- 1Key Laboratory of Control Technology and Standard for Agro-product Safety and Quality, Jiangsu Key Laboratory for Food Quality and Safety-State Key Laboratory Cultivation Base of Ministry of Science and Technology, Institute of Food Safety and Nutrition, Jiangsu Academy of Agricultural Sciences, Nanjing, China
- 2Key Laboratory of Veterinary Biological Engineering and Technology, Institute of Veterinary Medicine, Ministry of Agriculture, Jiangsu Academy of Agricultural Sciences, Nanjing, China
- 3Key Laboratory for Animal Genetics, Breeding, Reproduction and Molecular Design of Jiangsu Province, College of Animal Science and Technology, Yangzhou University, Yangzhou, China
Bovine mastitis continues to be a complex disease associated with significant economic loss in dairy industries worldwide. The incidence rate of subclinical mastitis (IRSCM) can show substantial variation among different farms; however, the milk microbiota, which have a direct influence on bovine mammary gland health, have never been associated with the IRSCM. Here, we aimed to use high-throughput DNA sequencing to describe the milk microbiota from two dairy farms with different IRSCMs and to identify the predominant mastitis pathogens along with commensal or potential beneficial bacteria. Our study showed that Klebsiella, Escherichia–Shigella, and Streptococcus were the mastitis-causing pathogens in farm A (with a lower IRSCM), while Streptococcus and Corynebacterium were the mastitis-causing pathogens in farm B (with a higher IRSCM). The relative abundance of all pathogens in farm B (22.12%) was higher than that in farm A (9.82%). However, the genus Bacillus was more prevalent in farm A. These results may be helpful for explaining the lower IRSCM in farm A. Additionally, the gut-associated genera Prevotella, Ruminococcus, Bacteroides, Rikenella, and Alistipes were prevalent in all milk samples, suggesting gut bacteria can be one of the predominant microbial contamination in milk. Moreover, Listeria monocytogenes (a foodborne pathogen) was found to be prevalent in farm A, even though it had a lower IRSCM. Overall, our study showed complex diversity between the milk microbiota in dairy farms with different IRSCMs. This suggests that variation in IRSCMs may not only be determined by the heterogeneity and prevalence of mastitis-causing pathogens but also be associated with potential beneficial bacteria. In the future, milk microbiota should be considered in bovine mammary gland health management. This would be helpful for both the establishment of a targeted mastitis control system and the control of the safety and quality of dairy products.
Introduction
Mastitis, defined as inflammation of the mammary gland, is commonly associated with bacterial infection (McDougall et al., 2009). Bovine mastitis continues to be one of the major veterinary and economic issues that affects dairy industries worldwide (Ruegg, 2017). It is well known that the incidence rate of subclinical mastitis (IRSCM) can show substantial variation among different dairy farms, and the predominant mastitis-causing pathogens can also differ considerably (Olde Riekerink et al., 2008). To develop successful control programs for mastitis, it is very important to identify the predominant mastitis-causing pathogens (Ruegg, 2017). However, there were no bacteria detected by conventional approaches in 20–40% of milk samples from mastitis cases due to the low concentration of bacteria or to the fastidious nutritional and growth requirements (Taponen et al., 2009). In addition, recent studies suggested that mastitis may be associated not only with the mastitis pathogens but also with an imbalance of the milk microbiota (Oikonomou et al., 2012; Kuehn et al., 2013). From another perspective, milk microbiota can directly affect subsequent development of dairy products. Therefore, to establish a targeted mastitis control system and to improve the safety and quality of dairy products, it is of great importance to understand the bacterial community present in milk.
Over the last two decades, methods such as real-time PCR (Katholm et al., 2012; Holmoy et al., 2018), multiplex PCR (mPCR) (Shome et al., 2011), and denaturing gradient gel electrophoresis (DGGE) PCR (Kuang et al., 2009) have been used to identify bacterial DNA in milk samples. In recent years, increasing evidence has shown that sequencing of the 16S rRNA gene can identify almost the entire bacterial community, both commensal and pathogenic, since it can overcome the limitations of the culture-based bacterial detection method (Kennedy et al., 2016). Few studies have been carried out to understand the diversity of microbiota in healthy and mastitic milk samples (Kuehn et al., 2013; Oikonomou et al., 2014; Oultram et al., 2017). Previous studies reported significant differences between the microbiota of milk from healthy and mastitic quarters (Kuehn et al., 2013). Furthermore, samples derived from healthy quarters could be easily discriminated from samples derived from clinical mastitis and culture negative quarters based on their microbiota profiles (Oikonomou et al., 2014). Oultram et al. (2017) reported that 16S rRNA gene sequencing can be used to diagnose clinical mastitis, and Streptococcus uberis and Staphylococcus are identified in most cattle. In addition, sequence-based microbiota analyses were also used to understand the microbial diversity of feces (Oikonomou et al., 2013) and teats (Falentin et al., 2016), to assess the impact of transfer to a milk processing facility (Kable et al., 2016), and to identify possible sources of raw milk contamination (Doyle et al., 2017b). However, no study has been conducted to compare the microbiota in milk samples from different dairy farms, especially from farms with different IRSCMs.
Many studies have been conducted to determine the association between risk factors and the IRSCM; many risk factors, including age of the bovine, body condition score, stage of lactation, mammary regression, management practices, herd housing, milking machine, nutrition, weather and climate have been reported to be associated with the IRSCM (Olde Riekerink et al., 2008; Santman-Berends et al., 2016). However, the milk microbiota, which can have a direct impact on bovine mammary gland health, has never been associated with the IRSCM. Therefore, the specific objectives of this study were to use high-throughput DNA sequencing to investigate the milk microbiota from two dairy farms with different IRSCMs and to identify the predominant mastitis pathogens and the commensal or potential beneficial bacteria.
Materials and Methods
Sample Collection
Milk samples were collected from commercial dairy farms A (66 samples) and B (72 samples), which both located in Nanjing, Jiangsu province, China. The cows were all Holstein dairy cattle and were on twice-daily milking. The IRSCMs of farm A and farm B determined by herd veterinarians were 16.9% (306/1860) and 56.2% (930/1656), respectively. Two milk collections were obtained from 4-year-old cows at 25 and 26 May 2016, separately. Each milk sample was collected from one teat per cow. To exclude the effect of antibiotics on milk microbiota, the milk samples were collected from cows that had not been treated with antibiotics for at least 1 month. The sampling procedure was performed during the afternoon milking according to a previous study (Schukken et al., 2009). Briefly, the bovine teats were thoroughly washed with osmosis water and 70% ethanol and dried using individual paper towels. Then, the first three streams of milk were discarded, and the milk samples (about 30 mL) were collected using 50 mL sterile plastic tubes. In this study, the subclinical mastitis status was evaluated using milk somatic cell counts (SCC) calculated by Fossomatic 5000TM automatic equipment (Foss Electric, Hillerød, Denmark). Subclinical mastitis was suspected when the SCC were greater than 500,000 cells/mL, but with no inflammation of the udder (Shittu et al., 2012; Kulkarni and Kaliwal, 2013). Cows were considered healthy when the SCC were lower than 100,000 cells/mL with no inflammation of the udder (Shittu et al., 2012; Kulkarni and Kaliwal, 2013). The samples were transferred to the laboratory using a mobile refrigerator and stored at -70°C for later analysis. For comparison purposes, 32 milk samples collected from farm A (16 samples from healthy cows and 16 samples from cows with subclinical mastitis) and 32 milk samples collected from farm B (16 samples from healthy cows and 16 samples from cows with subclinical mastitis) were further analyzed.
DNA Extraction and High-Throughput Sequencing
To extract microbial DNA, 1.8 mL of each milk sample was centrifuged at 10000 g for 10 min to generate a pellet from which DNA was extracted using the Powerfood Microbial DNA Isolation kit (Mo Bio Laboratories Inc., Carlsbad, CA, United States) according to the manufacturer’s instructions. The DNA was then used as a template for polymerase chain reaction. The V1–V2 region of the bacterial 16S rRNA genes from each sample was amplified using the universal primer set 27F and 338R (Oikonomou et al., 2014), which contained an 8-base unique barcode that was used to tag the PCR products from each sample. After the quantification, qualification and purification of the PCR products, a sequencing library was generated using NEB Next® UltraTM DNA Library Prep Kit for Illumina (NEB, Ipswich, MA, United States). Finally, the library was sequenced using an Illumina HiSeq 2000 system (Illumina, Inc., San Diego, CA, United States), and 300 bp paired-end reads were generated. The reads were deposited in the National Center for Biotechnology Information (NCBI) Sequence Read Archive (SRA) with accession number SRP149195.
Bioinformatic Analysis
Paired-end reads were merged using FLASH (Magoc and Salzberg, 2011) and the effective reads were obtained after removing the chimeric sequences (Edgar et al., 2011). Sequences were analyzed by the UPARSE software package using the UPARSE-OTU and UPARSE-OUT ref algorithms (Edgar, 2013) and were assigned to the same operational taxonomic units (OTUs) with ≥97% similarity. The representative sequence for each OTU was selected and annotated for taxonomic information using QIIME (Caporaso et al., 2010) and the Ribosomal Database Project classifier (Cole et al., 2009). To facilitate comparison, the milk samples were classified into eight groups according to their origin: group AM (16 samples from mastitic cows in farm A), group AH (16 samples from healthy cows in farm A), group BM (16 samples from mastitic cows in farm B), group BH (16 samples from healthy cows in farm B), group A (which consisted of groups AM and AH), group B (which consisted of groups AH and BH). The number of obtained reads and the percent of the sequence that could be annotated for different taxonomic levels was calculated for each group. Subsequently, the ten most abundant microbial phyla and the predominant genera whose abundance was higher than 0.5% in at least one group were identified. A two-way hierarchically clustered heatmap of the bacterial distribution in different milk samples was conducted using ClustVis based on average linkage clustering and Euclidean distance (Metsalu and Vilo, 2015). To calculate the genus diversity within individual groups, Perl scripts packaged in QIIME (Caporaso et al., 2010) were used to analyze the alpha diversity (Shannon index). To compare similarities within the whole community, principal component analysis (PCA) based on weighted UniFrac distances was also conducted using Perl scripts packaged in QIIME (Caporaso et al., 2010). Linear discriminant analysis (LDA) effect size (LEfSe) (Segata et al., 2011) was used to assess the microbial compositional differences between the two groups of samples at the genus or higher taxonomic level. The Spearman’s correlation coefficient values of each pair of predominant genera were calculated using SPSS Statics (version 22.0, SPSS Inc.). To reduce false-positives caused by excessive mutual exclusions, the genera that existed in less than half of the milk samples were removed (Li et al., 2015). The co-occurrence network analysis and visualization were conducted by the Gephi open source graph visualization software tool (version 0.9.2), using the force-directed algorithm ForceAtlas2 (Bastian et al., 2009).
Statistical Analysis
Data were collected and analyzed using SPSS Statics (version 22.0, SPSS Inc., Chicago, IL, United States). The diversity among different groups was analyzed by analysis of variance (ANOVA), followed by Tukey’s and Dunnett’s T3 multiple comparison tests. In these analyses, P < 0.05 was considered to be statistically significant, while P < 0.01 was considered to be an extremely significant difference.
Results
Sequencing Results
The pyrosequencing of milk samples generated a total of 2,585,190 reads, of which 2,396,471 (92.7%) effective reads were ultimately analyzed by the RDP classifier after exclusion due to trimming and quality control. The number of effective reads per sample ranged from 30,693 to 44,837 (median 38,358; mean 38,089). The milk samples were classified into groups A, B, M, H, AM, AH, BM, and BH according to their origin. As shown in Figure 1A, the violin plots showed the distribution of the number of effective reads in each group. The medians of the number of effective reads in group A, B, M, H, AM, AH, BM, and BH were 37767, 39553, 38577, 37585, 38227, 36982, 39577, and 39274, respectively. No significant differences were observed among the number of effective reads of different groups. These data demonstrate that the relative abundance could be used in the following study. As shown in Figure 1B, more than 97.5% of the sequences in each group could be assigned to the level of phyla, class, order and family. Additionally, more than 85.4% of the sequences in each group could be assigned to the level of genus, and the percentage of sequences assigned to the level of species ranged from 31.6 to 36.8%.
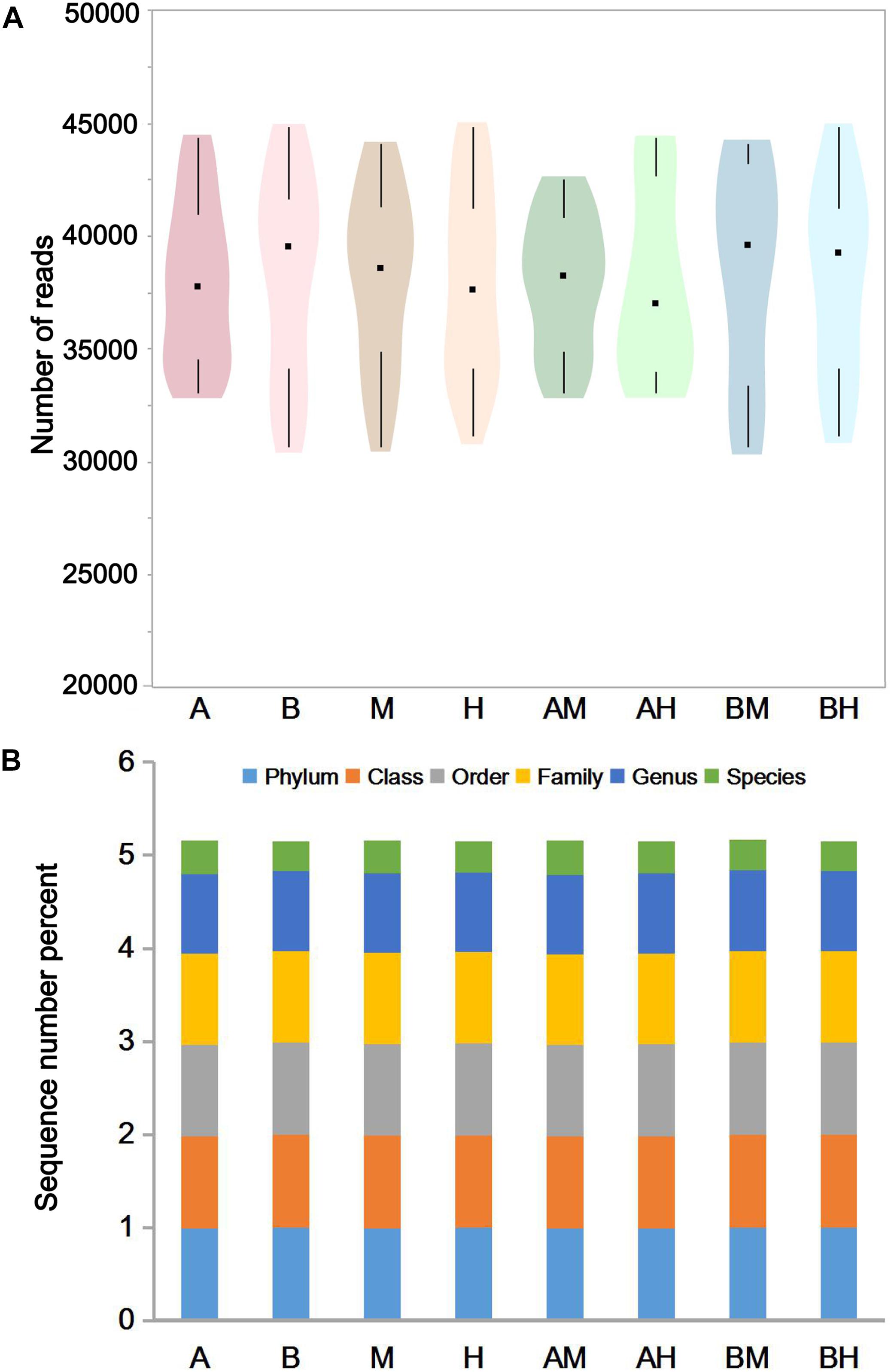
FIGURE 1. The number of effective reads in each group (A) and taxonomic classification of the effective reads at different levels (B). Violin plots show the distribution of the number of effective reads in each group, and the black points represent the medians of the number of effective reads (A). The colors represent the sequence number percent of effective reads annotated to this taxonomic level (B).
Microbiota of Milk Samples
The 10 most abundant microbial phyla (average abundance > 0.1%) are shown in Figure 2A. For each group, Proteobacteria was the major phylum with a prevalence that ranged from 39.96 to 48.30%, followed by Firmicutes (30.25–40.28%), Bacteroidetes (8.38–12.21%), and Actinobacteria (5.17–11.29%). All four phyla above were observed in all milk samples. At the genus level, a genus with a relative abundance higher than 0.5% in at least one group was defined as the predominant genus. We observed a total of 32 predominant genera as shown in Figure 2B. It was found that the prevalent genera were diverse in different groups. The six most prevalent microbial genera of group A were Halomonas, Ruminococcus, Listeria, Klebsiella, Escherichia–Shigella, and Acinetobacter, while the six most prevalent microbial genera of group B were Streptococcus, Halomonas, Atopostipes, Corynebacterium, Ruminococcus, and Comamonas. Some genera, such as Halomonas and Ruminococcus were prevalent in both groups A and B. When the samples were classified into groups M and H, our analysis demonstrated that Streptococcus, Halomonas, and Corynebacterium were the most abundant genera in group M, while Halomonas, Acinetobacter, and Atopostipes were the most abundant genera in group H. It was also noted that Halomonas, Acinetobacter, and Atopostipes were the most prevalent genera in group AM, while Streptococcus, Halomonas, and Corynebacterium were the most prevalent genera in group BM.
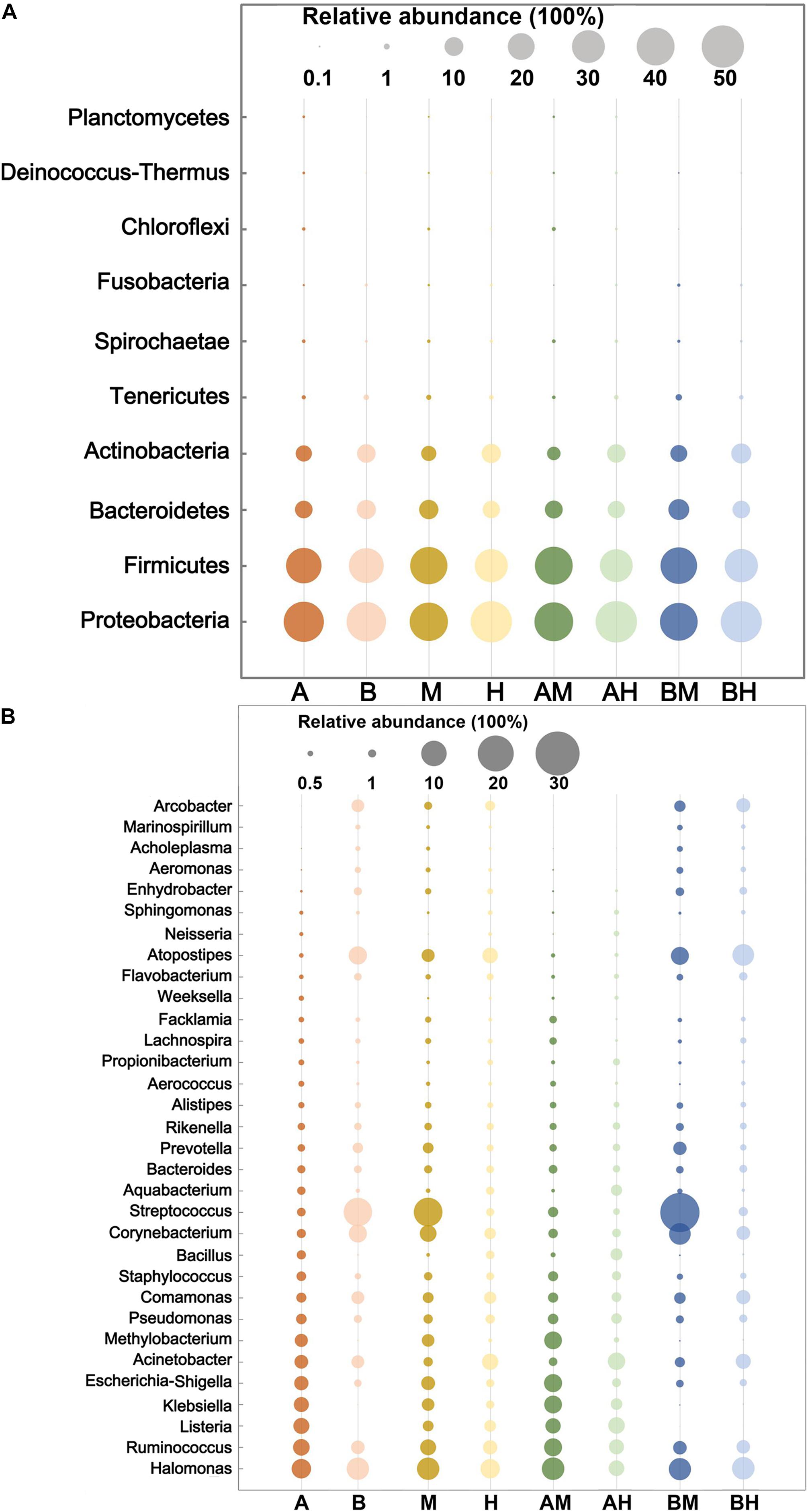
FIGURE 2. The 10 most abundant microbial phyla (A) and 32 genera with an abundance higher than 0.5% in at least one group (B). The size of the circles indicate the relative abundances of the phylum (A) or genus (B) in each group.
The hierarchically clustered heatmap of microbial profiles of each milk sample was generated (Figure 3). The prevalence of microbial genera in different samples was diverse. Some mastitic milk samples such as AM7, AM14, and BM13 were dominated by one genus, while others, especially the healthy milk samples including AH14, AH16, and BH15, showed a more balanced profile. Our results showed that 17 milk samples from group A and 23 milk samples from group B formed two distinct cluster (clades I and II), while the milk samples from groups M and H could not be completely separated. The milk samples of groups AM and AH and of groups BM and BH were also largely indistinguishable. This result suggested the microbial profiles were more similar in the milk samples collected from the same dairy farm.
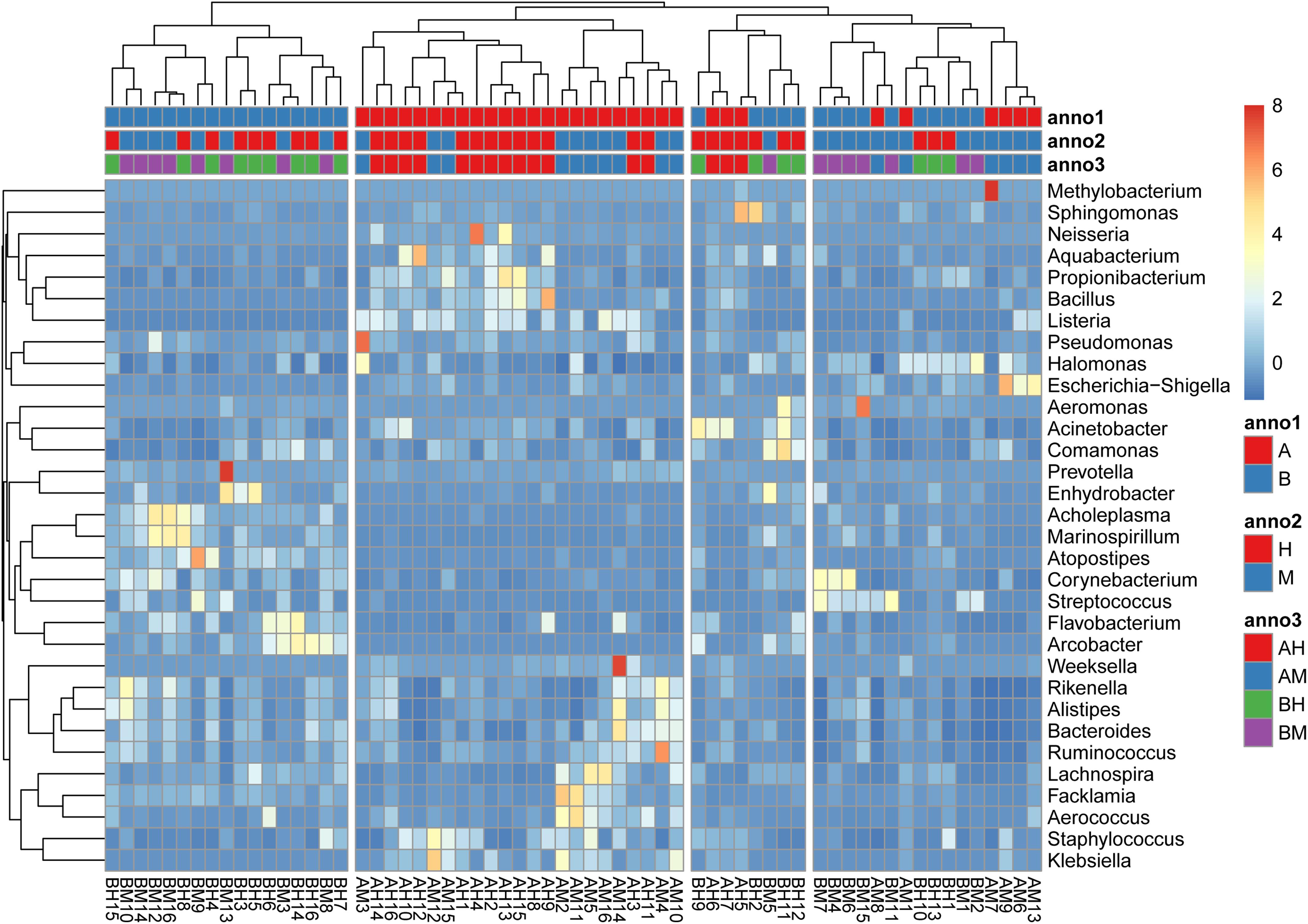
FIGURE 3. A hierarchically clustered heatmap of the microbial profiles of each milk sample. Annotations at the top of the heatmap show the clustering of milk samples. The dual hierarchical dendrogram shows the distribution of bacteria based on average linkage clustering and Euclidean distance. The color scale depicts the normalized relative abundance of each genus.
Alpha and Beta Diversities of Milk Microbiota
Alpha diversity can be used to determine the microbial diversity of a given sample (Bailey et al., 2013). To analyze the microbial diversity of each milk sample, the Shannon index, which reflects the species richness and evenness, was analyzed. The violin plots showed the distribution of the Shannon indices of milk samples in each group (Figure 4A). We detected no significant difference between the Shannon indices of groups A and B. However, the Shannon index of group M was significantly lower than that of group H. Additionally, similar trends were found between groups AM and AH and between groups BM and BH. These results indicated that the microbial diversity of healthy groups was higher than that of the mastitis groups. Beta diversity can determine the microbial diversity between different samples (Bailey et al., 2013). To compare whole microbial composition similarities, PCA was conducted using genus-level taxonomic profiles. As shown in Figure 4B, the clustering of the milk samples based on their microbiota made it possible to separate samples from groups A and B. However, there was no clear separation of milk samples from groups M and H within the PCA plots. The distribution of the milk samples from group AM revealed an overlap with group AH, and a similar result was found between groups BM and BH.
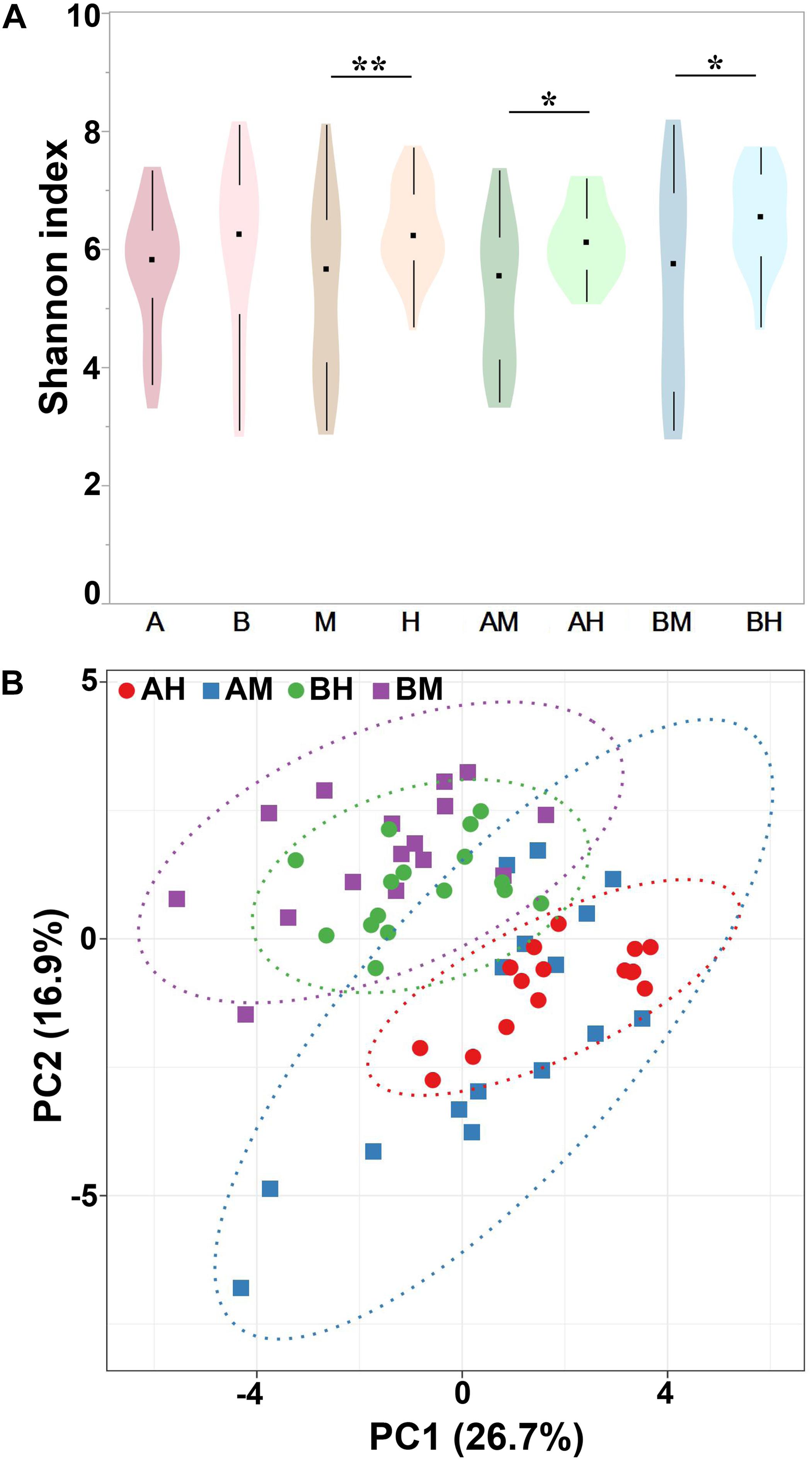
FIGURE 4. Shannon index of different groups (A) and PCA analysis of each milk sample (B). Violin plots show the distribution of the Shannon indices of milk samples in each group, and the black points represent the medians of the Shannon indices of milk samples. ∗P < 0.05, ∗∗P < 0.01 indicates a significant difference compared between the Shannon indices of two groups.
Compositional Differences Between Different Groups
To identify differences in microbial composition of milk samples between different groups, LEfSe was used to provide biomarkers at the genus-level with a linear discriminant analyses (LDA) score > 3 (P < 0.05). As shown in Figure 5A, 15 genera were statistically significantly different between group A and group B. Six genera (Listeria, Klebsiella, Escherichia–Shigella, Methylobacterium, Bacillus, and Weeksella) were significantly enriched in group A, while nine genera (Streptococcus, Atopostipes, Corynebacterium, Arcobacter, Flavobacterium, Enhydrobacter, Aeromonas, Marinospirillum, and Acholeplasma) were significantly enriched in group B. This result indicates that nearly half of the predominant bacterial genera were different between the two dairy farms. The comparison between groups M and H showed that Acinetobacter were more prevalent in group H, while Streptococcus were more prevalent in group M (Figure 5B).
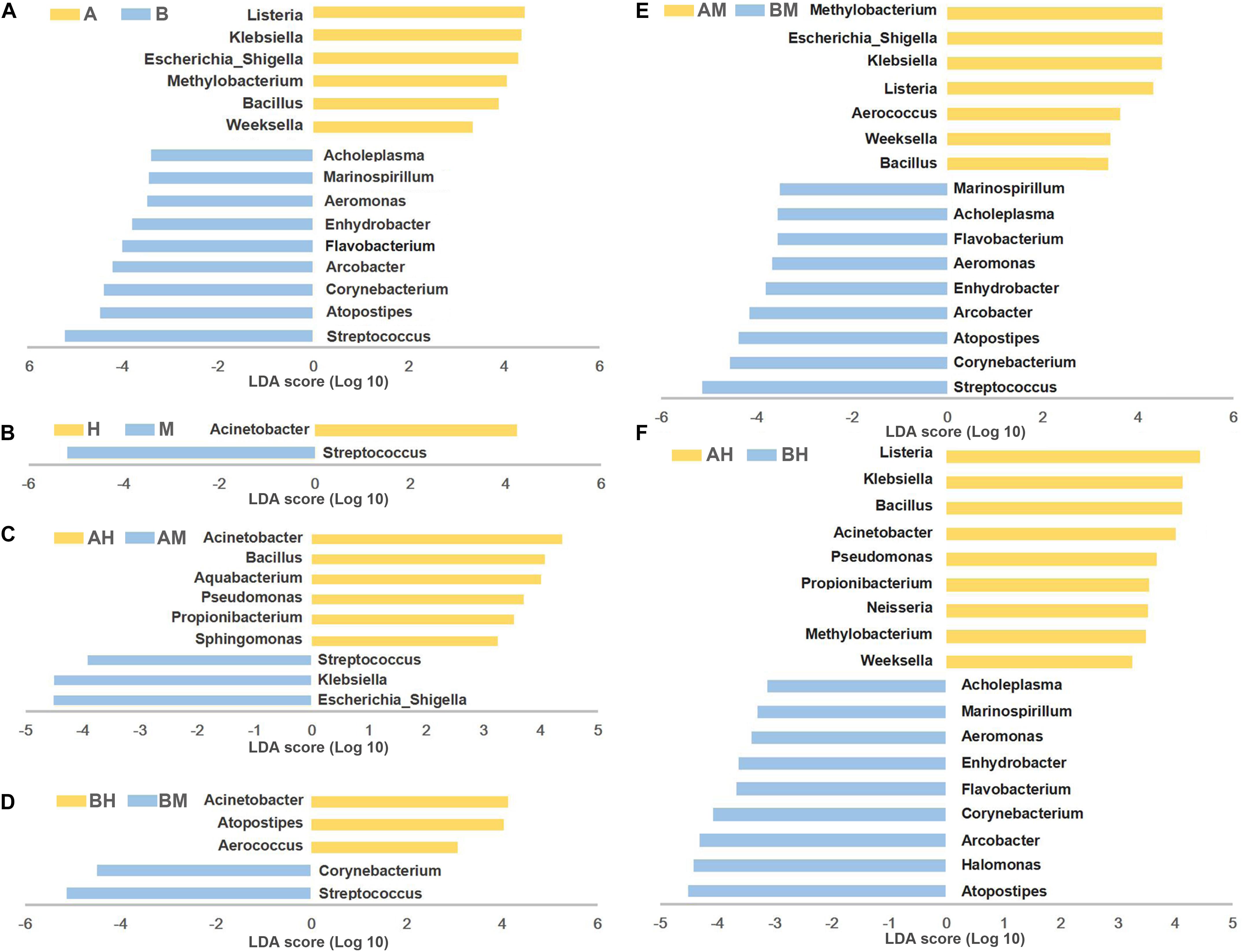
FIGURE 5. LEfSe analysis of microbiota. The microbial genera with significant differences in relative abundance compared between two groups are coded in blue and yellow as indicated in (A–F). Only the genera with an LDA score > 3 (P < 0.05) are depicted.
To identify the biomarkers between the bacterial composition of the healthy and mastitic milk samples obtained from the same dairy farm, the comparisons were also performed between groups AM and AH (Figure 5C) and between groups BM and BH (Figure 5D). Our results showed that three genera (Escherichia–Shigella, Klebsiella, and Streptococcus) were identified as biomarkers in group AM, while six genera (Acinetobacter, Bacillus, Aquabacterium, Pseudomonas, Propionibacterium, and Sphingomonas) were identified as biomarkers in group AH. The comparison between groups BM and BH showed that Acinetobacter, Atopostipes, and Aerococcus were more enriched in group BH, while Streptococcus and Corynebacterium were more enriched in group BM. Additionally, the comparisons between groups AM and BM and between groups AH and BH were also performed. As shown in Figures 5E,F, the different genera identified between groups AM and BM and between groups AH and BH were similar to the different genera identified between groups A and B.
Co-occurrence Network Analysis
The co-occurrence network analysis was applied in order to obtain a view of the potential relationships among predominant bacterial genera in milk samples. As shown in Figure 6, the co-occurrence network consisted of 20 nodes and 32 edges, with 27 positive and 5 negative correlation. Interestingly, five gut-associated genera (Prevotella, Ruminococcus, Bacteroides, Rikenella, and Alistipes) displayed positive correlation coefficients with each other, and each individual pairing between two genera showed a strong correlation (Spearman’s correlation coefficient value > 0.8), suggesting that these genera are likely to share a symbiotic or syntrophic relationship. It was also observed that the occurrence of Bacillus was negatively correlated with the occurrence of Staphylococcus and Comamonas, while the presence of Weeksella was negatively correlated with the presence of Aeromonas, Comamonas, and Atopostipes. Notably, although the co-occurrence network shed light on the complex relationships of bovine milk microbiota, empirical evidences are needed to support their natural presence.
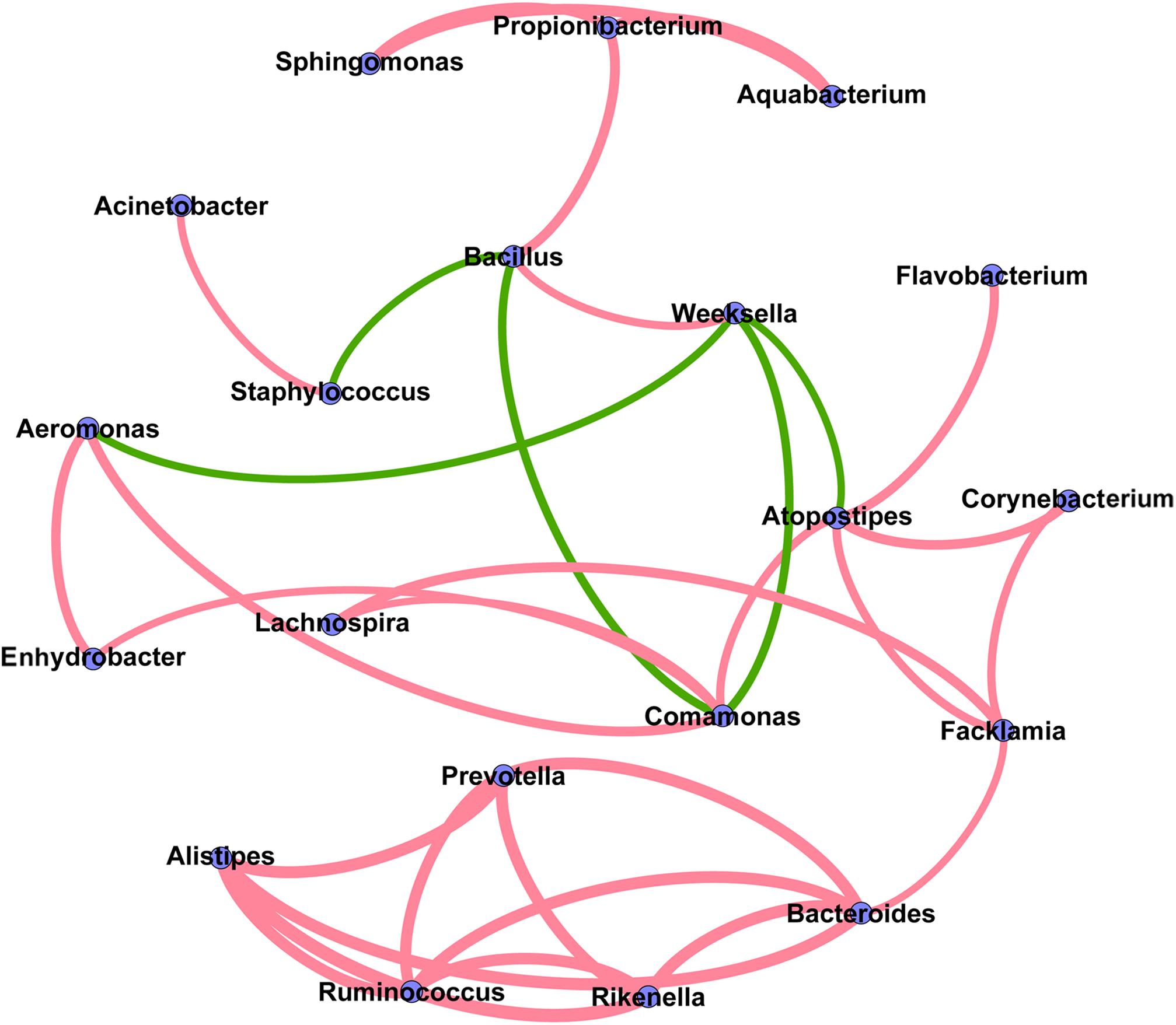
FIGURE 6. Co-occurrence network of the predominant bacterial genera in the milk samples. Each node represents one genus, and each pair of genera connected by the line has a calculated Spearman’s correlation coefficient value > 0.5 or <–0.5 (P-value < 0.05). Red connecting lines represent the positive significant correlations and green lines represent the negative significant correlations. The thickness of each connecting line between two nodes (edge) is proportional to the absolute value of Spearman’s correlation coefficient.
Discussion
Bovine mastitis is a highly prevalent disease in dairy herds and it is arguably the most important disease that affects the dairy industry worldwide (Ruegg, 2017). Here, we used high-throughput 16S rRNA gene sequencing to analyze the bacterial community of milk samples collected from two dairy farms with different IRSCMs. Over the past decade, different hypervariable (V) regions of 16S rRNA including V1–V2, V1–V3, V3, V3–V4, V3–V5, V4, V4–V5, V5, V5–V6, V5–V9, V6, and V6–V8 have been sequenced to describe the microbial community (Zhang et al., 2018). For bovine milk, V1–V2 region was used frequently to describe the bacterial diversity (Oikonomou et al., 2012, 2014; Kuehn et al., 2013; Rodrigues et al., 2017). It is recognized that the selection of V regions can affect estimates on OTU richness and diversity, and the use of different V regions means that results are often not directly comparable, diminishing the value of inferences that can be drawn (Sperling et al., 2017). Therefore, to compare with the bovine milk microbiota described in previous study (Oikonomou et al., 2012, 2014; Kuehn et al., 2013; Rodrigues et al., 2017), the V1–V2 region was selected to sequence in this study. However, as has been suggested by Sperling et al. (2017), more than one marker region is needed to provide more reliable inferences in the future study.
Contrary to previous studies that only focus on the comparison between microbial composition of healthy and mastitis milk samples (Oikonomou et al., 2012, 2014; Kuehn et al., 2013), we also focused on the microbial diversity between different farms. Our study revealed strong variation between the microbiota of milk samples, with some samples clearly dominated by one genus, whereas others displayed a more balanced profile. It was also noted that the milk samples collected from two farms (A and B) could be clearly separated, while there was no clear separation of healthy and mastitis samples collected from the same farm. However, the discriminant analysis made it possible to identify the genus markers between different groups.
In this study, one of our aims was to compare the microbial community of milk samples collected from farms A and B. There were six genera more prevalent in group A and nine genera more prevalent in group B. Among the six genera more prevalent in group A, Klebsiella and Escherichia–Shigella were more enriched in group AM compared to group AH, while Bacillus was more enriched in group AH. The relative abundance of Listeria, Methylobacterium, and Weeksella showed no significant difference between groups AH and AM. Both Klebsiella and Escherichia–Shigella (particularly Escherichia coli), have been recognized as the pathogens that cause environmental bovine mastitis, which is caused by pathogens present in the digestive tract of cows or their surroundings (Schukken et al., 2012). Contrarily, Bacillus was found to be more prevalent in group AH than group AM. In addition, our co-occurrence analysis showed there was a negative correlation between Bacillus and Staphylococcus, although this correlation had a weak coefficient value (0.51). These results suggest that Bacillus may contribute to the overall health of cows, however, which Bacillus species would be beneficial and its role warrants further investigation.
Although the 16S rRNA sequencing in our study was not insufficient for accurate taxonomic assignment at the species level, the percentage of sequences assigned to the level of species could range from 31.6 to 36.8% in different group. Our result showed that the sequences, which were assigned to Listeria at the genus level, were all assigned to Listeria monocytogenes at the species level. To determine which kind of Listeria species were present in farm A, we tried to isolate Listeria from 156 milk samples newly collected from farm A according to the previous study (Osman et al., 2016). Finally, 16 L. monocytogenes strains (positive rate 10.26%), but no L. innocua and L. ivanovii, were isolated from farm A. Thus, the Listeria sequences obtained from farm A in this study should be classified as L. monocytogenes at the species level. Bovine mastitis caused by L. monocytogenes is rare (Osman et al., 2016), in addition, the cases of short-live excretion of L. monocytogenes bacteria in milk samples do not show any symptoms, and cases of prolonged mastitis due to Listeria are not reported (Winter et al., 2004). However, L. monocytogenes has been involved in several outbreaks of listeriosis, occurring after consumption of contaminated milk and milk products worldwide (El Marnissi et al., 2016). Our study indicated that cows can be healthy reservoirs of L. monocytogenes, thus, there is a need for continued surveillance for the presence of Listeria in bovine milk. The Methylobacterium genus includes a group of strictly aerobic, Gram-negative bacteria which are ubiquitous and detected in soil, freshwater, and lake sediments (Bracke et al., 2014). These bacteria have been reported to cause opportunistic infections in immunocompromised hosts (Sanders et al., 2000). Recently, Methylobacterium was also observed as a contaminant in milk samples (Bracke et al., 2014), but no reports have shown a link to bovine mastitis. The genus Weeksella includes only one species, Weeksella virosa, that has been isolated from human clinical specimens (Sankar et al., 2015). Slenker et al. (2012) also described a fatal case of W. virosa sepsis in a young female with end-stage renal disease. However, similar to Methylobacterium, no research studies have reported a link between Weeksella and bovine mastitis.
Among the nine predominant genera more prevalent in group B, the relative abundances of Streptococcus and Corynebacterium were significantly higher in group BM, while Atopostipes was significantly higher in group BH. The relative abundances of six other genera (Arcobacter, Flavobacterium, Enhydrobacter, Aeromonas, Marinospirillum, and Acholeplasma) were similar between groups BH and BM. The Streptococcus genera, including Streptococcus agalactiae, Streptococcus uberis, and Streptococcus dysgalactiae, are well-known mastitis-causing pathogens (Klaas and Zadoks, 2017; Pang et al., 2017). Corynebacterium has also been identified as the pathogen associated with mastitis in dairy cows, often being described as contagious (Oultram et al., 2017). Previous studies reported that Corynebacterium could be detected in bulk tank milk samples collected from 894 China dairy herds at a frequency of 17.0% (Bi et al., 2016) and from 1242 dairy cows in Brazil at a frequency of 22.9% (Goncalves et al., 2016). In addition, in the microbiome of bulk tank milk, Streptococcus and Corynebacterium were encountered in significantly higher relative abundances in the HSCC (high somatic cell count) group when compared with the LSCC (low somatic cell count) (Rodrigues et al., 2017). Thus, we speculated that Streptococcus and Corynebacterium may have been responsible for the higher IRSCM in farm B.
In previous studies, Atopostipes isolated from pig manure was proposed as a new genus in 2004 (Samanta et al., 2015) and has recently been identified as a pig-specific fecal indicator (Jeong et al., 2011). Atopostipes is also found in the microbial community of the outer udder skin of calves (Yeoman et al., 2018). In addition, Atopostipes was exclusively present in feces and intestinal tissue of EAE (experimental autoimmune encephalomyelitis)-resistant rats (Stanisavljevic et al., 2016). However, Atopostipes has never been linked to bovine health or the safety of milk. During recent years, Arcobacter has emerged as an important foodborne and waterborne zoonotic pathogen worldwide and has been classified as a serious hazard to human health (Ramees et al., 2017). The presence of Arcobacter has been observed in cow milk (Yesilmen et al., 2014), cow feces (Van Driessche et al., 2005), and in milk filters in a water buffalo dairy farm in Italy (Serraino et al., 2013). In addition, Arcobacter has been isolated from a dairy herd that underwent an outbreak of mastitis (Logan et al., 1982). However, although Arcobacter has been associated with reproduction disorders and mastitis in livestock, it has also been isolated from healthy animals frequently (De Smet et al., 2011). Similarly, our results showed that Arcobacter was prevalent in farm B, but there was no significant difference between groups BM and BH.
Except for the genera discussed above, our study found no significant difference in the prevalence of 17 predominant genera between the two farms. Interestingly, five predominant genera (Prevotella, Ruminococcus, Bacteroides, Rikenella, and Alistipes), which were prevalent in all milk samples, were all typically gut-associated genera (Huws et al., 2011). Previous studies have also shown that Prevotella (Oikonomou et al., 2012, 2014), Ruminococcus (Oikonomou et al., 2012, 2014; Kable et al., 2016; Oultram et al., 2017), Bacteroides (Oikonomou et al., 2012, 2014; Oultram et al., 2017), and Alistipes (Gschwendtner et al., 2016) could be detected in cow milk. In addition, our study showed these five genera had strong positive correlation coefficients with each other. These findings suggest that gut bacteria can be one of the predominant microbial contamination in milk. The gut bacteria in milk can be contaminated from the herd feces (Doyle et al., 2017b). Alternatively, as described by several authors (Addis et al., 2016), the gut bacteria would also reach the mammary gland through an endogenous entero-mammary pathway. However, these gut-associated genera may not contribute to the appearance of bovine mastitis since there were no significant differences between their relative abundances in each group. Halomonas was identified as the most prevalent genus in both farms A and B. Halomonas, as a negative bacterium, has been detected in Danish raw milk cheeses (Masoud et al., 2011) and is found to be the most frequent OTU in short-ripened cheeses (Schornsteiner et al., 2014). As speculated by Ishikawa et al., Halomonas in dairy products originated in marine environments and was introduced via the sea salt added to cheese surfaces during washing and dry salting (Ishikawa et al., 2007). However, our study indicated that Halomonas is a frequent contaminant of milk microbiota, thus, the Halomonas in dairy products may come directly from raw milk.
In this study, we also focused on the bacterial genera which were more prevalent in group M and group H to identify the mastitis-associated pathogens and the potential beneficial bacteria present in both dairy farms. Streptococcus was identified as the core mastitis-associated pathogen in both farms A and B, while Acinetobacter was more prevalent in group H. In the assessment of the human milk microbial community, healthy controls possessed relatively more Acinetobacter in comparison to the mastitic group (Patel et al., 2017). It was also reported that Acinetobacter is a member of the core milk microbiota (Kable et al., 2016) and is frequently detected in raw milk (Quigley et al., 2013). Although the role of Acinetobacter in milk is still unknown, it is well documented that Acinetobacter is frequently associated with antibiotic resistance and human clinical infections (Dadar et al., 2012). Recently, it has been shown that Acinetobacter strains isolated from raw milk also exhibited antibiotic resistance (Gurung et al., 2013). Thus, although Acinetobacter may be important for the healthy status of cows, it is needed to examine the antibiotic resistance and genetic characteristics of Acinetobacter strains in milk samples since they could be a public health concern.
Additionally, the microbial diversity was also compared between groups AH and AM, and between groups BH and BM. Our study indicated that Klebsiella, Escherichia–Shigella, and Streptococcus were the pathogens that caused mastitis in farm A, while Streptococcus and Corynebacterium were the mastitis-causing pathogens in farm B. These genera are all well-recognized mastitis pathogens. It was also observed that Propionibacterium was identified as a biomarker in group AH. Previous studies have shown that Propionibacterium was present in all milk samples obtained from healthy quarters of bovine (Oikonomou et al., 2014), and the abundance of Propionibacterium is negatively correlated with the total bacterial count in cow milk (Li et al., 2018). In addition, Propionibacterium was only identified in the teats of non-infected quarters of dairy cows by denaturing gradient gel electrophoresis (DGGE) (Braem et al., 2012). These studies indicate that Propionibacterium may be potential beneficial bacteria for cows and warrant further investigation into its role in milk.
In our study, it became apparent that the 16S rRNA gene sequencing approach can be very helpful for elucidating the microbiota of milk. In conclusion, our study found that the relative abundances of the pathogens Streptococcus and Corynebacterium in farm B were 15.74 and 6.38%, respectively, while the relative abundances of pathogens Klebsiella, Escherichia–Shigella, and Streptococcus in farm A were 4.41, 3.95, and 1.46%, respectively. The relative abundance of all pathogens in farm B (22.12%) was higher than that of all pathogens in farm A (9.82%). Of note, although Streptococcus was present in both farms A and B, the relative abundance of Streptococcus in farm B (15.74%) was significantly higher than that in farm A (1.46%). In contrast, the genera Bacillus were more enriched in both groups A and AH. These results may helpful for explaining the lower IRSCMs in farm A compared to farm B. Additionally, the genera including Halomonas and the gut-associated genera Prevotella, Ruminococcus, Bacteroides, Rikenella, and Alistipes were identified as the commensal bacteria prevalent in all milk samples. Moreover, we also found that L. monocytogenes was enriched in group A, suggesting there is a need for surveillance of the milk microbiota.
Admittedly, while 16S rRNA gene sequencing is a powerful approach to describe bacterial community and leads to the discovery of many unexpected evolutionary lineages, certain limitations do have to be considered (Salter et al., 2014; Oultram et al., 2017). These limitations, including choices relating to sample collection, sample storage and preservation, DNA extraction method, contaminating microbial, amplifying primers, sequencing technology, read length and depth and bioinformatics analysis techniques, can affect the OTU richness and diversity (Salter et al., 2014). However, to overcome these limitations, many efforts have been made to evaluate the influence of sampling technique (Metzger et al., 2018), sample storage condition (Doyle et al., 2017a) and DNA extraction methods (Lima et al., 2018), design new amplifying primers (Bahram et al., 2018), remove bacterial DNA contamination (Karstens et al., 2018), develop sequencing technology (Tedersoo et al., 2018) and bioinformatic analysis techniques (Gasc and Peyret, 2018). Recently, the full-length 16S rRNA sequencing technology (Pootakham et al., 2017) and method (Fuks et al., 2018) have been demonstrated to be useful for the accurate classification of the bacterial community composition at the species level. As 16S rRNA sequencing becomes cheaper and faster toperform, and with new technology developing, it is likely to become a cost-effective and more reliable approach to determine microbial community.
Overall, our study revealed complex diversity between the microbial communities of dairy farms with different IRSCMs. This suggests that varying IRSCMs may not only be determined by the heterogeneity and prevalence of mastitis-causing pathogens but also related to the potential beneficial bacteria. Further studies could therefore aim to sequence more samples collected from more dairy farms with different IRSCMs to determine the relationship between milk microbiota and IRSCMs. Here, we encourage that the milk microbiota be examined for bovine mammary gland health management, which can provide complementary information such as the raw milk microbial ecology, and the detection of fastidious bacteria and polymicrobial infections. The metataxonomic approach, which could be combined with the bacterial culture or targeted specific real-time PCR, would not only be helpful for establishment of a targeted mastitis control system but also for the control of the safety and quality of dairy products. This would not only be helpful for establishment of a targeted mastitis control system but also for the control of the safety and quality of dairy products.
Ethics Statement
The sampling method was approved by the Animal Care and Ethics Committee of Jiangsu Academy of Agricultural Sciences (SYXK2015-0020).
Author Contributions
MP and RaW designed the experiments. MP, HB, LS, TH, HaZ, YZ, HuZ, LZ, and RuW performed the experiments. MP, XX, LS, and TH analyzed the data. MP, XX, and RaW drafted the manuscript. KX and RaW coordination of research. All authors read and approved the final manuscript.
Funding
This work was supported by Natural Science Foundation of Jiangsu Province (BK20170600, BK20180297, BK20160585, and BK20161373), Autonomic Research of Jiangsu Key Laboratory for Food Quality and Safety-State Key Laboratory Cultivation Base of Ministry of Science and Technology (028074911709), Foundation of Jiangsu Academy of Agricultural Sciences (028046111673), National Natural Science Foundation of China (NSFC31701725 and NSFC31602078), and National Agricultural Product Quality and Safety Risk Assessment (GJFP201801203 and GJFP201800703).
Conflict of Interest Statement
The authors declare that the research was conducted in the absence of any commercial or financial relationships that could be construed as a potential conflict of interest.
Acknowledgments
The authors thank Jiangsu Collaborative Innovation Center of Meat Production and Processing, Quality and Safety Control, Nanjing, China. They also thank Dr. Jialiang Yang, Icahn School of Medicine at Mount Sinai, for providing help on bioinformatic analysis.
References
Addis, M. F., Tanca, A., Uzzau, S., Oikonomou, G., Bicalho, R. C., and Moroni, P. (2016). The bovine milk microbiota: insights and perspectives from -omics studies. Mol. Biosyst. 12, 2359–2372. doi: 10.1039/c6mb00217j
Kulkarni, A. G., and Kaliwal, B. B. (2013). Bovine mastitis: a review. Int. J. Recent Sci. Res. 4, 543–548.
Bahram, M., Anslan, S., Hildebrand, F., Bork, P., and Tedersoo, L. (2018). Newly designed 16S rRNA metabarcoding primers amplify diverse and novel archaeal taxa from the environment. Environ. Microbiol. Rep. doi: 10.1111/1758-2229.12684 [Epub ahead of print].
Bailey, V. L., Fansler, S. J., Stegen, J. C., and McCue, L. A. (2013). Linking microbial community structure to beta-glucosidic function in soil aggregates. ISME J. 7, 2044–2053. doi: 10.1038/ismej.2013.87
Bastian, M., Heymann, S., and Jacomy, M. (2009). “Gephi: an open source software for exploring and manipulating networks,” in Proceedings of the Third International ICWSM Conference, San Jose, CA.
Bi, Y., Wang, Y. J., Qin, Y., Guix Vallverdu, R., Maldonado Garcia, J., Sun, W., et al. (2016). Prevalence of bovine mastitis pathogens in bulk tank milk in China. PLoS One 11:e0155621. doi: 10.1371/journal.pone.0155621
Bracke, N., Van Poucke, M., Baert, B., Wynendaele, E., De Bels, L., Den Broeck, W. V., et al. (2014). Identification of a microscopically selected microorganism in milk samples. J. Dairy Sci. 97, 609–615. doi: 10.3168/jds.2013-6932
Braem, G., De Vliegher, S., Verbist, B., Heyndrickx, M., Leroy, F., and De Vuyst, L. (2012). Culture-independent exploration of the teat apex microbiota of dairy cows reveals a wide bacterial species diversity. Vet. Microbiol. 157, 383–390. doi: 10.1016/j.vetmic.2011.12.031
Caporaso, J. G., Kuczynski, J., Stombaugh, J., Bittinger, K., Bushman, F. D., Costello, E. K., et al. (2010). QIIME allows analysis of high-throughput community sequencing data. Nat. Methods 7, 335–336. doi: 10.1038/nmeth.f.303
Cole, J. R., Wang, Q., Cardenas, E., Fish, J., Chai, B., Farris, R. J., et al. (2009). The Ribosomal Database Project: improved alignments and new tools for rRNA analysis. Nucleic Acids Res. 37, D141–D145. doi: 10.1093/nar/gkn879
Dadar, M., Adel, M., and Zorriehzahra, J. (2012). “Emerging of a new antibiotic resistance bacteria pathogen, Acinetobacter lwoffii in water environments and their pathogenic effects,” in Proceedings of the 17th International and Iranian Congress of Microbiology, Tehran.
De Smet, S., De Zutter, L., and Houf, K. (2011). Small ruminants as carriers of the emerging foodborne pathogen Arcobacter on small and medium farms. Small Rumin. Res. 97, 124–129. doi: 10.1016/j.smallrumres.2011.02.004
Doyle, C. J., Gleeson, D., O’Toole, P. W., and Cotter, P. D. (2017a). High-throughput metataxonomic characterization of the raw milk microbiota identifies changes reflecting lactation stage and storage conditions. Int. J. Food Microbiol. 255, 1–6. doi: 10.1016/j.ijfoodmicro.2017.05.019
Doyle, C. J., Gleeson, D., O’Toole, P. W., and Cotter, P. D. (2017b). Impacts of seasonal housing and teat preparation on raw milk microbiota: a high-throughput sequencing study. Appl. Environ. Microbiol. 83:e02694-16. doi: 10.1128/AEM.02694-16
Edgar, R. C. (2013). UPARSE: highly accurate OTU sequences from microbial amplicon reads. Nat. Methods 10, 996–998. doi: 10.1038/nmeth.2604
Edgar, R. C., Haas, B. J., Clemente, J. C., Quince, C., and Knight, R. (2011). UCHIME improves sensitivity and speed of chimera detection. Bioinformatics 27, 2194–2200. doi: 10.1093/bioinformatics/btr381
El Marnissi, B., Belkhou, R., and Bennani, L. (2016). Study of Listeria monocytogenes contamination in raw milk and some Moroccan traditionaldairy derivatives (Lben andJben). Int. J. Infect. Dis. 45:277. doi: 10.1016/j.ijid.2016.02.614
Falentin, H., Rault, L., Nicolas, A., Bouchard, D. S., Lassalas, J., Lamberton, P., et al. (2016). Bovine teat microbiome analysis revealed reduced alpha diversity and significant changes in taxonomic profiles in quarters with a history of mastitis. Front. Microbiol. 7:480. doi: 10.3389/fmicb.2016.00480
Fuks, G., Elgart, M., Amir, A., Zeisel, A., Turnbaugh, P. J., Soen, Y., et al. (2018). Combining 16S rRNA gene variable regions enables high-resolution microbial community profiling. Microbiome 6:17. doi: 10.1186/s40168-017-0396-x
Gasc, C., and Peyret, P. (2018). Hybridization capture reveals microbial diversity missed using current profiling methods. Microbiome 6:61. doi: 10.1186/s40168-018-0442-3
Goncalves, J. L., Tomazi, T., Barreiro, J. R., Beuron, D. C., Arcari, M. A., Lee, S. H., et al. (2016). Effects of bovine subclinical mastitis caused by Corynebacterium spp. on somatic cell count, milk yield and composition by comparing contralateral quarters. Vet. J. 209, 87–92. doi: 10.1016/j.tvjl.2015.08.009
Gschwendtner, S., Alatossava, T., Kublik, S., Fuka, M. M., Schloter, M., and Munsch-Alatossava, P. (2016). N2 gas flushing alleviates the loss of bacterial diversity and inhibits psychrotrophic Pseudomonas during the cold storage of bovine raw milk. PLoS One 11:e0146015. doi: 10.1371/journal.pone.0146015
Gurung, M., Nam, H. M., Tamang, M. D., Chae, M. H., Jang, G. C., Jung, S. C., et al. (2013). Prevalence and antimicrobial susceptibility of Acinetobacter from raw bulk tank milk in Korea. J. Dairy Sci. 96, 1997–2002. doi: 10.3168/jds.2012-5965
Holmoy, I. H., Toft, N., Jorgensen, H. J., Mork, T., Solverod, L., and Nodtvedt, A. (2018). Latent class analysis of real time qPCR and bacteriological culturing for the diagnosis of Streptococcus agalactiae in cow composite milk samples. Prev. Vet. Med. 154, 119–123. doi: 10.1016/j.prevetmed.2018.03.019
Huws, S. A., Kim, E. J., Lee, M. R., Scott, M. B., Tweed, J. K., Pinloche, E., et al. (2011). As yet uncultured bacteria phylogenetically classified as Prevotella, Lachnospiraceae incertae sedis and unclassified Bacteroidales, Clostridiales and Ruminococcaceae may play a predominant role in ruminal biohydrogenation. Environ. Microbiol. 13, 1500–1512. doi: 10.1111/j.1462-2920.2011.02452.x
Ishikawa, M., Kodama, K., Yasuda, H., Okamoto-Kainuma, A., Koizumi, K., and Yamasato, K. (2007). Presence of halophilic and alkaliphilic lactic acid bacteria in various cheeses. Lett. Appl. Microbiol. 44, 308–313. doi: 10.1111/j.1472-765X.2006.02073.x
Jeong, J. Y., Park, H. D., Lee, K. H., Weon, H. Y., and Ka, J. O. (2011). Microbial community analysis and identification of alternative host-specific fecal indicators in fecal and river water samples using pyrosequencing. J. Microbiol. 49, 585–594. doi: 10.1007/s12275-011-0530-6
Kable, M. E., Srisengfa, Y., Laird, M., Zaragoza, J., McLeod, J., Heidenreich, J., et al. (2016). The core and seasonal microbiota of raw bovine milk in tanker trucks and the impact of transfer to a milk processing facility. mBio 7:e00836-16. doi: 10.1128/mBio.00836-16
Karstens, L., Asquith, M., Davin, S., Fair, D., Gregory, W. T., Wolfe, A. J., et al. (2018). Controlling for contaminants in low biomass 16S rRNA gene sequencing experiments. bioRxiv [Preprint]. doi: 10.1101/329854
Katholm, J., Bennedsgaard, T. W., Koskinen, M. T., and Rattenborg, E. (2012). Quality of bulk tank milk samples from Danish dairy herds based on real-time polymerase chain reaction identification of mastitis pathogens. J. Dairy Sci. 95, 5702–5708. doi: 10.3168/jds.2011-5307
Kennedy, R., Lappin, D. F., Dixon, P. M., Buijs, M. J., Zaura, E., Crielaard, W., et al. (2016). The microbiome associated with equine periodontitis and oral health. Vet. Res. 47:49. doi: 10.1186/s13567-016-0333-1
Klaas, I. C., and Zadoks, R. N. (2017). An update on environmental mastitis: challenging perceptions. Transbound. Emerg. Dis. 65(Suppl. 1), 166–185. doi: 10.1111/tbed.12704
Kuang, Y., Tani, K., Synnott, A. J., Ohshima, K., Higuchi, H., Nagahata, H., et al. (2009). Characterization of bacterial population of raw milk from bovine mastitis by culture-independent PCR–DGGE method. Biochem. Eng. J. 45, 76–81. doi: 10.1016/j.bej.2009.02.010
Kuehn, J. S., Gorden, P. J., Munro, D., Rong, R., Dong, Q., Plummer, P. J., et al. (2013). Bacterial community profiling of milk samples as a means to understand culture-negative bovine clinical mastitis. PLoS One 8:e61959. doi: 10.1371/journal.pone.0061959
Li, J., Zhang, J., Liu, L., Fan, Y., Li, L., Yang, Y., et al. (2015). Annual periodicity in planktonic bacterial and archaeal community composition of eutrophic Lake Taihu. Sci. Rep. 5:15488. doi: 10.1038/srep15488
Li, N., Wang, Y., You, C., Ren, J., Chen, W., Zheng, H., et al. (2018). Variation in raw milk microbiota throughout 12 months and the impact of weather conditions. Sci. Rep. 8:2371. doi: 10.1038/s41598-018-20862-8
Lima, S. F., Bicalho, M. L. S., and Bicalho, R. C. (2018). Evaluation of milk sample fractions for characterization of milk microbiota from healthy and clinical mastitis cows. PLoS One 13:e0193671. doi: 10.1371/journal.pone.0193671
Logan, E. F., Neill, S. D., and Mackie, D. P. (1982). Mastitis in dairy cows associated with an aerotolerant Campylobacter. Vet. Rec. 110, 229–230. doi: 10.1136/vr.110.10.229
Magoc, T., and Salzberg, S. L. (2011). FLASH: fast length adjustment of short reads to improve genome assemblies. Bioinformatics 27, 2957–2963. doi: 10.1093/bioinformatics/btr507
Masoud, W., Takamiya, M., Vogensen, F. K., Lillevang, S., Al-Soud, W. A., Sørensen, S. J., et al. (2011). Characterization of bacterial populations in Danish raw milk cheeses made with different starter cultures by denaturating gradient gel electrophoresis and pyrosequencing. Int. Dairy J. 21, 142–148. doi: 10.1016/j.idairyj.2010.10.007
McDougall, S., Parker, K. I., Heuer, C., and Compton, C. W. (2009). A review of prevention and control of heifer mastitis via non-antibiotic strategies. Vet. Microbiol. 134, 177–185. doi: 10.1016/j.vetmic.2008.09.026
Metsalu, T., and Vilo, J. (2015). ClustVis: a web tool for visualizing clustering of multivariate data using Principal Component Analysis and heatmap. Nucleic Acids Res. 43, W566–W570. doi: 10.1093/nar/gkv468
Metzger, S. A., Hernandez, L. L., Skarlupka, J. H., Suen, G., Walker, T. M., and Ruegg, P. L. (2018). Influence of sampling technique and bedding type on the milk microbiota: results of a pilot study. J. Dairy Sci. 101, 6346–6356. doi: 10.3168/jds.2017-14212
Oikonomou, G., Bicalho, M. L., Meira, E., Rossi, R. E., Foditsch, C., Machado, V. S., et al. (2014). Microbiota of cow’s milk; distinguishing healthy, sub-clinically and clinically diseased quarters. PLoS One 9:e85904. doi: 10.1371/journal.pone.0085904
Oikonomou, G., Machado, V. S., Santisteban, C., Schukken, Y. H., and Bicalho, R. C. (2012). Microbial diversity of bovine mastitic milk as described by pyrosequencing of metagenomic 16s rDNA. PLoS One 7:e47671. doi: 10.1371/journal.pone.0047671
Oikonomou, G., Teixeira, A. G., Foditsch, C., Bicalho, M. L., Machado, V. S., and Bicalho, R. C. (2013). Fecal microbial diversity in pre-weaned dairy calves as described by pyrosequencing of metagenomic 16S rDNA. Associations of Faecalibacterium species with health and growth. PLoS One 8:e63157. doi: 10.1371/journal.pone.0063157
Olde Riekerink, R. G., Barkema, H. W., Kelton, D. F., and Scholl, D. T. (2008). Incidence rate of clinical mastitis on Canadian dairy farms. J. Dairy Sci. 91, 1366–1377. doi: 10.3168/jds.2007-0757
Osman, K. M., Samir, A., Abo-Shama, U. H., Mohamed, E. H., Orabi, A., and Zolnikov, T. (2016). Determination of virulence and antibiotic resistance pattern of biofilm producing Listeria species isolated from retail raw milk. BMC Microbiol. 16:263. doi: 10.1186/s12866-016-0880-7
Oultram, J. W., Ganda, E. K., Boulding, S. C., Bicalho, R. C., and Oikonomou, G. (2017). A metataxonomic approach could be considered for cattle clinical mastitis diagnostics. Front. Vet. Sci. 4:36. doi: 10.3389/fvets.2017.00036
Pang, M., Sun, L., He, T., Bao, H., Zhang, L., Zhou, Y., et al. (2017). Molecular and virulence characterization of highly prevalent Streptococcus agalactiae circulated in bovine dairy herds. Vet. Res. 48:65. doi: 10.1186/s13567-017-0461-2
Patel, S. H., Vaidya, Y. H., Patel, R. J., Pandit, R. J., Joshi, C. G., and Kunjadiya, A. P. (2017). Culture independent assessment of human milk microbial community in lactational mastitis. Sci. Rep. 7:7804. doi: 10.1038/s41598-017-08451-7
Pootakham, W., Mhuantong, W., Yoocha, T., Putchim, L., Sonthirod, C., Naktang, C., et al. (2017). High resolution profiling of coral-associated bacterial communities using full-length 16S rRNA sequence data from PacBio SMRT sequencing system. Sci. Rep. 7:2774. doi: 10.1038/s41598-017-03139-4
Quigley, L., O’Sullivan, O., Stanton, C., Beresford, T. P., Ross, R. P., Fitzgerald, G. F., et al. (2013). The complex microbiota of raw milk. FEMS Microbiol. Rev. 37, 664–698. doi: 10.1111/1574-6976.12030
Ramees, T. P., Dhama, K., Karthik, K., Rathore, R. S., Kumar, A., Saminathan, M., et al. (2017). Arcobacter: an emerging food-borne zoonotic pathogen, its public health concerns and advances in diagnosis and control - a comprehensive review. Vet. Q. 37, 136–161. doi: 10.1080/01652176.2017.1323355
Rodrigues, M. X., Lima, S. F., Canniatti-Brazaca, S. G., and Bicalho, R. C. (2017). The microbiome of bulk tank milk: characterization and associations with somatic cell count and bacterial count. J. Dairy Sci. 100, 2536–2552. doi: 10.3168/jds.2016-11540
Ruegg, P. L. (2017). A 100-Year Review: mastitis detection, management, and prevention. J. Dairy Sci. 100, 10381–10397. doi: 10.3168/jds.2017-13023
Salter, S. J., Cox, M. J., Turek, E. M., Calus, S. T., Cookson, W. O., Moffatt, M. F., et al. (2014). Reagent and laboratory contamination can critically impact sequence-based microbiome analyses. BMC Biol. 12:87. doi: 10.1186/s12915-014-0087-z
Samanta, A. K., Jayaram, C., Jayapal, N., Sondhi, N., Kolte, A. P., Senani, S., et al. (2015). Assessment of fecal microflora changes in pigs supplemented with herbal residue and prebiotic. PLoS One 10:e0132961. doi: 10.1371/journal.pone.0132961
Sanders, J. W., Martin, J. W., Hooke, M., and Hooke, J. (2000). Methylobacterium mesophilicum infection: case report and literature review of an unusual opportunistic pathogen. Clin. Infect. Dis. 30, 936–938. doi: 10.1086/313815
Sankar, S. A., Lo, C. I., Fall, B., Sambe-Ba, B., Mediannikov, O., Diallo, I., et al. (2015). Noncontiguous finished genome sequence and description of Weeksella massiliensis sp. nov. New Microbes New Infect. 8, 89–98. doi: 10.1016/j.nmni.2015.09.013
Santman-Berends, I., Swinkels, J. M., Lam, T., Keurentjes, J., and van Schaik, G. (2016). Evaluation of udder health parameters and risk factors for clinical mastitis in Dutch dairy herds in the context of a restricted antimicrobial usage policy. J. Dairy Sci. 99, 2930–2939. doi: 10.3168/jds.2015-10398
Schornsteiner, E., Mann, E., Bereuter, O., Wagner, M., and Schmitz-Esser, S. (2014). Cultivation-independent analysis of microbial communities on Austrian raw milk hard cheese rinds. Int. J. Food Microbiol. 180, 88–97. doi: 10.1016/j.ijfoodmicro.2014.04.010
Schukken, Y., Chuff, M., Moroni, P., Gurjar, A., Santisteban, C., Welcome, F., et al. (2012). The “other” gram-negative bacteria in mastitis: Klebsiella, Serratia, and more. Vet. Clin. North Am. Food Anim. Pract. 28, 239–256. doi: 10.1016/j.cvfa.2012.04.001
Schukken, Y. H., Gonzalez, R. N., Tikofsky, L. L., Schulte, H. F., Santisteban, C. G., Welcome, F. L., et al. (2009). CNS mastitis: nothing to worry about? Vet. Microbiol. 134, 9–14. doi: 10.1016/j.vetmic.2008.09.014
Segata, N., Izard, J., Waldron, L., Gevers, D., Miropolsky, L., Garrett, W. S., et al. (2011). Metagenomic biomarker discovery and explanation. Genome Biol. 12:R60. doi: 10.1186/gb-2011-12-6-r60
Serraino, A., Florio, D., Giacometti, F., Piva, S., Mion, D., and Zanoni, R. G. (2013). Presence of Campylobacter and Arcobacter species in in-line milk filters of farms authorized to produce and sell raw milk and of a water buffalo dairy farm in Italy. J. Dairy Sci. 96, 2801–2807. doi: 10.3168/jds.2012-6249
Shittu, A., Abdullahi, J., Jibril, A., Mohammed, A. A., and Fasina, F. O. (2012). Sub-clinical mastitis and associated risk factors on lactating cows in the Savannah Region of Nigeria. BMC Vet. Res. 8:134. doi: 10.1186/1746-6148-8-134
Shome, B. R., Das Mitra, S., Bhuvana, M., Krithiga, N., Velu, D., Shome, R., et al. (2011). Multiplex PCR assay for species identification of bovine mastitis pathogens. J. Appl. Microbiol. 111, 1349–1356. doi: 10.1111/j.1365-2672.2011.05169.x
Slenker, A. K., Hess, B. D., Jungkind, D. L., and DeSimone, J. A. (2012). Fatal case of Weeksella virosa sepsis. J. Clin. Microbiol. 50, 4166–4167. doi: 10.1128/JCM.01761-12
Sperling, J. L., Silva-Brandao, K. L., Brandao, M. M., Lloyd, V. K., Dang, S., Davis, C. S., et al. (2017). Comparison of bacterial 16S rRNA variable regions for microbiome surveys of ticks. Ticks Tick Borne Dis. 8, 453–461. doi: 10.1016/j.ttbdis.2017.02.002
Stanisavljevic, S., Lukic, J., Momcilovic, M., Miljkovic, M., Jevtic, B., Kojic, M., et al. (2016). Gut-associated lymphoid tissue, gut microbes and susceptibility to experimental autoimmune encephalomyelitis. Benef. Microbes 7, 363–373. doi: 10.3920/BM2015.0159
Taponen, S., Salmikivi, L., Simojoki, H., Koskinen, M. T., and Pyorala, S. (2009). Real-time polymerase chain reaction-based identification of bacteria in milk samples from bovine clinical mastitis with no growth in conventional culturing. J. Dairy Sci. 92, 2610–2617. doi: 10.3168/jds.2008-1729
Tedersoo, L., Tooming-Klunderud, A., and Anslan, S. (2018). PacBio metabarcoding of fungi and other eukaryotes: errors, biases and perspectives. New Phytol. 217, 1370–1385. doi: 10.1111/nph.14776
Van Driessche, E., Houf, K., Vangroenweghe, F., De Zutter, L., and Van Hoof, J. (2005). Prevalence, enumeration and strain variation of Arcobacter species in the faeces of healthy cattle in Belgium. Vet. Microbiol. 105, 149–154. doi: 10.1016/j.vetmic.2004.11.002
Winter, P., Schilcher, F., Bago, Z., Schoder, D., Egerbacher, M., Baumgartner, W., et al. (2004). Clinical and histopathological aspects of naturally occurring mastitis caused by Listeria monocytogenes in cattle and ewes. J. Vet. Med. 51, 176–179. doi: 10.1111/j.1439-0450.2004.00751.x
Yeoman, C. J., Ishaq, S. L., Bichi, E., Olivo, S. K., Lowe, J., and Aldridge, B. M. (2018). Biogeographical differences in the influence of maternal microbial sources on the early successional development of the bovine neonatal gastrointestinal tract. Sci. Rep. 8:3197. doi: 10.1038/s41598-018-21440-8
Yesilmen, S., Vural, A., Erkan, M. E., and Yildirim, I. H. (2014). Prevalence and antimicrobial susceptibility of Arcobacter species in cow milk, water buffalo milk and fresh village cheese. Int. J. Food Microbiol. 188, 11–14. doi: 10.1016/j.ijfoodmicro.2014.07.006
Keywords: milk microbiota, 16S rRNA gene sequencing, bovine mastitis, IRSCM, mastitis-causing pathogens
Citation: Pang M, Xie X, Bao H, Sun L, He T, Zhao H, Zhou Y, Zhang L, Zhang H, Wei R, Xie K and Wang R (2018) Insights Into the Bovine Milk Microbiota in Dairy Farms With Different Incidence Rates of Subclinical Mastitis. Front. Microbiol. 9:2379. doi: 10.3389/fmicb.2018.02379
Received: 07 June 2018; Accepted: 18 September 2018;
Published: 16 October 2018.
Edited by:
George Tsiamis, University of Patras, GreeceReviewed by:
Aggeliki Saridaki, Technical University of Crete, GreeceBeatrix Stessl, Veterinärmedizinische Universität Wien, Austria
Copyright © 2018 Pang, Xie, Bao, Sun, He, Zhao, Zhou, Zhang, Zhang, Wei, Xie and Wang. This is an open-access article distributed under the terms of the Creative Commons Attribution License (CC BY). The use, distribution or reproduction in other forums is permitted, provided the original author(s) and the copyright owner(s) are credited and that the original publication in this journal is cited, in accordance with accepted academic practice. No use, distribution or reproduction is permitted which does not comply with these terms.
*Correspondence: Ran Wang, cmFud2FuZ0BqYWFzLmFjLmNu