- 1State Key Laboratory of Urban and Regional Ecology, Research Centre for Eco-environmental Sciences, Chinese Academy of Sciences, Beijing, China
- 2Key Laboratory for Environmental Biotechnology, Research Center for Eco-Environmental Sciences, Chinese Academy of Sciences, Beijing, China
- 3College of Resources and Environment, University of Chinese Academy of Sciences, Beijing, China
- 4Faculty of Veterinary and Agricultural Sciences, The University of Melbourne, Melbourne, VIC, Australia
Paddy rice fields occupy broad agricultural area in China and cover diverse soil types. Microbial community in paddy soils is of great interest since many microorganisms are involved in soil functional processes. In the present study, Illumina Mi-Seq sequencing and functional gene array (GeoChip 4.2) techniques were combined to investigate soil microbial communities and functional gene patterns across the three soil types including an Inceptisol (Binhai), an Oxisol (Leizhou), and an Ultisol (Taoyuan) along four profile depths (up to 70 cm in depth) in mesocosm incubation columns. Detrended correspondence analysis revealed that distinctly differentiation in microbial community existed among soil types and profile depths, while the manifest variance in functional structure was only observed among soil types and two rice growth stages, but not across profile depths. Along the profile depth within each soil type, Acidobacteria, Chloroflexi, and Firmicutes increased whereas Cyanobacteria, β-proteobacteria, and Verrucomicrobia declined, suggesting their specific ecophysiological properties. Compared to bacterial community, the archaeal community showed a more contrasting pattern with the predominant groups within phyla Euryarchaeota, Thaumarchaeota, and Crenarchaeota largely varying among soil types and depths. Phylogenetic molecular ecological network (pMEN) analysis further indicated that the pattern of bacterial and archaeal communities interactions changed with soil depth and the highest modularity of microbial community occurred in top soils, implying a relatively higher system resistance to environmental change compared to communities in deeper soil layers. Meanwhile, microbial communities had higher connectivity in deeper soils in comparison with upper soils, suggesting less microbial interaction in surface soils. Structure equation models were developed and the models indicated that pH was the most representative characteristics of soil type and identified as the key driver in shaping both bacterial and archaeal community structure, but did not directly affect microbial functional structure. The distinctive pattern of microbial taxonomic and functional composition along soil profiles implied functional redundancy within these paddy soils.
Introduction
Soils cover most of the natural and artificial habitats of terrestrial ecosystems. Due to the high spatial heterogeneity in soil particles and large variation of soil physiochemical properties among soil types, soils are considered harboring the most diverse microbial groups in comparison with other ecosystems (Schimel and Schaeffer, 2012). For a long time, soil scientists have noticed that the soil natural properties determined by soil parent materials during soil formation period, such as pH, texture, and base saturation, etc., sustain soil biodiversity in nature and greatly affect the basic fertility and productivity of soil to a large degree (Anderson, 1988). Furthermore, anthropogenic activities such as tillage, fertilization, irrigation, and cultivation, etc., in soils exert considerable influence on the structure and functional performance of microbial communities via changing the soil properties, thus subsequently influence soil quality in the long term (Tripathi et al., 2015). To understand the diversity of microbial community and their function structure in soils, and their correlations with soil natural properties and human activities are therefore essential to evaluate the crops productivity and environmental sustainability of soil ecosystems, since microbes are responsible for the fertility and productivity of different soil types to a large degree (Anderson, 1988; Martiny et al., 2006).
Numerous recent studies based on culture-independent techniques have suggested that the diversity and community composition of soil microorganisms on large scales were greatly driven by soil pH, and some other soil properties such as organic matter and salinity (Lauber et al., 2009; Griffiths et al., 2011; Tripathi et al., 2015). These studies mainly concentrated on the microbial distribution pattern across large geographical distances, especially focusing on the surface soil, but rarely paid attention to subtle difference of microbial community among soil types. As soil type represents a consequence of the complex influences from soil parent materials and historical and present climatic conditions, it is difficult to attribute the influence of soil type on microbial community to a single factor (Cao et al., 2012; Zhao et al., 2016). For example, some studies suggested that certain microbial taxa would prefer to specific soil types, and soil bacterial community composition was distinct among soil types but could be hardly explained by a single soil chemical parameter (Nie et al., 2012; Tripathi et al., 2012). Moreover, it was observed that soils with different parent materials overwhelmingly supported distinct bacterial community structure after similar long term cultivation, as land use or management practices may mostly shift microbial community structures in top soils (Girvan et al., 2003; Sheng et al., 2015; Sun et al., 2015). Hence it is of interest to understand how much human disturbance could affect soil microbial community comparing to the effects from soil parent materials. Furthermore, the current knowledge on the differentiation of soil microorganism among various soil types and their potential significance are still very limited and deserved to be well depicted, considering the large body of pedodiversity and microbial diversity.
In addition to soil properties determined by soil parent materials, nutrient and oxygen fluctuating along soil profile depth can subsequently lead to a change in microbial communities with soil depth (Will et al., 2010; Wang et al., 2014; Stone et al., 2015). Compared to topsoil, sub-soil volume is much greater, and thus the microbial community and its function in sub-soil is not negligible. Some investigations focusing on specific microbes such as methane-oxidizing bacteria (Reim et al., 2012), ammonium oxidizing microbes (Wang et al., 2014; Lu et al., 2015), nitrifers and denitrifiers (Qin et al., 2016), and the rates of carbon and nitrogen cycling processes suggested great variation of functional microbes and the process they mediated along soil depth gradients (Durán et al., 2017), while few works have made efforts to compare the overall pattern of microbial functional genes in different soil depth layers. Paddy soils occupied large agricultural areas in China. Although the distribution of contrasting microbial community in different paddy soils and the functional analysis of microbes have been described recently (Sheng et al., 2015; Su et al., 2015), comparative investigations linked both taxonomic and functional structure are limited. Also, some recent studies attempted to exploring the influence of different land uses on both taxonomic and functional community of microbes combining high-throughput sequencing technique with functional gene array (Paula et al., 2014; Mendes et al., 2015), but these studies were mainly carried out in the same region within similar soil types.
Comparing to different management practices and land use types, soils with water-logged paddy rice cultivation receive relatively higher uniformity and similarity of management practice. It is still less understood whether uniform management would assimilate microbial community across different soil types, and the extent of inherent influences of parent materials on shaping microbial communities. Along a paddy soil profile, water would replace the gaseous phase and thus the oxygen status varies dramatically along the soil profile (Kögel-Knabner et al., 2010), but succession of microbial community structure along this oxygen gradient has rarely been studied (Noll et al., 2005). In our previous investigation, activity and diversity of the anaerobic ammonium oxidation (anammox) bacteria were examined in three paddy soil types, and the results revealed distinct pattern of anammox activity, diversity and abundance along depth gradient in different paddy soils (Bai et al., 2015). While the study only focused on the aspect of anammox process rather than a comprehensive observation on soil microbes and their functional genes. Therefore, in the present study, Miseq high-throughput sequencing and GeoChip techniques were combined to characterize the bacterial and archaeal community composition and functional structures across four soil profile depths among three distinct paddy soil types including Inceptisol, Oxisol, and Ultisol soil orders. This study aimed to (1) elaborately depict microbial community succession among different parent materials and explore the linkage between microbial community taxonomic and functional structure, and (2) to understand how much do soil inherent properties affect microbial community composition and its functional structure, and (3) if uniform flooding management would assimilate microbial community among different soil types.
Materials and Methods
Mesocosm Incubation and Soil Sampling
The paddy soils used for this study were freshly sampled from a greenhouse mesocosm incubation system as described in our previous study (Bai et al., 2015). Briefly, three paddy soils originally collected from Binhai (BH, 119.84° E, 34.01° N, Inceptisol), Leizhou (LZ, 110.04° E, 20.54° N, lateritic Oxisol), and Taoyuan (TY, 111.48° E, 28.90° N, Ultisol) in three rice production areas in Southeast China with the spatial distance more than 1000 km from each other, were incubated in mesocosms columns (50 cm in diameter and 70 cm in height) and received similar water, fertilization, and rice plantation management as in the field. For each soil type, two replicate columns were constructed, and one profile were sampled from each column at four soil depth intervals (A, 0–5 cm; B, 5–20 cm; C, 20–40 cm; D, 40–60 cm) at the tillering and heading growth stages of rice.
Soil Physicochemical Determinations
Soil pH and EC were measured with a pH meter and a conductivity meter, respectively. NO3- and NH4+ were extracted with 1 M KCl and determined by using a flow analyzer (AA3, SEAL analytical, Germany). Soil hot water-extractable carbon (HWC) was extracted with water at 70°C and determined by a carbon-nitrogen analyzer. Soil total C, N, and S were measured using an elemental analyzer (Vario EL III-Elementar, Germany). The concentrations of dissolved O2 along the soil profiles were also measured with an O2 microsensor electrode (Unisense, Denmark) precisely positioned by a micromanipulator (Unisense).
DNA Extraction and Purification
The total genomic DNA of microbes was extracted from 5 g of dry soil by using a protocol that included liquid nitrogen grinding and sodium dodecyl sulfate as previously described (Zhang et al., 2013). To remove humus and protein components, the DNA was purified with 0.5% low melting point agarose gel, and further purified by a phenol-chloroform-butanol extraction procedure. DNA quantity and quality were evaluated using a NanoDrop ND-1000 Spectrophotometer (NanoDrop Technologies Inc., Wilmington, DE, USA), and all the DNA sample reached final A260/A280 and A260/A230 ratios of >1.7 and 1.8. All DNA samples were stored at -40°C before downstream analysis.
DNA Microarray Hybridization, Scanning and Data Processing
Geochip 4.2 was utilized for analyzing functional structures of soil microbes. The experiments were carried out as previously described (Yang et al., 2013). Briefly, DNA samples were firstly labeled with Cy-5 fluorescent dye using a random priming method. Then the labeled DNA samples were purified with a QIA purification kit (Qiagen, Valencia, CA, USA) and further dried in a SpeedVac (ThermoSavant, Milford, MA, USA) at 45°C for 45 min. Hybridization buffer containing 40% formamide, 25% SSC, 1% SDS, 10 μg of unlabeled herring sperm DNA (Promega, Madison, WI, USA) was added to the dried DNA samples, then vortexed, spun down and incubated at 95°C for 5 min. Hybridizations were performed with a MAUI hybridization station (BioMicro, Salt Lake City, UT, USA). Subsequently, the microarray was scanned by a 100% laser power and 100% photomultiplier tube with a NimbleGen MS 200 Microarray Scanner (Roche, Madison, WI, USA) and signal intensities were quantified.
The obtained raw data was pre-analyzed and denoised with the online pipeline provided by Microarray Data manager1. Each sample was treated separately in this procedure. Briefly, values with signal-noise ratio less than 2 were firstly removed. Subsequently, the values of the signals detected by the probes within each sample were normalized with the lnMR method that ln(x+1) was divided by mean of total signal intensity of each sample, where x denotes a detected value by each probe within each sample.
Illumina Mi-Seq Sequencing and Sequence Analysis
The V4–V5 region of bacterial and archaeal 16S rRNA genes were sequenced with primer sets 515F (5′-GTGCCAGCMGCCGCGGTAA-3′)/806R (5′-GGACTACHVGGGTWTCTAAT-3′) and Arch519F (5′-CAGCCGCCGCGGTAA-3′)/Arch 915R (5′-GTGCTCCCCCGCCAATTCCT-3′), respectively. Based on the requirement of Illumina sequencing, Illumina adaptor A was added to the 5′-ends, and Illumina adaptor B and barcode were added to the 3′-ends of primers. The raw sequencing data were further processed with QIIME software. The FLASH method was firstly carried out to assemble the obtained sequences, and then the UPARSE method was utilized to filter chemira and repetitive sequences. The OTUs of bacterial and archaeal sequences were defined according to a similarity of 97%, and OTU tables were established for further analysis. The identification of bacterial and archaeal taxa was conducted based on the Greengenes database.
Statistical Analysis
Pearson correlation analysis and analysis of differences was carried out with Duncan analysis of one-way ANOVA by using SPSS software (IBM, version 19.0). The vegan package of R software (version 3.2.2) was utilized for conducting detrended correspondence analysis (DCA) which was used to study community and functional structures of soil microbes. Structural equation models (SEM) were developed according to an a priori model to determine the direct and indirect contribution of soil type, soil depth, pH, salinity, and HWC on microbial community and functional structures (as assessed by the first principal coordinate of the Bray–Curtis dissimilarity matrix). Soil type and depth were set as exogenous variables that latitude and longitude (first principal coordinate of the Bray–Curtis dissimilarity matrix) of the three soil sampling sites were substituted for soil type and soil depth was set as 5, 20, 40, and 60. SEM analysis was conducted by utilizing AMOS 22.0 (Amos, Development Corporation, Meadville, PA, USA). Adequate model fits were examined according to non-significant Chi-square test (P > 0.05), goodness fit index (GFI), Akaike value (AIC), and root mean square error of approximation (RMSEA).
Construction of Phylogenetic Molecular Ecological Networks
Phylogenetic molecular ecological networks (pMENs) were constructed based on random matrix theory with the bacterial and archaeal OTUs obtained in profile A and D from the three paddy soils in this study (Deng et al., 2012). Network construction was carried out by utilizing the online pipeline provided by the Institute of Environmental Genomics, University of Oklahoma2.
The average connectivity index (avgK) and modularity index were utilized to describe the topology structure of the networks. The avgK was used to describe the complexity of the networks, and modularity was utilized as a measurement of system resistance. The topological roles of different nodes were divided into four sub-categories based on the within-module connectivity (Zi) and the among module connectivity (Pi): (1) nodes with Zi > 2.5 and Pi < 0.62 were defined as module hubs; (2) nodes with Zi > 2.5 and Pi > 0.62 were defined as network hubs; (3) nodes with Zi < 2.5 and Pi < 0.62 were defined as peripherals; (4) nodes with Zi < 2.5 and Pi > 0.62 were defined as connectors (Olesen et al., 2006).
Networks Analysis of Soil Microbial Community and Functional Genes
The associations of microbial taxa and functional genes were analyzed using the Cytoscape plug-in CoNet (Soffer et al., 2015). The detected taxa and genes with a minimum occurrence of four across all the samples within certain depth layer were discarded in prior to calculation in order to minimize the artificial association bias (Li et al., 2015). The pairwise calculation was performed at the phylum level for archaea and bacteria expect that Proteobacteria were analyzed on the level of class, while functional genes were employed according to the gene category provided by functional gene array (Bai et al., 2013). Combination of correlation scores and P-values of Spearman correlation, Pearson correlation, Kullback–Leibler dissimilarity, and Bray–Curtis dissimilarity were utilized for all the pairwise correlation. Potential false-positive correlations and compositionality biases were eliminated by ReBoot procedure with 100 permutations, and the resultant distribution was further refined with 100 bootstraps. Then Brown method was utilized to combine the P-values for the four correlations measurement, and correlations found to be significant by less than two methods were discarded (Soffer et al., 2015). Only correlations with a coefficient above 0.8 and a significance level below 0.05 were considered statistically robust which were finally displayed as previously reported (Hu et al., 2016). The obtained pairwise correlations were used to construct the co-association networks. Network topology was explored using Cytoscape software and the Network Analyzer plug-in and was illustrated on the open-source interactive platform Gephi (Bastian and Heymann, 2009).
Results
Soil Physical and Chemical Properties
Chemical properties of the three soil columns are shown in Table 1. Significant differences with respect to properties were observed between soil types and along profiles within each soil type. Soil water content decreased dramatically along soil profiles. The pH ranged from 8.0 to 8.6 in BH columns, ranged from 6.8 to 7.2 in LZ columns, and ranged from 5.8 to 6.0 in TY soils. Soil salinity which was reflected by soil electrical conductivity (EC) was approximately 10-fold lower in TY than in BH and LZ soils. HWC peaked in the first two soils layers of the three soil types, and was 2- to 8-folds higher in BH and TY soils than in the LZ soil. DCA for measured soil properties in the three paddy soils showed a clear division in soil characteristics among the three paddy soils. In addition, a succession of soil chemical parameters along soil depth was observed along soil depth within each soil type (Supplementary Figure 1).
Diversity Based on the 16S rDNA and Microarray Analysis
A total of 48 samples covering 3 soil types, 4 depth layers, 2 mesocosm replicates, 2 growth stages were subjected to MiSeq sequencing and microarray assay to explore the diversity of species and functional genes. A total of 26075 bacterial OTUs and 3404 archaeal OTUs were generated after resampling with 42364 bacterial reads and 9456 archaeal reads per sample, respectively. No significant difference was found in alpha diversity of bacterial community except sample LZ-A presented the lowest Shannon indices. While Shannon indices of archaeal community was the lowest in layer A of BH and TY soils, and the highest and lowest Simpson indices were detected in TY-C and LZ-D, respectively (Table 2).
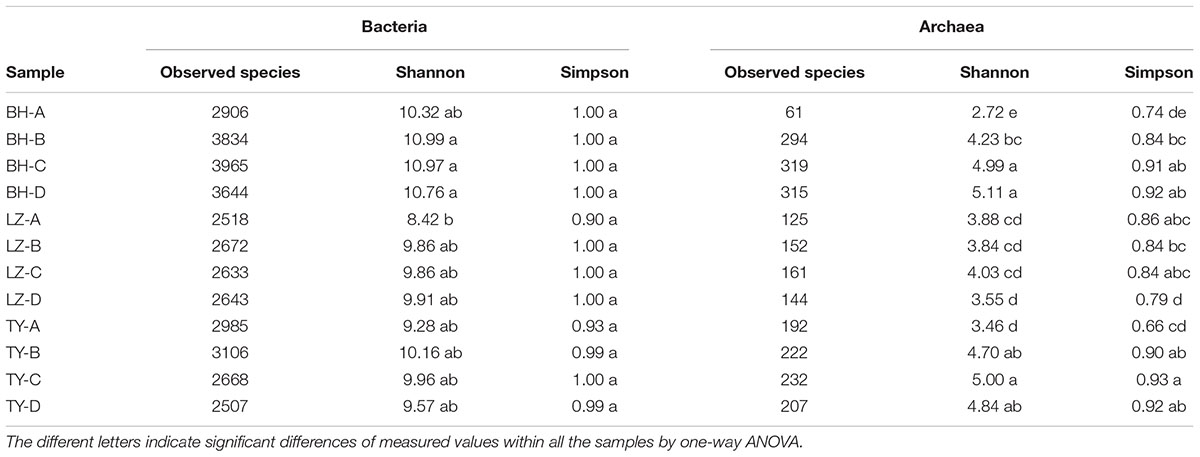
TABLE 2. Number of observed species, indices of Shannon and Simpson diversity of bacterial and archaeal community in four soil profiles of three paddy soils.
The number of detected genes, Shannon index, inverse Simpson index and Simpson evenness by Geochip were calculated to evaluate the functional diversity and structure of microbial communities. In total, 44350 genes were detected and ranged from 36860 to 40612 in different samples. Shannon and Simpson diversity in LZ samples were significantly lower than in BH and TY samples, and showed no significant difference between BH and TY samples. Approximately 80% of functional genes were shared by three soil types while around 5% of the genes were unique within each soil type (Table 3).
Community Similarity Based on the 16S rDNA and Microarray Analyses
Detrended correspondence analysis analysis of 16S rDNA Mi-seq sequences data indicated that bacterial and archaeal communities were clearly separated into three groups according to soil types, indicating the effects of soil type on microbial community composition (Figures 1A,B). A clear trend of community succession along soil profiles was also observed in the three soil types, with surface soils (0–5 cm) separated from sub-soils (5–20 cm, 20–40 cm, and 40–60 cm) markedly, and community structure in surface soils was much more heterogeneous compared to deeper soil layers (Supplementary Figure 2). Given the relative low variation in bacterial and archaeal community structure at the two paddy rice growth stages, samples within the same depth of each soil at the two time points were considered as replicates in the following analysis for the 16S rDNA data.
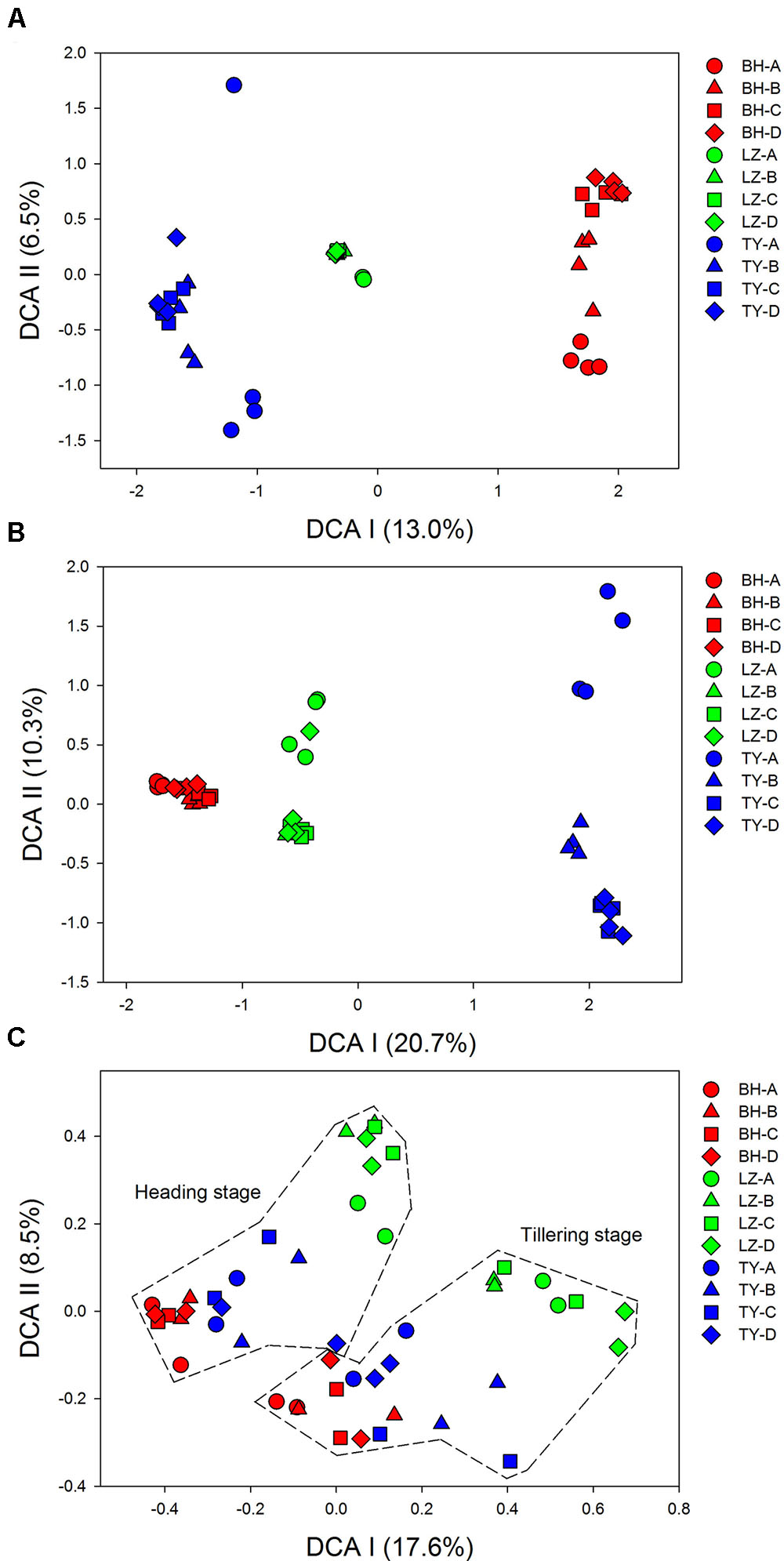
FIGURE 1. Detrended correspondence analysis (DCA) for microbial taxonomic and functional community. Results of (A) bacterial community, (B) archaeal community, and (C) all of the functional genes from four soil profiles (A, B, C, D) of three paddy soils (BH, LZ, TY).
Detrended correspondence analysis based on functional gene array data showed that samples from BH, LZ, and TY soils were well separated from each other by DCA I and DCA II, and the distance between BH and TY samples was closer than to LZ samples (Figure 1C). Unlike community pattern based on 16S rDNA, however, there was no clear differentiation in the structure of functional community between profile layers within each of the soil types. By contrast, samples of tillering and heading stages formed two clusters, which implied the significant difference of soil microbial functional structure between the two growth stages of paddy rice (Figure 1C and Supplementary Figure 2).
Phylogenetic Molecular Network Analysis of Microbial Communities
To further understand the similarity of microbial communities, networks of bacteria and archaea from the three soil profiles were constructed with OTU data obtained from Mi-seq sequencing (Table 4). In respect of the bacterial networks, the network size indicated by the numbers of nodes was highest (206) in bacteria community from layer B (Table 4). The coefficient of positive correlation among bacterial community in layer A was up to 93%, and 18–27% higher than in other layers. However, the network for bacteria in layer A had the lowest average degree (avgK = 3.68), which suggested higher connectivity of the nodes in deeper soil layers. Also, bacterial community in layer A presented the highest level of modularity, indicated its higher system resistance to changes in comparison with other layers. Meanwhile, module hubs, representing key nodes species in the networks, were detected in all the layers. Surface soil harbored the most module hubs (nine hubs) among the soil layers and layer B and C harbored six and eight hubs, while only one hub was defined in the bottom soils, suggesting a lower amount of generalization in this soil depth (Figure 2A and Table 4).
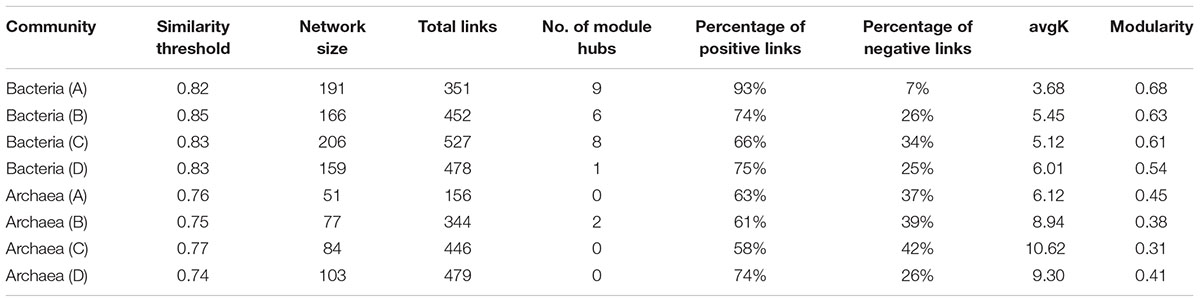
TABLE 4. Major topological properties of phylogenetic molecular ecological networks of bacterial and archaeal communities in four profile layers (A to D) of the three paddy soils.
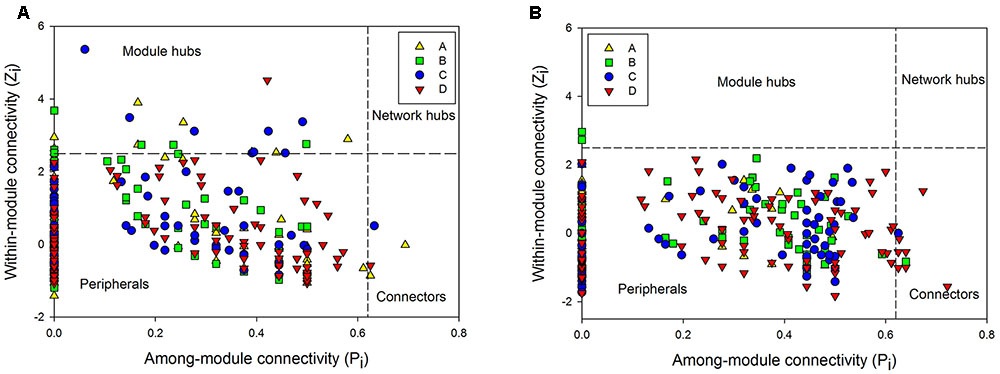
FIGURE 2. The Z-P plot indicating categories of nodes according to Zi (within-module connectivity) and Pi (among-module connectivity) of bacterial (A) and archaeal community (B) in four soil profile layers.
Depth-effects on the connection among microbes were also observed in the networks of the archaeal community. Similar to bacteria, the lowest avgK (6.12) but highest modularity (0.45) in the networks of archaeal community were recorded in layer A (Table 4), indicating that archaea had a lower connectivity in surface soils than in deeper soil layers and possessed a higher system resistance in comparison with other profile depths. In contrast to bacterial networks, module hub was hardly detected in all the archaeal networks (Table 4), suggesting the lack of generality in the archaeal community in these soils. However, more connector nodes between modules were identified in the archaeal community, especially in the bottom layer (Figure 2B). Although there was a slight decrease in the coefficient of positive correlation among archaeal nodes from profiles A to C, the highest coefficient was detected in the bottom layer of the archaeal community (Table 4).
Bacterial and Archaeal Community Composition Based on Mi-Seq Sequencing
Totally, 34 bacteria phyla were identified in all 48 samples of three soil types. The dominant phyla, including Acidobacteria, Actinobacteria, Bacteroidetes, Chloroflexi, Cyanobacteria, Firmicutes, Planctomycetes, Proteobacteria, and Verrucomicrobia, accounted for more than 95% of bacterial sequences (Figure 3A). The relative abundance of individual bacterial taxa varied distinctly among different paddy soil types and between surface and subsurface layers. Of the eight abundant bacteria phyla, the relative abundance of Acidobacteria, Chloroflexi, and Firmicutes were the lowest in the three surface soils (11.2–14%, 3.5–7.4%, and 3.6–5.8%, respectively) but were relatively higher in all the deep soil layers (11.2–29%, 7.7–17.8%, and 5.5–12.6%, respectively), and positively correlated with profile depth (P < 0.01, n = 48, Supplementary Table 1), also negatively correlated to soil HWC (P < 0.01, n = 48, Supplementary Table 1). Conversely, the abundance of Verrucomicrobia and β-proteobacteria decreased along soil depth in all the soil profiles and both positively correlated to the fluctuation of soil HWC along depth (P < 0.01 and P < 0.05, n = 48, Supplementary Tables 1, 2). Cyanobacteria mainly presented in surface soil layer with a proportion of 2.2–5.8% rather than 0.2–0.9% in deeper soils (Figure 3A).
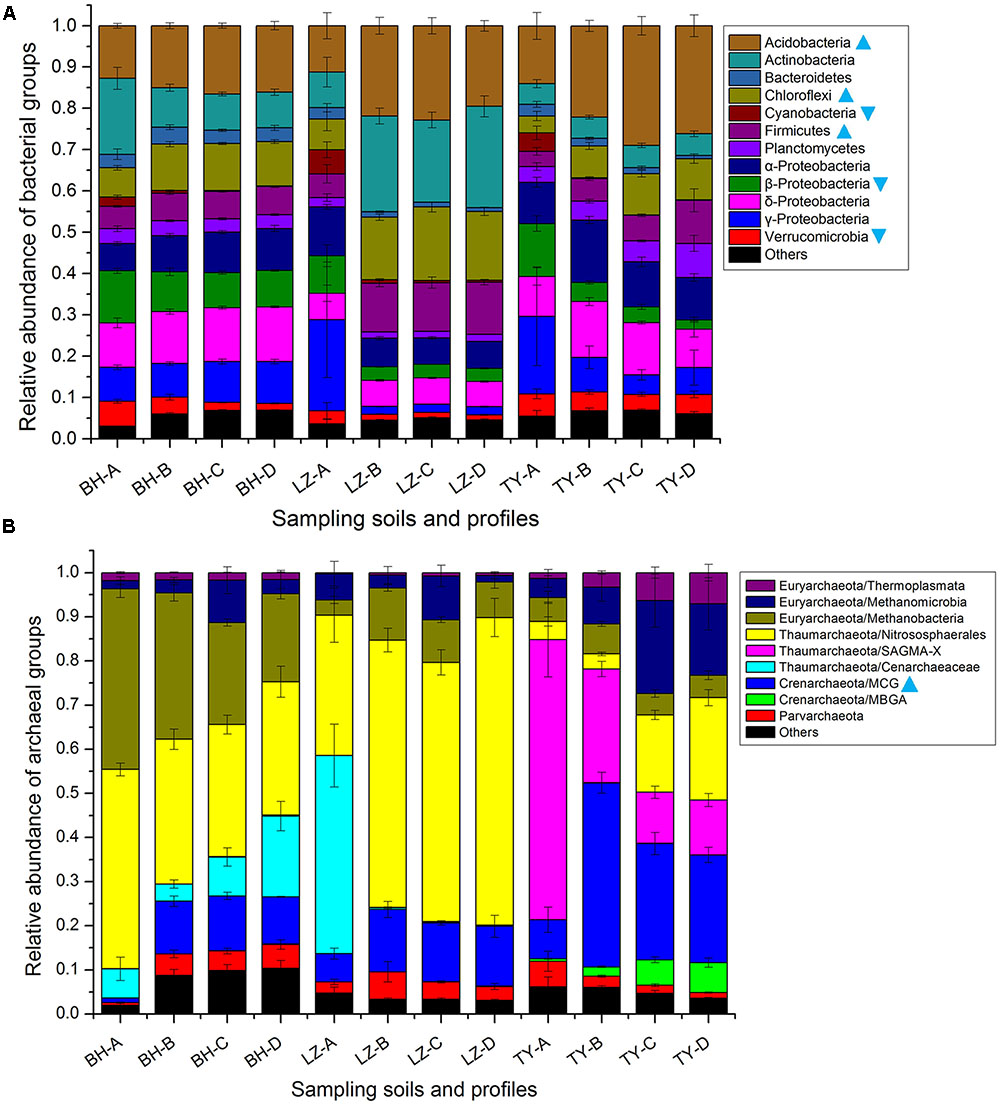
FIGURE 3. Dominant taxonomic groups of bacterial (A) and archaeal (B) groups in four soil profiles of three paddy soils. Cyan triangles denote that the relative abundance of the group uniformly decreased along depth layers within each soil type, cyan inverted triangles denote that the relative abundance of the group uniformly increased along depth layers.
As for archaea, total 3404 OTUs were retrieved and classified into four phyla, including Thaumarchaeota, Euryarchaeota, Crenarchaeota, and Parvarchaeota (Figure 3B). The Thaumarchaeota, which composed of Nitrososphaerales, Cenarchaeaceae, and SAGMA-X, was the most abundant phylum and predominated in the surface layers of three soil types with a relative abundance of 51.8–76.7%, but accounted for a relatively lower proportion between 29.1 and 70% in subsurface layers of three soil types (Figure 3B). Nitrososphaerales accounted for 30–45.1%, and 31.8–69.7% of Thaumarchaeota-affiliating sequences in BH and LZ profile soils, respectively, while it only accounted for 3.3–23.2% in the TY profile soil (Figure 3B). Euryarchaeota was the second abundant archaeal phylum and mainly composed of classes Methanobacteria, Methanomicrobia, and Thermoplasmata. Methanobacteria was approximately 2–3 times higher in BH soils than in LZ and TY soils, Methanomicrobia and Thermoplasmata were more frequently detected in the sub layers of the TY soil (Figure 3B). Converse to Thaumarchaeota phylum, the phylum Crenarchaeota accounted for a much lower proportion in surface layer than deep layers in three soil types, and was more abundant in TY deep layers than in corresponding layers of BH and LZ columns (Figure 3B). The phylum Crenarchaeota was composed of MCG group and MBGA group. MBGA group was only detected in TY profiles with an increasing trend from 3 to 56% along the depths (Figure 3B), while MCG group occupied much lower abundance (1–8.9%) in surface soils than in sub-soils (10.7–41.7%) in all three soil types. In contrast, the group SAGMA-X of Thaumarchaeota was predominant in the TY surface soil (63.4%) but sharply decreased in deep layers (11.5–25.8%), while only occupied 0.01–0.06% proportion of BH and LZ archaeal communities (Figure 3B). All these further suggested distinct bacterial and archaeal community composition among the three soil types and the depth-dependent distribution pattern of some microbial groups.
Pattern of Functional Gene Categories
Microbial functional genes were categorized based on the major metabolic processes to understand the pattern of functional microbial communities in different soil types and depth layers. Genes involved in carbon cycling were most abundant in all the samples, followed by genes related to metal resistance, organic remediation, and nitrogen cycling which also presented at a high level (Figure 4). DCA analysis did not identify significant differences in the gene abundance of different functional categories among depth layers within each soil type (data not shown). Normalized gene signal intensity of all the functional gene categories from different soil layers within each column were therefore combined. Results from one-way ANOVA indicated that the normalized signal intensity of most of the gene categories in BH and TY columns were significantly higher than in LZ columns (Figure 4).
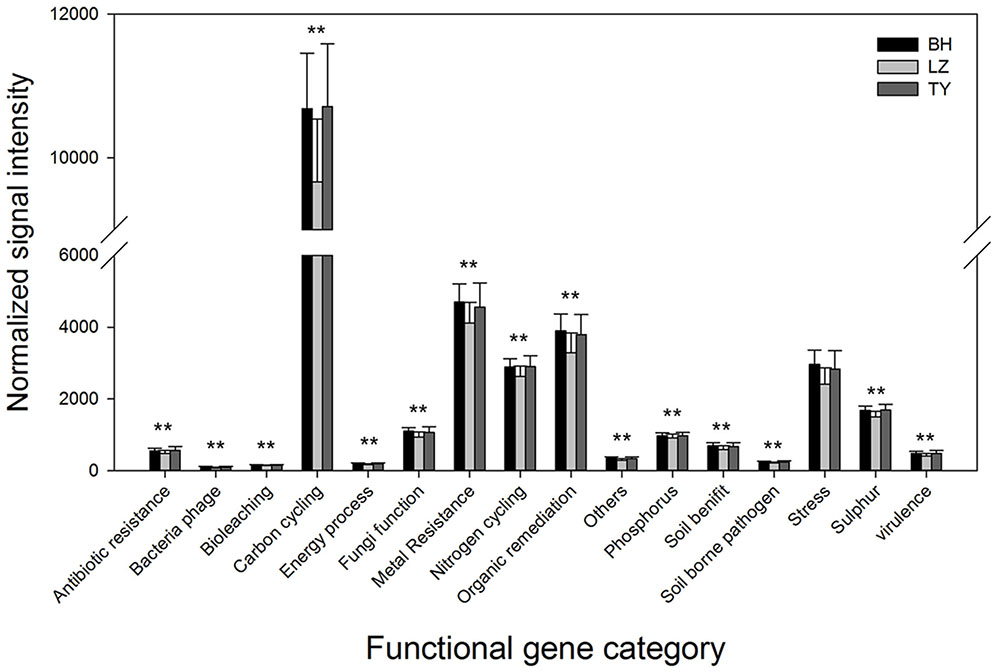
FIGURE 4. Abundance of different of categories of microbial functional genes in three paddy soils. ∗∗ Denotes that the abundance is significantly higher in BH and TY than in LZ soils.
Linkage among Microbial Community, Functional Community, and Soil Properties
Network analysis of microbial taxa and functional genes were generated to estimate the potential linkages between the microbial taxonomic community and overall functional structure obtained from GeoChip analysis (Figure 5). The average degree of the networks indicated the amount of total connections between microbes and their functions. For bacterial community, the highest average degree was detected in layer A (2.79), while archaeal community had lowest average degree in layer A (2.09) but relatively higher degree in both layers B and D (Table 5), indicating that bacterial community had complex connection with their functions in surface soil while archaea performed complex connections in deeper soils.
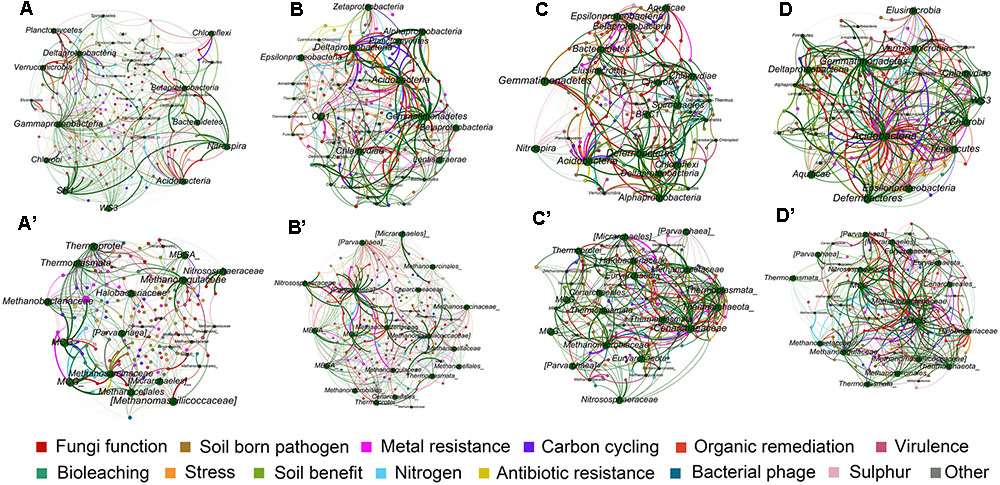
FIGURE 5. Mutualistic networks of interaction between bacterial community and functions of the four soil profile layers (A–D), and archaeal community and functions (A′–D′). Edges in green colors represent influence of microbes to ecological functions, and edges in other colors represent influence of each functional category to microbes.
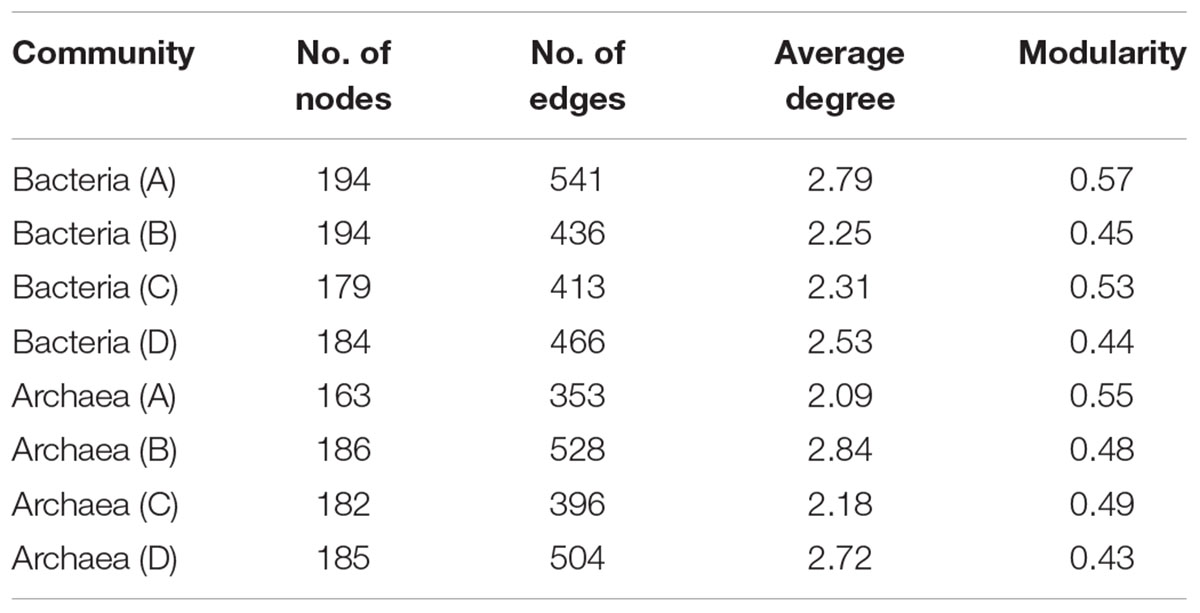
TABLE 5. Major topological properties of mutualistic networks between archaeal communities and functions, and between bacterial communities and functions from profiles A to D of the three paddy soils.
The number of edges that linked microbial taxonomic nodes with functional nodes reflects the linkage between microbial groups and functions, and the thickness of the edges showed the intensity of connection between nodes. Although layers B, C, and D of bacterial networks, and layers A and C of archaea presented overall lower average degrees (Figure 5 and Table 5), stronger connections indicated by number of edges between nodes were observed in these networks (Supplementary Table 3). Generally, the numbers of edges of the main dominant bacterial phyla detected by sequencing were similar among the profile layers (Figure 5 and Table 5). Specifically, Acidobacteria had relatively strong connections to ecological functions across all soil profile layers, while intensive connections between functions and some microbial groups such as δ-Proteobacteria, γ-Proteobacteria, Planctomycetes, and Nitrospira peaked in surface soils or upper layers (Supplementary Table 3). Most of the archaea groups presented weak connection with functions in the surface soils, while Methanomicrobia and Parvarchaeota in layers B and D, and Methanobacteria and MCG in layer D had strong connections with functions, and occupied nearly half of the archaeal connections in the two layers (Supplementary Table 4).
We further developed structure equation models (SEM) to explore how microbial communities and function associated. Parameters in the models included geographical distance of the soil originally located, soil depth, HWC, pH, and EC. The model for bacteria explained 98 and 38% of the variation in bacterial community and functional structure, respectively, along four depth layers of three paddy soils (Figure 6A). Soil pH, HWC, and geographical location were identified as the significant factors that shape the bacterial community structure, while microbial functional structure was solely affected by bacterial community (Figure 6A). Standardized total effects (including direct and indirect effects) obtained from standardized SEM indicated that soil pH contributed more to bacterial community structure than geographical distance and HWC (Supplementary Figure 3A). The model for archaea explained 91 and 25% of the variation in archaeal community and microbial functions, respectively. Archaeal community was mainly influenced by spatial distance, soil pH and EC (Figure 6B). Similar to bacteria, standardized SEM revealed that pH had stronger impact on archaeal community structure than geographical distance and EC (Supplementary Figure 3B).
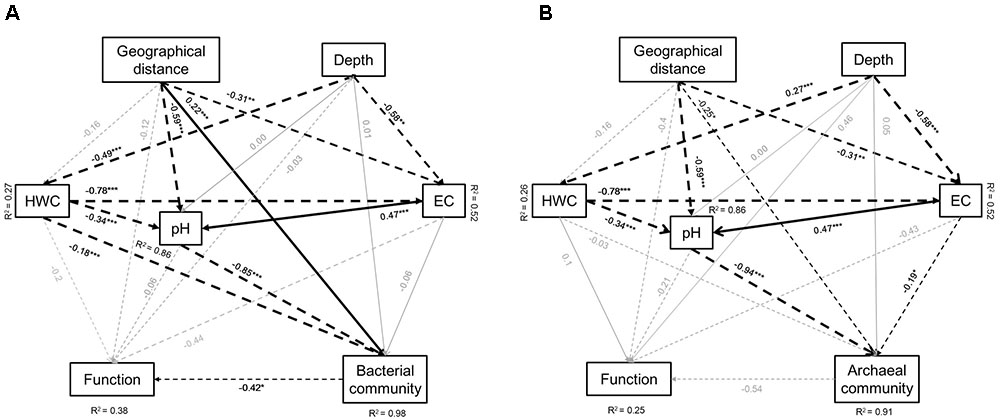
FIGURE 6. Effects of soil type (geographic distance), depth, soil HWC, pH and salinity (EC) on bacterial community and functional (A) and archaeal community and functional (B) structure. Geographical distance includes longitude and latitude of the soil sampling sites. Solid lines denote positive effects, and broken lines denote negative effects. Thickness of the arrows denotes significance and strength of the influence. R2 represent percentage of explanation of the models on the chosen factors. Significant level: ∗P < 0.05, ∗∗P < 0.01, ∗∗∗P < 0.001. Goodness-of-fit statistics are as following: (A) Chi-square = 0.000, degrees of freedom = 1, RMSEA = 0.000, AIC = 54, GFI = 1.000; (B) Chi-square = 0.000, degrees of freedom = 1, RMSEA = 0.000, AIC = 54, GFI = 1.000.
Discussion
Distinct Differentiation of Microbial Community Structure Within Soil Types and Depth Layers Revealed by 16S rDNA and Network Analysis
One of the main purposes of this study was to reveal the effects of soil parent material and profile depth on both microbial community and functional structures. In particular, Illumina Mi-Seq sequencing combining GeoChip techniques and further network analysis facilitated the work and revealed manifest differentiation of bacterial and archaeal community structure among three paddy soil types and between surface and deeper soils within each soil type. Some previous investigations have concerned the microbial community characteristics across different sampling sites and depths and found that layer depths had overwhelming influence on microbial community structure than sampling location (Fierer et al., 2003; Hartmann et al., 2009; Eilers et al., 2012; Steven et al., 2013). However, these studies were mainly carried out in sites with similar soil properties. The three soil types tested in the present study represented distinct pedogenic history and therein possessed varied soil chemical properties. Further SEM analysis identified soil pH, HWC and salinity as the critical influencing factors significantly influencing microbial community structure (Figure 6). Specifically, soil types had a greater direct effect on pH (P < 0.001) comparing to soil salinity (P < 0.01) and HWC (P > 0.05) while pH was also significantly adjusted by HWC and salinity, and pH had stronger impact on bacterial and archaeal community than HWC and salinity in SEMs (Figure 6 and Supplementary Figure S3). Generally, pH is the most representative characteristics of various parent materials of the soil chemical parameters (Imaya et al., 2005). Accordingly, it is not surprising to see that soil parent materials significantly affect microbial community structure via the determination of soil pH in this study. It either well explained why the observed variation of bacterial and archaeal community structure in this study were less among profile depths than among soil types, since the fluctuation of pH was smaller along profiles than that among soil types (7.96–8.62 in BH profile; 6.79–7.15 in LZ profile; 5.96–6.09 in TY profile). The results were consistent with numerous previous studies showing that soil pH is a critical factor in determining soil microbial community structure on large scale (Lauber et al., 2009; Griffiths et al., 2011; Kuramae et al., 2012; Thomson et al., 2015; Tripathi et al., 2015).
Furthermore, although the main division was caused preferentially by soil types, bacterial and archaeal community structure significantly changed along the four profile depths in this study. Particularly, a large separation of microbial community between surface (0–5 cm) and deep layers (5–60 cm) within each soil was indicated in DCA analysis (Figure 2 and Supplementary Figure S1), which was most likely caused by more uniform physicochemical characteristics shared by deeper soil layers (Eilers et al., 2012). SEM analysis clearly showed that soil HWC was greatly driven by depth and significantly affected bacterial community in this study (Figure 6A). Similarly, Hartmann et al. (2009) also suggested that the availability of resources is one of the main factors determining soil microbial community composition along depth gradients, and copiotrophic microbes favored surface soils while oligotrophs preferred deeper soils. These results further revealed a nutrient-effects on microbial community along soil depth layers. In addition to pH and HWC, many previous studies also suggested that salinity has considerable impacts on activity, biomass and community structure of soil microbes (Asghar et al., 2012; Yan and Marschner, 2013; Teng et al., 2014; Morrissey and Franklin, 2015). In relation to that, our SEMs indicated archaeal community structure was significantly shaped by soil salinity while salinity was significantly influenced by both soil types and depth gradients (Figure 6B). Such pattern implied parent material and depth determined archaeal status via the changing of salinity in these soils.
Network analysis of 16S rDNA data further suggested depth-effects on the connection among microbes. Firstly, the modularity of bacterial and archaeal communities was the highest in the top soils and decreased with soil depth (Table 4), indicating a higher degree of habitat heterogeneity for microbes in upper soils (Wang et al., 2015). Also, modularity could serve as an indicator of system resistance (Carpenter et al., 2012). Therefore, the highest modularity of bacterial and archaeal network in top layers was suggestive of a relatively higher system resistance to changes compared to networks in deeper soil layers. Similarly, the lowest avgK value of the network in top layers indicated a low intensive interaction within bacteria and archaea (Table 4). Consequently, the higher modularity and lower avgK value in top layers both suggested that the bacterial and archaeal community in the surface soils would be more resistant and less influenced by disturbance than deeper soils according to network theory (Saavedra et al., 2011). Interestingly, despite bacterial and archaeal community had similar patterns of modularity along soil depth, the higher percentages of negative links within the bacterial community in layers B, C, and D were observed, while the higher proportions of negative links within archaeal community were detected in upper soils (Table 4), suggesting the competition on resources within bacterial and archaeal community was fiercer in deeper and surface soils, respectively. Such a phenomenon might imply a division of positive interactions of the two kingdoms within soils and archaea might be more adapted to the extreme environments in deeper soils.
Variation of Main Bacterial and Archaeal Groups in Different Soil Types and Depth Layers Revealed by 16S rDNA Analysis
The microbial taxonomy analysis based on 16S rRNA gene suggested that Acidobacteria, Actinobacteria, Proteobacteria, Chloroflexi, Firmicutes, and Verrucomicrobia occupied approximately 80–90% of the bacterial communities in the three soil types, and some microbial taxa showed specific distribution patterns according to soil types or profiles. For instance, the highest relative abundance of Acidobacteria was recorded in the acidic TY Ultisols (14–29%), while the lowest was in the alkaline BH Inceptisols (12.7–16.6%). Pearson correlation analysis further proved that the relative abundance of Acidobacteria negatively correlated with soil pH (P < 0.01, r = -0.48, n = 48). The results were consistent with the previous studies’ conclusions that Acidobacteria are sensitive to pH change and this phylum thrives in relatively acidic soils (Will et al., 2010; Mendes et al., 2015).
Except to the bacterial groups such as Planctomycetes, α-Proteobacteria, and δ-Proteobacteria evenly distributed in each layer, the other groups showed consistently preference to a certain depth layer in the three soil types. Particularly, Cyanobacteria, Verrucomicrobia, and β-proteobacteria, were more abundant in all surface samples than in any other sub-soils. Cyanobacteria presented in the surface abundantly but hardly detected in sub-soils, which could be explained by their phototrophic metabolic characteristic. A higher relative abundance of β-proteobacteria was also reported in an early study in the oxic zone of paddy soils (Lüdemann et al., 2000), indicated the class prefers a relatively oxygen-rich condition. The highest relative abundance of Verrucomicrobia were observed in surface soils for three soil types in the present study. Similarly, Verrucomicrobia was found to peak at middle depth layers (20–40 cm) in upland soils and it was suggested that Verrucomicrobia might prefer a relative anoxic circumstance rather than oxic and extreme anoxic conditions in previous studies (Hansel et al., 2008; Eilers et al., 2012). For the paddy soils used in this study, the surface samples at two sampling points were flooded or at least water-saturated and therein represented an oxic/anoxic interface. The highest relative abundance of Verrucomicrobia in this layer and decreasing trend along profile depth further suggested its preference to such oxic/anoxic habitat.
By contrast, Firmicutes, Chloroflexi, and Acidobacteria were more abundant in sub-soils than in surfaces in all three soil types. Increasing of Firmicutes along soil profiles was observed in all three soil types, which is consistent with the observations in previous studies (Hansel et al., 2008; Li et al., 2014), and could be explained by their adaptation to low-nutrient environments in deeper soil layers as Firmicutes usually thrive in extreme conditions through spore-forming (Li et al., 2014). The decline tendency of Chloroflexi and Acidobacteria along soil depth coincided with a recent study in a colluvial soil in which the relative abundance of two phyla decreased along the profile up to 80 cm depth but fluctuated in deeper soils of 80–380 cm (Sagova-Mareckova et al., 2016). Moreover, the strong inverse relationship between carbon availability and the abundance of Acidobacteria were frequently observed in various soil systems and Acidobacteria was supposed to be “oligotrophic” (Fierer et al., 2007; Hansel et al., 2008). The clear decrease in abundance of Acidobacteria in carbon-poor deep profiles observed in this study further supported this hypothesis. Besides these groups showing consistent trend in three soil types, some predominant bacterial phyla such as α-, γ-Proteobacteria, and Actinobacteria did not show consistent shifts in relative abundance along the profiles of three soil types, suggesting the specific microbial community characteristics in different soil types (Eilers et al., 2012).
Taxonomy results of archaeal 16S rRNA gene reads showed that Thaumarchaeota was the most predominant phyla (36.7–76.7%) across each layer and three soil types in this study, while Crenarchaeota only accounted for 1.03–43.8% of archaeal reads, which was consistent with the observation that Thaumarchaeota dominated archaeal community in soil systems (Hu et al., 2013; Chronakova et al., 2015; Schneider et al., 2015; Tripathi et al., 2015). Generally, Thaumarchaeota was more abundant in all surface soils, and such a pattern was similar to a previous finding that Thaumarchaeota was the only archaeal group in aerobic top soils, in contrast to the more diverse archaeal community in deeper soil layers (Mikkonen et al., 2014). Likewise, proteins from Thaumarchaeota were mainly found in relatively oxic environments along a gradient of oxygen and redox in oxygen minimum zones (Hawley et al., 2014), suggesting the distribution of Thaumarchaeota was probably oxygen dependent. It was not surprise as the archaeal ammonia oxidizers are mainly affiliated within this phylum. Specifically, Nitrososphaerales within the phylum Thaumarchaeota was widely detected, with high relative abundance especially in all profile layers of BH and LZ soils, suggesting high abundance of ammonia oxidizers. Interestingly, Cenarchaeaceae and SAGMA-X within Thaumarchaeota showed contrasting distribution patterns across soil samples in the present study. Cenarchaeaceae was only found in BH soils and top soils of LZ columns (6.6–44.8%) while SAGMA-X was mainly detected in TY profiles and predominated in surface of TY soils (63.4%) (Figure 3). However, these two groups were rarely recorded in previous reports except that Cenarchaeaceae was detected in the fluid of a borehole in South Africa and the SAGMA cluster was found in a study in rivers (Herfort et al., 2009; Hernández-Torres et al., 2015). The ecological significance and potential function of these two groups deserved to be further investigation in the future.
The phylum Crenarchaeota, accounted for 9.4–43.8% in TY soils, while only accounted for 1.03–14.1% in BH and LZ soils. The higher relative abundance of Crenarchaeota in sub-soil layers was mainly determined by the presence of the MCG group, which was previously found more abundant in subsurface soils with anoxic environments (Mikkonen et al., 2014), presumably due to their anaerobic characteristic. Similarly, some subgroups under this class were recently detected in river sediments and were reported to preferentially occupy deeper layers of the sediments with reducing conditions (Lazar et al., 2015).
The phylum Euryarchaeota was detected in all profile depths with the proportion varying between 9.7 and 44.6% and mainly composed of Methanobacteria and Methanomicrobia in this study. The proportions in the present study were clearly higher than the proportion (∼15%) in upland soils according to previously reported (Hu et al., 2013), as the representative Euryarchaeota-affiliating class Methanobacteria and Methanomicrobia were commonly detected in anaerobic environments such as aquatic ecosystems, wetlands, and paddy soils and responsible for methanogenesis (Mao et al., 2015; Tong et al., 2015). Although the two classes were both detected in all the samples, the ratio of Methanobacteria and Methanomicrobia proportion varied markedly among soils types, ranging from 22.22 in BH soils to 0.23 in TY soils. Similarly, it was observed that the relative abundance of Methanomicrobia increased largely in the anoxic zone in a lake while Methanobacteria was absent in a previous report (Hugoni et al., 2015). Conversely, Methanobacteria was widely identified in another study in a water-flooded oil reservoir without detecting Methanomicrobia (Hernández-Torres et al., 2015). All these imply that the two classes probably compete for niche and substrate and replace each other under specific soil conditions.
Microbial Functional Structure in Different Paddy Soils and Linkage with Community Structure
Analyzing microbial functional genes of key enzymes related to biogeochemistry and metabolic pathways is essential to link microbial communities to their ecological functions. Functional gene array has been proved being a more effective approach to accomplish this task compared to conventional molecular ecology techniques (He et al., 2010, 2012; Kang et al., 2013). The detected genes in this study involved in diverse functions such as carbon degradation, methane oxidation and production, nitrogen and sulfur cycling, phosphorus utilization, heavy metal and antibiotic resistance, organic remediation and other categories, suggesting versatile ecological processes occurrence in these paddy soils. Revealing the pattern of these genes would be helpful to understand and predict relative functional processes performed by these gene categories (Bai et al., 2013; Zhang et al., 2013). However, DCA and SEMs analysis did not figure out similar effects from environmental factors on functional structure as the effect on bacterial community, except that the functional structure separated between the two rice growth stages in DCA plot (Figure 1C), suggesting the factors relative with plant growth potentially affected the structure of soil microbial functions to a greater degree even than soil type. Although significant effect of soil properties on microbial functional structure was not detected by SEMs, microbial functional gene diversity and abundance in the LZ soils (Oxisol) was significantly lower than in the BH soils (Inceptisol) and the TY soils (Ultisol) (Figure 4 and Table 3), and the functional structure in the BH and TY soils were more similar compared to the LZ samples in DCA plot (Figure 1). Such pattern might be attributed to the complex influences from soil type but not to a single factor as suggested before (Cao et al., 2012; Zhao et al., 2016). On the other hand, significant effects of bacterial community structure on functional structure while the weak relationship between archaeal community and functional structure were detected by SEM (Figure 6), possibly due to the low amount of archaeal probes in the functional gene array analysis.
To establish the linkage for microbial community composition and their function, network analysis between microbial taxa and functional genes were generated in the present study, and the analysis has further proved the known abundant microbial groups such as α- and β-proteobacteria and Acidobacteria could play essential roles in regulating ecological functions. However, the networks also suggested that the predominated microbial groups in soils did not necessary provide intense functional potentials, while less abundant microbial groups might take greater parts in soil ecological processes. For instance, the two dominant Phyla, Firmicute and Actinobacteria were detected in all soils but their numbers of connections with functions only ranged between 2 and 11 in the networks. On the contrary, some groups with lower relative abundance such as ε-Proteobacteria and Parvarchaota had more than 32 and 157 connections with functions (Supplementary Tables 3, 4).
Although both bacterial and archaeal community composition shifted greatly along soil profiles, the general functional structure only varied among soil types but not across the profile depths in the present study. The possible reason for the distinctive pattern of microbial taxonomic and functional composition along soil profiles could be that some microbial groups with less abundant take greater parts in soil ecological processes as mentioned above. Furthermore, the result was consistent with previous observation that the change of microbial community composition does not necessarily connect to a change of microbial functions, and could be explained as the functional redundancy of soil microorganisms (Comte and Giorgio, 2010). Given the possibility that most of microbes inhabiting in soils possessed similar functional genes, the fluctuation in taxonomic structure along soil profile gradients would not necessarily alter the microbial function structure. Such ability could serve as a fundamental property of soil microbes which is essential to environmental perturbation (Comte and Giorgio, 2010). The similar weak linkages between microbial taxonomic and functional community structure were previously observed in Antarctic soils (Yergeau et al., 2007, 2012; Chong et al., 2015), subtropical broadleaf forests (Ding et al., 2015), fen peatlands (Haynes et al., 2015), and in microbial stream biofilms (Dopheide et al., 2015), which further corroborated the theory.
Conclusion
By combining Illumina Mi-Seq sequencing with Geochip techniques, the study demonstrated manifest separation of bacterial and archaeal community and function structure among soil types, and visible but relatively slight shift of community structure along soil depth within each soil type, suggesting the overwhelming effect of soil parent material characteristics mainly via determination of soil pH, even under uniform rice cultivation management. Bacterial community showed significantly shifted along soil depth gradients mainly driven by organic carbon, while archaeal community variation along soil depth was mainly determined by salinity. Moreover, bacterial and archaeal taxa showed specific distribution patterns according to soil types or profiles, dependent on their ecophysiological properties. Especially, archaeal community composition showed contrasting patterns among the three paddy soils. Network analysis of bacterial and archaeal community indicated paddy soils harbored a higher degree of habitat heterogeneity for microbes in upper soils, and thus endowed microbes with higher system resistance and less intensive interaction in the surface soils compare to deeper soils. The relatively weak alignment between microbial community and functional structure suggested possible functional redundancy, and implied the potential resistance and resilience of microbial communities to environmental disturbance within these paddy soils.
Author Contributions
RB was responsible to most of the laboratorial works, data processing, and article writing. J-TW conducted the construction of network analysis of microbial community and functional genes, and contributed to the analysis of sequencing data and construction of structure equation models. YD provided essential ideas to the article writing, as well as assistance in constructing phylogenetic molecular ecological networks. J-ZH provided essential ideas to the experimental design and article writing. KF contributed to the construction of phylogenetic molecular ecological networks. L-MZ provided essential ideas to the experimental design, and was responsible for mesocosm setup, article writing and revising.
Conflict of Interest Statement
The authors declare that the research was conducted in the absence of any commercial or financial relationships that could be construed as a potential conflict of interest.
Acknowledgments
This work was financially supported by the National Science Foundation of China (No. 41322007), Chinese Academy of Sciences (XDB15020200), MOST (2014BAD14B00), and Youth Innovation Promotion Association of Chinese Academy of Sciences. We’d like to thank Dr. Ying Gao’s assistance in the analysis of GeoChip data, and Prof. Phillip Chalk for the language polishing for the manuscript. We are also grateful to Dr. Yu Dai, Dr. Chaolei Yuan, and Dr. Guiyou Zhang’s help for soil collection and the analysis of soil chemical properties.
Supplementary Material
The Supplementary Material for this article can be found online at: http://journal.frontiersin.org/article/10.3389/fmicb.2017.00945/full#supplementary-material
Footnotes
References
Anderson, D. (1988). The effect of parent material and soil development on nutrient cycling in temperate ecosystems. Biogeochemistry 5, 71–97. doi: 10.1007/BF02180318
Asghar, H. N., Setia, R., and Marschner, P. (2012). Community composition and activity of microbes from saline soils and non-saline soils respond similarly to changes in salinity. Soil Biol. Biochem. 47, 175–178. doi: 10.1016/j.soilbio.2012.01.002
Bai, R., Xi, D., He, J.-Z., Hu, H.-W., Fang, Y.-T., and Zhang, L.-M. (2015). Activity, abundance and community structure of anammox bacteria along depth profiles in three different paddy soils. Soil Biol. Biochem. 91, 212–221. doi: 10.1016/j.soilbio.2015.08.040
Bai, S., Li, J., He, Z., Van Nostrand, J. D., Tian, Y., Lin, G., et al. (2013). GeoChip-based analysis of the functional gene diversity and metabolic potential of soil microbial communities of mangroves. Appl. Microbiol. Biotechnol. 97, 7035–7048. doi: 10.1007/s00253-012-4496-z
Bastian, M., and Heymann, S. (2009). “Gephi: an open source software for exploring and manipulating networks,” in Proceedings of the International AAAI Conference on Weblogs and Social Media, San Jose, CA.
Cao, P., Zhang, L. M., Shen, J. P., Zheng, Y. M., Di, H. J., and He, J. Z. (2012). Distribution and diversity of archaeal communities in selected Chinese soils. FEMS Microbiol. Ecol. 80, 146–158. doi: 10.1111/j.1574-6941.2011.01280.x
Carpenter, S., Arrow, K., Barrett, S., Biggs, R., Brock, W., Crépin, A.-S., et al. (2012). General resilience to cope with extreme events. Sustainability 4, 3248–3259. doi: 10.3390/su4123248
Chong, C. W., Pearce, D. A., and Convey, P. (2015). Emerging spatial patterns in Antarctic prokaryotes. Front. Microbiol. 6:1058. doi: 10.3389/fmicb.2015.01058
Chronakova, A., Schloter-Hai, B., Radl, V., Endesfelder, D., Quince, C., Elhottova, D., et al. (2015). Response of archaeal and bacterial soil communities to changes associated with outdoor cattle overwintering. PLoS ONE 10:e0135627. doi: 10.1371/journal.pone.0135627
Comte, J., and Giorgio, P. A. D. (2010). Linking the patterns of change in composition and function in bacterioplankton successions along environmental gradients. Ecology 91, 1466–1476. doi: 10.1890/09-0848.1
Deng, Y., Jiang, Y.-H., Yang, Y., He, Z., Luo, F., and Zhou, J. (2012). Molecular ecological network analyses. BMC Bioinformatics 13:113. doi: 10.1186/1471-2105-13-113
Ding, J., Zhang, Y., Wang, M., Sun, X., Cong, J., Deng, Y., et al. (2015). Soil organic matter quantity and quality shape microbial community compositions of subtropical broadleaved forests. Mol. Ecol. 24, 5175–5185. doi: 10.1111/mec.13384
Dopheide, A., Lear, G., He, Z., Zhou, J., and Lewis, G. D. (2015). Functional gene composition, diversity and redundancy in microbial stream biofilm communities. PLoS ONE 10:e0123179. doi: 10.1371/journal.pone.0123179
Durán, J., Morse, J. L., Rodríguez, A., Campbell, J. L., Christenson, L. M., Driscoll, C. T., et al. (2017). Differential sensitivity to climate change of C and N cycling processes across soil horizons in a northern hardwood forest. Soil Biol. Biochem. 107, 77–84. doi: 10.1016/j.soilbio.2016.12.028
Eilers, K. G., Debenport, S., Anderson, S., and Fierer, N. (2012). Digging deeper to find unique microbial communities: the strong effect of depth on the structure of bacterial and archaeal communities in soil. Soil Biol. Biochem. 50, 58–65. doi: 10.1016/j.soilbio.2012.03.011
Fierer, N., Morse, J. L., Berthrong, S. T., Bernhardt, E. S., and Jackson, R. B. (2007). Environmental controls on the landscape-scale biogeography of stream bacterial communities. Ecology 88, 2162–2173. doi: 10.1890/06-1746.1
Fierer, N., Schimel, J. P., and Holden, P. A. (2003). Variations in microbial community composition through two soil depth profiles. Soil Biol. Biochem. 35, 167–176. doi: 10.1016/S0038-0717(02)00251-1
Girvan, M. S., Bullimore, J., Pretty, J. N., Osborn, A. M., and Ball, A. S. (2003). Soil type is the primary determinant of the composition of the total and active bacterial communities in arable soils. Appl. Environ. Microbiol. 69, 1800–1809. doi: 10.1128/aem.69.3.1800-1809.2003
Griffiths, R. I., Thomson, B. C., James, P., Bell, T., Bailey, M., and Whiteley, A. S. (2011). The bacterial biogeography of British soils. Environ. Microbiol. 13, 1642–1654. doi: 10.1111/j.1462-2920.2011.02480.x
Hansel, C. M., Fendorf, S., Jardine, P. M., and Francis, C. A. (2008). Changes in bacterial and archaeal community structure and functional diversity along a geochemically variable soil profile. Appl. Environ. Microbiol. 74, 1620–1633. doi: 10.1128/AEM.01787-07
Hartmann, M., Lee, S., Hallam, S. J., and Mohn, W. W. (2009). Bacterial, archaeal and eukaryal community structures throughout soil horizons of harvested and naturally disturbed forest stands. Environ. Microbiol. 11, 3045–3062. doi: 10.1111/j.1462-2920.2009.02008.x
Hawley, A. K., Brewer, H. M., Norbeck, A. D., Pasa-Tolic, L., and Hallam, S. J. (2014). Metaproteomics reveals differential modes of metabolic coupling among ubiquitous oxygen minimum zone microbes. Proc. Natl. Acad. Sci. U.S.A. 111, 11395–11400. doi: 10.1073/pnas.1322132111
Haynes, K. M., Preston, M. D., McLaughlin, J. W., Webster, K., and Basiliko, N. (2015). Dissimilar bacterial and fungal decomposer communities across rich to poor fen peatlands exhibit functional redundancy. Can. J. Soil Sci. 95, 219–230. doi: 10.4141/cjss-2014-062
He, Z., Deng, Y., Van Nostrand, J. D., Tu, Q., Xu, M., Hemme, C. L., et al. (2010). GeoChip 3.0 as a high-throughput tool for analyzing microbial community composition, structure and functional activity. ISME J. 4, 1167–1179. doi: 10.1038/ismej.2010.46
He, Z., Deng, Y., and Zhou, J. (2012). Development of functional gene microarrays for microbial community analysis. Curr. Opin. Biotechnol. 23, 49–55. doi: 10.1016/j.copbio.2011.11.001
Herfort, L., Kim, J. H., Coolen, M. J. L., Abbas, B., Schouten, S., Herndl, G. J., et al. (2009). Diversity of Archaea and detection of crenarchaeotal amoA genes in the rivers Rhine and Têt. Aquat. Microb. Ecol. 55, 189–201. doi: 10.3354/ame01294
Hernández-Torres, J., Castillo Villamizar, G. A., Salgar-Chaparro, S. J., Silva-Plata, B. A., Serna Daza, O. D., Martínez-Pérez, F., et al. (2015). Prokaryotic community characterization in a mesothermic and water- flooded oil reservoir in Colombia. Geomicrobiol. J. 33, 110–117. doi: 10.1080/01490451.2015.1039674
Hu, H. W., Wang, J. T., Li, J., Li, J. J., Ma, Y. B., Chen, D., et al. (2016). Field-based evidence for copper contamination induced changes of antibiotic resistance in agricultural soils. Environ. Microbiol. 18, 3896–3909. doi: 10.1111/1462-2920.13370
Hu, H.-W., Zhang, L.-M., Yuan, C.-L., and He, J.-Z. (2013). Contrasting Euryarchaeota communities between upland and paddy soils exhibited similar pH-impacted biogeographic patterns. Soil Biol. Biochem. 64, 18–27. doi: 10.1016/j.soilbio.2013.04.003
Hugoni, M., Domaizon, I., Taib, N., Biderre-Petit, C., Agogue, H., Galand, P. E., et al. (2015). Temporal dynamics of active Archaea in oxygen-depleted zones of two deep lakes. Environ. Microbiol. Rep. 7, 321–329. doi: 10.1111/1758-2229.12251
Imaya, A., Ohta, S., Tanaka, N., and Inagaki, Y. (2005). General chemical properties of brown forest soils developed from different parent materials in the submontane zone of the Kanto and Chubu Districts, Japan. Soil Sci. Plant Nutr. 51, 873–884. doi: 10.1111/j.1747-0765.2005.tb00122.x
Kang, S., Van Nostrand, J. D., Gough, H. L., He, Z., Hazen, T. C., Stahl, D. A., et al. (2013). Functional gene array-based analysis of microbial communities in heavy metals-contaminated lake sediments. FEMS Microbiol. Ecol. 86, 200–214. doi: 10.1111/1574-6941.12152
Kögel-Knabner, I., Amelung, W., Cao, Z., Fiedler, S., Frenzel, P., Jahn, R., et al. (2010). Biogeochemistry of paddy soils. Geoderma 157, 1–14. doi: 10.1016/j.geoderma.2010.03.009
Kuramae, E. E., Yergeau, E., Wong, L. C., Pijl, A. S., van Veen, J. A., and Kowalchuk, G. A. (2012). Soil characteristics more strongly influence soil bacterial communities than land-use type. FEMS Microbiol. Ecol. 79, 12–24. doi: 10.1111/j.1574-6941.2011.01192.x
Lauber, C. L., Hamady, M., Knight, R., and Fierer, N. (2009). Pyrosequencing-based assessment of soil pH as a predictor of soil bacterial community structure at the continental scale. Appl. Environ. Microbiol. 75, 5111–5120. doi: 10.1128/AEM.00335-09
Lazar, C. S., Biddle, J. F., Meador, T. B., Blair, N., Hinrichs, K. U., and Teske, A. P. (2015). Environmental controls on intragroup diversity of the uncultured benthic archaea of the miscellaneous Crenarchaeotal group lineage naturally enriched in anoxic sediments of the White Oak River estuary (North Carolina, USA). Environ. Microbiol. 17, 2228–2238. doi: 10.1111/1462-2920.12659
Li, B., Yang, Y., Ma, L., Ju, F., Guo, F., Tiedje, J. M., et al. (2015). Metagenomic and network analysis reveal wide distribution and co-occurrence of environmental antibiotic resistance genes. ISME J. 9, 2490–2502. doi: 10.1038/ismej.2015.59
Li, C., Yan, K., Tang, L., Jia, Z., and Li, Y. (2014). Change in deep soil microbial communities due to long-term fertilization. Soil Biol. Biochem. 75, 264–272. doi: 10.1016/j.soilbio.2014.04.023
Lu, S., Liu, X., Ma, Z., Liu, Q., Wu, Z., Zeng, X., et al. (2015). Vertical segregation and phylogenetic characterization of ammonia-oxidizing bacteria and archaea in the sediment of a freshwater aquaculture pond. Front. Microbiol. 6:1539. doi: 10.3389/fmicb.2015.01539
Lüdemann, H., Arth, I., and Liesack, W. (2000). Spatial changes in the bacterial community structure along a vertical oxygen gradient in flooded paddy soil cores. Appl. Environ. Microbiol. 66, 754–762. doi: 10.1128/AEM.66.2.754-762.2000
Mao, T.-T., Yin, R., and Deng, H. (2015). Effects of copper on methane emission, methanogens and methanotrophs in the rhizosphere and bulk soil of rice paddy. Catena 133, 233–240. doi: 10.1016/j.catena.2015.05.024
Martiny, J. B., Bohannan, B. J., Brown, J. H., Colwell, R. K., Fuhrman, J. A., Green, J. L., et al. (2006). Microbial biogeography: putting microorganisms on the map. Nat. Rev. Microbiol. 4, 102–112. doi: 10.1038/nrmicro1341
Mendes, L. W., de Lima Brossi, M. J., Kuramae, E. E., and Tsai, S. M. (2015). Land-use system shapes soil bacterial communities in Southeastern Amazon region. Appl. Soil Ecol. 95, 151–160. doi: 10.1016/j.apsoil.2015.06.005
Mikkonen, A., Santalahti, M., Lappi, K., Pulkkinen, A. M., Montonen, L., and Suominen, L. (2014). Bacterial and archaeal communities in long-term contaminated surface and subsurface soil evaluated through coextracted RNA and DNA. FEMS Microbiol. Ecol. 90, 103–114. doi: 10.1111/1574-6941.12376
Morrissey, E. M., and Franklin, R. B. (2015). Evolutionary history influences the salinity preference of bacterial taxa in wetland soils. Front. Microbiol. 6:1013. doi: 10.3389/fmicb.2015.01013
Nie, M., Meng, H., Li, K., Wan, J. R., Quan, Z. X., Fang, C. M., et al. (2012). Comparison of bacterial and fungal communities between natural and planted pine forests in subtropical China. Curr. Microbiol. 64, 34–42. doi: 10.1007/s00284-011-0031-1
Noll, M., Matthies, D., Frenzel, P., Derakshani, M., and Liesack, W. (2005). Succession of bacterial community structure and diversity in a paddy soil oxygen gradient. Environ. Microbiol. 7, 382–395. doi: 10.1111/j.1462-2920.2004.00700.x
Olesen, J. M., Bascompte, J., Dupont, Y. L., and Jordano, P. (2006). The smallest of all worlds: pollination networks. J. Theor. Biol. 240, 270–276. doi: 10.1016/j.jtbi.2005.09.014
Paula, F. S., Rodrigues, J. L., Zhou, J., Wu, L., Mueller, R. C., Mirza, B. S., et al. (2014). Land use change alters functional gene diversity, composition and abundance in Amazon forest soil microbial communities. Mol. Ecol. 23, 2988–2999. doi: 10.1111/mec.12786
Qin, H. L., Zhang, Z. X., Lu, J., Zhu, Y. J., Webster, R., Liu, X. L., et al. (2016). Change from paddy rice to vegetable growing changes nitrogen-cycling microbial communities and their variation with depth in the soil. Eur. J. Soil Sci. 67, 650–658. doi: 10.1111/ejss.12365
Reim, A., Luke, C., Krause, S., Pratscher, J., and Frenzel, P. (2012). One millimetre makes the difference: high-resolution analysis of methane-oxidizing bacteria and their specific activity at the oxic-anoxic interface in a flooded paddy soil. ISME J. 6, 2128–2139. doi: 10.1038/ismej.2012.57
Saavedra, S., Stouffer, D. B., Uzzi, B., and Bascompte, J. (2011). Strong contributors to network persistence are the most vulnerable to extinction. Nature 478, 233–235. doi: 10.1038/nature10433
Sagova-Mareckova, M., Zadorova, T., Penizek, V., Omelka, M., Tejnecky, V., Pruchova, P., et al. (2016). The structure of bacterial communities along two vertical profiles of a deep colluvial soil. Soil Biol. Biochem. 101, 65–73. doi: 10.1016/j.soilbio.2016.06.026
Schimel, J. P., and Schaeffer, S. M. (2012). Microbial control over carbon cycling in soil. Front. Microbiol. 3:348. doi: 10.3389/fmicb.2012.00348
Schneider, D., Engelhaupt, M., Allen, K., Kurniawan, S., Krashevska, V., Heinemann, M., et al. (2015). Impact of lowland rainforest transformation on diversity and composition of soil prokaryotic communities in Sumatra (Indonesia). Front. Microbiol. 6:1339. doi: 10.3389/fmicb.2015.01339
Sheng, R., Qin, H., O’Donnell, A. G., Huang, S., Wu, J., and Wei, W. (2015). Bacterial succession in paddy soils derived from different parent materials. J. Soils Sediments 15, 982–992. doi: 10.1007/s11368-014-1058-2
Soffer, N., Zaneveld, J., and Vega Thurber, R. (2015). Phage-bacteria network analysis and its implication for the understanding of coral disease. Environ. Microbiol. 17, 1203–1218. doi: 10.1111/1462-2920.12553
Steven, B., Gallegos-Graves, L. V., Belnap, J., and Kuske, C. R. (2013). Dryland soil microbial communities display spatial biogeographic patterns associated with soil depth and soil parent material. FEMS Microbiol. Ecol. 86, 101–113. doi: 10.1111/1574-6941.12143
Stone, M. M., Kan, J., and Plante, A. F. (2015). Parent material and vegetation influence bacterial community structure and nitrogen functional genes along deep tropical soil profiles at the Luquillo Critical Zone Observatory. Soil Biol. Biochem. 80, 273–282. doi: 10.1016/j.soilbio.2014.10.019
Su, J. Q., Ding, L. J., Xue, K., Yao, H. Y., Quensen, J., Bai, S. J., et al. (2015). Long-term balanced fertilization increases the soil microbial functional diversity in a phosphorus-limited paddy soil. Mol. Ecol. 24, 136–150. doi: 10.1111/mec.13010
Sun, L., Gao, J., Huang, T., Kendall, J. R., Shen, Q., and Zhang, R. (2015). Parental material and cultivation determine soil bacterial community structure and fertility. FEMS Microbiol. Ecol. 91, 1–10. doi: 10.1093/femsec/fiu010
Teng, Y., Su, J., Wang, J., Dai, N., Li, J., Song, L., et al. (2014). Soil microbial community response to seawater intrusion into coastal aquifer of Donghai Island, South China. Environ. Earth Sci. 72, 3329–3338. doi: 10.1007/s12665-014-3236-3
Thomson, B. C., Tisserant, E., Plassart, P., Uroz, S., Griffiths, R. I., Hannula, S. E., et al. (2015). Soil conditions and land use intensification effects on soil microbial communities across a range of European field sites. Soil Biol. Biochem. 88, 403–413. doi: 10.1016/j.soilbio.2015.06.012
Tong, H., Liu, C., Li, F., Luo, C., Chen, M., and Hu, M. (2015). The key microorganisms for anaerobic degradation of pentachlorophenol in paddy soil as revealed by stable isotope probing. J. Hazard. Mater. 298, 252–260. doi: 10.1016/j.jhazmat.2015.05.049
Tripathi, B. M., Kim, M., Singh, D., Lee-Cruz, L., Lai-Hoe, A., Ainuddin, A. N., et al. (2012). Tropical soil bacterial communities in Malaysia: pH dominates in the equatorial tropics too. Microb. Ecol. 64, 474–484. doi: 10.1007/s00248-012-0028-8
Tripathi, B. M., Kim, M., Tateno, R., Kim, W., Wang, J., Lai-Hoe, A., et al. (2015). Soil pH and biome are both key determinants of soil archaeal community structure. Soil Biol. Biochem. 88, 1–8. doi: 10.1016/j.soilbio.2015.05.004
Wang, F., Liang, Y., Jiang, Y., Yang, Y., Xue, K., Xiong, J., et al. (2015). Planting increases the abundance and structure complexity of soil core functional genes relevant to carbon and nitrogen cycling. Sci. Rep. 5:14345. doi: 10.1038/srep14345
Wang, J., Zhang, L., Lu, Q., Raza, W., Huang, Q., and Shen, Q. (2014). Ammonia oxidizer abundance in paddy soil profile with different fertilizer regimes. Appl. Soil Ecol. 84, 38–44. doi: 10.1016/j.apsoil.2014.06.009
Will, C., Thurmer, A., Wollherr, A., Nacke, H., Herold, N., Schrumpf, M., et al. (2010). Horizon-specific bacterial community composition of German grassland soils, as revealed by pyrosequencing-based analysis of 16S rRNA genes. Appl. Environ. Microbiol. 76, 6751–6759. doi: 10.1128/AEM.01063-10
Yan, N., and Marschner, P. (2013). Response of soil respiration and microbial biomass to changing EC in saline soils. Soil Biol. Biochem. 65, 322–328. doi: 10.1016/j.soilbio.2013.06.008
Yang, Y., Wu, L., Lin, Q., Yuan, M., Xu, D., Yu, H., et al. (2013). Responses of the functional structure of soil microbial community to livestock grazing in the Tibetan alpine grassland. Glob. Change Biol. 19, 637–648. doi: 10.1111/gcb.12065
Yergeau, E., Bokhorst, S., Kang, S., Zhou, J., Greer, C. W., Aerts, R., et al. (2012). Shifts in soil microorganisms in response to warming are consistent across a range of Antarctic environments. ISME J. 6, 692–702. doi: 10.1038/ismej.2011.124
Yergeau, E., Kang, S., He, Z., Zhou, J., and Kowalchuk, G. A. (2007). Functional microarray analysis of nitrogen and carbon cycling genes across an Antarctic latitudinal transect. ISME J. 1, 163–179. doi: 10.1038/ismej.2007.24
Zhang, Y., Lu, Z., Liu, S., Yang, Y., He, Z., Ren, Z., et al. (2013). Geochip-based analysis of microbial communities in alpine meadow soils in the Qinghai-Tibetan plateau. BMC Microbiol. 13:72. doi: 10.1186/1471-2180-13-72
Keywords: paddy soil, GeoChip, Mi-Seq sequencing, microbial community, soil profile, soil type, network analysis
Citation: Bai R, Wang J-T, Deng Y, He J-Z, Feng K and Zhang L-M (2017) Microbial Community and Functional Structure Significantly Varied among Distinct Types of Paddy Soils But Responded Differently along Gradients of Soil Depth Layers. Front. Microbiol. 8:945. doi: 10.3389/fmicb.2017.00945
Received: 16 February 2017; Accepted: 11 May 2017;
Published: 29 May 2017.
Edited by:
Florence Abram, NUI Galway, IrelandReviewed by:
Hamed Azarbad, Institut National de la Recherche Scientifique – Institut Armand-Frappier, CanadaWeidong Kong, Institute of Tibetan Plateau Research (CAS), China
Copyright © 2017 Bai, Wang, Deng, He, Feng and Zhang. This is an open-access article distributed under the terms of the Creative Commons Attribution License (CC BY). The use, distribution or reproduction in other forums is permitted, provided the original author(s) or licensor are credited and that the original publication in this journal is cited, in accordance with accepted academic practice. No use, distribution or reproduction is permitted which does not comply with these terms.
*Correspondence: Li-Mei Zhang, emhhbmdsbUByY2Vlcy5hYy5jbg==