- 1Beijing Engineering Research Center of Food Environment and Public Health, Minzu University of China, Beijing, China
- 2College of Food Science and Engineering, Dalian Ocean University, Dalian, China
- 3College of life and Environmental Science, Minzu University of China, Beijing, China
Background: Fibromyalgia (FM) is a syndrome characterized by chronic and widespread musculoskeletal pain. A number of studies have implied a potential association between gut microbiota and FM. However, the casual association between gut microbiota and FM remains unknown.
Method: Mendelian randomization (MR) study was conducted using the summary statistics of genetic variants from the genome-wide association study (GWAS). Inverse variance weighted (IVW), combined with MR-Egger and weighted median were used to investigate the causal association between 119 gut microbiota genera and FM. Sensitivity analyses were performed on the MR results, including heterogeneity test, leave-one-out test and pleiotropy test.
Results: A total of 1,295 single nucleotide polymorphism (SNPs) were selected as instrumental variables (IVs), with no significant heterogeneity and pleiotropy according to the sensitivity analyses. Five gut microbiota genera were found to have significant casual association with FM. Coprococcus2 (OR = 2.317, p-value = 0.005, 95% CI: 1.289–4.167), Eggerthella (OR = 1.897, p-value = 0.001, 95% CI: 1.313–2.741) and Lactobacillus (OR = 1.576, p-value =0.020, 95% CI: 1.073–2.315) can increase the risk of FM. FamillyXIIIUCG001 (OR = 0.528, p-value = 0.038, 95% CI: 0.289–0.964) and Olsenella (OR = 0.747, p-value = 0.050, 95% CI: 0.557–1.000) can decrease the risk of FM.
Conclusion: This MR study found that gut microbiota is casually associated with FM. New insights into the mechanisms of FM mediated by gut microbiota are provided.
1 Introduction
Fibromyalgia (FM) is an enigmatic syndrome characterized by chronic and widespread musculoskeletal pain. The global prevalence of FM is estimated at 2.7%, and is more in women than men (Sarzi-Puttini et al., 2020). Fibromyalgia can have an extremely negative impact on the psychological and physical condition of patients, causing a decreased quality of their life (Favretti et al., 2023). However, FM remains poorly understood, lacking effective preventive and therapeutic strategies. Multiple factors have been explored in the development of FM, and the gut-brain axis and gut microbiota are proposed as a promising mechanism (Giorgi et al., 2023).
The gut-brain axis is getting increasingly focused. It is a bidirectional interaction between the central and enteric nervous systems, involving multiple pathways including immune, neural, endocrine, and metabolic routes (Mayer et al., 2022). And the gut microbiota, a diverse and dynamic community of microorganisms that inhabit the digestive tract, is considered a key factor that modifies the gut-brain axis (Guo et al., 2019; Ibrahim et al., 2022). It has been reported that gut microbiota is associated with rheumatic diseases (Yu et al., 2021; Zhao et al., 2022) and neurological diseases (Generoso et al., 2021; Socała et al., 2021; Chen et al., 2021b) and plays a critical role in their development. It has also been reported that gut microbiota is associated with FM (Minerbi et al., 2019; Minerbi and Fitzcharles, 2020), but this association is correlative rather than causal.
Mendelian randomization (MR) is a method that utilizes genetic variants as instrumental variables to assess the causal association between exposures and outcomes (Emdin et al., 2017). Compared with observational epidemiological studies, MR is inherently less prone to confounding and reverse causation because of the random allocation of genotypes in the forming of a zygote during gestation (Davey Smith and Hemani, 2014). It can generate robust results when assessing the causal effects of modifiable exposures on disease outcomes.
In this study, we used the genome-wide association study (GWAS) summary statistics, to conduct a MR study to investigate the causal association between gut microbiota and FM.
2 Materials and methods
2.1 Data sources
Genetic variants for gut microbiota were obtained from the GWAS summary statistic of MiBioGen consortium (MiBioGen; Kurilshikov et al., 2021). This dataset includes 18,340 samples from 24 cohorts. Microbiota quantitative trait loci mapping was performed to identify host genetic variants that were mapped to genetic loci associated with the abundance levels of gut microbiota. In this dataset, genus was the lowest taxonomic level, and 131 genera were identified, with 12 unknown genera (Kurilshikov et al., 2021). Therefore, 119 bacterial genera were included in this MR study for analysis. Genetic variants for FM were obtained from GWAS summary statistics of FinnGen consortium R9 release data (Kurki et al., 2023). This dataset includes 168,378 European participants consisted of 737 cases and 167,641 controls. Each participating cohort had previously obtained ethical approvals, so no additional ethical approvals or informed consents were required.
2.2 Instrumental variable selection
To identify the potential instrumental variables, the single nucleotide polymorphisms (SNPs) for each bacterial genus were screened based on the locus-wide significance threshold (p < 1.0×10−5). Then, to eliminate non-random associations between allelic variants at different loci, the linkage disequilibrium (LD) between these SNPs were calculated on the base of clumping window size = 10,000 kb and R2 < 0.001, using the European population as reference.
2.3 Two-sample and bidirectional MR
Multiple MR methods were used to investigate the causal association between each bacterial genus and FM, including inverse variance weighted (IVW), MR-Egger and weighted media.
We used the IVW method as the primary analysis. IVW is one of the most commonly used method in MR that uses the meta-analysis method to aggregate the Wald values of SNPs and derive an overall estimate of the effect (Burgess et al., 2013). In the absence of heterogeneity and horizontal pleiotropy, we prefer IVW to estimate the causal associations. MR-Egger is another commonly used method in MR that relaxes the assumption of no horizontal pleiotropy. Based on the Instrument strength independent of direct effect (InSIDE) assumption, it estimates the causal effect by fitting a regression model that includes the intercept term and the weighted average of the ratio estimates. However, incorporating the intercept term in regression correspondingly causes statistically less efficient estimates of causal effects (Bowden et al., 2015). When there is horizontal pleiotropy, the results obtained from the MR-Egger are preferred. The weighted median method is a robust method in MR that provides stable estimates even when up to 50% of the instruments are invalid (Hartwig et al., 2017). When there is heterogeneity but no horizontal pleiotropy present, the results obtained from the weighted median method are preferred.
Bidirectional MR was used to exclude the possible causal effect of FM on gut microbiota. The reverse MR analyses were only conducted for bacterial genera that were significantly causal associated with FM.
2.4 Sensitivity analysis
Sensitivity analyses were conducted on the MR results, including heterogeneity test, leave-one-out test, pleiotropy test.
To assess the heterogeneity of instrumental variables (IVs), the Cochran’s IVW Q statistics were calculated. If there is a p-value <0.05, it indicates the presence of heterogeneity. To assess the horizontal pleiotropy, the MR-Egger regression was used. If the intercept term has a significant deviation (p-value <0.05) from zero, it indicates the presence of horizontal pleiotropy. The MR-PRESSO analysis was also used to detect and reduce horizontal pleiotropy. It can detect and remove significant outliers (p-value <0.05) but it requires a minimum of 50% valid genetic variants and relies on the InSIDE assumptions (Verbanck et al., 2018). The Leave-one-out test was performed by excluding each of IV in turn to evaluate whether any of them exerted a disproportionate impact on the result. The F-statistic (Staiger and Stock, 1997) of each IV was calculated to evaluate their strength. IVs with F-statistic <10 were considered as weak instrumental variables, which may introduce weak instrumental bias to the result.
2.5 Statistical analysis
All statistical analyses were conducted using R version 4.3.0, using the TwoSampleMR (Hemani et al., 2018) and forestplot (Gordon and Lumley, 2022) R packages.
3 Results
We identified a total of 1,295 SNPs as IVs for the gut microbiota, as is shown in Supplementary Table S1. Based on the MR results (Supplementary Table S2), we found that Coprococcus2, Eggerthella, Lactobacillus, FamilyXIIUC001 and Olsenella were causal associated with FM in at least one MR method (Figure 1).
We focused on the results of the IVW method, while considering the results of other methods as reference. As shown in Figure 1, Coprococcus2 (OR = 2.317, p-value = 0.005, 95% CI: 1.289–4.167), Eggerthella (OR = 1.897, p-value = 0.001, 95% CI: 1.313–2.741) and Lactobacillus (OR = 1.576, p-value =0.020, 95% CI: 1.073–2.315) could increase the risk of FM. FamillyXIIIUCG001 (OR = 0.528, p-value = 0.038, 95% CI: 0.289–0.964) and Olsenella (OR = 0.747, p-value = 0.050, 95% CI: 0.557–1.000) could decrease the risk of FM. The weighted median method also supported the result of Eggerthella, Lactobacillus and FamilyXIIIUCG001, while the weighted median method for Coprococcus2 and Olsenella, and the MR-Egger method did not find significant casual association, and their p-values were all greater than 0.1. Forest plots that reflected the single effect of IVs of these bacterial genera were shown in Supplementary Figure S1. In addition, the reverse MR analyses (Supplementary Table S3) did not find FM have any significant casual effect on gut microbiota.
We conducted a series of sensitivity analysis to ensure the reliability and robustness of the results. There was no significant heterogeneity of IVs as indicated by the non-significant results of Cochran’s IVW Q test (Supplementary Table S4). On the visual inspection of funnel plots (Figure 2), the MR-Egger method showed a presence of potential outliers, while the IVW method did not. And there was no significant horizontal pleiotropy according to the results from intercept analysis in the MR-Egger regression (Supplementary Table S5) and MR-PRESSO analysis (Supplementary Table S6). On the visual inspection of scatter plot (Figure 3), only the MR-Egger method for Eggerthella showed a significant deviation of the slopes and intercept term compared to the other two methods. The results of leave-one-out plots (Figure 4) were similar after excluding each IV from the study, which indicated that no single SNP had a disproportionate impact on the total results. The F-statistics of the IVs (Supplementary Table S7) were all greater than 10, indicating that the results are unlikely to be affected by weak instrumental bias.
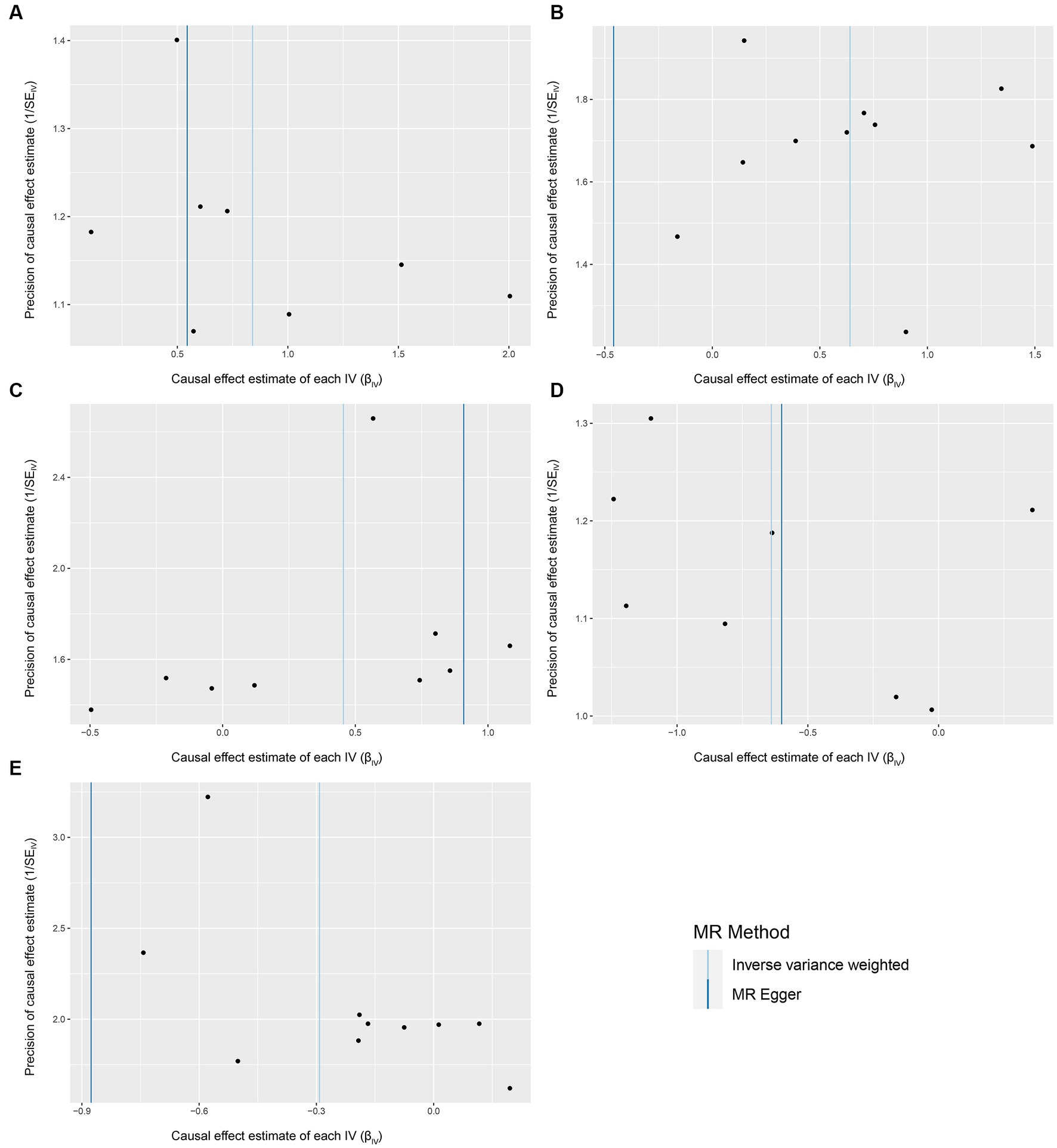
Figure 2. Funnel plots for the causal association between gut microbiota and FM. (A) Coprococcus2; (B) Eggerthella; (C) Lactobacillus; (D) FamillyXIIIUCG001; (E) Olsenella; funnel plots are scatter plots where the estimated causal effects of IVs are plotted against a measure of their precision. The X-axis represents the precision of the causal estimates, and the Y-axis represents the estimated causal effect of each genetic variant. In an unbiased scenario, where there is no heterogeneity, the scatter points on the funnel plot should exhibit a symmetric distribution resembling an inverted funnel shape.
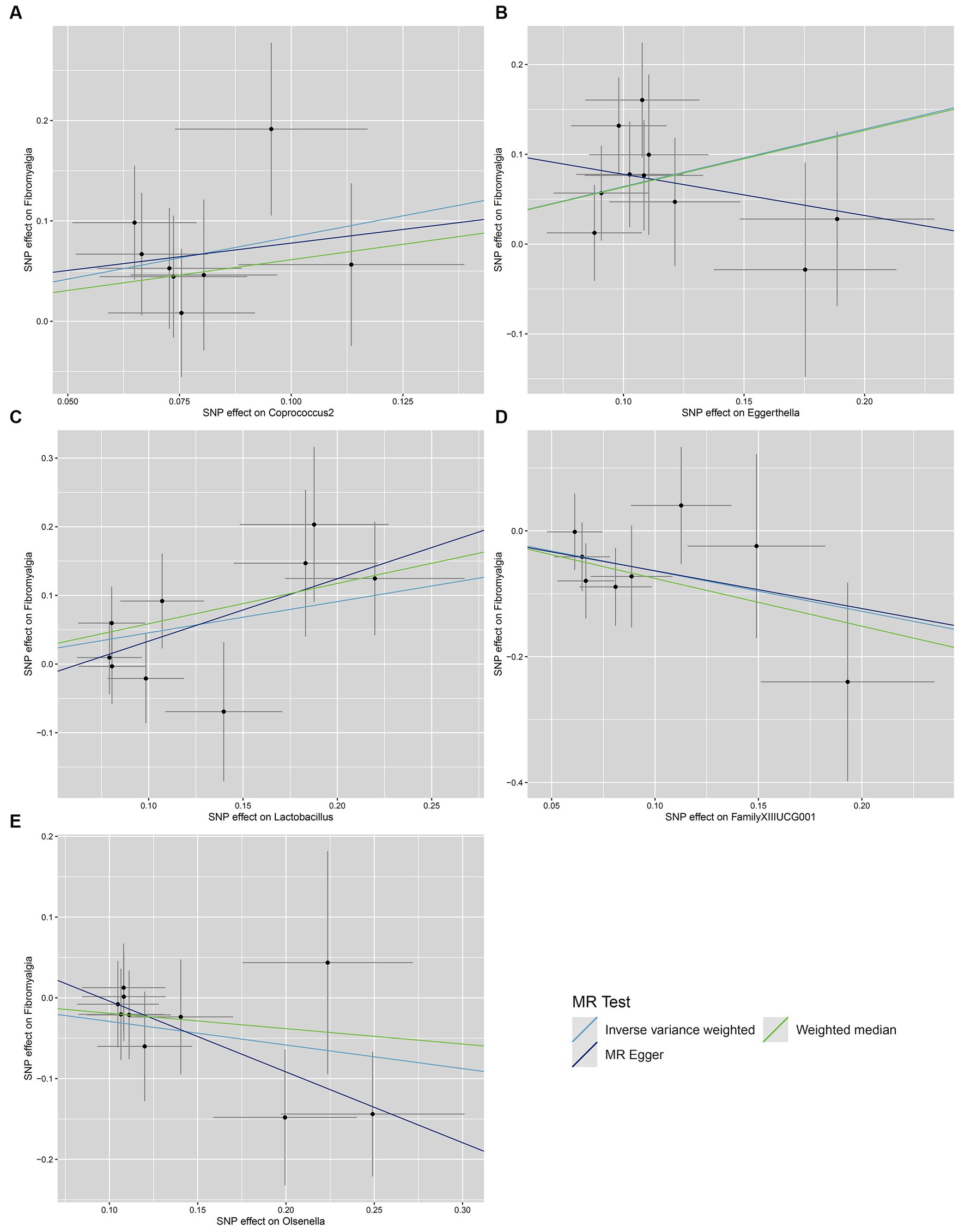
Figure 3. Scatter plots for the causal association between gut microbiota and FM. (A) Coprococcus2; (B) Eggerthella; (C) Lactobacillus; (D) FamillyXIIIUCG001; (E) Olsenella; scatter plots are used to visualize the association of IVs with exposure (gut microbiota) and outcome (FM). The X-axis and Y-axis, respectively, represent the effects of IVs on the exposure and outcome, with vertical and horizontal lines indicating the 95% confidence intervals for IVs. The slope of each line corresponding to the estimated MR effect of each method.
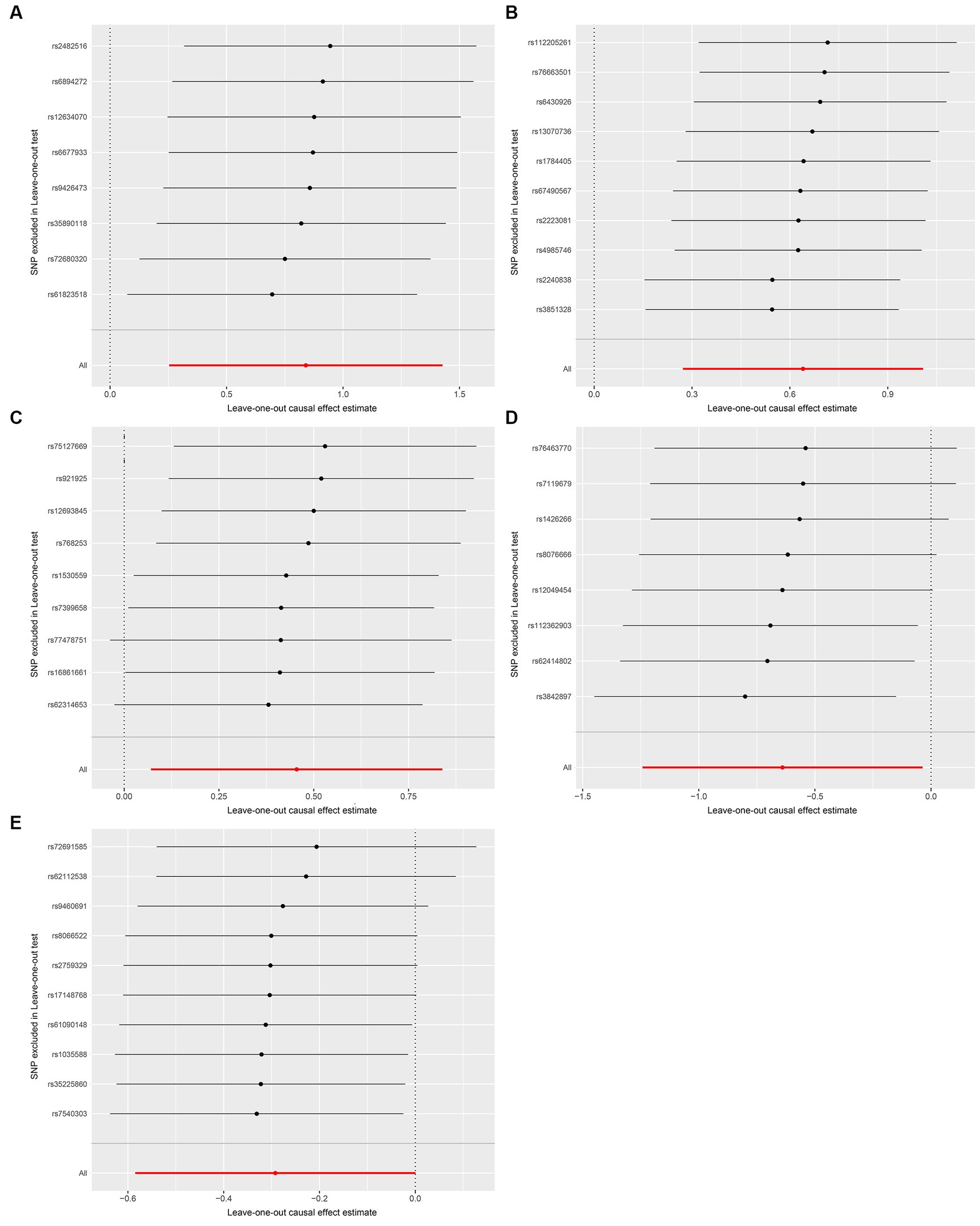
Figure 4. Leave-one-out plots for the causal association between gut microbiota and FM. (A) Coprococcus2; (B) Eggerthella; (C) Lactobacillus; (D) FamillyXIIIUCG001; (E) Olsenella; the leave-one-out plots are used to visualize the overall estimate of IVW (red horizontal line) and its estimates after the removal of each IV (black horizontal line).
4 Discussion
Gut microbiota is a crucial element of human health, intricately involved in various physiological functions within the human body (Zhang et al., 2020). Microbiota-gut-brain axis provide us a new way to understand FM. Potential associations between gut microbiota and FM has been identified, although these associations are correlative rather than causal (Clos-Garcia et al., 2019; Albayrak et al., 2021; Minerbi et al., 2022a,b). If a causal relationship between gut microbiota and FM is established, the way may be paved for the development of microbiota-based therapies and diagnostics for FM. In this study, we conducted a series of MR analyses to investigate the causal association between gut microbiota and FM, using the GWAS summary statistic from MiBioGen and FinnGen consortium. We found that Coprococcus2, Eggerthella, Lactobacillus, FamillyXIIIUCG001 and Olsenella were casually associated with FM.
Coprococcus2, Eggerthella and Lactobacillus were found to increase the risk of FM. Coprococcus2 is a bacterial genus producing short chain fatty acids (SCFAs), particularly butyric acid. Butyric acid and butyrate are often considered to maintain intestinal barrier function because it promotes the expression of mucus, antimicrobial peptides, and tight junction proteins (Garofalo et al., 2023). However, the effects of butyrate may be dose-dependent, as high concentrations of butyrate have been observed to induce intestinal cell apoptosis, thereby damage the intestinal barrier (Huang et al., 2014). It has also been observed an increase in the abundance of butyrate-producing bacteria and level of butyrate in patients with FM (Minerbi et al., 2019; Erdrich et al., 2020). In addition, high concentrations and mixtures of SCFAs can promote microglia activation and amplify the inflammatory process (Pender et al., 2000; Sampson et al., 2016). We proposed that it is the imbalance rather than deficiency of SCFAs that contribute to the development of FM. Coprococcus2 may increase the risk of FM by overproducing butyric acid.
Although there is a lack of research about the association between Eggerthella and FM, increased abundance of Eggerthella has been reported in various disorders, such as depression (Barandouzi et al., 2020; Liu et al., 2022), rheumatoid arthritis (Chen et al., 2021a), schizophrenia (Karpiński et al., 2023), systemic lupus erythematosus (He et al., 2016), and multiple sclerosis (Chang and Choi, 2023), all of which are common comorbidities of FM. Eggerthella can increase intestinal permeability and promote the production of pro-inflammatory cytokines (Salem et al., 2019). It can use ornithine as substrate to generate energy, producing citrulline and carbamyol phosphate as byproducts. And increased levels of citrulline in the gut participate in citrullination, leading to antibody production by the immune response (Chen et al., 2016). It can also activate Th17 cells, inducing an inflammatory response mediated by the Th17/Treg cell imbalance (Liu et al., 2022). These findings indicate the potential causal association between Eggerthella and FM.
Lactobacillus could increase the risk of FM. This is counterintuitive because Lactobacillus is regarded as a probiotic (Azad et al., 2018). In fact, the role of Lactobacillus is relatively heterogeneous. Certain species of Lactobacillus and have been shown to have potential association with FM. For example, Lactobacillus plantarum IS-10506 can upregulate serotonin (5-hydroxytryptamine, 5-HT) levels (Ranuh et al., 2019), while Lactobacillus acidophilus (Cao et al., 2018b) and Lactobacillus rhamnosus (Cao et al., 2018a) can downregulate 5-HT levels. Serotonergic dysfunction is one major hypothesis of fibromyalgia, which is supported by the efficacy of drugs that alter serotonin metabolism (Wolfe et al., 2013; Chinn et al., 2016). Another example, the Lactobacillus ruminis can stimulate the production of tumor necrosis factor (TNF) (Taweechotipatr et al., 2009). TNF can not only cross the blood-brain barrier but also increases its permeability (Banks et al., 1995; Tsao et al., 2001). TNF is also an activator of microglia (Klapal et al., 2016). We speculated that Lactobacillus ruminis may be involved in membrane dysfunction triggered by microglia through elevated levels of TNF, thus contributing to the development of FM. Unfortunately, due to the lack of statistic of genetic variant for bacterial species, we can only obtain the overall effect of the genus Lactobacillus.
FamilyXIIIUCG001 and Olsenella were found to decrease the risk of FM. There is limited research on these two bacterial genera, but we believe that they may be of significant importance for probiotic treatment of FM. In anhedonia-like phenotype rodents with neuropathic pain, the abundance of FamilyXIIIUCG001 were lower in susceptible group than in sham group and resilient group (Yang et al., 2019). In addition, in mice with chronic social defeat stress-induced depressive-like behavior receiving Clostridium butyricum miyairi 588 treatment, FamilyXIIIUCG001 was negatively correlated with depression-like behavior which is often comorbid with FM (Liu et al., 2014; Tian et al., 2019). As for Olsenella, a Mendelian randomization study has demonstrated that Olsenella can decrease the risk of Migraine (He et al., 2023). Another study found that Olsenella is negatively associated with the risk of low back pain (Su et al., 2023).
This study has several strengths. Firstly, we investigated the causal association between gut microbiota and FM through MR method, which can exclude the interference of confounding factors and reversing causation. Secondly, we obtained the summary statistics of genetic variants of diseases from the publicly available GWAS datasets, ensuring the reliability of IVs in the MR analysis. Thirdly, to account for bias related to study design, we performed several MR sensitivity analyses. Fourthly, we conducted reverse MR to assess the reversing causation, which could also contribute to the robustness of the study.
However, there are also several limitations in this study. Firstly, to perform sensitivity analysis, a broader range of genetic variations should be included as instrumental variables. Therefore, we did not use the traditional GWAS significance threshold (p < 5E–8) to screen SNPs. Secondly, the smallest taxonomic level in the original GWAS study is the genus level. Therefore, we failed to investigate the causal association with FM of gut microbiota at species level, which can be more accurate. Further research is needed to obtain the GWAS statistics of genetic variants for bacterial species. Thirdly, most of participants in these GWAS were European. This leads to the concern that our results may not be entirely applicable to other descent.
5 Conclusion
This MR study found that gut microbiota, including Coprococcus2, Eggerthella, Lactobacillus, FamillyXIIIUCG001 and Olsenella were casually associated with FM. It could contribute to the development of effective preventive and therapeutic strategies for FM.
Data availability statement
The datasets presented in this study can be found in online repositories. The names of the repository/repositories and accession number(s) can be found in the article/Supplementary material.
Ethics statement
Ethical approval was not required for the study involving humans in accordance with the local legislation and institutional requirements. Written informed consent to participate in this study was not required from the participants or the participants’ legal guardians/next of kin in accordance with the national legislation and the institutional requirements.
Author contributions
ZW: Data curation, Formal analysis, Investigation, Methodology, Software, Visualization, Writing – original draft, Writing – review & editing. DJ: Data curation, Formal analysis, Validation, Writing – original draft, Writing – review & editing. MZ: Validation, Writing – review & editing. YT: Validation, Writing – review & editing. YH: Conceptualization, Funding acquisition, Project administration, Resources, Supervision, Writing – review & editing.
Funding
The author(s) declare financial support was received for the research, authorship, and/or publication of this article. This study was funded by Double First Class University Plan (MOE, China Ministry of Education) and the Minzu University 985 Academic Team-Building Fund (No. 2018MDTD25C) and Beijing Municipal Science and Technology Commission.
Conflict of interest
The authors declare that the research was conducted in the absence of any commercial or financial relationships that could be construed as a potential conflict of interest.
Publisher’s note
All claims expressed in this article are solely those of the authors and do not necessarily represent those of their affiliated organizations, or those of the publisher, the editors and the reviewers. Any product that may be evaluated in this article, or claim that may be made by its manufacturer, is not guaranteed or endorsed by the publisher.
Supplementary material
The Supplementary material for this article can be found online at: https://www.frontiersin.org/articles/10.3389/fmicb.2023.1305361/full#supplementary-material
References
Albayrak, B., Süsgün, S., Küçükakkaş, O., Akbaş, F., Yabacı, A., and Özçelik, S. (2021). Investigating of relation between fibromyalgia syndrome and intestinal microbiota. Mikrobiyol. Bul. 55, 146–160. doi: 10.5578/mb.20219903
Azad, M. A. K., Sarker, M., Li, T., and Yin, J. (2018). Probiotic species in the modulation of gut microbiota: an overview. Biomed. Res. Int. 2018, 1–8. doi: 10.1155/2018/9478630
Banks, W. A., Kastin, A. J., and Broadwell, R. D. (1995). Passage of cytokines across the blood-brain barrier. Neuroimmunomodulation 2, 241–248. doi: 10.1159/000097202
Barandouzi, Z. A., Starkweather, A. R., Henderson, W. A., Gyamfi, A., and Cong, X. S. (2020). Altered composition of gut microbiota in depression: a systematic review. Front Psychiatry 11:541. doi: 10.3389/fpsyt.2020.00541
Bowden, J., Davey Smith, G., and Burgess, S. (2015). Mendelian randomization with invalid instruments: effect estimation and bias detection through egger regression. Int. J. Epidemiol. 44, 512–525. doi: 10.1093/ije/dyv080
Burgess, S., Butterworth, A., and Thompson, S. G. (2013). Mendelian randomization analysis with multiple genetic variants using summarized data. Genet. Epidemiol. 37, 658–665. doi: 10.1002/gepi.21758
Cao, Y. N., Feng, L. J., Liu, Y. Y., Jiang, K., Zhang, M. J., Gu, Y. X., et al. (2018a). Effect of Lactobacillus rhamnosus GG supernatant on serotonin transporter expression in rats with post-infectious irritable bowel syndrome. World J. Gastroenterol. 24, 338–350. doi: 10.3748/wjg.v24.i3.338
Cao, Y. N., Feng, L. J., Wang, B. M., Jiang, K., Li, S., Xu, X., et al. (2018b). Lactobacillus acidophilus and Bifidobacterium longum supernatants upregulate the serotonin transporter expression in intestinal epithelial cells. Saudi J. Gastroenterol. 24, 59–66. doi: 10.4103/sjg.SJG_333_17
Chang, S.-H., and Choi, Y. (2023). Gut dysbiosis in autoimmune diseases: association with mortality. Front. Cell. Infect. Microbiol. 13:1157918. doi: 10.3389/fcimb.2023.1157918
Chen, Y., Ma, C., Liu, L., He, J., Zhu, C., Zheng, F., et al. (2021a). Analysis of gut microbiota and metabolites in patients with rheumatoid arthritis and identification of potential biomarkers. Aging 13, 23689–23701. doi: 10.18632/aging.203641
Chen, J., Wright, K., Davis, J. M., Jeraldo, P., Marietta, E. V., Murray, J., et al. (2016). An expansion of rare lineage intestinal microbes characterizes rheumatoid arthritis. Genome Med. 8:43. doi: 10.1186/s13073-016-0299-7
Chen, Y., Xu, J., and Chen, Y. (2021b). Regulation of neurotransmitters by the gut microbiota and effects on cognition in neurological disorders. Nutrients 13:2099. doi: 10.3390/nu13062099
Chinn, S., Caldwell, W., and Gritsenko, K. (2016). Fibromyalgia pathogenesis and treatment options update. Curr. Pain Headache Rep. 20:25. doi: 10.1007/s11916-016-0556-x
Clos-Garcia, M., Andrés-Marin, N., Fernández-Eulate, G., Abecia, L., Lavín, J. L., van Liempd, S., et al. (2019). Gut microbiome and serum metabolome analyses identify molecular biomarkers and altered glutamate metabolism in fibromyalgia. EBioMedicine 46, 499–511. doi: 10.1016/j.ebiom.2019.07.031
Davey Smith, G., and Hemani, G. (2014). Mendelian randomization: genetic anchors for causal inference in epidemiological studies. Hum. Mol. Genet. 23, R89–R98. doi: 10.1093/hmg/ddu328
Emdin, C. A., Khera, A. V., and Kathiresan, S. (2017). Mendelian randomization. JAMA 318, 1925–1926. doi: 10.1001/jama.2017.17219
Erdrich, S., Hawrelak, J. A., Myers, S. P., and Harnett, J. E. (2020). Determining the association between fibromyalgia, the gut microbiome and its biomarkers: a systematic review. BMC Musculoskelet. Disord. 21:181. doi: 10.1186/s12891-020-03201-9
Favretti, M., Iannuccelli, C., and Di Franco, M. (2023). Pain biomarkers in fibromyalgia syndrome: current understanding and future directions. Int. J. Mol. Sci. 24:10443. doi: 10.3390/ijms241310443
Garofalo, C., Cristiani, C. M., Ilari, S., Passacatini, L. C., Malafoglia, V., Viglietto, G., et al. (2023). Fibromyalgia and irritable bowel syndrome interaction: a possible role for gut microbiota and gut-brain axis. Biomedicine 11:1701. doi: 10.3390/biomedicines11061701
Generoso, J. S., Giridharan, V. V., Lee, J., Macedo, D., and Barichello, T. (2021). The role of the microbiota-gut-brain axis in neuropsychiatric disorders. Braz. J. Psychiatry 43, 293–305. doi: 10.1590/1516-4446-2020-0987
Giorgi, V., Bazzichi, L., Batticciotto, A., Pellegrino, G., Di Franco, M., Sirotti, S., et al. (2023). Fibromyalgia: one year in review 2023. Clin. Exp. Rheumatol. 41, 1205–1213. doi: 10.55563/clinexprheumatol/257e99
Gordon, M., and Lumley, T. (2022). forestplot: advanced forest plot using ‘grid’ graphics Available at: https://cran.r-project.org/web//packages//forestplot/forestplot.pdf
Guo, R., Chen, L. H., Xing, C., and Liu, T. (2019). Pain regulation by gut microbiota: molecular mechanisms and therapeutic potential. Br. J. Anaesth. 123, 637–654. doi: 10.1016/j.bja.2019.07.026
Hartwig, F. P., Davey Smith, G., and Bowden, J. (2017). Robust inference in summary data Mendelian randomization via the zero modal pleiotropy assumption. Int. J. Epidemiol. 46, 1985–1998. doi: 10.1093/ije/dyx102
He, Z., Shao, T., Li, H., Xie, Z., and Wen, C. (2016). Alterations of the gut microbiome in Chinese patients with systemic lupus erythematosus. Gut Pathog. 8:64. doi: 10.1186/s13099-016-0146-9
He, Q., Wang, W., Xiong, Y., Tao, C., Ma, L., Ma, J., et al. (2023). A causal effects of gut microbiota in the development of migraine. J. Headache Pain 24:90. doi: 10.1186/s10194-023-01609-x
Hemani, G., Zheng, J., Elsworth, B., Wade, K. H., Haberland, V., Baird, D., et al. (2018). The MR-base platform supports systematic causal inference across the human phenome. eLife 7. doi: 10.7554/eLife.34408
Huang, X. Z., Li, Z. R., Zhu, L. B., Huang, H. Y., Hou, L. L., and Lin, J. (2014). Inhibition of p38 mitogen-activated protein kinase attenuates butyrate-induced intestinal barrier impairment in a Caco-2 cell monolayer model. J. Pediatr. Gastroenterol. Nutr. 59, 264–269. doi: 10.1097/mpg.0000000000000369
Ibrahim, I., Krishnan, S., Ayariga, J., Xu, J., Robertson, B., Sreepriya, M., et al. (2022). Modulatory effect of gut microbiota on the gut-brain, gut-bone axes, and the impact of cannabinoids. Meta 12:1247. doi: 10.3390/metabo12121247
Karpiński, P., Żebrowska-Różańska, P., Kujawa, D., Łaczmański, Ł., Samochowiec, J., Jabłoński, M., et al. (2023). Gut microbiota alterations in schizophrenia might be related to stress exposure: findings from the machine learning analysis. Psychoneuroendocrinology 155:106335. doi: 10.1016/j.psyneuen.2023.106335
Klapal, L., Igelhorst, B. A., and Dietzel-Meyer, I. D. (2016). Changes in neuronal excitability by activated microglia: differential Na+ current upregulation in pyramid-shaped and bipolar neurons by TNF-α and IL-18. Front. Neurol. 7:44. doi: 10.3389/fneur.2016.00044
Kurilshikov, A., Medina-Gomez, C., Bacigalupe, R., Radjabzadeh, D., Wang, J., Demirkan, A., et al. (2021). Large-scale association analyses identify host factors influencing human gut microbiome composition. Nat. Genet. 53, 156–165. doi: 10.1038/s41588-020-00763-1
Kurki, M. I., Karjalainen, J., Palta, P., Sipilä, T. P., Kristiansson, K., Donner, K. M., et al. (2023). FinnGen provides genetic insights from a well-phenotyped isolated population. Nature 613, 508–518. doi: 10.1038/s41586-022-05473-8
Liu, Y.-T., Shao, Y.-W., Yen, C.-T., and Shaw, F.-Z. (2014). Acid-induced hyperalgesia and anxio-depressive comorbidity in rats. Physiol. Behav. 131, 105–110. doi: 10.1016/j.physbeh.2014.03.030
Liu, L., Wang, H., Zhang, H., Chen, X., Zhang, Y., Wu, J., et al. (2022). Toward a deeper understanding of gut microbiome in depression: the promise of clinical applicability. Adv. Sci. 9:e2203707. doi: 10.1002/advs.202203707
Mayer, E. A., Nance, K., and Chen, S. (2022). The gut-brain axis. Annu. Rev. Med. 73, 439–453. doi: 10.1146/annurev-med-042320-014032
MiBioGen. MiBioGen consortium. Available at: https://mibiogen.gcc.rug.nl/menu/main/home/
Minerbi, A., Brereton, N. J. B., Anjarkouchian, A., Moyen, A., Gonzalez, E., Fitzcharles, M. A., et al. (2022a). Dietary intake is unlikely to explain symptom severity and syndrome-specific microbiome alterations in a cohort of women with fibromyalgia. Int. J. Environ. Res. Public Health 19:3254. doi: 10.3390/ijerph19063254
Minerbi, A., and Fitzcharles, M. A. (2020). Gut microbiome: pertinence in fibromyalgia. Clin. Exp. Rheumatol. 38, 99–104.
Minerbi, A., Gonzalez, E., Brereton, N. J. B., Anjarkouchian, A., Dewar, K., Fitzcharles, M. A., et al. (2019). Altered microbiome composition in individuals with fibromyalgia. Pain 160, 2589–2602. doi: 10.1097/j.pain.0000000000001640
Minerbi, A., Gonzalez, E., Brereton, N., Fitzcharles, M.-A., Chevalier, S., and Shir, Y. (2022b). Altered serum bile acid profile in fibromyalgia is associated with specific gut microbiome changes and symptom severity. Pain 164, e66–e76. doi: 10.1097/j.pain.0000000000002694
Pender, S. L. F., Quinn, J. J., Sanderson, I. R., and MacDonald, T. T. (2000). Butyrate upregulates stromelysin-1 production by intestinal mesenchymal cells. Am. J. Physiol. Gastrointest. Liver Physiol. 279, G918–G924. doi: 10.1152/ajpgi.2000.279.5.G918
Ranuh, R., Athiyyah, A. F., Darma, A., Risky, V. P., Riawan, W., Surono, I. S., et al. (2019). Effect of the probiotic Lactobacillus plantarum IS-10506 on BDNF and 5HT stimulation: role of intestinal microbiota on the gut-brain axis. Iran J. Microbiol. 11, 145–150. doi: 10.18502/ijm.v11i2.1077
Salem, F., Kindt, N., Marchesi, J. R., Netter, P., Lopez, A., Kokten, T., et al. (2019). Gut microbiome in chronic rheumatic and inflammatory bowel diseases: similarities and differences. United European Gastroenterol J 7, 1008–1032. doi: 10.1177/2050640619867555
Sampson, T. R., Debelius, J. W., Thron, T., Janssen, S., Shastri, G. G., Ilhan, Z. E., et al. (2016). Gut microbiota regulate motor deficits and Neuroinflammation in a model of Parkinson’s disease. Cell 167, 1469–1480.e12. doi: 10.1016/j.cell.2016.11.018
Sarzi-Puttini, P., Giorgi, V., Marotto, D., and Atzeni, F. (2020). Fibromyalgia: an update on clinical characteristics, aetiopathogenesis and treatment. Nat. Rev. Rheumatol. 16, 645–660. doi: 10.1038/s41584-020-00506-w
Socała, K., Doboszewska, U., Szopa, A., Serefko, A., Włodarczyk, M., Zielińska, A., et al. (2021). The role of microbiota-gut-brain axis in neuropsychiatric and neurological disorders. Pharmacol. Res. 172:105840. doi: 10.1016/j.phrs.2021.105840
Staiger, D., and Stock, J. H. (1997). Instrumental variables regression with weak instruments. Econometrica 65, 557–586. doi: 10.2307/2171753
Su, M., Tang, Y., Kong, W., Zhang, S., and Zhu, T. (2023). Genetically supported causality between gut microbiota, gut metabolites and low back pain: a two-sample Mendelian randomization study. Front. Microbiol. 14:1157451. doi: 10.3389/fmicb.2023.1157451
Taweechotipatr, M., Iyer, C., Spinler, J. K., Versalovic, J., and Tumwasorn, S. (2009). Lactobacillus saerimneri and Lactobacillus ruminis: novel human-derived probiotic strains with immunomodulatory activities. FEMS Microbiol. Lett. 293, 65–72. doi: 10.1111/j.1574-6968.2009.01506.x
Tian, T., Xu, B., Qin, Y., Fan, L., Chen, J., Zheng, P., et al. (2019). Clostridium butyricum miyairi 588 has preventive effects on chronic social defeat stress-induced depressive-like behaviour and modulates microglial activation in mice. Biochem. Biophys. Res. Commun. 516, 430–436. doi: 10.1016/j.bbrc.2019.06.053
Tsao, N., Hsu, H. P., Wu, C. M., Liu, C. C., and Lei, H. Y. (2001). Tumour necrosis factor-alpha causes an increase in blood-brain barrier permeability during sepsis. J. Med. Microbiol. 50, 812–821. doi: 10.1099/0022-1317-50-9-812
Verbanck, M., Chen, C. Y., Neale, B., and Do, R. (2018). Detection of widespread horizontal pleiotropy in causal relationships inferred from Mendelian randomization between complex traits and diseases. Nat. Genet. 50, 693–698. doi: 10.1038/s41588-018-0099-7
Wolfe, F., Brähler, E., Hinz, A., and Häuser, W. (2013). Fibromyalgia prevalence, somatic symptom reporting, and the dimensionality of polysymptomatic distress: results from a survey of the general population. Arthritis Care Res. 65, 777–785. doi: 10.1002/acr.21931
Yang, C., Fang, X., Zhan, G., Huang, N., Li, S., Bi, J., et al. (2019). Key role of gut microbiota in anhedonia-like phenotype in rodents with neuropathic pain. Transl. Psychiatry 9:57. doi: 10.1038/s41398-019-0379-8
Yu, X. H., Yang, Y. Q., Cao, R. R., Bo, L., and Lei, S. F. (2021). The causal role of gut microbiota in development of osteoarthritis. Osteoarthr. Cartil. 29, 1741–1750. doi: 10.1016/j.joca.2021.08.003
Zhang, D., Li, S., Wang, N., Tan, H. Y., Zhang, Z., and Feng, Y. (2020). The cross-talk between gut microbiota and lungs in common lung diseases. Front. Microbiol. 11:301. doi: 10.3389/fmicb.2020.00301
Keywords: gut microbiota, fibromyalgia, Mendelian randomization, causal association, gut-brain axis
Citation: Wang Z, Jiang D, Zhang M, Teng Y and Huang Y (2024) Causal association between gut microbiota and fibromyalgia: a Mendelian randomization study. Front. Microbiol. 14:1305361. doi: 10.3389/fmicb.2023.1305361
Edited by:
Edoardo Pasolli, University of Naples Federico II, ItalyReviewed by:
Joseph Atia Ayariga, Alabama State University, United StatesHaibo Shi, South China University of Technology, China
Copyright © 2024 Wang, Jiang, Zhang, Teng and Huang. This is an open-access article distributed under the terms of the Creative Commons Attribution License (CC BY). The use, distribution or reproduction in other forums is permitted, provided the original author(s) and the copyright owner(s) are credited and that the original publication in this journal is cited, in accordance with accepted academic practice. No use, distribution or reproduction is permitted which does not comply with these terms.
*Correspondence: Yaojiang Huang, eWFvamlhbmdAbXVjLmVkdS5jbg==
†These authors have contributed equally to this work