- 1School of Environmental Science and Engineering, Guangzhou University, Guangzhou, China
- 2Jiangsu Key Laboratory of Marine Bioresources and Environment, Jiangsu Ocean University, Lianyungang, China
- 3King Abdullah University of Science and Technology (KAUST), Biological and Environmental Sciences and Engineering Division (BESE), Thuwal, Saudi Arabia
Ocean acidification is recognized as a major anthropogenic perturbation of the modern ocean. While extensive studies have been carried out to explore the short-term physiological responses of phytoplankton to ocean acidification, little is known about their lipidomic responses after a long-term ocean acidification adaptation. Here we perform the lipidomic analysis of a marine diatom Phaeodactylum tricornutum following long-term (∼400 days) selection to ocean acidification conditions. We identified a total of 476 lipid metabolites in long-term high CO2 (i.e., ocean acidification condition) and low CO2 (i.e., ambient condition) selected P. tricornutum cells. Our results further show that long-term high CO2 selection triggered substantial changes in lipid metabolites by down- and up-regulating 33 and 42 lipid metabolites. While monogalactosyldiacylglycerol (MGDG) was significantly down-regulated in the long-term high CO2 selected conditions, the majority (∼80%) of phosphatidylglycerol (PG) was up-regulated. The tightly coupled regulations (positively or negatively correlated) of significantly regulated lipid metabolites suggest that the lipid remodeling is an organismal adaptation strategy of marine diatoms to ongoing ocean acidification. Since the composition and content of lipids are crucial for marine food quality, and these changes can be transferred to high trophic levels, our results highlight the importance of determining the long-term adaptation of lipids in marine producers in predicting the ecological consequences of climate change.
Introduction
Diatoms are responsible for ∼20% of global primary production and play crucial roles in carbon and silicon biogeochemical cycles (Field et al., 1998). Their fixed carbon is partitioned into either carbohydrates or lipids (Kroth et al., 2008). The lipids of diatoms consist of almost all lipid classes, including both polar lipids (Guschina and Harwood, 2006) and non-polar lipids [free fatty acids, sterols, glycerols and especially triacylglycerols (TAGs)]. TAGs of diatoms have been increasingly studied for their potential as nutritional sources and for biofuel production (Hildebrand et al., 2012; Shahidi and Ambigaipalan, 2018). Fatty acid profiles of diatoms are enriched with medium-chain and very long-chain polyunsaturated fatty acids (PUFAs), namely ω3 fatty acids such as eicosapentaenoic acid (EPA) (Dunstan et al., 1993; Guschina and Harwood, 2006). In aquatic food webs, PUFAs are exclusively synthesized by phytoplankton and cannot be synthesized de novo by metazoans, and therefore must be acquired by non-phytoplanktonic organisms via their diet (Hixson et al., 2015). As such, PUFAs are important determinants of food quality and, consequently, an important indicator for the health and optimal functioning of marine and freshwater food webs (Dalsgaard et al., 2003). The polar lipid fraction of diatoms mainly consists of digalactosyldiacylglycerol (DGDG), monogalactosyldiacylglycerol (MGDG), sulfoquino vosyldiacylglycerol (SQDG), phosphatidylinositol (PI), phosphatidylglycerol (PG), phosphatidylcholine (PC), and minor lipids such as betaine lipids (Stonik and Stonik, 2015).
Global change induces many alterations in marine environments, such as ocean acidification (Gattuso et al., 2015). The molecular, physiological, biochemical and ecological responses of diatoms to ocean acidification have been studied extensively in the last two decades (Gao et al., 2012; Hennon et al., 2015; Petrou et al., 2019; Shi et al., 2019; Li et al., 2021; see also comprehensive reviews by Gao and Campbell, 2014; Bach and Taucher, 2019 and references therein). It has been reported that the lipid contents and fatty acid compositions of diatoms are highly dependent on CO2 concentrations (Bermúdez et al., 2015; Wang et al., 2017; Abreu et al., 2020). For example, ocean acidification decreased the content of PUFAs in the marine diatom Cylindrotheca fusiformis by ∼3% (Bermúdez et al., 2015). The mechanisms through which CO2 affects the composition of fatty acids in microalgae are still unclear, however, it has been suggested that elevated CO2 can enhance the synthesis and accumulation of saturated fatty acids (Sato et al., 2003). This response reduces cell membrane fluidity, which helps the organism to cope with pH reductions and facilitates the regulation of cell homeostasis (Lane and Burris, 1981; Rossoll et al., 2012). More recently, it has been reported that the operation of carbon concentration mechanisms (CCMs) of microalgae are tightly coupled with their lipid metabolisms (Renberg et al., 2010; Abreu et al., 2020) and that the CCMs of diatoms are partially down-regulated under ocean acidification conditions (Trimborn et al., 2009; Wu et al., 2010; Hopkinson et al., 2011; Yang and Gao, 2012). The down-regulations of CCMs led to decreased photorespiration and symptoms of oxidative stress because of an increase in the electron sink constituted by CO2 fixation (Raven, 2010; Renberg et al., 2010). These changes consequently altered the composition of lipids (such as DGDG, MGDG, and triacylglycerol, given their importance for cell functioning, especially in stress-responses (e.g., oxidative stress) (Bréhélin et al., 2007; Abreu et al., 2020). However, most published studies have analyzed responses of lipid metabolisms in the short term only (i.e., 1–2 weeks), and were thus unable to resolve long-term responses to ocean acidification conditions. Since diatoms are characterized by large population sizes, standing genetic variations and short generation times (Reusch and Boyd, 2013; Collins et al., 2020), they have a high potential to adapt to acidifying oceans, as recently indicated (Li et al., 2017; Zhong et al., 2021).
To address this fundamental knowledge gap, we carried out a ∼400 days selection experiment with the model marine diatom Phaeodactylum tricornutum, and employed a lipidomics approach to investigate long-term responses in the lipid metabolism of diatoms to different emission scenarios associated with ocean acidification.
Materials and Methods
Culture Conditions
Cultures of Phaeodactylum tricornutum Bohlin bac-2, obtained from the Institute of Oceanology at the Chinese Academy of Sciences, were maintained in half-strength Guillard’s “F” solution (Guillard and Ryther, 1962). Prior to the long-term experiments, the cultures were kept in 15°C plant growth chambers (HP1000G-D, Ruihua) under a photon flux of 100 μmol photons m–2 s–1 with a light:dark cycle of 12 h:12 h (HP1000G-D, Ruihua). To initiate the long-term selection experiments, the single-clone cultures were diluted into triplicates (500 mL each) and grown at low CO2 (400 μatm, ambient CO2 condition, denoted LC) and high CO2 (1,000 μatm, projected year 2100 high CO2 according to the high emission scenario RCP 8.5, IPCC, 2014, denoted HC) levels. The LC condition was attained by pre-aerating the medium with the ambient outdoor air, while the HC treatment was achieved within a plant growth chamber (HP1000G-D, Ruihua). In the chamber, the target CO2 level of 1,000 μatm was obtained by mixing air and pure CO2 gas. The CO2 partial pressure was continuously monitored and maintained at 1,000 ± 50 μatm. Triplicate semi-continuous batch cultures were grown for ∼400 days under the two selection regimes (i.e., LC, HC). After the 400-days selection period, the cells have grown for approximately 885 and 883 generations under LC and HC conditions, respectively. The initial cell concentration was 50 cells mL–1 and the cell densities were maintained within a range of ∼4.0 × 104 to 5.0 × 105 cells mL–1 at the time of dilution. The cultures were inoculated every 5–7 days to restore the cell density to the initial level (i.e., batch growth cycle) with fresh medium equilibrated with the corresponding target CO2 levels. To main a stable carbonate chemistry over each batch growth cycle (pH variations < 0.1 units), the cultures were maintained in closed polycarbonate bottles that were completely filled with culture medium to prevent head space gas exchange. Instead of analyzing the carbonate chemistry parameters on a weekly basis for a ∼400 days experiment, we measured the parameters before running the long-term selection experiments to ensure the semi-continuous culture approaches are reliable (Jin et al., 2013). In the pilot experiment, pH and dissolved inorganic carbon (DIC) were measured before and after the renewal of the medium in LC and HC cultures of P. tricornutum.
Lipid Extraction
At the end of the long-term selection experiment, six replicate samples (n = 6) of the HC- and LC-selected cells were collected in the middle of the photoperiod, centrifuged (8,000 g, 10 min), flash frozen in liquid nitrogen, and stored at −80°C until further analysis. The pellets of freeze-dried cells were placed into a glass tube with a Teflon lined cap, and extracted in 0.75 mL methanol. Then, 2.5 mL of methyl tert-butyl ether (MTBE) was added and the mixture was incubated in a shaker at room temperature for 1 h. Phase separation was induced by adding 0.625 mL of UHPLC -grade water. After 10 min of incubation at room temperature, the sample was centrifuged at 1,000 g for 10 min. The upper organic phase was collected, while the lower phase was re-extracted with 1 mL of solvent mixture [MTBE/methanol/water (10:3:2.5, v/v/v)] and the resulting upper phase was collected again. The combined upper phases were dried with a Termovap sample concentrator (Ecom, Czechia). To speed up sample drying, 100 μL of MS-grade methanol was added to the upper phase after 25 min of centrifugation. Extracted lipids were dissolved in 100 μL CHCl3/ methanol/water (60:30:4.5, v/v/v) for storage until further analysis using liquid chromatography with tandem-mass spectrometry (LC-MS/MS).
Liquid Chromatography With Tandem-Mass Spectrometry Analysis
Liquid chromatography with tandem-mass spectrometry analysis was performed using a Thermo VanquishTM UHPLC (ThermoFisher Scientific). Samples were injected into a Thermo Accucore C30 column using a 20-min linear gradient at a flow rate of 0.35 mL min–1. The column temperature was set at 40°C. Mobile phase buffer A was acetonitrile / water (6/4) with 10 mM ammonium acetate and 0.1% formic acid, whereas buffer B was acetonitrile/isopropanol (1/9) with 10 mM ammonium acetate and 0.1% formic acid. The solvent gradient was set as follows: 30% B, initial; 43% B, 8 min; 50% B, 8.1 min; 70% B, 17 min; 99% B, 24 min; 30% B, 27.1 min; 30% B, 31 min. The MS condition was set as follows: Q-Exactive mass series spectrometer was selected in the m/z 114-1700 scanning range, and the MS/MS scan was used for a data-dependent full scan. The Q-Exactive mass series spectrometer was operated in negative polarity mode with a spray voltage of 3 kV, capillary temperature of 350°C, sheath gas flow of 20 arbitrary units and auxiliary gas flow of five arbitrary units.
Lipid Identification
The raw data files generated by the HPLC-MS/MS were processed using the software Compound Discoverer 3.0 (CD3.0, Thermo Fisher) to perform peak alignment, peak picking, and quantitation for each metabolite. The main parameters were set as follows: retention time tolerance: 0.2 min, actual mass tolerance: 5 ppm, signal intensity tolerance: 30%, signal/noise ratio: 3, and minimum intensity: 100,000. Peak intensities were normalized to the total spectral intensity. The normalized data were used to predict the molecular formula based on additive ions, molecular ion peaks and fragment ions. Peaks were matched with the databases LIPID MAPS1 and LipidBlast to obtain accurate qualitative and relative quantitative results. Then the identified metabolites were annotated using the Human Metabolome Database (HMDB)2 and LIPID MAPS database.
Quality Evaluation of Lipid Metabolites Data
Quality control samples (QCs) were obtained by collecting an equal amount of mixture from each replicate sample. The consistency analysis was performed between QCs and our culture samples. Before the analysis, three QCs were used to stabilize the analysis system and to remove the acquired data before data processing. All QCs were used to monitor the robustness of sample preparation and the stability of instrumental analysis by analyzing batch random inserts. During the whole instrumental analysis process, all samples were analyzed randomly to avoid inter-batch differences (Wang et al., 2015). In order to evaluate the overfitting of the model, 200 permutation tests were performed in the partial least squares discriminant analysis (PLS-DA) model (Broadhurst and Kell, 2006).
Statistical Analysis
After metabolic information collection and data pre-processing, the resulting matrix was imported into the software metaX (Wen et al., 2017) for unsupervised principal component analysis (PCA) and supervised PLS-DA (Roede et al., 2014). Identification of metabolites has a variable importance in the projection (VIP) graphs (99% confidence) (Roede et al., 2014). For each multivariate model, the calculated R2 value reflects the goodness of fit. The parameter Q2 in the PLS-DA represents the predictive ability of the model (Roede et al., 2014). A Q2 value close to 0.5 reflects a good model. We applied univariate analysis (t-test) to calculate the statistical significance (p-value). Metabolites with VIP > 1, p < 0.05 and fold change (FC) ≥ 2 or FC ≤ 0.5 were considered to be differential metabolites. Volcano plots were used to filter metabolites of interest, based on Log2 (FC) and -log10 (p-value) of metabolites. For clustering heat maps, the data were normalized using z-scores of the intensity areas of differential metabolites, and plotted using the pheatmap package in R (R version R.3.6.1) and TBtools (Chen et al., 2020). The correlations between differential metabolites were analyzed using the cor() function in R (method = Pearson). Statistical significances of correlation between differential metabolites were calculated by the function cor.mtest() in R. P-values < 0.05 were considered as statistically significant, and the correlation plots were generated using the corrplot package in R. The Kyoto Encyclopedia of Genes and Genome (KEGG) database was used for enrichment analysis and pathway analysis of differential metabolites. Chi-square test was used to test the differences in lipid metabolite compositions between long-term HC- and LC-selected cells.
Results
Sample Quality Control
The Pearson correlation coefficient between QC samples based on the peak area value found high correlation coefficients (∼0.99) of QC samples, which indicated a stability of the whole detection process and a high data quality (Supplementary Figure 1).
Overall Metabolites Description
We identified a total of 476 lipid metabolites in long-term HC- and LC-selected P. tricornutum cells (Supplementary Table 1). At the lipid category level, the majority of lipid metabolites were glycerophospholipids (GP) (49%), followed by glycerolipids (GL) (29%). Other lipid categories, such as sphingolipids (SP) and fatty acyls (FA), contributed relatively small proportions (SP: 15%; FA: 6%) in the detected lipid metabolites. At the lipid class level, the main lipid classes identified in long-term HC- and LC-selected P. tricornutum cells were glycosyldiradylglycerols, accounting for 27% of total lipid metabolites (Supplementary Table 1). Other identified lipid classes, such as glycerophosphoglycerols, glycerophosphocholines, and ceramides, represented 19, 15, and 13% of the total lipid metabolite pool (Supplementary Table 1).
Changes in Lipid Metabolites Between Long-Term HC- and LC-Selected Cells
The principal component analysis (PCA) of total lipid metabolites showed that PC1 and PC2 explain 43.4 and 31.1% of variation, respectively (Supplementary Figure 2). Although there were some variations among the six replicate samples due to the nature of the lipidomics analysis, our results showed there was a satisfactory separation of lipid metabolites between long-term HC- and LC-selected cells (Supplementary Figure 2). This discrimination was further evidenced by the PLS-DA scores plot (Supplementary Figure 3).
We found that of the identified 476 lipid metabolites, 74 significantly regulated different between long-term HC- and LC-selected cells (Table 1). Of those 74 lipid metabolites, 44 were significantly up-regulated, while the remaining 30 were significantly down-regulated in the long-term HC-selected cells compared to those under LC-selection (Table 1 and Figures 1, 2). Overall, the lipid classes MGDG (19%), HBMP (18%), PE (12%), and PG (12%) dominated in these 74 differentially regulated lipid metabolites (Table 1). However, the frequency of lipid classes differed significantly between down- and up-regulated lipid metabolites (χ2 = 65.317, p < 0.001, df = 16, n = 74) (Table 1). Specifically, MGDG (43%) was the most abundant in the down-regulated lipid metabolites, while HBMP (23%) and PE (20%) dominated in the up-regulated lipids (Table 1). We also found that the majority of the differently regulated PG lipids (seven out of nine) were up-regulated in long-term high CO2 selected cells (Table 1).
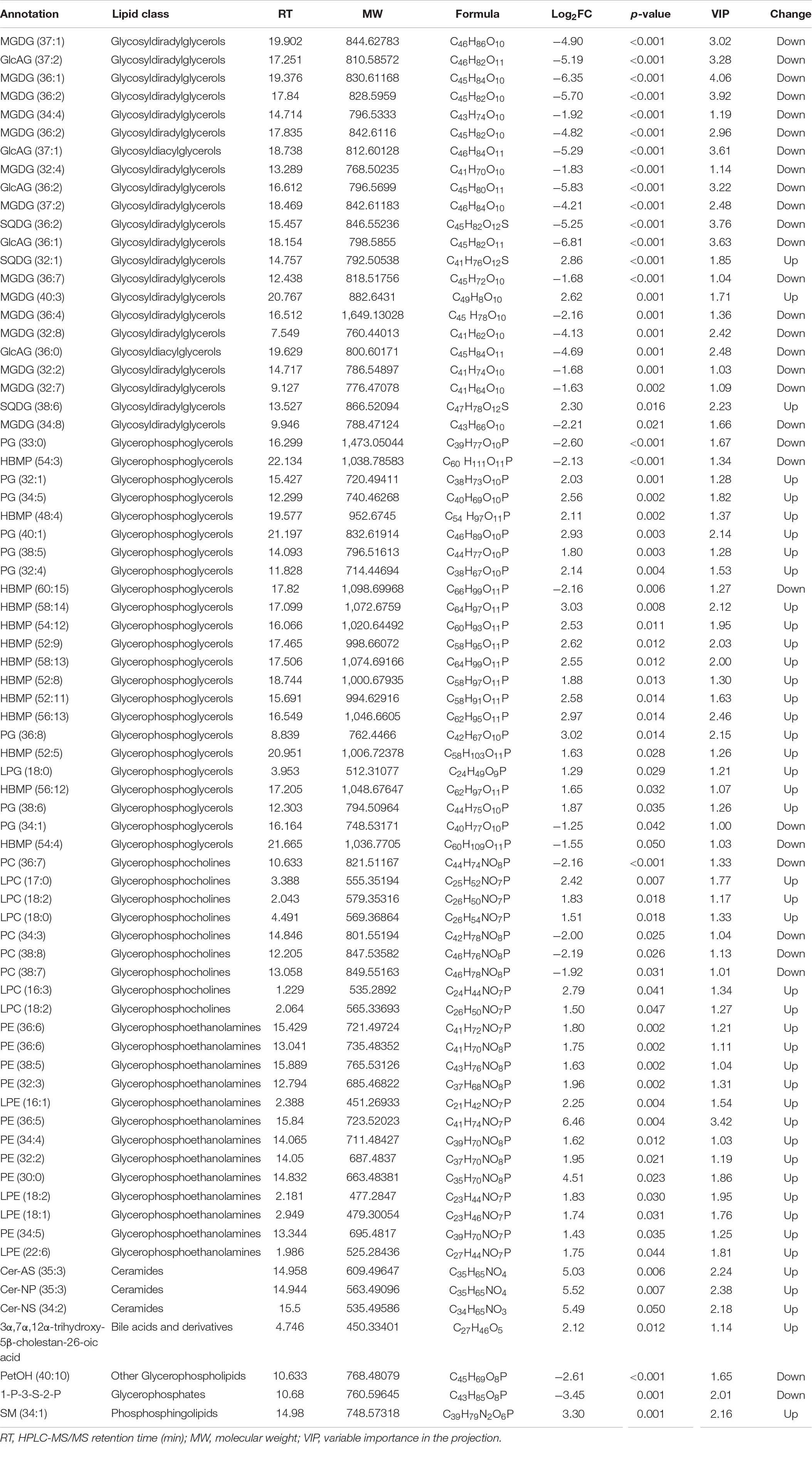
Table 1. Lipid metabolites that showed greater than twofold alterations in abundance in Phaeodactylum tricornutum selected under low (400 μam, ambient CO2 condition) or high CO2 (1,000 μam, projected year 2100 high CO2 according to high emission scenario RCP 8.5) for nearly 400 days.
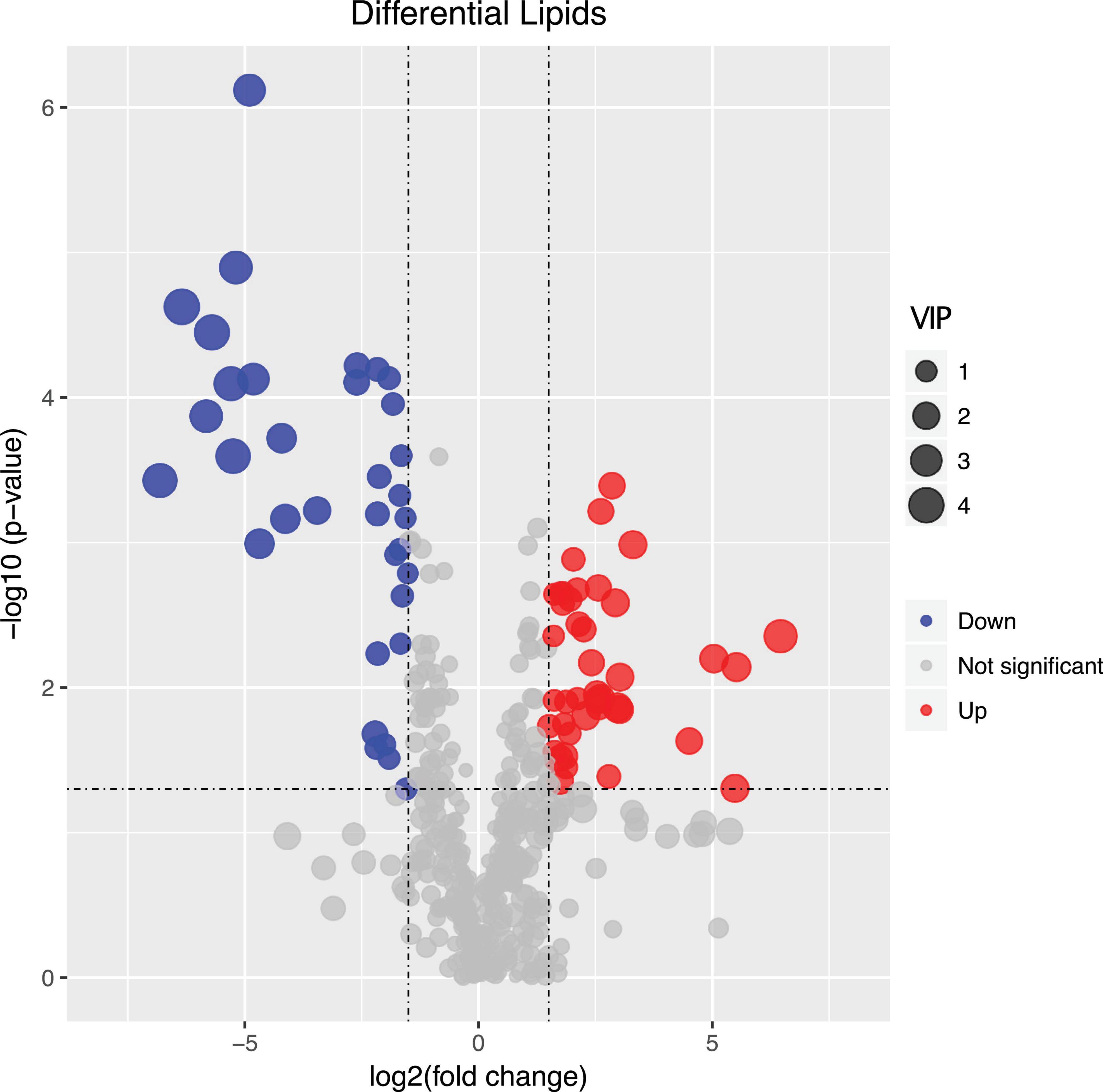
Figure 1. Volcano plot displaying the differentially regulated lipid metabolites between long-term low (400 μatm, LC) and high (1,000 μatm, HC) CO2 selected Phaeodactylum tricornutum cells. The dots represented in blue (down-regulated) and red (up-regulated) are differentially regulated lipid metabolites with > 2-fold change and a p-value of <0.05. VIP value represents the importance projection value of the metabolite obtained from the PLS-DA model.
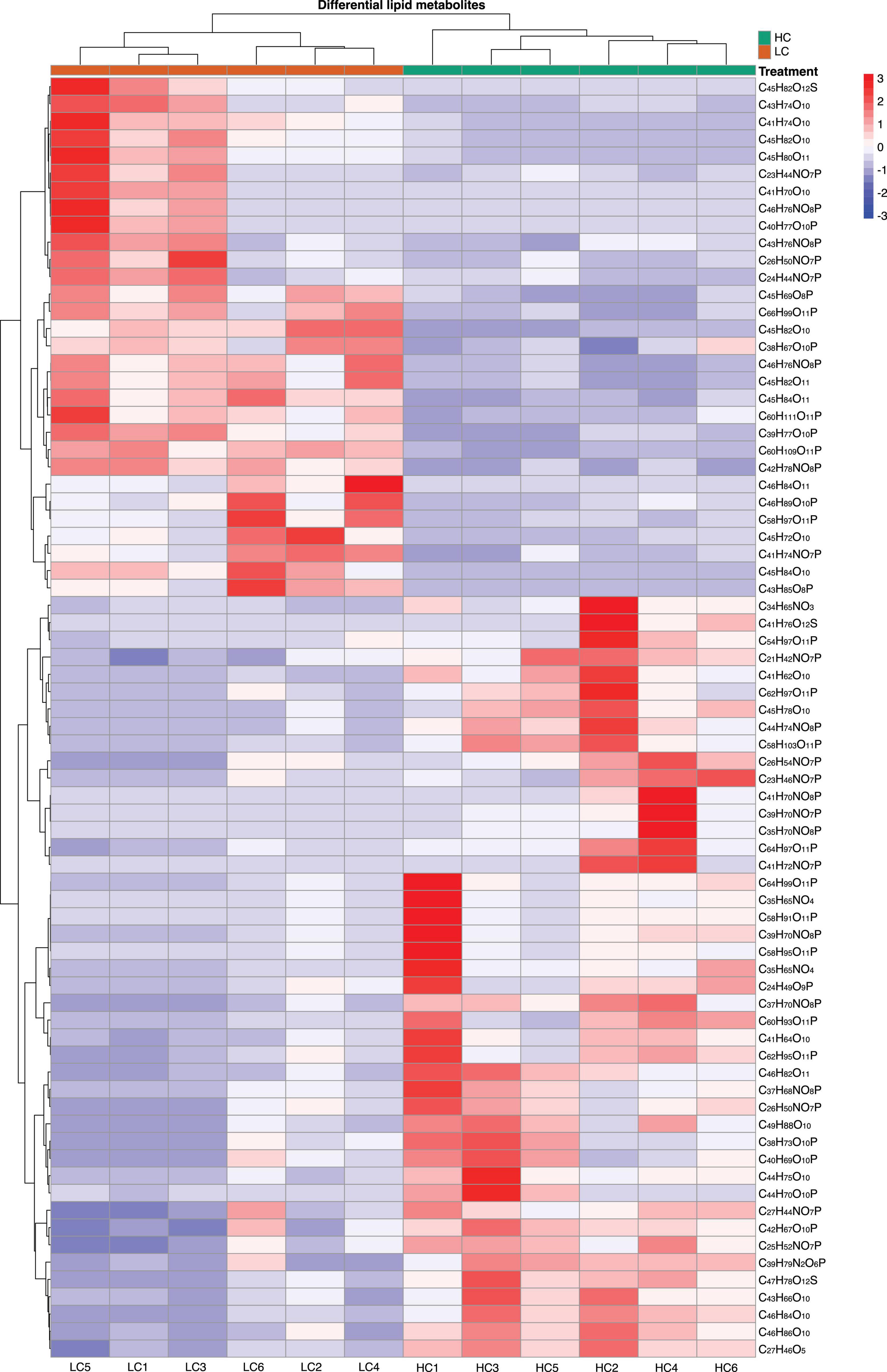
Figure 2. Heatmap displaying the log2-fold change in lipid metabolite between low (400 μatm, LC) and high (1,000 μatm, HC) CO2 selected Phaeodactylum tricornutum cells.
Correlations of Differently Regulated Metabolites
We further explored the correlations between differently regulated metabolites. Our results showed that MGDG (37:1, C46H86O10) were positively correlated with MGDG (34:4, C43H74O10), MGDG (32:4, C46H86O10), MGDG (37:2, C46H84O10) and GlcADG (37:1, C46H82O11) [Pearson correlation coefficients (r): 0.70–0.86] (Figure 3). PC (36:7, C44H74NO8P) were positively correlated with three MGDGs (36:2, C45H82O10; 34:4, C43H74O10, 32:4, C41H70O10) (r: 0.70–0.87). PC (36:7, C44H74NO8P) was positively correlated with GlcADG (37:1, C46H84O11) (r: 0.80), SQDG (36:2, C45H82O12S) (r: 0.74), HBMP (54:3, C60H111O11P) (r: 0.80), PG (33:0, C39H77O10P) (r: 0.73), and PEtOH (40:10, C45H69O81P) (r: 0.99) (Figure 3). In contrast, PC was negatively correlated with SQDG (32:1, C41H76O12S) (r: −0.70) and MGDGs (40:3, C49H88O10) (r: −0.73) (Figure 3). There were also pronounced negative correlations of two metabolites (MGDG, 40:3, C49H88O10; SQDG, 32:1, C41H76O12S) with the other metabolites presented in Figure 3 [e.g., SQDG (C41H76O12S) with PG (C39H77O10P), r: −0.6]. In summary, the up- and down-regulations of the metabolites were tightly coupled either by negative or positive correlations.
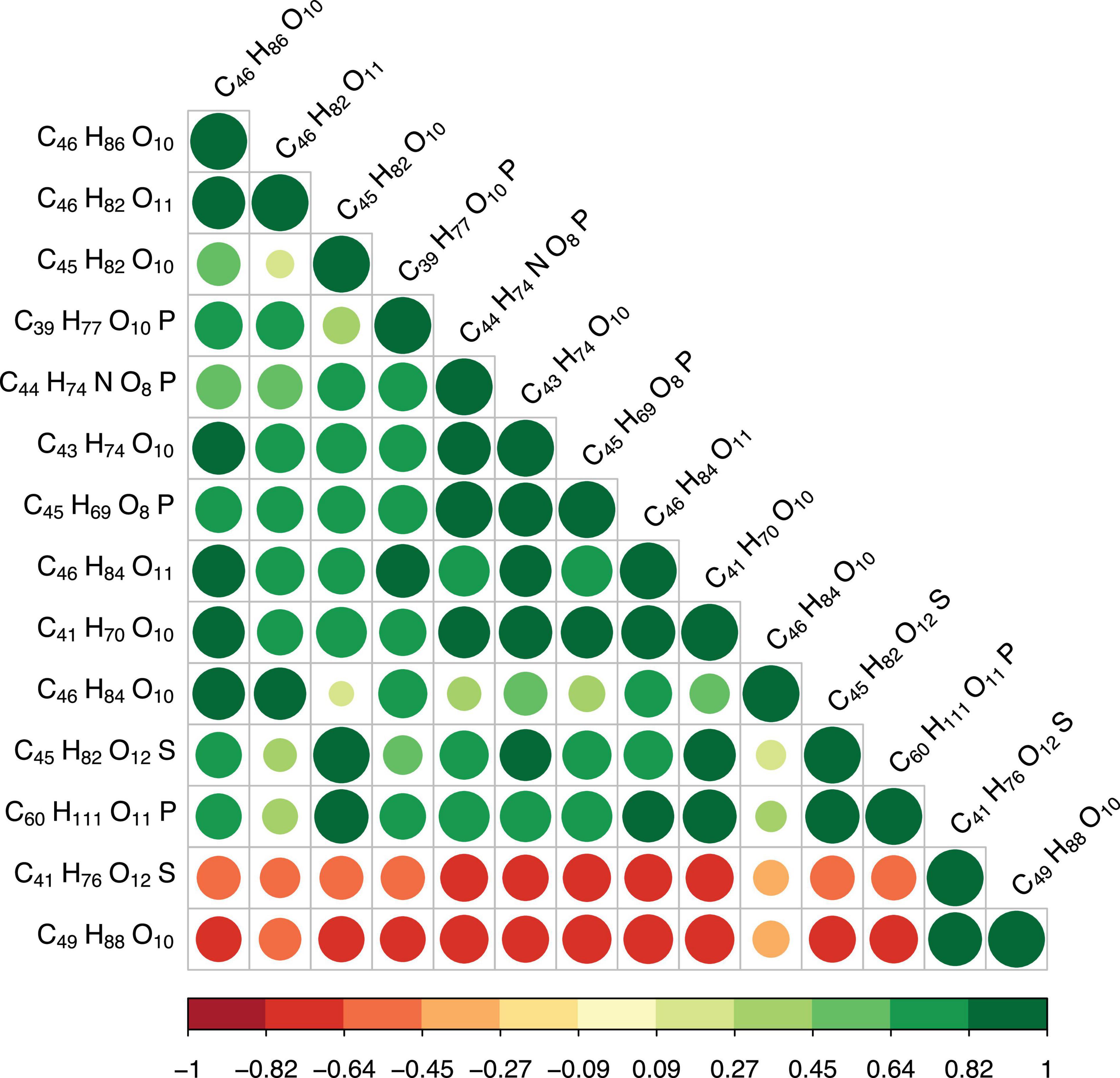
Figure 3. Pearson correlations between differential metabolites (top 20). The size of the circle represents the magnitude of correlation. Green circles indicate positive correlations, red negative correlations. The value of the correlation coefficient (r) is shown in the color bar below the graphs.
Discussion
Our results show that after 400 days (corresponding to almost 900 generations) of long-term high CO2 selection, the marine diatom species P. tricornutum revealed significant changes in lipid metabolisms. One of the most apparent findings of the present study is that MGDG, the main glycerolipid in non-plastid membranes, was significantly down-regulated in long-term LC selected cells. Our results are in agreement with the results of a previous short-term (6 h) study with the green algae species Chlamydomonas reinhardtii (Abreu et al., 2020). MGDG is a hexagonal II phase polar membrane lipid, whose role is to give the membrane a high internal lateral pressure among the fatty acyl chains and a pressure on the membrane proteins (Kruijff, 1997). Due to its non-bilayer lipid properties, different ratios of MGDG to other phase properties lipids may affect the lateral pressure on membrane proteins (Kruijff, 1997). For instance, it has been widely reported that a lower ratio of DGDG (a lamellar phase lipid) to MGDG reflects increased sensitivities of microalgae to various abiotic stresses (e.g., low temperature, CO2 limitation, salt stress, nitrogen starvation) (Du et al., 2018; Liu et al., 2019). Since no significant changes of DGDG were detected in the present study, the down-regulations of MGDG in long-term HC selected cells resulted in an increased ratio of DGDG:MGDG. Therefore, our results suggest that long-term high CO2 selection may be not stressful for P. tricornutum.
It is also worth noting that the down-regulations of MGDG would result in an increased conductivity of the thylakoid and in an increase of luminal pH, causing the activity of violaxanthin de-epoxidase to decrease (Aronsson et al., 2008). In addition, the down-regulations of MGDG are also likely to decrease the violaxanthin availability from the membrane for violaxanthin de-epoxidase (Schaller et al., 2010). Consequently, such a decrease in de-epoxidase would lead to a low efficiency of the violaxanthin-zeaxanthin interconversion, which is a key process of the xanthophyll cycle, a crucial regulatory component for energy dissipation in diatoms (Miloslavina et al., 2009; Goss and Jakob, 2010; Lepetit et al., 2017). This is evidenced by previous studies, in which diatoms in high CO2 conditions exhibited higher non-photochemical quenching (NPQ) (i.e., a lower energy dissipation capacity) (e.g., Gao et al., 2012; Wu et al., 2017; Li et al., 2019). These findings were further supported by our recent investigations, in which we found three proteins, namely the precursor protein of zeaxanthin epoxidase (ZEP2), zeaxanthin epoxidase (ZEP1) and violaxanthin de-epoxidase (VDE), were significantly down-regulated in HC selected cells (data not shown). To summarize, our results suggest that the MGDG down-regulation in long-term HC selected P. tricornutum cells is likely an adaptation strategy to cope with the long-term high CO2 conditions.
PE is one of the major phospholipids (phosphoglycerides) in most algae species (Guschina and Harwood, 2009). Very long chain polyunsaturated fatty acid can be incorporated into PE by acyltransferase reactions of the Kennedy pathway (Sayanova et al., 2017; Li-Beisson et al., 2019). The production of PE by algae was recognized as a response to abiotic stresses, such as light, temperature and nutrient. For instance, it was evidenced that the content of PE significantly increased with increasing temperature (Thompson, 1996). Both dark exposure and phosphate starvation were found to lead to a decrease of PE content in microalgae species (McLarnon-Riches et al., 1998; Khozin-Goldberg and Cohen, 2006). For the response of PE to high CO2, the degree of unsaturation of PE was reported to decrease in response to high CO2 by decreasing the contents of C18:3 at the sn-2 position of PE (Sato et al., 2003). Our results indicated that rather than the unsaturation of PE, the concentration of PE was significantly enhanced under long-term high CO2 selection.
In addition to PE, the majority of the differently regulated PG lipids (seven out of nine) were up-regulated in long-term high CO2 selected cells (Figure 4). PG is the major phospholipid in chloroplasts, and it contains an uncommon trans-3-hexadecenoic acid (C16:1t), located exclusively at position sn-2 of the glycerol backbone in all eukaryotic photosynthetic organisms (Boudière et al., 2014; Figure 4). PG play an important role in the light harvesting complex trimerization process and in the functioning of the photosystem (Loll et al., 2007). Along with MGDG and PE, PG is also sensitive to various abiotic stressors. It is well recognized that the contents of PG in various algal species, including diatoms, decrease under nutrient starvation (Van Mooy et al., 2009; Abida et al., 2015; Wang et al., 2019), elevated temperatures (Feijão et al., 2017, 2020), and low pH conditions (Vítová et al., 2016). In the present study, we found that ∼80% of the significantly regulated PGs were up-regulated after long-term high CO2 selection (Figure 4). This may be due to adaptation driven by long-term high CO2 selection. While the content of PG may decrease after short-term high CO2 selection, its content may be partially or completely reversed over the long-term high CO2 selection period. Such an adaptive response has been evidenced in multiple previous studies (Lohbeck et al., 2012; Jin et al., 2020). For instance, the fatty acid and lipid contents of three diatoms partly or entirely recovered following a long-term exposure (∼2 years) to warming conditions (+4°C) (Jin et al., 2020). Thus, our results suggest a high potential of diatoms to adapt their lipid metabolites to long-term high CO2 selection.
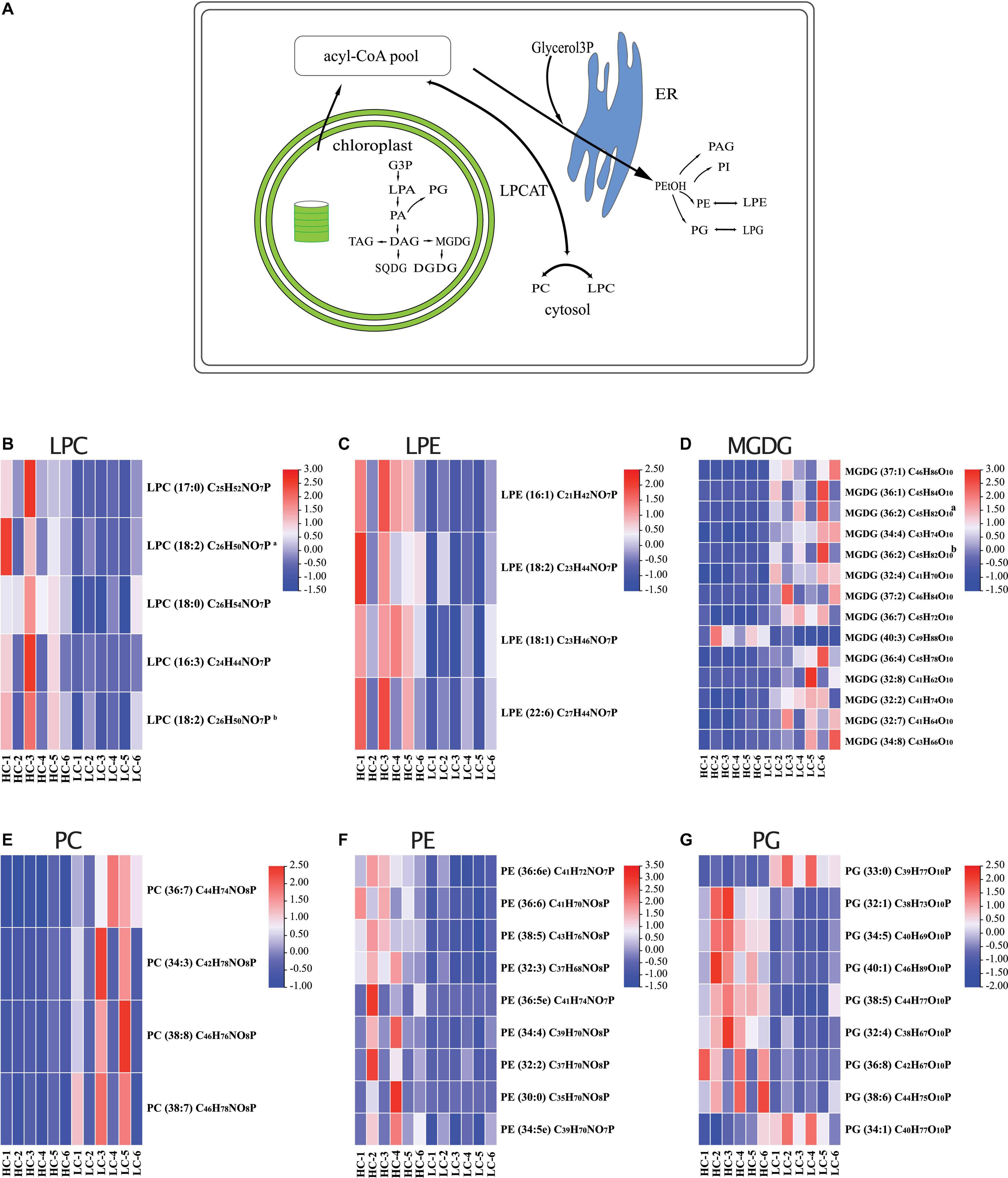
Figure 4. (A) Model of lipid metabolism changes in Phaeodactylum tricornutum after long-term high CO2 selection. The heatmaps display the log2-fold changes in lysophosphatidylcholine [LPC, (B)], lysophosphatidylethanolamine LPE (C), monogalactosyldiacylglycerol [MGDG, (D)], PC, phosphatidylcholine [PC, (E)], phosphatidylethanolamine [PE, (F)], and phosphatidylglycerol [PG, (G)].
It is known that an increase of SQDG could compensate the absence of PG in plastids (Jouhet et al., 2010; Boudière et al., 2014; Abida et al., 2015), thus the contents of SQDG and PG are expected to be negatively correlated, as shown in our study. Under stressful conditions, such as nutrient starvation, a PG-to-SQDG replacement was considered to be a ubiquitous phenomenon in photosynthetic organisms, enabling the preservation of an anionic lipid environment to the photosystems in the thylakoids (Boudière et al., 2014; Nakamura and Li-Beisson, 2016). Besides a PG-to-SQDG replacement, there was also a PC-to-DGTA replacement occurring in the diatom P. tricornutum, which was also considered to be a strategy to cope with environmental stresses (Abida et al., 2015). Since we observed multiple negative or positive correlations between different lipid metabolites (e.g., positive correlation between PC and PEtOH; negative correlations between MGDG and PC), our results suggest several more lipid remodeling mechanisms that have not been identified yet. We propose that the underlying mechanisms for lipid remodeling in response to various abiotic stressors in diatoms should be further investigated as part of future studies.
It is also recognized that the lipid metabolism of microalgae was regulated by some mineral elements such as silicon and calcium. For instance, lipid production in marine diatoms Chaetoceros gracilis and Thalassiosira pseudonana was reported to increase under silicon limitation conditions (Zendejas et al., 2012; Adams and Bugbee, 2014). Since high CO2 was reported to affect the biogenic silica cellular contents of diatoms (Xu et al., 2014), such a change induced by high CO2 is expected to regulate the lipid metabolism as well. Calcium (Ca2+), a secondary messenger, plays a crucial role in the signal transduction through the activation of various receptors associated with metabolic in coping with various environmental changes (Chen et al., 2014). Calcium can bind with calmodulin and then affect the energy metabolism by regulating the cyclic electron flow in photophosphorylation and respiratory oxidative phosphorylation in microalgae (Chen et al., 2015). It is known that an appropriate concentration of calcium can stimulate the activity of Acetyl-CoA carboxylase that catalyzes the conversion of acetyl-coenzyme A to malonyl-CoA during the fatty acid biosynthesis (Gorain et al., 2013). However, how would high CO2 interact with calcium to regulate the lipid modeling of diatoms (through energy metabolism or signal transduction) remains unknown. Hence, we recommend that the underlying mechanisms for lipid remodeling in response to high CO2 interacting with essential (or non-essential) elements in diatoms are warranted for further investigations.
In conclusion, our observations suggest that long-term high CO2 selection triggered substantial changes in lipid metabolites of the marine diatom P. tricornutum (Figure 4A). Some lipid metabolites (such as PGs) showed high adaptive potential to the selection. The tightly coupled regulations (positively or negatively correlated) of lipid metabolites reveal that the lipid remodeling is an organismal adaptation strategy of marine diatoms to ongoing ocean acidification. The composition and concentration of lipid are crucial for marine food quality, and their changes can be transferred to high trophic levels (Rossoll et al., 2012; Jin et al., 2015, 2020). Therefore, our results outline the importance of investigating the long-term responses of lipids of primary producers to prolonged ocean acidification conditions, and assessing the ecological consequences.
Data Availability Statement
The original contributions presented in the study are included in the article/Supplementary Material, further inquiries can be directed to the corresponding author/s.
Author Contributions
PJ and JX conceptualized the study. ZL, HL, JP, PL, QH, YG, and JZ acquired the data. PJ and FL performed the statistical analysis and generated the figures with JW and SO. PJ drafted the manuscript, SO contributed substantially to the editing of the manuscript. All authors discussed and approved the manuscript.
Funding
This study was supported by the National Natural Science Foundation of China (Nos: 41806141 and 41890803).
Conflict of Interest
The authors declare that the research was conducted in the absence of any commercial or financial relationships that could be construed as a potential conflict of interest.
Publisher’s Note
All claims expressed in this article are solely those of the authors and do not necessarily represent those of their affiliated organizations, or those of the publisher, the editors and the reviewers. Any product that may be evaluated in this article, or claim that may be made by its manufacturer, is not guaranteed or endorsed by the publisher.
Supplementary Material
The Supplementary Material for this article can be found online at: https://www.frontiersin.org/articles/10.3389/fmicb.2021.748445/full#supplementary-material
Supplementary Figure 1 | Pearson correlations between Quality control samples (QCs).
Supplementary Figure 2 | The principal component analysis (PCA) of total lipid metabolites.
Supplementary Figure 3 | Score plot from partial least squares discriminant analysis (PLS-DA) of the lipids in low (400 μatm, LC) and high (1,000 μatm, HC) CO2 selected Phaeodactylum tricornutum cells. The PLS-DA showed a clear separation between the HC and LC selected cells with acceptable goodness of fit (R2 = 0.97) and predictive power (Q2 = 0.91).
Footnotes
References
Abida, H., Dolch, L. J., Meï, C., Villanova, V., Conte, M., Block, M. A., et al. (2015). Membrane glycerolipid remodeling triggered by nitrogen and phosphorus starvation in Phaeodactylum tricornutum. Plant Physiol. 167, 118–136. doi: 10.1104/pp.114.252395
Abreu, I. N., Aksmann, A., Bajhaiya, A. K., Benlloch, R., Giordano, M., Pokora, W., et al. (2020). Changes in lipid and carotenoid metabolism in Chlamydomonas reinhardtii during induction of CO2-concentrating mechanism: cellular response to low CO2 stress-sciencedirect. Algal Res. 52:102099. doi: 10.1016/j.algal.2020.102099
Adams, C., and Bugbee, B. (2014). Enhancing lipid production of the marine diatom Chaetoceros gracilis: synergistic interactions of sodium chloride and silicon. J. Appl. Phycol. 26, 1351–1357. doi: 10.1007/s10811-013-0156-7
Aronsson, H., Schöttler, M. A., Kelly, A. A., Sundqvist, C., Dörmann, P., Karim, S., et al. (2008). Mongalactosyldiacylglycerol deficiency in Arabidopsis affects pigment composition in the prolamellar body and impairs thylakoid membrane ernergization and photoprotection. Plant Physiol. 148, 580–592.
Bach, L. T., and Taucher, J. (2019). CO2 effects on diatoms: a synthesis of more than a decade of ocean acidification experiments with natural communities. Ocean Sci. 15, 1159–1175. doi: 10.5194/os-15-1159-2019
Bermúdez, R., Feng, Y., Roleda, M. Y., Tatters, A. O., Hutchins, D. A., Larsen, T., et al. (2015). Long-term conditioning to elevated pCO2 and warming influences the fatty and amino acid composition of the diatom Cylindrotheca fusiformis. PLoS One 10:e0123945. doi: 10.1371/journal.pone.0123945
Boudière, L., Michaud, M., Petroutsos, D., Rébeillé, F., Falconet, D., Bastien, O., et al. (2014). Glycerolipids in photosynthesis: composition, synthesis and trafficking. Biochim. Biophys. Acta Bioenerg. 1837, 470–480. doi: 10.1016/j.bbabio.2013.09.007
Bréhélin, C., Kessler, F., and van Wijk, K. J. (2007). Plastoglobules: versatile lipoprotein particles in plastids. Trends Plant Sci. 12, 260–266. doi: 10.1016/j.tplants.2007.04.003
Broadhurst, D. I., and Kell, D. B. (2006). Statistical strategies for avoiding false discoveries in metabolomics and related experiments. Metabolomics 2, 171–196. doi: 10.1007/s11306-006-0037-z
Chen, C., Chen, H., Zhang, Y., Thomas, H., Frank, M. H., He, Y., et al. (2020). TBtools-an integrative toolkit developed for interactive analyses of big biological data. Mol. Plant 13, 1194–1202. doi: 10.1016/j.molp.2020.06.009
Chen, H., Hu, J., Qiao, Y., Chen, W., Rong, J., Zhang, Y., et al. (2015). Ca2 + -regulated cyclic electron flow supplies ATP for nitrogen starvation induced lipid biosynthesis in green alga. Sci. Rep. 5:15117. doi: 10.1038/srep15117
Chen, H., Zhang, Y., He, C., and Wang, Q. (2014). Ca2+ signal transduction related to neutral lipid synthesis in an oil-producing green alga Chlorella sp. C2. Plant Cell Physiol. 55, 634–644. doi: 10.1093/pcp/pcu015
Collins, S., Boyd, P. W., and Doblin, M. A. (2020). Evolution, microbes, and changing ocean conditions. Annu. Rev. Mar. Sci. 12, 181–208. doi: 10.1146/annurev-marine-010318-095311
Dalsgaard, J., John, M. S., Kattner, G., Müller-Navarra, D., and Hagen, W. (2003). Fatty acid trophic markers in the pelagic marine environment. Adv. Mar. Biol. 46, 225–340. doi: 10.1016/S0065-2881(03)46005-7
Du, Z. Y., Lucker, B. F., Zienkiewicz, K., Miller, T. E., Zienkiewicz, A., Sears, B. B., et al. (2018). Galactoglycerolipid lipase pgd1 is involved in thylakoid membrane remodeling in response to adverse environmental conditions in Chlamydomonas. Plant Cell 30, 447–465. doi: 10.1105/tpc.17.00446
Dunstan, G. A., Volkman, J. K., Barrett, S. M., and Garland, C. D. (1993). Changes in the lipid composition and maximisation of the polyunsaturated fatty acid content of three microalgae grown in mass culture. J. Appl. Phycol. 5, 71–83. doi: 10.1007/BF02182424
Feijão, E., Franzitta, M., Cabrita, M. T., Caçador, I., Duarte, B., Gameiro, C., et al. (2020). Marine heat waves alter gene expression of key enzymes of membrane and storage lipids metabolism in Phaeodactylum tricornutum. Plant Physiol. Biochem. 156, 357–368. doi: 10.1016/j.plaphy.2020.09.022
Feijão, E., Gameiro, C., Franzitta, M., Duarte, B., Caçador, I., Cabrita, M. T., et al. (2017). Heat wave impacts on the model diatom Phaeodactylum tricornutum: searching for photochemical and fatty acid biomarkers of thermal stress. Ecol. Indic. 95, 1026–1037. doi: 10.1016/j.ecolind.2017.07.058
Field, C. B., Behrenfeld, M. J., Randerson, J. T., and Falkowski, P. (1998). Primary production of the biosphere: integrating terrestrial and oceanic components. Science 281, 237–240. doi: 10.1126/science.281.5374.237
Gao, K. S., and Campbell, D. (2014). Photophysiological responses of marine diatoms to elevated CO2 and decreased pH: a review. Funct. Plant Biol. 41, 449–459. doi: 10.1071/FP13247
Gao, K. S., Xu, J., Gao, G., Li, Y., Hutchins, D. A., Huang, B., et al. (2012). Rising CO2 and increased light exposure synergistically reduce marine primary productivity. Nat. Clim. Change 2, 519–523. doi: 10.1038/nclimate1507
Gattuso, J. P., Magnan, A., Billé, R., Cheung, W., Howes, E., Joos, F., et al. (2015). Contrasting futures for ocean and society from different anthropogenic CO2 emissions scenarios. Science 349:aac4722. doi: 10.1126/science.aac4722
Gorain, P. C., Bagchi, S. K., and Mallick, N. (2013). Effects of calcium, magnesium and sodium chloride in enhancing lipid accumulation in two green microalgae. Environ. Technol. 34, 1887–1894. doi: 10.1080/09593330.2013.812668
Goss, R., and Jakob, T. (2010). Regulation and function of xanthophyll cycle-dependent photoprotection in algae. Photosynth. Res. 106, 103–122. doi: 10.1007/s11120-010-9536-x
Guillard, R. R. L., and Ryther, J. H. (1962). Studies of marine planktonic diatoms. I. Cyclotella nana Hustedt, and Detonula confervacea (Cleve) Gran. Can. J. Microbiol. 8, 229–239. doi: 10.1139/m62-029
Guschina, I. A., and Harwood, J. L. (2006). Lipids and lipid metabolism in eukaryotic algae. Prog. Lipid Res. 45, 160–186. doi: 10.1016/j.plipres.2006.01.001
Guschina, I. A., and Harwood, J. L. (2009). “Algal lipids and effect of the environment on their biochemistry,” in Lipid in Aquatic Ecosystems, eds M. Kainz, M. Brett, and M. Arts (New York, NY: Springer), 1–24. doi: 10.1007/978-0-387-89366-2_1
Hennon, G. M. M., Ashworth, J., Groussman, R. D., Berthiaume, C., and Morales, R. L. (2015). Diatom acclimation to elevated CO2 via cAMP signalling and coordinated gene expression. Nat. Clim. Change 5, 761–765. doi: 10.1038/nclimate2683
Hildebrand, M., Davis, A. K., Smith, S. R., Traller, J. C., and Abbriano, R. (2012). The place of diatoms in the biofuels industry. Biofuels 3, 221–240. doi: 10.4155/BFS.11.157
Hixson, S. M., Sharma, B., Kainz, M. J., Wacker, A., and Arts, M. T. (2015). Production, distribution, and abundance of long-chain omega-3 polyunsaturated fatty acids: a fundamental dichotomy between freshwater and terrestrial ecosystems. Environ. Rev. 23, 414–424. doi: 10.1139/er-2015-0029
Hopkinson, B. M., Dupont, C. L., Allen, A. E., and Morel, F. M. M. (2011). Efficiency of the CO2-concentrating mechanism of diatoms. Proc. Natl. Acad. Sci. U.S.A. 108, 3830–3837. doi: 10.1073/pnas.1018062108
IPCC (2014). Synthesis Report. Contribution of Working Groups I. II and III to the Fifth Assessment Report of the Intergovernmental Panel on Climate Change. Geneva: IPCC, 151.
Jin, P., Gao, K., and Beardall, J. (2013). Evolutionary responses of a coccolithophorid Gephyrocapsa oceanica to ocean acidification. Evolution 67, 1869–1878. doi: 10.1111/evo.12112
Jin, P., Hutchins, D. A., and Gao, K. (2020). The impacts of ocean acidification on marine food quality and its potential food chain consequences. Front. Mar. Sci. 7:543979. doi: 10.3389/fmars.2020.543979
Jin, P., Wang, T., Liu, N., Dupont, S., Beardall, J., Boyd, P. W., et al. (2015). Ocean acidification increases the accumulation of toxic phenolic compounds across trophic levels. Nat. Commun. 6:8714. doi: 10.1038/ncomms9714
Jouhet, J., Dubots, E., Maréchal, E., and Block, M. A. (2010). “Lipid trafficking in plant photosynthetic cells,” in Lipids in Photosynthesis, Vol. 30, eds H. Wada and N. Murata (Dordrecht: Springer), 349–372. doi: 10.1007/978-90-481-2863-1_16
Khozin-Goldberg, I., and Cohen, Z. (2006). The effect of phosphate starvation on the lipid and fatty acid composition of the fresh water eustigmatophyte Monodus subterraneus. Phytochemistry 67, 696–701. doi: 10.1016/j.phytochem.2006.01.010
Kroth, P. G., Chiovitti, A., Gruber, A., Martin-Jezequel, V., Mock, T., Parker, M. S., et al. (2008). A model for carbohydrate metabolism in the diatom Phaeodactylum tricornutum deduced from comparative whole genome analysis. PLoS One 3:e1426. doi: 10.1371/journal.pone.0001426
Lane, A. E., and Burris, J. E. (1981). Effects of environmental pH on the internal pH of Chlorella pyrenoidosa, Scenedesmus quadricauda, and Euglena mutabilis. Plant Physiol. 68, 439–442. doi: 10.1104/pp.68.2.439
Lepetit, B., Gélin, G., Lepetit, M., Sturm, S., Vugrinec, S., Rogato, A., et al. (2017). The diatom Phaeodactylum tricornutum adjusts nonphotochemical fluorescence quenching capacity in response to dynamic light via fine-tuned Lhcx and xanthophyll cycle pigment synthesis. New Phytol. 214, 205–218. doi: 10.1111/nph.14337
Li, F. T., Beardall, J., Collins, S., and Gao, K. S. (2017). Decreased photosynthesis and growth with reduced respiration in the model diatom Phaeodactylum tricornutum grown under elevated CO2 over 1800 generations. Glob. Change Biol. 23, 127–137. doi: 10.1111/gcb.13501
Li, H., Xu, T., Ma, J., Li, F., and Xu, J. (2021). Physiological responses of Skeletonema costatum to the interactions of seawater acidification and the combination of photoperiod and temperature. Biogeosciences 18, 1439–1449. doi: 10.5194/bg-18-1439-2021
Li, W., Ding, J., Li, F., Wang, T., Yang, Y., Li, Y., et al. (2019). Functional responses of smaller and larger diatoms to gradual CO2 rise. Sci. Total Environ. 680, 79–90.
Li-Beisson, Y., Thelen, J. J., Fedosejevs, E., and Harwood, J. L. (2019). The lipid biochemistry of eukaryotic algae. Prog. Lipid Res. 74, 31–68. doi: 10.1016/j.plipres.2019.01.003
Liu, X., Ma, D., Zhang, Z., Wang, S., Du, S., Deng, X., et al. (2019). Plant lipid remodeling in response to abiotic stresses. Environ. Exp. Bot. 165, 174–184. doi: 10.1016/j.envexpbot.2019.06.005
Lohbeck, K. T., Riebesell, U., and Reusch, T. B. H. (2012). Adaptive evolution of a key phytoplankton species to ocean acidification. Nat. Geosci. 5, 346–351. doi: 10.1038/ngeo1441
Loll, B., Kern, J., Saenger, W., Zouni, A., and Biesiadka, J. (2007). Lipids in photosystem II: interactions with protein and cofactors. Biochim. Biophys. Acta Bioenerg. 1767, 509–519. doi: 10.1016/j.bbabio.2006.12.009
McLarnon-Riches, C. J., Rolph, C. E., Greenway, D. L. A., and Robinson, P. K. (1998). Effects of environmental factors and metals on Selenastrum capricornutum. Phytochemistry 49, 1241–1247. doi: 10.1016/S0031-9422(98)00095-8
Miloslavina, Y., Grouneva, I., Lambrev, P. H., Lepetit, B., Goss, R., Wilhelm, C., et al. (2009). Ultrafast fluorescence study on the location and mechanism of nonphotochemical quenching in diatoms. Biochim. Biophys. Acta Bioenerg. 1787, 1189–1197. doi: 10.1016/j.bbabio.2009.05.012
Nakamura, Y., and Li-Beisson, Y. (2016). Lipids in Plant and Algae Development. Cham: Springer International Publishing. doi: 10.1007/978-3-319-25979-6
Petrou, K., Baker, K. G., Nielsen, D. A., Hancock, A. M., Schulz, K. G., and Davidson, A. T. (2019). Acidification diminishes diatom silica production in the Southern Ocean. Nat. Clim. Change 9, 781–786. doi: 10.1038/s41558-019-0557-y
Raven, J. A. (2010). Inorganic carbon acquisition by eukaryotic algae: four current questions. Photosynth. Res. 106, 123–134. doi: 10.1007/s11120-010-9563-7
Renberg, L., Johansson, A. I., Shutova, T., Stenlund, H., Aksmann, A., Raven, J. A., et al. (2010). A metabolomic approach to study major metabolite changes during acclimation to limiting CO2 in Chlamydomonas reinhardtii. Plant Physiol. 154, 187–196. doi: 10.1104/pp.110.157651
Reusch, T. B., and Boyd, P. W. (2013). Experimental evolution meets marine phytoplankton. Evolution 67, 1849–1859. doi: 10.1111/evo.12035
Roede, J. R., Uppal, K., Park, Y., Tran, V., and Jones, D. P. (2014). Transcriptome-metabolome wide association study (TMWAS) of maneb and paraquat neurotoxicity reveals network level interactions in toxicologic mechanism. Toxicol. Rep. 1, 435–444. doi: 10.1016/j.toxrep.2014.07.006
Rossoll, D., Bermúdez, R., Hauss, H., Schulz, K., Riebesell, U., Sommer, U., et al. (2012). Ocean acidification-induced food quality deterioration constrains trophic transfer. PLoS One 7:e34737. doi: 10.1371/journal.pone.0034737
Sato, N., Tsuzuki, M., and Kawaguchi, A. (2003). Glycerolipid synthesis in Chlorella kessleri 11 h: II. Effect of the CO2 concentration during growth. Mol. Cell Biol. Lipids 1633, 35–42. doi: 10.1016/S1388-1981(03)00070-2
Sayanova, O., Mimouni, V., Ulmann, L., Manceau, A., Pasquet, V., Schoefs, B., et al. (2017). Modulation of lipid biosynthesis by stress in diatoms. Philos. Trans. R. Soc. B Biol. Sci. 372:20160407. doi: 10.1098/rstb.2016.0407
Schaller, S., Latowski, D., Jemioła-Rzemiñska, M., Wilhelm, C., Strzałka, K., and Goss, R. (2010). The main thylakoid membrane lipid monogalactosyldiacylglycerol (MGDG) promotes the de-epoxidation of violaxanthin associated with the light-harvesting complex of photosystem II (LHCII). Biochim. Biophys. Acta Bioenerg. 1797, 414–424. doi: 10.1016/j.bbabio.2009.12.011
Shahidi, F., and Ambigaipalan, P. (2018). Omega-3 polyunsaturated fatty acids and their health benefits. Annu. Rev. Food Sci. Technol. 9, 345–381. doi: 10.1146/annurev-food-111317-095850
Shi, D., Hong, H., Su, X., Liao, L., Chang, S., and Lin, W. (2019). The physiological response of marine diatoms to ocean acidification: differential roles of seawater pCO2 and pH. J. Phycol. 55, 521–533. doi: 10.1111/jpy.12855
Stonik, V., and Stonik, I. (2015). Low-molecular-weight metabolites from diatoms: structures, biological roles and biosynthesis. Mar. Drugs 13, 3672–3709. doi: 10.3390/md13063672
Thompson, G. A. (1996). Lipids and membrane function in green algae. Biochim. Biophys. Acta 1302, 17–45. doi: 10.1016/0005-2760(96)00045-8
Trimborn, S., Wolf-Gladrow, D., Richter, K. U., and Rost, B. (2009). The effect of pCO2 on carbon acquisition and intracellular assimilation in four marine diatoms. J. Exp. Mar. Biol. Ecol. 376, 26–36. doi: 10.1016/j.jembe.2009.05.017
Van Mooy, B. A. S., Fredricks, H. F., Pedler, B. E., Dyhrman, S. T., Karl, D. M., Koblížek, M., et al. (2009). Phytoplankton in the ocean use non-phosphorus lipids in response to phosphorus scarcity. Nature 458, 69–72. doi: 10.1038/nature07659
Vítová, M., Goecke, F., Sigler, K., and Øezanka, T. (2016). Lipidomic analysis of the extremophilic red alga Galdieria sulphuraria in response to changes in pH. Algal Res. 13, 218–226. doi: 10.1016/j.algal.2015.12.005
Wang, S., Sirbu, D., Thomsen, L., Kuhnert, N., Ullrich, M. S., and Thomsen, C. (2019). Comparative lipidomic studies of Scenedesmus sp. (Chlorophyceae) and Cylindrotheca closterium (Bacillariophyceae) reveal their differences in lipid production under nitrogen starvation. J. Phycol. 55, 1246–1257. doi: 10.1111/jpy.12887
Wang, T., Tong, S., Liu, N., Li, F., Wells, M. L., and Gao, K. (2017). The fatty acid content of plankton is changing in subtropical coastal waters as a result of OA: results from a mesocosm study. Mar. Environ. Res. 132, 51–62. doi: 10.1016/j.marenvres.2017.10.010
Wang, X., Zhang, M., Ma, J., Zhang, Y., Hong, G., Sun, F., et al. (2015). Metabolic changes in paraquat poisoned patients and support vector machine model of discrimination. Biol. Pharm. Bull. 38, 470–475. doi: 10.1248/bpb.b14-00781
Wen, B., Mei, Z., Zeng, C., and Liu, S. (2017). metaX: a flexible and comprehensive software for processing metabolomics data. BMC Bioinformatics 18:183. doi: 10.1186/s12859-017-1579-y
Wu, Y., Campbell, D. A., and Gao, K. S. (2017). Short-term elevated CO2 exposure stimulated photochemical performance of a coastal marine diatom. Mar. Environ. Res. 125, 42–48. doi: 10.1016/j.marenvres.2016.12.001
Wu, Y., Gao, K., and Riebesell, U. (2010). CO2-induced seawater acidification affects physiological performance of the marine diatom Phaeodactylum tricornutum. Biogeosciences 7, 2915–2923. doi: 10.5194/bg-7-2915-2010
Xu, J., Gao, K., Li, Y., and Hutchins, D. A. (2014). Physiological and biochemical responses of diatoms to projected ocean changes. Mar. Ecol. Prog. Ser. 515, 73–81. doi: 10.3354/meps11026
Yang, G. Y., and Gao, K. S. (2012). Physiological responses of the marine diatom Thalassiosira pseudonana to increased pCO2 and seawater acidity. Mar. Environ. Res. 79, 142–151. doi: 10.1016/j.marenvres.2012.06.002
Zendejas, F. J., Benke, P. I., Lane, P. D., Simmons, B. A., and Lane, T. W. (2012). Characterization of the acylglycerols and resulting biodiesel derived from vegetable oil and microalgae (Thalassiosira pseudonana and Phaeodactylum tricornutum). Biotechnol. Bioeng. 109, 1146–1154. doi: 10.1002/bit.24395
Keywords: food quality, adaptation, ocean acidification, lipidomics, diatoms
Citation: Jin P, Liang Z, Lu H, Pan J, Li P, Huang Q, Guo Y, Zhong J, Li F, Wan J, Overmans S and Xia J (2021) Lipid Remodeling Reveals the Adaptations of a Marine Diatom to Ocean Acidification. Front. Microbiol. 12:748445. doi: 10.3389/fmicb.2021.748445
Received: 28 July 2021; Accepted: 09 September 2021;
Published: 14 October 2021.
Edited by:
Yuanyuan Feng, Shanghai Jiao Tong University, ChinaReviewed by:
Zou Dinghui, South China University of Technology, ChinaJin Zhou, Tsinghua University, China
Copyright © 2021 Jin, Liang, Lu, Pan, Li, Huang, Guo, Zhong, Li, Wan, Overmans and Xia. This is an open-access article distributed under the terms of the Creative Commons Attribution License (CC BY). The use, distribution or reproduction in other forums is permitted, provided the original author(s) and the copyright owner(s) are credited and that the original publication in this journal is cited, in accordance with accepted academic practice. No use, distribution or reproduction is permitted which does not comply with these terms.
*Correspondence: Jianrong Xia, anJ4aWFAZ3podS5lZHUuY24=