- 1Immunology, Department of Clinical and Experimental Medicine, University of Florence, Florence, Italy
- 2Department of Biology, University of Florence, Florence, Italy
- 3Department of Surgery and Translational Medicine, University of Florence, Florence, Italy
- 4Neuromusculoskeletal Department (Interdisciplinary Internal Medicine), Azienda Ospedaliera Universitaria Careggi, Florence, Italy
In this study Next-Generation Sequencing (NGS) was used to analyze and compare human microbiota from three different compartments, i.e., saliva, feces, and cancer tissue (CT), of a selected cohort of 10 Italian patients with colorectal cancer (CRC) vs. 10 healthy controls (saliva and feces). Furthermore, the Fusobacterium nucleatum abundance in the same body site was investigated through real-time quantitative polymerase chain reaction (qPCR) to assess the association with CRC. Differences in bacterial composition, F. nucleatum abundance in healthy controls vs. CRC patients, and the association of F. nucleatum with clinical parameters were observed. Taxonomic analysis based on 16S rRNA gene, revealed the presence of three main bacterial phyla, which includes about 80% of reads: Firmicutes (39.18%), Bacteroidetes (30.36%), and Proteobacteria (10.65%). The results highlighted the presence of different bacterial compositions; in particular, the fecal samples of CRC patients seemed to be enriched with Bacteroidetes, whereas in the fecal samples of healthy controls Firmicutes were one of the major phyla detected though these differences were not statistically significant. The CT samples showed the highest alpha diversity values. These results emphasize a different taxonomic composition of feces from CRC compared to healthy controls. Despite the low number of samples included in the study, these results suggest the importance of microbiota in the CRC progression and could pave the way to the development of therapeutic interventions and novel microbial-related diagnostic tools in CRC patients.
Introduction
Colorectal cancer (CRC) is the third most frequent tumor worldwide and is considered as the fourth leading cause of cancer deaths (Rowland, 2009), accounting for about 1.2 million new cases and 600,000 deaths per year. The CRC etiology is still not fully understood, but the development of colorectal carcinogenesis is a heterogeneous progression with different sets of genetic and epigenetic variations, and is influenced by dietary patterns, environmental conditions, host immunity, and microbial adhesion (as reviewed in Russo et al., 2016). A large number of microbes is able to live and thrive in the human gut, forming huge and complex communities, which, in turn, may play key roles in the CRC development (Warren et al., 2013; Gao et al., 2017).
Also, the association between some bacteria with the initiation and progression of neoplasia, e.g., Helicobacter pylori to gastric cancer (Amedei et al., 2014), has been well-established. This infection is the cause of approximately 5.5% of all cancers (Parkin, 2006). Whilst the fine processes of cancerogenesis have not been fully elucidated, it is reasonable to prevent its risk by targeting the possible microbial etiologic agent(s), as previous studies have suggested a potential dysbiosis of gut microbiota in CRC patients (Akin and Tözün, 2014). Many works have detected the hypothetical pathogens linked to CRC pathogenesis, aimed to improve CRC prevention and treatment (as reviewed in Russo et al., 2016). In the last years, the 16S rRNA gene sequencing approach has been widely used as an effective tool to analyze the complex microbial community (Eckburg et al., 2005). The obtained results suggested that the breakdown of the intestinal microbiota structure could promote carcinogenesis and CRC development.
Other studies of CRCs reported the presence of links between the intestinal microbiota and bacterial metabolites and the tumor development in murine colitis-associated CRC models (Gao et al., 2015; O'Keefe, 2016). Indeed, fermenting bacteria may produce protective molecules in the colon such as butyrate, the conjugate base of butyric acid, which has been shown to exert a beneficial effect on colon cells (Lupton, 2004; Pessione, 2012). On the other hand, the antibiotic suppression of intestinal microbiota has been shown to reduce the lipoperoxidation associated with the proliferation of colon carcinogenesis in murine models (Martin et al., 2015).
The presence of specific microorganisms in the microbiota and the subsequent inflammation (often microbial driven) and cancer are linked (Mantovani, 2009; Ben-Neriah and Karin, 2011). The inflammatory tumor process is actually added as a distinct cancer hallmark (Hanahan and Weinberg, 2011). Recent studies suggested that both the whole colon microbiota and specific pathogenic microbial strains likely play a crucial role in CRC development, in both direct and indirect ways (Candela et al., 2014; Lin and Zhang, 2017). In this context, Fusobacterium nucleatum appears to be particularly interesting. Indeed this nonspore-forming, anaerobic gram-negative oral commensal bacterium, is linked to periodontal disease, but several reports have shown a correlation with a wide range of disorders, including gastrointestinal and cardiovascular diseases, rheumatoid arthritis, respiratory tract infections, and Alzheimer's disease (for a review see Han, 2015). Furthermore, it is increasingly emerging that members of Fusobacterium genus, in particular F. nucleatum, inhabit stool and tumor tissues samples of patients affected by the CRC and colorectal adenomas (Ito et al., 2015). On the other hand, few studies suggested a correlation between F. nucleatum and colon cancer invasiveness (e.g., lymph node metastasis); however, this hypothesis has not been completely corroborated (Castellarin et al., 2012; Flanagan et al., 2014), even though an enrichment of F. nucleatum in CRC tissue has been detected, by both metagenomic and qPCR analyses of DNA from feces (Kostic et al., 2012).
In this study we used targeted metagenomics based on 16S rRNA gene to deeply explore three different human districts trying to define possible microbial markers of CRC not exclusively related to the tumor lesion.
Materials and Methods
Patients
In total, 10 Italian patients (four males and six females with an age range of 71–95 years) with colorectal adenocarcinoma (confirmed by histological analysis), undergoing surgical resections at the Unit of Surgery, University Hospital of Careggi (AOUC), University of Florence, between October 2015 and March 2016 and 10 healthy controls (six males and four females with an age range of 63–86 years) were recruited. The patients with other CRCs, or exposed to antibiotic therapy within 3 months prior to sample collection, as well those who have undertaken radiotherapy and chemotherapy before the surgical resection and those with comorbid malignancies of other organs were excluded. The 10 healthy subjects were selected based on age, and body mass index, no gastrointestinal disorders, no antibiotic use during the 3-month period prior to sample collection. All the 10 CRC patients were divided according to the pathological TNM (tumor-Node-Metastasis) staging system 7th edition and Dukes classification (Williams and Beart, 1992). The TNM classification uses three parameters to divide the patients into different stages: depth of tumor penetration into the gastric wall (T parameter), the number of metastatic regional lymph nodes involved (N parameter) and the presence of distant metastases (M parameter). Data collected included anthropometric measurements (height, weight), nutritional data (including the use of probiotics), clinical history and status and medication history (Table 1 and Table S1).
Unstimulated saliva and stool were collected from both patients and healthy controls, while CT samples were collected only from CRC patients. The day before surgery, in the early morning, saliva was collected by spitting after 1 min without swallowing into a sterile tube; stool samples were collected in a sterile container. CT samples were collected in sterile conditions during surgery. The oral health status of the patients was assessed as it can have a major impact on the salivary microbiota (Table S1). For ethical reasons, we collected only saliva and stool samples from healthy controls. After collection, saliva and stool samples were immediately frozen and stored at −80°C until DNA extraction. Fresh CRC tissues from each patient were collected in physiological solution (NaCl 0.9%). Samples were stored at −80° until use. The study protocols were approved by the ethics committee of AOUC Careggi and complied with the “Declaration of Helsinki.” A written approval was obtained from each participant.
Bacterial DNA Extraction
DNA was extracted from all samples using PowerLyzer® PowerSoil® DNA Isolation Kit (MO BIO laboratories, Inc., Carlsbad, California, USA) with some modifications to the manufacturer's instructions for fecal and saliva samples (Gao et al., 2015).
The modifications were performed on fecal samples as follows (Wesolowska-Andersen et al., 2014): 0.5 g of each stool sample was treated with 2.5 ml of PowerSoil® Bead Solution. Samples were vortexed and then centrifuged at room temperature for 5 min at 1,500 × g. 1 ml of the supernatant was transferred to a PowerLyzer® Glass Bead Tube and 750 ml of PowerSoil® Bead Solution were added. Each sample was incubated for 10 min at 65°C and then for 10 min at 95°C. By this step, manufacturer's instructions were followed.
The modifications on saliva samples were performed as follows (Aagaard et al., 2013): 2 ml of each saliva sample was centrifuged at room temperature for 15 min at 2,600 g. The pellet was resuspended with 750 μl of the PowerSoil® Bead Solution. Then, the sample was loaded into the PowerLyzer® Glass Bead Tube according to the manufacturer's instructions.
Quantity and purity of extracted DNA were checked by 0.8% agarose gel electrophoresis in Tris-EDTA buffer. Each sample was then quantified with the Qubit fluorometer (Life Technologies). All DNA samples were stored at −20°C until used.
16S rRNA Gene Analysis
Extracted DNA samples were sent to an external company (IGA Technology Services-Udine-Italy) for library construction and sequencing on the MiSeq Illumina platform with paired-end protocol. In particular, the V3-V4 region of bacterial 16S rRNA gene was amplified via PCR using specific primers (806R and 515F) as previously described (Checcucci et al., 2016). Raw sequences were processed following the UPARSE pipeline (Edgar, 2013). First, sequences were quality refined using StreamingTrim 1.0 (Bacci et al., 2014). Low quality segments were removed using a quality cutoff of 18 Phreds whereas constructs that might have been generated during sequencing procedures, were removed only if found in the top 50 residues of each sequence. Mate pairs were subsequently assembled using the “fastq_mergepairs” command of the USEARCH suite (Edgar et al., 2011). About 92% of the initial pairs were correctly merged, collecting more than 12 million sequences. Sequences were additionally filtered using the “fastq_filter” command of the USEARCH suite with a maximum error rate of 1.0 and a fixed length of 300 bp. Identical sequences were then merged (de-replication) and singleton sequences, namely those found only one time, were removed to reduce errors due to the amplification process (Morgan et al., 2014). De-replicated sequences with an identity higher than 97% were pooled together using the “cluster_otus” commands of the USEARCH suite. Putative chimeric sequences were automatically detected during this step, but an additional chimeric identification step was also included, using the “uchime_ref” command (Edgar et al., 2011) in combination with the latest RDP training set available (trainset16 02/2016) (Cole et al., 2014). Finally, 17′971 representative sequences were collected and used as reference for the clustering step that was performed using the “-usearch_global” command of the USEARCH suite with a sequence identity threshold of 97% (corresponding to the species level according to Konstantinidis and Tiedje (2007). More than 72% of the initial sequences were correctly assigned to an OTU (8′595′385 sequences). For each OTU (cluster), a single representative sequence was used for taxonomic annotation. Sequences were classified using the SINA standalone classifier in combination with the “Ref NR 99” database (release 123) (Pruesse et al., 2012). Clusters classified as “Eukaryota” were removed along with unclassified clusters retaining a set of 2,386 clusters classified as “Bacteria” or “Archaea.” A detailed description of the number of sequences collected at each step of analysis is reported in Table S2.
Real-Time Quantitative PCR
To estimate the relative amount of F. nucleatum over the total amount of bacteria, the DNA from each sample was assayed by real-time quantitative PCR (qPCR); the estimation of the total number of 16S rRNA gene copies in all samples was performed with bacterial primers Eub341F (5′- CCTACGGGAGGCAGCAG-3′) and Eub515R (5′-TACCGCGGCKGCTGGCA-3′) targeting the 16S rRNA gene, using a previously reported protocol (Abdelrhman et al., 2016); the value of F. nucleatum was assessed with specific primers: fuso-F (5′-CTTAGGAATGAGACAGAGATG-3′) and fuso-R (5′-TGATGGTAACATACGAAAGG-3′) (Periasamy and Kolenbrander, 2009), targeting a fragment of 16S rRNA gene. qPCR was performed in an QuantStudio™ 7 apparatus (Applied Biosystems), following the amplification program described in Periasamy and Kolenbrander (2009). F. nucleatum DSM 20482 (ATCC 10953) DNA was used as standard for qPCR quantification. Reactions were performed in triplicates in 10 μl final volume as described in Checcucci et al. (2016). qPCR results were analyzed by comparing the Cq values of the samples, representing the threshold cycles; Cq is a relative measure of the concentration of the target gene in the PCR reaction; lower Cq values indicate high amounts of targeted nucleic acid, while higher Cq values indicate lower amounts of the target nucleic acid. The presence of F. nucleatum has been calculated as the ratio between the Cq value of F. nucleatum 16S rRNA gene and the Cq value of the total bacterial community 16S rRNA gene amplicons. The results were analyzed with the Mann-Whitney pairwise post-hoc tests, using the PAST3 Software (Hammer et al., 2001).
Statistical Analysis
Statistical analyses on the bacterial community distribution were implemented in R (R Core Team, 2014) using the vegan package (version 2.3-2) (Oksanen et al., 2015). Analysis of similarity (ANOSIM, “anosim” function) was conducted to test the statistical significance of difference between distinctive bacterial communities.
Microbiome dataset was analyzed in R (R Core Team, 2014) with the help of additional libraries as reported below. Accumulation curves were calculated using the “specaccum” function (vegan package version 2.4) (Oksanen et al., 2015) adding sites in random order with 100 permutations for each step. Differences in bacterial population structure between different body sites were inspected using the “anosim” function (vegan package) with 1,000 permutations and plotted using the ggplot2 library (version 2.2) (Wickham, 2009). Hierarchical clustering of bacterial distribution was performed based on the average linkage method with Bray-Curtis dissimilarity indexes (“vegdist” function, vegan package) and visualized using custom scripts. OTU counts were normalized for each sample by dividing them by the total counts of all OTUs within that sample. Differences in the abundance of OTUs assigned to Fusobacterium genus between CRC and healthy patients were inferred using Student's t-test on normalized data (“t-test” function of the R stats package). The distribution of Fusobacterium representatives in biopsy samples of CRC patients was modeled using linear models and one-way analysis of variance (“lm” and “aov” functions of stats Package) on quantitative and qualitative factors, respectively. The LEfSe pipeline (Segata et al., 2011) was performed on OTU count collapsed according to shared taxonomic classifications to identify distinctive taxa of each body site here explored (saliva, feces, and biopsy). Differentially abundant OTUs were detected using differential abundance analysis with a zero-inflated log-normal model as implemented in the “fitFeatureModel” function of the metagenomeSeq R package, version 1.16 (Paulson et al., 2013).
Shannon, Chao 1, Evenness and Richness indices were used to estimate bacterial diversity in each sample (Tables S6, S7). The indices were computed using the “diversity” function of the vegan R package version 2.4. The percentage of coverage was calculated by Good's estimator (Good, 1953) using the formula: [1—(n/N)] × 100, where n is the number of sequences found once in a sample (singletons), and N is the total number of sequences in that sample. The Evenness index was calculated using the formula E = S/log(R), where S is the Shannon diversity index and R is the number of OTUs in the sample (the Richness). Different alpha diversity values were tested using mixed-effect model since our data contained observations that were not necessarily independent of one another, namely the patients (West et al., 2014). The “lme” function of the nlme R package (version 3.1) (Pinheiro et al., 2016) was used to construct a model for each diversity index measured (Table S3) to inspect different diversity level across different environment and between healthy and CRC patients. A random intercept model was built using subject ids as a random effect for each index considered.
Accession Number
The 16S rRNA gene sequence data generated in this study was submitted to the GenBank Sequence Read Archive accession number PRJNA356414.
Ethics Approval and Consent to Participate
The study was reviewed and approved by AOUC Careggi Institutional Review Board (Prot 2010/0012462). All study participants, or their legal guardian, have provided an informed written consent prior to the study enrollment in compliance with national legislation and the Code of Ethical Principles for Medical Research Involving Human Subjects of the World Medical Association (Declaration of Helsinki).
Results
Overall Comparison of the Oral, Fecal, and Cancer Tissue Microbiota
We examined the oral cavity and gut (feces and cancer tissue) bacterial communities of 10 patients with CRC and 10 age- and healthy-controls (Table S1). A dataset of 8,595,385 high-quality 16S rRNA sequences (n = 20 patient and control fecal samples, n = 20 patient and control saliva samples, n = 10 CT samples) was obtained. The final data set included 574 bacterial genera belonging to 36 different phyla with a different distribution across samples (Figure 1). Samples reported a Good's coverage estimator ranging from 99 to 100% indicating that roughly 1% of the reads in a given sample came from OTUs that appear only once in that sample (Table S3). The alpha diversity of samples from healthy patients and samples from CRC patients did not report significant differences. However, at body site level differences were observed. In particular, biopsy samples showed a higher level of both Shannon index and Evenness (Kruskal-Wallis test, χ2 = 9.9, p-values = 0.007 for Shannon index, and χ2 = 10.9, p-values = 0.004 for Evenness). The highest value of Evenness in biopsy samples together with the highest value of Shannon index revealed a high complexity of this environment. The analysis of the taxonomic composition revealed that more than 85% of the sequences collected were classified into five phyla: Firmicutes (39.18%), Bacteroidetes (30.36%), Proteobacteria (10.65%), Fusobacteria (5.15%), and Actinobacteria (4.22%). Differences in bacterial community composition were assessed using the analysis of similarity (ANOSIM function with 1,000 permutations). The different body sites showed different bacterial compositions (R = 0.834, P = 0.001), along with feces samples coming from controls and CRC patients (R = 0.201, P = 0.005). CT samples exhibited a composition of bacterial communities different from that present in stool samples in CRC patients (R = 0.61, P = 0.001). Accumulation curves reported in Figure 2 showed a different biodiversity level across body sites. In particular biopsy samples of patients affected by CRC were the ones reporting the highest level of bacterial diversity as confirmed by Kruskall-Wallis test reported in Figure 3. Hierarchical cluster analysis based on the log transformed abundances of OTUs showed that saliva samples are clustered together confirming that there is no statistical difference between the microbiota of CRC and healthy patients inhabiting this compartment (ANOSIM analysis: R = 0.049, P = 0.176). CT samples showed the highest alpha diversity value compared to other body sites, whereas the taxonomic diversity of samples from CRC and healthy patients was similar within the same body site (Table S4 and Figure 3). In contrast, CT samples seem to have a distinct pattern of bacterial distribution as reported in Figure 4 (cluster 3) with a wide distribution of bacterial genera belonging to the major phyla previously detected (Proteobacteria, Bacteroidetes, and Firmicutes).
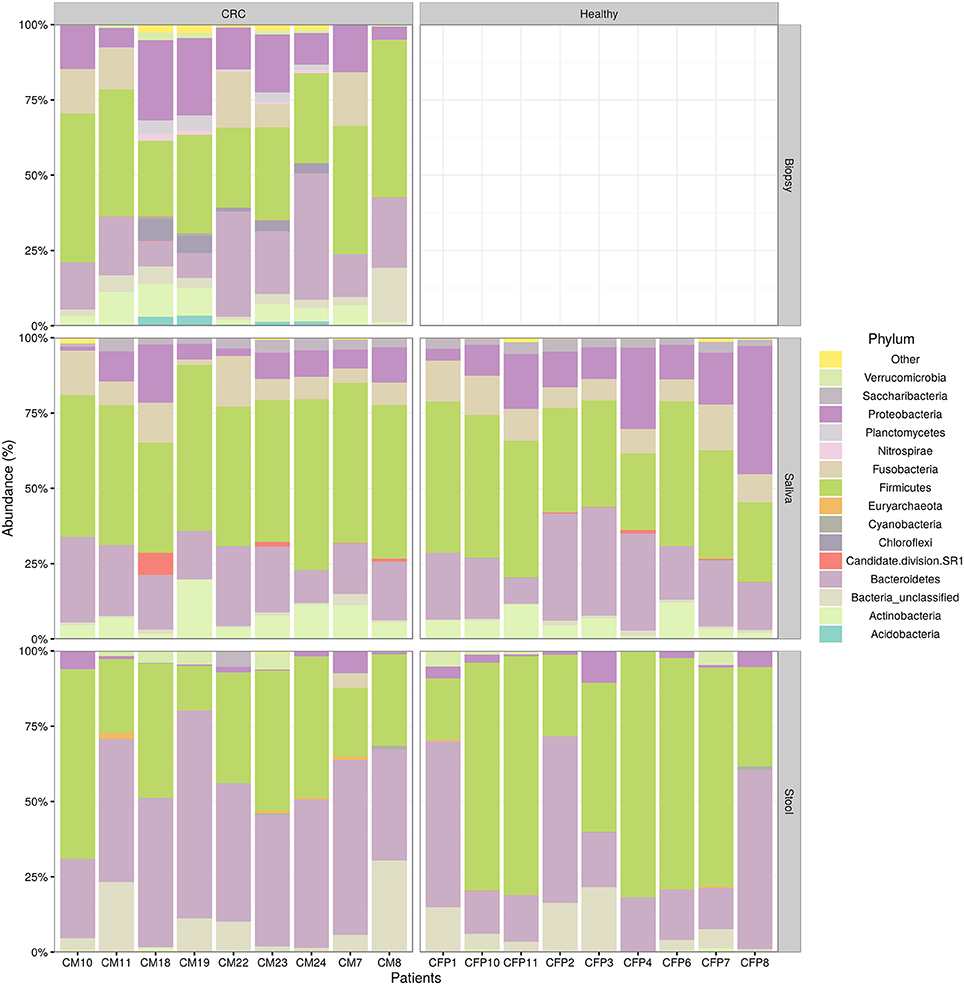
Figure 1. Taxonomic composition of CRC patient (and healthy controls) microbiota. Relative abundances bar plot showing the relative abundance of bacterial phyla in each sample. All phyla representing less than 5% of the total reads analyzed were included in the “Other” group.
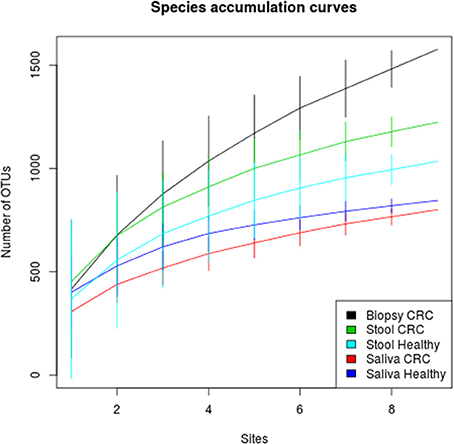
Figure 2. Species accumulation curves of healthy and CRC patients for each sampling body site. CT samples seem to have the highest alpha diversity whereas the bacterial diversity of CRC patients and controls is similar within each body site.
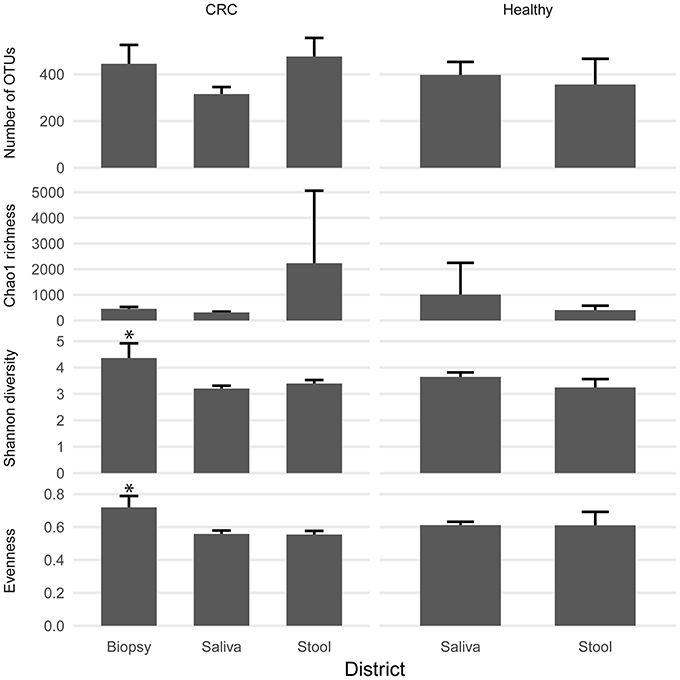
Figure 3. Biodiversity indices distribution according to sampling site and patient status. Patients with CRC and healthy ones reported similar diversity values for all the indices considered. CRC patients showed higher values of alpha diversity in biopsy samples. Asterisks indicate a significant Kruskal-Wallis test with alpha = 0.05.
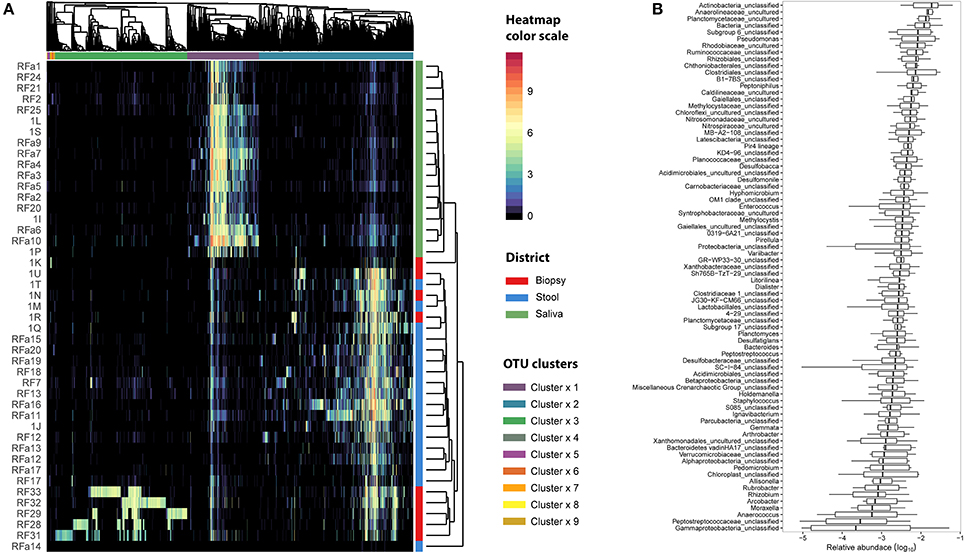
Figure 4. Microbiota distribution for each analyzed sample. (A) The number of reads assigned to each OTU was log transformed and reported using the color scale whereas sampling body sites were reported with different colors (red for biopsy samples, blue for stool samples, and green for saliva samples). Dendrograms were produced with average clustering method (UPGMA) based on Bray-Curtis distance. Clusters of OTUs were assessed cutting the dendrogram at 0.95 height. (B) The relative abundance of OTUs belonging to cluster 3 was collapsed at Genus level taking into account only the biopsy samples. Genera with an abundance lower than 1% were removed from the analysis. Relative abundance was computed dividing the number of 16S sequences assigned to each OTU by the total number of sequences obtained for each sample. Boxes denote the interquartile range (IQR) between the 25th and the 75th percentile (first and third quartiles), whereas the inner line represents the median. Whiskers represent the lowest and highest values within 1.5 times IQR from the first and third quartiles Outliers were reported using white circles.
qPCR Assay and Association of F. nucleatum Colonization with Clinicopathologic Features of CRC Patients
Several studies in literature suggested the role of F. nucleatum as cancer-promoting bacteria (Castellarin et al., 2012; Kostic et al., 2012; Flanagan et al., 2014; Ito et al., 2015; Ramos and Hemann, 2017). Members of Fusobacterium genus were detected through 16S rRNA analysis but this approach did not allow us to reach species resolution; thus we decided to further investigate the distribution of this genus using qPCR to confirm the presence of members of F. nucleatum species. In Figure 5, the quantification of F. nucleatum expressed as the ratio between the Cq value of F. nucleatum 16S rRNA gene, and the Cq value of the total bacterial community 16S rRNA gene amplicons is reported. Consequently, the lowest ratio values indicate higher F. nucleatum abundance in the samples. No significant differences between stool samples of healthy subjects and stool samples of CRC patients were observed (Table S5). Moreover, neither statistically significant differences were evidenced between saliva samples of healthy and CRC subjects. However, results highlighted that F. nucleatum abundance is higher in saliva than stool samples both in healthy subjects (p < 0.002) and in CRC patients (p < 0.01).
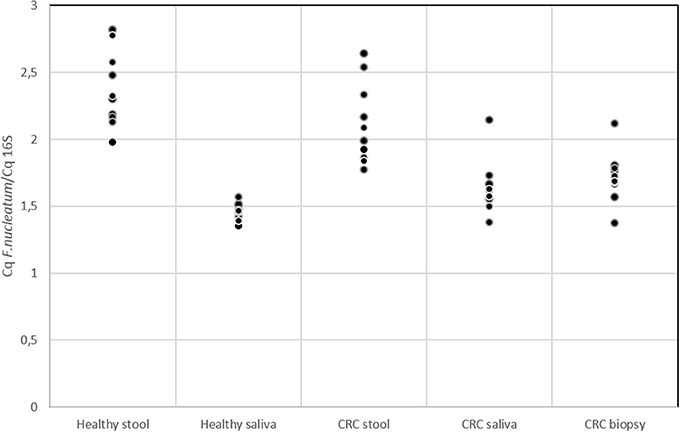
Figure 5. qPCR results for quantification of F. nucleatum respect to the total bacterial community; quantification is expressed as the ratio between the Cq value of F. nucleatum 16S rRNA gene and the Cq value of the total bacterial community 16S rRNA gene amplicons. The higher is the ratio value, the lowest is the quantification of the target amplicon in the sample.
NGS 16S rRNA data were then analyzed, taking into account all OTUs assigned to Fusobacterium genus. A search over the GenBank database by using the BLAST algorithm sorted out that all but OTU 300, matched with F. nucleatum sequences in the top-10 hits with an identity threshold ≥95%, confirming the feasibility of using NGS data for F. nucleatum DNA detection in our samples. Obtained results (Figure 6) confirmed qPCR data, revealing that stool samples had the lowest number of reads assigned to this genus in contrast with CT samples, which showed the highest number of sequences related to Fusobacterium genus. Neither NGS data were underlining differences between healthy controls and CRC patients.
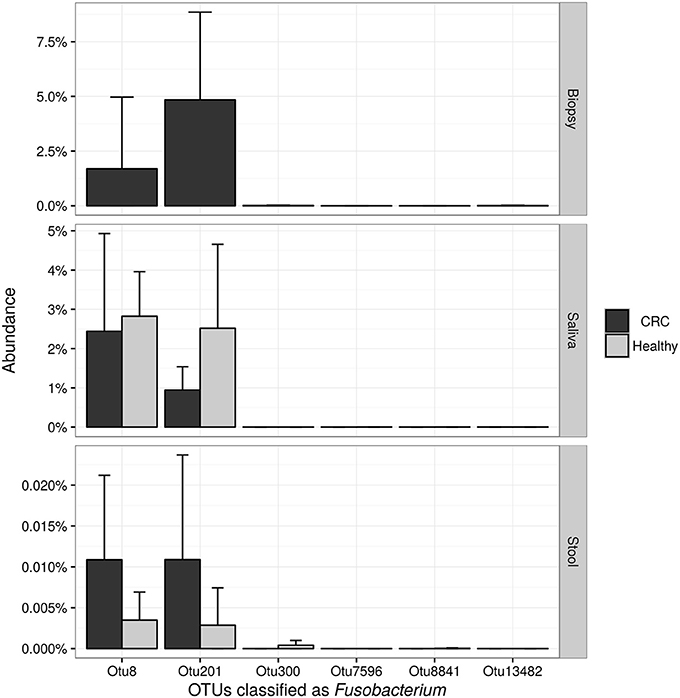
Figure 6. Barplots reporting the average number of sequences assigned to each OTU classified as Fusobacterium. Error bars represent the 95% confidence interval of the distribution.
Distinct Taxonomic Distribution in Different Body Sites
Linear discriminant analysis revealed a distinct microbial distribution in regard of sampling site (Figure 7A). In particular, saliva samples were mainly enriched in members of Actinobacteria, Saccharibacteria, Proteobacteria (Beta class), Fusobacteria, Firmicutes (mainly Negativicutes and Bacilli), and Bacteroidetes (exclusively represented by members of Flavobacteriia class and Prevotellaceae family) whereas stool samples presented a characteristic distribution of Bacteroidetes (in particular the Bacteroidia class) and Firmicutes (mainly Clostridia and Erysipelotrichia classes). Interestingly, biopsy samples were characterized by members of Proteobacteria, mainly Delta, Alpha, and Gamma classes, Planctomycetes, and Firmicutes (namely Lachnospiraceae family and Clostridiales family XI). Despite the increased abundance of members of Fusobacteria in saliva samples (Figure 7B), biopsy samples were enriched in members of Fusobacterium genus (Figure 7C). Interestingly, only one OTU classified as Fusobacterium was detected in cluster 3 (Figure 4A) reporting a mean abundance lower than 1%. On the contrary, collapsed counts based on shared taxonomic classifications showed a higher presence of members of Fusobacterium genus (2.9% in the whole dataset) and an even higher abundance in biopsy samples (5.9%, Figure 7C).
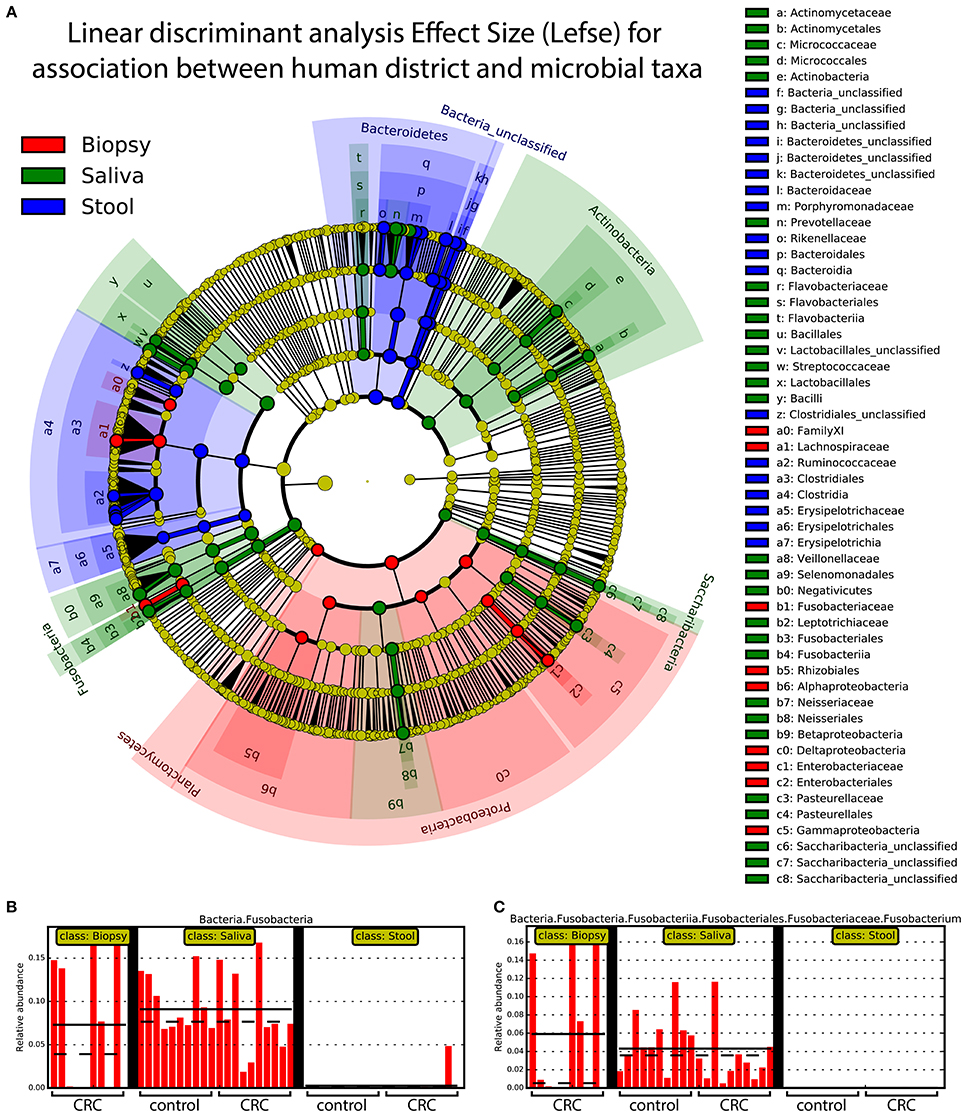
Figure 7. Linear discriminant analysis of association between sampling sites and microbial taxa. A linear discriminant analysis was performed using Lefse and considering the three body sites sampled in this study, namely: biopsy (red), saliva (green), and stool (blue). (A) Different body sites showed a characteristic taxonomic composition with major clades strongly associated with a particular site. Proteobacteria were mostly associated with biopsy samples whereas Fusobacteria and Bacteroidetes were mainly associated with saliva and stool samples, respectively. (B) Saliva samples reported highest values of Fusobacteria members even if (C) Fusobacterium genus was mainly found in biopsy samples. Each body site has been represented using different colors (A) whereas the relative abundance of Fusobacteria (B) and Fusobacterium clade (C) was reported for each subject. Samples coming from control and CRC patients were reported below (B,C).
Discussion
Comparison of Oral and Intestinal Microbiota in CRC Patients vs. Healthy Controls
The aim of this study was to explore, characterize, and compare the bacterial community composition in different body compartments (saliva, stool, and cancer tissue) of CRC Italian patients, through the NGS analysis. Data obtained revealed that the bacterial communities of the three analyzed body sites of both CRC patients and healthy individuals differed significantly in terms of bacterial community composition: the analysis of similarity showed different bacterial taxonomic compositions in saliva, stool, and biopsy. In addition, also linear discriminant analysis (performed with the “lefse“ pipeline to detect distinctive taxa of saliva, feces, and biopsy) revealed a distinct microbial distribution with respect to sampling sites. According to previous studies (Gao et al., 2015), the relative richness values of dominant phyla Bacteroidetes, Firmicutes, and Proteobacteria were all different in both CRC patients and healthy controls.
Saliva is a biological fluid that could be suitable for biomarker detection. The average adult produces more than 1,000 ml of saliva per day, which always flows into the gastrointestinal tract, thus, the salivary microbiota affects the development of intestinal microbiota in some respects. Our study shows that the microbial community of saliva is, as expected, very different from that inhabiting the other two body sites of the same patient. Variations of the salivary microbiota composition in oral health and disease conditions have been previously described (Belstrøm, 2015), suggesting that the oral microbial compositions may theoretically reflect the oral and general health status. According to previous data (Aas et al., 2005; Keijser et al., 2008; Lazarevic et al., 2009; Nasidze et al., 2009; Bik et al., 2010), we observed that sequences affiliated to the phylum Firmicutes dominates the bacterial communities in saliva samples, even though no significant difference was found between CRC patients and healthy controls The application of the linear discriminant analysis, showed an enrichment in members of Actinobacteria, Saccharibacteria, Proteobacteria (Beta class), Fusobacteria, Firmicutes (mainly Negativicutes and Bacilli), and Bacteroidetes (exclusively represented by members of Flavobacteriia class and Prevotellaceae family) in saliva samples. In particular, we observed an enrichment of Fusobacteria, in agreement with previously reported data (Bolstad et al., 1996). Stool samples exhibited a characteristic distribution of Bacteroidetes (in particular the Bacteroidia class) and Firmicutes (mainly Clostridia and Erysipelotrichia classes).
Our data suggest that bacterial communities and CRC might be further investigated for their possible correlations in order to assess the opportunity of detecting colon cancer through the analysis of specific fecal bacterial markers. In particular, the fecal-associated microbiota could be dynamically linked to colon cancer, which, in turn, may offer evidence for microflora-associated diagnostic, preventive, prognostic and therapeutic approaches for CRC. However, it is clear that additional studies will be required before a complete examination of these findings.
Because of the prognostic relevance of CRC detection and the prospective testing of fecal samples for bacterial biomarkers, we evaluated whether fecal microbiota profiles mirrored those findings for the colon mucosa tissue. For ethical reasons, healthy intestinal biopsies were not included in the pilot study; accordingly, only few studies evaluating the CRC mucosal microbial community included healthy individuals as controls (Dejea et al., 2014; Geng et al., 2014). As expected, we found that the microbiota compositions in neoplastic tissue significantly diverge from the intestinal lumen (stool) one, in agreement with Flemer et al. (2017). The relative abundance of dominant phyla Firmicutes, Bacteroidetes, Proteobacteria, Fusobacteria were all different in the two examined body sites (cancer tissue and stool). In particular, Proteobacteria and Fusobacteria were more abundant in cancer specimens, while a significantly higher abundance of Firmicutes and Fusobacteria were observed in the stools of CRC patients.
F. nucleatum Colonization in Patients with CRC vs. Healthy Controls
The presence of F. nucleatum in saliva, tumor tissue, and stool samples was investigated. It is not yet clear whether the presence of particular bacteria (not yet known) in the neoplastic microenvironment is indicative of a causative role in the CRC genesis and development (Gao et al., 2015). It has been previously suggested that the intestinal dysbiosis resulted in different types of colorectal pathologies, as well as CRC, but no specific direct link with the presence of this bacterium has been established (Tremaroli and Bäckhed, 2012; Allen-Vercoe and Jobin, 2014). Recently, NGS investigations provided much significant evidence in this CRC field, especially about the association of F. nucleatum with CRC. This bacterium is not a predominant species in stool samples and has been observed in cancer biopsies of CRC subjects by two independent research groups. Using whole-genome sequencing techniques, an enrichment of Fusobacterium spp. was observed, DNA sequences were detected in CT samples compared with the control ones (Kostic et al., 2012). Notably, among them, the most represented phylotype was F. nucleatum. On the other hand, using RNA-sequencing approaches, the group of Castellarin et al. (2012) detected a richness of Fusobacterium spp. in CRC vs. healthy tissues. On these premises, the two research groups have proposed a direct link between this bacterium and CRC pathogenesis. F. nucleatum is commonly found in periodontal plaque and, in the oral cavity, it is associated with viruses, which adhere to host tissue cells influencing the host's immune response (Bolstad et al., 1996). As expected qPCR data (Figure 5) highlighted that in saliva samples the presence of F. nucleatum is higher (with respect to total bacterial) than in stool (Bolstad et al., 1996). However, we cannot a priori exclude the possibility that our analysis detected also a mixture of closely related (and potentially still unknown) Fusobacterium strains/species. F. nucleatum is a key player in modifying intestinal inflammation levels (Rubinstein et al., 2013). In addition, in a study about colorectal adenomas, the abundance of F. nucleatum was found to positively correlate with inflammatory cytokine gene expression such as TNF (McCoy et al., 2013). TNF-α is produced during the inflammatory response and can promote survival, attachment, and proliferation of metastatic colon cancer cells in a mouse model of lung metastasis depending on the activation of NF-κB by inflammation and cancer cells (Luo et al., 2004). Moreover, through activation of NF-κB and STAT3, TNF-α can enhance epithelial-mesenchymal transition which are critical steps that allow polarized epithelial tumor cells to become mesenchymal like, enhancing cell migration and invasion (Yang and Weinberg, 2008). In light of possible roles of F. nucleatum in downregulating T cell-mediated antitumor immune responses (Kostic et al., 2013) and in promoting colorectal tumor progression, future investigations on a larger number of patients may be warranted to explore the impact of F. nucleatum on the T cell-based immunotherapy efficacy for CRC.
Conclusions
Here, we demonstrated a different bacterial taxonomic composition in CRC stool samples vs. healthy controls. We are aware that this study may suffer some limitations, such as the restricted number of enrolled patients, the not complete assessment of the oral health status (that might influence the composition of oral microbiota) and the lack of evaluation of other intestinal bacterial species (e.g., Escherichia coli and Bacteroides fragilis) (Arthur et al., 2012). In spite of this, our pilot study represents the first simultaneous comparison of the microbial compositions of the tree different body sites (saliva, stool, CT samples). Analyzing a single body niche to categorize CRC individuals does not enable researchers to comprehensively study the spatial variations of the microbiota in CRC. Therefore, the proposed strategy of characterizing the spatial community structures of CRC microbiota (according to Zhang et al., 2017) is crucial to improve our understanding of the mutual interplay between microflora and CRC presence.
However, to further validate these findings and to extend our knowledge about the variation of bacterial composition as a function of CRC (and vice versa), it will be helpful to account for a larger number of patients with longitudinal time points. This will promote the development of novel microbial-related diagnostic instruments and therapeutic approaches.
Availability of Data and Materials
The partial 16S rRNA gene sequence data generated in this study were submitted to the GenBank Sequence Read Archive accession number PRJNA356414.
Author Contributions
ER, RF, AA, and AM designed the research; AT, MR, EN, FR, FM, MM, and RB collected the samples; ER, CC, and CF performed the experiments; GB analyzed the data; ER, GB, CC, and CF wrote the paper; ER, AA, RF, AM, and PB supervised all the experimental work and revised the manuscript; all authors critically read and approved the manuscript.
Funding
The research was founded with a grant from the regional contribution of “The Programma Attuativo Regionale (Toscana) funded by FAS (now FSC), the Italian Ministry of University and Research (MIUR) and the Foundation ‘Ente Cassa di Risparmio di Firenze.’ CC, CF, GB, RB, and ER were granted by FAS.
Conflict of Interest Statement
The authors declare that the research was conducted in the absence of any commercial or financial relationships that could be construed as a potential conflict of interest.
Acknowledgments
The authors thank to all the CRC patients enrolled the Unit of Surgery, University Hospital of Careggi (AOUC), University of Florence, and the healthy controls. The authors thank Dr. Mirella Vivoli for the valuable help in revising the manuscript. We are also very grateful to referees for their comments and suggestions in improving the manuscript.
Supplementary Material
The Supplementary Material for this article can be found online at: https://www.frontiersin.org/articles/10.3389/fmicb.2017.02699/full#supplementary-material
Abbreviations
NGS, Next-Generation Sequencing; CT, cancer tissue; CRC, colorectal cancer; qPCR, Quantitative polymerase chain reaction; OTUs, operational taxonomic units.
References
Aagaard, K., Petrosino, J., Keitel, W., Watson, M., Katancik, J., Garcia, N., et al. (2013). The human microbiome project strategy for comprehensive sampling of the human microbiome and why it matters. FASEB J. 7, 1012–1022. doi: 10.1096/fj.12-220806
Aas, J. A., Paster, B. J., Stokes, L. N., Olsen, I., and Dewhirst, F. E. (2005). Defining the normal bacterial flora of the oral cavity. J. Clin. Microbiol. 43, 5721–5732. doi: 10.1128/JCM.43.11.5721-5732.2005
Abdelrhman, K. F. A., Bacci, G., Mancusi, C., Mengoni, A., Serena, F., and Ugolini, A. (2016). A first insight into the gut microbiota of the sea turtle Caretta caretta. Front. Microbiol. 7:1060. doi: 10.3389/fmicb.2016.01060
Akin, H., and Tözün, N. (2014). Diet, microbiota, and colorectal cancer. J. Clin. Gastroenterol. 48(Suppl. 1), S67–S69. doi: 10.1097/MCG.0000000000000252
Allen-Vercoe, E., and Jobin, C. (2014). Fusobacterium and Enterobacteriaceae: important players for CRC? Immunol. Lett. 162, 54–61. doi: 10.1016/j.imlet.2014.05.014
Amedei, A., Munari, F., Bella, C. D., Niccolai, E., Benagiano, M., Bencini, L., et al. (2014). Helicobacter pylori secreted peptidyl prolyl cis, trans-isomerase drives Th17 inflammation in gastric adenocarcinoma. Intern. Emerg. Med. 9, 303–309. doi: 10.1007/s11739-012-0867-9
Arthur, J. C., Perez-Chanona, E., Mühlbauer, M., Tomkovich, S., Uronis, J. M., Fan, T. J., et al. (2012). Intestinal inflammation targets cancer inducing activity of the microbiota. Science 338, 120–123. doi: 10.1126/science.1224820
Bacci, G., Bazzicalupo, M., Benedetti, A., and Mengoni, A. (2014). StreamingTrim 1.0: a java software for dynamic trimming of 16S rRNA sequence data from metagenetic studies. Mol. Ecol. Resour. 14, 426–434. doi: 10.1111/1755-0998.12187
Belstrøm, D. (2015). “Salivary microbiota in oral health and disease,” in Oral Infections and General Health: From Molecule to Chairside, ed A. M. L. Pedersen (Cham: Springer), 115–122.
Ben-Neriah, Y., and Karin, M. (2011). Inflammation meets cancer, with NF-κB as the matchmaker. Nat. Immunol. 12, 715–723. doi: 10.1038/ni.2060
Bik, E. M., Long, C. D., Armitage, G. C., Loomer, P., Emerson, J., Mongodin, E. F., et al. (2010). Bacterial diversity in the oral cavity of 10 healthy individuals. ISME J. 4, 962–974. doi: 10.1038/ismej.2010.30
Bolstad, A. I., Jensen, H. B., and Bakken, V. (1996). Taxonomy, biology, and periodontal aspects of Fusobacterium nucleatum. Clin. Microbiol. Rev. 9, 55–71.
Candela, M., Turroni, S., Biagi, E., Carbonero, F., Rampelli, S., Fiorentini, C., et al. (2014). Inflammation and colorectal cancer, when microbiota-host mutualism breaks. World J. Gastroenterol. 20, 908–922. doi: 10.3748/wjg.v20.i4.908
Castellarin, M., Warren, R. L., Freeman, J. D., Dreolini, L., Krzywinski, M., Strauss, J., et al. (2012). Fusobacterium nucleatum infection is prevalent in human colorectal carcinoma. Genome Res. 22, 299–306. doi: 10.1101/gr.126516.111
Checcucci, A., Azzarello, E., Bazzicalupo, M., Galardini, M., Lagomarsino, A., Mancuso, S., et al. (2016). Mixed nodule infection in Sinorhizobium meliloti-medicago sativa symbiosis suggest the presence of cheating behavior. Front. Plant Sci. 7:835. doi: 10.3389/fpls.2016.00835
Cole, J. R., Wang, Q., Fish, J. A., Chai, B., McGarrell, D. M., Sun, Y., et al. (2014). Ribosomal database project: data and tools for high throughput rRNA analysis. Nucleic Acids Res. 42, D633–D642. doi: 10.1093/nar/gkt1244
Dejea, C. M., Wick, E. C., Hechenbleikner, E. M., White, J. R., Mark Welch, J. L., Rossetti, B. J., et al. (2014). Microbiota organization is a distinct feature of proximal colorectal cancers. Proc. Natl. Acad. Sci. U.S.A. 111, 18321–18326. doi: 10.1073/pnas.1406199111
Eckburg, P. B., Bik, E. M., Bernstein, C. N., Purdom, E., Dethlefsen, L., Sargent, M., et al. (2005). Diversity of the human intestinal microbial flora. Science 308, 1635–1638. doi: 10.1126/science.1110591
Edgar, R. C. (2013). UPARSE: highly accurate OTU sequences from microbial amplicon reads. Nat. Methods 10, 996–998. doi: 10.1038/nmeth.2604
Edgar, R. C., Haas, B. J., Clemente, J. C., Quince, C., and Knight, R. (2011). UCHIME improves sensitivity and speed of chimera detection. Bioinformatics 27, 2194–2200. doi: 10.1093/bioinformatics/btr381
Flanagan, L., Schmid, J., Ebert, M., Soucek, P., Kunicka, T., Liska, V., et al. (2014). Fusobacterium nucleatum associates with stages of colorectal neoplasia development, colorectal cancer and disease outcome. Eur. J. Clin. Microbiol. Infect. Dis. 33, 1381–1390. doi: 10.1007/s10096-014-2081-3
Flemer, B., Lynch, D. B., Brown, J. M., Jeffery, I. B., Ryan, F. J., Claesson, M. J., et al. (2017). Tumour-associated and non-tumour-associated microbiota in colorectal cancer. Gut 66, 633–643. doi: 10.1136/gutjnl-2015-309595
Gao, R., Gao, Z., Huang, L., and Qin, H. (2017). Gut microbiota and colorectal cancer. Eur. J. Clin. Microbiol. Infect. Dis. 36, 757–769. doi: 10.1007/s10096-016-2881-8
Gao, Z., Guo, B., Gao, R., Zhu, Q., and Qin, H. (2015). Microbiota disbiosis is associated with colorectal cancer. Front. Microbiol. 6:20. doi: 10.3389/fmicb.2015.00020
Geng, J., Song, Q., Tang, X., Liang, X., Fan, H., Peng, H., et al. (2014). Co-occurrence of driver and passenger bacteria in human colorectal cancer. Gut Pathog. 6:26. doi: 10.1186/1757-4749-6-26
Good, I. J. (1953). The population frequencies of species and the estimation of population parameters. Biometrika 40, 237–264. doi: 10.1093/biomet/40.3-4.237
Hammer, Ø., Harper, D. A. T., and Ryan, P. D. (2001). PAST: paleontological statistics software package for education and data analysis. Palaeontol. Electronica 4:9
Han, Y. W. (2015). Fusobacterium nucleatum: a commensal-turned pathogen. Curr. Opin. 23, 141–147. doi: 10.1016/j.mib.2014.11.013
Hanahan, D., and Weinberg, R. A. (2011). Hallmarks of cancer: the next generation. Cell 144, 646–674. doi: 10.1016/j.cell.2011.02.013
Ito, M., Kanno, S., Nosho, K., Sukawa, Y., Mitsuhashi, K., Kurihara, H., et al. (2015). Association of Fusobacterium nucleatum with clinical and molecular features in colorectal serrated pathway: F. nucleatum and molecular features of serrated lesions. Int. J. Cancer 137, 1258–1268. doi: 10.1002/ijc.29488
Keijser, B. J., Zaura, E., Huse, S. M., van der Vossen, J. M., Schuren, F. H., Montijn, R. C., et al. (2008). Pyrosequencing analysis of the oral microflora of healthy adults. J. Dent. Res. 87, 1016–1020. doi: 10.1177/154405910808701104
Konstantinidis, K. T., and Tiedje, J. M. (2007). Prokaryotic taxonomy and phylogeny in the genomic era: advancements and challenges ahead. Curr. Opin. Microbiol. 10, 504–509. doi: 10.1016/j.mib.2007.08.006
Kostic, A. D., Chun, E., Robertson, L., Glickman, J. N., Gallini, C. A., Michaud, M., et al. (2013). Fusobacterium nucleatum potentiates intestinal tumorigenesis and modulates the tumor-immune microenvironment. Cell Host Microbe. 14, 207–215. doi: 10.1016/j.chom.2013.07.007
Kostic, A. D., Gevers, D., Pedamallu, C. S., Michaud, M., Duke, F., Earl, A. M., et al. (2012). Genomic analysis identifies association of fusobacterium with colorectal carcinoma. Genome Res. 22, 292–298. doi: 10.1101/gr.126573.111
Lazarevic, V., Whiteson, K., Huse, S., Hernandez, D., Farinelli, L., Osteras, M., et al. (2009). Metagenomic study of the oral microbiota by Illumina high-throughput sequencing. J. Microbiol. Methods 79, 266–271. doi: 10.1016/j.mimet.2009.09.012
Lin, L., and Zhang, J. (2017). Role of intestinal microbiota and metabolites on gut homeostasis and human diseases. BMC Immunol. 18:2. doi: 10.1186/s12865-016-0187-3
Luo, J. L., Maeda, S., Hsu, L. C., Yagita, H., and Karin, M. (2004). Inhibition of NF-kappaB in cancer cells converts inflammation- induced tumor growth mediated by TNFalpha to TRAIL-mediated tumor regression. Cancer Cell 6, 297–305. doi: 10.1016/j.ccr.2004.08.012
Lupton, J. R. (2004). Microbial degradation products influence colon cancer risk: the butyrate controversy. J. Nutr. 134, 479–482.
Martin, O. C. B., Lin, C., Naud, N., Tache, S., Raymond-Letron, I., Corpet, D. E., et al. (2015). Antibiotic suppression of intestinal microbiota reduces heme-induced lipoperoxidation associated with colon carcinogenesis in rats. Nutr. Cancer 67, 119–125. doi: 10.1080/01635581.2015.976317
McCoy, A. N., Araújo-Pérez, F., Azcárate-Peril, A., Yeh, J. J., Sandler, R. S., and Keku, T. O. (2013). Fusobacterium is associated with colorectal adenomas. PLoS ONE 8:e53653. doi: 10.1371/journal.pone.0053653
Morgan, M. J., Bass, D., Bik, H., Birky, C. W., Blaxter, M., Crisp, M. D., et al. (2014). Noise obscures the genetic signal of meiobiotal ecospecies in ecogenomic datasets. Proc. Biol. Sci. 281:20133076. doi: 10.1098/rspb.2013.3076
Nasidze, I., Li, J., Quinque, D., Tang, K., and Stoneking, M. (2009). Global diversity in the human salivary microbiome. Genome Res. 19, 636–643. doi: 10.1101/gr.084616.108
O'Keefe, S. J. (2016). Diet, microorganisms and their metabolites, and colon cancer. Nat. Rev. Gastroenterol. Hepatol. 13, 691–706. doi: 10.1038/nrgastro.2016.165
Oksanen, J., Guillaume Blanchet, F., Kindt, R., Legendre, P., Minchin, P. R., O'Hara, R. B., et al. (2015). Vegan: Community Ecology Package. R package version 2.3-2. 2015. Available online at: https://CRAN.R-project.org/package=vegan
Parkin, D. M. (2006). The global health burden of infection-associated cancers in the year 2002. Int. J. Cancer 118, 3030–3044. doi: 10.1002/ijc.21731
Paulson, J. N., Stine, O. C., Bravo, H. C., and Pop, M. (2013). Differential abundance analysis for microbial marker-gene surveys. Nature Methods 10, 1200–1202. doi: 10.1038/nmeth.2658
Periasamy, S., and Kolenbrander, P. E. (2009). Aggregatibacter actinomycetemcomitans builds mutualistic biofilm communities with Fusobacterium nucleatum and Veillonella species in saliva. Infect. Immun. 77, 3542–3551. doi: 10.1128/IAI.00345-09
Pessione, E. (2012). Lactic acid bacteria contribution to gut microbiota complexity: lights and shadows. Front. Cell Infect. Microbiol. 2:86. doi: 10.3389/fcimb.2012.00086
Pinheiro, J., Bates, D., DebRoy, S., Sarkar, D., and R Core Team (2016). _nlme: Linear and Nonlinear Mixed Effects Models_. R package version 3.1-128. Available online at: http://CRAN.R-project.org/package=nlme
Pruesse, E., Peplies, J., and Glöckner, F. O. (2012). SINA: accurate high-throughput multiple sequence alignment of ribosomal RNA genes. Bioinformatics 28, 1823–1829. doi: 10.1093/bioinformatics/bts252
Ramos, A., and Hemann, M. T. (2017). Drugs, bugs, and cancer: Fusobacterium nucleatum promotes chemoresistance in colorectal cancer. Cell 170, 411–413. doi: 10.1016/j.cell.2017.07.018
R Core Team (2014). R: A Language and Environment for Statistical Computing. Vienna: R Foundation for Statistical Computing. Available online at: http://www.R-project.org/
Rowland, I. R. (2009). The role of the gastrointestinal microbiota in colorectal cancer. Curr. Pharmaceut. Design 15, 1524–1527. doi: 10.2174/138161209788168191
Rubinstein, M. R., Wang, X., Liu, W., Hao, Y., Cai, G., and Han, Y. W. (2013). Fusobacterium nucleatum promotes colorectal carcinogenesis by modulating e-cadherin/β-catenin signaling via its fada adhesin. Cell Host Microbe. 14, 195–206. doi: 10.1016/j.chom.2013.07.012
Russo, E., Taddei, A., Ringressi, M. N., Ricci, F., and Amedei, A. (2016). The interplay between the microbiome and the adaptive immune response in cancer development. Ther. Adv. Gastroenterol. 9, 594–605. doi: 10.1177/1756283X16635082
Segata, N., Izard, J., Waldron, L., Gevers, D., Miropolsky, L., Garrett, W. S., et al. (2011). Metagenomic biomarker discovery and explanation. Genome Biol. 12:R60. doi: 10.1186/gb-2011-12-6-r60
Tremaroli, V., and Bäckhed, F. (2012). Functional interactions between the gut microbiota and host metabolism. Nature 489, 242–249. doi: 10.1038/nature11552
Warren, R. L., Freeman, D. J., Pleasance, S., Watson, P., Moore, R. A., Cochrane, K., et al. (2013). Co-occurrence of anaerobic bacteria in colorectal carcinomas. Microbiome 1:16. doi: 10.1186/2049-2618-1-16
Wesolowska-Andersen, A., Bahl, M. I., Carvalho, V., Kristiansen, K., Sicheritz-Pontén, T., Gupta, R., et al. (2014). Choice of bacterial DNA extraction method from fecal material influences community structure as evaluated by metagenomic analysis. Microbiome 2:19. doi: 10.1186/2049-2618-2-19
West, B. T., Welch, K. B., and Galecki, A. T. (2014). Linear Mixed Models: A Practical Guide Using Statistical Software. Boca Raton, FL: CRC Press.
Williams, S. T., and Beart, R. W. (1992). Staging of colorectal cancer. Semin. Surg. Oncol. 8, 89–93.
Yang, J., and Weinberg, R. A. (2008). Epithelial-mesenchymal transition: at the crossroads of development and tumor metastasis. Dev. Cell 14, 818–829. doi: 10.1016/j.devcel.2008.05.009
Keywords: colorectal cancer, gut microbiota, oral microbiota, Fusobacterium nucleatum, quantitative polymerase chain reaction, taxonomic analysis
Citation: Russo E, Bacci G, Chiellini C, Fagorzi C, Niccolai E, Taddei A, Ricci F, Ringressi MN, Borrelli R, Melli F, Miloeva M, Bechi P, Mengoni A, Fani R and Amedei A (2018) Preliminary Comparison of Oral and Intestinal Human Microbiota in Patients with Colorectal Cancer: A Pilot Study. Front. Microbiol. 8:2699. doi: 10.3389/fmicb.2017.02699
Received: 01 June 2017; Accepted: 26 December 2017;
Published: 12 January 2018.
Edited by:
Malka Halpern, University of Haifa, IsraelReviewed by:
Carlotta De Filippo, Consiglio Nazionale Delle Ricerche (CNR), ItalyNick Stephen Jakubovics, Newcastle University, United Kingdom
Eija Könönen, University of Turku, Finland
Copyright © 2018 Russo, Bacci, Chiellini, Fagorzi, Niccolai, Taddei, Ricci, Ringressi, Borrelli, Melli, Miloeva, Bechi, Mengoni, Fani and Amedei. This is an open-access article distributed under the terms of the Creative Commons Attribution License (CC BY). The use, distribution or reproduction in other forums is permitted, provided the original author(s) or licensor are credited and that the original publication in this journal is cited, in accordance with accepted academic practice. No use, distribution or reproduction is permitted which does not comply with these terms.
*Correspondence: Amedeo Amedei, YW1lZGVvLmFtZWRlaUB1bmlmaS5pdA==