- 1Department of Cardiology, Shibei Hospital, Shanghai, China
- 2Shanghai Jing’an District Pengpu Town Second Community Health Service Center, Shanghai, China
Introduction: Cardiovascular diseases, including myocardial infarction, remain a leading cause of death globally. Emerging evidence suggests the gut microbiota plays a crucial role in cardiovascular health. This study aims to explore the impact of gut microbiota on myocardial infarction using a mouse model.
Methods: The research utilizes a multi-omics approach, including 16S rDNA sequencing and LC-MS-based metabolomics to analyze fecal and serum samples from mice modeled to mimic myocardial infarction. This methodology allows for a comprehensive analysis of microbial populations and their metabolic output.
Results: The findings reveal a significant reduction in gut microbiota α-diversity in mice with induced myocardial infarction compared to healthy controls. Notably, there is an increase in populations of Fusobacteria and Clostridia. Metabolomic analysis indicates disruptions in amino acid and energy metabolism, suggesting a metabolic dysregulation linked to myocardial health.
Discussion: The study proposes a novel microbiota-metabolite-myocardium axis, where specific microbial metabolites may directly affect heart health. This connection points to the gut microbiota as a potential player in the pathogenesis of myocardial infarction and may open new therapeutic avenues targeting the gut microbiome to combat cardiovascular diseases.
1 Introduction
Myocardial infarction, a critical issue within cardiovascular health, emerges due to insufficient coronary arterial blood flow, leading to myocardial necrosis (Smit et al., 2020). This condition presents through various clinical symptoms, notably chest pain, ECG changes, and elevated cardiac enzymes (Gong et al., 2020). Myocardial infarction is classified into two primary types: ST-segment elevation myocardial infarction (STEMI) and non-ST-segment elevation myocardial infarction (NSTEMI), with NSTEMI being more subtle and less pronounced in its clinical manifestations (Byrne et al., 2024). The consequences of myocardial infarction are severe, potentially resulting in cardiogenic shock, arrhythmias, and significantly affecting patient quality of life, highlighting the critical nature of this condition (Jung et al., 2021; Samsky et al., 2021; Sterling et al., 2023). The incidence of myocardial infarction has been on the rise, with China reporting approximately 3 million new cases annually (Song et al., 2020). Despite advancements in medical and interventional treatments, the short-term mortality rate for myocardial infarction patients can reach up to 7.5%, and the five-year survival rate does not exceed 50–60% (Song et al., 2020). These statistics underscore myocardial infarction as a leading cause of cardiovascular mortality (Yang et al., 2022). Current treatment strategies, such as thrombolysis and recanalization, are vital yet insufficient to reverse ischemic injury (Widimsky et al., 2023). This underscores the necessity for a deeper understanding of myocardial infarction’s pathogenesis and the exploration of new therapeutic targets, emphasizing the ongoing quest for more effective treatments in the battle against this life-threatening condition.
One of the pivotal etiological factors of myocardial infarction is identified as coronary atherosclerosis, which precipitates thrombus formation leading to occlusion (Ambrose and McEnroe, 2022). The progression towards this occlusive thrombus is not linear but is facilitated by a cascade of physiological disruptions including inflammatory responses, oxidative stress, endothelial dysfunction, among others (Carresi et al., 2021). Beyond these, metabolic disorders have been recognized as significant contributors to the risk and development of acute myocardial infarction (Hasebe and Hasebe, 2022). Perturbations in energy metabolism, particularly within the tricarboxylic acid cycle, have been documented in the context of myocardial infarction, suggesting a critical impairment in energy substrate utilization during cardiac injury (Sanchez-Gimenez et al., 2023). Intriguingly, recent explorations have begun to unravel a potential linkage between myocardial infarction and gut microbiota dysbiosis, although the specific mechanistic pathways remain to be fully elucidated. This nascent area of research opens a novel vista for understanding myocardial infarction, positing the gut microbiome as a significant player in the modulation of cardiovascular health. The interplay of traditional factors such as coronary atherosclerosis and metabolic disorders with emerging insights into microbiota dysbiosis underscores the multifaceted nature of myocardial infarction pathogenesis, heralding a new era of comprehensive cardiovascular research and therapeutic strategies.
The human gut is a vast ecosystem, teeming with trillions of microbes, collectively termed the gut microbiome (Gomaa, 2020). The advent of advanced genomic sequencing technologies has facilitated a profound understanding of these microbial communities and the genetic material they harbor (Rezasoltani et al., 2020). This groundbreaking research has established the gut microbiome as a fundamental pillar of human physiology, exerting considerable influence over metabolism, immune function, and nutritional status (Wiertsema et al., 2021). Dysbiosis, a term denoting the disruption of this microbial equilibrium, has been implicated in a myriad of health conditions, notably type 2 diabetes and cardiovascular diseases (Tian et al., 2021). The modulation of the gut microbiome emerges as a promising avenue for the prevention and treatment of cardiometabolic disorders.
Recent investigations have highlighted alterations in the gut microbiota composition of patients with myocardial infarction, including an enhanced prevalence of Fusobacteria (Mutalub et al., 2022). These findings, albeit preliminary, underscore the potential interplay between gut microbiota and cardiovascular health. However, the exploration of this relationship is currently hampered by limitations such as small study cohorts and the reliance on singular methodological approaches. Comprehensive analyses focusing on the gut microbiota’s role in myocardial infarction are imperative for identifying pivotal pathogenic factors and novel therapeutic targets. Short-chain fatty acids (SCFAs), including acetate, propionate, and butyrate, are metabolites produced by gut microbes, such as those from the Lachnospiraceae and Ruminococcaceae families, through the fermentation of dietary carbohydrates (Song et al., 2021). These SCFAs are integral not only for the energy supply of colonocytes and the maintenance of intestinal barrier integrity but also for their systemic effects on cholesterol and lipid metabolism via receptor interactions (He et al., 2020).While prior research has concentrated on these and other gut microbiota-derived metabolites, such as TMAO and carnitine, offering insights into the pathogenesis of heart attacks, a holistic approach to study the disease in its entirety has been lacking (Wang et al., 2022).
The objective of this study is to develop a mouse model of myocardial infarction to methodically examine the variances in gut microbiota structure and metabolic profiles between afflicted and control groups. Through the integration of multi-omics data, this research aims to delineate the pathogenesis network of myocardial infarction, thereby providing a scientific foundation for the development of targeted therapeutic interventions. This methodology is poised to offer a comprehensive overview of the microbiota-cardiovascular axis, elucidating the connections between microbial metabolites and crucial host pathways and target genes. This endeavor is anticipated to facilitate personalized risk assessment and intervention strategies for cardiovascular diseases, leveraging a systems biology approach to unveil new mechanistic insights into myocardial infarction.
2 Methods
2.1 Experimental animals
Twenty healthy male C57BL/6 mice (SPF grade) weighing 20 ± 2 g at 8 weeks old were purchased from Shanghai SLAC Laboratory Animal Co., Ltd. After transport to the lab, they were housed in a specific pathogen-free environment at 22 ± 2°C and 60 ± 5% humidity with free access to food and water and a 12 h light/dark cycle. After acclimatization for 1 week, the mice were randomly divided into control and model groups (n = 10 each) using a random number table. Strict national experimental animal management regulations were followed to provide suitable breeding conditions. All animal experiments were approved by the ethics committee of Shibei Hospital. To induce the model of MI, occlusion of the left anterior descending coronary artery (LAD) was employed. In brief, mice were anaesthetized with R520 mobile small animal anesthesia machine (Reward Life Technology Co., Ltd., Shenzhen, China). The skin, fat and muscle were gently separated and then the fourth intercostal space on the left side of the chest was exposed. Left thoracotomy was performed to expose the heart, and the left anterior descending (LAD) coronary artery was visualized. An 8-0 nylon suture was passed under the LAD and ligated 1–2 mm below the tip of the left atrium. Occlusion was confirmed by observing blanching of the anterior wall of left ventricle post ligation. The lungs were inflated by increasing positive pressure ventilation before closing of thoracic cavity. Analgesics were administered post-surgery for pain management. Animal experiments were conducted after approval by the Ethics Committee of the Shanghai Shibei Hospital, and the ethical approval number is: SHIB-2022191.
After surgery, the mice were randomly divided into two groups (n = 10): (A) sham group (without LAD), (B) MI group (with LAD). The serum samples were collected for serum untargeted metabolomics analysis. All rats were sacrificed under the deeply anesthetized condition after echocardiographic examinations. The serum fecal and samples were collected for 16 s rDNA sequencing.
2.2 Assessment of cardiac function and histological analysis
Two weeks after the induction of MI, all mice were anaesthetized lightly with 1.5% isoflurane and mice undergo echocardiographic assessment (Vevo 2100) to evaluate cardiac function. Measurements include left ventricular ejection fraction (LVEF), left ventricular fractional shortening (LVFS). These parameters are used to assess the extent of cardiac dysfunction and remodeling following MI.
After echocardiographic assessment, mice are euthanized, and hearts are excised and fixed in formalin. Paraffin-embedded heart sections are stained with Masson’s trichrome to quantify fibrosis, with collagen fibers appearing blue and cardiac muscle fibers appearing red. Hematoxylin and eosin (H&E) staining is performed to evaluate general tissue morphology, including inflammation and necrosis. Terminal deoxynucleotidyl transferase dUTP nick end labeling (TUNEL) staining is used to detect apoptotic cells, with positive cells appearing as dark brown or black nuclei.
2.3 Metabolomics experiment
In the metabolomics study, 100 μL of serum samples were initially combined with 300 μL of chilled methanol, which included phenylalanine as an internal standard. This mixture was then vortexed for 3 min and subsequently centrifuged at 12,000 rpm for 10 min at a temperature of 4°C. The resulting supernatant was carefully transferred into autosampler vials, prepared for LC-MS/MS analysis.
The analysis of the metabolic profile was conducted using a Dionex Ultimate 3000 RS UHPLC system, coupled with a Q-Exactive Plus quadrupole-Orbitrap mass spectrometer. This system was equipped with a heated electrospray ionization (ESI) source from Thermo Fisher Scientific, Waltham, MA, United States, and was operated in both positive and negative ESI ion modes. For the chromatographic separation, an ACQUITY UPLC HSS T3 column (1.8 μm, 2.1 × 100 mm) was utilized in both ionization modes.
The separation was accomplished using a binary gradient elution system, comprising of solvent A (water with 0.1% formic acid, v/v) and solvent B (acetonitrile with 0.1% formic acid, v/v). The gradient program was set as follows: starting with 5% B at 0 min, maintaining 5% B till 2 min, increasing to 25% B at 4 min, 50% B at 8 min, 80% B at 10 min, reaching 100% B at 14 min and holding till 15 min, then returning to 5% B at 15.1 min and holding till 16 min. The flow rate was maintained at 0.35 mL/min, and the column temperature was controlled at 45°C. All samples were kept at a constant temperature of 4°C during the analysis. The injection volume for each sample was 2 μL, and the mass spectrometer was set to scan a mass range from m/z 100 to 1,000.
The resolution settings for the mass spectrometer were 70,000 for full MS scans and 17,500 for HCD MS/MS scans. The collision energies were adjusted to 10, 20, and 40 eV. The operational parameters for the mass spectrometer included a spray voltage of 3,800 V in the positive mode and 3,000 V in the negative mode, a sheath gas flow rate of 35 arbitrary units, an auxiliary gas flow rate of 8 arbitrary units, a capillary temperature of 320°C, an auxiliary gas heater temperature of 350°C, and an S-lens RF level set at 50. Quality control samples (QCs) were injected at regular intervals throughout the analytical run, providing a dataset to evaluate the repeatability of the analysis.
2.4 Data preprocessing and statistical analysis for metabolomics
The LC–MS data were processed using Progenesis QI V2.3 software (Nonlinear Dynamics, Newcastle, United Kingdom). This processing included several critical steps: baseline filtering, peak identification, integration, retention time correction, peak alignment, and normalization. Key settings for this process were a precursor tolerance of 5 ppm, product tolerance of 10 ppm, and a product ion threshold of 5%.
Compound identification hinged on the accurate mass-to-charge ratio (m/z), MS1/MS2 fragment ions, and isotopic distribution. This identification utilized several databases, including The Human Metabolome Database (HMDB), Lipid Maps (V2.3), Metlin, EMDB, and PMDB. The structures of the compounds were further elucidated using our library of internal standard metabolites, ensuring a match in exact masses, fragment ion masses, and retention times.
The data extracted underwent additional processing. Peaks with missing values (ion intensity = 0) in more than half of the samples within any group were removed. Zero values were substituted with half of the minimum observed value. Screening was also conducted based on the qualitative results of the compounds, discarding those with scores below 36 out of 60, as they were considered inaccurate.
A comprehensive data matrix was then compiled from both positive and negative ion data sets. This matrix was imported into R software for Principal Component Analysis (PCA), which helped in assessing the overall distribution of the samples and the stability of the analytical process.
For more detailed analysis, Orthogonal Partial Least-Squares-Discriminant Analysis (OPLS-DA) were employed. These methods were used to differentiate metabolites across various groups. To ensure the robustness of these models and prevent overfitting, a 7-fold cross-validation and 200 Response Permutation Testing (RPT) were performed.
Variable Importance of Projection (VIP) scores from the OPLS-DA model were utilized to determine the significance of each variable in group discrimination. A two-tailed student’s t-test was then applied to ascertain the statistical significance of the differences in metabolites between groups. Differential metabolites were ultimately selected based on VIP values exceeding 1.0 and p-values lower than 0.05.
2.5 Microbiological experiment
The extraction of DNA was carried out with the DNeasy PowerSoil Kit, strictly following the protocol provided by the manufacturer. The extracted DNA’s concentration was determined using the Quant-IT PicoGreen kit from Invitrogen. For the preparation of the sequencing library, protocols from the Illumina 16S Metagenomic Sequencing Library were utilized, specifically targeting the amplification of the V3 and V4 regions. A total of 2 ng of genomic DNA (gDNA) was amplified through PCR, employing a composition of 1× reaction buffer, 1 nM dNTP mix, 500 nM of each universal F/R PCR primer, and 2.5 U of Herculase II fusion DNA polymerase provided by Agilent Technologies, Santa Clara, CA, United States. The PCR cycling conditions were set as follows: an initial heat activation for 3 min at 95°C, followed by 25 cycles each consisting of 30 s at 95°C, 30 s at 55°C, and 30 s at 72°C, concluding with a final extension phase of 5 min at 72°C. The primer pair used for this initial amplification included universal primers with Illumina adapter overhang sequences, specifically V3-F: 5′-TACGGRAGGCAGCAG-3′ and V4-R: 5′-AGGGTATCTAATCCT-3′. This initial PCR product was then purified using AMPure beads from Agencourt Bioscience, Beverly, MA, United States. Post-purification, 10 μL of this product underwent further PCR amplification using the NexteraXT Indexed Primer for the final assembly of the library, following the same cycling conditions as the initial PCR, but limited to 10 cycles. The resultant PCR product was again purified using AMPure beads. This final purified product was quantified via qPCR, in line with the qPCR Quantification Protocol Guide from the KAPA Library Quantification kits designed for Illumina Sequencing Platforms, and its quality was assessed using a TapeStation D1000 ScreenTape from Agilent Technologies, Waldbronn, Germany. The final step involved paired-end sequencing (2 × 300 bp) performed by the MiSeq™ platform from Illumina.
2.6 16S rDNA data processing
Raw paired-end 16S rDNA sequences from the V3–V4 region were consolidated into consensus fragments using FLASH software (version 1.2.11). This step was followed by a filtration process where reads were clustered at 97% similarity with the aid of CD-HIT-OTU. This process involved the detection and removal of chimeric sequences and discarding reads of low quality, ambiguous nature, or those shorter than 400 bp. The high-quality reads that remained were then grouped into operational taxonomic units (OTUs) using a greedy algorithm.
Taxonomic categorization of these OTUs was carried out using the BLAST tool (version 2.4.0). Subsequent microbial community analyses, including evaluations of alpha-diversity and beta-diversity, as well as principal coordinate analyses (PCoA), were conducted utilizing the QIIME software (version 1.0). Alpha-diversity metrics such as Chao1, Shannon, and inverse Simpson indices were calculated. Beta-diversity, on the other hand, was assessed through PCoA based on weighted UniFrac distances.
The normality of data distribution was tested using Shapiro–Wilk’s test. Differences in relative abundance of microbial communities were examined using statistical approaches like Student’s t-test or Wilcoxon rank sum test, depending on the data suitability. To adjust for the risk of false findings due to multiple hypothesis testing, the false discovery rate (FDR) method was employed.
For the identification of potential metagenomic biomarkers, Linear Discriminant Analysis Effect Size (LEfSe) was utilized. This technique involves class comparison, checks for biological consistency, and estimates the size of the effects. Visual representation of differentially abundant functional categories, with an LDA score of 2.0 or higher, was achieved through heatmap and PCoA analyses.
Additionally, the Analysis of Similarities (ANOSIM) was used to compare matrices of rank dissimilarities. For functional gene enrichment analysis, the PICRUSt (Phylogenetic Investigation of Communities by Reconstruction of Unobserved States) approach was employed. This method estimates the proportional contributions of each sample’s KEGG categories, aiding in the understanding of functional aspects of the microbial communities.
2.7 Multi-omics data integration analysis
To trace the origin of metabolites through database search, researchers conduct Metabolic Pathway Enrichment Analysis (MPEA) based on different sources. By utilizing the powerful Sankey network, they integrate and visualize the correlations at both biological and statistical levels. MetOrigin1 was first utilized to conduct Spearman correlation analysis between gut microbiota and metabolomics. MetOrigin is a bioinformatics tool, aiming to identify which bacteria and how they participate in certain metabolic reactions, helping us to understand where metabolites come from: host, bacteria, or both.
3 Result
3.1 Echocardiographic and histological observations
H&E staining revealed disrupted cardiac architecture, increased inflammatory cell infiltration, and necrotic areas in the MI group (Figures 1A,B). Massons trichrome stain showed extensive fibrosis in the infarcted area of the MI group, with a significant increase in collagen deposition compared to the sham group (Figures 1C,D). TUNEL staining indicated a higher number of apoptotic cells in the peri-infarct region of the MI group, suggesting increased cell death following MI (Figures 1E,F). Echocardiography revealed that mice in the MI group have significantly reduced LVEF and LVFS compared to the sham group, indicating impaired left ventricular systolic function (Figures 1G,H).
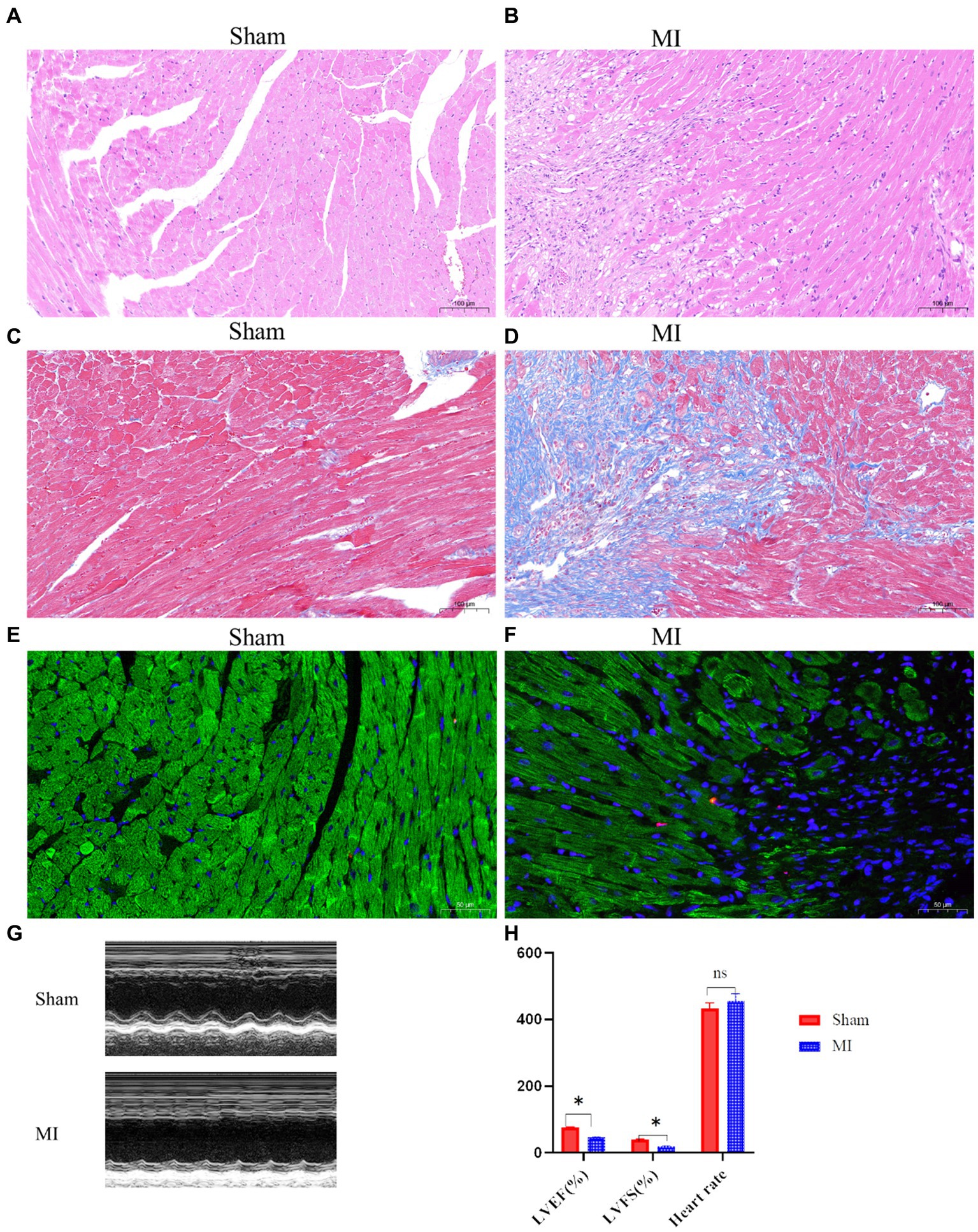
Figure 1. Cardiac function and histological changes in a mouse model of MI. (A,B) Hematoxylin and eosin (H&E) staining shows disrupted cardiac architecture and increased inflammatory cell infiltration in the MI group, whereas the sham group exhibits normal cardiac tissue morphology. (C,D) Masson’s trichrome staining reveals extensive fibrosis (blue areas) in the infarcted myocardium of the MI group, while minimal fibrosis is observed in the sham group. (E,F) Terminal deoxynucleotidyl transferase dUTP nick end labeling (TUNEL) staining indicates a higher number of apoptotic cells (dark nuclei) in the peri-infarct region of the MI group compared to the sham group. (G,H) Representative M-mode echocardiographic images demonstrate impaired left ventricular function in the MI group compared to the sham group.
3.2 Metabolomics analysis results
LC-MS technology was used to detect serum metabolomics profiles between both groups. After QC and standardization, approximately 3,000 features were obtained per sample. Unsupervised PCA clearly separated the two groups. OPLS-DA and random forest models verified good classification and prediction (Figure 2). Differential metabolites were sorted by VIP and validated by SAM, identifying 70 differences involving amino acid, lipid, carbohydrate pathways. Levels of Tetraazacyclododecanetetraacetic acid, MG (18:1(9Z)/0:0/0:0), 10-HDoHE, Acetyl-DL-Leucine, Isovalerylcarnitine, N-Acetyltryptophan, Allitridin, L-Leucine, etc. (Figure 3). Significantly increased in the MI group while PG (18:4/13:0), PA (12:0/20:4-2OH), PS (13:0/14:1), PA (a-13:0/20:5-OH(12)), PA (20:4-OH(11R)/14:1), PS (12:0/16:1), PC (16:0/5:0(CHO)), Trichloroethanol glucuronide, LysoPE (0:0/20:2(11Z, 14Z)), LysoPC (22:1(13Z)/0:0), 1-(9Z-Eicosenoyl)-sn-glycero-3-phosphocholine, D-Mannose etc. decreased (Table 1). Pathway analysis suggested microbial imbalance may have disturbed host D-Amino acid metabolism, Valine, leucine and isoleucine biosynthesis, Phenylalanine metabolism, Aminoacyl-tRNA biosynthesis and mTOR signaling pathway (Figure 4).
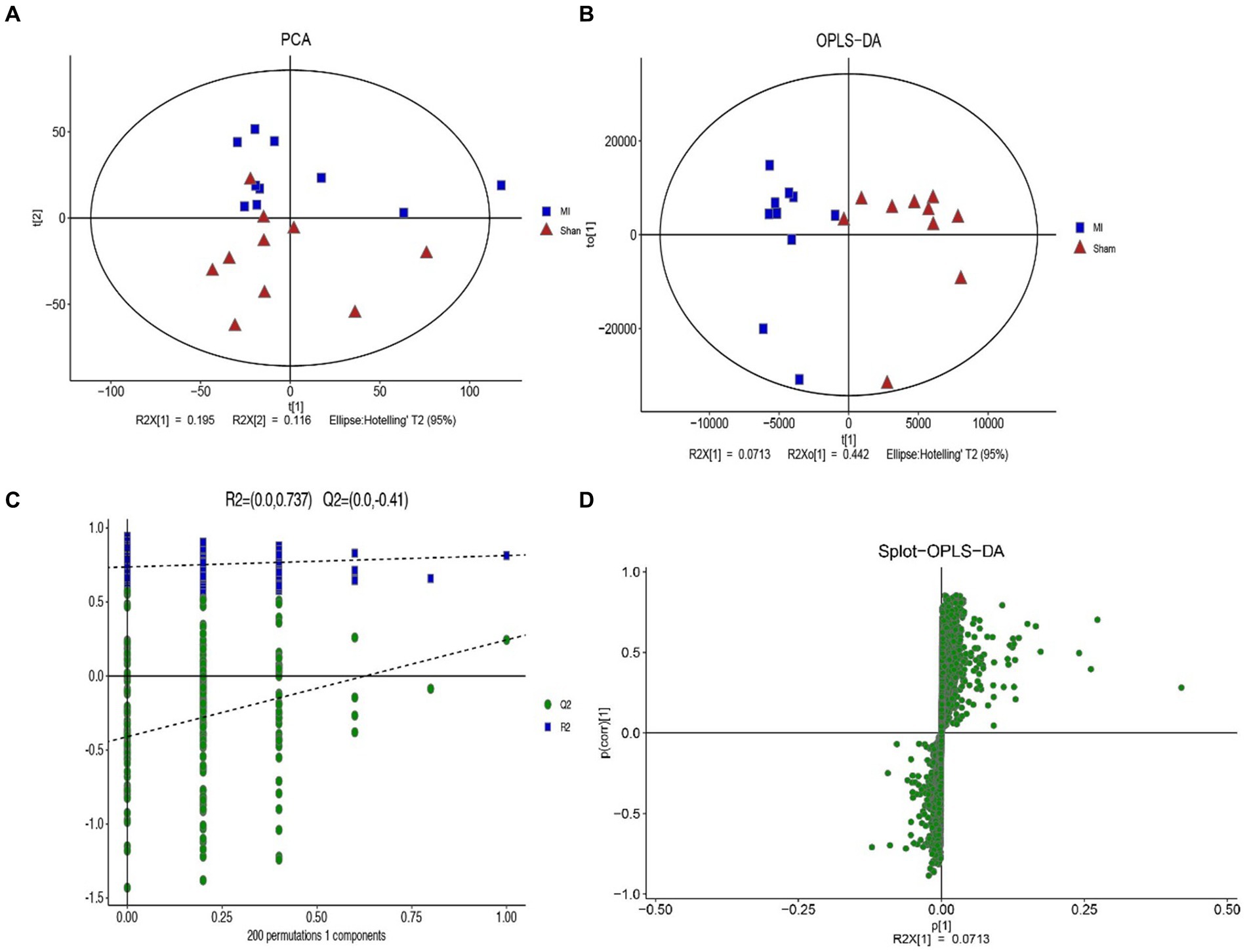
Figure 2. The difference of general serum metabolites with MI and sham group. (A) Principal component analysis (PCA) of general serum metabolites among two groups (R2X = 0.536). (B) OPLS-DA of serum metabolites R2X (cum) = 0.648, R2Y (cum) = 0.814. (C) The OPLS-DA permutation plot (R2 = 0.737, Q2 = −0.41). (D) S-plot of OPLS-DA modeling on MI-sham metabolic profiles.
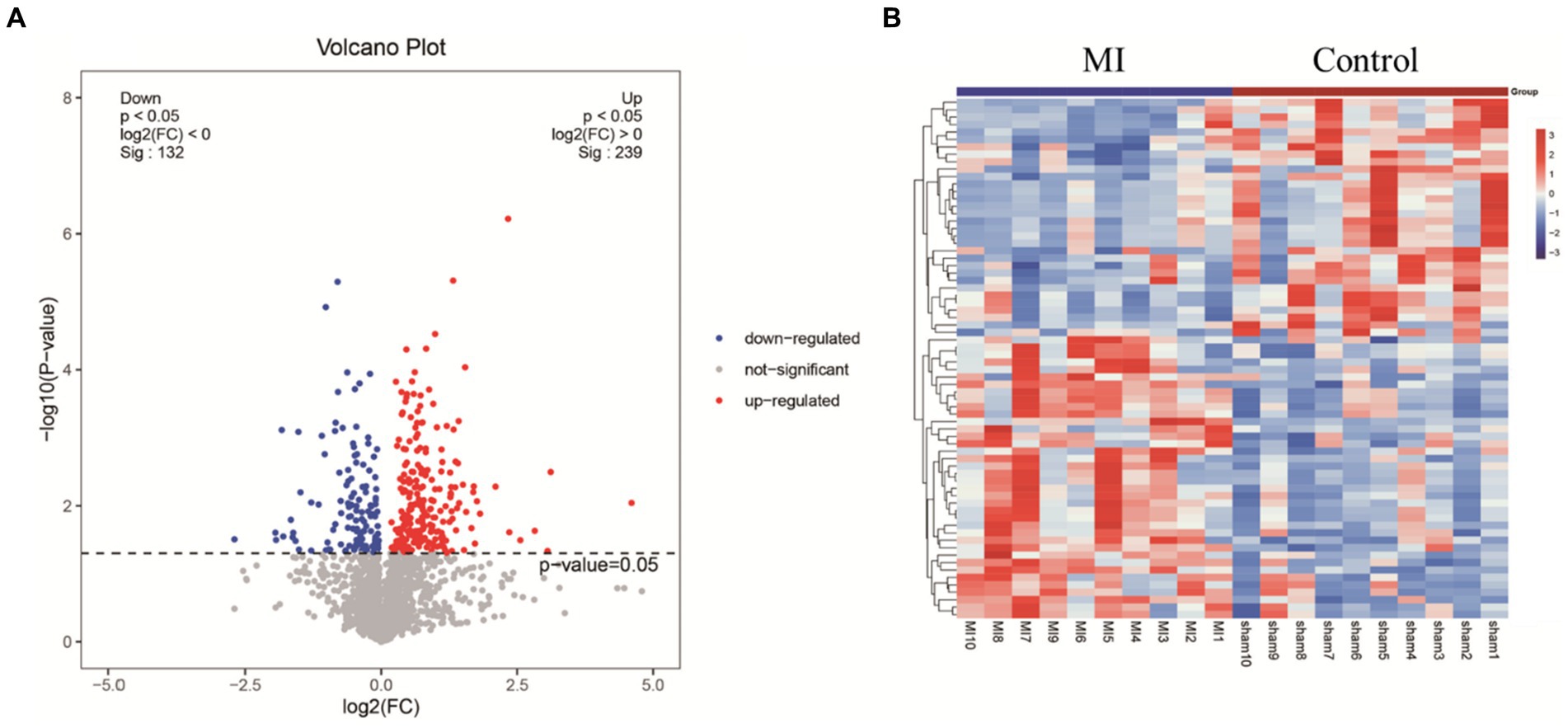
Figure 3. (A) Volcano plot highlights differentially expressed metabolite features between MI and sham group. The x-axis depicts fold change values between the two groups on a log scale, while the y-axis represents negative log transformed p-values from statistical significance tests. In this plot, up-regulated metabolites in MI locate to the right, whereas down-regulated species distribute to the left. (B) Hierarchical cluster heat map reveals distinct metabolite abundance patterns that discriminate between MI and sham group. Columns represent samples and rows denote metabolites. Red indicates metabolite upregulation, while blue signifies downregulation compared to the sham group.
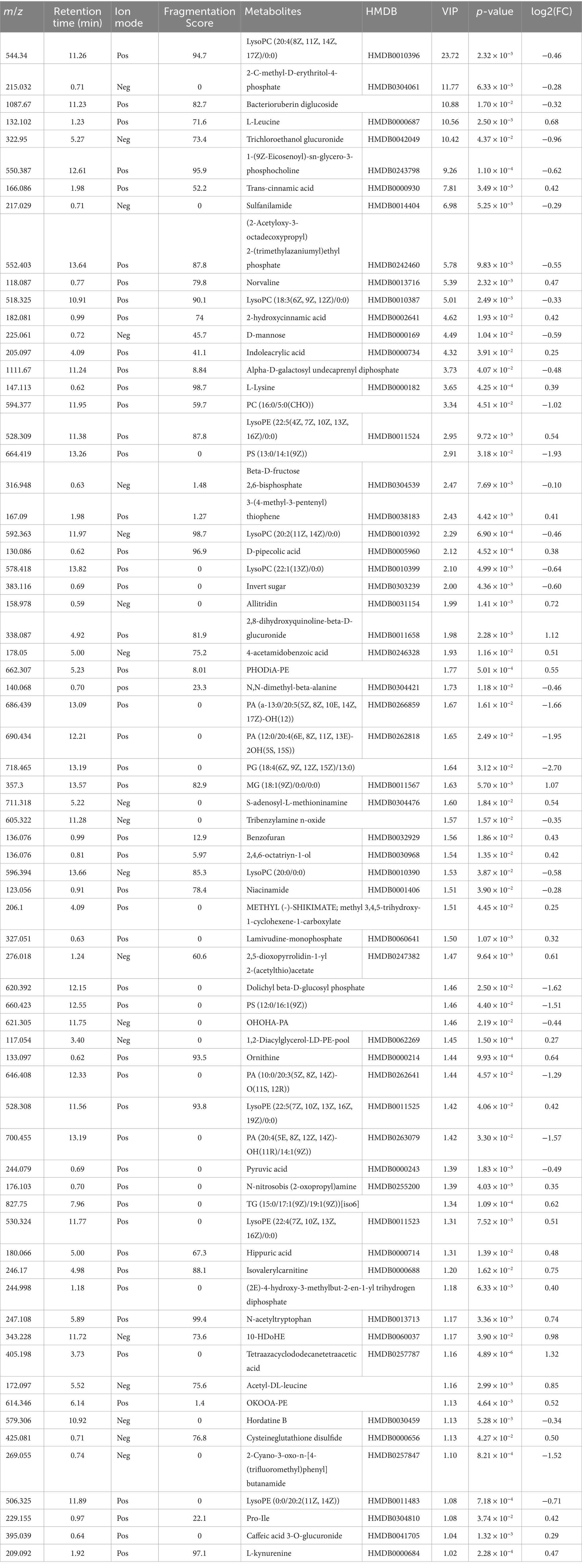
Table 1. Differential metabolites between myocardial infarction (MI) group and sham (Control) group.
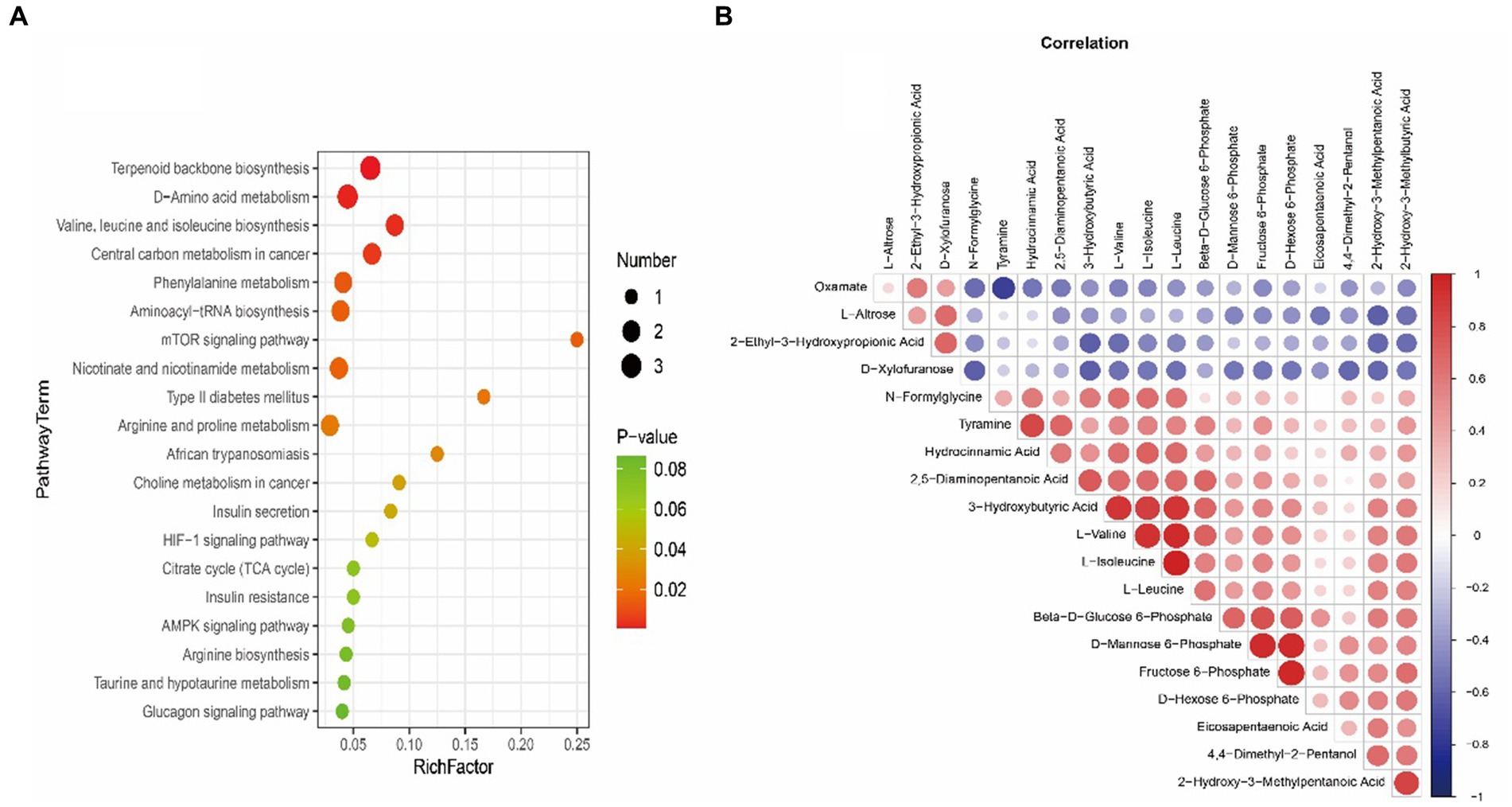
Figure 4. (A) Bubble plot exhibiting significantly enriched metabolic pathways associated with myocardial infarction (MI) identified through pathway enrichment analysis of differential metabolites. The x-axis denotes enrichment factors measuring pathway association strengths. Sizes of bubbles correlate with the number of enriched metabolites mapped to individual pathways. (B) Heatmap visualizing Spearman’s correlations between differential metabolites in myocardial infarction (MI) and sham groups. Positive correlations are shown in red and negative correlations in blue color gradients.
3.3 Microbiological analysis results
This project involved a total of 20 samples. In the differential statistical analysis of the project samples, using the t-test algorithm, we identified 125 differential ASVs, 24 differential genera, and 1 differential phylum. Meanwhile, employing the Wilcoxon algorithm resulted in 165 differential ASVs, 25 differential genera, and 2 differential phyla. The microbial composition of the thrombi varied widely across individuals. Bacteroidota (p) and Firmicutes (p) were the most abundant phyla among the detected microbes (Figure 5). We found 244 species, 151 families, 48 orders, 20 classes, and 14 phyla. Figure 5 displays the general landscape of the microbial composition of all samples at the genus level. Furthermore, taxonomic assignments at the other levels (phylum, class, order, family, and species) are also shown in Figure 5.
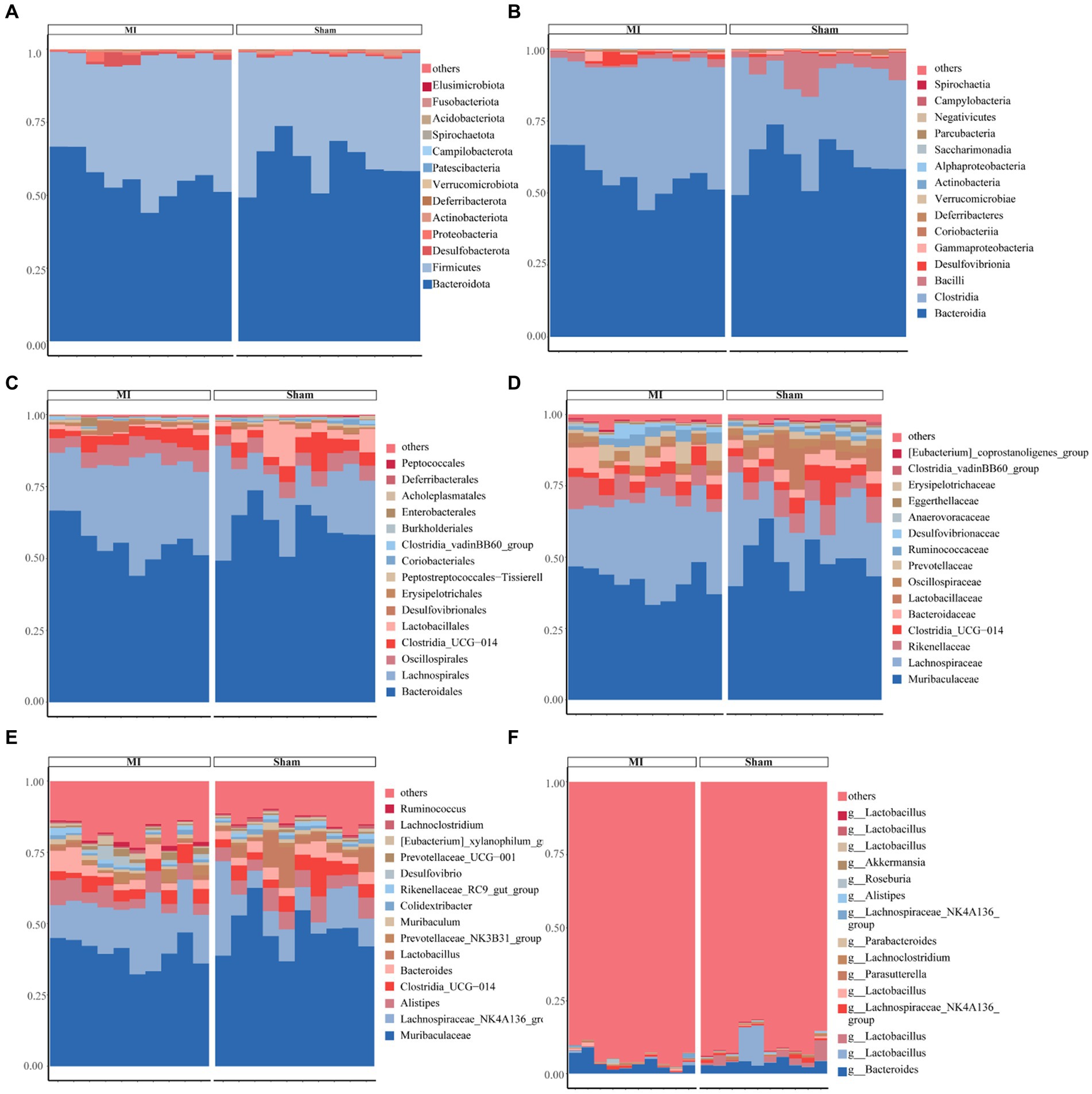
Figure 5. Bar plots exhibiting differential abundance of gut microbiota between MI and sham. (A) Phylum level: Bacteroidota and Firmicutes as dominant phyla. (B) Class level: Bacteroidia and Clostridia as dominant gut microbes. (C) Order level: Bacteroidales and Lachnospirales as dominant gut microbes in MI and sham controls. (D) Family level. (E) Genus level. (F) Species level.
First, microbial diversity was measured. The alpha-diversity of the stool microbiome, defined as the number of species present within each sample, did not differ significantly between groups (Figure 6). We assessed the relationships among bacterial communities from their beta-diversity to generate PCoA plots. OTUs of the thrombus microbiome relative to those of the gut and oral microbiomes displayed different clustering between groups (Figure 6), suggesting phylogenetic closeness between microbial communities within each group.
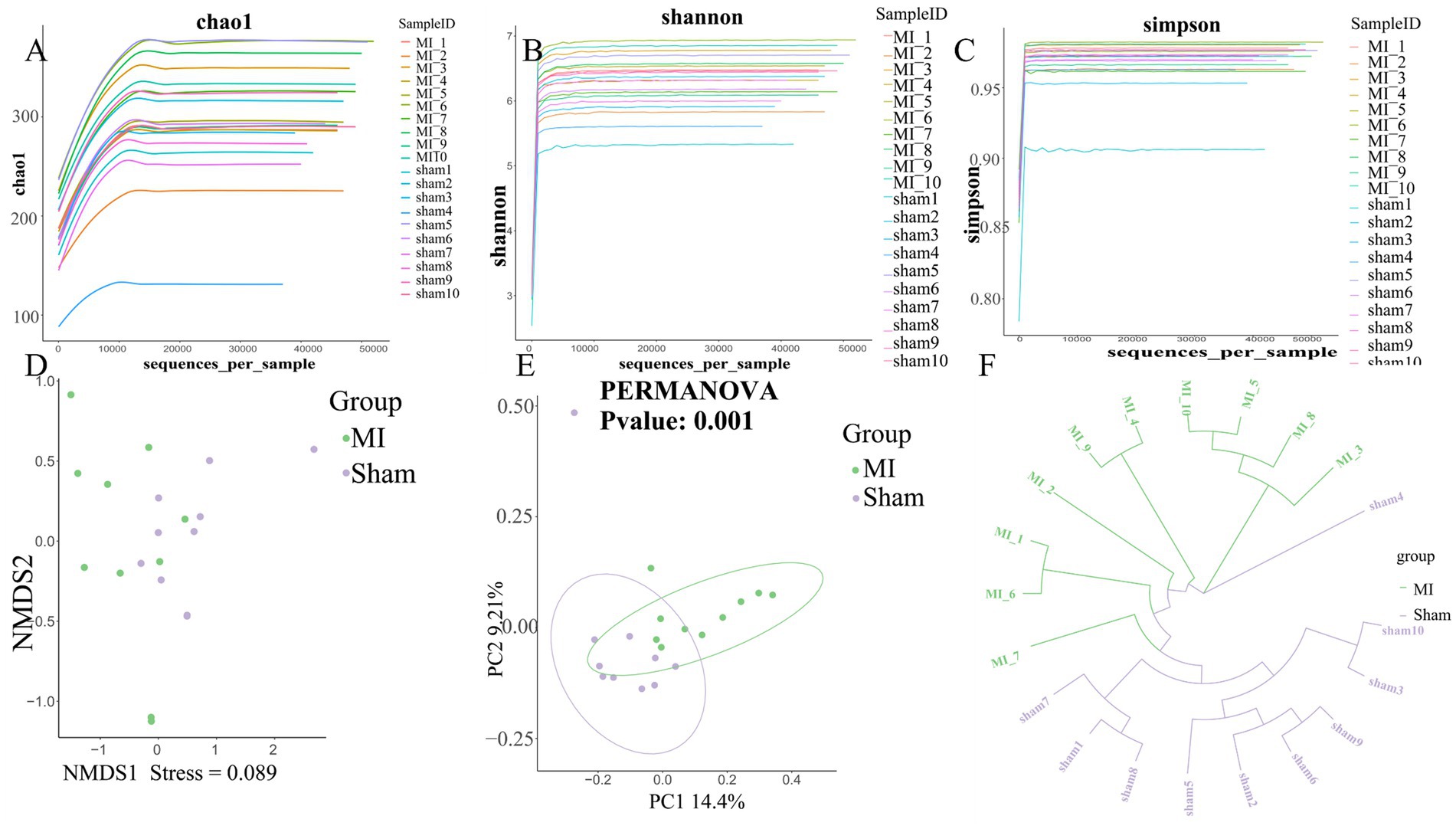
Figure 6. Comprehensive diversity analysis of microbial communities. (A) Alpha diversity, including (A) Chao; (B) Shannon index and (C) Simpson index; (D) Non-metric Multidimensional Scaling (NMDS) analysis; (E) Beta diversity analysis using Principal Coordinates Analysis (PCoA). (F) UPGMA Hierarchical Clustering Dendrogram of Samples.
LEfSe screened 81 clades from the gut microbiome. The MI group displayed dysbiosis of the gut microbiota. The prevalence of Verrucomicrobiota (p) was significantly higher in the MI group than in the sham group (LDA = 3.03, p = 0.049), Blautia(g), Bilophila(g), Butyricicoccus(g), Oscillibacter(g), Intestinimonas(g), Bifidobacterium(g), Ruminococcus(g), Butyricimonas and Parabacteroides(g) were significantly higher in the MI group than in the sham group, while that of Bacilli (c), Clostridiales (o), Lactobacillaceae (f), Lactobacillus (g), Morganella, Turicibacter, Incertae_Sedis, Dubosiella, Muribaculaceae, Caproiciproducens and Streptococcus were significantly lower in MI group. Short-chain fatty acid (SCFA)-producing bacteria displayed a higer abundance (Prevotella (g); Lachnospiraceae (f)) in MI group (Figure 7). The heatmap of the selected most differentially abundant features at the (A) phylum; (B) class; (C) order; (D) family; (E) genus; (F) species levels (Figure 8).
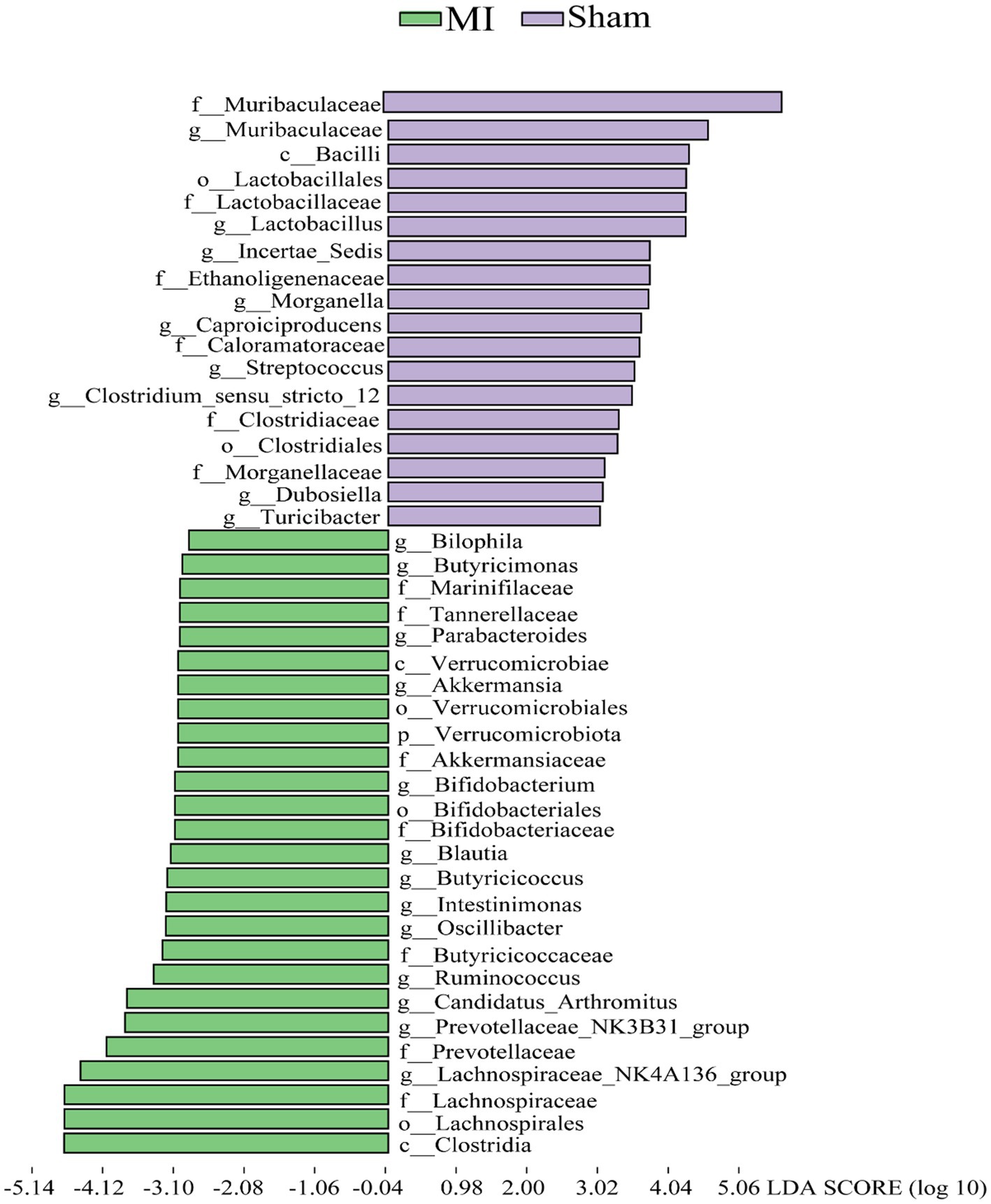
Figure 7. Histogram of the LDA scores for differentially abundant bacterial taxa in the stool sample between the MI group and sham groups. Green and Purple colors represent bacterial taxa that were significantly overrepresented in the MI group and sham groups, respectively.
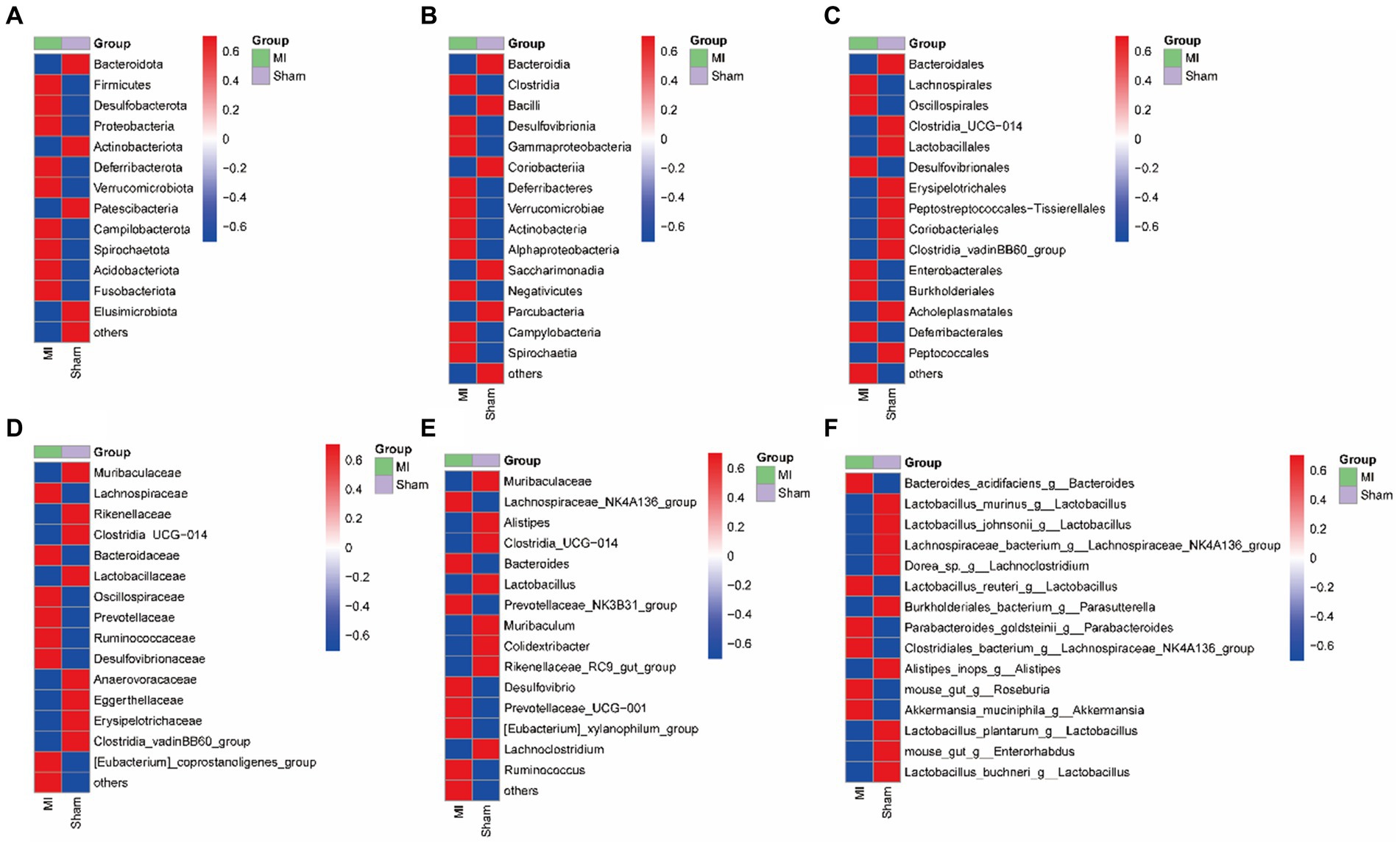
Figure 8. Heatmap of the selected most differentially abundant features at the (A) phylum; (B) class; (C) order; (D) family; (E) genus; (F) species levels. Blue represents lower abundance and red represents the highest abundance.
3.4 Different metabolic pathways of microbial communities between MI and sham group
Bacterial gene functions were predicted via 16S rRNA gene-based microbial compositions using the PICRUSt algorithm to make inferences from KEGG annotated databases. The MI and sham group exhibited significant differences among the 41 KEGG pathways (Figure 9). The MI microbiome displayed differences in Cellular Processes, Environmental Information Processing, Genetic Information Processing, Human Diseases, Metabolism and Organismal Systems in level 1. The MI microbiome displayed differences in amino acid metabolism, Carbohydrate metabolism, Cardiovascular diseases, Circulatory system, Cell motility and biosynthesis of other secondary metabolites in level 2.
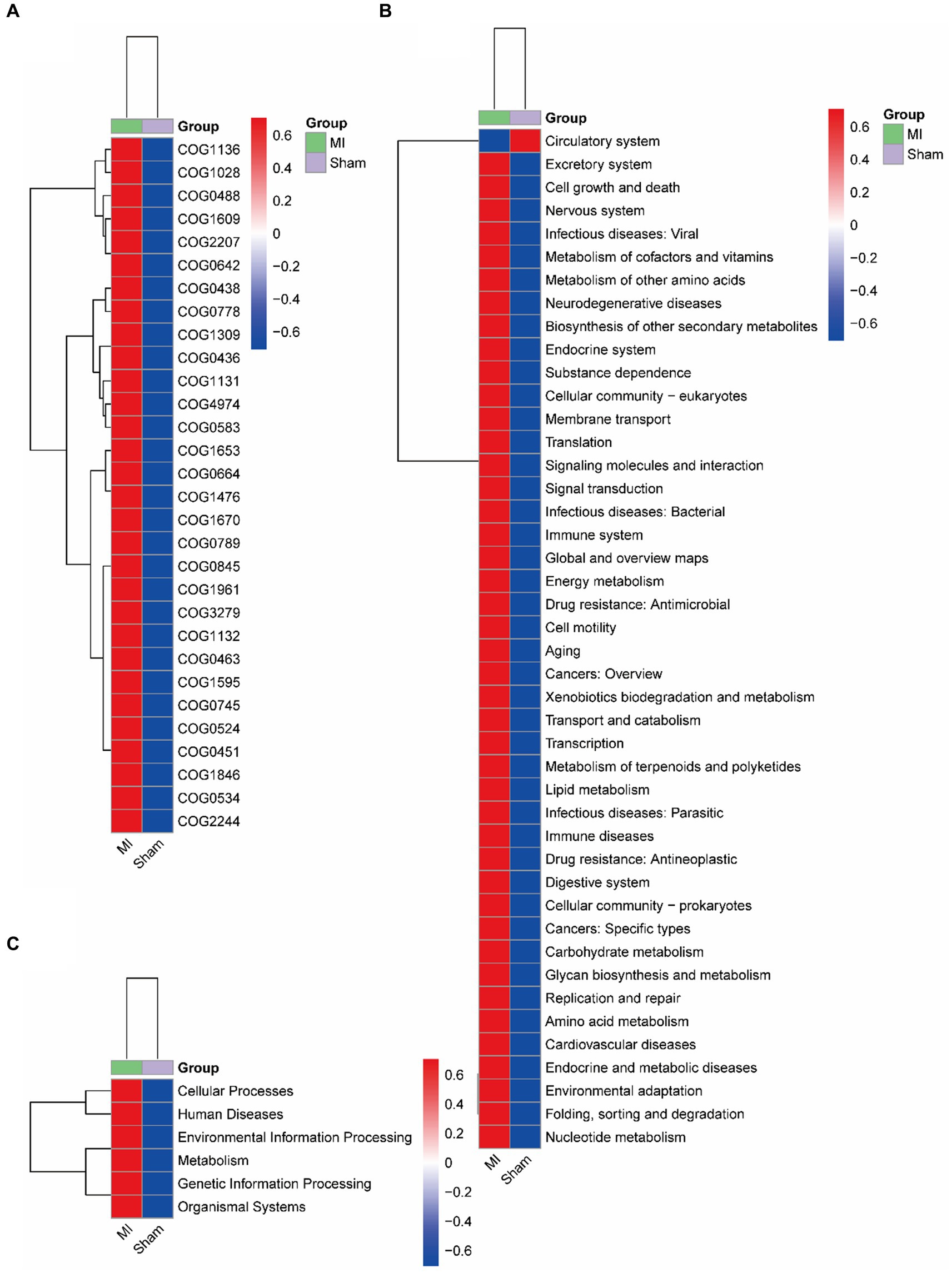
Figure 9. Metabolic implication. Different metabolic pathways of microbial communities between the: a gut microbiome and b oral microbiome groups using the PICRUSt algorithm.
3.5 Multi-omics association analysis results
We subsequently correlated the gut microbiota with serum metabolites to further explore the characteristics of microbiota in MI. The gut microbiota was further correlated with the blood metabolites. Eight blood metabolites, L-Leucine, L-Valine, L-Isoleucine, L-Lysine, L-Aspartic Acid, Ornithine Pyruvic acid and they were positive related with fecal contents of Ruminococcus, Intestinimonas, Lactobacillus, Parabacteroides, Bifidobacterium, Desulfovibrio, Blautia (p < 0.05). These metabolites were negative to Clostridium and Lactobacillus (Figure 10).
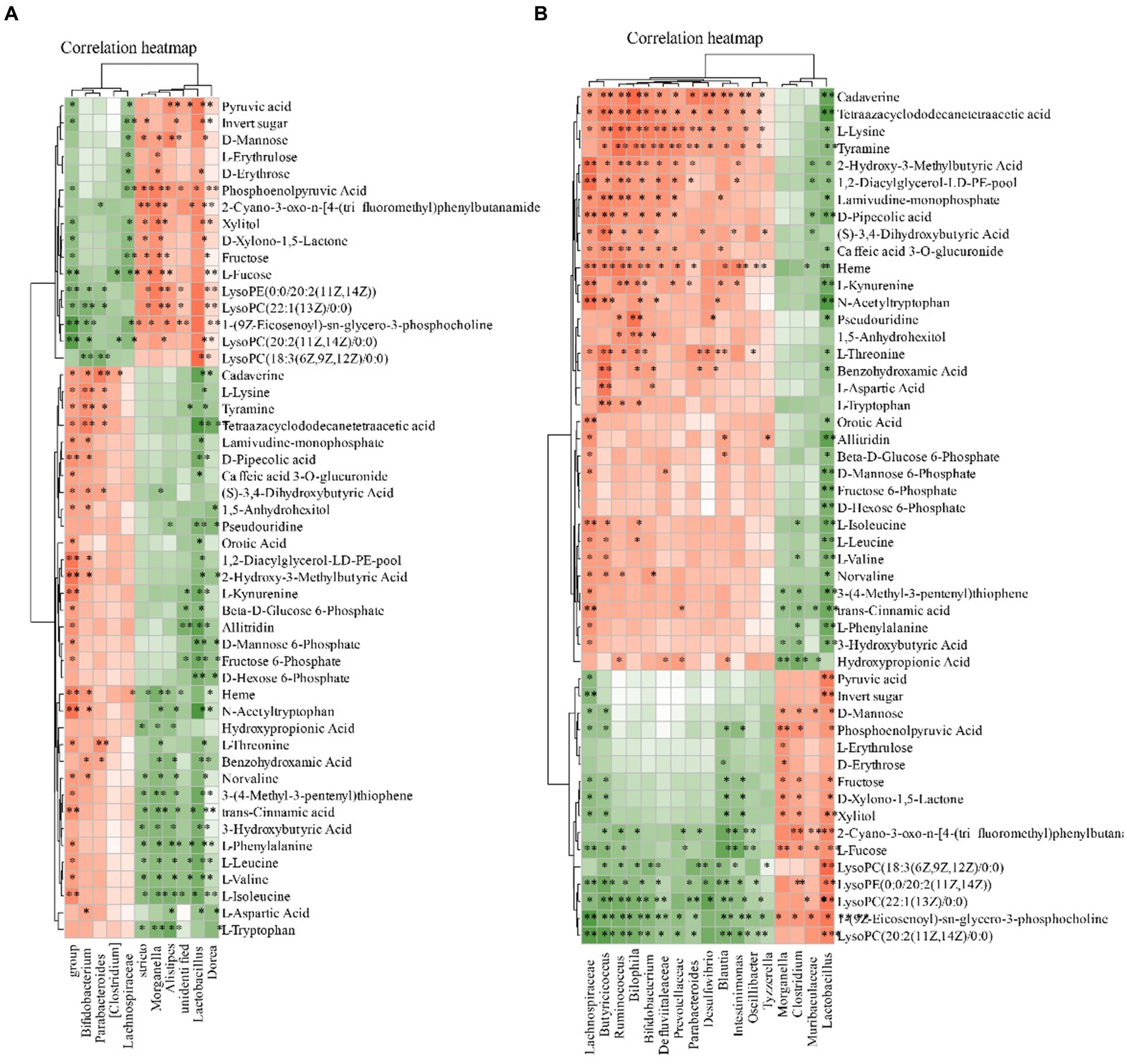
Figure 10. Relationship between the gut microbiota and serum metabolites. (A) Heatmap analysis of microbial-associated metabolite profiles at species level. (B) Heatmap analysis of microbial-associated metabolite profiles at genus level. The color gradient represents the strength and direction of associations: red indicates positive correlation, blue indicates negative correlation. Columns represent individual microbial taxa, and rows represent specific metabolites identified.
4 Discussion
Myocardial infarction (MI), universally recognized as a heart attack, remains at the forefront of global health challenges, contributing significantly to the morbidity and mortality rates worldwide, with an estimated annual incidence nearing 6 million cases (Amini et al., 2021). The pathogenesis of MI is characterized by a complex interplay involving lipid accumulation, inflammation, platelet activation, oxidative stress, and electrolyte imbalance, which collectively precipitate the acute rupture of coronary plaques and subsequent thrombosis (Smit et al., 2020).
Despite considerable advancements in medical interventions for MI, individuals recovering from such a cardiac event continue to face a heightened risk of recurrent ischemia and related comorbidities, which notably impair their quality of life. Emerging omics technologies promise a paradigm shift towards personalized healthcare, aiming to transcend the traditional one-size-fits-all approach. However, the underlying mechanisms of MI pathogenesis require further elucidation to inform the development of novel therapeutic interventions (Zhan et al., 2023). Among the novel discoveries in this domain is the “gut-heart axis,” a testament to the burgeoning recognition of the intestinal microenvironment’s influence on cardiovascular health (Moldovan et al., 2022). The gut microbiome, through its metabolic outputs, has been implicated in MI pathogenesis, affecting endothelial function, atherosclerosis development, thrombosis, and myocardial damage (Xu et al., 2023). Preliminary trials indicate that interventions aimed at restoring eubiosis-whether through nutritional or microbial means—have shown promise in mitigating MI effects. The identification of gut microbiome signatures specific to MI could herald the advent of novel diagnostic biomarkers and therapeutic targets (Dekkers et al., 2022). In conclusion, understanding the decoding mechanistic microbiome-metabolome interplay that governs MI opathology holds the potential to transform future prediction, prevention and precision management of this global threat. Therefore, multi-modal strategies targeting holistic gut-heart axis represent an innovative frontier to attenuate MI severity (Chen et al., 2023).
The serum metabolites identified in this study included amino acids like proline, branched-chain amino acids (Leucine, Isoleucine and Valine); lipids such as monoacylglycerols (MG), triacylglycerols (TG); neurotransmitter precursors for example tryptophan and kynurenine. Meanwhile, microbes belonging to common gut commensals and short chain fatty acids (SCFA) producers were detected, including Bifidobacterium, Lactobacillus, Ruminococcus.
Branched-chain amino acids (BCAAs), including leucine, isoleucine, and valine, are essential amino acids that undergo degradation through a shared metabolic pathway. The breakdown of BCAAs produces branched-chain α-keto acids (BCKAs), including α-ketoisocaproate (KIC), α-keto-β-methylvalerate (KMV), and α-ketoisovalerate (KIV). Defects in BCAA metabolism and elevated levels of BCKAs or BCAAs have been reported in pathologically stressed mouse hearts and human cardiomyopathy (Walejko et al., 2021; McGarrah and White, 2023). In cardiac muscle cells, they impede the activity of mitochondrial Complex I and induce the production of superoxides (McGarrah and White, 2023). Deficiencies in BCAA metabolism significantly contribute to heart failure and myocardial remodeling following chronic pressure overload or myocardial infarction. Mechanistic studies have revealed that BCKAs protect mitochondria and energy production, aiding in cell survival (Karwi and Lopaschuk, 2023). Importantly, BCKAs demonstrate protective effects in both cultured cells and intact hearts, regardless of whether administered as a pre-treatment or post-treatment (Dong et al., 2016). Previous studies have shown that BCKAs alleviate acute ischemic/reperfusion (I/R) injury in the heart, identifying a novel anti-necrotic function of BCAA metabolites and suggesting potential applications of BCKA-like compounds in the treatment of ischemic diseases (Dong et al., 2016).
This study reports an increased abundance of Verrucomicrobiota (p), Blautia(g), Bilophila(g), Butyricicoccus(g), Oscillibacter(g), Intestinimonas(g), Bifidobacterium(g), Ruminococcus(g), Butyricimonas and Parabacteroides(g). These diverse microbial ecosystems undertaking key physiological roles like nutrient digestion, vitamin synthesis, and immune modulation. The microbes, such as short chain fatty acid (SCFA)-producing Blautia, Butyricicoccus and Bifidobacterium; mucin-degraders like Oscillibacter; carbohydrate fermenting Ruminococcus and Intestinimonas. The major fermentation products of these bacteria, including SCFAs like butyrate and acetate, can be absorbed to provide energy, enhance gut barrier integrity, and regulate immunity. However, under disease states like myocardial infarction, the gut microbiota equilibrium gets disrupted, allowing expansion of opportunistic pathogens like Bilophila. This coupled with reduced beneficial symbionts may damage intestinal lining, increase exposure to microbial antigens and metabolites, ultimately overactivating inflammatory and oxidative pathways that accelerate cardiovascular damages. Therefore, characterization of myocardial infarction-associated gut ecosystem changes in a holistic manner is crucial. This allows better understanding of the microbial mediators and mechanisms contributing to cardiovascular pathogenesis. Targeted restoration of key commensals deficient in myocardial infarction patients through probiotics or prebiotics may mitigate gut dysfunction, dampen systemic inflammation, and improve disease outcomes.
The interconnections between gut microbiota, cardiovascular diseases, and renal function highlight the significant impact of gut-derived metabolites on general health. Gut-derived uremic toxins, such as indoxyl sulfate, p-cresyl sulfate, kynurenine, and kynurenic acid, have been identified as harmful metabolites that can lead to cardiovascular diseases in individuals with chronic kidney disease. These metabolites are produced by the gut microbiota and are problematic when kidney function is compromised, leading to their accumulation (El Chamieh et al., 2022). Prebiotics, which foster beneficial gut bacteria, can reduce the production of harmful uremic toxins like indoxyl sulfate and p-cresyl sulfate. These toxins are produced by pathogenic gut bacteria and have significant implications for kidney and cardiovascular health (Falconi et al., 2021). A healthy gut microbiota, supported by prebiotics, plays a vital role in managing these toxins, thereby indirectly supporting both kidney and cardiovascular health (Mitrea et al., 2023).
Our research conducted utilizing a multi-omics approach is comprehensive; however, it is constrained by a limited cohort size that may impede the generalizability of the results. Subsequent investigations could improve upon this limitation by including larger and more diverse study populations, potentially progressing to human clinical trials. Despite the aforementioned limitations, our study makes significant contributions to the field of microbiota research, specifically in elucidating the microbiota-metabolite-myocardium axis. Through the novel application of integrative multi-omics techniques, we conducted a thorough analysis of intricate interactions, yielding fresh perspectives on the impact of post-infarction gut microbiota alterations on myocardial health. This study expands upon existing knowledge and proposes potential clinical implications, including the identification of new biomarkers and therapeutic targets, which may enhance patient care in the context of cardiovascular diseases.
5 Conclusion
This study conducted a comprehensive analysis of the gut microbiomes and serum metabolites in myocardial infarction (MI) and sham groups, revealing significant differences in the composition and function of the microbiomes between the two groups. The findings suggest that microbial dysbiosis might be a contributing mechanism to acute myocardial infarction. The research also discovered microbial communities within human coronary thrombi, which correlated with those in the gut and oral microbiomes. These results pave the way for future research to further understand the clinical implications and to develop new therapeutic approaches. In conclusion, this study provides preliminary evidence that gut dysbiosis is involved in the pathogenesis of myocardial infarction via microbiota-metabolite axes, laying a foundation for new treatment strategies.
Data availability statement
The 16S rDNA has been deposited to NCBI with the accession number PRJNA1102168. The raw date of metabolomics has been deposited to MetaboLights with the accession number MTBLS9620.
Ethics statement
The animal study was approved by the Ethics Committee of Shibei Hospital. The study was conducted in accordance with the local legislation and institutional requirements.
Author contributions
ZG: Writing – original draft. YZ: Project administration, Writing – original draft. LZ: Investigation, Software, Writing – review & editing. PX: Data curation, Validation, Writing – original draft. NG: Validation, Visualization, Writing – review & editing. JL: Investigation, Methodology, Writing – original draft. XY: Supervision, Visualization, Writing – original draft. HC: Funding acquisition, Project administration, Resources, Writing – original draft, Writing – review & editing.
Funding
The author(s) declare that financial support was received for the research, authorship, and/or publication of this article. This work was supported by the Shanghai Jing’an District Discipline Construction Project (2021PY03) and the Jing’an District Health Research Project of Shanghai (2023MS10).
Conflict of interest
The authors declare that the research was conducted in the absence of any commercial or financial relationships that could be construed as a potential conflict of interest.
Publisher’s note
All claims expressed in this article are solely those of the authors and do not necessarily represent those of their affiliated organizations, or those of the publisher, the editors and the reviewers. Any product that may be evaluated in this article, or claim that may be made by its manufacturer, is not guaranteed or endorsed by the publisher.
Footnotes
References
Ambrose, J. A., and Mcenroe, D. J. (2022). In search of coronary thrombosis as the cause of myocardial infarction: unraveling a 20th-century mystery. Am. J. Med. 135, 560–565. doi: 10.1016/j.amjmed.2021.12.007
Amini, M., Zayeri, F., and Salehi, M. (2021). Trend analysis of cardiovascular disease mortality, incidence, and mortality-to-incidence ratio: results from global burden of disease study 2017. BMC Public Health 21:401. doi: 10.1186/s12889-021-10429-0
Byrne, R., Coughlan, J. J., Rossello, X., and Ibanez, B. (2024). The ‘10 commandments’ for the 2023 ESC guidelines for the management of acute coronary syndromes. Eur. Heart J. 45, 1193–1195. doi: 10.1093/eurheartj/ehad863
Carresi, C., Mollace, R., Macrì, R., Scicchitano, M., Bosco, F., Scarano, F., et al. (2021). Oxidative stress triggers defective autophagy in endothelial cells: role in atherothrombosis development. Antioxidants 10:387. doi: 10.3390/antiox10030387
Chen, H. C., Liu, Y. W., Chang, K. C., Wu, Y. W., Chen, Y. M., Chao, Y. K., et al. (2023). Gut butyrate-producers confer post-infarction cardiac protection. Nat. Commun. 14:7249. doi: 10.1038/s41467-023-43167-5
Dekkers, K. F., Sayols-Baixeras, S., Baldanzi, G., Nowak, C., Hammar, U., Nguyen, D., et al. (2022). An online atlas of human plasma metabolite signatures of gut microbiome composition. Nat. Commun. 13:5370. doi: 10.1038/s41467-022-33050-0
Dong, W., Zhou, M., Dong, M., Pan, B., Liu, Y., Shao, J., et al. (2016). Keto acid metabolites of branched-chain amino acids inhibit oxidative stress-induced necrosis and attenuate myocardial ischemia-reperfusion injury. J. Mol. Cell. Cardiol. 101, 90–98. doi: 10.1016/j.yjmcc.2016.11.002
El Chamieh, C., Liabeuf, S., and Massy, Z. (2022). Uremic toxins and cardiovascular risk in chronic kidney disease: what have we learned recently beyond the past findings? Toxins 14:280. doi: 10.3390/toxins14040280
Falconi, C. A., Junho, C., Fogaça-Ruiz, F., Vernier, I. C. S., Da Cunha, R. S., Stinghen, A. E. M., et al. (2021). Uremic toxins: an alarming danger concerning the cardiovascular system. Front. Physiol. 12:686249. doi: 10.3389/fphys.2021.686249
Gomaa, E. Z. (2020). Human gut microbiota/microbiome in health and diseases: a review. Antonie Van Leeuwenhoek 113, 2019–2040. doi: 10.1007/s10482-020-01474-7
Gong, W., Shi, H., Yan, M., Yan, Y., Wang, X., Li, S., et al. (2020). Clinical manifestation, timing course, precipitating factors, and protective factors of ventricular free wall rupture following ST-segment elevation myocardial infarction. Int. Heart J. 61, 651–657. doi: 10.1536/ihj.19-541
Hasebe, T., and Hasebe, N. (2022). Impact of risk factors related to metabolic syndrome on acute myocardial infarction in younger patients. Hypertens. Res. 45, 1447–1458. doi: 10.1038/s41440-022-00951-y
He, J., Zhang, P., Shen, L., Niu, L., Tan, Y., Chen, L., et al. (2020). Short-chain fatty acids and their association with signalling pathways in inflammation, glucose and lipid metabolism. Int. J. Mol. Sci. 21:6536. doi: 10.3390/ijms21176356
Jung, R. G., Di Santo, P., Mathew, R., Abdel-Razek, O., Parlow, S., Simard, T., et al. (2021). Implications of myocardial infarction on management and outcome in cardiogenic shock. J. Am. Heart Assoc. 10:e021570. doi: 10.1161/JAHA.121.021570
Karwi, Q. G., and Lopaschuk, G. D. (2023). Branched-chain amino acid metabolism in the failing heart. Cardiovasc. Drugs Ther. 37, 413–420. doi: 10.1007/s10557-022-07320-4
Mcgarrah, R. W., and White, P. J. (2023). Branched-chain amino acids in cardiovascular disease. Nat. Rev. Cardiol. 20, 77–89. doi: 10.1038/s41569-022-00760-3
Mitrea, L., Medeleanu, M., Pop, C. R., Rotar, A. M., and Vodnar, D. C. (2023). Biotics (pre-, pro-, post-) and uremic toxicity: implications, mechanisms, and possible therapies. Toxins 15:548. doi: 10.3390/toxins15090548
Moldovan, D. C., Ismaiel, A., Fagoonee, S., Pellicano, R., Abenavoli, L., and Dumitrascu, D. L. (2022). Gut microbiota and cardiovascular diseases axis. Minerva Med. 113, 189–199. doi: 10.23736/S0026-4806.21.07527-3
Mutalub, Y. B., Abdulwahab, M., Mohammed, A., Yahkub, A. M., Al-Mhanna, S. B., Yusof, W., et al. (2022). Gut microbiota modulation as a novel therapeutic strategy in cardiometabolic diseases. Food Secur. 11:2575. doi: 10.3390/foods11172575
Rezasoltani, S., Ahmadi Bashirzadeh, D., Nazemalhosseini Mojarad, E., Asadzadeh Aghdaei, H., Norouzinia, M., and Shahrokh, S. (2020). Signature of gut microbiome by conventional and advanced analysis techniques: advantages and disadvantages. Middle East J. Dig. Dis. 12, 5–11. doi: 10.15171/mejdd.2020.157
Samsky, M. D., Morrow, D. A., Proudfoot, A. G., Hochman, J. S., Thiele, H., and Rao, S. V. (2021). Cardiogenic shock after acute myocardial infarction: a review. JAMA 326, 1840–1850. doi: 10.1001/jama.2021.18323
Sanchez-Gimenez, R., Peiró, Ó. M., Bonet, G., Carrasquer, A., Fragkiadakis, G. A., Bulló, M., et al. (2023). TCA cycle metabolites associated with adverse outcomes after acute coronary syndrome: mediating effect of renal function. Front. Cardiovasc. Med. 10:1157325. doi: 10.3389/fcvm.2023.1157325
Smit, M., Coetzee, A. R., and Lochner, A. (2020). The pathophysiology of myocardial ischemia and perioperative myocardial infarction. J. Cardiothorac. Vasc. Anesth. 34, 2501–2512. doi: 10.1053/j.jvca.2019.10.005
Song, T., Guan, X., Wang, X., Qu, S., Zhang, S., Hui, W., et al. (2021). Dynamic modulation of gut microbiota improves post-myocardial infarct tissue repair in rats via butyric acid-mediated histone deacetylase inhibition. FASEB J. 35:e21385. doi: 10.1096/fj.201903129RRR
Song, J., Murugiah, K., Hu, S., Gao, Y., Li, X., Krumholz, H. M., et al. (2020). Incidence, predictors, and prognostic impact of recurrent acute myocardial infarction in China. Heart 107, 313–318. doi: 10.1136/heartjnl-2020-317165
Sterling, L. H., Fernando, S. M., Talarico, R., Qureshi, D., van Diepen, S., Herridge, M. S., et al. (2023). Long-term outcomes of cardiogenic shock complicating myocardial infarction. J. Am. Coll. Cardiol. 82, 985–995. doi: 10.1016/j.jacc.2023.06.026
Tian, R., Liu, H., Feng, S., Wang, H., Wang, Y., Wang, Y., et al. (2021). Gut microbiota dysbiosis in stable coronary artery disease combined with type 2 diabetes mellitus influences cardiovascular prognosis. Nutr. Metab. Cardiovasc. Dis. 31, 1454–1466. doi: 10.1016/j.numecd.2021.01.007
Walejko, J. M., Christopher, B. A., Crown, S. B., Zhang, G. F., Pickar-Oliver, A., Yoneshiro, T., et al. (2021). Branched-chain α-ketoacids are preferentially reaminated and activate protein synthesis in the heart. Nat. Commun. 12:1680. doi: 10.1038/s41467-021-21962-2
Wang, L., Wang, S., Zhang, Q., He, C., Fu, C., and Wei, Q. (2022). The role of the gut microbiota in health and cardiovascular diseases. Mol. Biomed. 3:30. doi: 10.1186/s43556-022-00091-2
Widimsky, P., Snyder, K., Sulzenko, J., Hopkins, L. N., and Stetkarova, I. (2023). Acute ischaemic stroke: recent advances in reperfusion treatment. Eur. Heart J. 44, 1205–1215. doi: 10.1093/eurheartj/ehac684
Wiertsema, S. P., Van Bergenhenegouwen, J., Garssen, J., and Knippels, L. M. J. (2021). The interplay between the gut microbiome and the immune system in the context of infectious diseases throughout life and the role of nutrition in optimizing treatment strategies. Nutrients 13:886. doi: 10.3390/nu13030886
Xu, H., Yang, F., and Bao, Z. (2023). Gut microbiota and myocardial fibrosis. Eur. J. Pharmacol. 940:175355. doi: 10.1016/j.ejphar.2022.175355
Yang, B., Bai, L., Zhang, Y., Cheng, Y., Zhao, C., Huang, B., et al. (2022). The value of different short-term risk scoring models in predicting long-term death of acute myocardial infarction. J. Clin. Med. 11:5054. doi: 10.3390/jcm11175054
Keywords: myocardial infarction, microbiota-metabolite-myocardium axis, 16S rDNA, metabolomics, LC-MS
Citation: Guo Z, Zhong Y, Zhou L, Xu P, Gao N, Lu J, Yan X and Cao H (2024) Unveiling the microbiota-metabolite-myocardium axis: a novel perspective on cardiovascular health. Front. Microbiol. 15:1389311. doi: 10.3389/fmicb.2024.1389311
Edited by:
Zhangran Chen, Xiamen University, ChinaReviewed by:
Zhenshang Xu, Qilu University of Technology, ChinaLaura Mitrea, University of Agricultural Sciences and Veterinary Medicine of Cluj-Napoca, Romania
Copyright © 2024 Guo, Zhong, Zhou, Xu, Gao, Lu, Yan and Cao. This is an open-access article distributed under the terms of the Creative Commons Attribution License (CC BY). The use, distribution or reproduction in other forums is permitted, provided the original author(s) and the copyright owner(s) are credited and that the original publication in this journal is cited, in accordance with accepted academic practice. No use, distribution or reproduction is permitted which does not comply with these terms.
*Correspondence: Huaming Cao, Y2htX2hhcHB5MzY1QDEyNi5jb20=
†These authors have contributed equally to this work