- 1Guangdong Provincial Key Laboratory of Plant Molecular Breeding, South China Agricultural University, Guangzhou, China
- 2State Key Laboratory of Agrobiotechnology, College of Biological Sciences, China Agricultural University, Beijing, China
- 3Institute of Environmental Science and Technology, College of Environment and Resource Science, Zhejiang University, Hangzhou, China
- 4College of Agronomy and Life Sciences, Yunnan Urban Agricultural Engineering and Technological Research Center, Kunming University, Kunming, China
Introduction: Understanding patterns and processes of microbial biogeography in soils is important for monitoring ecological responses to human activities, particularly in ecologically vulnerable areas such as the Qinghai-Xizang Plateau. Highland barley is the staple food of local people and has mainly been cultivated along the Yarlung Zangbo River valley in Xizang.
Methods: Here we investigated soil bacterial communities from 33 sampling sites of highland barley farmland in this region and compared them to those from wild ecosystems including alpine tundra, meadow, forest, and swamp. Additionally, the effects of environmental factors on bacterial communities, as well as the relative importance of stochastic and deterministic processes in shaping the beta diversity of soil bacterial communities in alpine ecosystems were assessed.
Results: In contrast to soils of wild ecosystems, these farmland samples harbored a highly homogeneous bacterial community without significant correlations with geographic, elevation, and edaphic distances. Discriminant bacterial taxa identified for farmland samples belong to Acidobacteria, with Acidobacteria Gp4 as the dominant clade. Although Acidobacteria were the most abundant members in all ecosystems, characterized bacterial taxa of meadow and forest were members of other phyla such as Proteobacteria and Verrucomicrobia. pH and organic matter were major edaphic attributes shaping these observed patterns across ecosystems. Null model analyses revealed that the deterministic assembly was dominant in bacterial communities in highland barley farmland and tundra soils, whereas stochastic assembly also contributed a large fraction to the assembly of bacterial communities in forest, meadow and swamp soils.
Discussion: These findings provide an insight into the consequences of human activities and agricultural intensification on taxonomic homogenization of soil bacterial communities in the Qinghai-Xizang Plateau.
1 Introduction
Microbial biogeography investigates the geographical variation of microorganisms across space and time, and uncovers the processes that cause this variation in microbial assemblage (Hanson et al., 2012; Xu et al., 2020; Wan et al., 2023). With the revolutionary advance in molecular phylogenetic approaches, sequencing techniques and computational methods in recent years (Kozich et al., 2013; Larin et al., 2024), extensive studies have successfully established the biogeographic patterns of bacteria across a broad spectrum of ecosystems (Nemergut et al., 2013). Now the major theme of this field has focused on identifying the mechanisms that shape these patterns (Hanson et al., 2012; Nemergut et al., 2013; Ruff et al., 2015; Cao et al., 2016; Kumar et al., 2017).
It has been acknowledged that edaphic variables, such as pH (Fierer and Jackson, 2006; Cao et al., 2016), salinity (Feng et al., 2023), and carbon availability (Fierer et al., 2007; Walsh et al., 2016), have a pivotal role in determining microbial biogeography. For example, it has been reported that the relative abundance of Acidobacteria, ubiquitous and abundant members of soil bacterial communities (Kielak et al., 2016; Delgado-Baquerizo et al., 2018), is negatively correlated with pH (Wang et al., 2023). These findings support a classic deterministic process of selection via abiotic features in determining microbial community assemblage. However, variation of microbial communities can also be affected by stochastic processes such as dispersal (Hawkins and Zeglin, 2022), evolutionary diversification (Shapiro et al., 2012), and ecological drift (Jones et al., 2023). Given the irreplaceable fundamental functions of microorganisms in biosphere, understanding both patterns and processes of microbial biogeography is not only helpful for us to investigate forces generating biodiversity, but also crucial for monitoring ecological responses to environmental changes caused by human activities.
Highland barley is the staple food for local people and an important livestock feed in the Qinghai-Xizang Plateau, which is one of the domestication centers for cultivated barley (Zeng et al., 2015). To improve the sustainable development of highland barley farmland in the ecologically vulnerable Qinghai-Xizang Plateau, it is important to know the characteristics of soil bacterial communities in the field. To this end, we firstly determined the beta diversity of soil bacterial communities in the highland barley farmland across 33 sampling sites along the Yarlung Zangbo River valley in the Qinghai-Xizang Plateau. The identified biogeographical pattern of soil bacterial communities and processes shaping it were compared to those of 33 soil samples in wild ecosystems (including tundra, swamp, meadow and forest), which are of different levels of ecological fragility.
2 Materials and methods
2.1 Sampling, DNA extraction, soil physicochemical properties characterization
Highland barley farmland soils were collected from 33 sampling sites in Xizang, China, with 12 samples collected along the Nyang Qu River (an upper tributary of Yarlung Zangbo River), 12 samples from the downstream of the Yarlung Zangbo River (where the Lhasa River joined), and nine samples from the midstream of the Yarlung Zangbo River (Supplementary Table S1 and Figure 1). Non-farmland soils were collected from 30 sites across eight alpine mountains (Mt. Bangla, Mt. Gangbala, Mt. Kaluola, Mt. Luobadui, Mt. Mila, Mt. Sejila, Mt. Pading and Mt. Zhongla) in the same region (with fifteen alpine meadow sites, nine alpine tundra sites, and six high-frigid forest sites), and three sites in Aralake swamp, a dry lakebed located in the Everest Wetland Nature Reserve (Supplementary Table S1 and Figure 1). Samples were collected during the growing season when the barley starts sprouting and the alpine plants flourishing and flowering. At each site, three areas (0.5 m x 0.5 m) were probed, with dominant vegetation recorded in situ. Soil samples (0–15 cm) from three areas were randomly collected and mixed as a single sample for each sampling site. Soil samples were stored in ice bags, transported to laboratory and preserved at −80°C before DNA extraction. Total DNA of soil samples were extracted using the Fast DNA™ SPIN Kit (MP biomedicals, Cleveland, OH, United States). The quality and quantity of DNA were checked with a NanoDrop device (ND-1000, Thermo Fisher, United States). Moreover, total N, available phosphorus (P), organic matter (OM), available potassium (K), electrical conductivity (EC), pH, and ion concentrations of Ca2+, Mg2+, Na+, Cl−, K+, HCO3−, and SO42− for air-dried soil samples were determined at the Plant Nutrient and Resource Research Institution, Beijing Academy of Agriculture and Forestry Sciences, China (Supplementary Table S1), using the methods described before (Wang et al., 2018). Non-parametric pairwise comparison (Dunn’s Post-Hoc test) between the 5 alpine ecotypes showed no significant difference based on the Euclidean distances of geographic (Supplementary Figure S1A) and elevation (Supplementary Figure S1B) characteristics, however, the edaphic distances of farmland are significantly lower than that of meadow and tundra (Supplementary Figure S1C, p-values = 9.85e−04 and 0.02, respectively). Climate data such as mean annual temperature (AMT) and annual precipitation (AP) of the sampling region were acquired from DIVA-GIS1 at the 2.5 arc-minutes resolution level. AMT and AP were then predicted and cross validated with linear model using latitude, longitude and altitude as explanatory variables in R before interpolating into sampling sites (Supplementary Table S1).
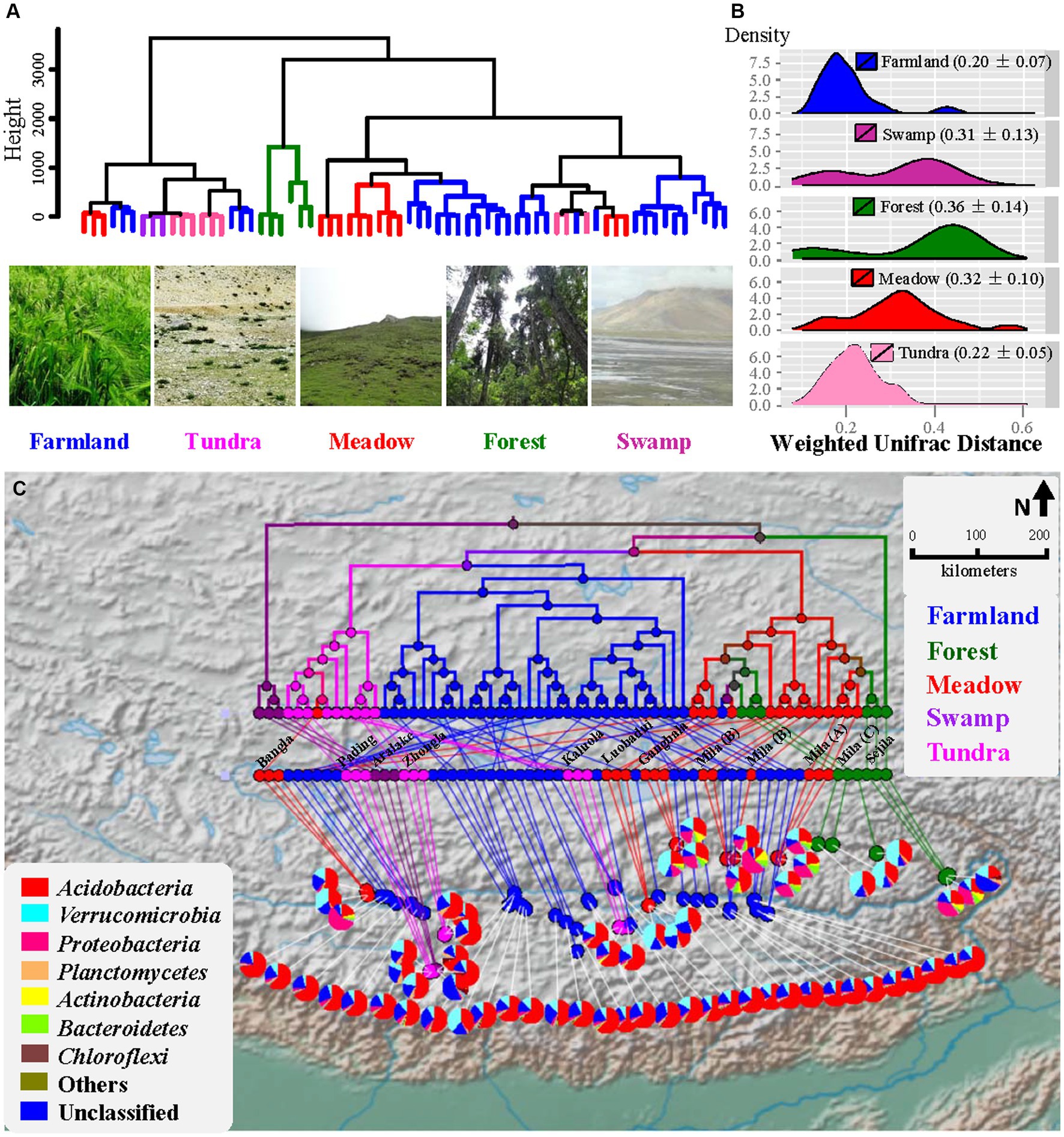
Figure 1. Beta diversity analysis of bacterial community. (A) UPGMA dendrogram based on geographic distance. Photos show plants of Xizang Highland barley, and the landscape of alpine tundra and meadow. (B) Density plot showing the distribution of weighted UniFrac distance (mean ± SD). (C) Geospatial biodiversity mapping analysis comparing bacterial communities across Xizang biomes using weighted UniFrac distance. Branches are colored based on the ecosystems they represent. Pie charts represent relative abundance of bacteria taxa at phylum level for each site. Geographic origins of soil samples from wild ecosystems are shown.
2.2 Library construction for 16S rRNA gene sequencing
PCR amplifications were performed on 66 DNA samples using the 515F/806R primer set (515F: GTGYCAGCMGCCGCGGTAA and 806R: GGACTACNVGGGTWTCTAAT), which targets the V4 region of the 16S rRNA gene (Caporaso et al., 2012). The PCR products with P5/P7 adapters and double indexes were purified with double volume of AMPure XP beads, and fluorescence quantified (Qubit 2.0 Fluorometer, ThermoFisher Scientific, United States) with Qubit dsDNA HS assay kit (Invitrogen, United States). Multiple samples were pooled together in equimolar concentrations. Then 10 ng of pooled PCR products were sequenced on the Illumina MiSeq platform (paired-end reads of 300 bp; Illumina, United States) at the National Human Genome Centre of China in Shanghai, China, according to the manufacturer’s manual.
2.3 Sequence processing and bacteria diversity analysis
Raw 16S rRNA gene fastaq files were processed using Mothur v.1.35.1 according to Miseq SOP (Kozich et al., 2013). Reads were firstly combined and screened to reduce sequencing errors, then aligned against the SILVA-based bacterial reference (Release 138.1). The aligned reads were further de-noised and chimeras were removed using the UCHIME algorithm (Edgar et al., 2011). Any sequences not belonging to bacterial 16S rRNA gene, such as homologs from archaea, chloroplasts, and mitochondria, were classified using the Bayesian classifier and removed before downstream analysis. The optimized sequences were then normalized to 4,052 sequences per sample (the smallest sequencing depth for tested samples) before calculating taxonomy-based diversity and phylogeny-based diversity using Mothur and FastTree, respectively, (Price et al., 2009; Schloss et al., 2009).
For taxonomy-based analysis, the quality-controlled subsample sequences were clustered into operational taxonomic units (OTUs) that share ≥97% sequence similarity. Rare OTUs constituting less than 0.001% of the total normalized filtered sequences were removed, for rare OTUs may result in inflated estimates of diversity (Bokulich et al., 2013). Taxonomic information was then assigned for the remaining OTUs. For phylogeny-based analysis, the subsample sequences with rare OTUs removed were analyzed using FastTree software to compute an approximately-maximum-likelihood phylogenetic tree (Price et al., 2009). The phylogenetic tree of the dominant clade of bacterial orders and their relative abundance across ecosystems were visualized in EvolView (Zhang et al., 2012).
Alpha diversity was analyzed using the number of OTUs observed, the inverse Simpson D (Simpson, 1949), as well as Faith’s phylogenetic diversity (PD) (Helmus et al., 2007) by randomly selecting 3,000 sequences per sample 1,000 times and calculating the average (Supplementary Table S2). Beta diversity was calculated either using the taxonomy-based Bray–Curtis dissimilarity metric or the phylogeny-based weighted UniFrac metric generated from the filtered sequences (evenly sampled at 3,000 reads per sample with 1,000 iterations). The resulting distances were further visualized by using GenGIS geographical mapping analysis (Parks et al., 2009). Analysis of similarity statistics (ANOSIM) (Clarke, 1993) and homogeneity of molecular variance (HOMOVA) (Stewart and Excoffier, 1996) were used to test significant differences in composition and variation of bacterial communities.
Discriminant bacteria from phylum to family level were identified for different ecosystems using linear discriminant analysis effect size (LEfSe) (Segata et al., 2011). Taxa with significant differential abundance (Kruskal–Wallis sum-rank test, p-value <0.05; LEfSe >4) were used to generate taxonomic cladograms illustrating differences between Xizang ecosystems. Indicator OTU analysis (Dufrene and Legendre, 1997) was used to identify potential specialist for each ecosystem at the OTU level. This approach can uncover OTU, which is present in most sites of a group and has a high relative abundance within that group compared to the other groups, with statistical significance evaluated using a randomization procedure. OTUs with IV > 0.5 (indicator value) and p-value <0.05 were classified as group specialists. The high abundant indicators (OTUs representing larger than 0.05% of the total filtered and normalized sequences) identified by this method were selected for species assemblages analysis using Kendall’s W coefficient of concordance (Legendre, 2005). An overall test of independence of all abundant indicator OTUs was first executed. If the null hypothesis was refused, correlated species were divided into groups and permutation tests were used to test the contribution of each OTU to the overall statistic within each group. OTUs representing larger than 0.05% of the total subsample sequences were used in the analysis to avoid inflated estimates of indicator OTUs caused by rare taxa.
2.4 Effects of environmental factors on bacterial communities
The variation in phylogenetic diversity was measured using null-model-based phylogenetic β-diversity metrics (βNTI) to classify community pairs into underlying drivers of deterministic and stochastic processes, as described in previous studies (Dini-Andreote et al., 2015; Jiao et al., 2022). Briefly, when βNTI is less than −2, it suggests a significantly lower phylogenetic turnover than expected under a null model of community assembly, indicating the presence of homogeneous selection, where similar species tend to coexist. Conversely, a βNTI greater than 2 indicates significantly higher phylogenetic turnover, suggesting variable selection, where dissimilar species coexist. If the absolute value of βNTI is less than 2, it indicates that stochastic processes, such as dispersal, drift, or speciation, play a dominant role in shaping the community structure. Mantel and Partial Mantel tests were performed based on weighted UniFrac distance and Euclidean distances (elevation, geographic, or edaphic distances) to study the correlation between environmental factors and soil bacterial communities. After removing highly correlated environment variables (Spearman ρ > 0.8), Hellinger transformed OTU abundance data were projected to environmental variables: geographic (G), elevation (A), edaphic attributes (E) for correlation analysis using redundancy analysis (RDA). The variance degree of bacterial taxa accounted by those three explanatory variables and their combined effects were further determined using the partitioning functions based on RDA analysis. These analyses were all carried out by using functions in the vegan package in R software (v 3.1.3 and v 3.2.2) (Legendre and Legendre, 2012; Oksanen et al., 2016). Partial correlation analysis was used to control the variation of other edaphic factors when the correlation between pH/OM and relative abundance of Acidobacteria was studied.
3 Results
3.1 Homogenous bacterial community in the barley field in contrast to other alpine ecosystems
From 66 soil samples of different ecosystems along the Yarlung Zangbo River valley (Figure 1A), 745,451 quality-trimmed sequences (an average of 253 bp) were generated with a reduction of 27.5% of total raw sequencing reads. In total, 5,329 OTUs were kept after removing rare OTUs and the number of observed OTUs per sample ranged from 352 to 779 (Supplementary Table S2). The highland barley farmland soils harbored the lowest variation in bacterial community structure as measured by using weighted UniFrac distance (Figure 1B and Supplementary Figure S1D) compared to those of forest (Dunn’s Post-Hoc test, p-values = 1.84e−04) and meadow (p-values = 3.72e−24), but showed no significant difference to those of tundra (p-values >0.05). HOMOVA (Table 1) showed that the amount of bacterial beta diversity within farmland samples was different from those of forest and meadow (all p-values <0.05), but not from those of tundra and swamp (all p-values >0.05). ANOSIM showed that the bacterial composition of farmland soils was different from those of wild ecosystems (all p-values <0.01; Table 1). Dissimilarity of bacterial composition between different wild ecosystems was also uncovered for 5/6 pairs (all p-values <0.05), except the meadow-forest pair (p-value >0.05).
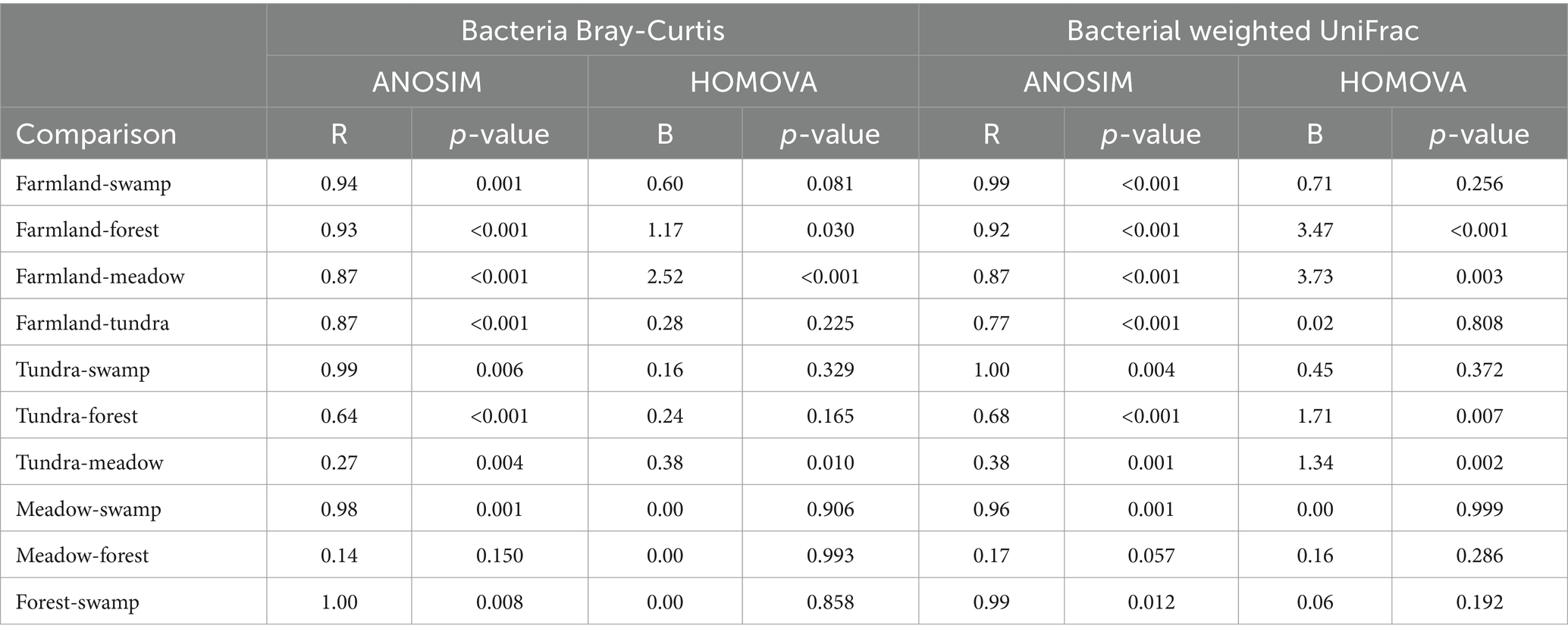
Table 1. Analysis of similarities (ANOSIM) and homogeneity of molecular variance (HOMOVA) of bacterial beta-diversity.
The variation patterns of the soil bacterial community within or between ecosystems were further analyzed using geospatial biodiversity mapping based on the pairwise weighted UniFrac distance (Figure 1C). Generally, independent of geographical distance between samples (Figure 1A), farmland samples formed a homogeneous cluster, which is closer to the alpine tundra cluster than the intermingled forest-meadow branches. A significant distance decay of community similarity was revealed for samples of tundra (Supplementary Figure S2, R2 = 0.19, p-value <0.01) and meadow (R2 = 0.12, p-value <0.001), but not for farmland (R2 = 0.0056, p-value >0.05) and forest samples (R2 = 0.0526, p-value >0.05). Swamp samples were not analyzed herein due to the small sample size and their immediate vicinity (less than 10 meters’ distance). In short, soil bacterial communities in the highland barley farmland were highly homogenous along the Yarlung Zangbo River valley compared to those of wild ecosystems investigated herein in the same region.
3.2 The relative importance of stochastic and deterministic processes in shaping the beta diversity of soil bacterial communities in alpine ecosystems in Xizang
Null model analyses revealed that the deterministic assembly was dominant in bacterial communities in highland barley farmland (93%) and tundra (84%) soils, whereas stochastic assembly also contributed a large fraction to the assembly of bacterial communities in forest (41%), meadow (40%) and swamp (67%) soils (Figure 2). In particular, the assembly of bacterial communities in highland barley farmland and tundra soil was governed by the homogeneous selection (βNTI < −2, Figure 2), in accordance with the homogenous bacterial community in these two ecosystems contrast to other alpine ecosystems. The relationships between phylogenetic diversity and major factors were also used to infer changes in the relative influences of deterministic and stochastic assembly processes, which was assessed using the Mantel tests that correlated the weighted UniFrac distance matrices with the Euclidean distance matrices of environmental variables. The highly correlated environmental variables (Spearman ρ > 0.8) among soil physicochemical properties, climatic and topographical features (Supplementary Table S1) were removed. The final variables used were as follows: latitude, longitude, altitude, organic matter, available phosphorus, available potassium, electrical conductivity, pH, Na+, Cl− and SO42−. All selected environmental features except K+ and SO42− exhibited significant differences between ecosystems (ANOVA, all p-values <0.01, Supplementary Table S3).
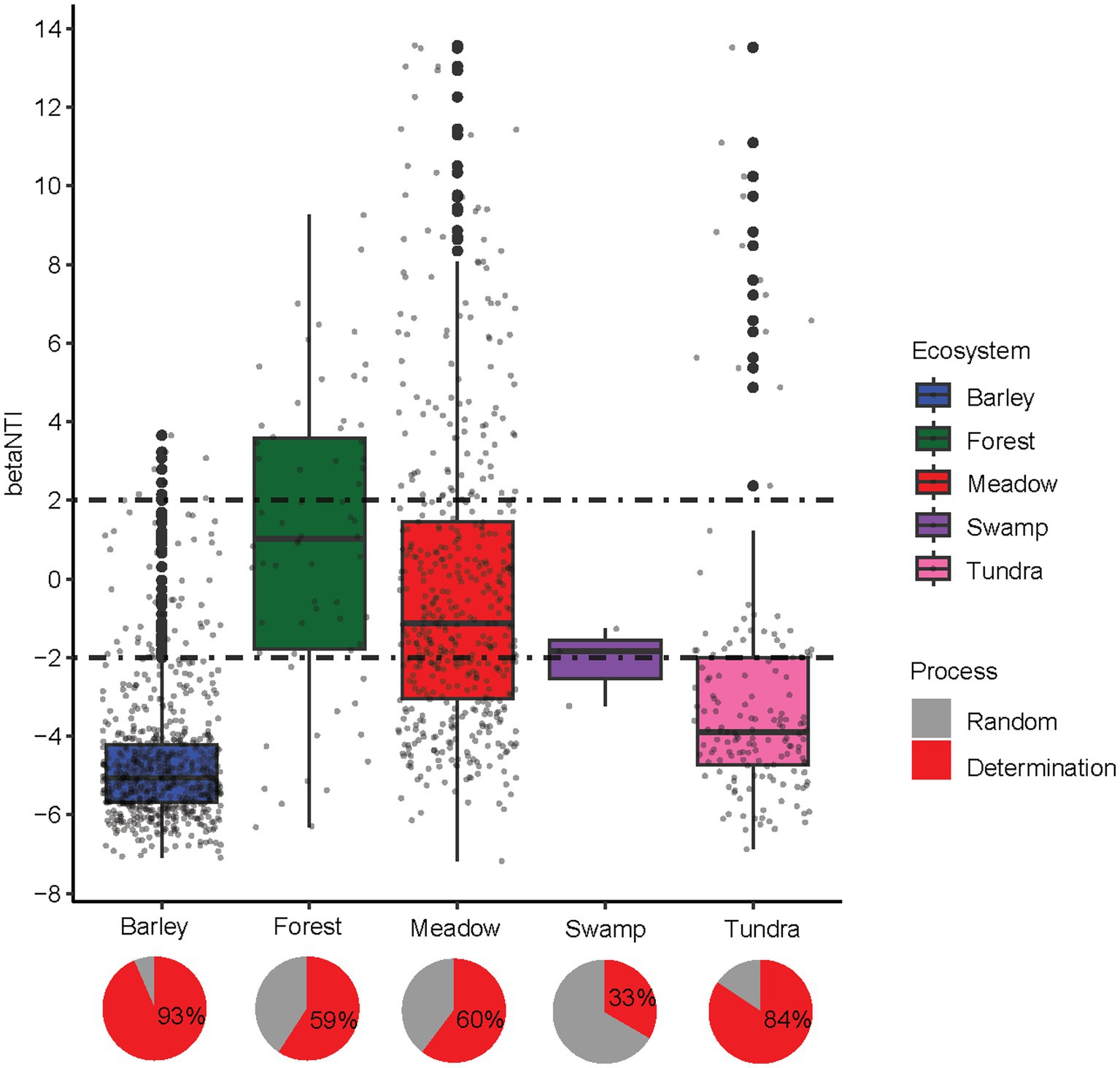
Figure 2. The distributions of between community analog (βNTI) across alpine ecosystems. The horizontal dashed lines indicate the βNTI values of +2 and − 2. Pie plots shows the relative contribution of each ecological process to community assembly.
The Mantel and Partial Mantel tests revealed that the beta diversity, based on weighted UniFrac distance (Table 2), within farmland samples was not correlated with the distance matrix of geographic (latitude and longitude), elevation (altitude) or edaphic characteristics (all p-values >0.05), indicated that the deterministic assembly of bacterial communities of highland barley farmland could not be explained by measured environmental variables. By contrast, the beta diversity of tundra samples correlated with edaphic distance (ρ = 0.396, p-value <0.05) in the Mantel test, and after controlling for geographic (ρ = 0.359, p-value <0.05) and elevation distances (ρ = 0.397, p-value <0.05) in the Partial Mantel test, indicating significant effects of deterministic factors. Similarly, the beta diversity of meadow samples correlated with geographic (ρ = 0.366, p-value <0.01) and edaphic distances (ρ = 0.510, p-value <0.001) in the Mantel test. The beta diversity of forest samples showed no correlation with elevation, geographic and edaphic factors (Table 2, all p-values >0.05), despite its high level of similarity to that of meadow samples (Figure 1B).
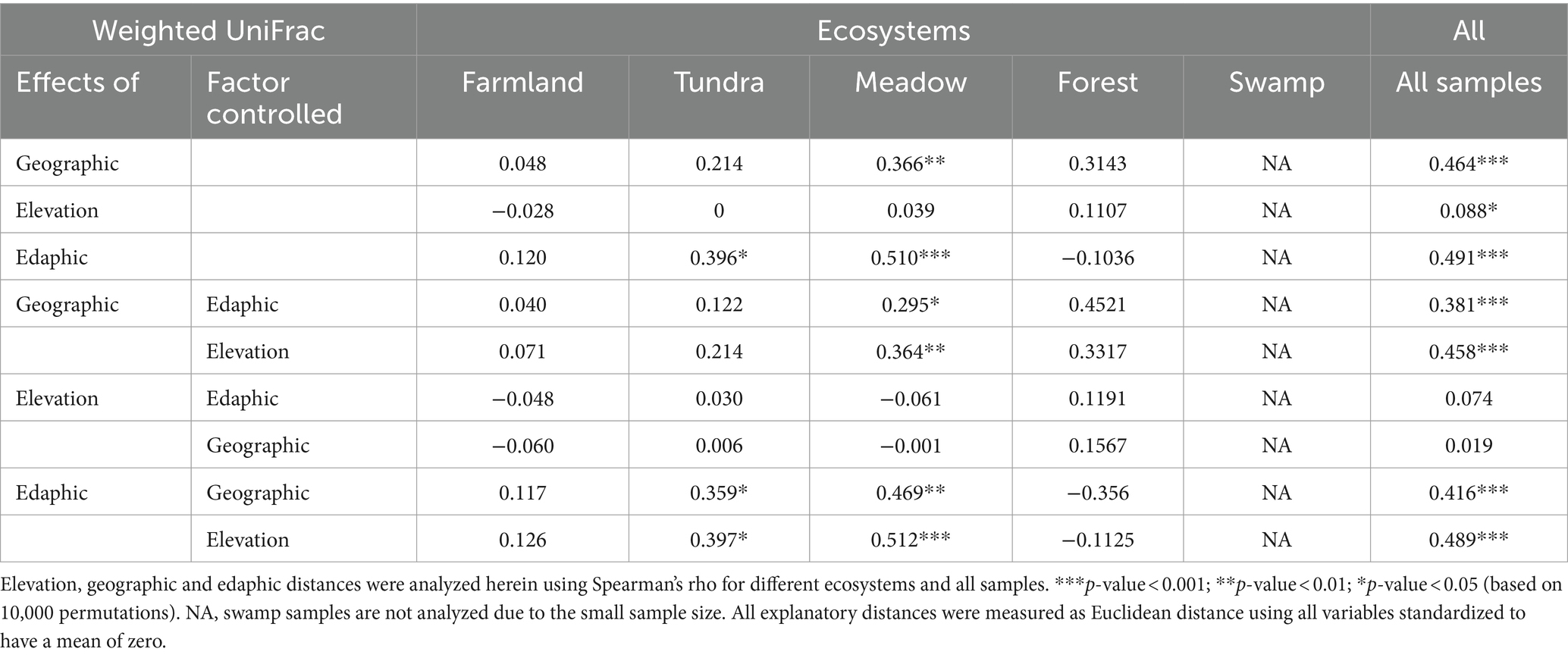
Table 2. Mantel and partial Mantel tests for the correlation between Weighted UniFrac distances of soil bacterial communities and the Euclidean distances of all explanatory variables.
3.3 Discriminant bacterial taxa in alpine ecosystems
Among the 66 soil samples collected from different ecosystems, bacterial communities were dominated by the phyla Acidobacteria (52 ± 16%, average ± SD), Verrucomicrobia (15 ± 11%), Proteobacteria (7 ± 12%), Planctomycetes (2 ± 1%), Bacteroidetes (1 ± 2%), Actinobacteria (1 ± 3%), and Chloroflexi (1 ± 2%) (Figures 1C, 3A). Relative abundances of these seven phyla were different across the five ecosystems (multivariate analysis of variance, p-value <0.001). Relative abundances of Acidobacteria in soil samples of farmland, swamp and tundra were higher than those from forest and meadow (Figure 3A, Tukey’s test, p-value <0.05), whereas Verrucomicrobia, Proteobacteria, Bacteroidetes and Actinobacteria showed the opposite pattern.
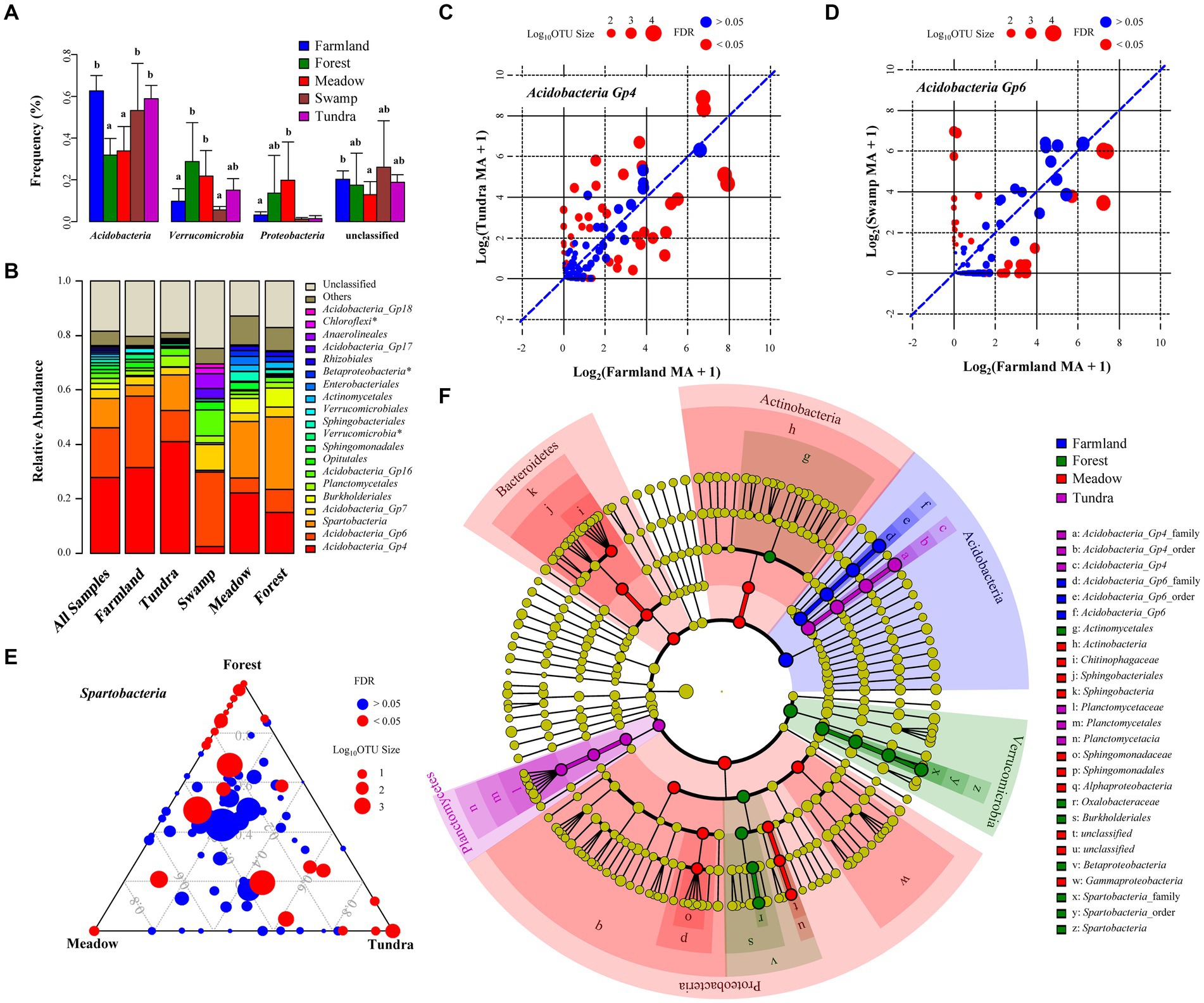
Figure 3. Discriminant bacterial taxa in alpine ecosystems. (A) Average relative abundance of major bacterial phyla. Error bars represent SD. Different letters indicate significant difference between ecosystems (p-value <0.05, Tukey’s test). (B) Relative abundance of the top 20 taxa at the order level. * represents the unclassified orders, for which phylum/class name is used. (C-E) Comparison of relative abundance of all OTUs classified as Acidobacteria_Gp4 in the farmland and tundra groups (C), Acidobacteria_Gp6 in the farmland and swamp groups (D), and Spartobacteria among mountain biomes (E). OTUs represented by red points are those of significantly different abundance between groups (Kruskal-Wallis test, p-value <0.05). The points are sized according to their log10-transformed number of reads in two groups. The y = x blue dashed line indicates equal average relative abundance. (F) Indicator bacterial taxa identified by linear discriminant analysis effect size (LEfSe).
Notably, Acidobacteria_Gp4, Acidobacteria_Gp6 and Spartobacteria dominated bacterial community composition, from class to family level. These three clades accounted for 56.9% of the total sequence in all soil samples (Figure 3B). Acidobacteria_Gp4 was 31.6 and 40.9% of the total sequences in farmland and tundra, respectively. Acidobacteria_Gp6 accounted for 26.2 and 27.2% of the total sequences in farmland and swamp, respectively. Spartobacteria was 26.8, 20.8 and 13.1% of the overall sequences in forest, alpine meadow and tundra, respectively (Figure 3B). Although Acidobacteria_Gp4 dominated in both farmland and tundra soils, one quarter of its OTUs (361 OTUs in total) showed biased distribution between these two ecosystems (Kruskal Wallis test with FDR-adjusted p-value <0.05; Figure 3C and Supplementary Figure S3). Similarly, 14.1% of OTUs belonging to Acidobacteria_Gp6 (340 OTUs in total) were different in relative abundance between farmland and swamp (Figure 3D and Supplementary Figure S4), 27.7% of OTUs within Spartobacteria (139 OTUs in total) were different in relative abundance between forest, alpine meadow and tundra (Figure 3E and Supplementary Figure S5). These results imply the existence of potential discriminant bacterial taxa for each ecosystem.
LEfSe identified 37 significantly discriminant bacterial taxa for Xizang ecosystems (Kruskal–Wallis sum-rank test, p-value <0.05; LEfSe >4), such as Acidobacteria_Gp6 clade (from phylum to family level) for the highland barley farmland, Acidobacteria_Gp4 and Planctomycetaceae clades for tundra, Oxalobacteraceae and Spartobacteria clades in forest soils, Sphingomonadaceae and Chitinophagaceae clades in alpine meadow soils (Figure 3F). Swamp samples were not analyzed in LEfSe due to small sample size. Indicator species analysis identified 50 indicator OTUs with IV > 0.5 (indicator value) and p-value <0.05 (Supplementary Table S4 and Supplementary Figure S6). Acidobacteria_Gp4 OTUs have more probability being identified as indicator taxa for farmland (5 OTUs) and tundra (4 OTUs), while Proteobacteria OTUs are more frequently identified as indicator taxa for forest and meadow. To elucidate potential relationship among these indicator OTUs, Kendall’s W coefficient of concordance was used to identify local assemblages of the indicator OTUs and clustered the 50 indicator OTUs into three assemblages (with 17, 12 and 21 OTUs for each assemblage) (Supplementary Table S4 and Supplementary Figure S6). 17/18 indicator OTUs identified for farmland and alpine tundra groups belong to the same Kendall’s group (corrected p-value <0.05). These results are consistent with the beta diversity pattern and indicates that agricultural practices in highland barley farmland and soil degradation in alpine tundra might have consistency in their effects on soil microbial communities.
3.4 Environmental factors shaping the beta diversity of bacterial communities
RDA showed that 31.6% (based on adjusted R2, p-value <0.001) of the variance of bacterial communities among tested samples could be explained by those selected environmental and spatial factors. Variation partitioning analysis (Figure 4A) further revealed that edaphic factors alone captured 12.7% of variance (p-value <0.001), while very limited variation could be attributed to pure effects of altitude (0.9%, p-value = 0.02) and geographic distance (2.5%, p-value = 0.316). By contrast, the combined effects of edaphic factors with either altitude (23.3%) or geographic distance (24.9%) apparently may play a greater role in shaping the observed beta diversity (Figure 4A). As shown in the RDA analysis (Figure 4B), pH and organic matter were the major environmental factors along the first axis (22.5% of total variance, p-value <0.001). The farmland cluster was separated from those of wild ecosystems, particularly meadow and forest, along these two edaphic factors. The meadow and forest samples were enriched with certain OTUs belonging to Proteobacteria and Verrucomicrobia while the farmland samples harbored more OTUs of Acidobacteria (Figure 4B). Significant correlations between some OTUs and other individual edaphic factors such as phosphorus, SO42−, EC, Na+ and Cl− were also observed (Figure 4B).
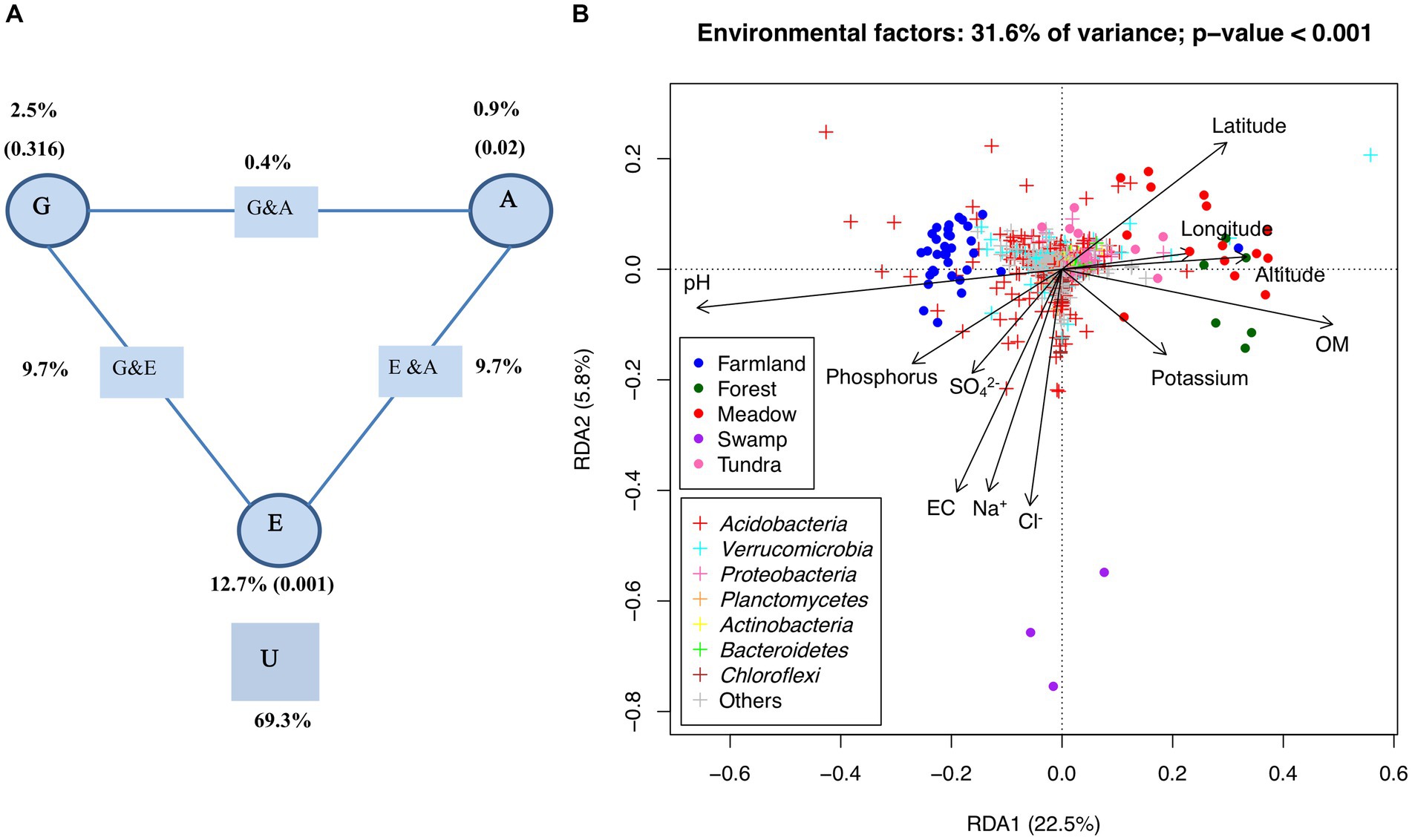
Figure 4. Deterministic factors shaping the bacterial communities. (A) Variation partition analysis of OTUs among geographic (“G”), elevation (“A”), edaphic attributes (“E”), and their interactions (“G x A,” “G x E,” and “E x A”). U, Unexplained variation (residual). The values in parentheses are p-values. (B) RDA of OTUs and environmental attributes. The percentage of variation explained by RDA1 and RDA2 is shown, both of the eigenvalues (RDA1 and RDA2) and all of the environmental variables are significant in permutation tests (α = 0.05). OM, organic matter; phosphorus, available phosphorus; potassium, available potassium; EC, electrical conductivity.
Acidobacteria was the most abundant clade in tested samples. As shown in the heat map and scatterplots of the abundance of Acidobacteria subgroups corresponding to pH and organic matter (Figures 5A–E), relative abundance of Acidobacteria correlated negatively with soil organic matter (R2 = 0.34, Spearman ρ = −0.54, p-value <0.001) and positively with soil pH (R2 = 0.55, ρ = 0.70, p-value <0.001), i.e., higher in farmland and tundra soils and lower in the organic-rich acidic meadow and forest soils. This differs from the pattern uncovered from earlier reported data in North and South America (Jones et al., 2009) (Figures 5B,C). This discrepancy could be explained at the subgroup level, i.e., subgroups 1 (abundance range: 0 ~ 4.13%), 2 (0 ~ 5.20%), 3 (0 ~ 1.11%), 12 (0 ~ 0.05%), 13 (0 ~ 0.19%) and 15 (0%) showing negative correlations with pH were apparently less abundant than subgroups 4 (1.25 ~ 66.03%), 6 (2.46 ~ 43.28%), 7 (0.77 ~ 14.28%), 16 (0.27 ~ 12.62%), and 17 (0.10 ~ 5.33%) showing positive correlations with pH (Figures 5A,D,E).
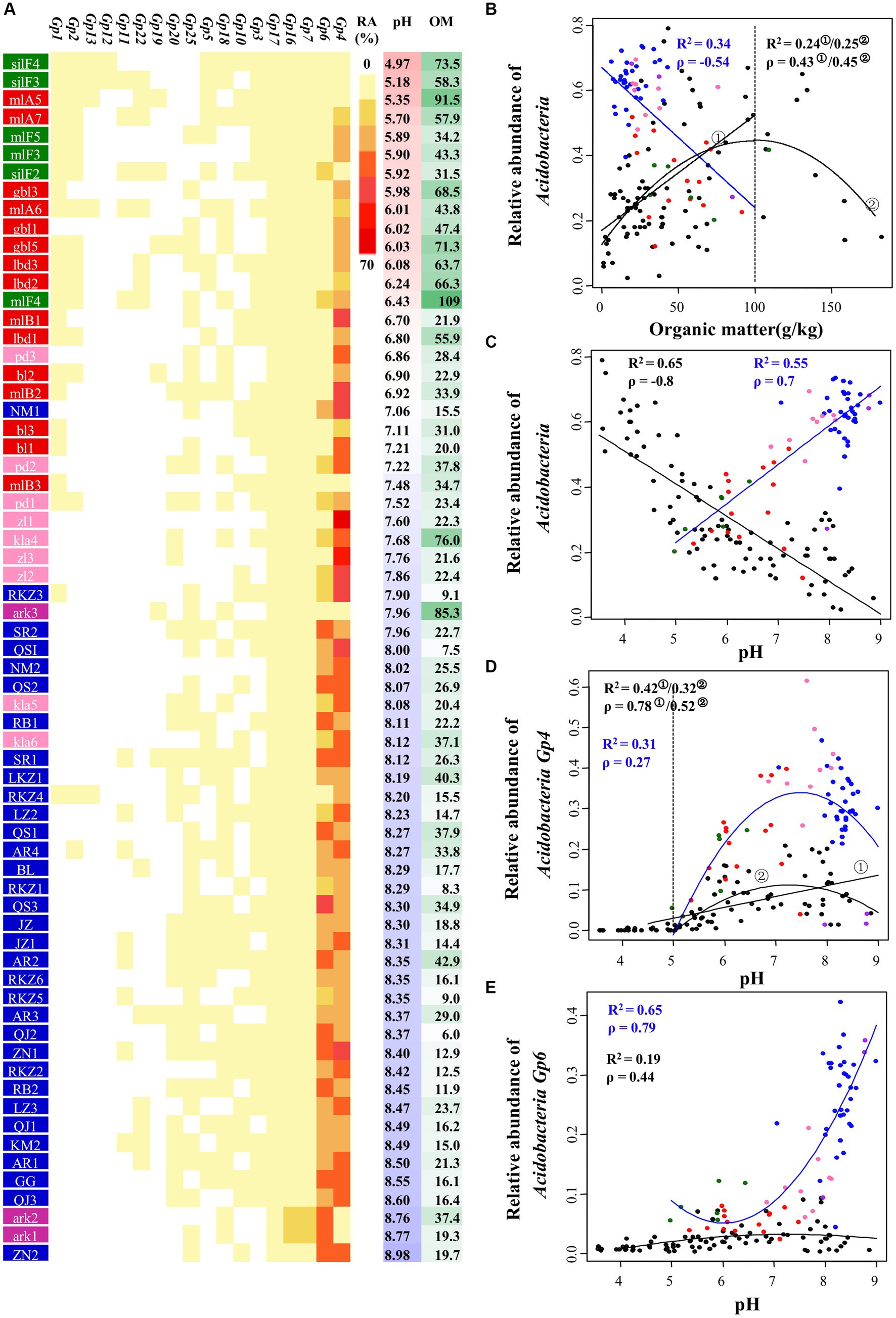
Figure 5. Relationship between the relative abundance of Acidobacteria and pH or organic matter in 66 soil bacterial communities. (A) The relative abundance of each Acidobacteria subgroup in each sample (ranging from 0 to 66.0%). OM, organic matter. (B,C) The effect of OM (B) or pH (C) on the abundance of Acidobacteria. (D,E) The effect of pH on the abundance of Acidobacteria_Gp4 (D) and Acidobacteria_Gp6 (E). Samples were colored according to different ecosystems: farmland (blue), forest (green), meadow (red), swamp (purple), tundra (pink). Results from the research by Jones et al. (2009) were included in our analysis (black dots). Both Spearman’s rank correlation (ρ) and regression analysis (R2) were used for testing the correlations, all p-values <0.001.
In addition to the strong positive correlation with pH, relative abundance of Acidobacteria showed lower levels of correlation with EC (ρ = 0.414), available phosphorus (ρ = 0.365), Na+ (ρ = 0.297) and SO42− (ρ = 0.288) (all p-values <0.05). When these edaphic factors were individually controlled, the partial correlation coefficient of pH and relative abundance of Acidobacteria ranged from 0.65 to 0.8 (all p-values <0.001). If the other edaphic factors were all controlled, the coefficient was 0.41 (p-value = 0.0007). This implies that the positive correlation between pH and relative abundance of Acidobacteria was robust in our test samples. By contrast, the partial correlation coefficient was −0.17 (p-value >0.05) between organic matter and Acidobacteria abundance when the other edaphic factors were all controlled, indicating a combined effect by organic matter and other edaphic attributes.
4 Discussion
Understanding patterns and processes of bacterial biogeography is an important way to evaluate the ecological effect caused by human activities (Geremia et al., 2016; Wu et al., 2021; Xue et al., 2021). Soil biodiversity is strongly influenced by external drivers such as climate change and nitrogen deposition but also by land-use management (Wall et al., 2015). Evidence is mounting that soil biodiversity loss and simplification of soil community composition impair multiple ecosystem functions, including plant diversity, decomposition, nutrient retention, and nutrient cycling (Wagg et al., 2014). The Qinghai-Xizang Plateau, an ecologically vulnerable region, has undergone studies investigating soil bacterial communities in individual wild ecosystems like alpine meadows and lake sediments (Xiong et al., 2012; Yang et al., 2014; Yuan et al., 2014; Wang et al., 2022; Yang et al., 2022). In the present study, we compared soil bacterial communities in the highland barley farmland to those of wild alpine ecosystems including tundra, meadow, forest and swamp along the Yarlung Zangbo River valley, which is the major region for highland barley cultivation.
Soil bacterial communities were highly homogeneous in the highland barley farmland compared to those of meadow, forest and swamp. The observed low beta diversity in farmland samples might be partially due to the relatively simple vegetation coverage by highland barley compared to that of meadow, forest and swamp. This view is supported by a report of soil microbial communities along four sites of a Qinghai-Xizang mountainous grassland (Yang et al., 2014), where vegetation diversity was revealed as one of the major factors shaping the variations of microbial communities. Although farmland samples showed a similar level of beta diversity to those from tundra, no significant relationship was found between the beta diversity of farmland samples and their corresponding geographic, elevation and edaphic distances. This is in contrast to the observations in meadow samples in this study and earlier reports on bacterial communities in lake sediments and alpine meadows in the Qinghai-Xizang Plateau (Xiong et al., 2012; Yang et al., 2014; Yuan et al., 2014). It might be reasonable to speculate that transport via human activities, such as those in the farmland, could be more efficient than dispersal via wind, water, and other hitchhiking mechanisms in wild ecosystems (Papke and Ward, 2004). Recent studies demonstrated that land conversion to agricultural land results in taxonomic and functional homogenization of soil bacteria and fungi, mainly driven by the increase in the geographic ranges of taxa in croplands (Peng et al., 2024) and disproportionate negative effects of agricultural cultivation on rare microbial taxa (Banerjee et al., 2024). These results align with our perspective, indicating that agricultural activities lead to microbial homogenization and has profound implications for the structure and function of soil ecosystems, highlighting the importance of agricultural sustainability in protecting biodiversity.
The dominant phylum was Acidobacteria in all ecosystems tested in this study, and this is in line with earlier studies of soil bacterial communities across continents suggesting Acidobacteria as ubiquitous and abundant members (Zinger et al., 2009; Yuan et al., 2014; Sun et al., 2020; Sui et al., 2022). Moreover, farmland, tundra and swamp soils characterized with higher pH and lower organic matter than meadow and forest soils had a higher abundance of Acidobacteria. This is consistent with a negative correlation between abundance of Acidobacteria and organic carbon availability, and a strong positive correlation between pH and Acidobacteria abundance. These results are inconsistent with an earlier report in North and South America soils (Jones et al., 2009) but could be partially explained at the subgroup level of Acidobacteria, since subgroups such as 1, 2, 3, 12 and 13 which have negative correlations with pH (Jones et al., 2009) were less abundant in test samples of this study than those subgroups (4, 6, 7, 16, and 17) that have positive correlations with pH. Therefore, the general conclusion of the correlation between Acidobacteria abundance and pH/organic carbon availability proposed earlier (Fierer et al., 2007; Jones et al., 2009) might be misleading. These findings suggest that more studies should be compared to improve our understanding of the ecological significance of Acidobacteria (Kielak et al., 2016), and it is important to investigate bacterial communities at a high-resolution level (Liao et al., 2023).
Agricultural intensification can reduce the diversity and density of beneficial organisms; less-intensive practices such as enhancing crop diversity by rotation and intercropping, and reducing soil tillage are beneficial to soil biodiversity and the sustainability of agriculture (Wall et al., 2015; Bender et al., 2016). In this study, a considerable similarity level of bacterial communities between farmland and tundra soils was found. Tundra is characterized by its lower vegetation coverage than meadow and forest, and represents one of the most vulnerable ecosystems on the planet (Malanson et al., 2009; Sistla and Schimel, 2013; Stark et al., 2015). Future action should be taken to increase the crop diversity of barley farmland system by using rotation and intercropping crops (Bardgett and van der Putten, 2014; Bender et al., 2016; Blaser et al., 2016). Moreover, similar edaphic conditions (such as pH and organic matter) between the highland barley farmland and tundra suggest that the nutrient management of highland barley farmland soils should be carried out. Particularly pH and organic matter representing the edaphic factors with major effects on soil bacterial community could be managed in agriculture practices (van Rijssel et al., 2022; Wang et al., 2023). While this study provides valuable insights into the bacterial biogeography of highland barley farmland and wild alpine ecosystems, it has some limitations. Firstly, our study focused on the taxonomic biogeography of bacterial communities alone, a more comprehensive analysis incorporating multiple microbial groups (e.g., bacteria, fungi and archaea) and microbial function could provide a broader perspective on the effects of land-use change. Secondly, here we focused on a relatively small geographical scale in the Qinghai-Xizang Plateau, limiting the generalizability of the findings. Broader spatial sampling across different ecoregions and elevations could provide a more comprehensive understanding of bacterial biogeography patterns in alpine ecosystems.
5 Conclusion
An important aim of studies of soil bacterial community is to improve our understanding of the effects of human activities on the sustainability of agricultural soils. Our study highlights the distinctiveness of soil bacterial communities in highland barley farmland compared to those in wild alpine ecosystems along the Yarlung Zangbo River valley. The homogeneity observed in farmland bacterial communities, despite their similarity to tundra samples, but with no detectable correlations with abiotic effectors, suggests that agricultural activities in highland barley farmland may be having a profound effect on soil microbial diversity. This finding raises concerns about the potential ecological consequences of agricultural practices in this region, especially given the already fragile nature of the Qinghai-Xizang Plateau.
Data availability statement
The datasets presented in this study can be found in online repositories. The names of the repository/repositories and accession number(s) can be found in the article/Supplementary material.
Author contributions
XW: Visualization, Validation, Investigation, Funding acquisition, Conceptualization, Writing – review & editing, Writing – original draft, Supervision, Resources, Project administration, Methodology, Formal analysis, Data curation. YY: Writing – review & editing, Formal analysis. QN: Writing – review & editing. J-WG: Writing – review & editing. ZT: Writing – review & editing. XS: Supervision, Investigation, Funding acquisition, Conceptualization, Writing – review & editing. CT: Writing – original draft, Conceptualization, Writing – review & editing, Validation, Supervision, Resources, Funding acquisition.
Funding
The author(s) declare that financial support was received for the research, authorship, and/or publication of this article. This work was supported by the National Natural Science Foundation of China (32100227 and 32370016), projects in Xizang Autonomous Region (2016ZDKJZC and ZH20170001).
Acknowledgments
We thank Jin Kou, Zi Wang, Ke Feng, and Ying Shi for their assistance in collecting samples in the Qinghai-Xizang Plateau. We also thank Prof. J. Peter W. Young for polishing the language.
Conflict of interest
The authors declare that the research was conducted in the absence of any commercial or financial relationships that could be construed as a potential conflict of interest.
Publisher’s note
All claims expressed in this article are solely those of the authors and do not necessarily represent those of their affiliated organizations, or those of the publisher, the editors and the reviewers. Any product that may be evaluated in this article, or claim that may be made by its manufacturer, is not guaranteed or endorsed by the publisher.
Supplementary material
The Supplementary material for this article can be found online at: https://www.frontiersin.org/articles/10.3389/fmicb.2024.1418161/full#supplementary-material
Footnotes
References
Banerjee, S., Zhao, C., Garland, G., Edlinger, A., García-Palacios, P., Romdhane, S., et al. (2024). Biotic homogenization, lower soil fungal diversity and fewer rare taxa in arable soils across Europe. Nat. Commun. 15:327. doi: 10.1038/s41467-023-44073-6
Bardgett, R. D., and van der Putten, W. H. (2014). Belowground biodiversity and ecosystem functioning. Nature 515, 505–511. doi: 10.1038/nature13855
Bender, S. F., Wagg, C., and van der Heijden, M. G. (2016). An underground revolution: biodiversity and soil ecological engineering for agricultural sustainability. Trends Ecol. Evol. 31, 440–452. doi: 10.1016/j.tree.2016.02.016
Blaser, M. J., Cardon, Z. G., Cho, M. K., Dangl, J. L., Donohue, T. J., Green, J. L., et al. (2016). Toward a predictive understanding of earth's microbiomes to address 21st century challenges. MBio 7:16. doi: 10.1128/mBio.00714-16
Bokulich, N. A., Subramanian, S., Faith, J. J., Gevers, D., Gordon, J. I., Knight, R., et al. (2013). Quality-filtering vastly improves diversity estimates from Illumina amplicon sequencing. Nat. Methods 10, 57–59. doi: 10.1038/nmeth.2276
Cao, H., Chen, R., Wang, L., Jiang, L., Yang, F., Zheng, S., et al. (2016). Soil pH, total phosphorus, climate and distance are the major factors influencing microbial activity at a regional spatial scale. Sci. Rep. 6:25815. doi: 10.1038/srep25815
Caporaso, J. G., Lauber, C. L., Walters, W. A., Berg-Lyons, D., Huntley, J., Fierer, N., et al. (2012). Ultra-high-throughput microbial community analysis on the Illumina HiSeq and MiSeq platforms. ISME J. 6, 1621–1624. doi: 10.1038/ismej.2012.8
Clarke, K. R. (1993). Nonparametric multivariate analyses of changes in community structure. Aust. J. Ecol. 18, 117–143. doi: 10.1111/j.1442-9993.1993.tb00438.x
Delgado-Baquerizo, M., Oliverio, A. M., Brewer, T. E., Benavent-González, A., Eldridge, D. J., Bardgett, R. D., et al. (2018). A global atlas of the dominant bacteria found in soil. Science 359, 320–325. doi: 10.1126/science.aap9516
Dini-Andreote, F., Stegen, J. C., van Elsas, J. D., and Salles, J. F. (2015). Disentangling mechanisms that mediate the balance between stochastic and deterministic processes in microbial succession. Proc. Natl. Acad. Sci. USA 112, E1326–E1332. doi: 10.1073/pnas.1414261112
Dufrene, M., and Legendre, P. (1997). Species assemblages and indicator species: the need for a flexible asymmetrical approach. Ecol. Monogr. 67, 345–366. doi: 10.1890/0012-9615(1997)067[0345:SAAIST]2.0.CO;2
Edgar, R. C., Haas, B. J., Clemente, J. C., Quince, C., and Knight, R. (2011). UCHIME improves sensitivity and speed of chimera detection. Bioinformatics 27, 2194–2200. doi: 10.1093/bioinformatics/btr381
Feng, L., Zhang, Z., Yang, G., Wu, G., Yang, Q., and Chen, Q. (2023). Microbial communities and sediment nitrogen cycle in a coastal eutrophic lake with salinity and nutrients shifted by seawater intrusion. Environ. Res. 225:115590. doi: 10.1016/j.envres.2023.115590
Fierer, N., Bradford, M. A., and Jackson, R. B. (2007). Toward an ecological classification of soil bacteria. Ecology 88, 1354–1364. doi: 10.1890/05-1839
Fierer, N., and Jackson, R. B. (2006). The diversity and biogeography of soil bacterial communities. Proc. Natl. Acad. Sci. USA 103, 626–631. doi: 10.1073/pnas.0507535103
Geremia, R. A., Puscas, M., Zinger, L., Bonneville, J. M., and Choler, P. (2016). Contrasting microbial biogeographical patterns between anthropogenic subalpine grasslands and natural alpine grasslands. New Phytol. 209, 1196–1207. doi: 10.1111/nph.13690
Hanson, C. A., Fuhrman, J. A., Horner-Devine, M. C., and Martiny, J. B. H. (2012). Beyond biogeographic patterns: processes shaping the microbial landscape. Nat. Rev. Microbiol. 10, 497–506. doi: 10.1038/nrmicro2795
Hawkins, J. H., and Zeglin, L. H. (2022). Microbial dispersal, including Bison dung vectored dispersal, increases soil microbial diversity in a grassland ecosystem. Front. Microbiol. 13:825193. doi: 10.3389/fmicb.2022.825193
Helmus, M. R., Bland, T. J., Williams, C. K., and Ives, A. R. (2007). Phylogenetic measures of biodiversity. Am. Nat. 169, E68–E83. doi: 10.1086/511334
Jiao, S., Chu, H., Zhang, B., Wei, X., Chen, W., and Wei, G. (2022). Linking soil fungi to bacterial community assembly in arid ecosystems. iMeta 1:e2. doi: 10.1002/imt2.2
Jones, C. T., Meynell, L., Neto, C., Susko, E., and Bielawski, J. P. (2023). The role of the ecological scaffold in the origin and maintenance of whole-group trait altruism in microbial populations. BMC Ecol. Evol. 23:11. doi: 10.1186/s12862-023-02112-2
Jones, R. T., Robeson, M. S., Lauber, C. L., Hamady, M., Knight, R., and Fierer, N. (2009). A comprehensive survey of soil acidobacterial diversity using pyrosequencing and clone library analyses. ISME J. 3, 442–453. doi: 10.1038/ismej.2008.127
Kielak, A. M., Barreto, C. C., Kowalchuk, G. A., van Veen, J. A., and Kuramae, E. E. (2016). The ecology of Acidobacteria: moving beyond genes and genomes. Front. Microbiol. 7:744. doi: 10.3389/fmicb.2016.00744
Kozich, J. J., Westcott, S. L., Baxter, N. T., Highlander, S. K., and Schloss, P. D. (2013). Development of a dual-index sequencing strategy and curation pipeline for analyzing amplicon sequence data on the MiSeq Illumina sequencing platform. Appl. Environ. Microbiol. 79, 5112–5120. doi: 10.1128/AEM.01043-13
Kumar, M., Brader, G., Sessitsch, A., Maki, A., van Elsas, J. D., and Nissinen, R. (2017). Plants assemble species specific bacterial communities from common core taxa in three Arcto-alpine climate zones. Front. Microbiol. 8:12. doi: 10.3389/fmicb.2017.00012
Larin, A. K., Klimina, K. M., Veselovsky, V. A., Olekhnovich, E. I., Morozov, M. D., Boldyreva, D. I., et al. (2024). An improved and extended dual-index multiplexed 16S rRNA sequencing for the Illumina HiSeq and MiSeq platform. BMC Genom. Data 25:8. doi: 10.1186/s12863-024-01192-3
Legendre, P. (2005). Species associations: the Kendall coefficient of concordance revisited. J. Agric. Biol. Environ. Stat. 10, 226–245. doi: 10.1198/108571105X46642
Legendre, P., and Legendre, L. (2012). Numerical ecology (developments in environmental modelling). second Edn. New York: Elsevier.
Liao, H., Ji, Y., and Sun, Y. (2023). High-resolution strain-level microbiome composition analysis from short reads. Microbiome 11:183. doi: 10.1186/s40168-023-01615-w
Malanson, G. P., Brown, D. G., Butler, D. R., Cairns, D. M., Fagre, D. B., and Walsh, S. J. (2009). “Chapter 3 ecotone dynamics: Invasibility of alpine tundra by tree species from the subalpine forest,” in Developments in earth surface processes. eds. D. R. Butler, G. P. Malanson, S. J. Walsh, and D. B. Fagre (Elsevier), 35–61.
Nemergut, D. R., Schmidt, S. K., Fukami, T., O'Neill, S. P., Bilinski, T. M., Stanish, L. F., et al. (2013). Patterns and processes of microbial community assembly. Microbiol. Mol. Biol. Rev. 77, 342–356. doi: 10.1128/MMBR.00051-12
Oksanen, J., Blanchet, F. G., Friendly, M., Kindt, R., Legendre, P., McGlinn, D., et al. (2016). Vegan: Community ecology package. R package version 2.4–1. Available at: https://CRAN.R-project.org/package=vegan
Papke, R. T., and Ward, D. M. (2004). The importance of physical isolation to microbial diversification. FEMS Microbiol. Ecol. 48, 293–303. doi: 10.1016/j.femsec.2004.03.013
Parks, D. H., Porter, M., Churcher, S., Wang, S., Blouin, C., Whalley, J., et al. (2009). GenGIS: a geospatial information system for genomic data. Genome Res. 19, 1896–1904. doi: 10.1101/gr.095612.109
Peng, Z., Qian, X., Liu, Y., Li, X., Gao, H., An, Y., et al. (2024). Land conversion to agriculture induces taxonomic homogenization of soil microbial communities globally. Nat. Commun. 15:3624. doi: 10.1038/s41467-024-47348-8
Price, M. N., Dehal, P. S., and Arkin, A. P. (2009). FastTree: computing large minimum evolution trees with profiles instead of a distance matrix. Mol. Biol. Evol. 26, 1641–1650. doi: 10.1093/molbev/msp077
Ruff, S. E., Biddle, J. F., Teske, A. P., Knittel, K., Boetius, A., and Ramette, A. (2015). Global dispersion and local diversification of the methane seep microbiome. Proc. Natl. Acad. Sci. USA 112, 4015–4020. doi: 10.1073/pnas.1421865112
Schloss, P. D., Westcott, S. L., Ryabin, T., Hall, J. R., Hartmann, M., Hollister, E. B., et al. (2009). Introducing mothur: open-source, platform-independent, community-supported software for describing and comparing microbial communities. Appl. Environ. Microbiol. 75, 7537–7541. doi: 10.1128/AEM.01541-09
Segata, N., Izard, J., Waldron, L., Gevers, D., Miropolsky, L., Garrett, W. S., et al. (2011). Metagenomic biomarker discovery and explanation. Genome Biol. 12:R60. doi: 10.1186/gb-2011-12-6-r60
Shapiro, B. J., Friedman, J., Cordero, O. X., Preheim, S. P., Timberlake, S. C., Szabo, G., et al. (2012). Population genomics of early events in the ecological differentiation of bacteria. Science 336, 48–51. doi: 10.1126/science.1218198
Sistla, S. A., and Schimel, J. P. (2013). Seasonal patterns of microbial extracellular enzyme activities in an arctic tundra soil: identifying direct and indirect effects of long-term summer warming. Soil Biol. Biochem. 66, 119–129. doi: 10.1016/j.soilbio.2013.07.003
Stark, S., Männistö, M. K., Ganzert, L., Tiirola, M., and Häggblom, M. M. (2015). Grazing intensity in subarctic tundra affects the temperature adaptation of soil microbial communities. Soil Biol. Biochem. 84, 147–157. doi: 10.1016/j.soilbio.2015.02.023
Stewart, C. N., and Excoffier, L. (1996). Assessing population genetic structure and variability with RAPD data: application to Vaccinium macrocarpon (American cranberry). J. Evol. Biol. 9, 153–171. doi: 10.1046/j.1420-9101.1996.9020153.x
Sui, X., Frey, B., Yang, L., Liu, Y., Zhang, R., Ni, H., et al. (2022). Soil Acidobacterial community composition changes sensitively with wetland degradation in northeastern of China. Front. Microbiol. 13:1052161. doi: 10.3389/fmicb.2022.1052161
Sun, H., Wu, Y., Zhou, J., Bing, H., and Zhu, H. (2020). Climate influences the alpine soil bacterial communities by regulating the vegetation and the soil properties along an altitudinal gradient in SW China. Catena 195:104727. doi: 10.1016/j.catena.2020.104727
van Rijssel, S. Q., Veen, G. F., Koorneef, G. J., Bakx-Schotman, J. M. T., ten Hooven, F. C., Geisen, S., et al. (2022). Soil microbial diversity and community composition during conversion from conventional to organic agriculture. Mol. Ecol. 31, 4017–4030. doi: 10.1111/mec.16571
Wagg, C., Bender, S. F., Widmer, F., and van der Heijden, M. G. A. (2014). Soil biodiversity and soil community composition determine ecosystem multifunctionality. Proc. Natl. Acad. Sci. USA 111, 5266–5270. doi: 10.1073/pnas.1320054111
Wall, D. H., Nielsen, U. N., and Six, J. (2015). Soil biodiversity and human health. Nature 528, 69–76. doi: 10.1038/nature15744
Walsh, E. A., Kirkpatrick, J. B., Pockalny, R., Sauvage, J., Spivack, A. J., Murray, R. W., et al. (2016). Relationship of bacterial richness to organic degradation rate and sediment age in subseafloor sediment. Appl. Environ. Microbiol. 82, 4994–4999. doi: 10.1128/AEM.00809-16
Wan, W., Gadd, G. M., Gu, J., Liu, W., Chen, P., Zhang, Q., et al. (2023). Beyond biogeographic patterns: processes shaping the microbial landscape in soils and sediments along the Yangtze River. mLife 2, 89–100. doi: 10.1002/mlf2.12062
Wang, X. L., Cui, W. J., Feng, X. Y., Zhong, Z. M., Li, Y., Chen, W. X., et al. (2018). Rhizobia inhabiting nodules and rhizosphere soils of alfalfa: a strong selection of facultative microsymbionts. Soil Biol. Biochem. 116, 340–350. doi: 10.1016/j.soilbio.2017.10.033
Wang, S., Jiao, C., Zhao, D., Zeng, J., Xing, P., Liu, Y., et al. (2022). Disentangling the assembly mechanisms of bacterial communities in a transition zone between the alpine steppe and alpine meadow ecosystems on the Tibetan plateau. Sci. Total Environ. 847:157446. doi: 10.1016/j.scitotenv.2022.157446
Wang, C., Yu, Q.-Y., Ji, N.-N., Zheng, Y., Taylor, J. W., Guo, L.-D., et al. (2023). Bacterial genome size and gene functional diversity negatively correlate with taxonomic diversity along a pH gradient. Nat. Commun. 14:7437. doi: 10.1038/s41467-023-43297-w
Wu, H., Hao, B., Cai, Y., Liu, G., and Xing, W. (2021). Effects of submerged vegetation on sediment nitrogen-cycling bacterial communities in Honghu Lake (China). Sci. Total Environ. 755:142541. doi: 10.1016/j.scitotenv.2020.142541
Xiong, J., Liu, Y., Lin, X., Zhang, H., Zeng, J., Hou, J., et al. (2012). Geographic distance and pH drive bacterial distribution in alkaline lake sediments across Tibetan plateau. Environ. Microbiol. 14, 2457–2466. doi: 10.1111/j.1462-2920.2012.02799.x
Xu, X., Wang, N., Lipson, D., Sinsabaugh, R., Schimel, J., He, L., et al. (2020). Microbial macroecology: in search of mechanisms governing microbial biogeographic patterns. Glob. Ecol. Biogeogr. 29, 1870–1886. doi: 10.1111/geb.13162
Xue, R., Zhao, K., Yu, X., Stirling, E., Liu, S., Ye, S., et al. (2021). Deciphering sample size effect on microbial biogeographic patterns and community assembly processes at centimeter scale. Soil Biol. Biochem. 156:108218. doi: 10.1016/j.soilbio.2021.108218
Yang, Y., Gao, Y., Wang, S., Xu, D., Yu, H., Wu, L., et al. (2014). The microbial gene diversity along an elevation gradient of the Tibetan grassland. ISME J. 8, 430–440. doi: 10.1038/ismej.2013.146
Yang, F., Ma, C., and Fang, H. (2022). Simulation of critical transitions and vulnerability assessment of Tibetan plateau key ecosystems. J. Mt. Sci. 19, 673–688. doi: 10.1007/s11629-021-6960-7
Yuan, Y., Si, G., Wang, J., Luo, T., and Zhang, G. (2014). Bacterial community in alpine grasslands along an altitudinal gradient on the Tibetan plateau. FEMS Microbiol. Ecol. 87, 121–132. doi: 10.1111/1574-6941.12197
Zeng, X., Long, H., Wang, Z., Zhao, S., Tang, Y., Huang, Z., et al. (2015). The draft genome of Xizang Highland barley reveals adaptive patterns to the high stressful Tibetan plateau. Proc. Natl. Acad. Sci. USA 112, 1095–1100. doi: 10.1073/pnas.1423628112
Zhang, H., Gao, S., Lercher, M. J., Hu, S., and Chen, W. H. (2012). EvolView, an online tool for visualizing, annotating and managing phylogenetic trees. Nucleic Acids Res. 40, W569–W572. doi: 10.1093/nar/gks576
Keywords: microbial biogeography, Acidobacteria, pH, Xizang, highland barley
Citation: Wang X, Yang Y, Nan Q, Guo J-W, Tan Z, Shao X and Tian C (2024) Barley farmland harbors a highly homogeneous soil bacterial community compared to wild ecosystems in the Qinghai-Xizang Plateau. Front. Microbiol. 15:1418161. doi: 10.3389/fmicb.2024.1418161
Edited by:
Yong-Hong Liu, Chinese Academy of Sciences (CAS), ChinaCopyright © 2024 Wang, Yang, Nan, Guo, Tan, Shao and Tian. This is an open-access article distributed under the terms of the Creative Commons Attribution License (CC BY). The use, distribution or reproduction in other forums is permitted, provided the original author(s) and the copyright owner(s) are credited and that the original publication in this journal is cited, in accordance with accepted academic practice. No use, distribution or reproduction is permitted which does not comply with these terms.
*Correspondence: Xiaolin Wang, eGx3YW5nQHNjYXUuZWR1LmNu; Changfu Tian, Y2Z0aWFuQGNhdS5lZHUuY24=
†These authors have contributed equally to this work