- 1Department of Ecology and Evolution, University of Lausanne, Lausanne, Switzerland
- 2Department of Fundamental Microbiology, University of Lausanne, Lausanne, Switzerland
- 3Institute of Earth Surface Dynamics, University of Lausanne, Lausanne, Switzerland
- 4Center for Primary Care and Public Health (Unisanté), University of Lausanne, Lausanne, Switzerland
Archaeorhizomycetes, a widespread fungal class with a dominant presence in many soil environments, contains cryptic filamentous species forming plant-root associations whose role in terrestrial ecosystems remains unclear. Here, we apply a correlative approach to identify the abiotic and biotic environmental variables shaping the distribution of this fungal group. We used a DNA sequencing dataset containing Archaeorhizomycetes sequences and environmental variables from 103 sites, obtained through a random-stratified sampling in the Western Swiss Alps along a wide elevation gradient (>2,500 m). We observed that the relative abundance of Archaeorhizomycetes follows a “humped-shaped” curve. Fitted linear and quadratic generalized linear models revealed that both climatic (minimum temperature, precipitation sum, growing degree-days) and edaphic (carbon, hydrogen, organic carbon, aluminum oxide, and phyllosilicates) factors contribute to explaining the variation in Archaeorhizomycetes abundance. Furthermore, a network inference topology described significant co-abundance patterns between Archaeorhizomycetes and other saprotrophic and ectomycorrhizal fungal taxa. Overall, our results provide strong support to the hypothesis that Archaeorhizomycetes in this area have clear ecological requirements along wide, elevation-driven abiotic and biotic gradients. Additionally, correlations to soil redox parameters, particularly with phyllosilicates minerals, suggest Archaeorhizomycetes might be implied in biological rock weathering. Such soil taxa-environment studies along wide gradients are thus a useful complement to latitudinal field observations and culture-based approaches to uncover the ecological roles of cryptic soil organisms.
Introduction
Microorganisms, like soil fungi, were recently shown to follow similar biogeographic patterns to macroorganisms, such as vascular plants, and were shown to be influenced by similar variables, such as climate and soil composition (Pellissier et al., 2014; Tedersoo et al., 2014). As for macroorganisms, their distributions can thus be expected to be, at least partly, driven by their environmental requirements (i.e., if speaking at the level of their constituent species, by their environmental niche).
As formalized by Hutchinson (Hutchinson, 1957), the realized environmental niche – or ecological niche (Martínez-Meyer et al., 2013) – describes the N-dimensional envelope of abiotic conditions, constrained by biotic interactions, which allow populations of a particular species to develop and persist (Pulliam, 2000; Wiens et al., 2010). Typically captured from field observations, the ecological niche concept has greatly facilitated our understanding of the distribution of species (Araújo and Guisan, 2006; Soberón and Nakamura, 2009). The same approach can be applied to higher taxonomic levels to assess the envelope of environmental requirements of all species within a group (Hadly et al., 2009; Smith et al., 2019), although in such case, the positive population growth criteria cannot be applied to the whole group.
Mountain regions with wide elevation-driven environmental gradients, in particular, provide a unique opportunity for studying environmental requirements of taxa because of the strong eco-physiological and abiotic variations observed within a confined geographic area (Becker et al., 2007; Pottier et al., 2013; Descombes et al., 2016). Elevational gradients also offer several features that make them suitable for microbial biogeographical studies: sampling strategies can be more easily based on stratifying environmental information (Hirzel and Guisan, 2002); steep changes over short distances allow reproducible field experiments (Pellissier et al., 2013); and the elevation forms a natural laboratory to track climate change and land use on species diversity (Becker et al., 2007). More recently, biogeographical studies have started focusing on the distribution of soil microorganisms along elevational gradients (Bryant et al., 2008; Fierer et al., 2011; Wang et al., 2012; Pellissier et al., 2014; Tedersoo et al., 2014; Yashiro et al., 2016), but so far very few have attempted to determine the environmental requirements of cryptic soil fungi as done here along wide continuous gradients.
Correlative studies revealing clear environmental determinism of mycorrhizal fungi at global (Tedersoo et al., 2014) or regional (Pellissier et al., 2014; Jarvis et al., 2015) scales strongly support that environmental requirements also govern the distribution and assemblage of soil fungi across latitudinal and elevational gradients. These studies describe edaphic factors, such as pH, phosphate, and nitrogen concentration (Pellissier et al., 2014; Shen et al., 2014; Rincón et al., 2015), and climatic variables, such as mean annual temperature or growing degree-days, as predictors of fungi distributions (Pellissier et al., 2013, 2014; Tedersoo et al., 2014). Other studies revealed the importance of biotic interactions for fungi, such as with plants (e.g., fungi as predictors of plants in Pellissier et al., 2013), but these studies have mostly been limited to mycorrhizal fungal species (e.g., van der Heijden et al., 1998, 2015; Tedersoo et al., 2010, 2012). Yet, most insights about the environmental requirements of fungi in nature have so far been restricted to contrasting a few different environmental conditions at a limited number of sites, often mainly contrasting different soil horizons (depth) or soil composition (e.g., Dickie et al., 2002; Dickie, 2007; Taylor et al., 2014), whereas several studies focused only on mycorrhizal fungi (e.g., Buée et al., 2007; Lekberg et al., 2007). These studies inform on the drivers of fungal communities in specific habitats and soil horizons, but do not provide a continuous and comprehensive view of fungal requirements (i.e., fitting continuous responses along environmental gradients) that account for multiple environmental factors (see Guisan et al., 2017). Although Hutchinson’s niche concept was originally defined – and should best be used – at the species level, the idea that taxonomic groups above and below the species level can also strive within a clear envelope of environmental conditions also led some authors to apply the niche concept to infra-specific (Pearman et al., 2010; Banta et al., 2012) or supra-specific (Hadly et al., 2009; Smith et al., 2019), levels. Although not formally corresponding to Hutchinson’s definition, the latter case can inform on the environmental requirements of an entire fungal group or clade (e.g., genus, family, order, or class). Advancing our understanding of the ecological requirements and spatial distributions of soil fungi at multiple taxonomic depth will require sampling soils across large regions spanning wide elevation and other abiotic and biotic gradients (e.g., Pellissier et al., 2014; Tedersoo et al., 2014 for soil fungi, Yashiro et al., 2016, 2018 for soil bacteria).
Archaeorhizomycetes comprise a deep branching phylogenetic (i.e., ancient) class of non-mycorrhizal and largely non-cultured fungi (but see Menkis et al., 2014) belonging to the subphylum Taphrinomycotina (Ascomycota) and so far only includes one described order, (Archaeorhizomycetales), one family (Archaeorhizomycetaceae), and one genus (Archaeorhizomyces) (Rosling et al., 2011), but the classification may still evolve given this lineage is still largely uncharacterized. It comprises hundreds of cryptically reproducing filamentous species without known fruiting structures, globally distributed in different world regions and in various terrestrial ecosystems (Porter et al., 2008; Rosling et al., 2011, 2013). These include soils of pacific coastal temperate conifer rainforest (Levy-Booth et al., 2018), cold temperate Nothofagus forests (Fernández-Martínez et al., 2017), of boreal forests (Clemmensen et al., 2015), and of arctic tundra (Borg Dahl et al., 2017), where Archaeorhizomycetes can locally dominate some soil compartments (e.g., in rhizosphere, Urbina et al., 2018; in arctic soils, Borg Dahl et al., 2017), but with overall high variations in relative abundances (e.g., along a deglaciation chronosequence; Fernández-Martínez et al., 2017). As first proposed by Rosling et al. (2011), together with the more detailed description of the type species Archaeorhizomyces finlayi, Archaeorhizomycetes are non-pathogenic plant root and rhizosphere associated fungi with putative saprotrophic activity (Rosling et al., 2011). The presence of sequence signatures has suggested Archaeorhizomycetes to occupy plant rhizospheres in deeper soil horizons with low pH and high nutrient turnover (Rosling et al., 2013; Clemmensen et al., 2015; Carrino-Kyker et al., 2016; Urbina et al., 2018). Archaeorhizomycetes spp. often dominate such sites and are commonly associated with mycorrhizal fungi (Choma et al., 2016). In addition, they seem to show specificity for certain plant types (e.g., Tsuga, Picea, and Pinus spp.; Rosling et al., 2013). Despite this wealth of information (Rosling et al., 2013), the absence of systematic sampling of fungal communities along environmental gradients makes the ecological role and trophic status of Archaeorhizomycetes in terrestrial ecosystems still elusive (Choma et al., 2016; Urbina et al., 2018). Since most Archaeorhizomycetes remain uncultured and their taxonomic diversity is poorly understood (Hughes Martiny et al., 2006), addressing their biogeography through molecular tools is cumbersome and, even if still prone to biases due to the used bioinformatic workflows (Rosling et al., 2013; Choma et al., 2016), large progress on their biogeographic determinants can still be expected through running a large and systematic metagenomics-based field survey.
The advance we bring here is to use a large soil metagenomics dataset sampled across wide environmental gradients in the Western Swiss Alps (Yashiro et al., 2016, 2018). The area was sampled in a random-stratified strategy aiming to cover a gradual change in abiotic (i.e., edaphic and climatic variables) and potentially biotic conditions. We mine this metagenomics DNA sample set specifically for Archaeorhizomycetes (indeed, here with only one genus, Archaeorhizomyces, included), in order to better assess the realized environmental requirements (“niche”) of this group in this area and along the abiotic gradients investigated, and its potential biotic relationships with plants and other fungi. We used a statistical approach to quantify those abiotic and biotic factors correlated to Archaeorhizomycetes abundance, and this way identify the envelope of suitable environmental conditions for this group (and the currently single order, family and genus included; thus overall at a supra-specific level; Hadly et al., 2009; Smith et al., 2019). More specifically, we aimed to answer the following three questions: (i) Do Archaeorhizomycetes (and here at least the single Archaeorhizomyces genus it includes) exhibit geographic patterns along environmental – and especially elevational – gradients? (ii) Are there abiotic factors that significantly explain the observed patterns? (iii) Are there significant correlations between Archaeorhizomycetes marker sequence abundances and that of other organisms such as plant species and fungi?
Materials and Methods
Analytical Framework
We used a large environmental DNA sample set and environmental variables from 103 sites obtained by a random-stratified sampling across wide spatial and elevational gradients in the Western Swiss Alps during summer 2012 (July–September). Fungal marker sequences including Archaeorhizomycetes were amplified from the purified environmental DNAs, sequenced and analyzed to operational taxonomic units (OTUs) as described below. Those abiotic and biotic variables with the greatest correlation with Archaeorhizomycetes OTUs were selected using Spearman rank correlations. Next, we fitted generalized linear models (GLMs) with the abundance of Archaeorhizomycetes sequences as the response variable and the selected environmental variables as predictors to identify the significant abiotic variables explaining this whole group’s “ecological niche.” Lastly, we analyzed the correlation of Archaeorhizomycetes with a set of biotic variables, using network inference analyses, to identify significant biotic relationships of Archaeorhizomycetes with other fungal groups, including also all the previously identified significant abiotic variables.
Study Area and Data Collection
This study was performed in the Western Swiss Alps, across an area of ca. 700 km2 spanning an elevation gradient from 374 to 3210 m (Figure 1). This area had already been extensively investigated to understand plant and soil bacteria distributions along an elevation gradient (Dubuis et al., 2011, 2013; Ndiribe et al., 2013; Pottier et al., 2013; Yashiro et al., 2016, 2018; Descombes et al., 2017)1. Soil samples were collected from 103 plots of 4 m2 of open (non-forested) vegetation between 800 and 3000 m in elevation, as described previously (Yashiro et al., 2016, 2018) using a balanced random-stratified sampling design (Hirzel and Guisan, 2002) based on elevation, slope, and aspect. Forest areas were excluded to allow a better comparison of fungal composition in grassland-like habitats along the full elevation gradient. From each sampling site, we measured a total of 49 local edaphic factors (for details on the specific methods, see Buri, 2014; Yashiro et al., 2016; Buri et al., 2017), and 7 climatic variables at 25 m resolution (Descombes et al., 2017; Supplementary Table S1). The percent plant cover for 448 plant species across all plots was inventoried as previously described (Dubuis et al., 2011, 2013).
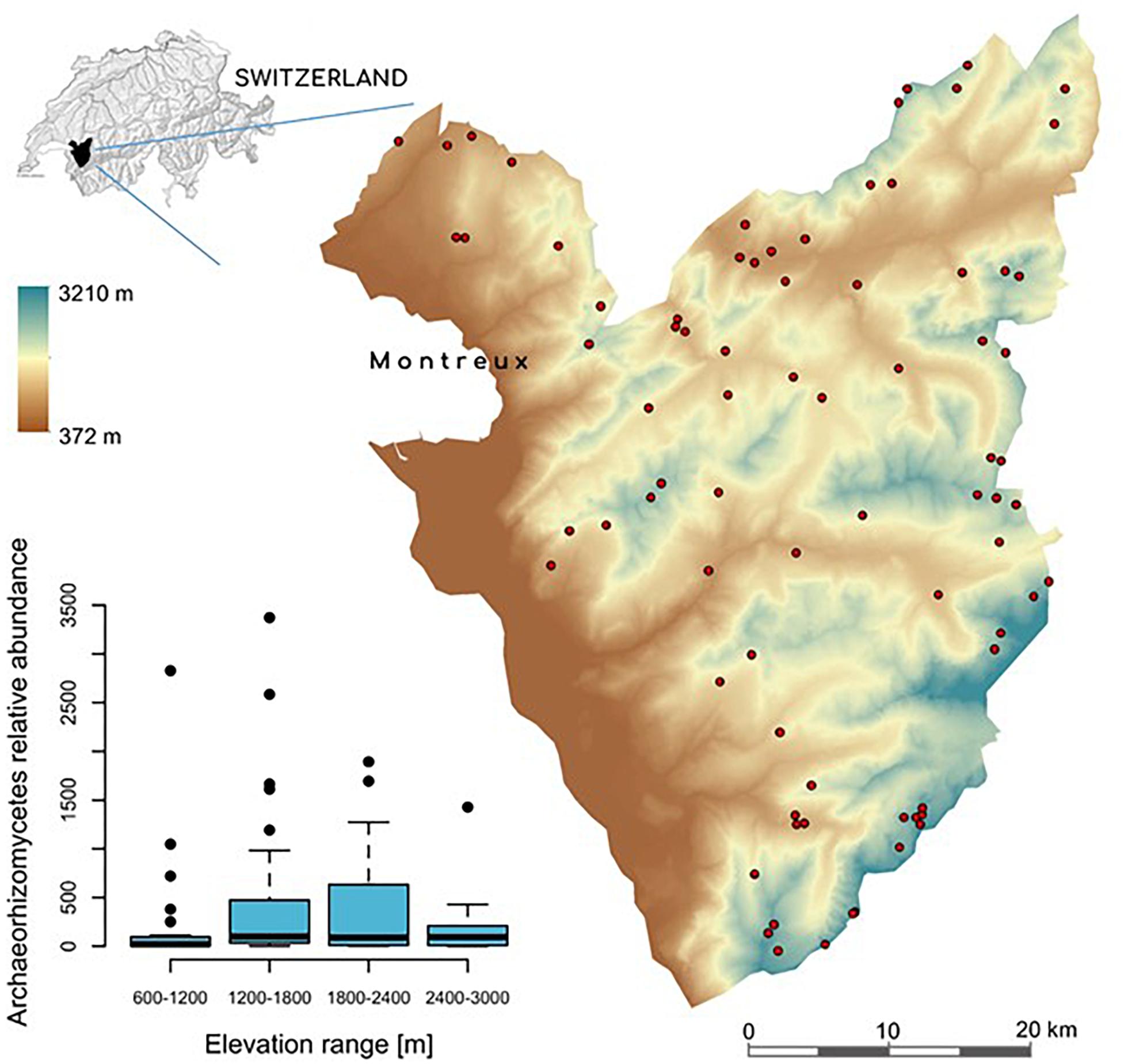
Figure 1. Digital elevation model (DEM) of the study area in the Western Swiss Alps (700 km2). The small map on the top left shows the location of the study area, colored in black, within Switzerland. Red dots correspond to the 103 sampling sites across the >2,500 m-wide elevation gradient. The boxplot on the bottom left, represents the relative abundance of Archaeorhizomycetes OTUs across all samples, categorized in elevational bins.
Molecular Analyses
DNA samples had been extracted and purified from mixed and sieved soil cores (five per site), as described previously (Yashiro et al., 2016). To address fungal diversity, we exploited sequence variation in the fungal internal transcribed spacer region (ITS1), as proposed by Schmidt et al. (2013). ITS1-regions were amplified with primers ITS1F, 5′-GAACCWGCGGARGGATC-3′ (Schmidt et al., 2013) and ITS2, 5′-GCTGCGTTCTTCATCGATGC-3′ (White et al., 1990). Both ITS-primers were coupled at the 5′ end to specific sets of barcode sequences to allow sample multiplexing. In silico tests to evaluate coverage (Supplementary Figure S1) and details of the used ITS-primer barcode couples are provided in the Supplementary Tables S2, S3.
PCR amplifications were carried out in a total volume of 50 μl containing 21 μl H2O, 10 μl of 5×-concentrated PrimerSTAR buffer (Takara, Shiga, Japan), 10 μl of 5 M Betaine solution, 4 μl of a (10 mM mixture of all four deoxy-nucleotides, 0.6 μl of PrimerSTAR HS DNA polymerase (Takara, Shiga, Japan), 2 μl barcoded primer-pair (final concentration 0.2 μM), and 2 μl DNA sample (DNA elution, 25-folds). PCRs were performed on a GeneAmp model 9700 (Applied Biosystems, Foster City, CA, United States) with the following conditions: initial denaturation at 98°C for 2 min, followed by 30 cycles of each, 98°C for 1 min, 57°C for 5 s, and 72°C for 40 s, and a final extension at 72°C for 5 min. The obtained amplicons were purified by using a QIAquick PCR Purification kit (Qiagen, CA, United States) and eluted in 20 μl of elution buffer (Qiagen, CA, United States). Purified PCR products were quantified using the Quant-IT assay kit (Life Technologies, Carlsbad, CA, United States), and pooled to approximate equimolar amounts. Libraries were prepared with the TruSeq DNA sample preparation kit (Illumina, San Diego, CA, United States) for paired-end sequencing (2 × 101 nt) (to see the overall PCR strategy, Supplementary Figure S2). Sequencing was carried out on an Illumina HiSeq 2500 platform at the Genomic Technologies Facility of the University of Lausanne.
Archaeorhizomycetes and Fungal OTUs
Raw fastq reads were demultiplexed and quality filtered according to both the Illumina adapter and the specific paired-barcodes coupled to the ITS primers. Trimming, removal of short reads and initial removal of anomalous reads were done using custom Bash scripts (Yashiro et al., 2016). Forward and reverse paired-end reads were then trimmed to 92 nt, concatenated, and prepared for QIIME 1.8 (Caporaso et al., 2010). A reference dataset was created from the fungal ITS QIIME/UNITE dataset (version 12.11). This dataset contains non-redundant fungal ITS1 sequences trimmed and concatenated in the same way as the paired-end fastq reads (Supplementary Figure S2). Given that only the outer regions of the ITS1 were used for taxonomy assignment, our method does not bias against fungi with very long ITS1 regions. To evaluate the effect of trimming and concatenation in shorter ITS1 regions, such as those of Archaeorhizomycetes, we compared the ITS1 region length between the complete and the concatenated Archaeorhizomycetes sequences obtained from the dataset (UNITE-QIIME 12.11) (Supplementary Figure S3). Sequences between 183 and 192 bp were obtained (20 bp of the 18S, 50 bp of the 5.8S region and a variable region of 112 bp for Archaeorhizomyces finlayi (Schoch et al., 2014) or 121 bp for Archaeorhizomyces borealis (Menkis et al., 2014). Consequently, the concatenated sequence of 184 bp is not expected to present a sufficient overlap in the middle sequence to be detected with a software.
High quality paired-end reads were processed using open-reference OTU picking (Rideout et al., 2014), a hybrid method that implements both closed-reference and de novo clustering using the UCLUST algorithm (Edgar et al., 2011). Referenced and de novo OTUs were combined into a single OTU table (for more details on the overall strategy of fungal metabarcoding using HiSeq Illumina, see Supplementary Figure S4). OTU clusters with less than ten reads were removed (Pagni et al., 2013; Schmidt et al., 2013). To account for differences in sequencing per site, all samples were rarefied (Gotelli and Chao, 2013). Because de novo OTUs for fungi clusters were not very different to referenced OTUs as shown by Procrustes analyses (see Thioulouse et al., 2018) in QIIME 1.8 (Caporaso et al., 2010; Supplementary Figure S5), and because they are not currently well defined (Westcott and Schloss, 2015), all the subsequent analyses were done using the relative abundance of referenced OTUs.
Preselection of Biotic and Abiotic Variables
To use a reasonable number of explanatory variables in the analyses (typically a 1:10 ratio between variables and observations), an initial filtering step was performed using Spearman rank correlations (Clarke and Ainsworth, 1993) to select the biotic and abiotic variables most correlated with the total relative abundance of Archaeorhizomycetes reads. The selection was performed separately within two groups of explanatory variables: biotic (222 fungal OTUs at genus level; 448 plant species) and edaphic (49 variables). No functional fungal group was considered here as defining them is still difficult (Frac et al., 2018). In addition, seven climatic variables already used in previous studies (e.g., Pellissier et al., 2013; Yashiro et al., 2016; Supplementary Table S4) were then added. The three sets of selected variables were jointly visualized by a co-abundance matrix using ggplot2 in R (Wickham, 2009).
GLM Analyses on Abiotic Variables
To identify significant abiotic (i.e., edaphic and climatic) variables explaining Archaeorhizomycetes’ abundance, linear and second order polynomial functions were fitted with GLMs in R (Version 3.2.1, R Development Core Team, 2015) using a Gaussian distribution and identity link function (McCullagh and Nelder, 1989). After analyzing residuals versus predicted values (Supplementary Figures S6A, S7A), and testing the assumptions of normality (Nelder and Wedderburn, 1972; Supplementary Figures S6B, S7B), GLMs were fitted on the ten edaphic and seven climatic variables with untransformed and log-transformed Archaeorhizomycetes abundances (Supplementary Figure S8). A backward stepwise approach based on the Akaike information criterion (AIC) (Burnham and Anderson, 2004) was used to retain the final variables in the multiple regression models, choosing the model with the smallest AIC. Values were corrected for multiple comparisons using the Benjamini-Hochberg method (Benjamini and Hochberg, 1995).
Network Analyses
A co-abundance network inference analysis between Archaeorhizomycetes, the 12 selected biotic variables (i.e., fungal OTUs and plant species) and the abiotic variables selected from the previous correlation and GLM analyses was performed using the SparCC Python module based on Spearman correlations (Friedman and Alm, 2012). To measure the significance of the predicted relationships, pseudo p-values defined as the percentage of times a correlation at least as extreme as the observed were obtained by bootstrapping (1000 resampled datasets) (Friedman and Alm, 2012) were used. To convert the pseudo p-values into significance values, pseudo p-values of near zero were converted to a large positive value by multiplying by 1 × 10-8 and applying a logarithm. Positive converted values >1 were considered significant. To visualize the p-values in the network, we used the igraph R package (Csárdi and Nepusz, 2006).
Results
Fungal OTUs and Archaeorhizomycetes Abundance
A total of 46.9 million high-quality paired-end reads across all sites were obtained. Open-reference clustering using a threshold of 97% identity indicated a high variation of the number of reads per site (Supplementary Table S5). Rarefaction curves at 21,500 reads per site showed asymptotic or nearly asymptotic behavior for most sites (Supplementary Figure S9), suggesting that most of the fungal diversity was captured. From a total of 2.2 million rarefied reads, only 2,094 OTUs (37.48%) could be reference-clustered, whereas 3,497 OTUs (62.52%) were de novo clustered (Supplementary Figure S10). Among the referenced sequences, 36,635 ITS1 sequences were classified as Archaeorhizomycetes (Archaeorhizomyces). This number corresponded to 13.3% of total fungal OTUs (i.e., 278 OTUs) assigned after rarefaction. Archaeorhizomycetes OTUs mostly belonged to four groups of non-cultured Archaeorhizomycetes, represented by GenBank accession numbers DQ182455, GU174301, GU174341, and GU174343 (Rosling et al., 2011). Ranking abundance curve showed that Archaeorhizomycetes was the most abundant and prevalent across sites (Supplementary Figure S11). Relative abundance of Archaeorhizomycetes varied considerably (min = 0; median = 78; mean = 378; max = 3372) and displayed a significant “humped-shape” curve as a function of elevation, characterized by highest abundances at middle elevations (Figure 1).
Preselection of Abiotic and Biotic Variables
Based on the Spearman correlation analyses, 12 biotic variables (six fungal OTUs and six plant species; Supplementary Tables S6, S7, respectively) and ten abiotic edaphic factors (Supplementary Table S8) were selected and used with the seven abiotic topo-climatic predictors in further fungi-environment analyses. Among abiotic edaphic factors, the abundance of Archaeorhizomycetes was most positively correlated with total organic compounds (organic material, carbon, nitrogen, and hydrogen), mineral components [magnesium oxide (MgO), potassium oxide (K2O), aluminum oxide (Al2O3)] and parent material (phyllosilicates) and negatively correlated with quartz content (Figure 2A). Regarding the biotic variables, OTUs of the fungal genera Cephalosporium (taxon synonymous Acremonium), Exophiala and Typhula were the most positively correlated with Archaeorhizomycetes OTUs, whereas those of Omphalotus, Suillus, and Tubeufia were the most negatively correlated with Archaeorhizomycetes (Figure 2B). Archaeorhizomycetes OTU abundance was most positively correlated with the plant species Carex flacca, Helianthemum nummularium, Alchemilla coriacea, Anthyllis vulneraria, Leucanthemum vulgare, and Polygonum viviparum (listed in order of highest to lowest correlation strength; Figure 2B and Supplementary Table S7).
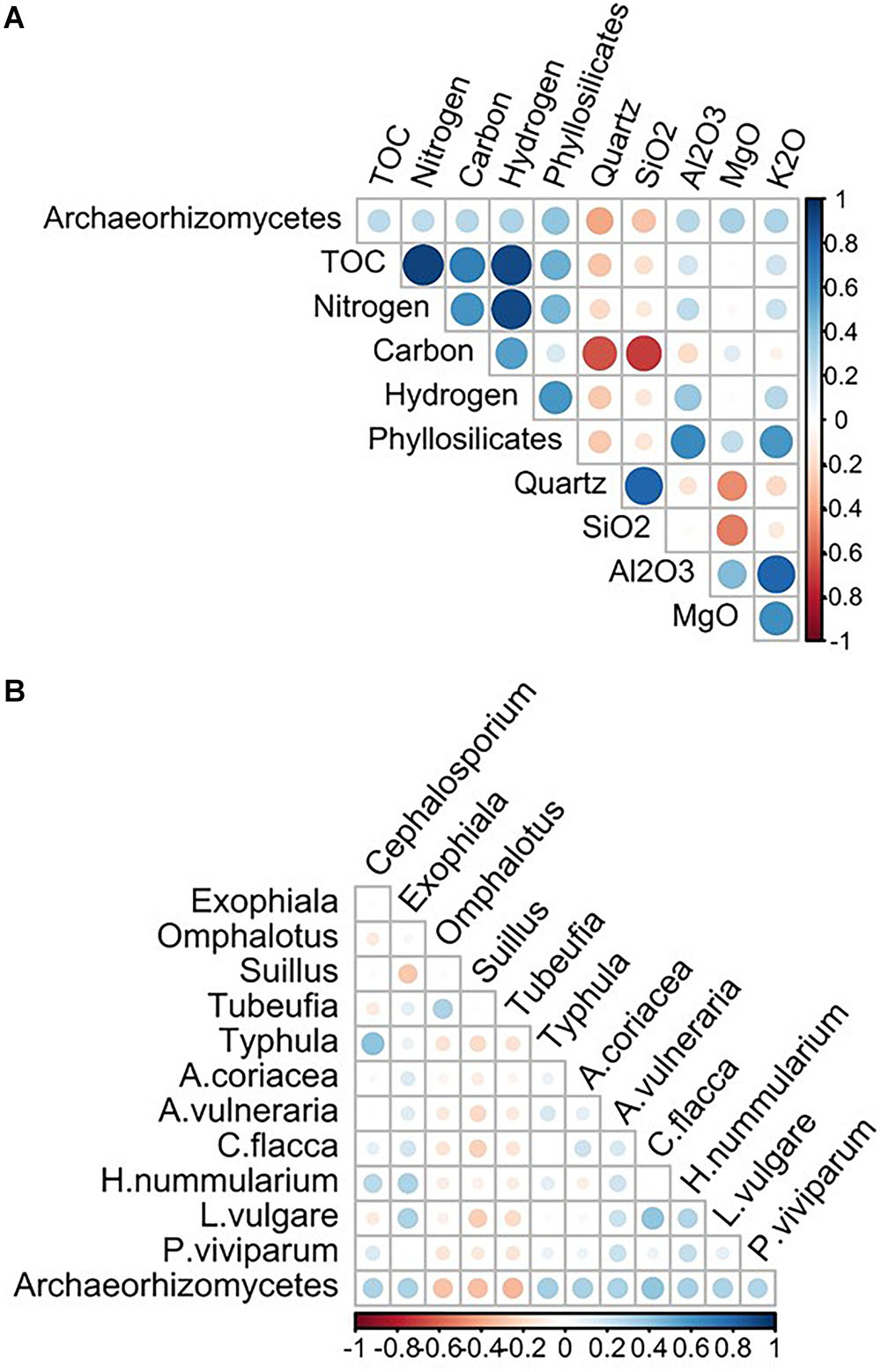
Figure 2. Archaeorhizomycetes OTU relative abundance correlations with 10 edaphic factors (A) abiotic and 12 biotic variables (B), of which 6 fungal genera and 6 plant species. Correlations are described as Spearman correlations, with positive interactions in blue, and negative in red. The shading color and size of the circles correspond to the strength of the correlation, on an absolute scale between 0 and 1.
Archaeorhizomycetes’ Abiotic Environmental Requirements
Multivariate GLMs were obtained between observed Archaeorhizomycetes OTU abundance as the response variable and different edaphic and climatic variables as explanatory variables (Table 1, full models in Supplementary Table S9). After stepwise selection and Benjamini-Hochberg correction for multiple testing, the GLM analysis revealed that Archaeorhizomycetes relative abundance in the soil was most significantly explained by evapotranspiration, growing degree-days, monthly sum precipitation and minimum temperature for the climatic predictors (Table 1), as well as by total organic carbon, carbon, nitrogen, hydrogen, phyllosilicates and aluminum oxide for the edaphic predictors (Table 1). The goodness-of-fit (adjusted pseudo R2) of the model with these selected abiotic factors was 0.58. Inspection of univariate response curves in GLMs further revealed a linear increase of Archaeorhizomycetes relative abundance with phyllosilicate concentrations (Figure 3A), and “humped-shaped” dependencies with total organic carbon, growing degree-days and evapotranspiration (Figures 3B–D, respectively).
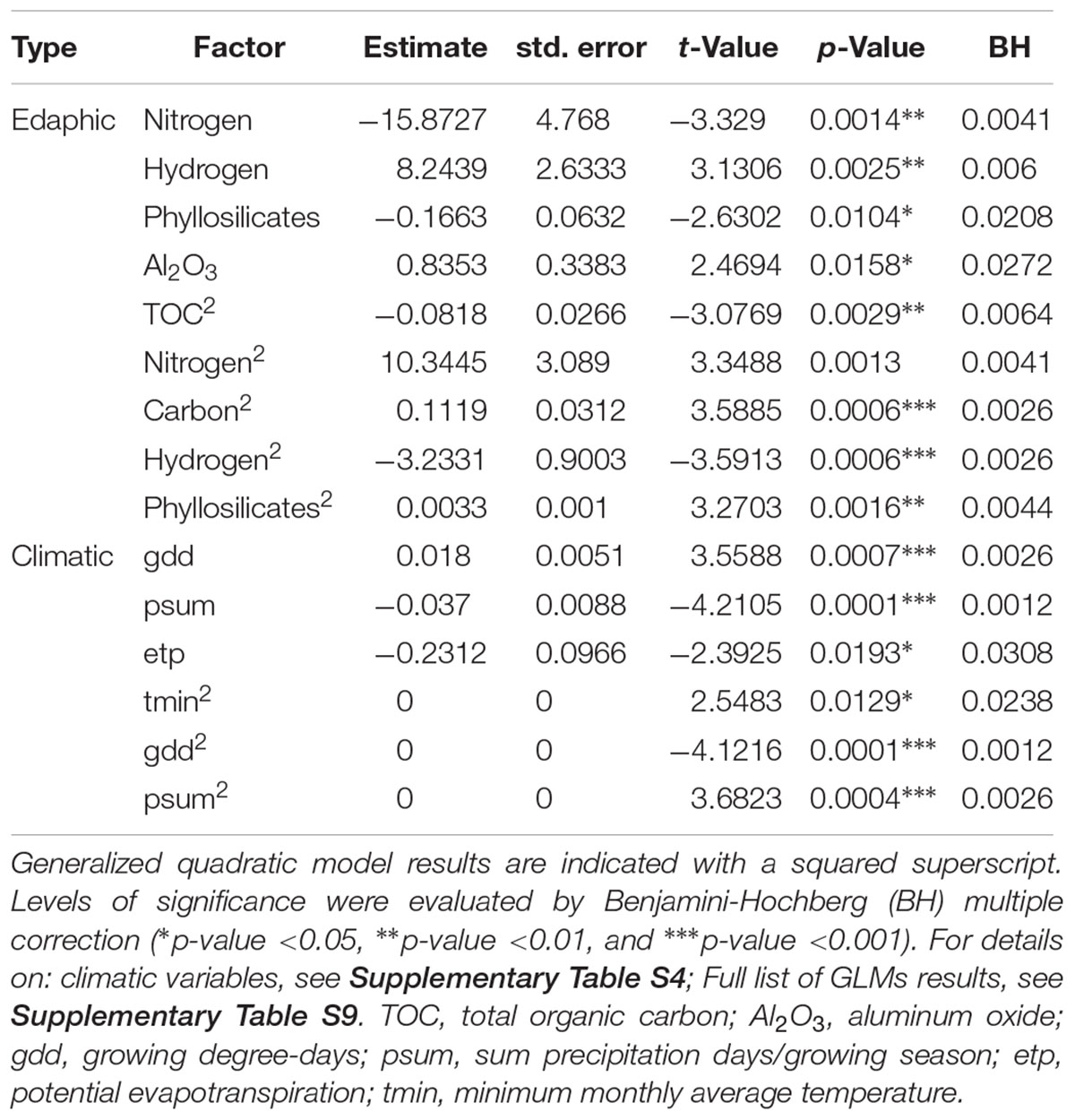
Table 1. Generalized linear model (GLM) and generalized quadratic model most significant results of Archaeorhizomycetes and edaphic and climatic factors.
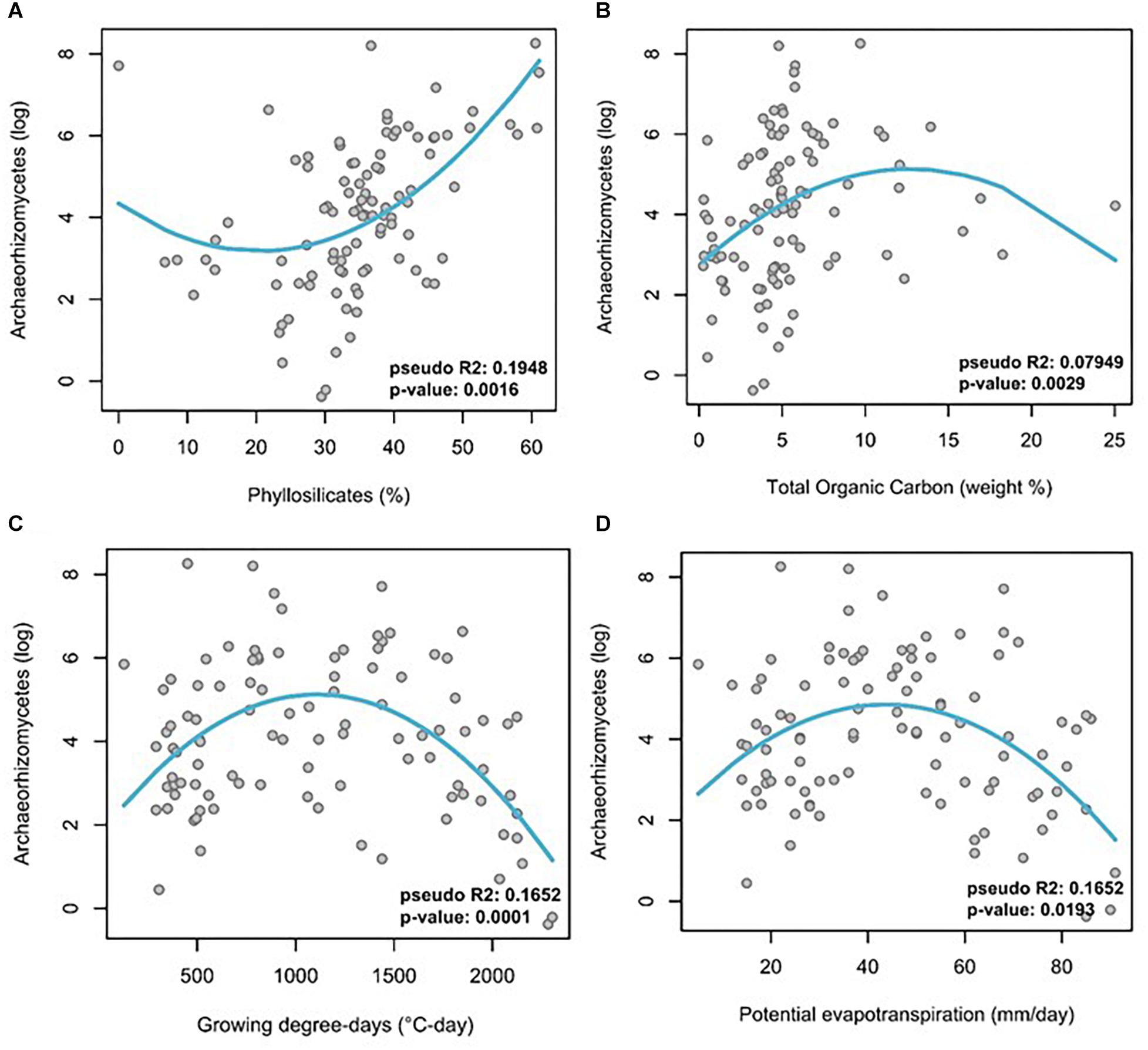
Figure 3. Log-transformed relative abundance of Archaeorhizomycetes OTUs across all samples as a function of abiotic factors. (A) phyllosilicates, (B) total organic carbon, (C) growing degree-days, and (D) potential evapotranspiration. Trend curves were calculated by mean of a univariate GLM using Gaussian family and identity link function. Note the unimodal “humped-shaped” curves for three of the abiotic factors, and a reverse unimodal curve for phyllosilicates.
Global Abiotic and Biotic Network Inference
Once the most explanatory abiotic factors were identified from the GLMs, correlation patterns were further explored using network inference (Figure 4). The network object consisted of 23 nodes and 75 significant edges (an average clustering coefficient of 0.46). Edaphic and climatic variables clustered together (see blue and dark green nodes, Figure 4). The Archaeorhizomycetes environmental edges (in pink) associated significantly with minimum temperature, evapotranspiration, growing degree-days and monthly sum of precipitation (Figure 4). Once the biotic variables were added, phyllosilicate concentrations remained the unique significant edaphic factor directly associated with variations in Archaeorhizomycetes abundances (Figure 4). Regarding the biotic variables, Archaeorhizomycetes abundances correlated significantly with the fungal plant pathogen Typhula (GenBank AF193350), the saprotrophic fungus Exophiala (GenBank AB488490), the Suillus ectomycorrhizal fungal families (GenBank GU187544, GU553371, L54082, L54112), and the genera Tubeufia (GenBank AY916461) and Omphalotus (GenBank AY313271), both involved in vegetation decaying processes (Figure 4, details on the fungi detected are in Supplementary Table S6). None of the plant species considered had a significant direct correlation with Archaeorhizomycetes.
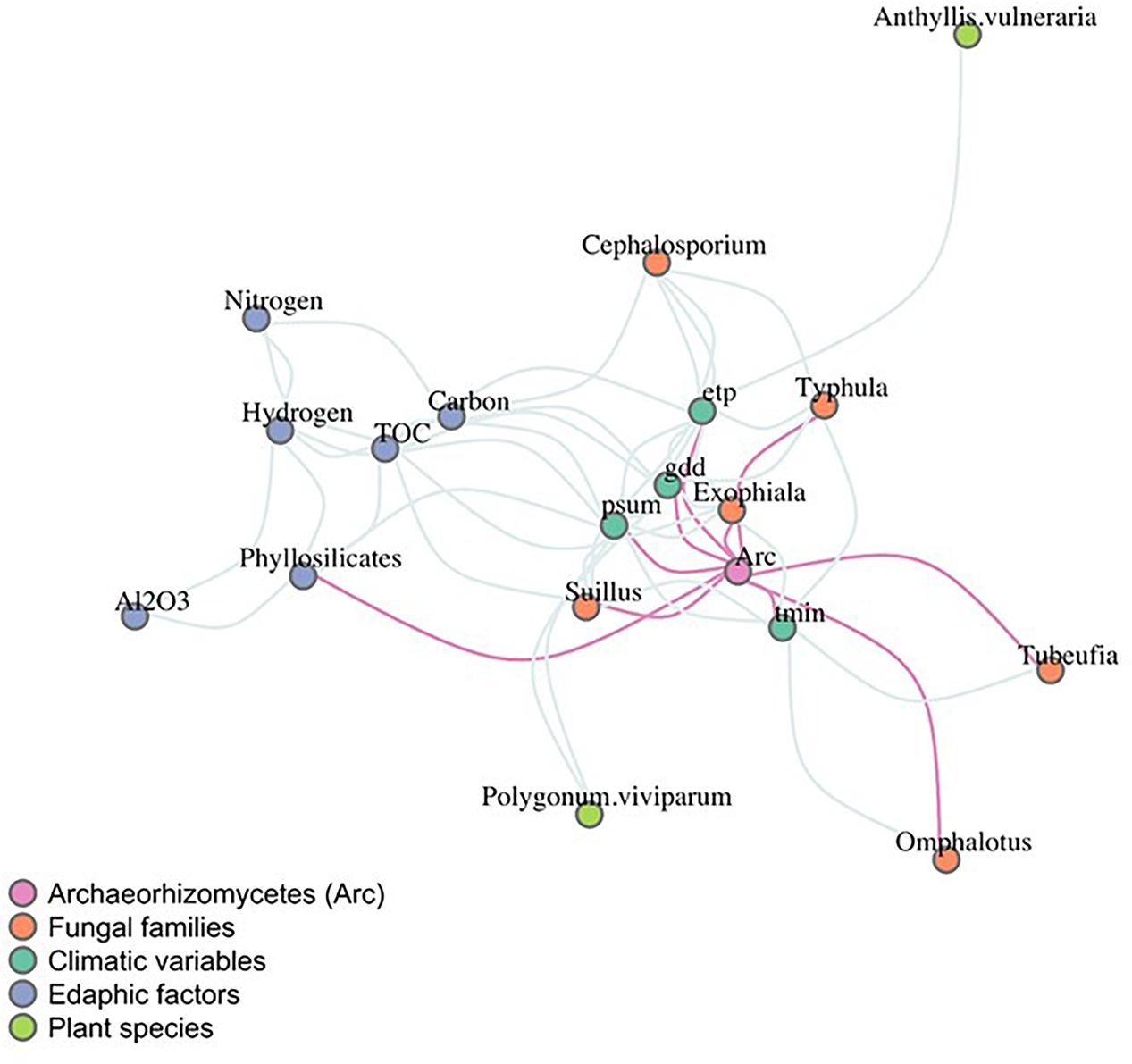
Figure 4. Inference network of co-occurrences between Archaeorhizomycetes, biotic, climatic, and abiotic variables. Nodes are colored according to the variable type as indicated in the color legend. Direct connections to Archaeorhizomycetes in pink represent the significant predicted relationships using the pseudo p-values. Tmin, minimum monthly average temperature; gdd, growing degree-days; etp, potential evapotranspiration; TOC, total organic carbon; psum, sum precipitation days/growing season.
Discussion
Soil fungi play fundamental roles in the functioning of terrestrial ecosystems (van der Heijden et al., 2008). Yet, the distribution and ecological role of most cryptic fungi are currently still under-determined (Tedersoo et al., 2014; Choma et al., 2016), limiting a more comprehensive understanding of natural soil ecosystems. The ancient fungal class Archaeorhizomycetes, one of the most ubiquitous and abundant cryptic groups of soil fungi, is described to have root endophytic properties and saprotrophic potential in the lab (Rosling et al., 2011). However, their precise environmental requirements and their ecological role in natural ecosystems remained largely unexplored. Here, we used a multivariate framework to explore and quantify the abiotic and biotic environmental requirements of the fungal class of Archaeorhizomycetes, and at least of the single Archaeorhizomyces genus it contains.
Our study revealed three major findings. First, Archaeorhizomycetes abundances are not evenly distributed across the elevation gradient, and thus in geographic space as well, but rather follow a “humped-shaped” relationship along elevation (Figure 1). Second, the environmental requirements of Archaeorhizomycetes are defined by different climatic (notably: minimum temperature, evapotranspiration, growing degree-days and sum precipitation) and edaphic factors (in particular: carbon, nitrogen, hydrogen, total organic carbon, aluminum oxide and phyllosilicates) (Table 1 and Supplementary Table S9). Third, network inference further revealed significant correlations between Archaeorhizomycetes, fungal saprophytes belonging to the genera Typhula and Exophiala, and the ectomycorrhizal Suillus. Overall, these results strongly support our hypothesis that the spatial variation in Archaeorhizomycetes abundance is at least partly driven by environmental determinism along wide abiotic gradients and by positive or negative associations with other fungal taxa. Unexpectedly, significant correlations to soil redox parameters, in particular with phyllosilicates minerals, suggest a potential involvement of Archaeorhizomycetes in rocks’ biological weathering. This would, however, require in vitro experiments (e.g., as suggested in Rosling et al., 2009) to be formally demonstrated.
Archaeorhizomycetes represented around 13% of the total referenced OTUs across the alpine landscape. This suggests that Archaeorhizomycetes are indeed important members of diverse terrestrial ecosystems, as concluded from previous studies (Rosling et al., 2011, 2013; Menkis et al., 2014). The maximum relative abundance of Archaeorhizomycetes among the sites sampled here was found at a mid to high elevations (1800–2400 m), suggesting that fungi in this group prefer environmental conditions found in subalpine and alpine vegetation belts. Although Archaeorhizomycetes have an apparent wide distribution (Rosling et al., 2013), our data thus indicate that particular environmental preferences can still be identified. From our sampling of open-habitats only, our results confirm that Archaeorhizomycetes accommodate well in treeless soil conditions with low temperatures, as previously shown for tundra biomes (Schadt et al., 2003; Borg Dahl et al., 2017). However, as Archaeorhizomycetes were also observed in boreal (Clemmensen et al., 2015) and cold Nothofagus forests (Fernández-Martínez et al., 2017), additional sampling within forests should be conducted within our study area to assess whether they also colonize forest soils and in which specific forest types.
Different environmental variables contributed to explain part of the variation in the relative abundance of Archaeorhizomycetes, and thus to define their environmental requirements in this region along the abiotic gradients considered. Notably, the climatic variables growing degree-days, minimum temperature, and precipitation correlated strongly with observed Archaeorhizomycetes abundances, suggesting that, in our open mountain habitats, they prefer colder and more humid conditions (Table 1 and Figure 3D). Our finding is in accordance with previous descriptions of Archaeorhizomycetes as being spring seasonal in tundra environments (Schadt et al., 2003). Borg Dahl et al. (2017) also showed Archaeorhizomycetes in cold tundra environments to be affected by shading and warming. A positive correlation with total organic carbon suggests that Archaeorhizomycetes proliferate at a higher carbon content in the soil, and thus, potentially depend on surrounding organic carbon sources, as had been proposed initially (Rosling et al., 2011). Other studies confirmed their dominance in the rhizosphere with putatively higher nutrient turnovers and deeper soils (Clemmensen et al., 2015; Fernández-Martínez et al., 2017), as well as in soils with lower pH and more available phosphorus (Carrino-Kyker et al., 2016). Further notable significant associations with edaphic factors aluminum oxide (Al2O3), hydrogen, and particularly phyllosilicates suggest that Archaeorhizomycetes may play a role in biological weathering (although this has not yet been shown experimentally for this group; see, e.g., weathering reactions in Box 1 in Hoffland et al., 2004). The extent of fungal biological rock weathering has been increasingly studied (Landeweert et al., 2001; Hoffland et al., 2004; Gadd, 2007; Shashank et al., 2016), with different fungi having been described as excreting organic acids, phenolic compounds, or siderophores that dissolve minerals (Hoffland et al., 2004). Trees may further enhance the activity of fungi for mineral weathering (Quirk et al., 2012), but our samples excluded sites with trees and our data therefore cannot confirm or refute possible Archaeorhizomycetes associations with trees and enhanced weathering. This would be a suitable direction for future research in our area.
Significant positive, as well as negative correlations, between Archaeorhizomycetes’ and other fungal abundances were inferred from network analysis (Figures 2B, 4). One of the positively associated fungi, Typhula, consists of psychrophilic soil fungi with saprophytic and pathogenic activity on dormant plant hosts during winter (Chang et al., 2006). Other co-occurring fungi are Exophiala species, also described as having saprotrophic and potential pathogenic activity (Barnes et al., 2016). However, negatively correlated fungi, such as the Tubeufia and Omphalotus genera, also include saprotrophic species (Fukasawa et al., 2010; Chaiwan et al., 2017). Archaeorhizomycetes were also negatively correlated to Suillus spp., described as ectomycorrhizal (Jarvis et al., 2015) and implicated in weathering processes (Hoffland et al., 2004; for more details on the correlated fungi see Supplementary Table S6). These correlations with other fungal taxa suggest that Archaeorhizomycetes might benefit in some cases from carbohydrates or nutrients released from organic matter according to substrate-dependent vertical partitioning of the resources across soil horizons. As different fungal species were shown to have coinciding fundamental niches, their realized niches could differ with respect to competition for nutrients (Gadgil and Gadgil, 1971; Bending, 2003; Lekberg et al., 2007; Bödeker et al., 2016) and lead them to occupy different soil depths (i.e., “niche” partitioning). This idea could also be tested in our area in further experimental approaches of combined consumption of organic matter by saprotrophic and ectomycorrhizal fungi, as suggested by Bödeker et al. (2016). We did not find any significant correlations between plant and Archaeorhizomycetes distributions, suggesting that they might not have specific root-associations as specific plant colonizers, at least not across the range of open grassland-type habitats investigated here. This is in contrast to studies describing potential associations of Archaeorhizomycetes with plant growth (Schadt et al., 2003) and thus would need to be further investigated. An interesting avenue to further explore could be whether distinct plants in different vegetation states along a successional gradient (e.g., from pioneer to late successional) have active root associations with Archaeorhizomycetes.
Although an increasing number of studies have proposed MiSeq Illumina for fungi in recent years (Schmidt et al., 2013; Bálint et al., 2014), at the time of our study we only had access to HiSeq Illumina 2500. Even though this technology is recognized for its great depth coverage (Taberlet et al., 2012), at that time it only allowed amplification of 100 bases forward and 100 bases reverse (although this has recently improved significantly). To counter that and to reference as many fungal sequences as possible, we decided to adapt the fungal reference dataset UNITE 12.11 and to use a hybrid method which generates both referenced and de novo OTUs (for more details about the strategy, see Supplementary Figures S1–S3). We recognize the high read recovery and later low usability of the HiSeq Illumina reads, in our case from 46.9 million high-quality reads, we used only 2.2 million after rarefaction (i.e., 20-fold factor). This data reduction came from the experimental decision to include in the rarefaction procedure (Gotelli and Chao, 2013) the sampling sites with very low sequencing reads (Supplementary Table S5). Despite this, rarefaction curves at 21,500 sequences showed that we managed to cover most of the fungal diversity across the study area (Supplementary Figure S9), as also shown by the Procrustes analysis plots comparing the non-metric multidimensional scaling (NMDS) topologies from de novo and referenced OTUs (Supplementary Figure S5). Here, we observed that de novo and referenced OTU picking correlate in almost 97% of cases, suggesting that de novo OTU clustering is highly dependent on the reference dataset (Rideout et al., 2014) and technically a pseudo de novo clustering strategy. For this reason, our analyses were performed only on the referenced OTUs. At the same time, we acknowledge that we may have underexplored the fungal diversity, which could have altered specific observations and conclusions made in this study. Indeed, the trimming of HiSeq sequences to 92 nt and their concatenation (184 nt overall), might have affected the OTU identification and/or underestimated the overall abundance of ITS1 regions shorter than 184 nt. Nevertheless, the length of the concatenated sequence is long enough to cover that of described ITS1 Archaeorhizomycetes sequence – A. borealis (192 nt; Menkis et al., 2014) and A. finlayi (183 nt; Schoch et al., 2014). This is supported by the findings of Tedersoo et al. (2015) who described a similar length for the variable region of ITS1 Archaeorhizomycetes. The representability of our dataset at Archaeorhizomycetes family level is supported by the diversity of Archaeorhizomycetes OTUs detected (n = 16).
We also acknowledge that the environmental envelope approach used here could be criticized for being applied to a whole fungal group. Based on current taxonomic knowledge, the Archaeorhizomycetes class includes so far only one genus Archaeorhizomyces (within a single family and single order; Rosling et al., 2011) and thus the approach used here can at least be considered reasonable at the genus level, where well-defined environmental requirements can already be identified in some cases (e.g., for plant, Androsace species being specialized in cold high-altitude or high-latitude climates; Boucher et al., 2012). Furthermore, environmental envelope studies are also found at coarser taxonomic levels (Smith et al., 2019), when for instance assessing niche conservatism across phylogenetic trees (Hadly et al., 2009). Another potential limitation when using environmental envelope approaches is the selection of the proper environmental variables in the model. Previous knowledge on the taxa is traditionally used to direct precise analyses (Austin, 2007; Mod et al., 2016; Petitpierre et al., 2017), but this information was absent in our study. Our idea instead was to reduce complexity by using pairwise Spearman rank correlations (Dormann et al., 2013). A limitation present in other studies is the use of presence-only or presence-absence data, rather than abundances, for quantifying and modeling environmental requirements and distributions of various organisms (e.g., plants, vertebrates and insects; Pellissier et al., 2012; Dubuis et al., 2013; Maiorano et al., 2013). It was, however, a strength of our study to use relative abundance from sequencing datasets directly, rather than transforming them into presence-absence data, which could have resulted in a loss of explanatory or predictive power. Still, environmental niche theory for microorganisms and the technical framework to use OTU relative abundance data in analyses and modeling are still in the early stages of development and would benefit from further improvements.
Finally, although our study identified several potential abiotic and biotic preferences of Archaeorhizomycetes (and here mainly of the Archaeorhizomyces genus), further support to these conclusions should come from experimental approaches (as, e.g., in Fukami and Morin, 2003, see Lee-Yaw et al., 2016), which could be optimally designed along environmental gradients based on our models and results. Likewise, our study shows the usefulness of large-scale DNA sets and next-generation amplicon sequencing as a template framework to uncover the ecological roles and environmental requirements of other cryptic soil organisms along wide natural environmental gradients, which for many are still uncharacterized (Choma et al., 2016; Hibbett, 2016; Peay et al., 2016). This is still of high importance because, despite significant advances in recent years regarding the descriptions of environmental niches for macroorganisms, still much remains to be known about the environmental requirements and niches of microorganisms, opening promising avenues for future research.
Author Contributions
AG, JvdM, and HN-H designed the initial soil metagenomics project in the Swiss Alps. EP-F, EY, AG, HN-H, and JvdM designed the initial sampling survey. EP-F and EY conceived the soil DNA sampling protocole, with AB for the soil physical properties. EP-F, ES, AG, and JvdM designed the specific analyses for this study. AB conducted all soil analyses. EP-F and EY performed the DNA extraction and ITS fungal Illumina pre-processing. EP-F performed the sequence data analyses for the determination of fungal OTUs. EP-F performed the correlative analyses and wrote the initial manuscript. All authors contributed to the interpretation of results and further refinements of the manuscript.
Funding
This study was supported by the Swiss National Science Foundation, grant numbers PDFMP3_135129 and 31003A-1528661, and by the European Commission FP7-PEOPLE-2010-IIF MP-Alps (grant agreement 273965).
Conflict of Interest Statement
The authors declare that the research was conducted in the absence of any commercial or financial relationships that could be construed as a potential conflict of interest.
Acknowledgments
We thank Anne Dubuis for advices regarding the sampling strategy, Jean Nicolas Pradervand, Wim Hordijk, and Madeleine Scriba for the support during the sampling campaign, Glacier 3000 and the TSP train (Aigle-Sépey-Diablerets) for support with the sampling logistic, Thierry Adatte and Jorge Spangenberg for helping AB use the soil analyses facilities, and Marco Pagni for the discussions about the open-reference strategy. Part of the computations were performed at the Vital-IT Center for high-performance computing (http://www.vital-it.ch) of the Swiss Institute of Bioinformatics (SIB).
Supplementary Material
The Supplementary Material for this article can be found online at: https://www.frontiersin.org/articles/10.3389/fmicb.2019.00656/full#supplementary-material
All embedded in one single document (Data Sheet 1.pdf).
Footnote
References
Araújo, M. B., and Guisan, A. (2006). Five (or so) challenges for species distribution modelling. J. Biogeogr. 33, 1677–1688. doi: 10.1111/j.1365-2699.2006.01584.x
Austin, M. (2007). Species distribution models and ecological theory: a critical assessment and some possible new approaches. Ecol. Modell. 200, 1–19. doi: 10.1016/j.ecolmodel.2006.07.005
Bálint, M., Schmidt, P.-A., Sharma, R., Thines, M., and Schmitt, I. (2014). An Illumina metabarcoding pipeline for fungi. Ecol. Evol. 4, 2642–2653. doi: 10.1002/ece3.1107
Banta, J. A., Ehrenreich, I. M., Gerard, S., Chou, L., Wilczek, A., Schmitt, J., et al. (2012). Climate envelope modelling reveals intraspecific relationships among flowering phenology, niche breadth and potential range size in Arabidopsis thaliana. Ecol. Lett. 15, 769–777. doi: 10.1111/j.1461-0248.2012.01796.x
Barnes, C. J., van der Gast, C. J., Burns, C. A., McNamara, N. P., and Bending, G. D. (2016). Temporally variable geographical distance effects contribute to the assembly of root-associated fungal communities. Front. Microbiol. 7:195. doi: 10.3389/fmicb.2016.00195
Becker, A., Körner, C., Brun, J.-J., Guisan, A., and Tappeiner, U. (2007). Ecological and land use studies along elevational gradients. Mt. Res. Dev. 27, 58–65. doi: 10.1659/0276-4741(2007)27[58:EALUSA]2.0.CO;2
Bending, G. D. (2003). Litter decomposition, ectomycorrhizal roots and the “Gadgil” effect. New Phytol. 158, 228–229. doi: 10.1046/j.1469-8137.2003.00753.x
Benjamini, Y., and Hochberg, Y. (1995). Controlling the false discovery rate: a practical and powerful approach to multiple testing. J. R. Stat. Soc. Ser. B 57, 289–300. doi: 10.1111/j.2517-6161.1995.tb02031.x
Bödeker, I. T. M., Lindahl, B. D., Olson,Å, and Clemmensen, K. E. (2016). Mycorrhizal and saprotrophic fungal guilds compete for the same organic substrates but affect decomposition differently. Funct. Ecol. 30, 1967–1978. doi: 10.1111/1365-2435.12677
Borg Dahl, M., Priemé, A., Brejnrod, A., Brusvang, P., Lund, M., Nymand, J., et al. (2017). Warming, shading and a moth outbreak reduce tundra carbon sink strength dramatically by changing plant cover and soil microbial activity. Sci. Rep. 7:16035. doi: 10.1038/s41598-017-16007-y
Boucher, F. C., Thuiller, W., Roquet, C., Douzet, R., Aubert, S., Alvarez, N., et al. (2012). Reconstructing the origins of high-alpine niches and cushion life form in the genus Androsace S.L. (Primulaceae). Evolution 66, 1255–1268. doi: 10.1111/j.1558-5646.2011.01483.x
Bryant, J. A., Lamanna, C., Morlon, H., Kerkhoff, A. J., Enquist, B. J., and Green, J. L. (2008). Microbes on mountainsides: contrasting elevational patterns of bacterial and plant diversity. Proc. Natl. Acad. Sci. U.S.A. 105, 11505–11511. doi: 10.1073/pnas.0801920105
Buée, M., Courty, P. E., Mignot, D., and Garbaye, J. (2007). Soil niche effect on species diversity and catabolic activities in an ectomycorrhizal fungal community. Soil Biol. Biochem. 39, 1947–1955. doi: 10.1016/J.SOILBIO.2007.02.016
Buri, A. (2014). Predicting Plant Distribution: Does Edaphic Factor Matter?. Master’s thesis, University of Lausanne, Lausanne.
Buri, A., Cianfrani, C., Pinto-Figueroa, E., Yashiro, E., Spangenberg, J. E., Adatte, T., et al. (2017). Soil factors improve predictions of plant species distribution in a mountain environment. Prog. Phys. Geogr. 41, 703–722. doi: 10.1177/0309133317738162
Burnham, K. P., and Anderson, D. R. (2004). Model selection and multimodel inference: a practical information-theoretic approach. Ecol. Modell. 172, 96–97. doi: 10.1016/j.ecolmodel.2003.11.004
Caporaso, J. G., Kuczynski, J., Stombaugh, J., Bittinger, K., Bushman, F. D., Costello, E. K., et al. (2010). QIIME allows analysis of high- throughput community sequencing data. Nat. Methods 7, 335–336. doi: 10.1038/nmeth0510-335
Carrino-Kyker, S. R., Kluber, L. A., Petersen, S. M., Coyle, K. P., Hewins, C. R., DeForest, J. L., et al. (2016). Mycorrhizal fungal communities respond to experimental elevation of soil pH and P availability in temperate hardwood forests. FEMS Microbiol. Ecol. 92, 1–19. doi: 10.1093/femsec/fiw024
Chaiwan, N., Lu, Y., Tibpromma, S., Bhat, D., Hyde, K., and Boonmee, S. (2017). Neotubeufia gen. nov. and Tubeufia guangxiensis sp. nov. (Tubeufiaceae) from freshwater habitats. Mycosphere 8, 1443–1456. doi: 10.5943/mycosphere/8/9/9
Chang, S. W., Scheef, E., Abler, R. A. B., Thomson, S., Johnson, P., and Jung, G. (2006). Distribution of Typhula spp. and Typhula ishikariensis varieties in Wisconsin, Utah, Michigan, and Minnesota. Phytopathology 96, 926–933. doi: 10.1094/PHYTO-96-0926
Choma, M., Bárta, J., Šantrůčková, H., and Urich, T. (2016). Low abundance of Archaeorhizomycetes among fungi in soil metatranscriptomes. Sci. Rep. 6:38455. doi: 10.1038/srep38455
Clarke, K. R., and Ainsworth, M. (1993). A method of linking multivariate community structure to environmental variables. Mar. Ecol. Prog. Ser. 92, 205–219. doi: 10.3354/meps092205
Clemmensen, K. E., Finlay, R. D., Dahlberg, A., Stenlid, J., Wardle, D. A., and Lindahl, B. D. (2015). Carbon sequestration is related to mycorrhizal fungal community shifts during long-term succession in boreal forests. New Phytol. 205, 1525–1536. doi: 10.1111/nph.13208
Csárdi, G., and Nepusz, T. (2006). The igraph software package for complex network research. InterJournal Complex Syst. 1695, 1–9.
Descombes, P., Marchon, J., Pradervand, J.-N., Bilat, J., Guisan, A., Rasmann, S., et al. (2017). Community-level plant palatability increases with elevation as insect herbivore abundance declines. J. Ecol. 105, 142–151. doi: 10.1111/1365-2745.12664
Descombes, P., Pradervand, J.-N., Golay, J., Guisan, A., and Pellissier, L. (2016). Simulated shifts in trophic niche breadth modulate range loss of alpine butterflies under climate change. Ecography 39, 796–804. doi: 10.1111/ecog.01557
Dickie, I. A. (2007). Host preference, niches and fungal diversity. New Phytol. 174, 230–233. doi: 10.1111/j.1469-8137.2007.02055.x
Dickie, I. A., Xu, B., and Koide, R. T. (2002). Vertical niche differentiation of ectomycorrhizal hyphae in soil as shown by T-RFLP analysis. New Phytol. 156, 527–535. doi: 10.2307/1513974
Dormann, C. F., Elith, J., Bacher, S., Buchmann, C., Carl, G., Carré, G., et al. (2013). Collinearity: a review of methods to deal with it and a simulation study evaluating their performance. Ecography 36, 27–46. doi: 10.1111/j.1600-0587.2012.07348.x
Dubuis, A., Giovanettina, S., Pellissier, L., Pottier, J., Vittoz, P., and Guisan, A. (2013). Improving the prediction of plant species distribution and community composition by adding edaphic to topo-climatic variables. J. Veg. Sci. 24, 593–606. doi: 10.1111/jvs.12002
Dubuis, A., Pottier, J., Rion, V., Pellissier, L., Theurillat, J.-P., and Guisan, A. (2011). Predicting spatial patterns of plant species richness: a comparison of direct macroecological and species stacking modelling approaches. Divers. Distrib. 17, 1122–1131. doi: 10.1111/j.1472-4642.2011.00792.x
Edgar, R. C., Haas, B. J., Clemente, J. C., Quince, C., and Knight, R. (2011). UCHIME improves sensitivity and speed of chimera detection. Bioinformatics 27, 2194–2200. doi: 10.1093/bioinformatics/btr381
Fernández-Martínez, M. A., Pérez-Ortega, S., Pointing, S. B., Allan Green, T. G., Pintado, A., Rozzi, R., et al. (2017). Microbial succession dynamics along glacier forefield chronosequences in Tierra del Fuego (Chile). Polar Biol. 40, 1939–1957. doi: 10.1007/s00300-017-2110-7
Fierer, N., Mccain, C. M., Meir, P., Zimmermann, M., Rapp, J. M., Silman, M. R., et al. (2011). Microbes do not follow the elevational diversity patterns of plants and animals. Ecology 92, 797–804. doi: 10.1890/10-1170.1
Frac, M., Hannula, S. E., Bełka, M., and Jȩdryczka, M. (2018). Fungal biodiversity and their role in soil health. Front. Microbiol. 9:707. doi: 10.3389/fmicb.2018.00707
Friedman, J., and Alm, E. J. (2012). Inferring correlation networks from genomic survey data. PLoS Comput. Biol. 8:e1002687. doi: 10.1371/journal.pcbi.1002687
Fukami, T., and Morin, P. J. (2003). Productivity-biodiversity relationships depend on the history of community assembly. Nature 424, 423–426. doi: 10.1038/nature01795
Fukasawa, Y., Takashi, O., and Takeda, H. (2010). Beech log decomposition by wood-inhabiting fungi in a cool temperate forest floor: a quantitative analysis focused on the decay activity of a dominant basidiomycete Omphalotus guepiniformis. Ecol. Res. 25, 959–966. doi: 10.1007/s11284-010-0720-4
Gadd, G. M. (2007). Geomycology: biogeochemical transformations of rocks, minerals, metals and radionuclides by fungi, bioweathering and bioremediation. Mycol. Res. 111, 3–49. doi: 10.1016/J.MYCRES.2006.12.001
Gadgil, R. L., and Gadgil, P. D. (1971). Mycorrhiza and litter decomposition. Nature 233:133. doi: 10.1038/233133a0
Gotelli, N. J., and Chao, A. (2013). “Measuring and Estimating Species Richness, Species Diversity, and Biotic Similarity from Sampling Data,” in Encyclopedia of Biodiversity, ed. S. A. Levin (Waltham, MA: Academic Press), 195–211. doi: 10.1016/B978-0-12-384719-5.00424-X
Guisan, A., Thuiller, W., and Zimmermann, N. E. (2017). Habitat Suitability and Distribution Models: With Applications in R. Cambridge: Cambridge University Press. doi: 10.1017/9781139028271
Hadly, E. A., Spaeth, P. A., and Li, C. (2009). Niche conservatism above the species level. Proc. Natl. Acad. Sci. U.S.A. 106, 19707–19714. doi: 10.1073/pnas.0901648106
Hibbett, D. (2016). The invisible dimension of fungal diversity. Science 351, 1150–1151. doi: 10.1126/science.aae0380
Hirzel, A., and Guisan, A. (2002). Which is the optimal sampling strategy for habitat suitability modelling. Ecol. Modell. 157, 331–341. doi: 10.1016/S0304-3800(02)00203-X
Hoffland, E., Kuyper, T. W., Wallander, H., Plassard, C., Gorbushina, A. A., Haselwandter, K., et al. (2004). The role of fungi in weathering. Front. Ecol. Environ. 2, 258–264. doi: 10.1890/1540-92952004002
Hughes Martiny, J. B., Bohannan, B. J. M., Brown, J. H., Colwell, R. K., Fuhrman, J. A., Green Jessica, L., et al. (2006). Microbial biogeography: putting microorganisms on the map. Nat. Rev. Microbiol. 4, 102–112. doi: 10.1038/nrmicro1341
Hutchinson, G. E. (1957). Concluding remarks. Cold Spring Harb. Symp. Quant. Biol. 22, 415–427. doi: 10.1101/SQB.1957.022.01.039
Jarvis, S. G., Woodward, S., and Taylor, A. F. S. (2015). Strong altitudinal partitioning in the distributions of ectomycorrhizal fungi along a short (300 m) elevation gradient. New Phytol. 206, 1145–1155. doi: 10.1111/nph.13315
Landeweert, R., Hoffland, E., Finlay, R. D., Kuyper, T. W., and Van Breemen, N. (2001). Linking plants to rocks: ectomycorrhizal fungi mobilize nutrients from minerals. Trends Ecol. Evol. 16, 248–254. doi: 10.1016/S0169-5347(01)02122-X
Lee-Yaw, J. A., Kharouba, H. M., Bontrager, M., Mahony, C., Csergo, A. M., Noreen, A. M. E., et al. (2016). A synthesis of transplant experiments and ecological niche models suggests that range limits are often niche limits. Ecol. Lett. 19, 710–722. doi: 10.1111/ele.12604
Lekberg, Y., Koide, R. T., Rohr, R. J., Aldrich-Wolfe, L., and Morton, J. B. (2007). Role of niche restrictions and dispersal in the composition of arbuscular mycorrhizal fungal communities. J. Ecol. 95, 95–105. doi: 10.1111/j.1365-2745.2006.01193.x
Levy-Booth, D. J., Giesbrecht, I. J. W., Kellogg, C. T. E., Heger, T. J., D’Amore, D. V., Keeling, P. J., et al. (2018). Seasonal and ecohydrological regulation of active microbial populations involved in DOC, CO2, and CH4 fluxes in temperate rainforest soil. ISME J. doi: 10.1038/s41396-018-0334-3 [Epub ahead of print].
Maiorano, L., Amori, G., Capula, M., Falcucci, A., Masi, M., Montemaggiori, A., et al. (2013). Threats from climate change to terrestrial vertebrate hotspots in Europe. PLoS One 8:e74989. doi: 10.1371/journal.pone.0074989
Martínez-Meyer, E., Díaz-Porras, D., Peterson, A. T., and Yáñez-Arenas, C. (2013). Ecological niche structure and rangewide abundance patterns of species. Biol. Lett. 9:20120637. doi: 10.1098/rsbl.2012.0637
McCullagh, P., and Nelder, J. A. (1989). Generalized Linear Models, 2nd Edn. London: Chapman and Hall. doi: 10.1007/978-1-4899-3242-6
Menkis, A., Urbina, H., James, T. Y., and Rosling, A. (2014). Archaeorhizomyces borealis sp. nov. and a sequence-based classification of related soil fungal species. Fungal Biol. 118, 943–955. doi: 10.1016/J.FUNBIO.2014.08.005
Mod, H. K., Scherrer, D., Luoto, M., and Guisan, A. (2016). What we use is not what we know: environmental predictors in plant distribution models. J. Veg. Sci. 27, 1308–1322. doi: 10.1111/jvs.12444
Ndiribe, C., Pellissier, L., Antonelli, S., Dubuis, A., Pottier, J., Vittoz, P., et al. (2013). Phylogenetic plant community structure along elevation is lineage specific. Ecol. Evol. 3, 4925–4939. doi: 10.1002/ece3.868
Nelder, J. A., and Wedderburn, R. W. M. (1972). Generalized linear models. J. R. Stat. Soc. A. 135, 370–384. doi: 10.2307/2344614
Pagni, M., Niculita-Hirzel, H., Pellissier, L., Dubuis, A., Xenarios, I., Guisan, A., et al. (2013). Density-based hierarchical clustering of pyro-sequences on a large scale—the case of fungal ITS1. Bioinformatics 29, 1268–1274. doi: 10.1093/bioinformatics/btt149
Pearman, P. B., D’amen, M., Graham, C. H., Thuiller, W., and Zimmermann, N. E. (2010). Within-taxon niche structure: niche conservatism, divergence and predicted effects of climate change. Ecography 33, 990–1003. doi: 10.1111/j.1600-0587.2010.06443.x
Peay, K. G., Kennedy, P. G., and Talbot, J. M. (2016). Dimensions of biodiversity in the Earth mycobiome. Nat. Rev. Microbiol. 14, 434–447. doi: 10.1038/nrmicro.2016.59
Pellissier, L., Niculita-Hirzel, H., Dubuis, A., Pagni, M., Guex, N., Ndiribe, C., et al. (2014). Soil fungal communities of grasslands are environmentally structured at a regional scale in the Alps. Mol. Ecol. 23, 4274–4290. doi: 10.1111/mec.12854
Pellissier, L., Pinto-Figueroa, E., Niculita-Hirzel, H., Moora, M., Villard, L., Goudet, J., et al. (2013). Plant species distributions along environmental gradients: do belowground interactions with fungi matter? Front. Plant Sci. 4:500. doi: 10.3389/fpls.2013.00500
Pellissier, L., Pradervand, J.-N., Pottier, J., Dubuis, A., Maiorano, L., and Guisan, A. (2012). Climate-based empirical models show biased predictions of butterfly communities along environmental gradients. Ecography 35, 1–9. doi: 10.2307/23258172
Petitpierre, B., Broennimann, O., Kueffer, C., Daehler, C., and Guisan, A. (2017). Selecting predictors to maximize the transferability of species distribution models: lessons from cross-continental plant invasions. Glob. Ecol. Biogeogr. 26, 275–287. doi: 10.1111/geb.12530
Porter, T. M., Schadt, C. W., Rizvi, L., Martin, A. P., Schmidt, S. K., Scott-Denton, L., et al. (2008). Widespread occurrence and phylogenetic placement of a soil clone group adds a prominent new branch to the fungal tree of life. Mol. Phylogenet. Evol. 46, 635–644. doi: 10.1016/j.ympev.2007.10.002
Pottier, J., Dubuis, A., Pellissier, L., Maiorano, L., Rossier, L., Randin, C. F., et al. (2013). The accuracy of plant assemblage prediction from species distribution models varies along environmental gradients. Glob. Ecol. Biogeogr. 22, 52–63. doi: 10.1111/j.1466-8238.2012.00790.x
Pulliam, H. R. (2000). On the relationship between niche and distribution. Ecol. Lett. 3, 349–361. doi: 10.1046/j.1461-0248.2000.00143.x
Quirk, J., Beerling, D. J., Banwart, S. A., Kakonyi, G., Romero-Gonzalez, M. E., and Leake, J. R. (2012). Evolution of trees and mycorrhizal fungi intensifies silicate mineral weathering. Biol. Lett. 8, 1006–1011. doi: 10.1098/rsbl.2012.0503
R Development Core Team (2015). R: A Language and Environment for Statistical Computing. Vienna: R Foundation for Statistical Computing.
Rideout, J. R., He, Y., Navas-Molina, J., Walters, W., Ursell, L. K., Gibbons, S. M., et al. (2014). Subsampled open-reference clustering creates consistent, comprehensive OTU definitions and scales to billions of sequences. PeerJ 2:e545. doi: 10.7717/peerj.545
Rincón, A., Santamaría-Pérez, B., Rabasa, S. G., Coince, A., Marçais, B., and Buée, M. (2015). Compartmentalized and contrasted response of ectomycorrhizal and soil fungal communities of Scots pine forests along elevation gradients in France and Spain. Environ. Microbiol. 17, 3009–3024. doi: 10.1111/1462-2920.12894
Rosling, A., Cox, F., Cruz-Martinez, K., Ihrmark, K., Grelet, G.-A., Lindahl, B. D., et al. (2011). Archaeorhizomycetes: unearthing an ancient class of ubiquitous soil fungi. Science 333, 876–879. doi: 10.1126/science.1206958
Rosling, A., Roose, T., Herrmann, A. M., Davidson, F. A., Finlay, R. D., and Gadd, G. M. (2009). Approaches to modelling mineral weathering by fungi. Fungal Biol. Rev. 23, 138–144. doi: 10.1016/j.fbr.2009.09.003
Rosling, A., Timling, I., and Taylor, D. L. (2013). “Archaeorhizomycetes: patterns of distribution and abundance in soil,” in Genomics of Soil- and Plant-Associated Fungi. Soil Biology, eds B. Horwitz, P. Mukherjee, M. Mukherjee, and C. Kubicek (Heidelberg: Springer), 333–349. doi: 10.1007/978-3-642-39339-6
Schadt, C. W., Martin, A. P., Lipson, D. A., and Schmidt, S. K. (2003). Seasonal dynamics of previously unknown fungal lineages in tundra soils. Science 301, 1359–1361. doi: 10.1126/science.1086940
Schmidt, P.-A., Bálint, M., Greshake, B., Bandow, C., Römbke, J., and Schmitt, I. (2013). Illumina metabarcoding of a soil fungal community. Soil Biol. Biochem. 65, 128–132. doi: 10.1016/j.soilbio.2013.05.014
Schoch, C. L., Robbertse, B., Robert, V., Vu, D., Cardinali, G., Irinyi, L., et al. (2014). Finding needles in haystacks: linking scientific names, reference specimens and molecular data for Fungi. Database 2014:bau061. doi: 10.1093/database/bau061
Shashank, B. S., Sharma, S., Sowmya, S., Latha, R. A., Meenu, P. S., and Singh, D. N. (2016). State-of-the-art on geotechnical engineering perspective on bio-mediated processes. Environ. Earth Sci. 75:270. doi: 10.1007/s12665-015-5071-6
Shen, C., Liang, W., Shi, Y., Lin, X., Zhang, H., Wu, X., et al. (2014). Contrasting elevational diversity patterns between eukaryotic soil microbes and plants. Ecology 95, 3190–3202. doi: 10.1890/14-0310.1
Smith, A. B., Godsoe, W., Rodríguez-Sánchez, F., Wang, H.-H., and Warren, D. (2019). Niche estimation above and below the species level. Trends Ecol. Evol. 34, 260–273. doi: 10.1016/j.tree.2018.10.012
Soberón, J., and Nakamura, M. (2009). Niches and distributional areas: concepts, methods, and assumptions. Proc. Natl. Acad. Sci. U.S.A. 106, 19644–19650. doi: 10.1073/pnas.0901637106
Taberlet, P., Coissac, E., Pompanon, F., Brochmann, C., and Willerslev, E. (2012). Towards next-generation biodiversity assessment using DNA metabarcoding. Mol. Ecol. 21, 2045–2050. doi: 10.1111/j.1365-294X.2012.05470.x
Taylor, D. L., Hollingsworth, T. N., McFarland, J. W., Lennon, N. J., Nusbaum, C., and Ruess, R. W. (2014). A first comprehensive census of fungi in soil reveals both hyperdiversity and fine-scale niche partitioning. Ecol. Monogr. 84, 3–20. doi: 10.1890/12-1693.1
Tedersoo, L., Anslan, S., Bahram, M., Põlme, S., Riit, T., Liiv, I., et al. (2015). Shotgun metagenomes and multiple primer pair-barcode combinations of amplicons reveal biases in metabarcoding analyses of fungi. MycoKeys 10, 1–43. doi: 10.3897/mycokeys.10.4852
Tedersoo, L., Bahram, M., Põlme, S., Kõljalg, U., Yorou, N., Wijesundera, R., et al. (2014). Global diversity and geography of soil fungi. Science 346, 1078–1097. doi: 10.1126/science.aaa1185
Tedersoo, L., Bahram, M., Toots, M., Diédhiou, A. G., Henkel, T. W., Kjøller, R., et al. (2012). Towards global patterns in the diversity and community structure of ectomycorrhizal fungi. Mol. Ecol. 21, 4160–4170. doi: 10.1111/j.1365-294X.2012.05602.x
Tedersoo, L., May, T. W., and Smith, M. E. (2010). Ectomycorrhizal lifestyle in fungi: global diversity, distribution, and evolution of phylogenetic lineages. Mycorrhiza 20, 217–263. doi: 10.1007/s00572-009-0274-x
Thioulouse, J., Dray, S., Dufour, A.-B., Siberchicot, A., Jombart, T., and Pavoine, S. (2018). Multivariate Analysis of Ecological Data With ade4. New York, NY: Springer-Verlag, doi: 10.1007/978-1-4939-8850-1
Urbina, H., Breed, M. F., Zhao, W., Lakshmi Gurrala, K., Andersson, S. G. E., Ågren, J., et al. (2018). Specificity in Arabidopsis thaliana recruitment of root fungal communities from soil and rhizosphere. Fungal Biol. 122, 231–240. doi: 10.1016/j.funbio.2017.12.013
van der Heijden, M. G., Bruin, S., De Luckerhoff, L., van Logtestijn, R. S., and Schlaeppi, K. (2015). A widespread plant-fungal-bacterial symbiosis promotes plant biodiversity, plant nutrition and seedling recruitment. ISME J. 10, 1–11. doi: 10.1038/ismej.2015.120
van der Heijden, M. G. A., Bardgett, R. D., and van Straalen, N. M. (2008). The unseen majority: soil microbes as drivers of plant diversity and productivity in terrestrial ecosystems. Ecol. Lett. 11, 296–310. doi: 10.1111/j.1461-0248.2007.01139.x
van der Heijden, M. G. A., Klironomos, J. N., Ursic, M., Moutoglis, P., Streitwolf-Engel, R., Boller, T., et al. (1998). Mycorrhizal fungal diversity determines plant biodiversity, ecosystem variability and productivity. Nature 396, 69–72. doi: 10.1038/23932
Wang, J., Soininen, J., Zhang, Y., Wang, B., Yang, X., and Shen, J. (2012). Patterns of elevational beta diversity in micro-and macroorganisms. Glob. Ecol. Biogeogr. 21, 743–750. doi: 10.1111/j.1466-8238.2011.00718.x
Westcott, S. L., and Schloss, P. D. (2015). De novo clustering methods outperform reference-based methods for assigning 16S rRNA gene sequences to operational taxonomic units. PeerJ 3:e1487. doi: 10.7717/peerj.1487
White, T. J., Bruns, S., Lee, S., and Taylor, J. (1990). “Amplification and direct sequencing of fungal ribosomal RNA genes for phylogenetics,” in PCR Protocols: A Guide to Methods and Applications, eds M. A. Innis, D. H. Gelfand, J. J. Sninsky, and T. J. White (New York, NY: Academic Press, Inc), 315–322.
Wickham, H. (2009). ggplot2: Elegant Graphics for Data Analysis. New York, NY: Springer, doi: 10.1007/978-0-387-98141-3
Wiens, J. J., Ackerly, D. D., Allen, A. P., Anacker, B. L., Buckley, L. B., Cornell, H. V., et al. (2010). Niche conservatism as an emerging principle in ecology and conservation biology. Ecol. Lett. 13, 1310–1324. doi: 10.1111/j.1461-0248.2010.01515.x
Yashiro, E., Pinto-Figueroa, E., Buri, A., Spangenberg, J. E., Adatte, T., Niculita-Hirzel, H., et al. (2016). Local environmental factors drive divergent grassland soil bacterial communities in the western Swiss Alps. Appl. Environ. Microbiol. 82, 6303–6316. doi: 10.1128/AEM.01170-16
Yashiro, E., Pinto-Figueroa, E., Buri, A., Spangenberg, J. E., Adatte, T., Niculita-Hirzel, H., et al. (2018). Meta-scale mountain grassland observatories uncover commonalities as well as specific interactions among plant and non-rhizosphere soil bacterial communities. Sci. Rep. 8:5758. doi: 10.1038/s41598-018-24253-x
Keywords: Archaeorhizomyces, fungi, Illumina sequencing, abiotic and biotic requirements, correlation analyses, network analysis, phyllosilicates, Swiss Alps
Citation: Pinto-Figueroa EA, Seddon E, Yashiro E, Buri A, Niculita-Hirzel H, van der Meer JR and Guisan A (2019) Archaeorhizomycetes Spatial Distribution in Soils Along Wide Elevational and Environmental Gradients Reveal Co-abundance Patterns With Other Fungal Saprobes and Potential Weathering Capacities. Front. Microbiol. 10:656. doi: 10.3389/fmicb.2019.00656
Received: 11 July 2018; Accepted: 15 March 2019;
Published: 04 April 2019.
Edited by:
Rosa Margesin, University of Innsbruck, AustriaReviewed by:
Petra Fransson, Swedish University of Agricultural Sciences, SwedenAnna Rosling, Uppsala University, Sweden
Copyright © 2019 Pinto-Figueroa, Seddon, Yashiro, Buri, Niculita-Hirzel, van der Meer and Guisan. This is an open-access article distributed under the terms of the Creative Commons Attribution License (CC BY). The use, distribution or reproduction in other forums is permitted, provided the original author(s) and the copyright owner(s) are credited and that the original publication in this journal is cited, in accordance with accepted academic practice. No use, distribution or reproduction is permitted which does not comply with these terms.
*Correspondence: Antoine Guisan, antoine.guisan@unil.ch
†Co-last authorship