- 1Key Laboratory of Animal Genetics, Breeding and Reproduction in the Plateau Mountainous Region, Ministry of Education, College of Animal Science, Guizhou University, Guiyang, China
- 2Department of Agricultural, Food and Nutritional Science, University of Alberta, Edmonton, AB, Canada
- 3Department of Veterinary and Animal Sciences, Faculty of Health and Medical Sciences, University of Copenhagen, Frederiksberg, Denmark
Introduction: Gut microbiota are associated with the health and performance of ruminant species, and they are affected by altitude, host genetics, and sex. However, there has been little research on comparing the fecal microbiota of indigenous small ruminants such as sheep and goats in Guizhou province, China. In the present study, we revealed the effect of altitude, genetics, and sex on fecal microbiota profiles and enterotypes in indigenous small ruminants of Guizhou province, China.
Methods: Fecal samples were collected from Hei and Qianbei Ma goats and Weining sheep in the Chinese province of Guizhou. 16S rRNA gene sequencing targeting the V3–V4 region was performed using the Illumina MiSeq platform. Sequences were processed using QIIME2, and the qualified sequences were processed using the plugin DADA2 to generate amplicon sequence variants (ASVs). The statistical analysis was performed using R studio.
Results: The fecal microbial profile was found to vary by herd (influenced by genetics/altitude) and sex. All samples were categorized into two enterotypes. The first enterotype is dominated by UCG-005, and the second enterotype is dominated by the Christensenellaceae_R-7_group, which may be highly driven by the host's genetics (breed). The predicted functional profiles of the fecal microbiota were also assigned to two clusters that corresponded exactly to the enterotypes. Cluster 1 of the functional profiling was characterized by biosynthesis pathways, and cluster 2 was characterized by energy metabolism pathways.
Discussion: Our findings may provide new insights into the fecal microbial community and enterotypes in small ruminants by herds, offering clues for understanding the mechanisms by which the fecal microbiota contribute to divergent host phenotypes in indigenous small ruminants in Guizhou.
Introduction
In southwest China, the altitude of Guizhou province ranges from 200 m (east) to 2,800 m (west), and it is considered the Guizhou Plateau. Multiple domestic animal species, such as cattle, sheep, and goats, are pastured in this area by farmers. In 2022, the ruminant population in Guizhou was 875 million (with cattle accounting for 485 million and sheep and goats making up 390 million), contributing to approximately 3% of the local agricultural output. Among them, small ruminants raised in this area contribute to a large proportion of the livestock industry (5%), and are thus of great economic value.
It has been shown that the composition of gut microbiota is influenced by common factors including genetics, sex, and altitude (Moeller and Sanders, 2020; Bai et al., 2022; Guo et al., 2022). In addition, rumen microbiota are essential for converting recalcitrant plant materials into nutrients for the host and for maintaining bovine health (Lopes et al., 2019; Virgínio Júnior and Bittar, 2021), while fecal microbiota are indicators of hindgut microbiota, which are involved in the last stage of plant material digestion and absorption and in supporting host health (Mao et al., 2015; Guo et al., 2020; Wang et al., 2023). Therefore, the impact of altitude, genetics, and sex on rumen and fecal microbiota of ruminants has attracted increasing attention. Recent studies have suggested that the fecal microbiome and metabolomics of Sanhe heifers (Zhang et al., 2023), as well as rumen microbiota composition and fermentation profiles, change with altitudes (Han et al., 2022). In addition, the composition and function of the fecal microbiota in wild blue sheep vary with sex (Zhu et al., 2020), as do the composition and fermentation profiles of rumen microbiota (Guo et al., 2022). Furthermore, the evenness of fecal bacterial (Mahayri et al., 2022) and the composition of rumen bacteria (Wu et al., 2020) are affected by host genetics. These results indicate that altitude, host genetics, and sex are important factors that can affect the diversity and composition of gut microbiota. Although some studies have been conducted to explore the rumen microbiota community of local goats in Guizhou (Tian et al., 2021, 2022; Zhou et al., 2022), there has been little research on comparing the fecal microbiota of indigenous small ruminants such as sheep and goats in Guizhou province, China.
In the current study, we collected fecal samples from three individual herds consisting of two goat breeds and one sheep breed living between 1,000 and 2,200 m above sea level (a.s.l) in a high-mountain ecosystem. We included both male and female animals from each population to determine the effect of altitude, genetics, and sex on fecal microbiota profiles and enterotypes in small ruminants.
Materials and methods
Animals and sampling
The sheep and goats used in this study were aged between 2 and 4 years, and the experiment was conducted between July and August 2022. In this study, 35 fecal samples were collected from Weining sheep (H) (F = 23 and M = 12), 23 from Qianbei Ma goats (L) (F = 11 and M = 12), and 32 from Hei goats (M) (F = 16 and M = 16), raised in Weining (26°36′N, 103°36′E; 2,200 m), Xishui (28°19′N, 106°12′E; 1,040 m), and Weining (26°44′N, 104°39′E; 1,900 m), respectively, in Guizhou, China. The Weining sheep (a dual-purpose breed for wool and meat) and Hei goats (meat) grazed freely in the natural pasture, and the dominant and associated plant genera included Digitaria, Poa, Agrostis, and Festuca. The Weining sheep and Hei goats were corralled the night before the sample collection. The Qianbei Ma goats (a dual-purpose breed for wool and meat) were housed indoors and fed a basal diet twice daily (9:00 and 17:00), with free access to water, as described in a previous study (Fu et al., 2022), and weaned at 3 months of age. Before the morning feeding and grazing, the fecal samples were collected from the rectum of the animals using clean disposable latex gloves and were frozen in liquid nitrogen immediately and then stored at −80°C until further analysis.
DNA extraction and amplicon sequencing
Total genomic DNA was extracted from the fecal samples using a QIAamp DNA stool mini kit (Qiagen, Valencia, CA, US) following the manufacturer's instructions. The concentration of the extracted DNA was checked using a Nanodrop spectrophotometer (Thermo Scientific, Wilmington, DE, US). The qualified DNA was then amplified using the primer set 338F/806R targeting the hypervariable V3–V4 regions of bacterial 16S rRNA following standard PCR conditions (Kim et al., 2021). The qualified PCR products were sequenced using the Illumina MiSeq platform (2 × 300 paired-end sequencing runs). All the data used in this study are available in the National Center for Biotechnology Information Sequence Read Archive (NCBI SRA) under the accession number PRJNA1006184.
Bioinformatics and statistical analysis
Sequences were processed using QIIME2 (v 2022.2) (Bolyen et al., 2019). After demultiplexing, the sequences were quality filtered, the chimera was removed, and the subsequent reads were merged using the plugin DADA2 to generate amplicon sequence variants (ASVs) (Callahan et al., 2016). Then, a taxonomic classification of the ASVs was obtained using the plugin q2-feature-classifier against the SILVA 132 99% reference database (Kim et al., 2021). For diversity analysis, the sequences were rarefied to 16,006 reads per sample to avoid uneven sequencing depth. Furthermore, the alpha diversity was determined using Shannon and Chao1 indices, while the beta diversity was estimated based on the Bray–Curtis distances, which was then presented in a principal coordinate analysis (PCoA) plot. Finally, microbial functional predictions and pathway inferences were performed using PICRUSt2 based on the MetaCyc database (Caicedo et al., 2020). The statistical data analysis was conducted using R studio (version 3.5.3). For example, the differential abundance of the taxonomic composition (at the phylum and genus levels) and the Metacyc pathways among herds were determined by conducting linear discriminant analysis effect size (LEfSe) (Segata et al., 2011). A differential analysis of the alpha diversity among herds was conducted using the Kruskal–Wallis test followed by Dunn's post hoc multiple-comparison text. A Mann–Whitney U test was performed to compare the median similarities of the alpha diversity between female and male animals within each herd. Differences in the microbial composition within each herd were calculated using DESeq2 (Love et al., 2014). The effect of herd and sex on the beta diversity was evaluated using PERMANOVA with the default parameter (Anderson, 2014). Taxa that occurred in at least 25% of the animals within each herd and had a relative abundance of >0.1% were retained for the subsequent analysis.
Enterotype clusters at the genus level were calculated using the Jensen–Shannon divergence (JSD) distance and the Partitioning Around Medoids (PAM) clustering algorithm (Mobeen et al., 2018). The optimal number of clusters was determined using the Calinski–Harabasz (CH) index, while the robustness of the clusters was evaluated using the Silhouette index (Mobeen et al., 2018). The Mann–Whitney U test was employed to determine the differential microbial taxa between enterotypes, and the taxa with the highest relative abundances among these differential microorganisms were regarded as the proxy of the enterotypes (Huang et al., 2022). Values were represented as the mean ± standard error of the mean (SEM) unless otherwise noted. Statistical significance was set at a p-value of < 0.05.
Results
Fecal microbiota characteristics in small ruminants among different herds
After quality control, a total of 1,794,289 sequences with an average of 19,936 sequences per sample were obtained and assigned to 8,564 ASVs (Table 1). The Good's coverage for all animals was found to be over 99.97%, indicating that the sequencing depth covered most of the fecal bacteria. The Shannon and Chao1 indices were higher in the M herd among the three herds (Figure 1A), and the bacterial community displayed a clear separation based on the herd under study (PERMANOVA P = 0.001, Figure 1B).
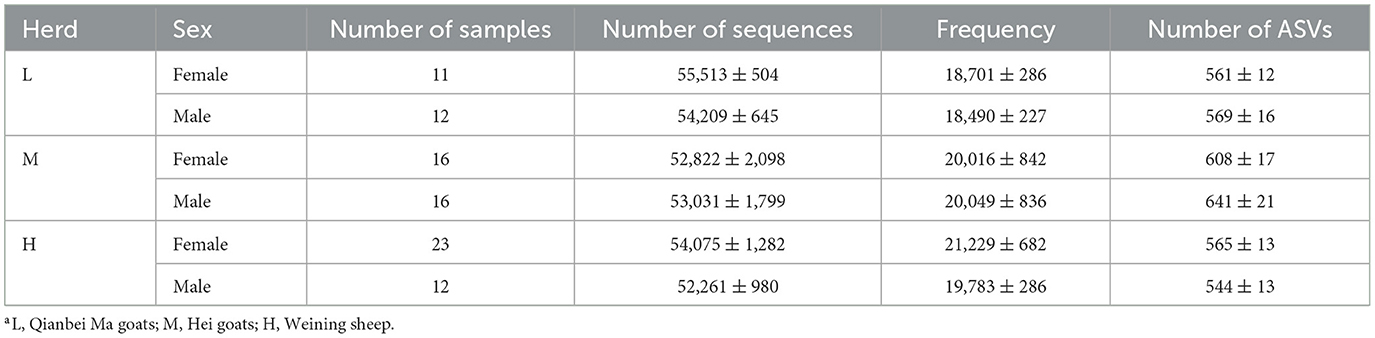
Table 1. The detailed information of the sample of different herdsa and sequence features obtained using the DADA2 algorithm.
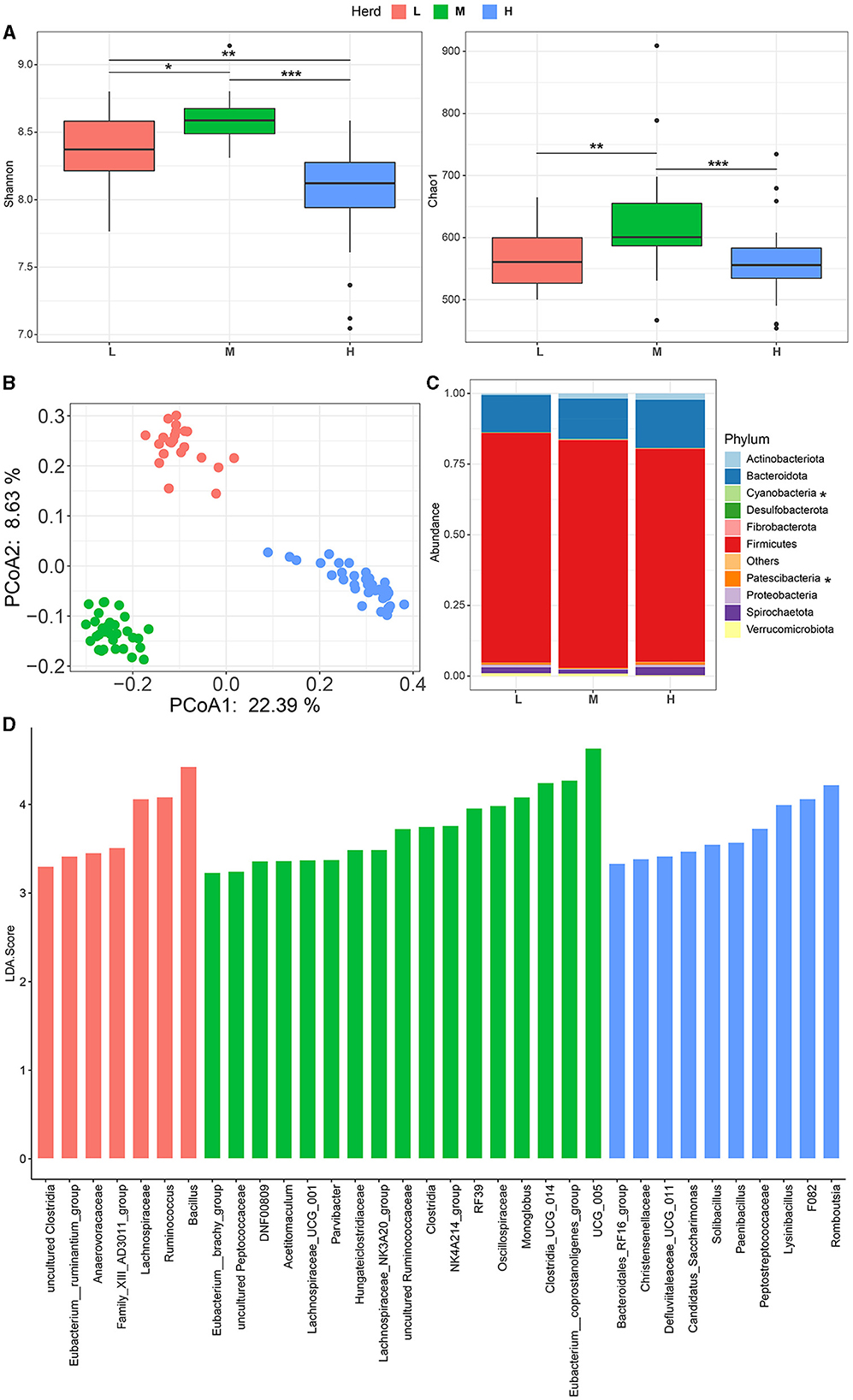
Figure 1. Fecal microbial community composition in small ruminants among different herds. Alpha (A) and Beta (B) diversities at different herds. (C) Fecal bacterial composition at the phylum level. (D) Differential fecal bacterial taxa within different herds. * p< 0.05, ** p< 0.01, and *** p< 0.001. L, Qianbei Ma goats; M, Hei goats; H, Weining sheep.
For the taxonomic profile, a total of 11 phyla were identified according to the selected criteria mentioned in the Materials and Methods section, with Firmicutes (78.7 ± 0.01%) and Bacteroidota (14.8 ± 0.01%) (synonym Bacteroidetes) being the predominant taxa in the fecal microbiome (Figure 1C, Supplementary Table S1). The relative abundances of Cyanobacteria and Patescibacteria were significantly different between different herds (p < 0.05, Figure 1C). In total, 108 genera were identified based on the selected criteria, of which UCG-005 (12.1 ± 0.005%) was the dominant genus, followed by Christensenellaceae_R-7_group (11.2 ± 0.004%), f.Lachnospiraceae (5.7 ± 0.002%), UCG-010 (3.7 ± 0.001%), Clostridia_UCG-014 (3.4 ± 0.002%), and Rikenellaceae_RC9_gut_group (3.0 ± 0.002%) (Supplementary Table S2). Among these, a total of 34 bacterial genera were differentially identified among different herds (Figure 1D). Specifically, the fecal microbiota of the L herd was enriched with Bacillus (L: 5.1%, M: 0, and H: 1.1%) and Ruminococcus (L: 3.7%, M: 1.9%, and H: 1.4%) (Figure 1D, Supplementary Table S2). Moreover, the relative abundances of UCG_005 (M: 16%, L: 13.1%, and H: 7.9%) and Clostridia_UCG_014 (M: 5.2%, L: 3.3%, and H: 1.9%) were found to be overrepresented in the M herd (Figure 1D, Supplementary Table S2). In addition, the relative abundances of Romboutsia (H: 4.5%, L: 1.5%, and M: 2.3%) and F082 (H: 2.4%, L: 0.3%, and M: 0.2%) were greater in the H herd (Figure 1D, Supplementary Table S2). The Venn diagram showed that 51 genera were shared by all herds and that the L herd and M herd shared more taxa with each other than with the H herd. Each herd had unique taxa, and the number of unique genera in the H herd (n = 17) was greater than that in the M herd (n = 8) and the L herd (n = 9) (Supplementary Figure S1).
Effect of sex on the composition of the fecal microbiota
To determine whether sex affects the fecal microbiota profile, the microbial composition between female and male animals within each herd was compared. The alpha diversity (Shannon and Chao1) indices did not differ between the female and male animals in the L herd and H herd, whereas the Shannon index was greater in male animals than in female animals in the M herd (Figure 2A). The principal coordinate analysis (PCoA) based on Bray–Curtis distances displayed a clear separation between female and male animals within each herd (PERMANOVA p < 0.05, Figure 2B).
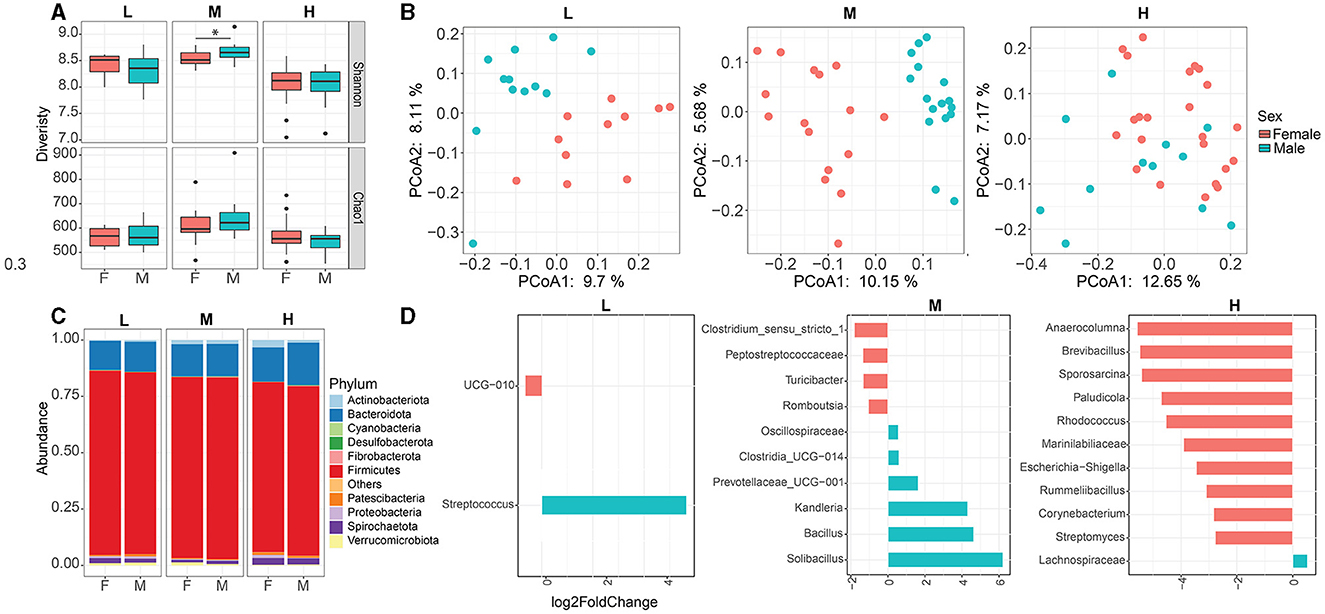
Figure 2. Effect of sex on fecal microbial community composition within each herd. Alpha (A) and Beta (B) diversities between female and male animals within each herd. (C) Fecal microbial composition between female and male animals at the phylum level. (D) Differential microbial taxa between female and male animals within each herd. * p< 0.05. L, Qianbei Ma goats; M, Hei goats; H, Weining sheep.
Taxonomic profiles were analyzed to evaluate and determine variations in the bacterial structure between female and male animals within each herd. Overall, the dominant phyla were Firmicutes (female vs. male 80.4% vs. 80.6%), Bacteroidota (female vs. male: 14.2% vs. 14.3%), and Actinobacteriota (female vs. male: 1.9% vs. 1.8%) in the M herd, and the relative abundances of Fibrobacterota (female vs. male: 0.1% vs. 0.4%) and Desulfobacterota (female vs. male: 0.07% vs. 0.1%) were greater in male animals than in female animals (p < 0.05). In addition, the relative abundance of Verrucomicrobiota (female vs. male: 1.3% vs. 0.6%) was greater in female animals than in male animals in the M herd. Similarly, the predominant phyla in the L herd and H herd were Firmicutes (L: 81.8% vs. 80.6%; H: 75.5% vs. 75.2%), Bacteroidota (L: 12.9% vs. 13.3%; H: 15.2% vs. 18.8%), and Actinobacteriota (0.35% vs. 0.75%). Patescibacteria (0.46% vs. 0.98%) were lesser in female animals than in male animals in the L herd (p < 0.05, Figure 2C). Furthermore, the relative abundance of Actinobacteriota was greater in female animals than in male animals in the H herd (Figure 2C). At the genus level, the top four most abundant genera were UCG-005, Christensenellaceae_R-7_group, Lachnospiraceae, and UCG-010 within each herd (Supplementary Table S2), and the relative abundances of Lachnospiraceae and UCG-010 were distinct between female and male animals from the L herd and H herd (Figure 2D).
Enterotype profile of bacterial community
A total of 90 fecal samples were divided into enterotypes 1 and 2 using principal coordinate analysis (PCoA) (Figure 3A), and the enterotypes were mainly driven by genera UCG-005 (enterotype 1, n = 54) and Christensenellaceae_R-7_group (enterotype 2, n = 36) (Figure 3B), respectively. All samples from the M herd and 22 samples from the L herd belonged to enterotype 1, whereas all samples from the H herd and 1 sample from the L herd belonged to enterotype 2 (Figure 3C).
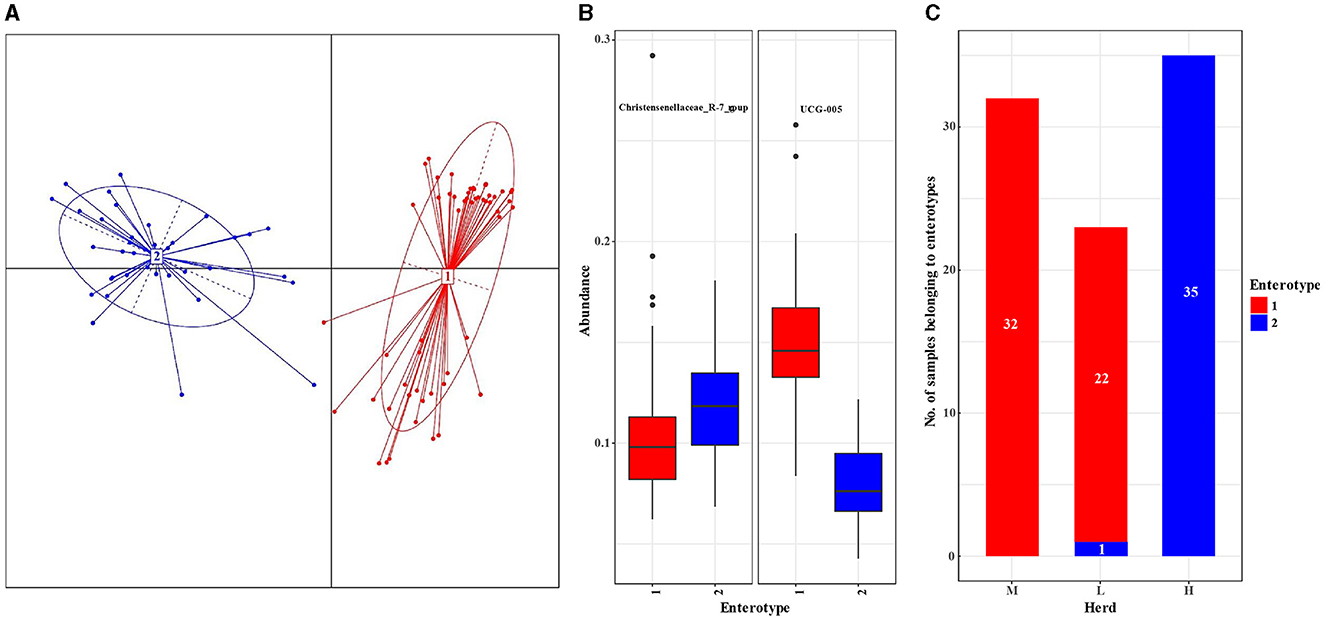
Figure 3. Analysis of enterotypes in the fecal microbiota in small ruminants among different herds. (A) Principal coordinate analysis plot based on the Jensen–Shannon divergence distance at the genus level. (B) Relative abundances of representative genera in each enterotype. (C) Number of samples belonging to the enterotypes of each herd.
The fecal bacterial community functions of enterotypes 1 and 2 were predicted and stratified, and the metabolic pathway abundances were investigated. Non-metric multidimensional scaling (NMDS) based on Bray–Curtis distances was used to depict the clustering of metabolic functional profiles, and two functional clusters (1 and 2) that corresponded to enterotypes 1 and 2 were obtained (ANOSIM R = 0.57, p < 0.001, Figure 4A). A total of 371 MetaCyc metabolic pathways potentially encoded by fecal bacteria were predicted, of which 211 metabolic pathways were differentially represented between clusters 1 and 2, with 92 pathways being enriched in cluster 1 and 119 in cluster 2 [Linear discriminant analysis (LDA) > 2, p < 0.05, Supplementary Table S3]. Moreover, the abundances of the top 20 ranked metabolic pathways belonged mainly to biosynthesis (amino acid biosynthesis, fatty acid and lipid biosynthesis, and nucleoside and nucleotide biosynthesis), while only three metabolic pathways mapped to the generation of precursor metabolites and energy (glycolysis, pentose phosphate, and fermentation pathways) (Figure 4B). Of these, 10 were overrepresented in cluster 2 (Figure 4B).
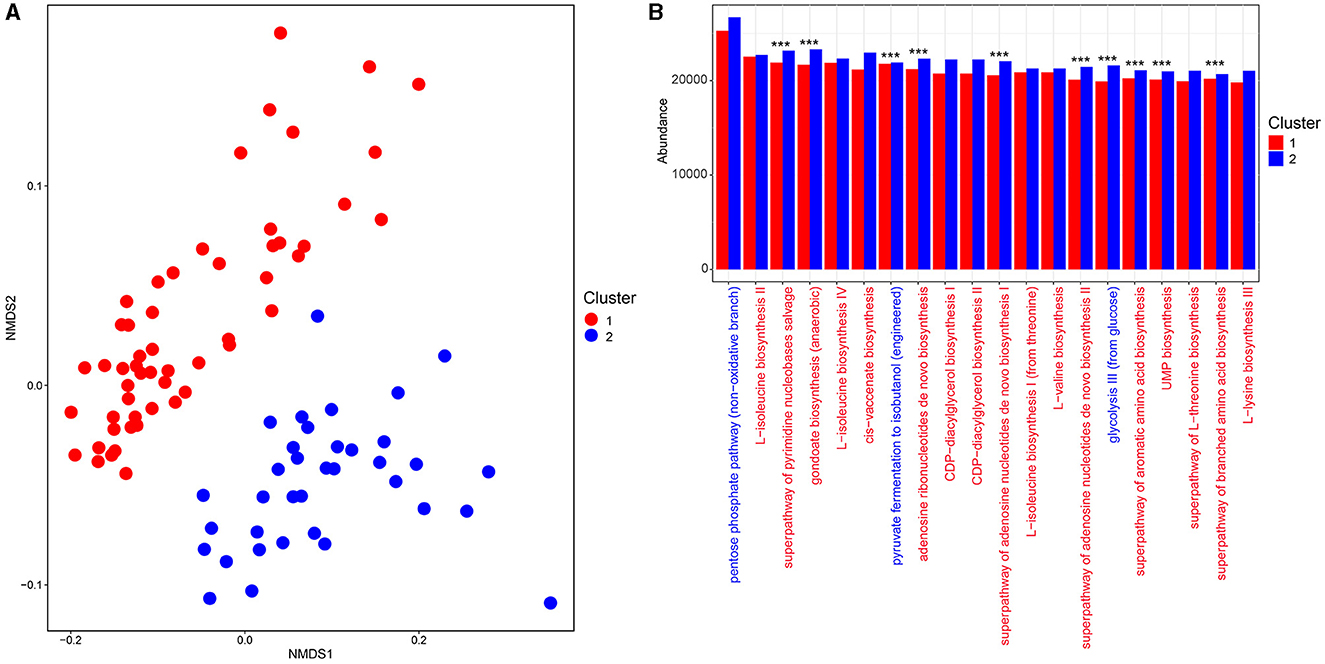
Figure 4. Comparison of functional pathways between enterotype groups. (A) The non-metric multidimensional scaling (NMDS) plot of the functional profile of the fecal bacterial communities between enterotypes 1 and 2 based on the Bray–Curtis distances. (B) The top 20 ranked MetaCyc pathways between enterotypes 1 and 2. The red font represents the MetaCyc pathways belonging to biosynthesis, while the blue font represents the MetaCyc pathways belonging to the generation of precursor metabolites and energy. *** p< 0.001.
Discussion
Small ruminants play an important role in the global food economy, and their fecal microbiota play key roles in host metabolism and productivity performance. However, considering the importance of fecal microbial composition to the host, few studies have been conducted to explore it and its potential influential factors on indigenous small ruminants in the Chinese province of Guizhou. This study provides a comprehensive overview of the fecal bacterial microbial profiles across three local ruminant breeds (two goat breeds and one sheep breed) living in different areas of Guizhou and demonstrates that fecal microbial communities differ according to sex and herd (genetics/altitude). Furthermore, two enterotypes were identified, with enterotype 1 dominated by UCG-005 and enterotype 2 by Christensenellaceae_R-7_group. In addition, the predicted functional profiles of the fecal microbiota were divided into two functional clusters that corresponded to the identified enterotypes.
In the present study, the Shannon diversity was significantly different among ruminant species. This is consistent with previous reports on other ruminant species (O'Donnell et al., 2017; Chang et al., 2020), where the diet varied across species. However, a recent study reported that there was no significant difference in the alpha diversity of fecal microbiota between sheep and goats when they were fed the same diet (Shabana et al., 2021). This discrepancy suggests that diet supersedes host species in shaping the fecal microbiota (Wei et al., 2021). Moreover, the Firmicutes/Bacteroidota ratio varied across species. It was reported that Firmicutes and Bacteroidetes are involved in the digestion of carbohydrates and proteins, and the Firmicutes/Bacteroidota ratio is positively associated with average daily gain (ADG) and the milk fat composition in bovines (Maslen et al., 2023). In addition, host-specific taxa were found in each ruminant species. For instance, Bacteroidales_BS11_gut_group was detected uniquely in the H herd, Succiniclasticum was detected uniquely in the L herd, while Olsenella was detected uniquely in the M herd. Bacteroidales_BS11_gut_group specializes in fermenting hemicellulose monomeric sugars and acetate production (Solden et al., 2017). Succiniclasticum, a type of amylolytic bacteria, possesses the ability to convert succinate to propionate (Zhang et al., 2018). Olsenella could produce lactic acid from glucose fermentation (Gaowa et al., 2021). Thus, we hypothesized that variations in the composition of fecal microbiota among different species may contribute to the differences in the feed utilization of the host since fecal microbiota are host-specific and associated with host phenotypes (Mallott and Amato, 2021; Andrade et al., 2022).
In addition, the Shannon diversity was significantly higher in the L herd (low altitude) and M herd (median altitude) compared to the H herd (high altitude), which is consistent with a previous study on Tibetan humans and pigs (Zeng et al., 2020). However, a recent study on mammals (macaques, humans, and dogs) found that Shannon diversity was higher in high-altitude populations than in their low-altitude counterparts (Zhao et al., 2023), and similar observations were also seen in mice under simulated high-altitude environments (Wang F. et al., 2022) and in Tibetans (Lan et al., 2017). These controversial results indicate that altitude is not the only driver of fecal microbiota alpha diversity since genetic background, life environments, and diet also act as the deterministic drivers in shaping it (Zeng et al., 2020). Recent studies have reported that the profile of fecal microbiota is mainly influenced by altitudes in indigenous animals (Ma et al., 2019) and that the yak fecal microbial community shows a clear clustering of taxa according to altitudes (Liu et al., 2021). Thus, we speculated that variations in altitude may have contributed to the differences in the fecal bacterial community of the small ruminants in the current study. Firmicutes and Bacteroidota were the most abundant phyla in the fecal microbiota across altitudes; this finding is supported by the results of the previous studies on ruminants (Lei et al., 2018; Cui et al., 2019; Liu et al., 2019), highlighting that they are essential components of the fecal microbiota in ruminants. In this study, the Firmicutes/Bacteroidota ratio showed a noticeable downward trend in the H herd (high altitude) compared to the L herd (low altitude) and the M herd (medium altitude). However, multiple studies have consistently indicated that small ruminants living at high altitudes, such as Tibetan antelope, European mouflon, and blue sheep, exhibit a higher Firmicutes/Bacteroidota ratio compared to their low-altitude counterparts (Ma et al., 2019; Sun et al., 2019). Moreover, it has been demonstrated that the Firmicutes/Bacteroidota ratio influences cardiorespiratory fitness (Durk et al., 2019) and is treated as an indicator of gut dysbiosis (Yu et al., 2019). In addition, the Firmicutes/Bacteroidota ratio changed with diet (Zhang et al., 2018b) and was positively associated with energy harvest (Wang F. et al., 2022). Furthermore, the high ratio of Firmicutes to Bacteroidetes was beneficial to the utilization of plant cellulose in pikas (Li et al., 2016). Based on these findings, we postulated that changes in the ratio of Firmicutes to Bacteroidota across altitudes facilitates a high-altitude adaptation of the host (Wang X. et al., 2022), which may be attributed to noticeable differences in dietary habits (Li and Zhao, 2015).
At the genus level, the relative abundances of Romboutsia, Lysinibacillus, Peptostreptococcaceae, Christensenellaceae, Defluviitaleaceae_UCG_011, Candidatus_Saccharimonas, Paenibacillus, and Solibacillus were greater in the H herd (high altitude) than in other herds (low and medium altitudes). The microbes that are overrepresented in the high-altitude area probably perform important functions for the host. For instance, members of Lysinibacillus isolated from the Taihu Lake are iron-reducing strains (Li et al., 2023), and hypoxia signaling links erythropoiesis with iron homeostasis (Renassia and Peyssonnaux, 2019). Furthermore, Paenibacillus spp. can produce a variety of exopolysaccharides (EPSs) that have been proven to have antioxidative properties, and antioxidants play a crucial role in the adaptation of animals to high-altitude environments (Zeng et al., 2017). In addition, Paenibacillus polymyxa 10 could enhance the growth performance of broilers by improving intestinal health (Wang et al., 2021). Christensenellaceae contributes to the host health by modulating lipid metabolism (Shen et al., 2021). Moreover, Solibacillus acts as probiotics against pathogens, such as Aeromonas and Pseudomonas (Ayoola et al., 2023). Taken together, these potential probiotics in the H herd may help animals improve metabolic function and maintain intestinal homeostasis to adapt to high-altitude environments. More research is warranted to substantiate the ecological functions of these bacteria in high-altitude small ruminants. Contrary to the observed potential probiotics, two potential pathogenetic bacteria were also enriched in the H herd. Romboutsia functions as a potential pathobiont in ulcerative colitis (Wang F. et al., 2022), while Defluviitaleaceae_UCG_011 is positively associated with intestinal dysbiosis (Yang et al., 2023). These results suggest that high altitude may increase the number of pathogenic bacteria that induce intestinal dysfunction (Wan et al., 2022) and climate change may lead to wider spreading of pathogens (Rocklöv and Dubrow, 2020). The relative abundances of fiber-degrading bacteria such as Bacillus and Ruminococcus (Pandit et al., 2018) were enriched in the L herd (low altitude) compared to other herds. Ruminococcus converts cellulose, hemicellulose, and other polysaccharides into acetate and succinate (La Reau and Suen, 2018) and is positively associated with fumaric acid concentration that inhibits rumen methane production (Hu et al., 2020). Bacillus produces amino acids, stimulates protein synthesis, and is a major reservoir of lipolytic enzymes (Mukendi et al., 2018). In this study, the relative abundance of UCG_005 was greater in the M herd (medium altitude) than in the other herds. Members of this genus enhance fiber digestion (Yang et al., 2020) and are related to the production of ruminal acetate and total short-chain fatty acids (SCFAs) (Li et al., 2021). In addition, UCG_005 produces butyric acids that enhance the intestinal surface area, improving calcium absorption (Zhou et al., 2023). The differentially abundant profile in fecal bacteria across altitudes suggests that the fecal microbiota follow a divergent pattern in functional adaptation according to the external environment, genetic background, or diet resources (Zhang et al., 2018b).
In the present study, the fecal microbiota composition differed between male and female animals within each herd, which is consistent with the findings of a recent study on fecal microbiota composition in different ruminant species on the Qinghai-Tibetan Plateau that identified sex as one of the key factors influencing the fecal microbiota composition (Wang X. et al., 2022). Sex-related differences in fecal microbiota composition were also observed in Hanwoo cattle (Sim et al., 2022), Chinese forest musk deer (Zhao et al., 2019), and Dromedary camels in Saudi Arabia (Elbir and Alhumam, 2022). In this study, a greater abundance of UCG−010 in male animals and a greater abundance of Streptococcus in female animals in the L herd were observed. UCG−010 is crucial for fiber degradation (mainly in cellulose digestion) and biohydrogenation process of converting dietary polyunsaturated fatty acids (PUFAs) to saturated fatty acids (SFAs) (Guerra et al., 2022). Streptococcus is a key probiotic in ruminant feedings (Kulkarni et al., 2022), and a bacteriocin produced by Streptococcus bovis can reduce methane emissions from ruminants (Lee et al., 2002). In addition, the abundance of Anaerocolumna was higher in female animals than in male animals, whereas Lachnospiraceae was greater in male animals than in female animals in the H herd. Anaerocolumna has the potential to degrade lignin and chitin (Ueki et al., 2019; Xu et al., 2021), while Lachnospiraceae plays an important role in fiber digestion (Yang et al., 2020). These findings suggest that the fecal microbiota community varies with sex, which may be ascribed to different sex hormones (Kim, 2023) and diet preferences between male and female animals (Lee et al., 2017).
The predicted outcomes of the bacterial community structure (enterotype) can be highly dependent on host genetics rather than on altitude and the sex of the collected sample, as demonstrated in the current study. This finding is in contradiction with previous studies on other ruminant species and humans, where the enterotypes were diet-driven (Wu et al., 2011; Hicks et al., 2018; Couch et al., 2021). However, it has been demonstrated that host genetics is an important contributor to the enterotype of individual fecal microbiota in humans and pigs (Lim et al., 2014; Ma et al., 2022), and the gut microbiome is host-specific (Mallott and Amato, 2021). Thus, the division of fecal microbiota into different enterotypes between goats and sheep could be expected. In this study, enterotype 1 and enterotype 2 were characterized by UCG-005 and Christensenellaceae_R-7_group, respectively. Consistent with our observation of the enrichment of biosynthesis pathways (i.e., amino acid biosynthesis and fatty acids) in enterotype 1, species of UCG-005 are associated with acetate and SCFA production and increased intestinal absorption of nutrients (Li et al., 2021; Zhou et al., 2023). In addition, the pathways associated with energy metabolism (glycolysis II from fructose 6-phosphate, glycolysis I from glucose 6-phosphate, and aerobic respiration I cytochrome c) were enriched in enterotype 2. Christensenellaceae_R-7_group plays an important role in degrading carbohydrates and amino acids to acetate and ammonia, with acetic and butyric acids as fermentation end products (Chen et al., 2020), contributing to the enrichment of energy metabolism pathways in enterotype 2. In our study, the pathways associated with energy metabolism were significantly enriched in the H herd (high altitude) compared to the other herds. This finding is supported by a study on Tibetans and Tibetan pigs, where the relative abundances of energy metabolism pathways were significant in high-altitude groups (Zeng et al., 2020). These findings suggest that fecal microbiota helps the host in adapting to high-altitude energy requirements by modulating relevant metabolic functions.
One of the limitations of the current study was the lack of data on blood oxygen levels. It was reported that blood oxygen level is an important factor that affects the intestinal microbial community (Zhang et al., 2018a) via maintaining the balance between aerobic and anaerobic environments in the intestinal epithelium (Bai et al., 2022); thus, data on blood oxygen level should be taken into consideration in future studies. Furthermore, samples from different ruminant species at the same altitude were not collected. Future studies with more and larger-scale samples from different ruminant species at the same altitude (below 1,000 m and above 3,000) are warranted to verify the findings in this study.
In conclusion, this study characterized bacterial fecal microbiota in indigenous small ruminants living in different areas of Guizhou. The microbiota were clustered into two enterotypes led by UCG-005 (E1) and Christensenellaceae_R-7_group (E2), which were highly dependent on host genetics (breeds), although altitude and sex also contributed to the differences in the fecal microbiota community. In addition, the fecal microbiota enterotypes corresponded to the clustering of functional prediction profiles, confirming the existence of these two enterotypes in our animal cohort. These results provide detailed and novel insights into the composition, function, and enterotypes in the fecal microbiota of local sheep and goats in Guizhou, which may help us understand how fecal microbiota contribute to different host phenotypes in indigenous small ruminants and their adaptation mechanism in high-altitude environments.
Data availability statement
The datasets presented in this study can be found in online repositories. The names of the repository/repositories and accession number(s) can be found below: https://www.ncbi.nlm.nih.gov/, PRJNA1006184.
Ethics statement
The animal study was approved by the Animal Ethics Committee of Guizhou University. The study was conducted in accordance with the local legislation and institutional requirements.
Author contributions
WG: Conceptualization, Formal analysis, Writing – original draft, Writing – review & editing. TL: Data curation, Visualization, Writing – review & editing. WW: Formal analysis, Methodology, Writing – review & editing. YY: Methodology, Software, Writing – review & editing. AN: Conceptualization, Supervision, Visualization, Writing – review & editing. MZ: Conceptualization, Supervision, Validation, Visualization, Writing – review & editing. XC: Funding acquisition, Project administration, Writing – review & editing.
Funding
The author(s) declare financial support was received for the research, authorship, and/or publication of this article. This work was supported by the National Natural Science Foundation of China (NSFC, No. 32260835), the Science and Technology Project of Guizhou Province (Qian Kehe Foundation-ZK [2022] Key 033), and the Guizhou High Level Innovative Talents Project (Qian Kehe Platform Talents [2022] 021-1).
Acknowledgments
We would like to thank Compute Canada (http://www.computecanada.ca) for computing resources and guidance during data analysis.
Conflict of interest
The authors declare that the research was conducted in the absence of any commercial or financial relationships that could be construed as a potential conflict of interest.
Publisher's note
All claims expressed in this article are solely those of the authors and do not necessarily represent those of their affiliated organizations, or those of the publisher, the editors and the reviewers. Any product that may be evaluated in this article, or claim that may be made by its manufacturer, is not guaranteed or endorsed by the publisher.
Supplementary material
The Supplementary Material for this article can be found online at: https://www.frontiersin.org/articles/10.3389/fmicb.2024.1415230/full#supplementary-material
References
Anderson, M. J. (2014). “Permutational multivariate analysis of variance (PERMANOVA),” in Wiley StatsRef: Statistics Reference Online, 1–15.
Andrade, B. G. N., Bressani, F. A., Cuadrat, R. R. C., Cardoso, T. F., Malheiros, J. M., de Oliveira, P. S. N., et al. (2022). Stool and ruminal microbiome components associated with methane emission and feed efficiency in nelore beef cattle. Front. Genet. 13:812828. doi: 10.3389/fgene.2022.812828
Ayoola, M. B., Pillai, N., Nanduri, B. Jr, M. J. R., and Ramkumar, M. (2023). Predicting foodborne pathogens and probiotics taxa within poultry-related microbiomes using a machine learning approach. Animal Micr. 57, 1–27. doi: 10.1186/s42523-023-00260-w
Bai, X., Liu, G., Yang, J., Zhu, J., Wang, Q., Zhou, Y., et al. (2022). Changes in the gut microbiota of rats in high-altitude hypoxic environments. Microbiol. Spectr. 10, e01626–e01622. doi: 10.1128/spectrum.01626-22
Bolyen, E., Rideout, J. R., Dillon, M. R., Bokulich, N. A., Abnet, C. C., Al-Ghalith, G. A., et al. (2019). Reproducible, interactive, scalable and extensible microbiome data science using QIIME 2. Nat. Biotechnol. 37, 852–857. doi: 10.1038/s41587-019-0209-9
Caicedo, H. H., Hashimoto, D. A., Caicedo, J. C., Pentland, A., and Pisano, G. P. (2020). Overcoming barriers to early disease intervention. Nat. Biotechnol. 38, 669–673. doi: 10.1038/s41587-020-0550-z
Callahan, B. J., McMurdie, P. J., Rosen, M. J., Han, A. W., Johnson, A. J. A., and Holmes, S. P. (2016). DADA2: High-resolution sample inference from Illumina amplicon data. Nat. Methods 13, 581–583. doi: 10.1038/nmeth.3869
Chang, J., Yao, X., Zuo, C., Qi, Y., Chen, D., and Ma, W. (2020). The gut bacterial diversity of sheep associated with different breeds in Qinghai province. BMC Vet. Res. 16, 1–9. doi: 10.1186/s12917-020-02477-2
Chen, R., Li, Z., Feng, J., Zhao, L., and Yu, J. (2020). Effects of digestate recirculation ratios on biogas production and methane yield of continuous dry anaerobic digestion. Bioresour. Technol. 316:123963. doi: 10.1016/j.biortech.2020.123963
Couch, C. E., Stagaman, K., Spaan, R. S., Combrink, H. J., Sharpton, T. J., Beechler, B. R., et al. (2021). Diet and gut microbiome enterotype are associated at the population level in African buffalo. Nat. Commun. 12:2267. doi: 10.1038/s41467-021-22510-8
Cui, X., Wang, Z., Yan, T., Chang, S., Wang, H., and Hou, F. (2019). Rumen bacterial diversity of Tibetan sheep (Ovis aries) associated with different forage types on the Qinghai-Tibetan Plateau. Can. J. Microbiol. 65, 859–869. doi: 10.1139/cjm-2019-0154
Durk, R. P., Castillo, E., Márquez-Magaña, L., Grosicki, G. J., Bolter, N. D., Matthew Lee, C., et al. (2019). Gut microbiota composition is related to cardiorespiratory fitness in healthy young adults. Int. J. Sport Nutr. Exerc. Metab. 29, 249–253. doi: 10.1123/ijsnem.2018-0024
Elbir, H., and Alhumam, N. A. (2022). Sex differences in fecal microbiome composition and function of dromedary camels in Saudi Arabia. Animals 12:3430. doi: 10.3390/ani12233430
Fu, K., Chen, X., Guo, W., Zhou, Z., Zhang, Y., Ji, T., et al. (2022). Effects of N acetylcysteine on the expression of genes associated with reproductive performance in the goat uterus during early gestation. Animals 12:2431. doi: 10.3390/ani12182431
Gaowa, N., Li, W., Gelsinger, S., Murphy, B., and Li, S. (2021). Analysis of host jejunum transcriptome and associated microbial community structure variation in young calves with feed-induced acidosis. Metabolites 11:414. doi: 10.3390/metabo11070414
Guerra, V., Tiago, I., Aires, A., Coelho, C., Nunes, J., Martins, L. O., et al. (2022). The gastrointestinal microbiome of browsing goats (Capra hircus). PLoS ONE 17:e0276262. doi: 10.1371/journal.pone.0276262
Guo, J., Li, P., Zhang, K., Zhang, L., Wang, X., Li, L., et al. (2020). Distinct stage changes in early-life colonization and acquisition of the gut microbiota and its correlations with volatile fatty acids in goat kids. Front. Microbiol. 11:584742. doi: 10.3389/fmicb.2020.584742
Guo, X., Sha, Y., Lv, W., Pu, X., Liu, X., Luo, Y., et al. (2022). Sex differences in rumen fermentation and microbiota of Tibetan goat. Microbl. Cell Fact 21, 1–12. doi: 10.1186/s12934-022-01783-8
Han, L., Xue, W., Cao, H., Chen, X., Qi, F., Ma, T., et al. (2022). Comparison of rumen fermentation parameters and microbiota of yaks from different altitude regions in tibet, China. Front. Microbiol. 12:807512. doi: 10.3389/fmicb.2021.807512
Hicks, A. L., Lee, K. J., Couto-Rodriguez, M., Patel, J., Sinha, R., Guo, C., et al. (2018). Gut microbiomes of wild great apes fluctuate seasonally in response to diet. Nat. Commun. 9:1786. doi: 10.1038/s41467-018-04204-w
Hu, Y., He, Y., Gao, S., Liao, Z., Lai, T., Zhou, H., et al. (2020). The effect of a diet based on rice straw co-fermented with probiotics and enzymes versus a fresh corn Stover-based diet on the rumen bacterial community and metabolites of beef cattle. Sci. Rep. 10:10721. doi: 10.1038/s41598-020-67716-w
Huang, K., Wang, Y., Bai, Y., Luo, Q., Lin, X., Yang, Q., et al. (2022). Gut microbiota and metabolites in atrial fibrillation patients and their changes after catheter ablation. Microbiol. Spectr. 10, e01077–e01021. doi: 10.1128/spectrum.01077-21
Kim, H. S., Whon, T. W., Sung, H., Jeong, Y. S., Jung, E. S., Shin, N. R., et al. (2021). Longitudinal evaluation of fecal microbiota transplantation for ameliorating calf diarrhea and improving growth performance. Nat. Commun. 12, 1–16. doi: 10.1038/s41467-020-20389-5
Kim, M. (2023). Assessment of the gastrointestinal microbiota using 16S ribosomal RNA gene amplicon sequencing in ruminant nutrition. Anim. Biosci. 36, 364–373. doi: 10.5713/ab.22.0382
Kulkarni, N. A., Chethan, H. S., Srivastava, R., and Gabbur, A. B. (2022). Role of probiotics in ruminant nutrition as natural modulators of health and productivity of animals in tropical countries: an overview. Trop. Anim. Health Prod. 54:110. doi: 10.1007/s11250-022-03112-y
La Reau, A. J., and Suen, G. (2018). The Ruminococci: key symbionts of the gut ecosystem. J. Microbiol. 56, 199–208. doi: 10.1007/s12275-018-8024-4
Lan, D., Ji, W., Lin, B., Chen, Y., Huang, C., Xiong, X., et al. (2017). Correlations between gut microbiota community structures of Tibetans and geography. Sci. Rep. 7:16982. doi: 10.1038/s41598-017-17194-4
Lee, J. R., Muckerman, J. E., Wright, A. M., Davis, D. J., Childs, T. E., Gillespie, C. E., et al. (2017). Sex determines effect of physical activity on diet preference: association of striatal opioids and gut microbiota composition. Behav. Brain Res. 334, 16–25. doi: 10.1016/j.bbr.2017.07.018
Lee, S. S., Hsu, J. T., Mantovani, H. C., and Russell, J. B. (2002). The effect of bovicin HC5, a bacteriocin from Streptococcus bovis HC5, on ruminal methane production in vitro1. FEMS Microbiol. Lett. 217, 51–55. doi: 10.1111/j.1574-6968.2002.tb11455.x
Lei, Y., Zhang, K., Guo, M., Li, G., Li, C., Li, B., et al. (2018). Exploring the spatial-temporal microbiota of compound stomachs in a pre-weaned goat model. Front. Microbiol. 9:01846. doi: 10.3389/fmicb.2018.01846
Li, H., Li, T., Beasley, D. A. E., Heděnec, P., Xiao, Z., Zhang, S., et al. (2016). Diet diversity is associated with beta but not alpha diversity of pika gut microbiota. Front. Microbiol. 7:01169. doi: 10.3389/fmicb.2016.01169
Li, H., Ma, L., Li, Z., Yin, J., Tan, B., Chen, J., et al. (2021). Evolution of the gut microbiota and its fermentation characteristics of ningxiang pigs at the young stage. Animals 11:638. doi: 10.3390/ani11030638
Li, L., and Zhao, X. (2015). Comparative analyses of fecal microbiota in Tibetan and Chinese Han living at low or high altitude by barcoded 454 pyrosequencing. Sci. Rep. 5:14682. doi: 10.1038/srep14682
Li, Y., Liu, H., Ye, D., Jiang, Q., Cui, X., Li, J., et al. (2023). Novel dissimilatory fermentative iron-reducing strains isolated from the sediments of taihu lake. Available at SSRN:4333573. doi: 10.2139/ssrn.4333573
Lim, M. Y., Rho, M., Song, Y. M., Lee, K., Sung, J., and Ko, G. (2014). Stability of gut enterotypes in Korean monozygotic twins and their association with biomarkers and diet. Sci. Rep. 4:7348. doi: 10.1038/srep07348
Liu, C., Wu, H., Liu, S., Chai, S., Meng, Q., and Zhou, Z. (2019). Dynamic Alterations in Yak Rumen Bacteria Community and Metabolome Characteristics in Response to Feed Type. Front. Microbiol. 10:01116. doi: 10.3389/fmicb.2019.01116
Liu, W., Wang, Q., Song, J., Xin, J., Zhang, S., Lei, Y., et al. (2021). Comparison of gut microbiota of yaks from different geographical regions. Front. Microbiol. 12:666940. doi: 10.3389/fmicb.2021.666940
Lopes, D. R. G., La Reau, A. J., De Souza Duarte, M., Detmann, E., Bento, C. B. P., Mercadante, M. E. Z., et al. (2019). The bacterial and fungal microbiota of nelore steers is dynamic across the gastrointestinal tract and its fecal-associated microbiota is correlated to feed efficiency. Front. Microbiol. 10:01263. doi: 10.3389/fmicb.2019.01263
Love, M. I., Huber, W., and Anders, S. (2014). Moderated estimation of fold change and dispersion for RNA-seq data with DESeq2. Genome Biol. 15, 1–21. doi: 10.1186/s13059-014-0550-8
Ma, N., Sun, Y., Chen, J., Qi, Z., Liu, C., and Ma, X. (2022). Micro-coevolution of genetics rather than diet with enterotype in pigs. Front Nutr 9:846974. doi: 10.3389/fnut.2022.846974
Ma, Y., Ma, S., Chang, L., Wang, H., Ga, Q., Ma, L., et al. (2019). Gut microbiota adaptation to high altitude in indigenous animals. Biochem. Biophys. Res. Commun. 516, 120–126. doi: 10.1016/j.bbrc.2019.05.085
Mahayri, T. M., Mattiello, S., and Celozzi, S. (2022). Host species affects bacterial evenness, but not diversity : comparison of fecal bacteria of cows and goats offered the same diet. Animals 12:2011. doi: 10.3390/ani12162011
Mallott, E. K., and Amato, K. R. (2021). Host specificity of the gut microbiome. Nat Rev Microbiol 19, 639-653. doi: 10.1038/s41579-021-00562-3
Mao, S., Zhang, M., Liu, J., and Zhu, W. (2015). Characterising the bacterial microbiota across the gastrointestinal tracts of dairy cattle: membership and potential function. Sci. Rep. 5:16116. doi: 10.1038/srep16116
Maslen, B. N., Duff, C., Clark, S. A., Van der Werf, J., White, J. D., and Pant, S. D. (2023). Increased yearling weight gain is associated with a distinct faecal microbial profile. Animals 13:3062. doi: 10.3390/ani13193062
Mobeen, F., Sharma, V., and Prakash, T. (2018). Enterotype variations of the healthy human gut microbiome in different geographical regions. Bioinformation 14, 560–573. doi: 10.6026/97320630014560
Moeller, A. H., and Sanders, J. G. (2020). Roles of the gut microbiota in the adaptive evolution of mammalian species. Philos. Trans. R. Soc. B. 375, 1–10. doi: 10.1098/rstb.2019.0597
Mukendi, G. M., Mitema, A., Nelson, K., and Feto, N. A. (2018). “Bacillus species of ruminant origin as a major potential sources of diverse lipolytic enzymes for industrial and therapeutic applications,” in Bacilli in Agrobiotechnology: Plant Stress Tolerance, Bioremediation, and Bioprospecting (Cham: Springer International Publishing), 255–283. doi: 10.1007/978-3-030-85465-2_12
O'Donnell, M. M., Harris, H. M. B., Ross, R. P., and O'Toole, P. W. (2017). Core fecal microbiota of domesticated herbivorous ruminant, hindgut fermenters, and monogastric animals. Microbiology Open 6:e00509. doi: 10.1002/mbo3.509
Pandit, R. J., Hinsu, A. T., Patel, S. H., Jakhesara, S. J., Koringa, P. G., Bruno, F., et al. (2018). Microbiota composition, gene pool and its expression in Gir cattle (Bos indicus) rumen under different forage diets using metagenomic and metatranscriptomic approaches. Syst. Appl. Microbiol. 41, 374–385. doi: 10.1016/j.syapm.2018.02.002
Renassia, C., and Peyssonnaux, C. (2019). New insights into the links between hypoxia and iron homeostasis. Curr. Opin. Hematol. 26, 125–130. doi: 10.1097/MOH.0000000000000494
Rocklöv, J., and Dubrow, R. (2020). Climate change: an enduring challenge for vector-borne disease prevention and control. Nat. Immunol. 21, 479–483. doi: 10.1038/s41590-020-0648-y
Segata, N., Izard, J., Waldron, L., Gevers, D., Miropolsky, L., Garrett, W. S., et al. (2011). Metagenomic biomarker discovery and explanation. Genome Biol. 12, 1–18. doi: 10.1186/gb-2011-12-6-r60
Shabana, I. I., Albakri, N. N., and Bouqellah, N. A. (2021). Metagenomic investigation of faecal microbiota in sheep and goats of the same ages. J. Taibah Univ. Sci. 15, 1–9. doi: 10.1080/16583655.2020.1864930
Shen, T., Yue, Y., He, T., Huang, C., Qu, B., Lv, W., et al. (2021). The association between the gut microbiota and Parkinson's disease, a meta-analysis. Front. Aging Neurosci. 13:636545. doi: 10.3389/fnagi.2021.636545
Sim, S., Lee, H., Yoon, S., Seon, H., Park, C., and Kim, M. (2022). The impact of different diets and genders on fecal microbiota in Hanwoo cattle. J. Anim. Sci. Technol. 64, 897–910. doi: 10.5187/jast.2022.e71
Solden, L. M., Hoyt, D. W., Collins, W. B., Plank, J. E., Daly, R. A., Hildebrand, E., et al. (2017). New roles in hemicellulosic sugar fermentation for the uncultivated Bacteroidetes family BS11. ISME J. 11, 691–703. doi: 10.1038/ismej.2016.150
Sun, G., Zhang, H., Wei, Q., Zhao, C., Yang, X., Wu, X., et al. (2019). Comparative analyses of fecal microbiota in European mouflon (Ovis orientalis musimon) and blue sheep (Pseudois nayaur) living at low or high altitudes. Front. Microbiol. 10:1735. doi: 10.3389/fmicb.2019.01735
Tian, X., Wang, X., Li, J., Luo, Q., Ban, C., and Lu, Q. (2022). The effects of selenium on rumen fermentation parameters and microbial metagenome in goats. Fermentation 8:240. doi: 10.3390/fermentation8050240
Tian, X. Z., Li, J. X., Luo, Q. Y., Zhou, D., Long, Q. M., Wang, X., et al. (2021). Effects of purple corn anthocyanin on blood biochemical indexes, ruminal fluid fermentation, and rumen microbiota in goats. Front. Vet. Sci. 8:715710. doi: 10.3389/fvets.2021.715710
Ueki, A., Tonouchi, A., Kaku, N., and Ueki, K. (2019). Anaerocolumna chitinilytica sp. nov., a chitin-decomposing anaerobic bacterium isolated from anoxic soil subjected to biological soil disinfestation. Int. J. Syst. Evol. Microbiol. 71:004999. doi: 10.1099/ijsem.0.004761
Virgínio Júnior, G. F., and Bittar, C. M. M. H. (2021). Microbial colonization of the gastrointestinal tract of dairy calves - A review of its importance and relationship to health and performance. Anim. Health Res. Rev. 22, 97–108. doi: 10.1017/S1466252321000062
Wan, Z., Zhang, X., Jia, X., Qin, Y., Sun, N., Xin, J., et al. (2022). Lactobacillus johnsonii YH1136 plays a protective role against endogenous pathogenic bacteria induced intestinal dysfunction by reconstructing gut microbiota in mice exposed at high altitude. Front. Immunol. 13:1007737. doi: 10.3389/fimmu.2022.1007737
Wang, B., Gong, L., Zhou, Y., Tang, L., Zeng, Z., Wang, Q., et al. (2021). Probiotic Paenibacillus polymyxa 10 and Lactobacillus plantarum 16 enhance growth performance of broilers by improving the intestinal health. Anim. Nutr. 7, 829–840. doi: 10.1016/j.aninu.2021.03.008
Wang, D., Tang, G., Zhao, L., Wang, M., Chen, L., Zhao, C., et al. (2023). Potential roles of the rectum keystone microbiota in modulating the microbial community and growth performance in goat model. J. Anim. Sci. Biotechnol. 14, 1–15. doi: 10.1186/s40104-023-00850-3
Wang, F., Zhang, H., Xu, T., Hu, Y., and Jiang, Y. (2022). Acute exposure to simulated high-altitude hypoxia alters gut microbiota in mice. Arch. Microbiol. 204, 1–7. doi: 10.1007/s00203-022-03031-4
Wang, X., Zhang, Z., Li, B., Hao, W., Yin, W., Ai, S., et al. (2022). Depicting fecal microbiota characteristic in yak, cattle, yak- cattle hybrid and tibetan sheep in different eco-regions of qinghai-tibetan plateau. Microbiol. Spectr. 10, e00021–22. doi: 10.1128/spectrum.00021-22
Wei, X., Dong, Z., Cheng, F., Shi, H., Zhou, X., Li, B., et al. (2021). Seasonal diets supersede host species in shaping the distal gut microbiota of Yaks and Tibetan sheep. Sci. Rep. 11:22626. doi: 10.1038/s41598-021-99351-4
Wu, D., Vinitchaikul, P., Deng, M., Zhang, G., Sun, L., Gou, X., et al. (2020). Host and altitude factors affect rumen bacteria in cattle. Braz. J. Microbiol. 51, 1573–1583. doi: 10.1007/s42770-020-00380-4
Wu, G. D., Chen, J., Hoffmann, C., Bittinger, K., Chen, Y., Keilbaugh, S. A., et al. (2011). Linking long-term dietary patterns with gut microbial enterotypes. Science 334, 105–109. doi: 10.1126/science.1208344
Xu, C., Su, X., Wang, J., Zhang, F., Shen, G., Yuan, Y., et al. (2021). Characteristics and functional bacteria in a microbial consortium for rice straw lignin-degrading. Bioresour. Technol. 331:125066. doi: 10.1016/j.biortech.2021.125066
Yang, C., Tsedan, G., Liu, Y., and Hou, F. (2020). Shrub coverage alters the rumen bacterial community of yaks (Bos grunniens) grazing in alpine meadows. J. Anim. Sci. Technol. 62, 504–520. doi: 10.5187/jast.2020.62.4.504
Yang, J., Lee, R., Schulz, Z., Hsu, A., Pai, J., Yang, S., et al. (2023). Mixed nuts as healthy snacks: effect on tryptophan metabolism and cardiovascular risk factors. Nutrients 15:569. doi: 10.3390/nu15030569
Yu, X., Zhang, X., Jin, H., Wu, Z., Yan, C., Liu, Z., et al. (2019). Zhengganxifeng decoction affects gut microbiota and reduces blood pressure via renin-angiotensin system. Biol. Pharm. Bull. 42, 1482–1490. doi: 10.1248/bpb.b19-00057
Zeng, B., Zhang, S., Xu, H., Kong, F., Yu, X., Wang, P., et al. (2020). Gut microbiota of Tibetans and Tibetan pigs varies between high and low altitude environments. Microbiol. Res. 235:126447. doi: 10.1016/j.micres.2020.126447
Zeng, B., Zhao, J., Guo, W., Zhang, S., Hua, Y., Tang, J., et al. (2017). High-altitude living shapes the skin microbiome in humans and pigs. Front. Microbiol. 8:1929. doi: 10.3389/fmicb.2017.01929
Zhang, H., Shao, M., Huang, H., Wang, S., Ma, L., Wang, H., et al. (2018). The dynamic distribution of small-tail Han sheep microbiota across different intestinal segments. Front. Microbiol. 9:32. doi: 10.3389/fmicb.2018.00032
Zhang, W., Jiao, L., Liu, R., Zhang, Y., Ji, Q., Zhang, H., et al. (2018a). The effect of exposure to high altitude and low oxygen on intestinal microbial communities in mice. PLoS ONE 13:e0203701. doi: 10.1371/journal.pone.0203701
Zhang, W., Li, N., Tang, X., Liu, N., and Zhao, W. (2018b). Changes in intestinal microbiota across an altitudinal gradient in the lizard Phrynocephalus vlangalii. Ecol. Evol. 8, 4695–4703. doi: 10.1002/ece3.4029
Zhang, X., Wang, W., Cao, Z., Yang, H., Wang, Y., and Li, S. (2023). Effects of altitude on the gut microbiome and metabolomics of Sanhe heifers. Front. Microbiol. 14:1076011. doi: 10.3389/fmicb.2023.1076011
Zhao, G., Ma, T., Tang, W., Li, D., Mishra, S. K., Xu, Z., et al. (2019). Gut microbiome of Chinese forest musk deer examined across gender and age. Biomed Res. Int. 2019:9291216. doi: 10.1155/2019/9291216
Zhao, J., Yao, Y., Dong, M., Xiao, H., Xiong, Y., Yang, S., et al. (2023). Diet and high altitude strongly drive convergent adaptation of gut microbiota in wild macaques, humans, and dogs to high altitude environments. Front. Microbiol. 14:1067240. doi: 10.3389/fmicb.2023.1067240
Zhou, J., Cheng, J., Liu, L., Luo, J., and Peng, X. (2023). Lactobacillus acidophilus (LA) fermenting astragalus polysaccharides (APS) improves calcium absorption and osteoporosis by altering gut microbiota. Foods 12:275. doi: 10.3390/foods12020275
Zhou, W., Wu, X., Su, C., Li, L., Xu, L., Akhtar, R. W., et al. (2022). Exploring the rumen microbial community in Guizhou White goats at different ages. Rev. Bras. Zootec. 51:e20210070. doi: 10.37496/rbz5120210070
Keywords: fecal microbiota, goat, sheep, enterotypes, Guizhou
Citation: Guo W, Liu T, Wang W, Yu Y, Neves ALA, Zhou M and Chen X (2024) Survey of the fecal microbiota of indigenous small ruminants living in different areas of Guizhou. Front. Microbiol. 15:1415230. doi: 10.3389/fmicb.2024.1415230
Received: 10 April 2024; Accepted: 22 July 2024;
Published: 08 August 2024.
Edited by:
Einar Vargas-Bello-Pérez, University of Reading, United KingdomReviewed by:
Zhiyuan He, Beijing University of Agriculture, ChinaYuxin Yang, Northwest A&F University, China
Copyright © 2024 Guo, Liu, Wang, Yu, Neves, Zhou and Chen. This is an open-access article distributed under the terms of the Creative Commons Attribution License (CC BY). The use, distribution or reproduction in other forums is permitted, provided the original author(s) and the copyright owner(s) are credited and that the original publication in this journal is cited, in accordance with accepted academic practice. No use, distribution or reproduction is permitted which does not comply with these terms.
*Correspondence: Mi Zhou, bXpob3UyQHVhbGJlcnRhLmNh; Xiang Chen, eGNoZW4yQGd6dS5lZHUuY24=