD within the population-based colorectal cancer screening programme of the Basque Country (https://www.osakidetza.euskadi.eus/programa-cribado-cancer-colorrectal/webosk00-oskenf/es/). Both patients were asymptomatic at diagnosis, which was made according to ECCO criteria, during a complete screening colonoscopy after a positive fecal occult blood test (cut-off 20 µg Hb/g). Clinical, endoscopic and histologic findings confirmed the diagnosis of CD by the presence of chronic infiltrate and absence of any enteropathogen or alternative diagnosis after a detailed differential diagnosis. This methodology has been used in previous reports by our group (4–6) and recognized by international consensus as a potential target for disease intervention studies (7).
The frozen vials of PBMCs were rapidly thawed in a 37°C water bath for 2 min and diluted in 4 mL of DMEM medium (Gibco # 41966). Cells were centrifuged at 500 x g for 10 min at room temperature. The supernatant was removed, and the cell pellet was resuspended in 3 mL of cold 1X phosphate-buffered saline (PBS, Thermo Fisher Scientific). Live cells were sorted by FACSfusion using DAPI. Cells were resuspended with PBS at a concentration of 1.000 cells/mL for single-cell RNA sequencing (scRNAseq).
Single cell library was prepared using Chromium Next GEM Chip G Single Cell Kit, Chromium Next GEM Single Cell 3’ Kit v3.1 and Dual Index Kit TT Set A, following Chromium Next GEM Single Cell 3’ Reagent Kits v3.1 (Dual Index) user guide. The sequencing process was carriedout on a NovaSeq 6000 sequencer, whereas alignment and gene count was performed using 10X Genomics Cell Ranger 7.0.0 and the human reference GRCh38.
For the control dataset, two healthy non-IBD controls were selected from two distinct PBMC scRNAseq datasets, after reassuring that each sample underwent the same 10X Genomics 3’ V3 protocol that was implemented in our study. The first sample was selected from the publicly available PBMC scRNAseq control dataset provided by 10X Genomics (8) and the second sample was an untreated healthy individual selected from the publicly available scRNAseq dataset of PBMCs (1M-scBloodNL) provided by Oelen et al. (9).
We performed the following quality control to each sample individually using SCANPY (10). We filtered out genes with gene count <10 across all cells. We removed cells with high mitochondrial content and removed mitochondrial genes from the list of quantified genes. We normalized total counts per cell to 10,000. We calculated cell-cycle scores using the “scanpy_usage” github repository (https://github.com/scverse/scanpy_usage) to score S and G2M phases and removed cycling cells. We predicted and removed doublets using SCRUBLET (11). We finally performed cell-type annotation using AZIMUTH (12) that utilized the AZIMUTH human PBMC reference dataset (13).
We next merged the samples and performed batch correction with SCVI-TOOLS (14) in order to correct data integration from different studies. Finally, for each cell-type we performed differential gene expression analysis with SCANPY using the batch corrected gene expression estimated by SCVI-TOOLS. Using the identified differential expressed genes, we performed pathway enrichment analysis and functional annotation. Enrichment analysis was performed with the Cytoscape plug-in ClueGO (15) where databases from KEGG, Reactome and WikiPathways were queried for pathway enrichment and annotation. For the functional annotation of proteins we utilized the PANTHER API (16).
3 Results
Initially, we evaluated the pre-processing pipeline that included the merging of the datasets. We did not observe formation of separate clusters between the samples for the same cell-types Figure 1 right panel), indicating successful batch correction concerning integration from different studies Luecken et al. (17). The cell-type annotation identified B cells (B), CD4+ and CD8+ T-cells (CD4T, CD8T), dendritic cells (DC), monocytes (Mono) and natural killer cells (NK) (Figure 1 left panel). The analytical pipeline included 17,021 cells and 3,000 quantified genes. Considering each cell type individually, we identified 49 differentially expressed genes (DEG) between CD patients and controls, from which 30 were DEGs in all cell types.
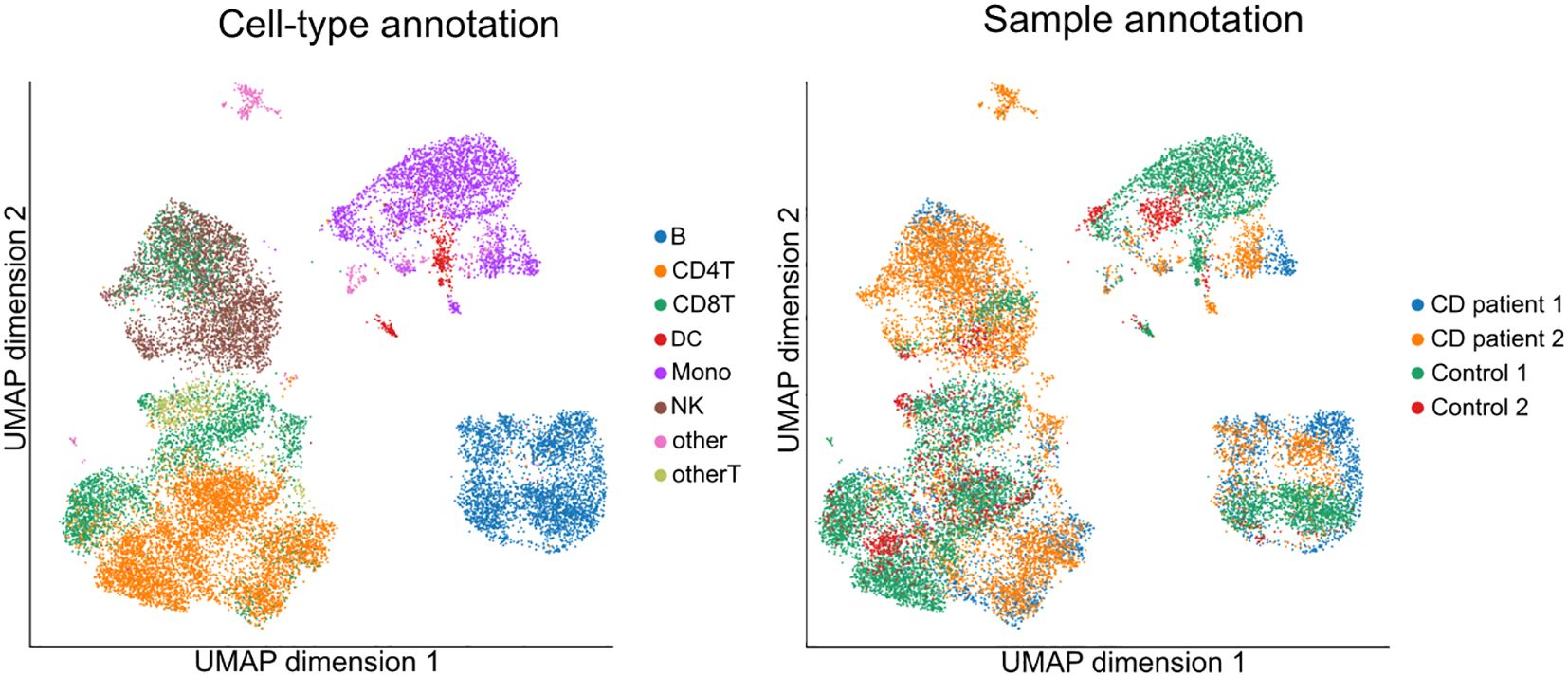
Figure 1. Uniform manifold approximation and projection (UMAP) of cells annotated by celltype (left panel) and sample (right panel).
Building on this list of 49 detected DEGs, we report four insights into the preclinical period of the disease. First, we observed greater similarity between CD8T and DC cells since they were the cell types with higher fraction of DEGs (Figure 2A). This suggests a higher contribution of these cell-types in the separation of the two groups. On the other hand, gene fold-changes between the two groups showed similar expression patterns between the CD4T and B cells as well as between CD8T and NK cells (Figure 2B).
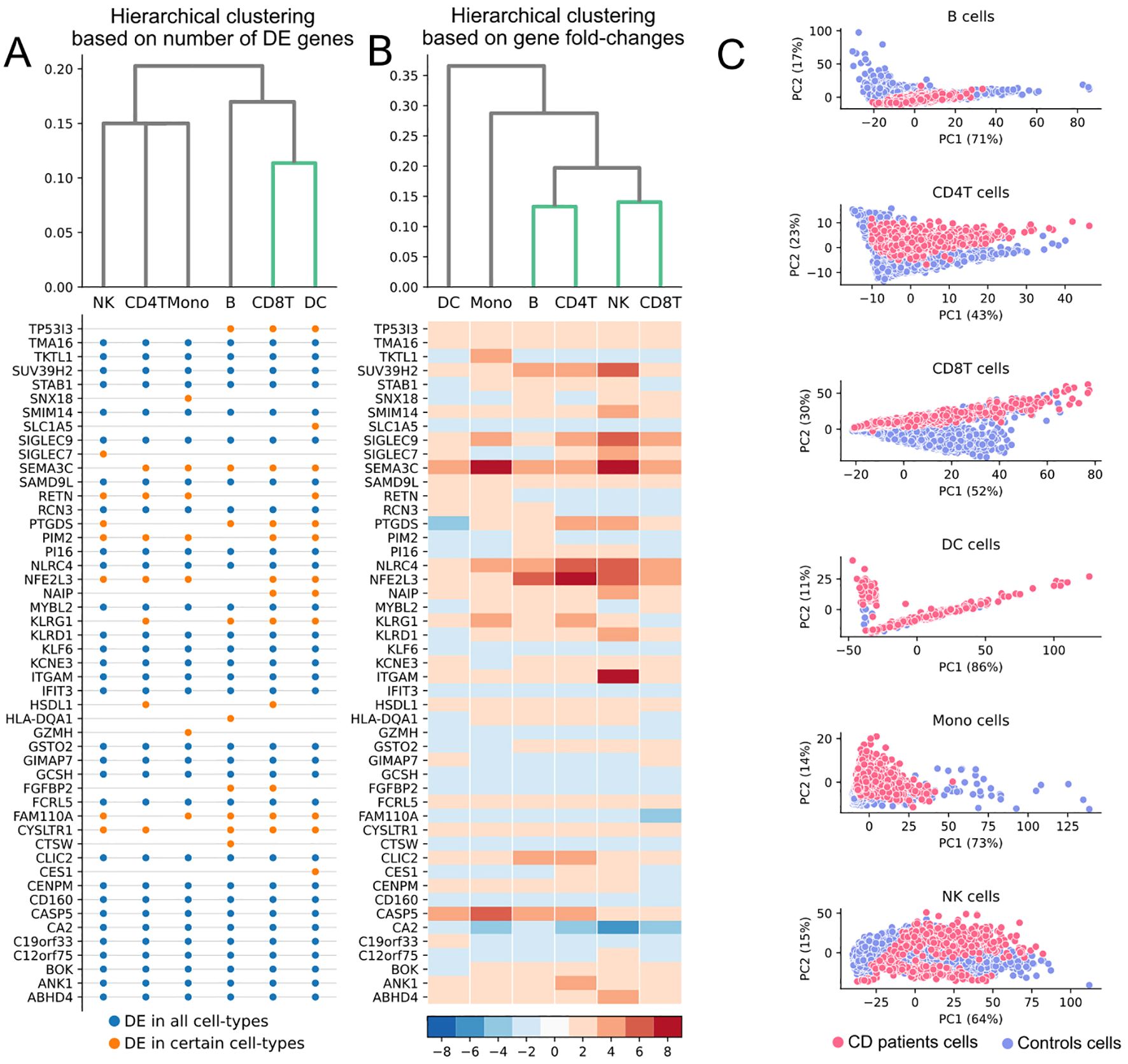
Figure 2. Clusterings based on 49 DEGs identified between CD patients and controls. (A) Hierarchical clustering based on the fraction of the 49 DEGs that were differentially expressed in each cell-type. (B) Hierarchical clustering based on the fold-changes of the 49 DEGs between CD patients and controls in each cell-type. (C) PCA using the 49 DEGs in each cell-type.
Second, pathway analysis revealed significant enrichment of the pathway that is related to immunoregulatory interactions between lymphoid and non-lymphoid cells (Figure 3). Five genes belonged to this enriched pathway, namely CD160, KLRD1, KLRG1, SIGLEC7 and SIGLEC9. All cell-types displayed significant alterations in the mRNA levels of CD160, KLRD1 and SIGLEC9, whereas SIGLEC7 was differentially expressed only in NK cells, and KLRG1 in all cells except NK and Mono cells. Furthermore, functional annotation analysis identified the gene products of FCRL5, KLRD1, CES1, HLA-DQA1, IFIT3, PI16 as proteins related to immunological defense.
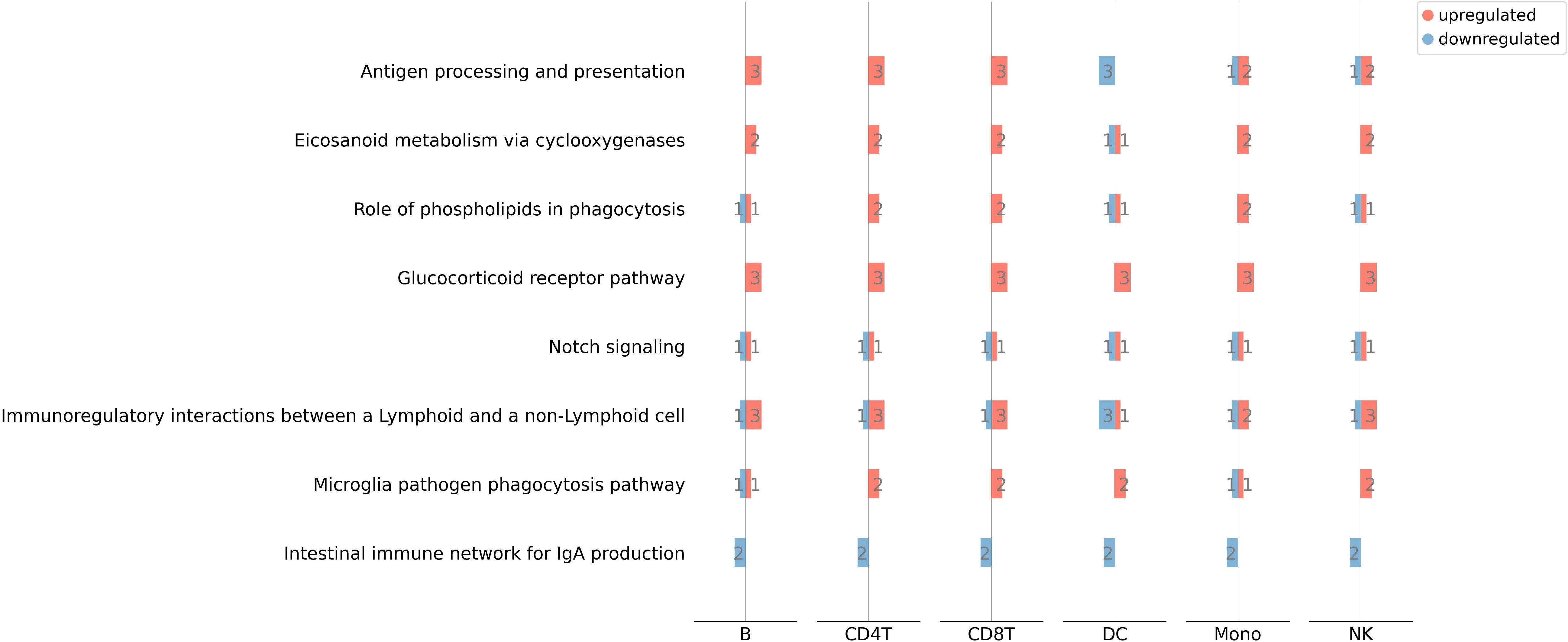
Figure 3. Pathway enrichment analysis of 30 genes that were differentially expressed across all cell types.
Third, we identified significant transcriptomic alterations in genes previously related to the symptomatic phase of IBD, or reported as potential biomarkers. The differentially expressed FCRL5 and PIM2 have been reported as significantly upregulated in plasma cells of mesenteric adipose tissue of CD patients (18). We observed significant downregulation of CD160 that has been related to the development of the IBD pathogenesis (19). The gene CA2 has been identified as a biomarker in ileal CD (20) and it was differentially expressed in all cell-types in the current study. Whole blood transcriptomic data analysis included CYSLTR1 among the 100 genes that could be potentially associated with IBD (21), and it was differentially expressed and upregulated in almost all cell-types of CD patients in our study. The serum levels of NLRC4 have been reported significantly lower in CD patients using enzyme-linked immunosorbent assay (22). Although NLRC4 was differentially expressed across all cell-types in our study, we observed upregulated levels in the CD patients. Additionally, it has been reported that the activation of NLRC4 is mediated by NAIP (23) which was differentially expressed and upregulated in the CD8T and DC cells of the CD patients in the current study.
Fourth, principal component analysis (PCA) using the 49 DEGs showed high captured variance with the first two PCs in all cell-types (Figure 2C). Of note, we observed greater separation between patients and controls in the CD8T cells. The separation occurred mainly across the PC2 that captured the highest variance in the CD8T cells compared to the other cell-types. The genes that exhibited strong negative correlation (≤-0.8) with the PC2 and were differentially expressed in CD8T cells were KLRD1, ITGAM, SMIM14, SAMD9L, PTGDS, KLRG1, whereas the genes GIMAP7, KLF6, FGFBP2 showed strong positive correlation (≥0.8). Interestingly, KLRD1 and KLRG1 have been suggested to be among the targets of the T-box transcription factor Eomesodermin (Eomes) during the shifting of CD8+ tissue-resident memory T cells towards an inflammatory differentiation state in UC patients (24). Thus, capturing such transcriptomic events in the preclinical phase highlights the potential role of CD8 T-cells during the initial events of the immunopathogenesis of CD. This hypothesis is inline with previous studies, postulating that the transcriptomic profile of this cell-type is able to predict a more frequently relapsing disease (25). Additionally, the serum protein levels of CXCL9, which is involved in T-cell trafficking, have recently been found significantly associated with the future manifestation of CD pathogenesis (26).
4 Discussion
IBD represents a chronic and progressive disease with a complex pathophysiology. Although the immunological events that drive its pathophysiology remain unknown, various studies hint their presence years before the onset of the first symptoms (3). In addition, life style and environmental factors make their own contribution to the disease onset, modulating its progression and increasing its complexity. Thus, longitudinal studies that integrate multi-omic data are necessary to study the transition of IBD patients from the preclinical phase.
Our pilot study is the first that incorporates PBMC single-cell expression data of CD asymptomatic patients. Overall, we observed distinct transcriptomic profiles of these patients compared to healthy individuals, with small intragroup differences. Of note, although correction methods were applied to minimize technical biases, differences in sequencing platforms, sample processing, and population structure between datasets may still introduce residual confounding effects, which we acknowledge as a limitation of the study.
Nevertheless, our preliminary results revealed significant transcriptomic alterations in previously reported genes and highlighted CD8T cells as the cell-type that has greater impact on the separation of the two groups. In a systematic review conducted by Chen et al. (27), subsets of CD8T populations have exhibited prognostic potential to predict either post-operative endoscopic recurrence, risk of surgery or future response to vedolizumab treatment. Thus, the emergence of patterns associated with CD8T cells in our preliminary results are encouraging findings and evoke anticipation for larger sample size attempts towards characterization of the preclinical stage of IBD, such as the EARLY study, that will serve to validate the first principles described here.
5 Conclusion
Our preliminary findings directly support our aim of exploring how subclinical inflammation shapes the immunologic landscape during the preclinical phase of CD. The identification of distinct transcriptomic profiles compared to healthy controls and the prominent role of CD8T cells suggest that certain immunologic alterations can be detectable during the asymptomatic phase. Thus, providing the first single-cell approximation, our pilot study adds novel transcriptomic insights that complement the emerging picture about the molecular changes that precede the onset of symptomatic IBD and paves the way for the discovery of new markers allowing the development of potential disease intervention strategies at an early stage in this disease.
Data availability statement
The datasets presented in this study can be found in online repositories. The names of the repository/repositories and accession number(s) can be found below: https://www.ncbi.nlm.nih.gov/sra/PRJNA970312, PRJNA970312.
Ethics statement
The studies involving humans were approved by Comité de ética de la investigación con medicamentos de Euskadi (CEIm-E). The studies were conducted in accordance with the local legislation and institutional requirements. The participants provided their written informed consent to participate in this study. Written informed consent was obtained from the individual(s) for the publication of any potentially identifiable images or data included in this article.
Author contributions
DK: Data curation, Formal Analysis, Investigation, Methodology, Writing – original draft, Writing – review & editing. LE-M: Methodology, Writing – review & editing. AP: Resources, Writing – review & editing. MA: Funding acquisition, Resources, Writing – review & editing. IR-L: Conceptualization, Funding acquisition, Resources, Supervision, Writing – review & editing. UM: Conceptualization, Funding acquisition, Resources, Supervision, Writing – review & editing.
Funding
This work was supported by grants from the Spanish Ministry of Science MCIN/AEI/10.13039/501100011033 and by ERDF/EU (PID2019-108244RA-I00 and PID2023-151379OB-I00 to U.M.M, PID2019-107956RA-I00 to A.P., RYC2020-030632-I to U.M.M, RYC2018-024183-I to A.P., CEX2021-001136-S to U.M.M and D.K), ”la Caixa” Foundation (ID 100010434, fellowship code LCF/BQ/LI18/11630001 to U.M.M and LCF/PR/HR21/52410009 to A.P., and the European Research Council (ERC) (grant number: ERC-2018-StG 804236-NEXTGEN-IO to A.P. IR-L is funded by a research grant from Gobierno Vasco – Eusko Jaurlaritza (grant number: 2020111061 and 2023222006). The EARLY study is funded by an IIS Grant from AbbVie, Programa Estatal de Generación de Conocimiento y Fortalecimiento Científico y Tecnológico del sistema de I+D+i (PID2019-108244RA-I00), GETECCU-Pfizer grant, Ayudas a Proyectos de Investigación y Desarrollo en Salud del Departamento de Salud del Gobierno Vasco (2020111061), and the 10x Genomics Core Lab Grant Program. The funder was not involved in the study design, collection, analysis, interpretation of data, the writing of this article or the decision to submit it for publication.
Conflict of interest
The authors declare that the research was conducted in the absence of any commercial or financial relationships that could be construed as a potential conflict of interest.
Generative AI statement
The author(s) declare that no Generative AI was used in the creation of this manuscript.
Publisher’s note
All claims expressed in this article are solely those of the authors and do not necessarily represent those of their affiliated organizations, or those of the publisher, the editors and the reviewers. Any product that may be evaluated in this article, or claim that may be made by its manufacturer, is not guaranteed or endorsed by the publisher.
References
1. Leibovitzh H, Lee S-H, Xue M, Garay JAR, Hernandez-Rocha C, Madsen KL, et al. Altered gut microbiome composition and function are associated with gut barrier dysfunction in healthy relatives of patients with crohn’s disease. Gastroenterology. (2022) 163:1364–76. doi: 10.1053/j.gastro.2022.07.004
PubMed Abstract | PubMed Abstract | Crossref Full Text | Google Scholar
2. Porter CK, Riddle MS, Gutierrez RL, Princen F, Strauss R, Telesco SE, et al. Cohort profile of the proteomic evaluation and discovery in an ibd cohort of tri-service subjects (predicts) study: Rationale, organization, design, and baseline characteristics. Contemp Clin trials Commun. (2019) 14:100345. doi: 10.1016/j.conctc.2019.100345
PubMed Abstract | PubMed Abstract | Crossref Full Text | Google Scholar
3. Rudbaek JJ, Agrawal M, Torres J, Mehandru S, Colombel J-F, and Jess T. Deciphering the different phases of preclinical inflammatory bowel disease. Nat Rev Gastroenterology Hepatology. (2024) 21:86–100. doi: 10.1038/s41575-023-00854-4
PubMed Abstract | PubMed Abstract | Crossref Full Text | Google Scholar
4. Rodríguez-Lago I, Agirre U, Mateos B, Mañosa M, Márquez-Mosquera L, and Menchén L. Natural history, immunological and genetic characteristics of preclinical inflammatory bowel disease (EARLY): study protocol for a prospective cohort study. Therap Adv Gastroenterol. (2025) 18:17562848251338647. doi: 10.1177/17562848251338647
PubMed Abstract | PubMed Abstract | Crossref Full Text | Google Scholar
5. Rodríguez-Lago I, Ramírez C, Merino O, Azagra I, Maiz A, Zapata E, et al. Early microscopic findings in preclinical inflammatory bowel disease. Digestive Liver Dis. (2020) 52:1467–72. doi: 10.1016/j.dld.2020.05.052
PubMed Abstract | PubMed Abstract | Crossref Full Text | Google Scholar
6. Rodríguez-Lago I, Aguirre U, Ramírez de la Piscina P, Muñagorri A, Zapata E, Higuera R, et al. Subclinical bowel inflammation increases healthcare resources utilization and steroid use before diagnosis of inflammatory bowel disease. United Eur Gastroenterology J. (2023) 11:9–18. doi: 10.1002/ueg2.12352
PubMed Abstract | PubMed Abstract | Crossref Full Text | Google Scholar
7. Torres J, Halfvarson J, Rodríguez-Lago I, Hedin CR, Jess T, Dubinsky M, et al. Results of the seventh scientific workshop of ecco: precision medicine in ibd—prediction and prevention of inflammatory bowel disease. J Crohn’s Colitis. (2021) 15:1443–54. doi: 10.1093/ecco-jcc/jjab048
PubMed Abstract | PubMed Abstract | Crossref Full Text | Google Scholar
8. Zheng GX, Terry JM, Belgrader P, Ryvkin P, Bent ZW, Wilson R, et al. Massively parallel digital transcriptional profiling of single cells. Nat Commun. (2017) 8:14049. doi: 10.1038/ncomms14049
PubMed Abstract | PubMed Abstract | Crossref Full Text | Google Scholar
9. Oelen R, de Vries DH, Brugge H, Gordon MG, Vochteloo M, single-cell eQTLGen consortium, et al. Single-cell rna-sequencing of peripheral blood mononuclear cells reveals widespread, context-specific gene expression regulation upon pathogenic exposure. Nat Commun. (2022) 13:3267. doi: 10.1038/s41467-022-30893-5
PubMed Abstract | PubMed Abstract | Crossref Full Text | Google Scholar
10. Wolf FA, Angerer P, and Theis FJ. Scanpy: large-scale single-cell gene expression data analysis. Genome Biol. (2018) 19:1–5. doi: 10.1186/s13059-017-1382-0
PubMed Abstract | PubMed Abstract | Crossref Full Text | Google Scholar
11. Wolock SL, Lopez R, and Klein AM. Scrublet: computational identification of cell doublets in single-cell transcriptomic data. Cell Syst. (2019) 8:281–91. doi: 10.1016/j.cels.2018.11.005
PubMed Abstract | PubMed Abstract | Crossref Full Text | Google Scholar
12. Hao Y, Hao S, Andersen-Nissen E, Mauck WM, Zheng S, Butler A, et al. Integrated analysis of multimodal single-cell data. Cell. (2021) 184:3573–87. doi: 10.1016/j.cell.2021.04.048
PubMed Abstract | PubMed Abstract | Crossref Full Text | Google Scholar
13. Stuart T, Butler A, Hoffman P, Hafemeister C, Papalexi E, Mauck WM, et al. Comprehensive integration of single-cell data. Cell. (2019) 177:1888–902. doi: 10.1016/j.cell.2019.05.031
PubMed Abstract | PubMed Abstract | Crossref Full Text | Google Scholar
14. Gayoso A, Lopez R, Xing G, Boyeau P, Valiollah Pour Amiri V, Hong J, et al. A python library for probabilistic analysis of single-cell omics data. Nat Biotechnol. (2022) 40:163–6. doi: 10.1038/s41587-021-01206-w
PubMed Abstract | PubMed Abstract | Crossref Full Text | Google Scholar
15. Bindea G, Mlecnik B, Hackl H, Charoentong P, Tosolini M, Kirilovsky A, et al. Cluego: a cytoscape plug-in to decipher functionally grouped gene ontology and pathway annotation networks. Bioinformatics. (2009) 25:1091–3. doi: 10.1093/bioinformatics/btp101
PubMed Abstract | PubMed Abstract | Crossref Full Text | Google Scholar
16. Mi H, Ebert D, Muruganujan A, Mills C, Albou L-P, Mushayamaha T, et al. Panther version 16: a revised family classification, tree-based classification tool, enhancer regions and extensive api. Nucleic Acids Res. (2021) 49:D394–403. doi: 10.1093/nar/gkaa1106
PubMed Abstract | PubMed Abstract | Crossref Full Text | Google Scholar
17. Luecken MD, Büttner M, Chaichoompu K, Danese A, Interlandi M, Müller MF, et al. Benchmarking atlas-level data integration in single-cell genomics. Nat Methods. (2022) 19:41–50. doi: 10.1038/s41592-021-01336-8
PubMed Abstract | PubMed Abstract | Crossref Full Text | Google Scholar
18. da Silva FAR, Pascoal LB, Dotti I, Setsuko Ayrizono M, Aguilar D, Rodrigues BL, et al. Whole transcriptional analysis identifies markers of b, t and plasma cell signaling pathways in the mesenteric adipose tissue associated with crohn’s disease. J Trans Med. (2020) 18:1–14. doi: 10.1186/s12967-020-02220-3
PubMed Abstract | PubMed Abstract | Crossref Full Text | Google Scholar
19. Mei K, Chen Z, Wang Q, Luo Y, Huang Y, Wang B, et al. The role of intestinal immune cells and matrix metalloproteinases in inflammatory bowel disease. Front Immunol. (2023) 13:1067950. doi: 10.3389/fimmu.2022.1067950
PubMed Abstract | PubMed Abstract | Crossref Full Text | Google Scholar
20. Perez K, Ngollo M, Rabinowitz K, Hammoudi N, Seksik P, Xavier RJ, et al. Metaanalysis of ibd gut samples gene expression identifies specific markers of ileal and colonic diseases. Inflammatory Bowel Dis. (2022) 28:775–82. doi: 10.1093/ibd/izab311
PubMed Abstract | PubMed Abstract | Crossref Full Text | Google Scholar
21. Nowak JK, Szymańska CJ, Glapa-Nowak A, Duclaux-Loras R, Dybska E, Ostrowski J, et al. Unexpected actors in inflammatory bowel disease revealed by machine learning from whole-blood transcriptomic data. Genes. (2022) 13:1570. doi: 10.3390/genes13091570
PubMed Abstract | PubMed Abstract | Crossref Full Text | Google Scholar
22. Irak K, Bayram M, Cifci S, and Sener G. Serum levels of nlrc4 and mcp-2/ccl8 in patients with active crohn’s disease. PloS One. (2021) 16:e0260034. doi: 10.1371/journal.pone.0260034
PubMed Abstract | PubMed Abstract | Crossref Full Text | Google Scholar
23. Duncan JA and Canna SW. The nlrc 4 inflammasome. Immunological Rev. (2018) 281:115–23. doi: 10.1111/imr.2018.281.issue-1
24. Boland BS, He Z, Tsai MS, Olvera JG, Omilusik KD, Duong HG, et al. Heterogeneity and clonal relationships of adaptive immune cells in ulcerative colitis revealed by single-cell analyses. Sci Immunol. (2020) 5:eabb4432. doi: 10.1126/sciimmunol.abb4432
PubMed Abstract | PubMed Abstract | Crossref Full Text | Google Scholar
25. Biasci D, Lee JC, Noor NM, Pombal DR, Hou M, Lewis N, et al. A blood-based prognostic biomarker in ibd. Gut. (2019) 68:1386–95. doi: 10.1136/gutjnl-2019-318343
PubMed Abstract | PubMed Abstract | Crossref Full Text | Google Scholar
26. Leibovitzh H, Lee S-H, Garay JAR, Espin-Garcia O, Xue M, Neustaeter A, et al. Immune response and barrier dysfunction-related proteomic signatures in preclinical phase of crohn’s disease highlight earliest events of pathogenesis. Gut. (2023) 72:1462–71. doi: 10.1136/gutjnl-2022-328421
PubMed Abstract | PubMed Abstract | Crossref Full Text | Google Scholar
27. Chen R, Li C, Zheng J, Fan Z, Li L, Chen M, et al. Lymphocyte subsets for predicting inflammatory bowel disease progression and treatment response: a systematic review. Front Immunol. (2024) 15:1403420. doi: 10.3389/fimmu.2024.1403420
PubMed Abstract | PubMed Abstract | Crossref Full Text | Google Scholar
Keywords: Crohn’s disease, scRNAseq, PBMC, preclinical, IBD
Citation: Kioroglou D, Egia-Mendikute L, Palazon A, Barreiro-de Acosta M, Rodríguez-Lago I and Marigorta UM (2025) Single-cell analysis reveals significant transcriptomic alterations in preclinical Crohn’s disease. Front. Immunol. 16:1539830. doi: 10.3389/fimmu.2025.1539830
Received: 04 December 2024; Accepted: 28 April 2025;
Published: 22 May 2025.
Edited by:
Olga Maria Nardone, Federico II University Hospital, ItalyReviewed by:
Maria Manuela Estevinho, Centro Hospitalar de Vila Nova de Gaia, PortugalJingtong Wu, Xiamen University, China
Copyright © 2025 Kioroglou, Egia-Mendikute, Palazon, Barreiro-de Acosta, Rodríguez-Lago and Marigorta. This is an open-access article distributed under the terms of the Creative Commons Attribution License (CC BY). The use, distribution or reproduction in other forums is permitted, provided the original author(s) and the copyright owner(s) are credited and that the original publication in this journal is cited, in accordance with accepted academic practice. No use, distribution or reproduction is permitted which does not comply with these terms.
*Correspondence: Urko M. Marigorta, dW1hcnRpbmV6QGNpY2Jpb2d1bmUuZXM=; Iago Rodríguez-Lago, aWFnby5yLmxhZ29AZ21haWwuY29t