- Department of General Surgery, The First Medical Center, Chinese People’s Liberation Army (PLA) General Hospital, Beijing, China
Background: TNFRSF12A is abnormally expressed in various malignancies, especially in stomach adenocarcinoma (STAD), which is related to tumor invasiveness and prognosis of patients. This study examined the expression pattern of TNFRSF12A in STAD and predicted immunotherapy response.
Methods: Data were derived from The Cancer Gene Atlas (TCGA), Gene Expression Omnibus (GEO), and Gene Expression Profiling Interactive Analysis (GEPIA) to analyze the expression pattern of TNFRSF12A in pan-cancer and STAD, as well as its correlation with clinical features. Biological pathways involved in TNFRSF12A were analyzed by “clusterProfiler” package. Immune cell infiltration was evaluated by “GSVA” and “CIBERSORT” packages. Immunotherapy response was assessed by TIDE score and tumor mutation burden (TMB) level. Expression level of TNFRSF12A in the single cell of STAD was analyzed by scRNA-seq. Finally, in vitro test detected the mRNA expression of TNFRSF12A in STAD cells, Wound healing and Transwell assays were performed to measure the capabilities of STAD cell to migrate and invade.
Results: TNFRSF12A was highly expressed in STAD. However, TNFRSF12A expression did not shown significant difference in relation to clinical features. TNFRSF12A exhibited notably positive correlation with many carcinogenic signaling pathways and immune cells infiltration such as T cells and macrophages. High TNFRSF12A expression group showed a higher TIDE score, Exclusion score, and TMB level than the low TNFRSF12A expression group, which indicated that STAD patients with high TNFRSF12A expression responded more poorly to immunotherapy. TNFRSF12A showed a positive relation with most of immune checkpoint genes. By scRNA-seq analysis, TNFRSF12A was chiefly expressed in Fibroblasts and Mast cells of STAD. Further, in vitro assays verified the high expression of TNFRSF12A in STAD cells, and the migration and invasion capabilities of STAD cells were notably suppressed by TNFRSF12A silencing (p<0.05).
Conclusion: The present study not only reveals the potential of TNFRSF12A as a therapeutic target for STAD, but also explores its great potential in STAD immunotherapy. This finding opens up a new way of thinking for the personalized treatment of STAD.
1 Introduction
As a frequently detected cancer globally (1, 2), gastric cancer (GC) has complicated pathogenesis, comprising environmental factor, genetic predisposition, and chronic inflammation (3–5). GC exhibits strong invasion and metastasis characteristics, which leads to the high incidence and mortality rates (6). Stomach adenocarcinoma (STAD) is a prevailing type of GC, accounting for about 90%, and has different molecular subtypes and clinical behaviors (7). Most patients have already been at the middle or advanced stages when diagnosed due to the nontypical clinical symptoms of early STAD (8). At present, endoscopic surveillance is the standard screening method that has made a breakthrough in the detection and therapy of STAD, but its high price and invasiveness is merely limited to high-risk patients (9). Surgical resection, chemotherapy, radiotherapy, and immunotherapy are commonly applied for STAD management (10). Nonetheless, the long-term survival probability of STAD is still disillusionary owing to tumor recurrence and metastasis (11). Immune checkpoint inhibitors (ICIs) have also been manifested to be resultful in controlling the development of STAD, yet they only benefit a small number of patients (12). Thus, the accurate diagnosis and effective treatment of STAD remains a serious challenge facing modern medicine; it is necessary to understand the pathogenesis of STAD and search for reliable markers.
With the deepening research on tumor microenvironment (TME), tumor-associated inflammation has been shown to regulate STAD proliferation, migration, and immune escape through cytokines and chemokines, which in turn affects patient survival (13–15). TNFRSF12A, alternatively known as Fn14, belongs to tumor necrosis factor (TNF) receptor superfamily and exerts its biological functions mainly by binding to its ligand TWEAK (16). TNFRSF12A can promote angiogenesis and regulate apoptosis, as well as also affect the immune escape ability of tumors by regulating immune cell infiltration in TME (17). It has been suggested that TNFRSF12A expression is abnormally upregulated in numerous carcinomas, such as colorectal cancer (18), glioma (19), and breast cancer (20), which is closely associated with tumor aggressiveness and patient prognosis. Particularly, TNFRSF12A has also been reported to be markedly overexpressed in GC tissues and cells, indicating a worse prognostic outcomes of GC (21). Given that STAD is a common subtype of gastric cancer, it is highly likely that TNFRSF12A also plays a critical role in the pathogenesis of STAD. Therefore, investigating the roles of TNFRSF12A in STAD is not only helpful to understand the development mechanism of STAD, but also could offer a novel therapeutic target in clinical practice.
We first acquired sample data from the public database to reveal the expression pattern of TNFRSF12A in pan-cancer and assess its correlation with patient prognosis and clinical features in STAD. Further, we analyzed the biological pathways involved in TNFRSF12A and evaluated the immune cell infiltration in STAD. TIDE score and TMB level were calculated to predict immunotherapy responses. Finally, the expression of TNFRSF12A in the single cell of STAD was assessed by scRNA-seq analysis. In vitro test was further conducted. This study confirmed the high expression of TNFRSF12A in STAD and the correlation of immune infiltration, suggesting that TNFRSF12A may be a therapeutic target for STAD. Meanwhile, the study also revealed the potential value of TNFRSF12A in the field of STAD immunotherapy, which provides key clues for in-depth investigation of the immunotherapeutic mechanism of STAD and the development of more effective therapeutic strategies.
2 Materials and methods
2.1 Data source and preprocessing
The RNA-seq data of TNFRSF12A in pan-cancer were obtained from the GEPIA database (http://gepia.cancer-pku.cn/).
Transcriptome data, clinical data, and mutation data of STAD were derived from the Cancer Gene Atlas (TCGA) database. Then, FPKM values were converted into TPM and log2 conversion was performed. The samples with complete survival data were reserved, including 32 normal samples and 350 tumor samples.
Clinical information and RNA-seq data of GSE66229 dataset as well as scRNA-seq data of GSE167297 dataset were all acquired from the GEO database. For GSE66229 dataset, the probes were transformed into gene symbols based on the annotation information, and the gene with the highest average expression was selected when corresponding to duplicate gene symbols or multiple probes.
2.2 Gene set enrichment analysis
Samples in TCGA-STAD cohort were divided by the median expression of TNFRSF12A into high and low expression groups to examine the relationship between TNFRSF12A expression and biological pathways. Kyoto Encyclopedia of Genes and Genomes (KEGG) enrichment analysis was conducted by GSEA using the gseKEGG function in the “clusterProfiler” R package (22), and the top5 KEGG pathways were screened based on the normalized enrichment score (NES). HALLMARK pathway scores were calculated using the “GSVA” R package (23), and the gene sets were derived from the MSigDB (https://www.gsea-msigdb.org/gsea/msigdb/). The relationship between HALLMARK pathways and TNFRSF12A expression was analyzed (p<0.05).
2.3 Immune cell infiltration analysis
Single sample GSEA (ssGSEA) was applied to calculate 28 types of tumor-infiltrating lymphocytes (TILs) scores with the “GSVA” R package (24), and the gene sets were obtained from a previous study (25). The “CIBERSORT” R package was employed to quantify the abundance of 22 types of immune cells in TCGA-STAD cohort (26). The correlation between immune cell infiltration and TNFRSF12A expression was analyzed.
2.4 Immunotherapy response assessment
The TIDE score and Exclusion score was calculated by TIDE algorithm (27), predicting the response of STAD patients to ICIs therapy. Moreover, the expressions of immune checkpoint genes and TMB were served as potential predictors of immunotherapy response. TMB was counted using the “maftools” R package (28).
2.5 Single cell data analysis
The scRNA-seq data of each sample in GSE167297 dataset was read by the Read10X function in the “Seurat” R package (29), retaining the cells with gene numbers of 200–2500 and mitochondrial gene ratio of <10%. Next, the SCTransform function was employed for normalization, and after principal component analysis, the “harmony” R package was applied for removing batch effects (30). Subsequently, tSNE dimensionality reduction was conducted by the RunTSNE function. The FindNeighbors and FindClusters functions (parameters: dims=1:20 and resolution=0.1) were employed to cluster the cells clustering. Cell types were annotated according to the marker genes offered by CellMarker2.0 database (31, 32).
2.6 Cell cultivation and transfection
Human gastric mucosa epithelial cell line GES-1 (CBP60512, Nanjing Cobioer Biotechnology Co., China) and STAD cell line AGS (CBP60476, Cobioer, China) were acquired beforehand. GES-1 cell line was cultivated in DMEM (CBP60512M, Cobioer, China) containing 10% fetal bovine serum (FBS), and AGS cell line was grown in RPMI-1640 (CBP60476M, Cobioer, China) encompassing 10% FBS. All cells were stored at an incubator of 5% CO2 and 37˚C. All cells were tested for mycoplasma contamination, identified as contamination-free and certified as short tandem repeat (STR).
Subsequently, to silence the TNFRSF12A in AGS cells, the small interfering (si) RNA of TNFRSF12A (si-TNFRSF12A#1 from Merck KGaA, Darmstadt, Germany and si-TNFRSF12A#2: 5’-AGGGAGAATTTATTAATAAAAGA-3’, Sangon Biotech (Shanghai) Co., Ltd., Shanghai, China) and negative control (si-NC) was applied to transfect the AGS cells through Lipofectamine 2000 (Invitrogen, USA).
2.7 Real-time quantitative PCR
Total RNA of GES-1 and AGS cells was collected utilizing the TRIzol reagent (15596026, Thermo Fisher). Then, the PrimeScript reverse transcriptase reagent Kit (RR037Q, Takara, Japan) was used to synthesize cDNA. RT−qPCR was carried out using the SYBR Green Universal Master Mix (4364344, Thermo Fisher) in a QuantStudio 3 Real-Time PCR System (Thermo Fisher). The primer pairs were designed by Sangon Biotech (Shanghai) Co., Ltd. The primer sequences for TNFRSF12A were 5’-GACCTGGACAAGTGCAT-3’ (forward) and 5’-GGTGGTGAACTTCTCTCTC-3’ (reverse), for TPSAB1 were 5’-CACCCACAGTTCTACACC-3’ (forward) and 5’-GGATCCAGTCCAAGTAGTAG-3’ (reverse), for DCN were 5’-ATGAAGGCCACTATCATCCTCC-3’ (forward) and 5’-GTCGCGGTCATCAGGAACTT-3’ (reverse), for GAPDH were 5’-CTGGGCTACACTGAGCACC-3’ (forward) and 5’-AAGTGGTCGTTGAGGGCAATG-3’ (reverse). GAPDH was applied as the housekeeping gene, and the relative mRNA expression levels of TNFRSF12A, TPSAB1, and DCN were calculated by 2-ΔΔCT method (33).
2.8 Wound healing assay
The ability of AGS cells to migrate could be measured by wound healing assay (34). In short, the transfected AGS cells were planted into 6-well plates and cultivated until a uniform monolayer was formed. An aseptic micropipette was applied to form scratch wound on the surface of AGS cells. After that, the wound images at 0 and 48h were obtained under an inverted microscope (Primo vert, ZEISS, Germany) and the wound closure rate of AGS cells was estimated employing the ImageJ software (version 1.42G) (32).
2.9 Transwell assay
The ability of AGS cells to invade was measured by Transwell assay (35). Briefly, diluted Matrigel (Corning, USA) was pre-coated in the Transwell chamber (8.0μm, Corning, USA). Next, the transfected AGS cells (2×104 cells/well) were suspended in 200µL serum-deleted RPMI-1640 medium and cultured in the upper chamber, whereas the lower chamber was supplemented with 600µL RPMI-1640 medium encompassing 10% FBS. After 48h incubation, the invaded AGS cells were fixed in 4% paraformaldehyde (ZY640017RE, Zeye, Shanghai, China) for 10 minutes, stained by 0.1% crystal violet (ZY-9248, Zeye, Shanghai, China) for 5 min, and washed twice using phosphate buffer solution. Under the Primo vert inverted microscope (ZEISS, Germany), the number of invaded AGS cells was counted from six randomly picked fields.
2.10 Statistical analysis
R software (version 4.2.0) and GraphPad Prism (version 8.0) were applied for statistical analysis. All experiments were performed in triplicate and data were shown as mean ± standard deviation. The difference between two continuous variables was compared by t test. For experiments involving three or more variables, we employed the analysis of variance (ANOVA) test to assess the overall differences among the groups. Subsequently, a Sidak’s multiple comparisons test was conducted to determine the specific pairwise differences between each group. The correlation analysis was conducted by Spearman method. To evaluate the survival differences, Kaplan-Meier (K-M) survival analysis was performed by “survminer” R package (36). A p<0.05 denoted a statistical significance level.
3 Results
3.1 TNFRSF12A expression in pan-cancer and its correlation with clinical features in STAD
The expression data of TNFRSF12A was acquired from the GEPIA database. It was found that compared to normal samples, TNFRSF12A was high-expressed in most tumors, such as STAD, colon adenocarcinoma (COAD), lung adenocarcinoma (LUAD), liver hepatocellular carcinoma (LIHC), and glioblastoma (GBM) (Figure 1A). Further, K-M curves demonstrated that the progression-free survival (PFS) and overall survival (OS) rates of high TNFRSF12A expression group in STAD were all lower than that of low TNFRSF12A expression group (Figures 1B, C). Meanwhile, the relationship between TNFRSF12A expression and clinicopathologic characteristics in STAD was analyzed by Spearman method. The TNFRSF12A expression was significantly different among different T stages (p=0.0069), and the expression of TNFRSF12A in T2, T3, and T4 stages was higher than that in T1 stage (Figure 1D). However, the expression of TNFRSF12A in different N stages (N0, N1, N2, N3), M stages (M0, M1), Stage (I, II, III, IV), or Grade (G1, G2, G3) showed no significant difference (p>0.05) (Figures 1E–H). This result implies that TNFRSF12A is highly expressed in most of the tumors, but there is no significant relationship between TNFRSF12A expression and clinicopathological features in STAD.
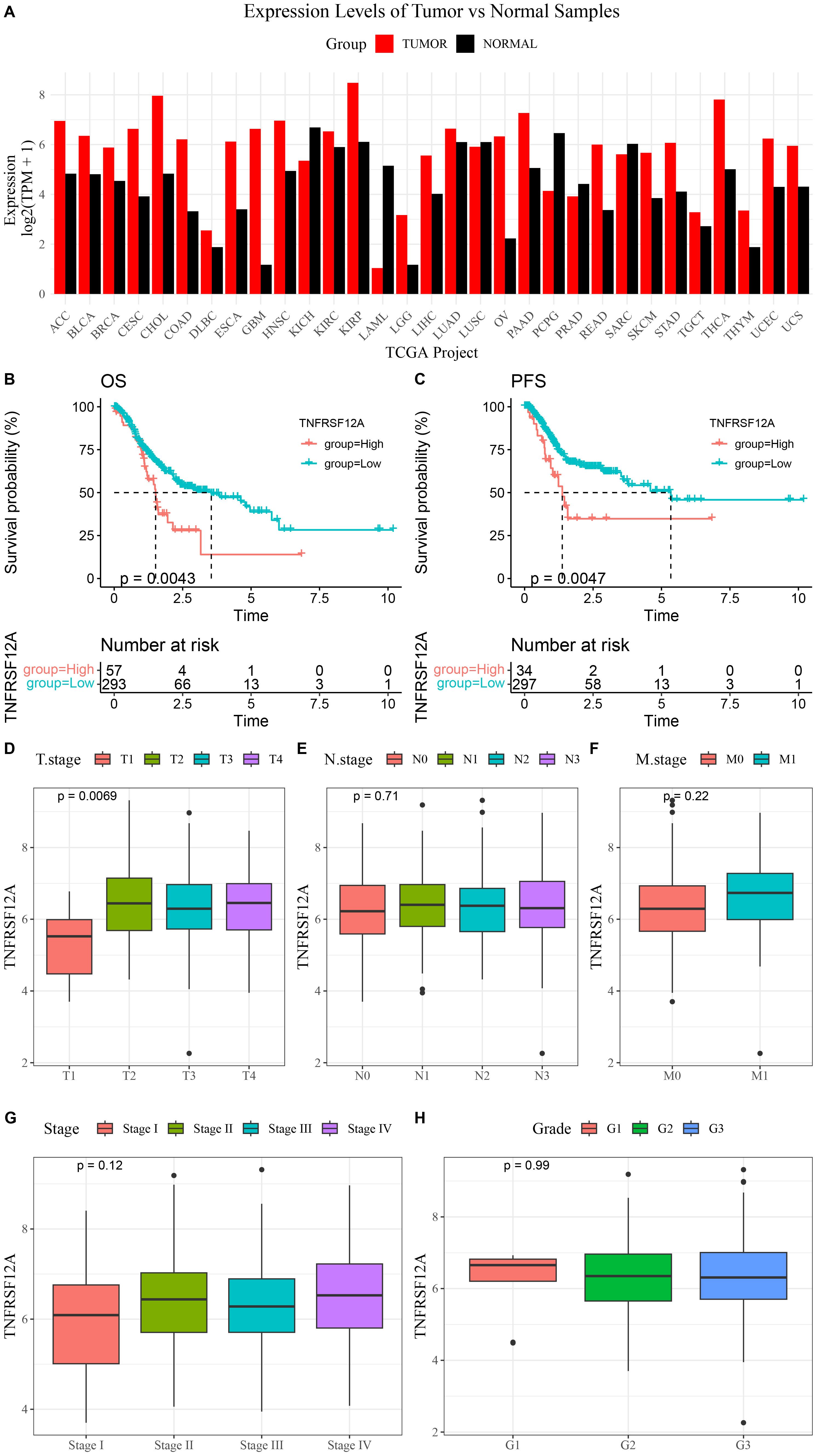
Figure 1. Expression of TNFRSF12A in pan-cancer and its correlation with clinical features in STAD. (A) Expression levels of TNFRSF12A in pan-cancer; (B) Kaplan-Meier (K-M) curve of overall survival (OS) for high and low TNFRSF12A expression groups in STAD; (C) K-M curve of progression-free survival (PFS) for high and low TNFRSF12A expression groups in STAD; (D) Relationship between TNFRSF12A expression and T stages in STAD; (E) Relationship between TNFRSF12A expression and N stages in STAD; (F) Relationship between TNFRSF12A expression and M stages in STAD; (G) Relationship between TNFRSF12A expression and Stage in STAD; (H) Relationship between TNFRSF12A expression and Grade in STAD.
3.2 TNFRSF12A expression and its relationship with clinical features in GSE66229 dataset
We further analyzed the relationship of TNFRSF12A with prognosis and clinicopathologic features in GSE66229 dataset. The expression of TNFRSF12A in STAD tissue was markedly higher than that in normal gastric tissue (Figure 2A). Based on K-M survival analysis, high TNFRSF12A expression group exhibited a lower disease-free survival (DFS) probability than low TNFRSF12A expression group (Figure 2B), indicating that STAD patients with a high TNFRSF12A expression may have a worse prognosis. Furthermore, there was no significant difference of TNFRSF12A expression in different Stage (I-IV), T stages, N stages, and M stages (p>0.05) (Figures 2C–F). This result implies that the TNFRSF12A high-expression group possessed a worse prognosis, but there was no significant difference in TNFRSF12A expression in STAD patients with different stages.
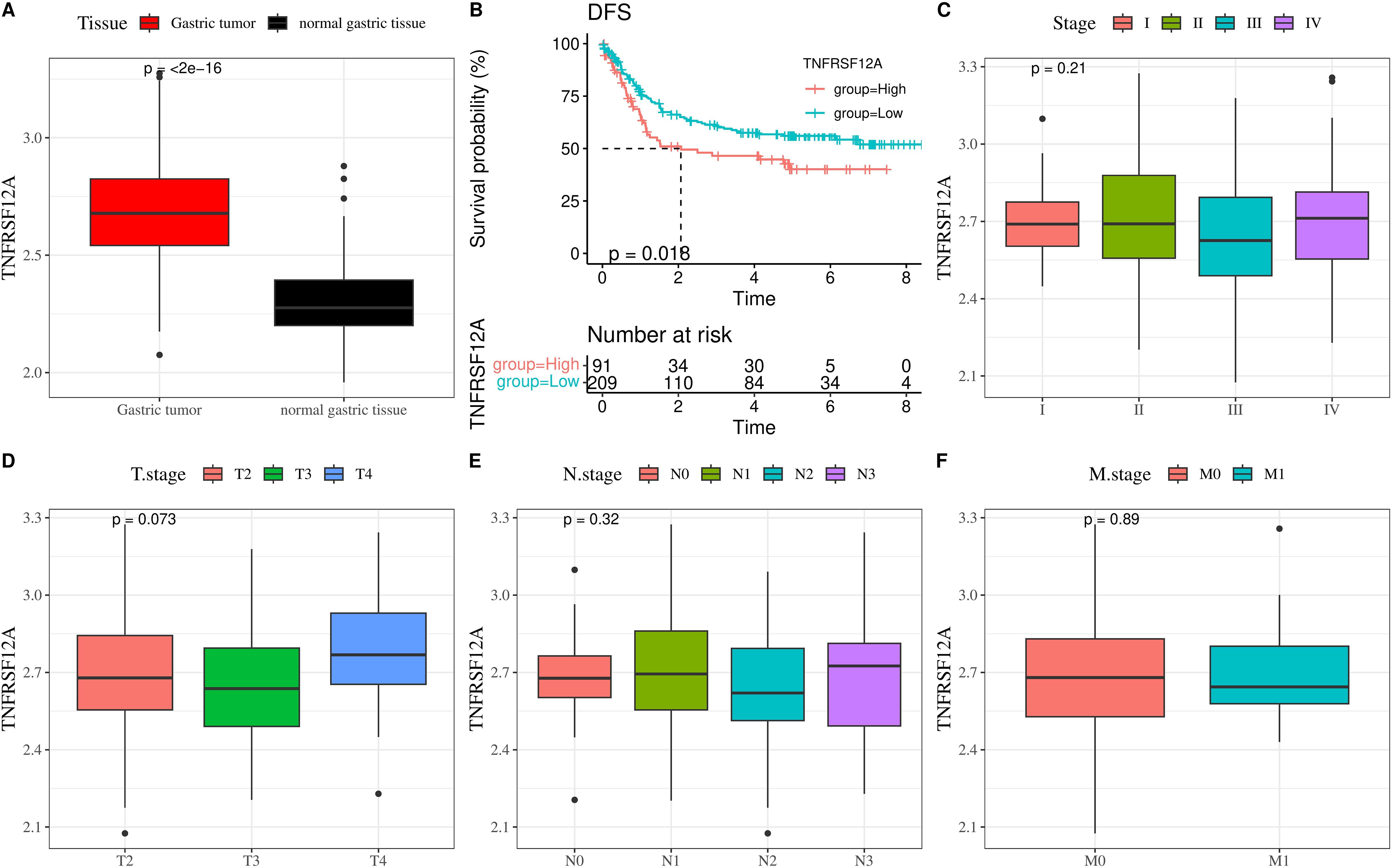
Figure 2. TNFRSF12A expression and its relationship with clinical features in GSE66229 dataset. (A) Expression level of TNFRSF12A in STAD tissue and normal gastric tissue; (B) K-M curve of disease-free survival (DFS) for high and low TNFRSF12A expression groups; (C) Correlation between TNFRSF12A expression and Stage; (D) Correlation between TNFRSF12A expression and T stage; (E) Correlation between TNFRSF12A expression and N stage; (F) Correlation between TNFRSF12A expression and M stage.
3.3 Correlation between TNFRSF12A expression and biological pathways
Biological pathways involved in TNFRSF12A were identified by GSEA, and the samples in TCGA-STAD cohort were split into high and low groups according to the median expression level of TNFRSF12A. KEGG enrichment analysis demonstrated that the high expression group mainly participated in the pathways of Bladder cancer, extracellular matrix (ECM)-receptor interaction, Hepatitis C, Proteasome, and Virion-Hepatitis viruses (Figure 3A). Whereas, low expression group was principally enriched in the Nicotine addiction, Pancreatic secretion, Primary immunodeficiency, Serotonergic synapse, and Taste transduction pathways (Figure 3B). Additionally, the relationship between TNFRSF12A expression and HALLMARK pathways was analyzed, suggesting that TNFRSF12A exhibited notably positive correlation with many carcinogenic signaling pathways, such as reactive oxygen species (ROS) pathway, TNFA signaling via NFKB, P53 pathway, glycolysis, epithelial-mesenchymal transition (EMT), etc (Figure 3C). These results provided new lights on the potential role of TNFRSF12A in STAD. This result shows that TNFRSF12A is closely associated with oncogenic signaling pathways, suggesting its potential as a biomarker.
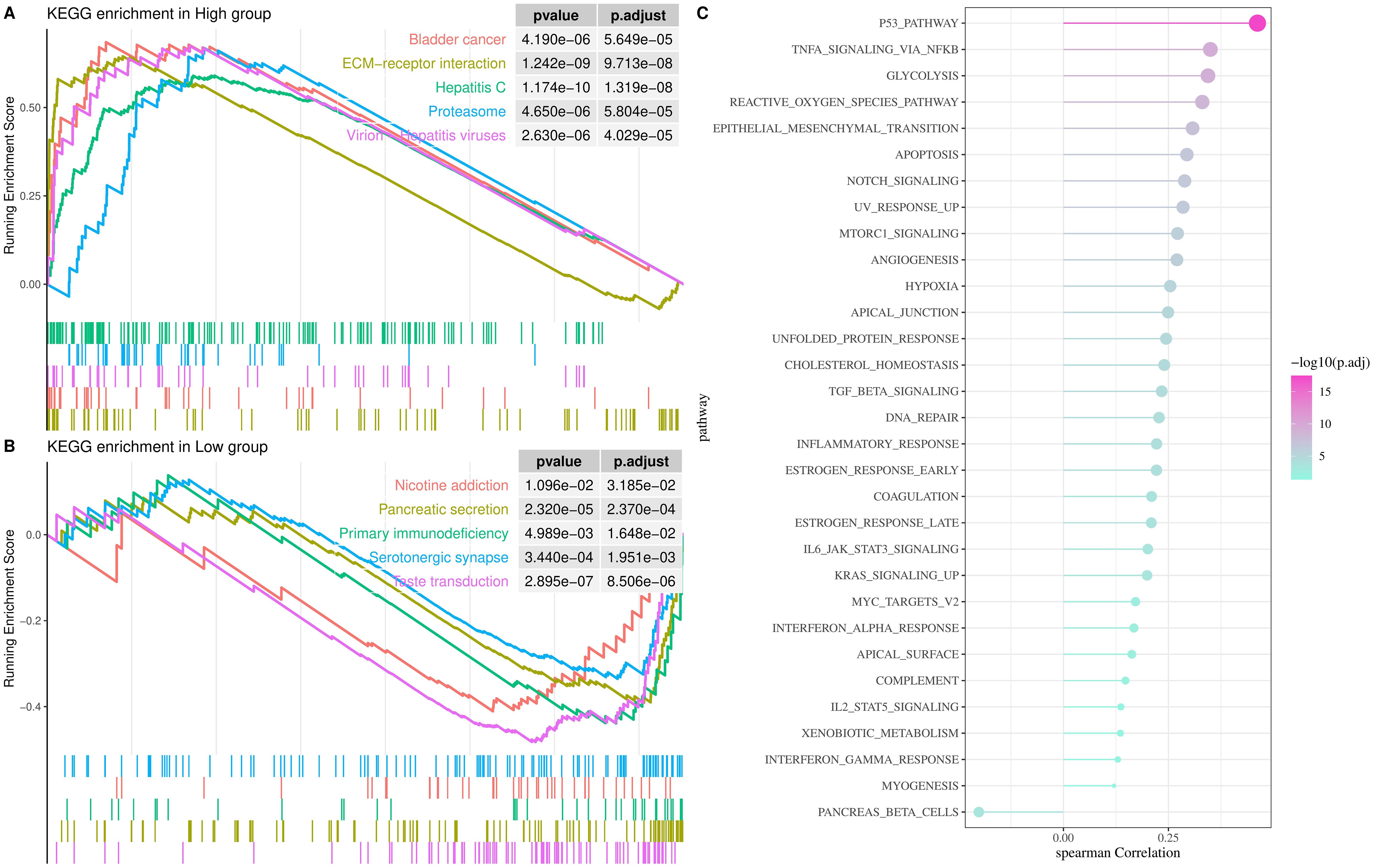
Figure 3. Analysis of biological pathways involved in TNFRSF12A in TCGA-STAD cohort. (A) KEGG enrichment pathways for high TNFRSF12A expression group; (B) KEGG enrichment pathways for low TNFRSF12A expression group; (C) Correlation between TNFRSF12A expression and HALLMARK pathways.
3.4 Relationship between TNFRSF12A expression and immune cell infiltration
The immune cell infiltration of TNFRSF12A in STAD was evaluated by ssGSEA and CIBERSORT algorithm. It was found that TNFRSF12A was significantly positively correlated with the infiltration of numerous immune cells, comprising Regulatory T cell, central memory CD8 T cell, T follicular helper cell, activated Dendritic cell, Natural Killer (NK) cell, Macrophage, Mast cells (MCs), central memory CD4 T cell, NKT cell, Neutrophils, and so on (Figures 4A, B). These findings manifested that TNFRSF12A may exert an essential role in regulating the TME in STAD.
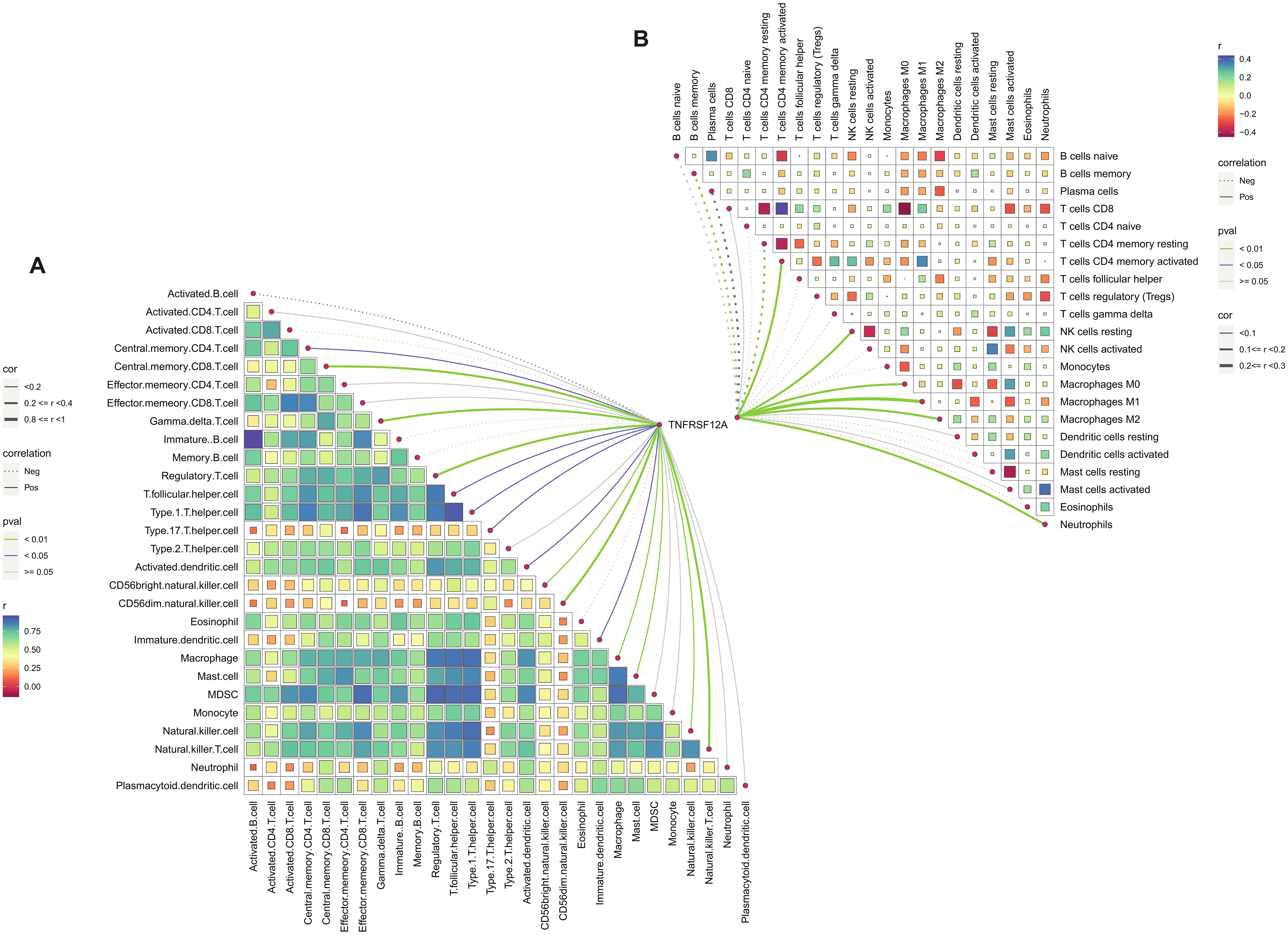
Figure 4. Correlation analysis of TNFRSF12A expression and immune cell infiltration. (A) Correlation between TNFRSF12A and 28 tumor-infiltrating lymphocytes (TILs) assessed by ssGSEA; (B) Correlation between TNFRSF12A and 22 immune cells evaluated by CIBERSORT.
3.5 Prediction of immunotherapy responses between high and low TNFRSF12A expression groups
TIDE score and Exclusion score of high TNFRSF12A expression group were all markedly higher than that of low TNFRSF12A expression group (Figures 5A, C), and TNFRSF12A exhibited positive relationship with TIDE score and Exclusion score (Figures 5B, D). This suggested that STAD patients with high TNFRSF12A expression might have a higher likelihood of immune escape and less benefit from ICIs therapy. Additionally, high TNFRSF12A expression group had a higher TMB compared to low TNFRSF12A expression group (Figure 5E), which may affect the therapeutic response of STAD patients to ICIs. TNFRSF12A was positively correlated with most of the immune checkpoint genes, including BTN2A2, IDO1, TDO2, ADORA2A, PDCD1, CTLA4, CD160, HAVCR2, TIGIT, KIR2DL3, etc (Figure 5F). These outcomes further demonstrated the important role of TNFRSF12A in evaluating the response of STAD patients to ICIs therapy, providing a potential target for clinical application.
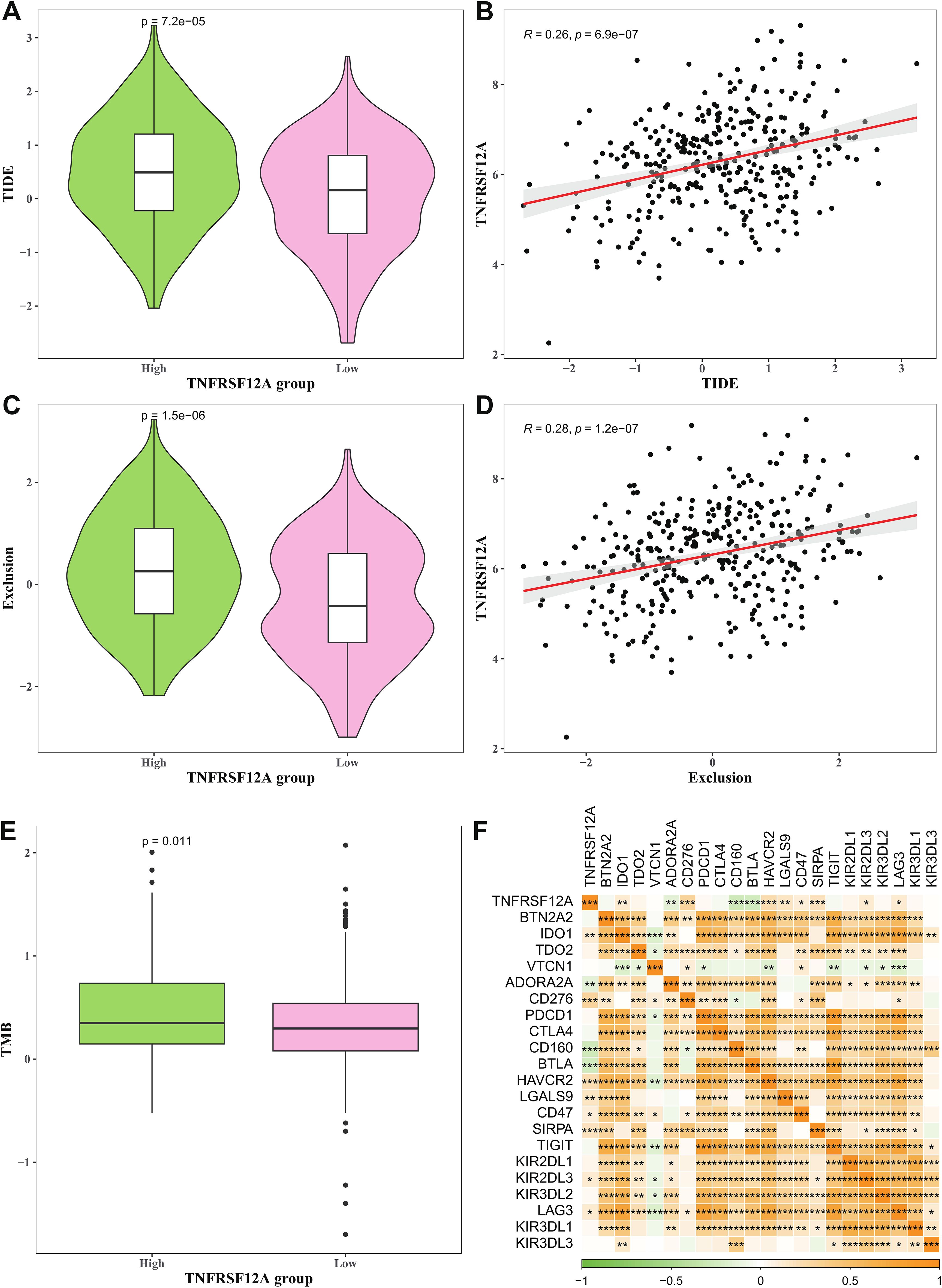
Figure 5. Prediction of immunotherapy response between high and low TNFRSF12A expression groups. (A) TIDE score in high and low TNFRSF12A expression groups; (B) Correlation between TNFRSF12A and TIDE score; (C) Exclusion score in high and low TNFRSF12A expression groups; (D) Correlation between TNFRSF12A and Exclusion score; (E) TMB in high and low TNFRSF12A expression groups; (F) Correlation between TNFRSF12A and immune checkpoint genes; *** means p<0.001, ** means p<0.01, * means p<0.05.
3.6 Expression of TNFRSF12A in the single cell of STAD
The scRNA-seq data of STAD in GSE167297 dataset was analyzed, revealing 10 cell clusters (Figure 6A). Then, 8 cell types were determined (Figure 6B), containing B/Plasma cells (CD79A, MZB1, MS4A1), Endothelial cells (EMCN, VWF, PLVAP), Epithelial cells (KRT18, EPCAM, KRT8), Fibroblasts (COL1A1, COL3A1, COL1A2, DCN), Mast cells (TPSAB1, CPA3), Myeloid cells (LYZ, S100A9), NKT cells (CD8A, NKG7, GZMA), and T cells (CD3E, IL7R) (Figure 6C). Further, the expression level of TNFRSF12A in each cell type was displayed by a violin plot, discovering that TNFRSF12A was primarily expressed in Fibroblasts and Mast cells (Figure 6D). Hence, we selected the marker genes of Fibroblasts (DCN) and Mast cells (TPSAB1) for subsequent in vitro validation assays.
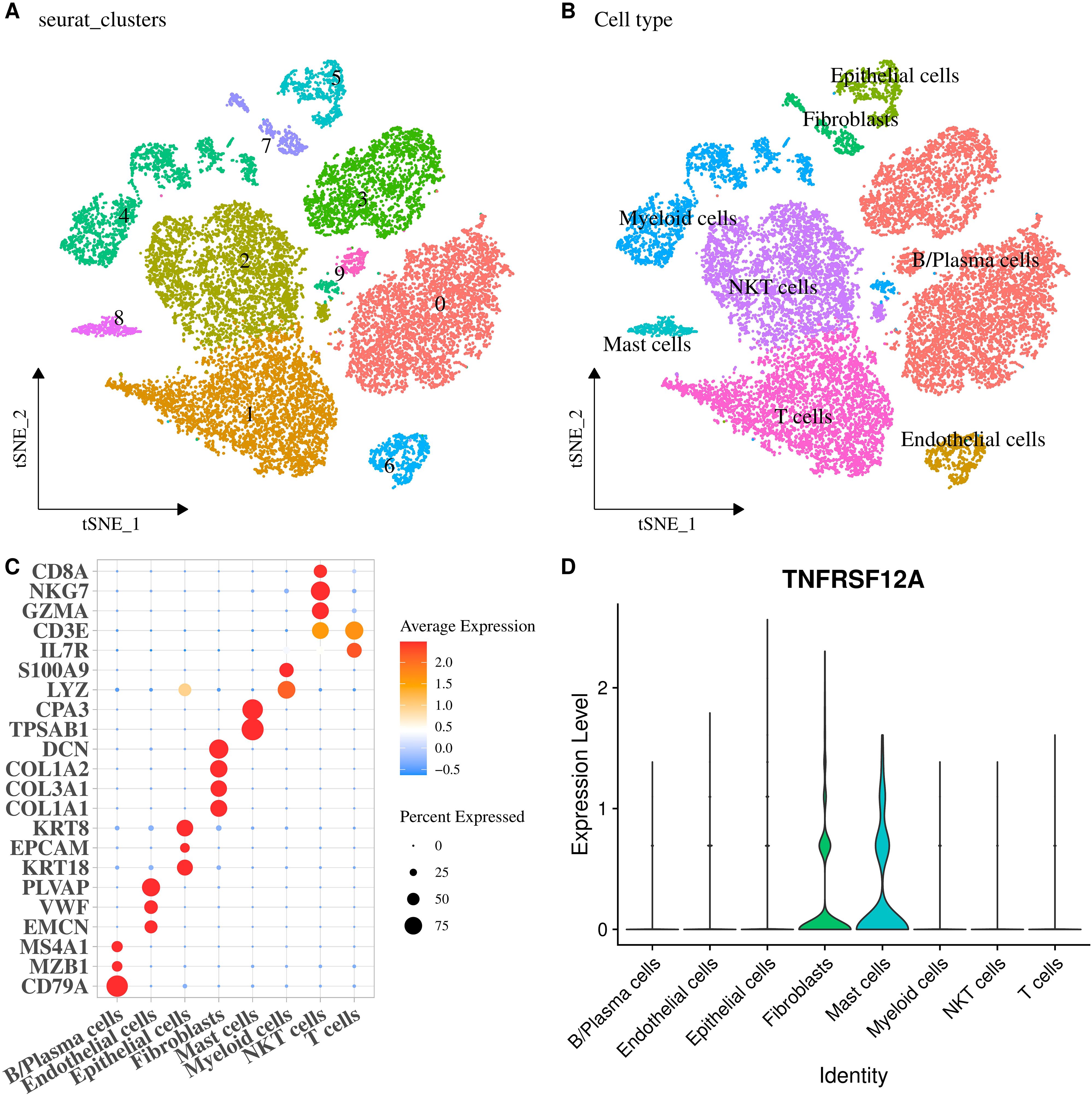
Figure 6. Expression of TNFRSF12A in the single cell of STAD. (A) The tSNE plot of cell clusters in STAD; (B) The tSNE plot of cell types in STAD; (C) Bubble diagram of marker genes expression in each cell type; (D) Expression levels of TNFRSF12A in each cell type.
3.7 TNFRSF12A silencing notably suppressed the migratory and invasive abilities of STAD cells
The relative mRNA expressions of TNFRSF12A, TPSAB1, and DCN in human gastric mucosa epithelial cell line GES-1 and STAD cell line AGS were detected through RT−qPCR. It was found that compared with GES-1 cells, TNFRSF12A, TPSAB1, and DCN were all highly expressed in AGS cells (Figure 7A). To perform siRNA transfection targeting TNFRSF12A while minimizing off-target effects, two targeting sequences were selected. The results of RT−qPCR confirmed the success of the transfection (p<0.05) (Figure 7B). Subsequently, si-TNFRSF12A#2 was chosen for the subsequent experiments. Thereafter, Wound healing assay displayed that the wound closure rate of AGS cells was significantly decreased via TNFRSF12A silencing (Figure 7C). Moreover, Transwell assay revealed that the number of invaded AGS cells was markedly declined via TNFRSF12A silencing (Figure 7D). These data supported that TNFRSF12A functioned crucially in STAD cell migration and invasion, which may be a promising target for controlling STAD progression.
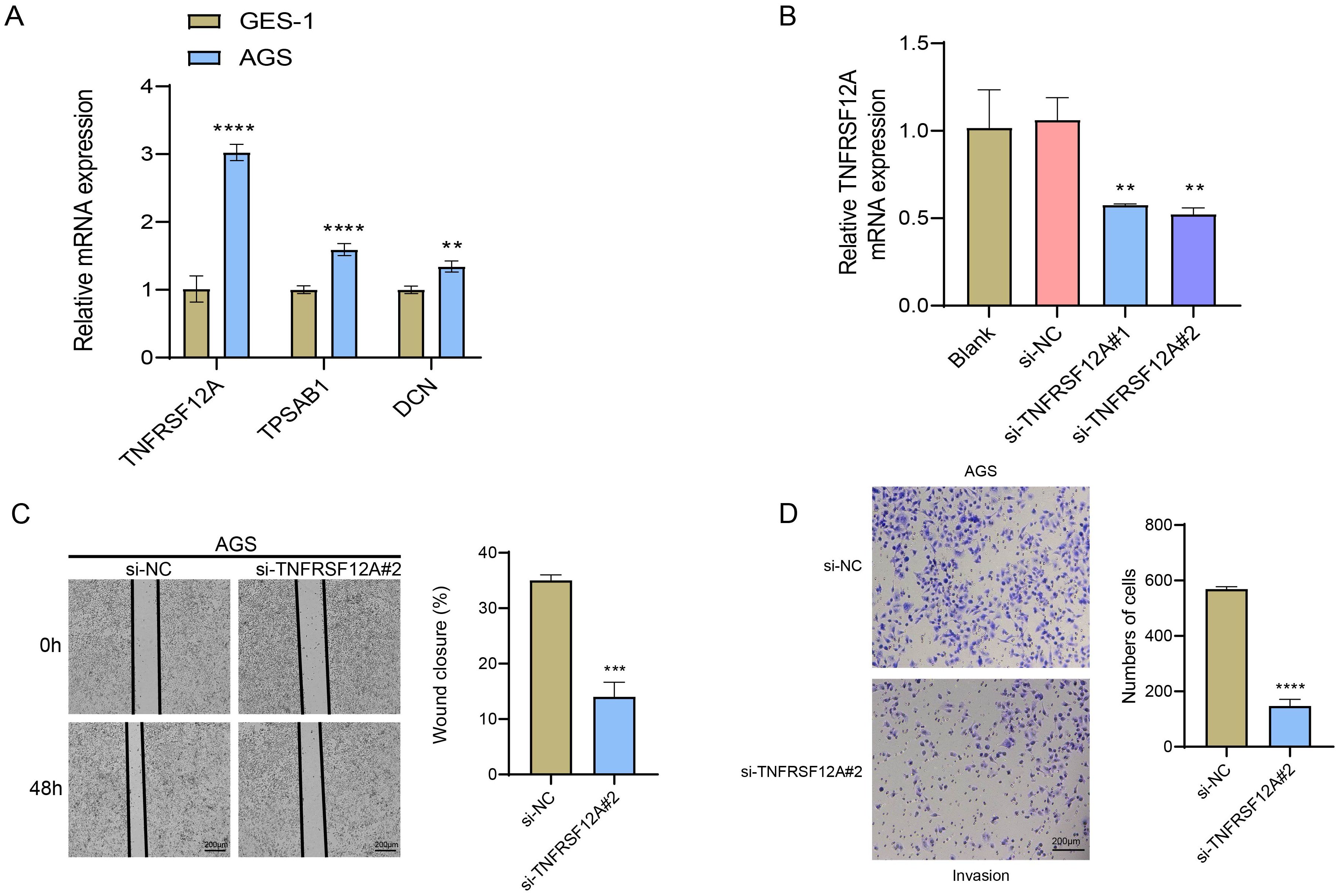
Figure 7. In vitro verification utilizing STAD cells. (A) Relative mRNA expression levels of TNFRSF12A, TPSAB1, and DCN in human gastric mucosa epithelial cell line GES-1 and STAD cell line AGS detected by RT−qPCR; (B) RT-qPCR to verify the success of TNFRSF12A transfection; (C) Cell migration of TNFRSF12A-silenced AGS assessed by Wound healing assay; (D) Cell invasion of TNFRSF12A-silenced AGS evaluated by Transwell assay. And ** indicates p<0.01, *** indicates p<0.001, **** indicates p<0.0001.
4 Discussion
TNFRSF12A is expressed in various human tissues, containing liver, heart, lung, and skeletal muscle (37). Numerous evidences have manifested that the dysregulation of TNFRSF12A plays an important part in the triggering and development of malignant tumors (38). TNFRSF12A is also found to be overexpressed in various carcinomas, which usually indicates a poor prognosis (39). In this study, we verified the high expression of TNFRSF12A in STAD by bioinformatics as well as in vitro experiments and found that it may affect STAD immunotherapy by influencing immune infiltration, a finding that provides a new therapeutic target for personalized treatment of STAD.
The biological pathways involved in TNFRSF12A were analyzed by GSEA in this study, suggesting that TNFRSF12A was positively related to many carcinogenic signaling pathways, such as P53 pathway, glycolysis, TNFA signaling via NFKB, ROS pathway, EMT, and so on. P53 as a tumor suppressor is mutated in around 50% of STAD, and these mutations are more frequent in intestinal tumors than diffuse tumors (40). The gene set “TNFA signaling via NFKB” contains 182 genes, such as TNF-α, NFKB, and the other inflammatory cytokines (41). TNF-α and NFKB are relevant to several important biological processes, including inflammatory response, immune regulation, tumorigenesis, and tumor cell apoptosis (42). In addition, glycolysis pathway as the main source of energy acquisition for cancer tissues significantly impacts the tumor growth, invasiveness, chemotherapy resistance, TME, and immune escape (43). Elevated glycolysis belongs to a part of the “Warburg effect”, enabling STAD cells to produce lactic acid, which provides energy for cell biosynthesis and cell division (44). Oxidative stress represents a disorder of antioxidant defense system implicated in the production of excessive ROS in tumor cells, which is connected with the angiogenesis, DNA damage, and tumor metastasis (45). Besides, EMT is a crucial process in the development of epithelial malignancies, including STAD, promoting the migration and invasion of cancer cells (46, 47). Hence, these carcinogenic signaling pathways may exert a crucial role in the triggering of STAD and its development, and these data can offer new clues to the potential role of TNFRSF12A in STAD.
Data from single-cell analyses show that TNFRSF12A was expressed predominantly in fibroblasts and mast cells. Santi et al. used the expression levels of α-SMA and TNFRSF12A to differentiate between cancer associated fibroblast (CAF) subpopulations (48). TNFRSF12A+ CAF plays a key role in the immunosuppression of intestinal-type gastric adenocarcinoma (IGAC) (a type of STAD) acts as a key mediator in immunosuppression and has potential as an immunomodulator (48). Furthermore, TNFRSF12A was found to act as a C2 ALOX5+ MCs and tumor cell key receptor in the communication pathway that plays a role in cervical cancer progression (49). These evidences imply that TNFRSF12A in CAF and MC may influence the development of STAD.
An increasing number of researches have proved that immune cell infiltration in TME is closely relevant to patient prognosis and conducive to the prediction of immunotherapy response in STAD (50). In this present study, we found that TNFRSF12A was notably positively related to the infiltration of most immune cells in STAD, for instance central memory CD4 T cell, Macrophage, Regulatory T cell, T follicular helper cell, central NK cell, memory CD8 T cell, NKT cell, activated Dendritic cell, Mast cell, Neutrophils. Macrophage serves as an important immunosuppressive cell and hampers the activation of NK cells and CD8 T cells (51). Mast cell can promote tumor proliferation and invasion directly, or indirectly via regulating TME (52). A study of tumor-bearing mice has indicated that Neutrophils can facilitate the tumorigenesis and aggressiveness of GC cells by mediating EMT (53). Thus, TNFRSF12A may play a pivotal role in regulating the TME in STAD. Furthermore, TIDE score and TMB are widely utilized as predictive indicators for STAD patients during ICIs therapy, contributing to the clinical decision-making (54). In this study, we found that the TIDE score, Exclusion score, and TMB level of high TNFRSF12A expression group were all markedly higher than that of low TNFRSF12A expression group, demonstrating that STAD patients with high TNFRSF12A expression might have stronger immune escape and poorer response to immunotherapy (55). In addition, this study demonstrated that TNFRSF12A was positively correlated with most of the immune checkpoint genes, and previous studies have also found that silencing of TNFRSF12A can inhibit GC cell viability, increase T cell proliferation, and affect the NF-kB pathway (21). These findings imply that TNFRSF12A is highly likely to have an important impact on the body’s immune response by regulating the proliferation process of T cells, which in turn suggests its potential role in the field of tumor immunotherapy. Taken together, these outcomes further emphasized the important role of TNFRSF12A in STAD, providing a promising therapeutic target for clinical application.
5 Conclusion
To conclude, the present work discovered that TNFRSF12A was high-expressed in STAD, which was linked to the low survival rate and poor prognosis. TNFRSF12A showed the positive correlation with many carcinogenic signaling pathways and immune cells infiltration. STAD patients with high TNFRSF12A expression may have stronger immune escape and poorer immunotherapy response. These outcomes could provide a new insight on the role of TNFRSF12A in STAD and supply a potential therapeutic target for STAD.
Data availability statement
The datasets presented in this study can be found in online repositories. The names of the repository/repositories and accession number(s) can be found in the article/supplementary material.
Author contributions
LS: Conceptualization, Formal Analysis, Methodology, Resources, Software, Validation, Writing – original draft, Writing – review & editing. LZ: Formal Analysis, Investigation, Methodology, Project administration, Resources, Visualization, Writing – original draft, Writing – review & editing. ZW: Conceptualization, Investigation, Methodology, Project administration, Validation, Writing – review & editing. XY: Investigation, Methodology, Project administration, Resources, Software, Supervision, Validation, Visualization, Writing – review & editing. JY: Conceptualization, Data curation, Formal Analysis, Investigation, Methodology, Project administration, Writing – review & editing. ZY: Methodology, Project administration, Resources, Software, Writing – original draft. LL: Conceptualization, Formal Analysis, Methodology, Resources, Visualization, Writing – original draft, Writing – review & editing. JL: Conceptualization, Funding acquisition, Investigation, Methodology, Resources, Supervision, Visualization, Writing – original draft, Writing – review & editing.
Funding
The author(s) declare that financial support was received for the research and/or publication of this article. This work was supported by the National Natural Science Foundation of China (No.82303414) and Project of PLA General Hospital Project (No.22QNCZ026).
Conflict of interest
The authors declare that the research was conducted in the absence of any commercial or financial relationships that could be construed as a potential conflict of interest.
Generative AI statement
The author(s) declare that no Generative AI was used in the creation of this manuscript.
Publisher’s note
All claims expressed in this article are solely those of the authors and do not necessarily represent those of their affiliated organizations, or those of the publisher, the editors and the reviewers. Any product that may be evaluated in this article, or claim that may be made by its manufacturer, is not guaranteed or endorsed by the publisher.
Glossary
COAD: colon adenocarcinoma
DFS: disease-free survival
ECM: extracellular matrix
EMT: epithelial-mesenchymal transition
FBS: fetal bovine serum
FPKM: fragments per kilobase of transcript per million fragments mapped
GBM: glioblastoma
GC: gastric cancer
GEO: Gene Expression Omnibus
GEPIA: Gene Expression Profiling Interactive Analysis
GSEA: gene set enrichment analysis
ICIs: immune checkpoint inhibitors
KEGG: Kyoto Encyclopedia of Genes and Genomes
K-M: Kaplan-Meier
LIHC: liver hepatocellular carcinoma
LUAD: lung adenocarcinoma
MSigDB: Molecular Signatures Database
NES: normalized enrichment score
NFKB: nuclear factor kappa B
NK: Natural Killer
OS: overall survival
PFS: progression-free survival
RNA-seq: RNA sequencing
ANOVA: analysis of variance
ROS: reactive oxygen species
RT−qPCR: real time quantitative PCR
scRNA-seq: single-cell RNA sequencing
si: small interfering
ssGSEA: single sample gene set enrichment analysis
STAD: stomach adenocarcinoma
TCGA: the Cancer Gene Atlas
TIDE: Tumor Immune Dysfunction and Exclusion
TILs: tumor-infiltrating lymphocytes
TMB: tumor mutation burden
TME: tumor microenvironment
TNF: tumor necrosis factor
TPM: transcripts per million
tSNE: t-Distributed Stochastic Neighbor Embedding
CAF: cancer associated fibroblast
MC: mast cell
IGAC: intestinal-type gastric adenocarcinoma
STR: short tandem repeat
References
1. Su X, Zhang J, Luo X, Yang W, Liu Y, Liu Y, et al. LncRNA LINC01116 promotes cancer cell proliferation, migration and invasion in gastric cancer by positively interacting with lncRNA CASC11. OncoTargets Ther. (2019) 12:8117–23. doi: 10.2147/OTT.S208133
2. Fatima S, Song Y, Zhang Z, Fu Y, Zhao R, Malik K, et al. Exploring the pharmacological mechanisms of P-hydroxylcinnamaldehyde for treating gastric cancer: A pharmacological perspective with experimental confirmation. Curr Mol Pharmacol. (2024) 17:e18761429322420. doi: 10.2174/0118761429322420241112051105
3. Jaroenlapnopparat A, Bhatia K, Coban S. Inflammation and gastric cancer. Dis (Basel Switzerland). (2022) 10:35. doi: 10.3390/diseases10030035
4. Chandarana CV, Mithani NT, Singh DV, Kikani UB. Vibrational spectrophotometry: A comprehensive review on the diagnosis of gastric and liver cancer. Curr Pharm Anal. (2024) 20:453–65. doi: 10.2174/0115734129322567240821052326
5. Song J, Xu X, He S, Wang N, Bai Y, Chen Z, et al. Myristicin suppresses gastric cancer growth via targeting the EGFR/ERK signaling pathway. Curr Mol Pharmacol. (2023) 16(7):712–24. doi: 10.2174/1874467216666230103104600
6. Wang H, Luo Y, Chu Z, Ni T, Ou S, Dai X, et al. Poria acid, triterpenoids extracted from Poria cocos, inhibits the invasion and metastasis of gastric cancer cells. Molecules (Basel Switzerland). (2022) 27:3629. doi: 10.3390/molecules27113629
7. Luo WZ, Li X, Wu XX, Shang YW, Meng DH, Chen YL, et al. MAGED4B is a poor prognostic marker of stomach adenocarcinoma and a potential therapeutic target for stomach adenocarcinoma tumorigenesis. Int J Gen Med. (2023) 16:1681–93. doi: 10.2147/IJGM.S401507
8. Zhou X, Nie H, Wang C, Yu X, Yang X, He X, et al. Prognostic value and therapeutic potential of NEK family in stomach adenocarcinoma. J Cancer. (2024) 15:3154–72. doi: 10.7150/jca.90197
9. Yu P, Chen P, Wu M, Ding G, Bao H, Du Y, et al. Multi-dimensional cell-free DNA-based liquid biopsy for sensitive early detection of gastric cancer. Genome Med. (2024) 16:79. doi: 10.1186/s13073-024-01352-1
10. Jin C, Lu X, Yang M, Hou S. Integrative analysis indicates the potential values of ANKRD53 in stomach adenocarcinoma. Discover Oncol. (2024) 15:188. doi: 10.1007/s12672-024-01054-5
11. Chen H, Zheng Z, Yang C, Tan T, Jiang Y, Xue W. Machine learning based intratumor heterogeneity signature for predicting prognosis and immunotherapy benefit in stomach adenocarcinoma. Sci Rep. (2024) 14:23328. doi: 10.1038/s41598-024-74907-2
12. Yang J, Jin F, Li H, Shen Y, Shi W, Wang L, et al. Identification of mitochondrial respiratory chain signature for predicting prognosis and immunotherapy response in stomach adenocarcinoma. Cancer Cell Int. (2023) 23:69. doi: 10.1186/s12935-023-02913-x
13. Ren N, Liang B, Li Y. Identification of prognosis-related genes in the tumor microenvironment of stomach adenocarcinoma by TCGA and GEO datasets. Bioscience Rep. (2020) 40:BSR20200980. doi: 10.1042/BSR20200980
14. Salleh EA, Lee YY, Zakaria AD, Jalil NAC, Musa M. Cancer-associated fibroblasts of colorectal cancer: Translational prospects in liquid biopsy and targeted therapy. Biocell. (2023) 47:2233–44. doi: 10.32604/biocell.2023.030541
15. Qiu H, Zhang X, Yu H, Gao R, Shi J, Shen T. Identification of potential targets of triptolide in regulating the tumor microenvironment of stomach adenocarcinoma patients using bioinformatics. Bioengineered. (2021) 12:4304–19. doi: 10.1080/21655979.2021.1945522
16. Xiong X, Hu T, Yin Z, Zhang Y, Chen F, Lei P. Research advances in the study of sleep disorders, circadian rhythm disturbances and Alzheimer’s disease. Front Aging Neurosci. (2022) 14. doi: 10.3389/fnagi.2022.944283
17. Liao M, Liao J, Qu J, Shi P, Cheng Y, Pan Q, et al. Hepatic TNFRSF12A promotes bile acid-induced hepatocyte pyroptosis through NFκB/Caspase-1/GSDMD signaling in cholestasis. Cell Death disease. (2023) 9:26. doi: 10.1038/s41420-023-01326-z
18. Wang C, Zhao Y, Chen Y, Shi Y, Yang Z, Wu W, et al. The oncogenic role of TNFRSF12A in colorectal cancer and pan-cancer bioinformatics analysis. Cancer Res Treat. (2024) 57:212–28. doi: 10.4143/crt.2024.408
19. Zhang Y, Yang X, Zhu XL, Wang ZZ, Bai H, Zhang JJ, et al. A novel immune-related prognostic biomarker and target associated with Malignant progression of glioma. Front Oncol. (2021) 11:643159. doi: 10.3389/fonc.2021.643159
20. Yang J, Min KW, Kim DH, Son BK, Moon KM, Wi YC, et al. High TNFRSF12A level associated with MMP-9 overexpression is linked to poor prognosis in breast cancer: Gene set enrichment analysis and validation in large-scale cohorts. PLoS One. (2018) 13:e0202113. doi: 10.1371/journal.pone.0202113
21. Xia L, Jiang L, Chen Y, Zhang G, Chen L. ThPOK transcriptionally inactivates TNFRSF12A to increase the proliferation of T cells with the involvement of the NF-kB pathway. Cytokine. (2021) 148:155658. doi: 10.1016/j.cyto.2021.155658
22. Song Z, Yu J, Wang M, Shen W, Wang C, Lu T, et al. CHDTEPDB: transcriptome expression profile database and interactive analysis platform for congenital heart disease. Congenit Heart Dis. (2023) 18:693–701. doi: 10.32604/chd.2024.048081
23. Zhang D, Wang M, Peng L, Yang X, Li K, Yin H, et al. Identification and validation of three PDAC subtypes and individualized GSVA immune pathway-related prognostic risk score formula in pancreatic ductal adenocarcinoma patients. J Oncol. (2021) 2021:4986227. doi: 10.1155/2021/4986227
24. Yuan L, Hu C, Yu P, Bao Z, Xia Y, Zhang B, et al. High HDAC5 expression correlates with a poor prognosis and the tumor immune microenvironment in gastric cancer. Ann Trans Med. (2022) 10:990. doi: 10.21037/atm-22-4325
25. Charoentong P, Finotello F, Angelova M, Mayer C, Efremova M, Rieder D, et al. Pan-cancer immunogenomic analyses reveal genotype-immunophenotype relationships and predictors of response to checkpoint blockade. Cell Rep. (2017) 18:248–62. doi: 10.1016/j.celrep.2016.12.019
26. Yuan G, Jia J, Wang Z, Xia Y, Pan Y, Zhang L, et al. Weighted gene co-expression network analysis of immune infiltration in nonalcoholic fatty liver disease. Endocrine Metab Immune Disord - Drug Targets. (2023) 23:1173–85. doi: 10.2174/1871530323666221208105720
27. Li X, Lei J, Shi Y, Peng Z, Gong M, Shu X. Developing a RiskScore Model based on Angiogenesis-related lncRNAs for Colon Adenocarcinoma Prognostic Prediction. Curr Med Chem. (2024) 31:2449–66. doi: 10.2174/0109298673277243231108071620
28. Deng Y, Lin Y, Zhou B, Jing Q, Zhang W. Identification of necroptosis-related subtypes and characterization of tumor microenvironment infiltration in non-small cell lung cancer. Curr Cancer Drug Targets. (2024) 24:80–93. doi: 10.2174/1568009623666230414140609
29. Zulibiya A, Wen J, Yu H, Chen X, Xu L, Ma X, et al. Single-cell RNA sequencing reveals potential for endothelial-to-mesenchymal transition in tetralogy of fallot. Congenit Heart Dis. (2023) 18:611–25. doi: 10.32604/chd.2023.047689
30. Chai R, Zhao Y, Su Z, Liang W. Integrative analysis reveals a four-gene signature for predicting survival and immunotherapy response in colon cancer patients using bulk and single-cell RNA-seq data. Front Oncol. (2023) 13:1277084. doi: 10.3389/fonc.2023.1277084
31. Hu C, Li T, Xu Y, Zhang X, Li F, Bai J, et al. CellMarker 2.0: an updated database of manually curated cell markers in human/mouse and web tools based on scRNA-seq data. Nucleic Acids Res. (2023) 51:D870–d6. doi: 10.1093/nar/gkac947
32. Hou B, Zhao LI, Ianhao Zhao T, Yang M, Zhu W, Chen X, et al. Chrysophanol inhibits the progression of gastric cancer by activating nod-like receptor protein-3. Biocell. (2023) 47:175–86. doi: 10.32604/biocell.2022.021359
33. Ou L, Liu H, Peng C, Zou Y, Jia J, Li H, et al. Helicobacter pylori infection facilitates cell migration and potentially impact clinical outcomes in gastric cancer. Heliyon. (2024) 10:e37046. doi: 10.1016/j.heliyon.2024.e37046
34. Song J, Fu Q, Liu G, Zhang C, Wang Y, Tao S, et al. TULP3 silencing suppresses cell proliferation, migration and invasion in gastric cancer via the PTEN/Akt/Snail pathway. Cancer Treat Res Commun. (2022) 31:100551. doi: 10.1016/j.ctarc.2022.100551
35. Wang W, Baral S, Liu B, Sun Q, Wang L, Ren J, et al. FANCA facilitates G1/S cell cycle advancement, proliferation, migration and invasion in gastric cancer. Acta Biochim Biophys Sinica. (2024) 56:973–85. doi: 10.3724/abbs.2024045
36. Li S, Chen S, Wang B, Zhang L, Su Y, Zhang X. A robust 6-lncRNA prognostic signature for predicting the prognosis of patients with colorectal cancer metastasis. Front Med. (2020) 7:56. doi: 10.3389/fmed.2020.00056
37. Ruiz BI, Lowman XH, Yang Y, Fan Q, Wang T, Wu H, et al. Alpha-ketoglutarate regulates Tnfrsf12a/Fn14 expression via histone modification and prevents cancer-induced cachexia. Genes. (2023) 14:1818. doi: 10.3390/genes14091818
38. Liu JY, Jiang L, He T, Liu JJ, Fan JY, Xu XH, et al. NETO2 promotes invasion and metastasis of gastric cancer cells via activation of PI3K/Akt/NF-κB/Snail axis and predicts outcome of the patients. Cell Death disease. (2019) 10:162. doi: 10.1038/s41419-019-1388-5
39. Wang Y, Zhang S, Xie X, Chen Z, Wu L, Yu Z, et al. Association of TNFRSF12A methylation with prognosis in hepatocellular carcinoma with history of alcohol consumption. Front Genet. (2019) 10:1299. doi: 10.3389/fgene.2019.01299
40. Till JE, Yoon C, Kim BJ, Roby K, Addai P, Jonokuchi E, et al. Oncogenic KRAS and p53 loss drive gastric tumorigenesis in mice that can be attenuated by E-cadherin expression. Cancer Res. (2017) 77:5349–59. doi: 10.1158/0008-5472.CAN-17-0061
41. Subramanian A, Tamayo P, Mootha VK, Mukherjee S, Ebert BL, Gillette MA, et al. Gene set enrichment analysis: a knowledge-based approach for interpreting genome-wide expression profiles. Proc Natl Acad Sci U S A. (2005) 102:15545–50. doi: 10.1073/pnas.0506580102
42. Xue J, Chen L, Cheng H, Song X, Shi Y, Li L, et al. The identification and validation of hub genes associated with acute myocardial infarction using weighted gene co-expression network analysis. J Cardiovasc Dev Dis. (2022) 9:30. doi: 10.3390/jcdd9010030
43. Liao Z, Xie Z. Construction of a disulfidptosis-related glycolysis gene risk model to predict the prognosis and immune infiltration analysis of gastric adenocarcinoma. Clin Trans Oncol. (2024) 26:2309–22. doi: 10.1007/s12094-024-03457-w
44. Zhong Z, Ye Z, He G, Zhang W, Wang J, Huang S. Low expression of A-kinase anchor protein 5 predicts poor prognosis in non-mucin producing stomach adenocarcinoma based on TCGA data. Ann Trans Med. (2020) 8:115. doi: 10.21037/atm.2019.12.98
45. Li G, Ping M, Zhang W, Wang Y, Zhang Z, Su Z. Establishment of the molecular subtypes and a risk model for stomach adenocarcinoma based on genes related to reactive oxygen species. Heliyon. (2024) 10:e27079. doi: 10.1016/j.heliyon.2024.e27079
46. Chen K, Xu J, Tong YL, Yan JF, Pan Y, Wang WJ, et al. Rab31 promotes metastasis and cisplatin resistance in stomach adenocarcinoma through Twist1-mediated EMT. Cell Death disease. (2023) 14:115. doi: 10.1038/s41419-023-05596-4
47. Xie X, Zhou Z, Song Y, Zhang X, Dang C, Zhang H. Mist1 inhibits epithelial-mesenchymal transition in gastric adenocarcinoma via downregulating the Wnt/β-catenin pathway. J Cancer. (2021) 12:4574–84. doi: 10.7150/jca.59138
48. Wang Q, Chen J, Wang Y, Li X, Ping X, Shen J, et al. The profiles of immunosuppressive microenvironment in the Lauren intestinal-type gastric adenocarcinoma. Cancer Immunol Immunother. (2025) 74:82. doi: 10.1007/s00262-024-03938-5
49. Zhao F, Hong J, Zhou G, Huang T, Lin Z, Zhang Y, et al. Elucidating the role of tumor-associated ALOX5+ mast cells with transformative function in cervical cancer progression via single-cell RNA sequencing. Front Immunol. (2024) 15:1434450. doi: 10.3389/fimmu.2024.1434450
50. Luo N, Fu M, Zhang Y, Li X, Zhu W, Yang F, et al. Prognostic role of M6A-associated immune genes and cluster-related tumor microenvironment analysis: A multi-omics practice in stomach adenocarcinoma. Front Cell Dev Biol. (2022) 10:935135. doi: 10.3389/fcell.2022.935135
51. Xiang Z, Cha G, Wang Y, Gao J, Jia J. Characterizing the crosstalk of NCAPG with tumor microenvironment and tumor stemness in stomach adenocarcinoma. Stem Cells Int. (2022) 2022:1888358. doi: 10.1155/2022/1888358
52. Wang M, Li Z, Peng Y, Fang J, Fang T, Wu J, et al. Identification of immune cells and mRNA associated with prognosis of gastric cancer. BMC cancer. (2020) 20:206. doi: 10.1186/s12885-020-6702-1
53. Wang Y, Li X, Zhang T, Li F, Shen Y, He Y, et al. Neutrophils promote tumor invasion via FAM3C-mediated epithelial-to-mesenchymal transition in gastric cancer. Int J Biol Sci. (2023) 19:1352–68. doi: 10.7150/ijbs.79022
54. Meijing Z, Tianhang L, Biao Y. N6-methyladenosine modification patterns and tumor microenvironment immune characteristics associated with clinical prognosis analysis in stomach adenocarcinoma. Front Cell Dev Biol. (2022) 10:913307. doi: 10.3389/fcell.2022.913307
55. Yan P, Cheng M, Wang L, Zhao W. A ferroptosis-related gene in Helicobacter pylori infection, SOCS1, serves as a potential prognostic biomarker and corresponds with tumor immune infiltration in stomach adenocarcinoma: In silico approach. Int immunopharmacol. (2023) 119:110263. doi: 10.1016/j.intimp.2023.110263
Keywords: TNFRSF12A, single-cell RNA sequencing, stomach adenocarcinoma, tumor microenvironment, immunotherapy
Citation: Sun L-D, Zhang L-L, Wan Z, Yang X-D, Yao J, Yang Z-L, Liu L and Liu J-Y (2025) TNFRSF12A expression in stomach adenocarcinoma and its preliminary role in predicting immunotherapy response. Front. Immunol. 16:1578068. doi: 10.3389/fimmu.2025.1578068
Received: 17 February 2025; Accepted: 11 April 2025;
Published: 05 May 2025.
Edited by:
Minghua Ren, First Affiliated Hospital of Harbin Medical University, ChinaReviewed by:
Jing Wang, Second Hospital of Jiaxing City, ChinaZeyan Yu, Guangxi Medical University Cancer Hospital, China
Copyright © 2025 Sun, Zhang, Wan, Yang, Yao, Yang, Liu and Liu. This is an open-access article distributed under the terms of the Creative Commons Attribution License (CC BY). The use, distribution or reproduction in other forums is permitted, provided the original author(s) and the copyright owner(s) are credited and that the original publication in this journal is cited, in accordance with accepted academic practice. No use, distribution or reproduction is permitted which does not comply with these terms.
*Correspondence: Lin Liu, Z2VvZmZyZXkzMDFAMTI2LmNvbQ==; Jun-Yan Liu, ODY0MDI0NzEzQHFxLmNvbQ==
†These authors have contributed equally to this work