- 1Centre for Ecology and Evolution in Microbial Model Systems, Linnaeus University, Kalmar, Sweden
- 2Department of Ecology, Environment and Plant Sciences, Stockholm University, Stockholm, Sweden
- 3Department of Biology and Environmental Sciences, Linnaeus University, Kalmar, Sweden
Climate change related warming is a serious environmental problem attributed to anthropogenic activities, causing ocean water temperatures to rise in the coastal marine ecosystem since the last century. This particularly affects benthic microbial communities, which are crucial for biogeochemical cycles. While bacterial communities have received considerable scientific attention, the benthic eukaryotic community response to climate change remains relatively overlooked. In this study, sediments were sampled from a heated (average 5°C increase over the whole year for over 50 years) and a control (contemporary conditions) Baltic Sea bay during four different seasons across a year. RNA transcript counts were then used to investigate eukaryotic community changes under long-term warming. The composition of active species in the heated and control bay sediment eukaryotic communities differed, which was mainly attributed to salinity and temperature. The family level RNA transcript alpha diversity in the heated bay was higher during May but lower in November, compared with the control bay, suggesting altered seasonal activity patterns and dynamics. In addition, structures of the active eukaryotic communities varied between the two bays during the same season. Hence, this study revealed that long-term warming can change seasonality in eukaryotic diversity patterns. Relative abundances and transcript expression comparisons between bays suggested that some taxa that now have lower mRNA transcripts numbers could be favored by future warming. Furthermore, long-term warming can lead to a more active metabolism in these communities throughout the year, such as higher transcript numbers associated with diatom energy production and protein synthesis in the heated bay during winter. In all, these data can help predict how future global warming will affect the ecology and metabolism of eukaryotic community in coastal sediments.
Introduction
Climate change is a world-wide environmental problem caused by anthropogenic greenhouse gas emissions that results in an increase in average surface temperature (IPCC et al., 2008). As the largest ecosystem on Earth with an important role in geochemical cycling of key elements such as Fe, S, and Mn, the marine ecosystem is influenced by climate change with associated effects such as an increased ocean surface temperature of 1°C in the last century (IPCC et al., 2008; Abraham et al., 2013; Garcia-Soto et al., 2021) and higher dissolved carbon dioxide (CO2) concentrations (Connell et al., 2013). This leads to further problems such as acidification, salinity changes, water stratification, deoxygenation, and sea level rise (Connell et al., 2013; Breitburg et al., 2018; Malhi et al., 2020). In addition, mounting evidence indicates that climate change alters the biodiversity and community compositions of marine ecosystems. These include tropical regions experiencing species loss while temperate regions might experience increasing diversity as species migrate to the poles (Alabia et al., 2020) that has potentially far reaching implications for ecosystem services (Staniczenko et al., 2017).
Despite only consisting of 4% of the earth’s total area and 11% of the world’s oceans, coastal zones contain more than a third of the world’s human population and contribute with 90% of the catch from marine fisheries (Barbier, 2017). Coastal areas also provide many additional ecosystem services including carbon storage in estuaries and sediments, contaminant removal, and storm plus flooding buffering (Canuel et al., 2012). The coastal areas are also responsible for the majority of atmospheric methane emission from the marine environment (Borges et al., 2016; Weber et al., 2019). Eukaryotes are abundant in coastal systems and play important ecological roles by serving as both primary producers and consumers (Nagarkar et al., 2018). For example, they graze on prokaryotes (Massana et al., 2004) and deliver energy to higher trophic levels through the food web. Moreover, eukaryotes are also involved in the benthic–pelagic exchange process between the sediment and open water (Marcus and Boero, 1998) with the transfer of individual organisms as well as elements such as phosphorous and nitrogen (Fanning et al., 1982). One important habitat for eukaryotes is the benthic sediment, although it has received less attention compared to the pelagic environment (Salonen et al., 2019). The eukaryotic communities in sediments are complex and diverse, including various benthic macrofauna (e.g., Bivalvia), meiofauna (e.g., nematodes, protists), and algae (e.g., diatoms) (Bik et al., 2012; Broman et al., 2019b). Moreover, sediment acts as a reservoir for resting stages of phytoplankton and zooplankton (Brendonck and De Meester, 2003; Suikkanen et al., 2010) and harbors larvae of semi-aquatic insects for some time before they emerge to the terrestrial phase (Mason et al., 2022). The coastal biodiversity is threated by climate change and anthropogenic activities (Holon et al., 2018), resulting in many species disappearing (Pan et al., 2013), and such that it may correspondingly affect ecosystem processes. For example, low sediment biodiversity can decrease the coastal ecosystem’s stability and resistance, making it vulnerable to invasive species or other forms of disturbance (Levin et al., 2001). Therefore, it is essential to better understand how the structure and activity of coastal benthic eukaryotic communities is influenced by global warming.
The Baltic Sea is one of the largest brackish water areas in the world that it is relatively isolated due to a narrow connection to the North Sea (Stigebrandt, 2001). This sea has suffered from a high level of eutrophication over an extended time due to anthropogenic nutrient loading and atmospheric deposition of primarily nitrogen and phosphorus (Knuuttila et al., 2011). This eutrophication contributes to increased biomass production and elevated oxygen consumption (Reusch et al., 2018). In the last century, the area of year-round hypoxic “dead zones” in the Baltic Sea has expanded ten-fold (Carstensen et al., 2014). Correspondingly, the dead zone eukaryotic community structure has also been modified with an increased abundance of species tolerating low oxygen conditions such as nematodes (Broman et al., 2020) and lower hatching of zooplankton resting stages (Broman et al., 2015). While higher global warming-related oxygen consumption (Schmidtko et al., 2017) will likely magnify the influence of eutrophication and algae blooms (Reusch et al., 2018), it is not well known how the benthic eukaryotic community and especially the active groups will respond.
This research was conducted in a Baltic Sea bay that has been used as a discharge recipient of warm water from a nuclear power plant for more than 50 years, which has raised the average water temperature by approximately 5.1°C above a nearby, unaffected, control bay. This temperature difference is within the same order of magnitude of the expected temperature increase for the Baltic Sea (Andersson et al., 2015). Therefore, this bay can be regarded as a natural laboratory to study the influence of long-term climate change in a Baltic Sea coastal ecosystem (Seidel et al., 2022a). Previous studies of this model system have uncovered that the sediment prokaryote communities in the heated versus the control bay show a weakened resilience with microbial RNA transcripts for stress in the heated bay despite exposure to > 50 years of warming (Seidel et al., 2022a). In addition, the sediment surface microbial community (< 2 cm of depth) in the warmed conditions exhibited a higher diversity due to shallowing of geochemical layers (Seidel et al., 2022b).
In this study, a metatranscriptomic dataset from the heated and control Baltic Sea bay sediments at four seasonal time points over a year was interrogated to investigate whether and how the eukaryotic community composition and their active functions differed between the two bays and change over time. It was hypothesized that heating of coastal marine system leads to a difference in the species composition of sediment-bound eukaryotic communities as well as different seasonal dynamics in their transcriptome signatures, such as overall increased activities in the winter.
Materials and methods
Sampling sites and sediment cores
The sediment sample cores were collected using a kajak gravity corer from three sites in each of the heated (B: N 057° 25.259′ E 016° 40.130′, D: N 057° 25.387′ E 016° 40.104′, and F: N 057° 25.220′ E 016° 39.895′) and control (K: N 057° 26.011′ E 016° 41.022′, L: N 057° 25.964′ E 016° 40.914′, and M: N 057° 25.907′ E 016° 40.992′) Baltic Sea bays (Figure 1). The water depths for the sites were: B- 3 m, D- 1.2 m, F- 2.4 m, K- 2.8 m, L- 1.6 m, M- 4.9 m. Samples were collected in May, June, and November 2018 plus March in 2019 as previously described (Broman et al., 2019a; Seidel et al., 2022b). Briefly, sediments were collected with a 50 cm-long kayak-type gravity corer, immediately sliced in the field, and the 0–1 cm sediment surface was aseptically retained for nucleic acid extractions along with chemical analyses (Broman et al., 2019a). All nucleic acid samples were flash frozen in liquid nitrogen before being returned to the laboratory on the same day and stored in a −80°C freezer.
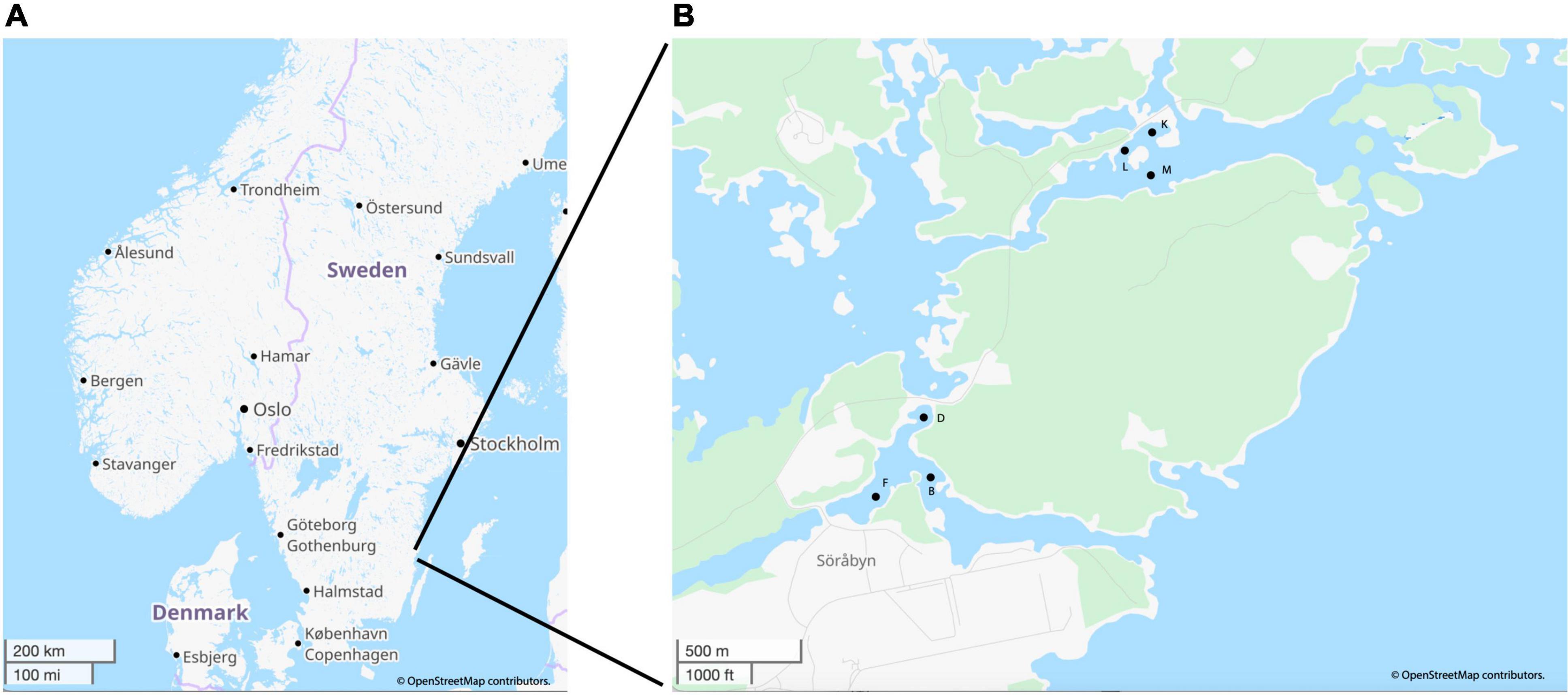
Figure 1. Map of the general sampling are near Oskarshamn, Sweden (A) and a zoomed in image of the heated and control bays with specific sampling points (B). Image generated and adapted in OpenStreetMap, licensed under CC BY-SA 2.0.
Temperature measurements and physiochemical analyses
Surface water temperature (1 m below the sea surface) was monitored in the two bays by using data loggers (HOBOware, Onset Computer Corporation, USA) at each of the sampling sites from December 2017 until November 2019. In addition, the bottom water temperature was measured in situ (Multiline™ sensor, WTW™) during each sampling time point (Seidel et al., 2022b). The chemical analyses of pore water including oxygen, pH, salinity, organic matter, nitrate, nitrite, ferrous iron, total iron, and sulfate on sediment were conducted as previously described (Broman et al., 2019a).
RNA extractions and sequencing
RNA was extracted from the sediment 0–1 cm depth fractions using the RNeasy® PowerSoil Total RNA Kit (QIAGEN) and the phenol/chloroform/isoamyl alcohol method as previously described (Seidel et al., 2022b). Total RNA samples were sent to the DOE Joint Genome Institute (JGI) at the Lawrence Berkeley National Laboratory, Berkeley, USA where they performed sequencing on the Illumina NovaSeq600 platform to produce sequences with 2 × 151 bp read length. Quality control was conducted to eliminate contaminants and ribosomal RNA reads by using BBDuk (v. 38.75) and BBMap (Bushnell, 2023) that resulted in an average of 64.83 % of the reads being retained [as previously reported (Seidel et al., 2022b)].
Bioinformatics and statistical analyses
The filtered mRNA reads provided by JGI were co-assembled by Megahit v.1.2.9 (Li et al., 2015) with default settings. TransDecoder v.5.5.0 (Haas, 2023) with default settings (first LongOrfs function then Predict function) was used to identify candidate coding regions within the transcript sequences, which generated open reading frames (ORFs) from the assembled contigs. After that, Bowtie2 with default settings v.2.3.5.1 (Langmead and Salzberg, 2012) was used align the sequencing reads. The Bowtie2 output was used to generate a counts table using FeatureCounts v.2.0.3 (Liao et al., 2014) with standard settings. Taxonomic annotation was performed using the software Eukulele v.2.0.2 (Krinos et al., 2023) in default settings against the reference PhyloDB v.1.075 database (Allen, 2020). Since the focus of this paper was on eukaryotes, only contigs annotated as within the Eukaryote domain were retained for further analyses. Functional annotation used the automatic annotation servers GhostKOALA in KEGG (Kyoto Encyclopedia of Genes and Genomes) website, which is an internal annotation tool for KEGG Orthology assignment (Kanehisa et al., 2016).
The Alpha Diversity indices (Shannon’s H and evenness) were normalized by scaling with ranked subsampling in package “SRS” (v.0.2.3), and then calculated by the package “vegan” (v.2.6-4) (Oksanen, 2010) in R (v.4.3.1). A linear regression model was used to test for significant differences between the bays and among the different sampling months. The model was created by the “stats” package (v.4.1.2) (R Core Team, 2018) and followed by additional pairwise comparison of bays on each sampling month using the package “emmeans” (v.1.8.5) (Lenth, 2023) in R. PerMANOVA with 999 permutations was used to test for statistical differences on eukaryotic RNA transcript community comparing both bays, using the “adonis()” function from the package “vegan” (v.2.6-4) (Oksanen, 2010) and the canonical correspondence analysis was also done by package “vegan” (v.2.6-4) (Oksanen, 2010) with “cca()” function. The variance inflation factor (VIF) based on the canonical correspondence analysis from the “vegan” package was used to determine if the measured environmental variables added new information to the differences between the two groups. Permutations ANOVA (n = 999 permutations) was used for testing the statistical significance of each environmental variable. Package “ANCOM-BC2” (v.3.16) (Lin and Peddada, 2020) was used for differential RNA transcript abundance analysis at phylum level between the bays in each sampling month.
Differential RNA transcript expression analysis was performed using the “edgeR” package (v. 3.36.0) in R (Robinson et al., 2010). The two bays (n = 12 per bay) were first modeled as contrast factors to give differentially expressed genes over the year. Then both bays and months were modeled together as contrast factors, with the different sampling sites as replicates (n = 3 per bay per month) to give differentially expressed genes among each month. To ensure the downstream statistical analysis would not be affected by low counts, a cutoff (> 113 counts and at least in three sample libraries) was set to filter out low count transcripts. The choice for this cutoff was based on 10/L where L was the minimum library size (88795 RNA transcripts) and at least for three libraries as each bay-month group contained three replicates (Chen et al., 2016). Then the differential expression analysis relative to fold change threshold was performed by “glmTreat()” function inside the edgeR package. This function is analogous to the TREAT method for microarrays and modifies the statistical test to detect expression changes greater than a specified threshold. The threshold used in this study was log2(1.5) as a standard value (Chen et al., 2016) to remove transcripts with fold changes below this threshold. The Benjamini–Hochberg method was used as p-adjustment type and the p-value setting was 0.05.
Results
RNA sequencing
The RNA sequencing generated a total of 1,437,330,588 reads in 24 sediment core samples (min. 28,035,392 and max. 68,172,798 reads) giving on average 64.83% mRNA reads after rRNA filtering. Assembly of the filtered RNA reads generated 4,214,024 contigs with 55.47% (2,337,566) assigned a taxonomy of which 12.72% (297,242) belonged to the Eukaryota (Supplementary Figure 1 and Supplementary Table 1). A rarefaction curve evaluation was done on the filtered eukaryotic RNA reads (Supplementary Figure 5).
RNA transcript based eukaryotic diversity
A canonical correspondence analysis of RNA transcript-based eukaryote beta diversity (Figure 2B) showed significantly different eukaryotic RNA transcript community compositions between the two bays (PERMANOVA, df = 1, F = 3.85, p = 0.001). Among the physiochemical parameters separating the eukaryotic communities, salinity and water depth were two significant variables from the permutation ANOVA test (Supplementary Tables 3, 4). Salinity and bottom water temperature best fitted the separation between the heated and control bay while water depth, bottom water oxygen concentrations, and other geochemical parameters contributed more to the site variation, especially site D in the heated bay (left top part in Figure 2B).
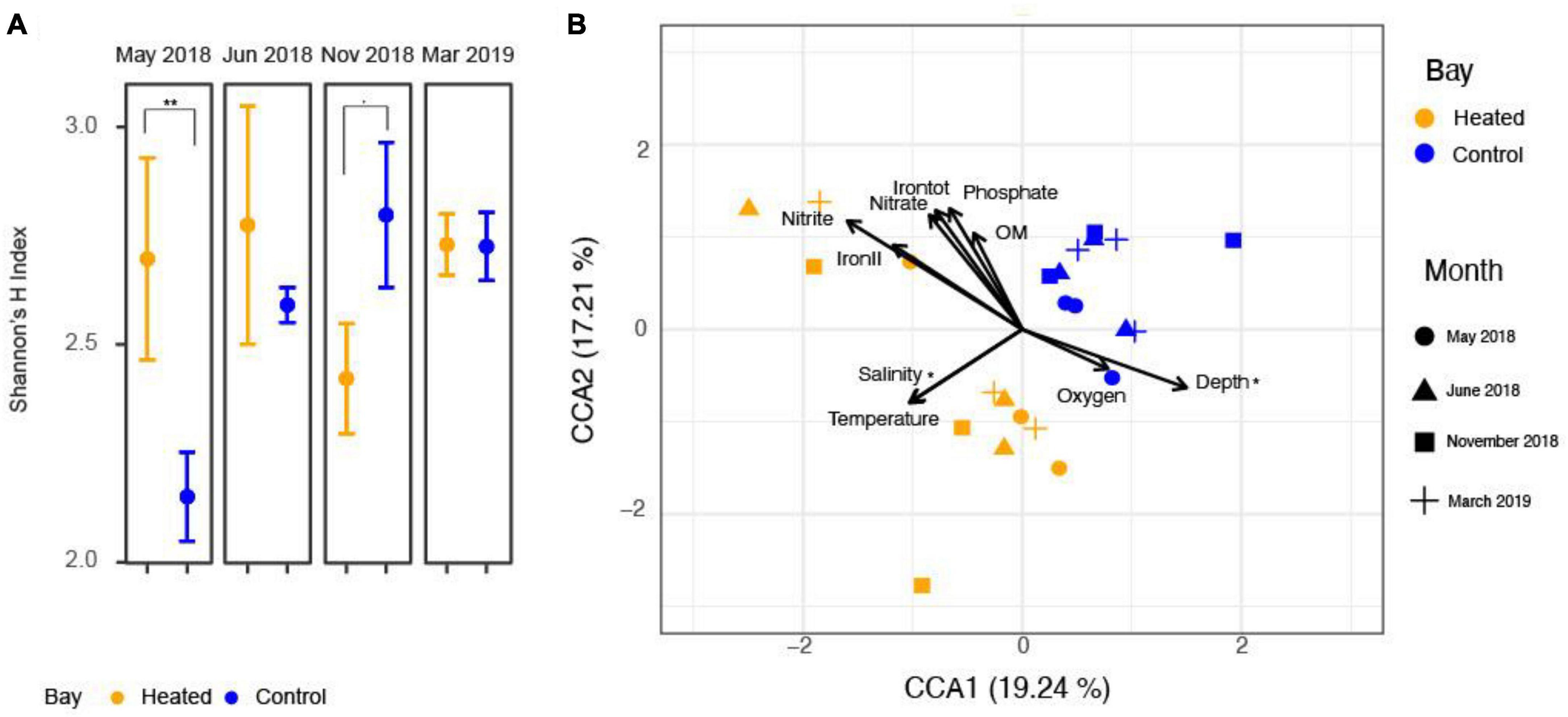
Figure 2. Shannon ìs H index in family level (A) along with canonical correspondence analysis based on mRNA transcripts with relevant physiochemical parameters (B) for the heated (orange) and control (blue) bays for each sampling month. The asterisk denotes statistically significant (p < 0.05), and the dot denotes a p-value between 0.05 and 0.1.
Seasonal patterns of eukaryotic community Shannon’s H index were observed between the two bays at the family level (ANOVA: Bay, df = 1, F = 0.84, p = 0.37; Month, df = 3, F = 1.87, p = 0.18; Bay-month interaction, df = 3, F = 3.84, p = 0.03; Figure 2A). Therefore, single effects were insignificant but there was an interactive effect of month and bay on Shannon’s H index. More specifically in May, the heated bay had a significantly higher Shannon’s H index diversity compared to the control bay (pairwise comparison, 2.80 ± 0.3 and 1.96 ± 0.3, p = 0.01) while the control bay had higher diversity in November (pairwise comparison, 2.96 ± 0.3 and 2.38 ± 0.3, p = 0.08). In June and March, there were no statistical differences between the heated and control bays (pairwise comparison, June: 2.92 ± 0.3 and 2.64 ± 0.2, p = 0.37, March: 2.85 ± 0.3 and 2.85 ± 0.3, p = 0.98). The Shannon’s evenness followed the same pattern as for the H index (Supplementary Figure 2).
Community composition on phylum and family level
The most abundant RNA transcript-based phylum was the diverse and often single-celled Stramenopiles (58% in total; Figure 3) encompassing both photosynthetic and non-photosynthetic members (Dorrell et al., 2017) that consisted of > 50% average relative abundance of all samples in both the heated plus control bays and all sampling months. The dominant family within the Stramenopiles was Bacillariophyta (combined unclassified Bacillariophyta and Bacillariophyta_X, i.e., diatoms), especially in the control bay during spring-summer (May and June). Alveolata was the second most abundant RNA transcript-based phylum (10%). However, the Alveolata displayed a different pattern with a tendency to higher relative abundance in the heated bay, especially during spring-summer (May and June). The dominant family within the Alveolata was the dinoflagellates Dinophyceae (combined unclassified Dinophyceae and Dinophyceae_X). Alveolata was followed in dominance by the Opisthokonta phylum (8%) that lacked a clear pattern between the two bays or among seasons but had a high relative abundance in site F in the heated bay compared to the other sites (especially in November with the Arthropoda family). The dominant families within the Opisthokonta were the aforementioned Arthropoda along with the marine gelatinous animals Ctenophora (Ctenophora unclassified and Ctenophora_X). However, the disparities in the community composition between the bays in each sampling month were small based on differential abundance analysis, and only the phyla Stramenopiles, Opisthokonta, and some rare taxa were statistically different in March (Supplementary Table 2).
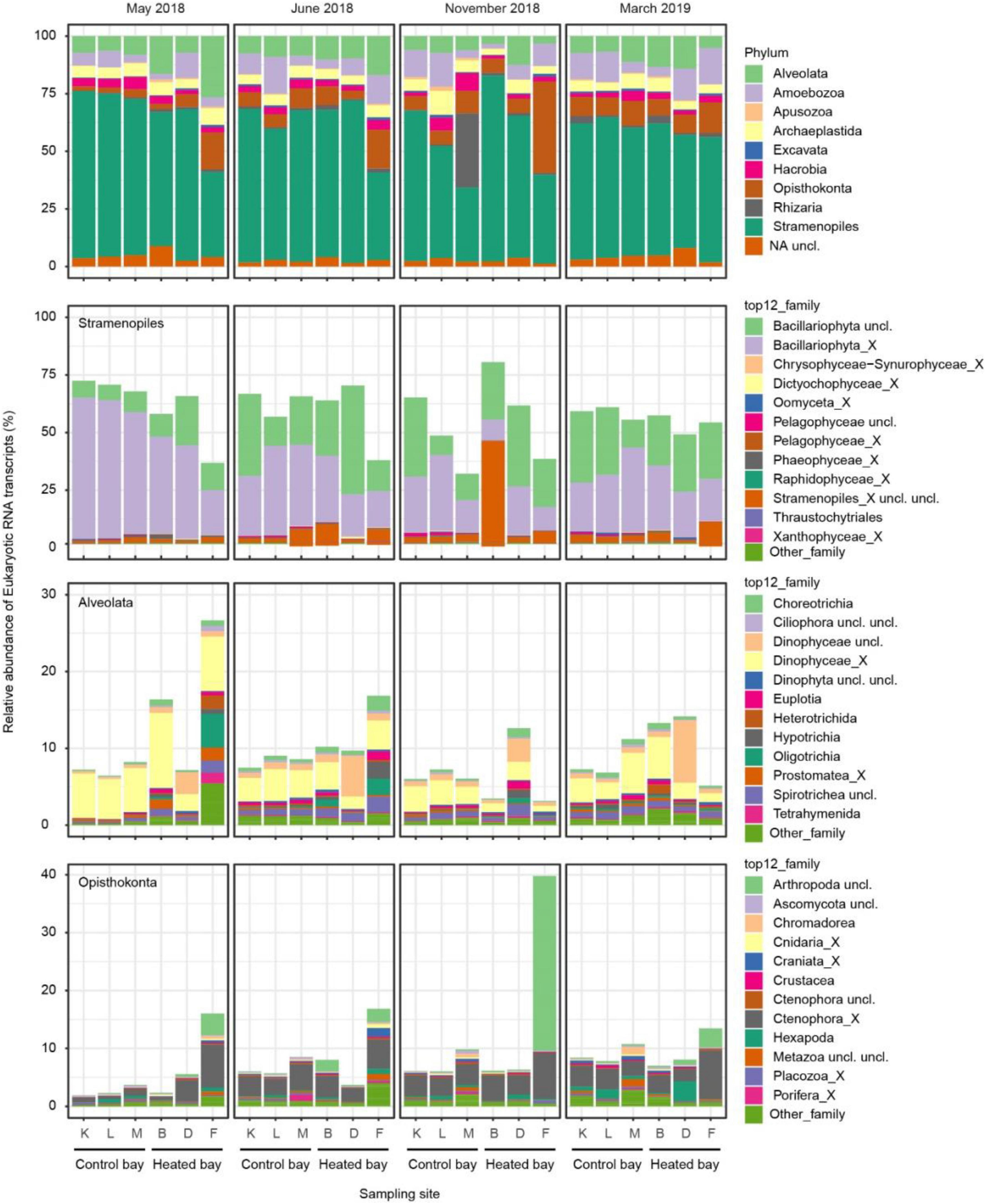
Figure 3. Relative abundance of eukaryotic mRNA transcripts at the level of phylum in the control and heated bays over the four sampling months (top). The Stramenopiles, Alevolata, and Opisthokonta phyla were further split into their respective relative abundance of families.
mRNA transcript based activity
After filtering out genes with insufficient counts for statistical analysis, eukaryotic RNA transcripts encoding 1,319 genes had a differential count between the two bays (lfc = log2 1.5, p < 0.05). In total for all sampling occasions, 147 genes had significantly higher transcript counts in the control bay compared to the heated bay and 213 were higher in the heated bay (Supplementary Figure 3). Disregarding effects of sampling month, common taxa such as Pelagophyceae, Bacillariophyta, and Dinophyta had significantly different RNA transcript counts in both bays that encoded diverse functions in cell metabolism. Many of the taxa displaying differential transcript counts were diatoms, dinoflagellates, and other algae that are typically primary producers containing chlorophyll with a pelagic or benthic lifestyle [e.g., in biofilms or as resting stages with some mRNA to maintain viability (Coyne and Craig Cary, 2005)]. Among the less common taxa, there were some bay-specific genes with differential transcript counts. For example, Spirotrichea (e.g., ciliates), the Ctenophora (gelatinous zooplankton with benthic stage), and Archaeplastida (Mamiellophyceae/Zygnemophyceae/Trebouxiophyceae) only had significantly more transcript counts in the heated bay. In contrast, a few Euglenozoa RNA transcripts were only significantly increased in the control bay.
Month-specific RNA transcript differences
The seasonal dynamics in significantly different RNA transcripts [lfc = log2(1.5), p = 0.05] was studied by considering sampling months separately (Figure 4). There were overall more significant increased transcript numbers in May (n = 87) in the heated bay compared to the control bay (n = 20; Figure 4), which was in line with the patterns in the community diversity (Figure 2A) whereby the alpha diversity was higher in the heated bay at that time point.
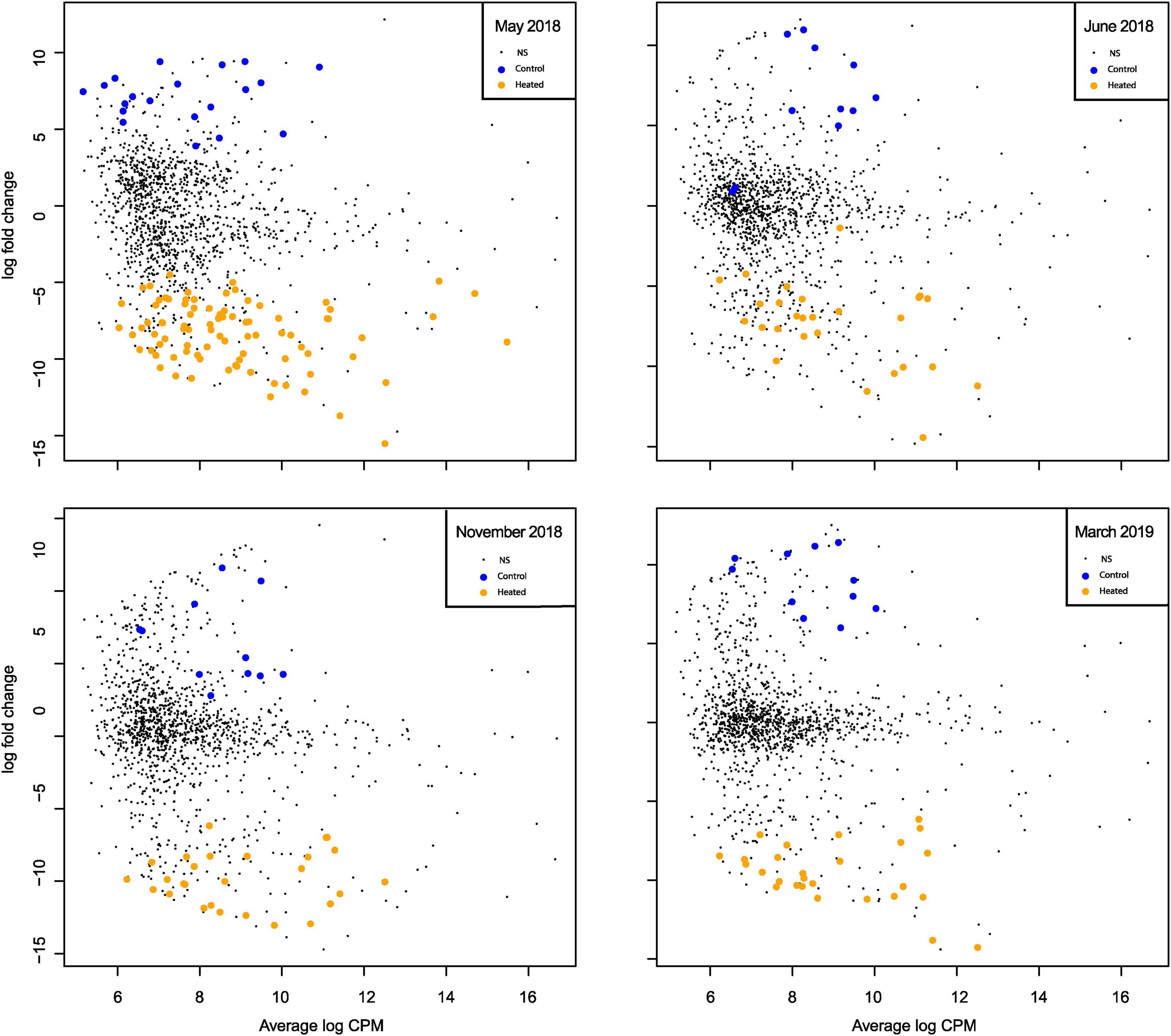
Figure 4. Mean-difference plot of transcript counts per million (CPM) between heated bay and control bay in the separate months. Genes with fold-changes significantly greater than 1.5 (p < 0.05) are highlighted for the heated (orange) and control (blue) bays for each sampling month. NS signifies genes with no significant difference between the two bays.
A greater number of statistically significant Bacillariophyta (diatom) gene transcripts were identified in the heated versus control bay sediments at all sampling times (Figure 5 and Supplementary Figure 4). This trend was stronger with more significant Bacillariophyta transcripts in May and June compared with the March and November sampling times. The RNA transcript signature in the heated bay was highly similar in the November and March samplings with transcripts encoding for the genes COX1-2, CYTB, and petB. These genes indicated growth (Rouzer and Marnett, 2009), metabolism (Shan et al., 1990; Rouzer and Marnett, 2009), or other energy demanding activities in the heated bay during winter whereas only one transcript encoding a heat shock gene [HSP20; (Liu et al., 2012)] had differentially significant RNA transcripts in the control bay for the November and March samples. Transcripts during the May and June sampling occasions were more diverse when comparing the two bays with the genes CPS1, COX1, clpC, and LSS/ERG7 having higher transcript numbers in the heated bay in May compared to the control bay. These related to various functions including maintaining the cell membrane (Ku et al., 1991), nitrogen homeostasis (Yougo et al., 1991), and energy production (Ku et al., 1991; Corey et al., 1994). In contrast, higher transcripts numbers were identified in the control bay for the genes CRYAB, COX2, ALDO, RIT2/RIN, and pps/ppsA that have various functions in e.g., energy production (Rahman et al., 1999) and carbohydrate metabolism (Hutchins et al., 2001; Chiba et al., 2015). Finally, differential Bacillariophyta transcript numbers higher in the heated bay in June included genes related to photosynthesis [psbV, psbC, and psaB (Ikeuchi et al., 1987; Smart and McIntosh, 1991)] and energy production [e.g., ALDO and COX1 (Ku et al., 1991)].
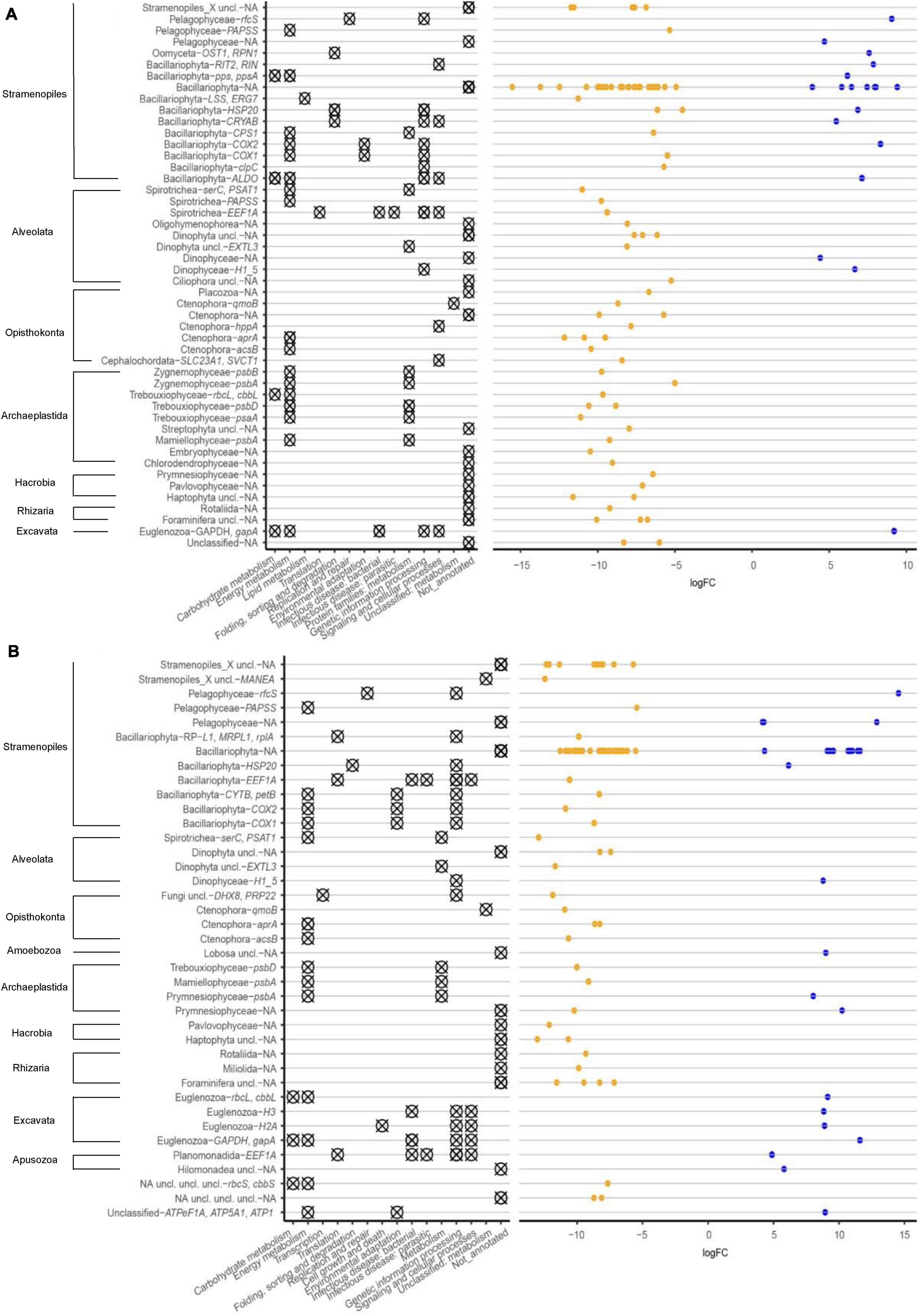
Figure 5. Taxonomy and metabolic functional groups of differential RNA transcript numbers (logFC) for genes between heated bay and control bay in May (A) and November (B) 2018. NA denotes genes that lacked an assigned function.
Genes assigned to the Ctenophora only had increased transcript counts in the heated bay in May, June, and November, which included aprA (Figure 5 and Supplementary Figure 4) and transcripts encoding acsB and qmoB were increased in May and November. Furthermore, RNA transcripts assigned to the Spirotrichea were only increased in the heated bay and included PSAT1 for all sampling points. In May, PAPSS, and EEF1A also had increased transcript counts.
Dinophyceae (Dinoflagellate) transcripts were generally less common without major differences between the bays (Figure 5 and Supplementary Figure 4). However, there were e.g., higher EXTL3 gene transcript counts in the heated bay at all sampling times. In contrast, a gene with significantly increased transcript counts in the control bay during May and November encodes histone H1/5 (H1_5). Pelagophyceae is a subclass of Stramenopiles, commonly called brown algae (Bringloe et al., 2020). Genes with transcripts assigned to the Pelagophyceae had higher transcript counts in both bays including rfcS in the control bay and PAPSS in the heated bay. Finally, transcripts for Euglenozoa genes also had higher counts in the control bay including gapA (glyceraldehyde 3-phosphate dehydrogenase).
Discussion
This study demonstrated that more than 50 years of heating of a coastal marine system has led to changes in the sediment-bound eukaryotic community structure and activity. Overall, the results showed that long-term warming increased the diversity of the eukaryotic community during spring and triggered changes in the seasonal activity patterns of common sediment eukaryotes. The likely main driver for these changes was temperature with on average 5.1°C higher temperatures in the heated compared to the control bay over an annual cycle (Seidel et al., 2022b). The canonical correspondence analysis separated the eukaryotic communities between the heated and control bay along the salinity differences among sampling sites. Although temperature was not significant, the direction of the temperature and salinity arrows were in the same direction. Other factors such as oxygen concentration is lowered in higher temperatures (Brewer and Peltzer, 2017) such that a lack of oxygen likely limited nitrification in the sediment (Liikanen et al., 2002). This resulted in less nitrite and nitrate, potentially explaining a portion of the observed among-site variation. This study complements and adds a layer of generality to previous studies suggesting significant effects of climate change on the more intensively studied prokaryotic communities (Seidel et al., 2022a,b, 2023).
A highly diversified eukaryote community can act as a reservoir of rare and dormant taxa, which provides ecosystems with a biological buffering capacity to handle climate change and cope with extreme weather events such as marine heat waves (Capo et al., 2016). The Shannon H index was significantly higher in the heated bay in May (Figure 2A). This was potentially explained by an earlier onset of summer conditions in the heated bay, leading to a more diverse active community in the warmer waters in the heated bay compared to the control bay (Salonen et al., 2019). The alpha diversity also varied in November with significantly higher family level alpha diversities in the control bay (Figure 2A). However, the number of statistically increased RNA transcript numbers was still higher in the heated bay (Figures 4, 5). These two opposing patterns may suggest the control bay entered cold winter conditions earlier, which might have caused the community to have a low number of transcripts from a wide range of taxa. While in the heated bay, there were still certain taxa that were more active and dominant while others had a low number of transcripts. In June and March, the active sediment eukaryotic communities in both heated bay and control bay were in summer and spring-bloom conditions. These conditions tended to provide settings for a more similar in alpha diversity. Recent climate change has already altered the marine eukaryotic diversity globally, e.g., in temperate zones and high latitude areas (Gao et al., 2018; Han et al., 2022) and the impacts of climate change can either work directly on the eukaryote’s life-cycle, or indirectly through food web interactions (Przytulska et al., 2015). Eukaryotic diversity in the marine ecosystem generally declines toward the poles, which is suggested to be primarily driven by decreasing of ocean temperatures (Ibarbalz et al., 2019). It is unknown how climate change will affect the sediment-bound eukaryotic diversity, but this study suggested that climate change will alter the community composition, but the magnitude of those changes can vary among different seasons. Other studies have also shown that temporal changes like year and season affect the diversity of sediment eukaryotes (Guardiola et al., 2016; Salonen et al., 2019; Broman et al., 2020; Lalzar et al., 2023). The community composition of sediment eukaryotes also changes in response to annual and seasonal abiotic fluctuations as well as to clines and gradients in environmental variables such as turbidity, nutrients, and human activities (Broman et al., 2015; Salonen et al., 2019; Vause et al., 2019). In all, the data suggested that large scale climate change induced shifts in seasonality that will have major effects on eukaryotic sediment organisms and the overall ecosystem structure and functioning.
Most previous marine sediment bound eukaryote research focuses on spatial variability in community composition (Salonen et al., 2019; Wang et al., 2022; Lalzar et al., 2023) or the temporal variability in the same area (Salonen et al., 2019). This study compared seasonal variability in community composition between two bays in close spatial distance, but with significant differences in water temperatures. This enabled inference of how environmental variation, such as climate change, affects community composition and transcriptomic activity without a significant spatial variance. Furthermore, while most previous studies use 18S rRNA gene data to determine eukaryotic community compositions, this study added the aspect of studying the transcript-based active members. The sediment-bound eukaryote community compositions in this study were dominated by the functionally important Bacillariophyta (diatoms) in both bays, followed by Dinophyceae (dinoflagellates), Ctenophores, and other less abundant taxa. Within these major groups there were both facultative sediment dwellers as well as those organisms that only spend part of their life cycle in the sediment, e.g., as resting stages (Fryxell, 1983; Lewis et al., 1999; McQuoid et al., 2002; Ellegaard and Ribeiro, 2018). This is in line with other studies demonstrating that eukaryotes in sediments have diverse communities with different dominance patterns, e.g., diatoms, maxillopods, or dinoflagellates (Salonen et al., 2019; Iburg et al., 2021; Lalzar et al., 2023). The largest phyla and family level community differences between the bays were present in spring and summer with a tendency toward more diatoms in the control bay (∼75% relative abundance), whereas dinoflagellates contributed more to the relative abundance in the heated bay, albeit not significantly. In contrast, during winter the community compositions between the two bays were more similar. Dinoflagellates are suggested to be favored (Vehmaa et al., 2011) while diatoms have a tendency to decrease (Wasmund et al., 1998) with mild winters. This shift in the relative abundances of diatoms and dinoflagellates is projected to lead to differences in the production of the system (Wei et al., 2004), which might result in lower benthic production and higher pelagic secondary production through energy transfer in the food web (Hjerne et al., 2019). Likewise, differences in the sediment-bound eukaryotic communities in terms of resting stages could lead to an altered benthic-pelagic coupling in coastal systems.
The differential RNA transcript counts showed a similar trend as the alpha diversity, with more increased transcripts in May and November compared to March and June samplings. The most common taxa among those differentially expressed transcripts were the functionally important Bacillariophyta (diatoms) and Dinophyceae (dinoflagellates) that are commonly found in sediments (Broman et al., 2017; Salonen et al., 2019). Bacillariophyta (diatoms) play a vital role in the marine ecosystem (Nelson et al., 1995) and tend to dominate phytoplankton communities in well-mixed coastal areas, where they can access sufficient light and nutrient resources (Morel and Price, 2003). The Bacillariophyta usually bloom in open waters during spring and then sink to the sediment in response to nutrient depletion, but there are also diatoms that solely live on surfaces and in the sediment (Smetacek, 1985; Vyverman, 1992; Dela-Cruz et al., 2006). A previous study found that diatoms dominate a Baltic Sea sediment transcriptome (Broman et al., 2017) with RNA reads associated with diatoms being linked to the thylakoid membrane in the chloroplast and photosynthesis. Furthermore, a low abundance of genes coding for the Calvin-Benson-Bassam cycle (to synthesize Rubisco for photosynthesis) is suggested to indicate that many of these diatoms were in resting stages (Thureborn et al., 2016; Broman et al., 2017). Differential RNA transcripts assigned to diatoms during winter in the heated bay were associated with energy production and protein synthesis, which may indicate an increased metabolism in the heated bay sediment-bound community during winter. In general, the results showed a pattern of early diatom activity in the heated bay suggesting future climate change might decrease the dominance and activity of diatoms in later spring development.
Dinoflagellates were another common component in the sediment eukaryotic community, but only a few genes had differential RNA transcripts that were predominantly in the heated bay. Likewise, the Ctenophores, Spirotrichea, Mamiellophyceae, Zygnemophyceae, and Trebouxiophyceae only had differential transcript numbers in the heated compared to the control bay. These transcripts were associated with various genes and commonly occurring categories included “regulating energy metabolism” and “protein families: metabolism,” which might indicate that the sediment bound eukaryotic community in the heated bay had a more active metabolism throughout the year. The Ctenophores are functionally important gelatinous zooplankton in marine food webs that predate other zooplankton and fish eggs (Gamble, 1994; Mills, 1995; Purcell, 1997). In agreement with this study, they are often abundant during summer phytoplankton blooms and are predicted to be favored by climate change (Mills, 2001; Javidpour et al., 2009). Spirotrichea is a diverse subgroup of the class Ciliophora (commonly termed ciliates) (Gao et al., 2016) that are important components of marine food webs, acting as the food resource for large zooplankton and predators of bacteria and phytoplankton (Weisse and Sonntag, 2016). Other taxa with differential transcripts in the heated bay were Mamiellophyceae, Zygnemophyceae, and Trebouxiophyceae that are phytoplanktonic green algae (Gontcharov et al., 2003; Leliaert et al., 2012). Transcripts from those taxa were mostly involved in photosynthesis and significantly increased in the heated bay especially in May, suggesting the photosynthesizing algal community activities were different between the bays, or due to differences in cell numbers. All these taxa had only differential transcript numbers in the heated bay and their diverse functions suggested that future climate change and warming can trigger more diverse community composition involved in various metabolism pathways.
In summary, this study demonstrated that long-term (> 50 years) heating of coastal waters (average 5°C, i.e., comparable to the expected temperature increase for the Baltic Sea by 2100) altered the community composition, seasonal dynamics, and the transcriptome of sediment-bound eukaryotes. Other studies suggest that sediment eukaryotes show promise to become a tool for environmental monitoring of coastal systems (Gielings et al., 2021). Therefore, the results from this study can help predict the influence of future global warming in sediment communities and their overlaying pelagic systems, with this study suggesting a generally more active metabolism during winter. However, the methodology for characterizing sediment eukaryotes is still under intense development (Reñé et al., 2020), and future work can be extended both spatially and temporally to gain a more comprehensive view of the sediment bound communities.
Data availability statement
The RNA transcript raw reads are available on the JGI Integrated Microbial Genomes and Microbiomes (IMG) database with the following references JGI proposal ID 503869. The R code used for analysis is available at GitHub: https://github.com/lsjmouse/eukaryotes_manuscript_R_analysis.
Author contributions
SL: Formal analysis, Methodology, Visualization, Writing – original draft, Writing – review & editing. EN: Formal analysis, Methodology, Software, Supervision, Visualization, Writing – original draft, Writing – review & editing. LS: Data curation, Methodology, Supervision, Writing – original draft, Writing – review & editing. MK: Funding acquisition, Supervision, Writing – original draft, Writing – review & editing. AF: Funding acquisition, Supervision, Writing – original draft, Writing – review & editing. MD: Funding acquisition, Methodology, Supervision, Writing – original draft, Writing – review & editing. SH: Funding acquisition, Methodology, Supervision, Writing – original draft, Writing – review & editing.
Funding
The authors declare financial support was received for the research, authorship, and/or publication of this article. The authors thank the Swedish Research Council for Sustainable Development, Formas (contract FR-2020/0008) to MD; the Swedish Research Council, Vetenskaprådet (contract 2020-03519) and the Magnus Bergvalls Stiftelse (Grant No. 2019-03116) to AF; The Crafoord Foundation (Grant No. 20170539) to SH; and the Swedish Research Council for Sustainable Development, Formas (contract 2022-01016_3) to MK. The computations were enabled by resources (projects NAISS 2023/22-893 and 2023/6-261) provided by the Swedish National Infrastructure for Computing (SNIC) at UPPMAX at Uppsala University, partially funded by the Swedish Research Council through grant agreement No. 2018-05973. A portion of this research was performed under the Facilities Integrating Collaborations for User Science (FICUS) initiative and used resources at the DOE Joint Genome Institute and the Environmental Molecular Sciences Laboratory, which are DOE Office of Science User Facilities. Both facilities are sponsored by the Office of Biological and Environmental Research and operated under Contract Nos. DE-AC02-05CH11231 (JGI) and DE-AC05-76RL01830 (EMSL) via a CSP FY18 Q3 New Investigator Proposal (CSP 503869) to SH. Open access funding provided by Linnaeus University.
Acknowledgments
The authors wish to thank OKG AB (Oskarshamns Nuclear Energy Company) for permission to work at the heated bay and surrounding areas.
Conflict of interest
The authors declare that the research was conducted in the absence of any commercial or financial relationships that could be construed as a potential conflict of interest.
Publisher’s note
All claims expressed in this article are solely those of the authors and do not necessarily represent those of their affiliated organizations, or those of the publisher, the editors and the reviewers. Any product that may be evaluated in this article, or claim that may be made by its manufacturer, is not guaranteed or endorsed by the publisher.
Supplementary material
The Supplementary material for this article can be found online at: https://www.frontiersin.org/articles/10.3389/fmicb.2024.1369102/full#supplementary-material
References
Abraham, J. P., Baringer, M., Bindoff, N. L., Boyer, T., Cheng, L., Church, J. A., et al. (2013). A review of global ocean temperature observations: Implications for ocean heat content estimates and climate change. Rev. Geophys. 51, 450–483. doi: 10.1146/annurev-marine-122414-034040
Alabia, I. D., Molinos, J. G., Saitoh, S.-I., Hirata, T., Hirawake, T., and Mueter, F. J. (2020). Multiple facets of marine biodiversity in the Pacific Arctic under future climate. Sci. Total Environ. 744:140913. doi: 10.1016/j.scitotenv.2020.140913
Allen, A. E. (2020). PhyloDB v.1.075. Available online at: https://allenlab.ucsd.edu/data/
Andersson, A., Meier, H. M., Ripszam, M., Rowe, O., Wikner, J., Haglund, P., et al. (2015). Projected future climate change and Baltic Sea ecosystem management. Ambio 44, 345–356.
Bik, H. M., Sung, W., De Ley, P., Baldwin, J. G., Sharma, J., Rocha-Olivares, A., et al. (2012). Metagenetic community analysis of microbial eukaryotes illuminates biogeographic patterns in deep-sea and shallow water sediments. Mol. Ecol. 21, 1048–1059. doi: 10.1111/j.1365-294X.2011.05297.x
Borges, A. V., Champenois, W., Gypens, N., Delille, B., and Harlay, J. (2016). Massive marine methane emissions from near-shore shallow coastal areas. Sci. Rep. 6:27908. doi: 10.1038/srep27908
Breitburg, D., Levin, L. A., Oschlies, A., Grégoire, M., Chavez, F. P., Conley, D. J., et al. (2018). Declining oxygen in the global ocean and coastal waters. Science 359:eaam7240.
Brendonck, L., and De Meester, L. (2003). Egg banks in freshwater zooplankton: Evolutionary and ecological archives in the sediment. Hydrobiologia 491, 65–84.
Brewer, P. G., and Peltzer, E. T. (2017). Depth perception: The need to report ocean biogeochemical rates as functions of temperature, not depth. Philos. Trans. R. Soc. A Math. Phys. Eng. Sci. 375:20160319. doi: 10.1098/rsta.2016.0319
Bringloe, T. T., Starko, S., Wade, R. M., Vieira, C., Kawai, H., De Clerck, O., et al. (2020). Phylogeny and evolution of the brown algae. Crit. Rev. Plant Sci. 39, 281–321.
Broman, E., Bonaglia, S., Holovachov, O., Marzocchi, U., Hall, P. O., and Nascimento, F. J. (2020). Uncovering diversity and metabolic spectrum of animals in dead zone sediments. Commun. Biol. 3:106. doi: 10.1038/s42003-020-0822-7
Broman, E., Brüsin, M., Dopson, M., and Hylander, S. (2015). Oxygenation of anoxic sediments triggers hatching of zooplankton eggs. Proc. R. Soc. B Biol. Sci. 282:20152025. doi: 10.1098/rspb.2015.2025
Broman, E., Raymond, C., Sommer, C., Gunnarsson, J. S., Creer, S., and Nascimento, F. J. (2019b). Salinity drives meiofaunal community structure dynamics across the Baltic ecosystem. Mol. Ecol. 28, 3813–3829. doi: 10.1111/mec.15179
Broman, E., Li, L., Fridlund, J., Svensson, F., Legrand, C., and Dopson, M. (2019a). Spring and late summer phytoplankton biomass impact on the coastal sediment microbial community structure. Microb. Ecol. 77, 288–303. doi: 10.1007/s00248-018-1229-6
Broman, E., Sachpazidou, V., Dopson, M., and Hylander, S. (2017). Diatoms dominate the eukaryotic metatranscriptome during spring in coastal ‘dead zone’ sediments. Proc. R. Soc. B Biol. Sci. 284:20171617. doi: 10.1098/rspb.2017.1617
Bushnell, B. (2023). BBMap. Available online at: https://sourceforge.net/projects/bbmap/ (accessed October 15, 2023).
Canuel, E. A., Cammer, S. S., Mcintosh, H. A., and Pondell, C. R. (2012). Climate change impacts on the organic carbon cycle at the land-ocean interface. Annu. Rev. Earth Planet. Sci. 40, 685–711.
Capo, E., Debroas, D., Arnaud, F., Guillemot, T., Bichet, V., Millet, L., et al. (2016). Long-term dynamics in microbial eukaryotes communities: A palaeolimnological view based on sedimentary DNA. Mol. Ecol. 25, 5925–5943. doi: 10.1111/mec.13893
Carstensen, J., Andersen, J. H., Gustafsson, B. G., and Conley, D. J. (2014). Deoxygenation of the Baltic Sea during the last century. Proc. Natl. Acad. Sci. U.S.A. 111, 5628–5633. doi: 10.1073/pnas.1323156111
Chen, Y., Lun, A. T., and Smyth, G. K. (2016). From reads to genes to pathways: Differential expression analysis of RNA-Seq experiments using Rsubread and the edgeR quasi-likelihood pipeline. F1000Res 5:1438. doi: 10.12688/f1000research.8987.2
Chiba, Y., Kamikawa, R., Nakada-Tsukui, K., Saito-Nakano, Y., and Nozaki, T. (2015). Discovery of PPi-type phosphoenolpyruvate carboxykinase genes in eukaryotes and bacteria. J. Biol. Chem. 290, 23960–23970. doi: 10.1074/jbc.M115.672907
Connell, S. D., Kroeker, K. J., Fabricius, K. E., Kline, D. I., and Russell, B. D. (2013). The other ocean acidification problem: CO2 as a resource among competitors for ecosystem dominance. Philos. Trans. R. Soc. B Biol. Sci. 368:20120442. doi: 10.1098/rstb.2012.0442
Corey, E., Matsuda, S., and Bartel, B. (1994). Molecular cloning, characterization, and overexpression of ERG7, the Saccharomyces cerevisiae gene encoding lanosterol synthase. Proc. Natl. Acad. Sci. U.S.A. 91, 2211–2215. doi: 10.1073/pnas.91.6.2211
Coyne, K. J., and Craig Cary, S. (2005). Molecular approaches to the investigation of viable dinoflagellate cysts in natural sediments from estuarine environments. J. Eukaryot. Microbiol. 52, 90–94. doi: 10.1111/j.1550-7408.2005.05202001.x
Dela-Cruz, J., Pritchard, T., Gordon, G., and Ajani, P. (2006). The use of periphytic diatoms as a means of assessing impacts of point source inorganic nutrient pollution in south-eastern Australia. Freshw. Biol. 51, 951–972.
Dorrell, R. G., Gile, G., Mccallum, G., Méheust, R., Bapteste, E. P., Klinger, C. M., et al. (2017). Chimeric origins of ochrophytes and haptophytes revealed through an ancient plastid proteome. Elife 6:e23717. doi: 10.7554/eLife.23717
Ellegaard, M., and Ribeiro, S. (2018). The long-term persistence of phytoplankton resting stages in aquatic ‘seed banks’. Biol. Rev. 93, 166–183. doi: 10.1111/brv.12338
Fanning, K. A., Carder, K. L., and Betzer, P. R. (1982). Sediment resuspension by coastal waters: A potential mechanism for nutrient re-cycling on the ocean’s margins. Deep Sea Res. A Oceanogr. Res. Papers 29, 953–965.
Gamble, J. (1994). Predation mortality of bay anchovy Anchoa mitchilli eggs and larvae due to scyphomedusae and ctenophores in Chesapeake Bay. Mar. Ecol. Progr. Ser. 114, 47–58.
Gao, F., Warren, A., Zhang, Q., Gong, J., Miao, M., Sun, P., et al. (2016). The all-data-based evolutionary hypothesis of ciliated protists with a revised classification of the phylum Ciliophora (Eukaryota, Alveolata). Sci. Rep. 6:24874. doi: 10.1038/srep24874
Gao, X., Lin, L., Yu, Y., and Zeng, Y. (2018). Microbial eukaryotic diversity with emphasis on picoprasinophytes under the sea ice of the central Arctic Ocean in summer. Curr. Sci. 115, 1709–1713.
Garcia-Soto, C., Cheng, L., Caesar, L., Schmidtko, S., Jewett, E. B., Cheripka, A., et al. (2021). An overview of ocean climate change indicators: Sea surface temperature, ocean heat content, ocean pH, dissolved oxygen concentration, arctic sea ice extent, thickness and volume, sea level and strength of the AMOC (Atlantic meridional overturning circulation). Front. Mar. Sci. 8:642372. doi: 10.3389/fmars.2021.642372
Gielings, R., Fais, M., Fontaneto, D., Creer, S., Costa, F. O., Renema, W., et al. (2021). DNA metabarcoding methods for the study of marine benthic meiofauna: A review. Front. Mar. Sci. 8:730063. doi: 10.3389/fmars.2021.730063
Gontcharov, A. A., Marin, B., and Melkonian, M. (2003). Molecular phylogeny of conjugating green algae (Zygnemophyceae, Streptophyta) inferred from SSU rDNA sequence comparisons. J. Mol. Evol. 56, 89–104. doi: 10.1007/s00239-002-2383-4
Guardiola, M., Wangensteen, O. S., Taberlet, P., Coissac, E., Uriz, M. J., and Turon, X. (2016). Spatio-temporal monitoring of deep-sea communities using metabarcoding of sediment DNA and RNA. PeerJ 4:e2807. doi: 10.7717/peerj.2807
Haas, B. (2023). TransDecoder. Available online at: https://github.com/TransDecoder/TransDecoder (accessed October 15, 2023).
Han, D., Shin, H., Lee, J.-H., Kang, C.-K., Kim, D.-G., and Hur, H.-G. (2022). Phylogenetic diversity and spatiotemporal dynamics of bacterial and microeukaryotic plankton communities in Gwangyang Bay of the Korean Peninsula. Sci. Rep. 12:2980. doi: 10.1038/s41598-022-06624-7
Hjerne, O., Hajdu, S., Larsson, U., Downing, A. S., and Winder, M. (2019). Climate driven changes in timing, composition and magnitude of the Baltic Sea phytoplankton spring bloom. Front. Mar. Sci. 6:482. doi: 10.3389/fmars.2019.00482
Holon, F., Marre, G., Parravicini, V., Mouquet, N., Bockel, T., Descamp, P., et al. (2018). A predictive model based on multiple coastal anthropogenic pressures explains the degradation status of a marine ecosystem: Implications for management and conservation. Biol. Conserv. 222, 125–135.
Hutchins, A. M., Holden, J. F., and Adams, M. W. (2001). Phosphoenolpyruvate synthetase from the hyperthermophilic archaeon Pyrococcus furiosus. J. Bacteriol. 183, 709–715. doi: 10.1128/JB.183.2.709-715.2001
Ibarbalz, F. M., Henry, N., Brandão, M. C., Martini, S., Busseni, G., Byrne, H., et al. (2019). Global trends in marine plankton diversity across kingdoms of life. Cell 179, 1084–1097.
Iburg, S., Izabel-Shen, D., Austin, ÅN., Hansen, J. P., Eklöf, J. S., and Nascimento, F. J. (2021). Effects of recreational boating on microbial and meiofauna diversity in coastal shallow ecosystems of the Baltic Sea. mSphere 6:e0012721. doi: 10.1128/mSphere.00127-21
Ikeuchi, M., Plumley, F. G., Inoue, Y., and Schmidt, G. W. (1987). Phosphorylation of photosystem II components, CP43 apoprotein, D1, D2, and 10 to 11 kilodalton protein in chloroplast thylakoids of higher plants. Plant Physiol. 85, 638–642. doi: 10.1104/pp.85.3.638
IPCC, Rajendra, K. P., and Andy, R. (2008). IPCC, 2007: Climate change 2007: Synthesis report. Geneva: IPCC.
Javidpour, J., Molinero, J. C., Peschutter, J., and Sommer, U. (2009). Seasonal changes and population dynamics of the ctenophore Mnemiopsis leidyi after its first year of invasion in the Kiel Fjord, Western Baltic Sea. Biol. Invas. 11, 873–882.
Kanehisa, M., Sato, Y., and Morishima, K. (2016). BlastKOALA and GhostKOALA: KEGG tools for functional characterization of genome and metagenome sequences. J. Mol. Biol. 428, 726–731. doi: 10.1016/j.jmb.2015.11.006
Knuuttila, S., Svendsen, L., Staaf, H., Kotilainen, P., Boutrup, S., Pyhala, M., et al. (2011). Fifth Baltic Sea pollution load compilation (PLC-5). Washington, DC: Helsinki Commission.
Krinos, A., Hu, S., Cohen, N., and Alexander, H. (2023). EUKulele. Available online at: https://github.com/AlexanderLabWHOI/EUKulele (accessed October 15, 2023).
Ku, C., Lu, Q., Ussuf, K., Weinstock, G., and Sanborn, B. (1991). Hormonal regulation of cytochrome oxidase subunit messenger RNAs in rat sertoli cells. Mol. Endocrinol. 5, 1669–1676. doi: 10.1210/mend-5-11-1669
Lalzar, M., Zvi-Kedem, T., Kroin, Y., Martinez, S., Tchernov, D., and Meron, D. (2023). Sediment microbiota as a proxy of environmental health: Discovering inter-and intrakingdom dynamics along the eastern mediterranean continental shelf. Microbiol. Spectr. 11:e0224222. doi: 10.1128/spectrum.02242-22
Langmead, B., and Salzberg, S. L. (2012). Fast gapped-read alignment with Bowtie 2. Nat. Methods 9, 357–359. doi: 10.1038/nmeth.1923
Leliaert, F., Smith, D. R., Moreau, H., Herron, M. D., Verbruggen, H., Delwiche, C. F., et al. (2012). Phylogeny and molecular evolution of the green algae. Crit. Rev. Plant Sci. 31, 1–46.
Lenth, R. V. (2023). R package emmeans. Available online at: https://github.com/rvlenth/emmeans (accessed October 15, 2023).
Levin, L. A., Boesch, D. F., Covich, A., Dahm, C., Erséus, C., Ewel, K. C., et al. (2001). The function of marine critical transition zones and the importance of sediment biodiversity. Ecosystems 4, 430–451.
Lewis, J., Harris, A., Jones, K., and Edmonds, R. (1999). Long-term survival of marine planktonic diatoms and dinoflagellates in stored sediment samples. J. Plankton Res. 21, 343–354.
Li, D., Liu, C.-M., Luo, R., Sadakane, K., and Lam, T.-W. (2015). MEGAHIT: An ultra-fast single-node solution for large and complex metagenomics assembly via succinct de Bruijn graph. Bioinformatics 31, 1674–1676. doi: 10.1093/bioinformatics/btv033
Liao, Y., Smyth, G. K., and Shi, W. (2014). featureCounts: An efficient general purpose program for assigning sequence reads to genomic features. Bioinformatics 30, 923–930. doi: 10.1093/bioinformatics/btt656
Liikanen, A., Murtoniemi, T., Tanskanen, H., Väisänen, T., and Martikainen, P. J. (2002). Effects of temperature and oxygenavailability on greenhouse gas and nutrient dynamics in sediment of a eutrophic mid-boreal lake. Biogeochemistry 59, 269–286.
Lin, H., and Peddada, S. D. (2020). Analysis of compositions of microbiomes with bias correction. Nat. Commun. 11:3514.
Liu, I.-C., Chiu, S.-W., Lee, H.-Y., and Leu, J.-Y. (2012). The histone deacetylase Hos2 forms an Hsp42-dependent cytoplasmic granule in quiescent yeast cells. Mol. Biol. Cell 23, 1231–1242. doi: 10.1091/mbc.E11-09-0752
Malhi, Y., Franklin, J., Seddon, N., Solan, M., Turner, M. G., Field, C. B., et al. (2020). Climate change and ecosystems: Threats, opportunities and solutions. Philos. Trans. R. Soc. B Biol. Sci. 375:20190104.
Marcus, N. H., and Boero, F. (1998). Minireview: The importance of benthic-pelagic coupling and the forgotten role of life cycles in coastal aquatic systems. Limnol. Oceanogr. 43, 763–768.
Mason, R. J., Rice, S., Johnson, M. F., Wood, P., and Vettori, D. (2022). Aquatic insect bioconstructions modify fine-sediment entrainment and mobility. J. Geophys. Res. Earth Surf. 127:e2021JF006399.
Massana, R., Balagué, V., Guillou, L., and Pedros-Alio, C. (2004). Picoeukaryotic diversity in an oligotrophic coastal site studied by molecular and culturing approaches. FEMS Microbiol. Ecol. 50, 231–243. doi: 10.1016/j.femsec.2004.07.001
McQuoid, M. R., Godhe, A., and Nordberg, K. (2002). Viability of phytoplankton resting stages in the sediments of a coastal Swedish fjord. Eur. J. Phycol. 37, 191–201.
Mills, C. E. (1995). Medusae, siphonophores, and ctenophores as planktivorous predators in changing global ecosystems. ICES J. Mar. Sci. 52, 575–581.
Mills, C. E. (2001). Jellyfish blooms: Are populations increasing globally in response to changing ocean conditions? Hydrobiologia 451, 55–68.
Morel, F. M., and Price, N. M. (2003). The biogeochemical cycles of trace metals in the oceans. Science 300, 944–947.
Nagarkar, M., Countway, P. D., Du Yoo, Y., Daniels, E., Poulton, N. J., and Palenik, B. (2018). Temporal dynamics of eukaryotic microbial diversity at a coastal Pacific site. ISME J. 12, 2278–2291. doi: 10.1038/s41396-018-0172-3
Nelson, D. M., Tréguer, P., Brzezinski, M. A., Leynaert, A., and Quéguiner, B. (1995). Production and dissolution of biogenic silica in the ocean: Revised global estimates, comparison with regional data and relationship to biogenic sedimentation. Glob. Biogeochem. Cycles 9, 359–372.
Oksanen, J. (2010). Vegan: Community ecology package. Available online at: http://vegan.r-forge.r-project.org/ (accessed March 2, 2024).
Pan, J., Marcoval, M. A., Bazzini, S. M., Vallina, M. V., and Marco, S. (2013). “Coastal marine biodiversity: Challenges and threats,” in Marine ecology in a changing world, eds A. H. Arias and M. C. Menendez (Boca Raton, FL: CRC Press), 43–67.
Przytulska, A., Bartosiewicz, M., Rautio, M., Dufresne, F., and Vincent, W. F. (2015). Climate effects on high latitude Daphnia via food quality and thresholds. PLoS One 10:e0126231. doi: 10.1371/journal.pone.0126231
Purcell, J. E. (1997). Pelagic cnidarians and ctenophores as predators: Selective predation, feeding rates, and effects on prey populations. Ann. Inst. Océanogr. 73, 739–775.
R Core Team (2018). R: A language and environment for statistical computing. 2014. Vienna: R Foundation for Statistical Computing.
Rahman, S., Taanman, J.-W., Cooper, J. M., Nelson, I., Hargreaves, I., Meunier, B., et al. (1999). A missense mutation of cytochrome oxidase subunit II causes defective assembly and myopathy. Am. J. Hum. Genet. 65, 1030–1039.
Reñé, A., Auladell, A., Reboul, G., Moreira, D., and López-García, P. (2020). Performance of the melting seawater-ice elution method on the metabarcoding characterization of benthic protist communities. Environ. Microbiol. Rep. 12, 314–323. doi: 10.1111/1758-2229.12834
Reusch, T. B., Dierking, J., Andersson, H. C., Bonsdorff, E., Carstensen, J., Casini, M., et al. (2018). The Baltic Sea as a time machine for the future coastal ocean. Sci. Adv. 4:eaar8195. doi: 10.1126/sciadv.aar8195
Robinson, M. D., Mccarthy, D. J., and Smyth, G. K. (2010). edgeR: A Bioconductor package for differential expression analysis of digital gene expression data. Bioinformatics 26, 139–140.
Rouzer, C. A., and Marnett, L. J. (2009). Cyclooxygenases: Structural and functional insights. J. Lipid Res. 50, S29–S34.
Salonen, I., Chronopoulou, P., Leskinen, E., and Koho, K. (2019). Metabarcoding successfully tracks temporal changes in eukaryotic communities in coastal sediments. FEMS Microbiol. Ecol. 95:fiy226. doi: 10.1093/femsec/fiy226
Schmidtko, S., Stramma, L., and Visbeck, M. (2017). Decline in global oceanic oxygen content during the past five decades. Nature 542, 335–339. doi: 10.1038/nature21399
Seidel, L., Broman, E., Nilsson, E., Ståhle, M., Ketzer, M., Pérez-Martínez, C., et al. (2023). Climate change-related warming reduces thermal sensitivity and modifies metabolic activity of coastal benthic bacterial communities. ISME J. 17, 855–869. doi: 10.1038/s41396-023-01395-z
Seidel, L., Broman, E., Ståhle, M., Nilsson, E., Turner, S., Hendrycks, W., et al. (2022a). Long-term warming of Baltic Sea coastal waters affects bacterial communities in bottom water and sediments differently. Front. Microbiol. 13:873281. doi: 10.3389/fmicb.2022.873281
Seidel, L., Ketzer, M., Broman, E., Shahabi-Ghahfarokhi, S., Rahmati-Abkenar, M., Turner, S., et al. (2022b). Weakened resilience of benthic microbial communities in the face of climate change. ISME Commun. 2:21. doi: 10.1038/s43705-022-00104-9
Shan, B., Vazquez, E., and Lewis, J. (1990). Interferon selectively inhibits the expression of mitochondrial genes: A novel pathway for interferon-mediated responses. EMBO J. 9, 4307–4314. doi: 10.1002/j.1460-2075.1990.tb07879.x
Smart, L. B., and McIntosh, L. (1991). Expression of photosynthesis genes in the Cyanobacterium Synechocystis sp. PCC 6803: psaA-psaB and psbA transcripts accumulate in dark-grown cells. Plant Mol. Biol. 17, 959–971. doi: 10.1007/BF00037136
Smetacek, V. (1985). Role of sinking in diatom life-history cycles: Ecological, evolutionary and geological significance. Mar. Biol. 84, 239–251.
Staniczenko, P. P., Lewis, O. T., Tylianakis, J. M., Albrecht, M., Coudrain, V., Klein, A.-M., et al. (2017). Predicting the effect of habitat modification on networks of interacting species. Nat. Commun. 8:792.
Stigebrandt, A. (2001). “Physical oceanography of the Baltic Sea,” in A systems analysis of the Baltic Sea, eds F. V. Wulff, L. A. Rahm, and P. Larsson (Berlin Heidelberg: Springer), 19–74.
Suikkanen, S., Kaartokallio, H., Hällfors, S., Huttunen, M., and Laamanen, M. (2010). Life cycle strategies of bloom-forming, filamentous Cyanobacteria in the Baltic Sea. Deep Sea Res. II Top. Stud. Oceanogr. 57, 199–209.
Thureborn, P., Franzetti, A., Lundin, D., and Sjöling, S. (2016). Reconstructing ecosystem functions of the active microbial community of the Baltic Sea oxygen depleted sediments. PeerJ 4:e1593. doi: 10.7717/peerj.1593
Vause, B. J., Morley, S. A., Fonseca, V. G., Jażdżewska, A., Ashton, G. V., Barnes, D. K., et al. (2019). Spatial and temporal dynamics of Antarctic shallow soft-bottom benthic communities: Ecological drivers under climate change. BMC Ecol. 19:27. doi: 10.1186/s12898-019-0244-x
Vehmaa, A., Larsson, P., Vidoudez, C., Pohnert, G., Reinikainen, M., and Engström-Öst, J. (2011). How will increased Dinoflagellate: Diatom ratios affect copepod egg production?–A case study from the Baltic Sea. J. Exp. Mar. Biol. Ecol. 401, 134–140.
Vyverman, W. (1992). Multivariate analysis of periphytic and benthic diatom assemblages from Papua New Guinea. Hydrobiologia 234, 175–193.
Wang, Z., Lei, M., Ji, S., Xie, C., Chen, J., Li, W., et al. (2022). Comparison in diversity of eukaryotic algae in surface sediments from different functional sea areas of Qingdao coast, the Yellow Sea, China: A metabarcoding approach. J. Oceanol. Limnol. 40, 2322–2342.
Wasmund, N., Nausch, G., and Matthäus, W. (1998). Phytoplankton spring blooms in the southern Baltic Sea–spatio-temporal development and long-term trends. J. Plankton Res. 20, 1099–1117.
Weber, T., Wiseman, N. A., and Kock, A. (2019). Global ocean methane emissions dominated by shallow coastal waters. Nat. Commun. 10:4584.
Wei, H., Sun, J., Moll, A., and Zhao, L. (2004). Phytoplankton dynamics in the Bohai Sea–observations and modelling. J. Mar. Syst. 44, 233–251.
Weisse, T., and Sonntag, B. (2016). “Ciliates in planktonic food webs: Communication and adaptive response,” in Biocommunication of ciliates, eds G. Witzany and M. Nowacki (Berlin: Springer), 351–372.
Keywords: marine, RNA transcripts, diversity, community structure, functional activity
Citation: Li S, Nilsson E, Seidel L, Ketzer M, Forsman A, Dopson M and Hylander S (2024) Baltic Sea coastal sediment-bound eukaryotes have increased year-round activities under predicted climate change related warming. Front. Microbiol. 15:1369102. doi: 10.3389/fmicb.2024.1369102
Received: 11 January 2024; Accepted: 05 March 2024;
Published: 26 March 2024.
Edited by:
Yunyun Zhuang, Ocean University of China, ChinaReviewed by:
Longhui Deng, Shanghai Jiao Tong University, ChinaJun Gong, Sun Yat-sen University, China
Copyright © 2024 Li, Nilsson, Seidel, Ketzer, Forsman, Dopson and Hylander. This is an open-access article distributed under the terms of the Creative Commons Attribution License (CC BY). The use, distribution or reproduction in other forums is permitted, provided the original author(s) and the copyright owner(s) are credited and that the original publication in this journal is cited, in accordance with accepted academic practice. No use, distribution or reproduction is permitted which does not comply with these terms.
*Correspondence: Songjun Li, c29uZ2p1bi5saUBsbnUuc2U=