- 1Yunnan Institute of Digestive Disease, The First Affiliated Hospital of Kunming Medical University, Kunming, China
- 2State Key Laboratory for Conservation and Utilization of Bio-Resources in Yunnan, School of Life Sciences, Yunnan University, Kunming, Yunnan, China
Objective: Colorectal adenomas (CRA) are the primary precancerous lesions leading to colorectal cancer (CRC). Early detection and intervention of CRA can significantly reduce the incidence of CRC. We investigated the relationships between the gut microbiome and the expression levels of PD-L1, IL-6, and IFN-γ at different CRA stages.
Methods: Participants were divided into normal, non-advanced adenoma (NAA), and advanced adenoma (AA) groups. PD-L1 expression in collected tissues was analyzed via immunohistochemistry (IHC) and Western blotting. Serum IL-6 and IFN-γ levels were measured using Enzyme-Linked Immunosorbent Assay (ELISA). 16S rRNA gene sequencing was used to examine gut microbiota changes, with correlation analysis to assess microbial influences on CRA progression.
Results: The main differences in bacterial composition among the three groups were found within the Firmicutes and Bacteroidetes phyla. In the normal vs. NAA comparison, Clostridium sensu stricto, Faecalimonas, Gemmiger, and Ruminococcus were more abundant in the normal group, while Solobacterium was enriched in the NAA group. For the normal vs. AA comparison, the normal group was enriched with Anaerostipes, Blautia, Clostridium sensu stricto, Intestinibacter, Phocaeicola, and Turicibacter, whereas Solobacterium was more abundant in the AA group. In the NAA vs. AA comparison, the NAA group exhibited higher levels of Blautia, Faecalimonas, and Turicibacter relative to the AA group. Anaerostipes and Blautia are positively correlated with taurine and hypotaurine metabolism, propanoate metabolism, and zeatin biosynthesis. PD-L1 protein levels progressively increase with CRA advancement. Additionally, Faecalimonas, and Solobacterium were negatively associated with IFN-γ, while Gemmiger, and Anaerostipes were positively associated with IL-6.
Conclusion: This study highlights the dynamic alterations in gut microbiota composition and their potential influence on the regulation of inflammatory cytokines and PD-L1 expression during CRA progression. The enrichment of protective taxa, such as Anaerostipes and Blautia, in the normal group emphasizes their potential role in mitigating adenoma progression. Dietary modulation to promote the proliferation of these beneficial bacteria could serve as a promising strategy to improve colorectal health. Future research should further explore the specific relationships between dietary components, gut microbiota, and metabolic pathways, and assess the effects of dietary interventions on gut health.
1 Introduction
Colorectal cancer (CRC) is the third most common malignant tumor globally and the second leading cause of cancer-related death, accounting for approximately 10% of all cancer-related deaths (Sung et al., 2021). In China, CRC has become the third most common malignant tumor (Zheng et al., 2019). Over 85% CRCs originate from colorectal adenomas (CRA), and the causal relationship between the two has been established (Fu et al., 2025). The “normal mucosa-adenoma-cancer” pathway is considered the main route for CRC formation (Mang, 2019). The reported prevalence of CRA in China is approximately 13.3%–18.1%, with the prevalence of advanced adenomas being about 3.5% (Hong et al., 2018; Pan et al., 2020). Advanced adenomas are significant precursors to CRC, with the risk of progression to CRC increasing significantly by approximately 30%–50% (Conteduca et al., 2013). Although much research has focused on the metastatic and therapeutic aspects of advanced CRC, studies on the CRA stage are relatively limited, particularly in the areas of gut microbiota and dietary influences on CRA. Therefore, this study aims to explore the biomarkers and microbiota changes during the CRA stage, combined with dietary interventions, to provide new insights for early diagnosis and intervention of CRA.
Dietary interventions, as a non-pharmacological treatment approach, have been widely applied in the management of various diseases. In the prevention and treatment of CRA and CRC, a well-balanced diet can significantly reduce disease risk and improve patient prognosis. Studies have shown that the intake of dietary fiber can lower the risk of CRC by increasing the abundance of beneficial gut microbiota and reducing carcinogens in the gut (Munteanu and Schwartz, 2024; Song et al., 2018). Therefore, dietary treatment holds great promise as an effective measure for preventing the progression of CRA and the occurrence of CRC.
The “normal mucosa-adenoma-cancer” process is a multifactorial and multistep complex process. The human gut microbiota, primarily composed of bacteria from the Firmicutes, Bacteroidetes, Actinobacteria, and Proteobacteria phyla, is essential for functions such as nutrient absorption and immune regulation (Adak and Khan, 2019). Dysbiosis, or imbalance in this microbiota, can disrupt these functions and contribute to the development of diseases like colorectal tumors. Specifically, dysbiosis is linked to CRA and CRC, potentially by damaging intestinal epithelial cells, altering metabolism, and producing carcinogens (Flemer et al., 2017; Sepich-Poore et al., 2021). This disruption may lead to abnormal crypt foci, promoting the formation of colorectal polyps and eventually cancer (Liang et al., 2020; Zouggar et al., 2020).
In the tumor microenvironment, pro-inflammatory cytokines like IFN-γ and IL-6 can upregulate PD-L1, a protein that helps tumors evade immune detection (Lim et al., 2016). This upregulation allows cancer cells to avoid being targeted by the immune system. The PD-1/PD-L1 pathway, increasingly recognized for its role in CRC, suppresses cytotoxic lymphocyte activity, facilitating immune escape (Chen et al., 2017; Wu et al., 2022). Additionally, changes in the gut microbiota composition and metabolism can contribute to CRC by influencing local inflammation and genotoxic stress (Cao et al., 2022). However, the exact relationship between PD-L1 expression (Freitas et al., 2021; Gatenbee et al., 2022; Miller et al., 2018) and plasma inflammatory cytokines (Huang et al., 2019; Shi et al., 2015) with disease progression remains unclear.
Our study aims to investigate changes in the gut microbiota, IL-6, IFN-γ, and PD-L1 across different stages of CRA. By identifying significant biomarkers associated with these stages, and regulating gut microbiota composition and the production of beneficial metabolic products through dietary interventions.
2 Materials and methods
2.1 Ethics statement
This study was approved by the Ethics Committees of the First Affiliated Hospital of Kunming Medical University (project license number: L-26/2022). It was conducted in accordance with the principles of the Declaration of Helsinki. Written informed consent was obtained from all participants included in the study. Additionally, all research activities were carried out following applicable guidelines and regulations.
2.2 Subjects
The study was conducted between 2022 and 2023 at the First Affiliated Hospital of Kunming Medical University, with a total of 30 participants involved. These individuals were categorized into three groups based on their colorectal health status: normal (n = 10), non-advanced adenoma (NAA, n = 10), and advanced stage adenoma (AA, n = 10). Each participant provided three types of samples: serum samples to measure inflammatory cytokines IL-6 and IFN-γ via Enzyme-Linked Immunosorbent Assay (ELISA), fecal samples for analyzing gut microbiota composition using 16S rRNA gene sequencing, and intestinal tissue samples for assessing PD-L1 protein expression through Western blotting (Figure 1A). According to the 2020 guidelines by the US Multi-Society Task Force on the endoscopic identification and management of malignant colorectal polyps[6], CRA were classified based on their pathological structural characteristics determined by Hematoxylin and Eosin (H&E) staining. These categories included tubular adenomas (villous component < 25%), villous adenomas (villous component > 75%), and tubulovillous adenomas (villous component between 25 and 75%). For classification purposes, normal samples displayed regular colorectal mucosa, NAA samples exhibited tubular adenomas with a gross specimen length of <10 mm, a villous component <25%, and no high-grade dysplasia, while AA samples showed tubulovillous adenomas with a villous component ranging from 25 to 75% (Figure 1B). Participants were included in the study if they were diagnosed with one of the three groups based on H&E staining (normal, non-advanced adenoma, or advanced stage adenoma). The exclusion criteria consisted of individuals with a history of colorectal polyps or colorectal surgery, a personal or family history of gastrointestinal tumors, inflammatory bowel disease, infectious diseases, metabolic disorders (e.g., diabetes, obesity, and hyperlipidemia), immune system diseases, or severe hepatic or renal conditions.
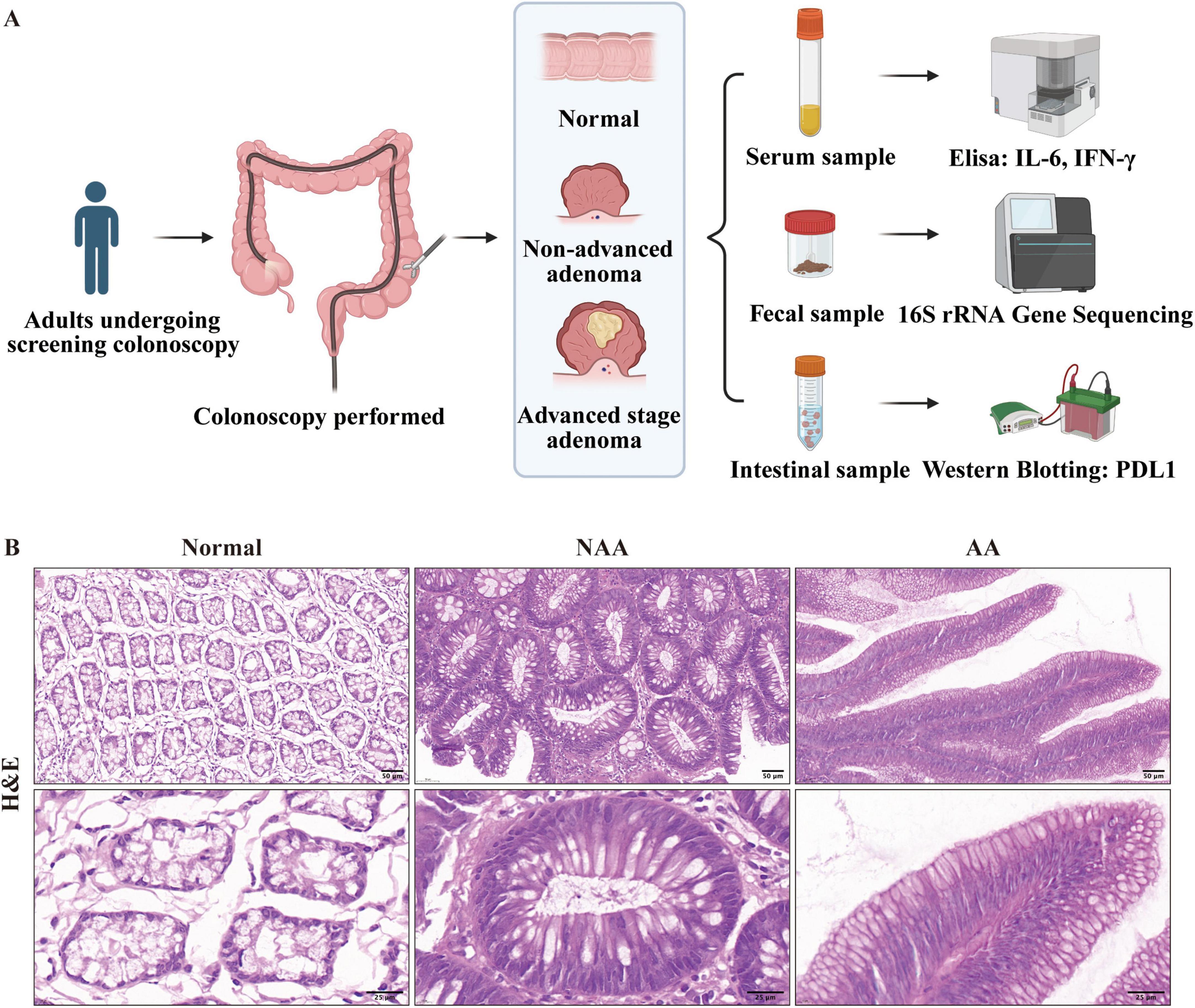
Figure 1. Cohort enrollment flowchart and Representative Hematoxylin and Eosin. (A) Cohort enrollment flowchart (provided by BioRender.com). (B) Representative Hematoxylin and Eosin (H&E) stained sections from each of the normal, NAA, and AA groups (30×, 100× magnification; scale bar: 50, 25 μm). Normal, normal group; NAA, non-advanced adenoma group; AA, advanced stage adenoma group.
2.3 Sample collection
All participants provided two samples of normal colonic mucosa or post-operative specimens, with the exact sampling locations detailed in Supplementary Table 1. One sample was stained with H&E, while the other was preserved in liquid nitrogen for subsequent PD-L1 protein expression detection through Western Blot and Immunohistochemistry (IHC). Prior to taking intestinal cleansing agents, fecal samples (5–10 g) were collected using single-use fecal sampling tubes, quickly frozen in liquid nitrogen for 16S rRNA sequencing. Blood samples (5 ml) were drawn from peripheral veins before colonoscopy, processed by centrifugation (3,000 rpm for 10 min at room temperature), and the serum was promptly frozen in liquid nitrogen for subsequent analysis of IL-6 and IFN-γ levels using ELISA.
2.4 Histology
Evaluated the expression of PD-L1 using IHC. Colorectal tissues were processed by embedding in OCT compound and then rapidly frozen in liquid nitrogen. These tissues were then sectioned into 5 μm slices. Tissue sections were baked overnight in an oven, dewaxed progressively with xylene and anhydrous ethanol, subjected to antigen retrieval with sodium citrate, cooled at room temperature, processed for membrane breakage, and blocked with sheep serum for 30 min. The sections were then incubated overnight with primary antibody, rewarmed for 1 h, incubated with secondary antibody, treated with horseradish peroxidase, visualized by DAB, dehydrated, and sealed. Colorectal sections underwent hematoxylin and eosin (H&E) staining for further examination. Image analysis was performed using ImageJ software.
2.5 Examination of inflammatory factors, and PDL1 level
The levels of serum inflammatory factors (IL-6, and IFN-γ) were determined using a commercial ELISA kit (IL-6, EK106/2-96; IFN-γ, EK180-96, Lianke Biotechnology Co., Ltd., Hangzhou, China) following the manufacturer’s instructions. Evaluated the protein expression of PD-L1 in colorectal tissue using Western blot. Fresh Colorectal tissues were pulverized under liquid nitrogen, and high-efficiency RIPA lysate (catalog number 89900, Thermo) was utilized for protein extraction. The resultant protein samples were then loaded (20 μg/10 μl per well) and resolved via sodium dodecyl sulfate-polyacrylamide gel electrophoresis (SDS-PAGE) on gels comprised of either 10 or 12.5% acrylamide. Subsequently, the separated proteins were transferred onto polyvinylidene fluoride (PVDF) membranes (catalog number ISEQ00010, Millipore). The membranes were then blocked with 5% skim milk in TBST for 2 h at room temperature. Following blocking, the membranes were incubated with primary antibodies targeted against PDL1 (1:1000) and GAPDH (1:1000). After thorough washing and incubation with appropriate secondary antibodies, the blots were visualized using a ChemiDoc XRS imaging system. The quantification of target proteins was achieved by normalizing their relative intensities in the experimental groups to those in the control group.
2.6 DNA extraction and 16S rRNA gene sequencing
Genomic DNA was extracted from fecal samples and the quality and concentration of purified DNA was measured with NanoDrop 2000. The isolated DNA was stored at −20°C for 16S amplified library preparation or qPCR. The hypervariable V3-V4 region of the 16S rRNA gene in 20 ng of DNA was amplified using gene-specific primers:5′ACTCCTACGGGAGGCAGCAG3′, R:5′ GGACTACHVGGGTWTCTAAT 3′. PCR conditions were 95°C for 3 min, 27 cycles of 95°C for 30 s, 55°C for 30 s and 72°C for 45 s, 72°C for 10 min and holding at 4°C. Two steps of amplification were performed to prepare the sequencing library using TransGen AP221-02. Quantification was performed using the QuantiFluor-ST™ (Promega), followed by paired-end sequencing on an Illumina platform.
2.7 16S rRNA gene amplicon sequencing analysis
Fastq files from the Illumina MiSeq run were processed through the QIIME2 pipeline as shown by Bolyen et al. (2019) using the DADA2 plugin (Callahan et al., 2016) to generate amplicon sequence variants (ASVs). Taxonomic classification was performed using the rdp_16S_v18_classifier database (Hung et al., 2022). While genus-level classifications are robust due to the resolution of the V3-V4 region of the 16S rRNA gene, species-level annotations were inferred based on probabilistic matches in the reference database. We performed multivariate testing between treatment groups and further performed post hoc comparisons when significant differences were observed. We determined alpha diversity indices: Pielou’s evenness, Shannon diversity and Observed species. Beta diversity was calculated based on the unweighted unifrac distance metric and visualized using principal co-ordinates analysis (PCoA).
2.8 Linear discriminant analysis and function predict
We identified taxa differentiating the normal, NAA, and AA groups using linear discriminant analysis effect size (LEfSe). LEfSe utilizes a non-parametric Kruskal-Wallis rank sum test to assess differential features with significantly different abundances between assigned taxa and performs LDA to estimate the effect size of each sequence variant as reported by Segata et al. (2011). LDA scores ranking differential taxa are displayed on a LEfSe bar chart according to their effect size. For LEfSe analysis data are first converted to log10 before the non-parametric Kruskal-Wallis rank sum test. The cut-off of the LDA score was set to 2, and significant features were considered with P-values lower than 0.05. To predict the metabolic pathways, phylogenetic investigation of communities by reconstruction of unobserved states (Picrust2) was performed from sequencing data, as shown before (Douglas et al., 2020). Fastq sequence files for each sample were processed using QIIME2. Representative sequences were used as input files for the Picrust2 analysis pipeline. Metabolic pathways were assigned based on the Kyoto Encyclopedia of Genes and Genomes (KEGG) (Langille et al., 2013).
2.9 Statistical analyses
Statistical evaluations in this study were conducted utilizing GraphPad Prism (ver. 9) and R (ver. 4.3.0). The microbial composition at different levels, KEGG as well as indices of alpha diversity were analyzed using Kruskal-Wallis test. Beta diversity was calculated using unweighted UniFrac distance, and a permutational multivariate analysis of variance (PERMANOVA) (Anderson, 2001) was performed in QIIME2 to confirm significant differences between groups. Spearman’s rank correlation method was used to analyze relationships between variables. To ensure consistency, data were preprocessed through normalization and scaling of variables before analysis. The data are presented as the mean ± standard error of the mean (SEM). Differences were deemed statistically significant at a threshold of p < 0.05.
3 Results
3.1 Microbial diversity analysis
3.1.1 Alpha diversity
The study examined changes in α-diversity and β-diversity of the gut microbiota across different stages of CRA using 16S rRNA gene sequencing. For alpha diversity, the observed species showed a slight decrease from the normal group to the NAA group and the AA group, with no statistically significant differences (ns) between the groups, except for a significant reduction (p < 0.05) between the normal and AA groups. The Pielou index was significantly higher in the normal group compared to the NAA and AA groups (p < 0.05). The NAA group exhibited the lowest Pielou index, while the AA group showed a slightly higher value compared to the NAA group, with no statistically significant difference (ns). The Shannon index progressively decreases from the normal group to the NAA group and further to the AA group, with a significant difference (p < 0.05) observed between the normal and AA groups. No statistically significant differences (ns) were observed between the normal and NAA groups or between the NAA and AA groups (Figure 2A).
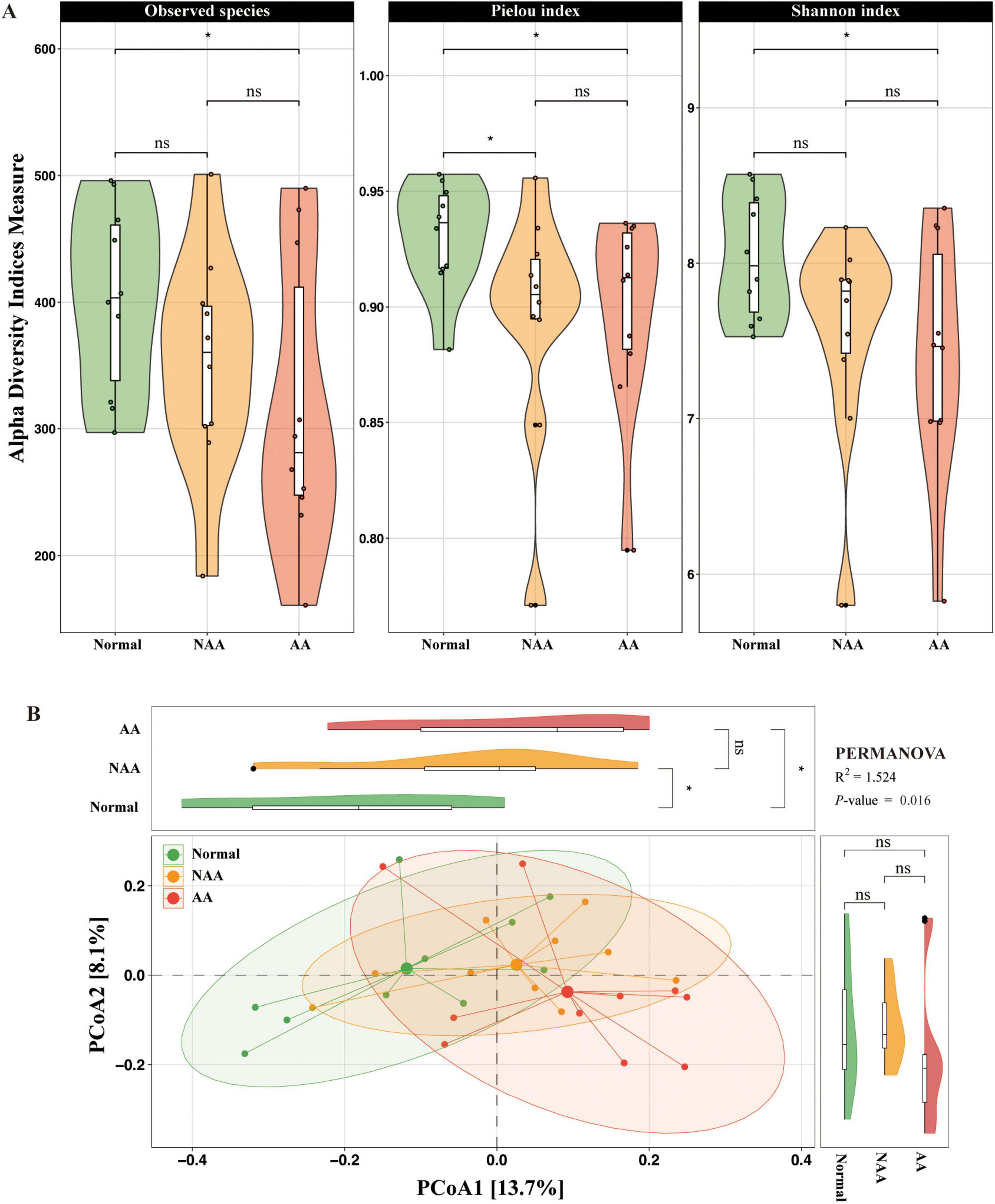
Figure 2. Gut microbiota profiling in normal, NAA, and AA groups. (A) Alpha diversity of microbial communities in normal, NAA, and AA groups, including observed species, Pielou index and Shannon index. (B) Coordination plot of principal co-ordinates analysis (PCoA) of microbial communities among normal, NAA, and AA groups. Normal, normal group; NAA, non-advanced adenoma group; AA, advanced stage adenoma group. The data are presented as means ± SD, n = 10. *P < 0.05.
3.1.2 Beta diversity
Principal co-ordinates analysis was performed at the species level to investigate the gut microbial community structure, revealing significant differences among the normal, NAA, and AA groups (PERMANOVA, R2 = 1.524, P-value = 0.016) (Figure 2B). PCoA1 explained 13.7% of the variation in the microbial community structure, while PCoA2 accounted for 8.1%. These results highlight distinct microbial community profiles across the three groups, with a significant shift from normal to NAA through AA.
3.2 Differential analysis of gut microbiota composition
The LEfSe algorithm was employed to pinpoint the key bacterial taxa responsible for the differences in gut microbiota across the normal, NAA, and AA group phenotypes. Our detailed exploration into the microbial composition at various taxonomic levels, as illustrated in Supplementary Figure 1.
At the family level (Figure 3A), the fecal microbiota primarily comprised three bacterial families: Clostridiaceae 1, Oscillospiraceae, and Turicibacteraceae. Compared to the NAA and AA groups, the gut microbiota of the normal group was enriched with Clostridiaceae 1 and Oscillospiraceae. Furthermore, compared to the AA group, both the normal and NAA groups exhibited enrichment of Turicibacteraceae in the gut microbiome.
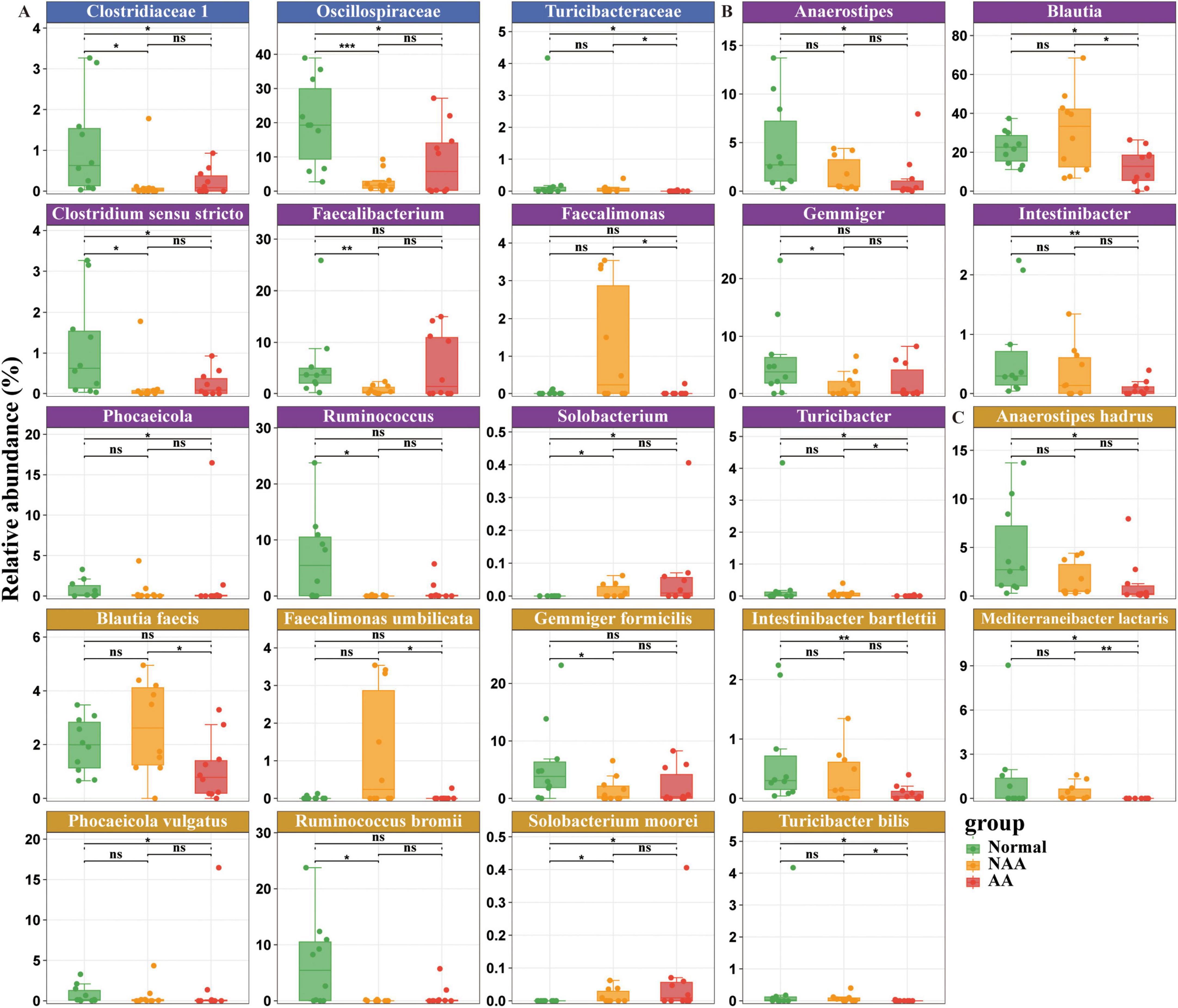
Figure 3. Differential gut microbiota composition at family, genus, species level between normal, NAA, and AA groups. (A) Family level. (B) Genus level. (C) Species level. Normal, normal group; NAA, non-advanced adenoma group; AA, advanced stage adenoma group. The data are presented as means ± SD, n = 10. *P < 0.05; **P < 0.01; ***P < 0.001.
At the genus level (Figure 3B), the fecal microbiota primarily comprised bacteria from the following genera: Anaerostipes, Blautia, Clostridium sensu stricto, Faecalibacterium, Faecalimonas, Gemmiger, Intestinibacter, Phocaeicola, Ruminococcus, Solobacterium, and Turicibacter. Compared to the NAA group, the gut microbiota of the normal group was enriched with Clostridium sensu stricto, Faecalimonas, Gemmiger, and Ruminococcus. In contrast, the NAA group shows an enrichment of Solobacterium when compared to the normal group. Compared to the AA group, the normal group’s gut microbiota is enriched with Anaerostipes, Blautia, Clostridium sensu stricto, Intestinibacter, Phocaeicola, and Turicibacter. Conversely, compared to the normal group, the AA group exhibits an enrichment of Solobacterium. Furthermore, compared to the AA group, the NAA group’s gut microbiota is enriched with Blautia, Faecalimonas, and Turicibacter.
At the species level (Figure 3C), the fecal microbiota primarily includes the following species: Anaerostipes hadrus, Blautia faecis, Faecalimonas umbilicata, Gemmiger formicilis, Intestinibacter bartlettii, Mediterraneibacter lactaris, Phocaeicola vulgatus, Ruminococcus bromii, Solobacterium moorei, and Turicibacter bilis. Compared to the NAA group, the gut microbiota of the normal group is enriched with Gemmiger formicilis and Ruminococcus bromii. Conversely, compared to the normal group, the NAA group shows an enrichment of Solobacterium moorei. Compared to the AA group, the normal group’s gut microbiota is enriched with Anaerostipes hadrus, Intestinibacter bartlettii, Mediterraneibacter lactaris, Phocaeicola vulgatus, and Turicibacter bilis. On the other hand, compared to the normal group, the AA group exhibited an enrichment of Solobacterium moorei. Furthermore, compared to the AA group, the NAA group’s gut microbiota is enriched with Blautia faecis, Faecalimonas umbilicata, Mediterraneibacter lactaris, and Turicibacter bilis.
3.3 Predicted KEGG functional pathways in the gut microbiome
In the normal vs. NAA comparison (Figure 4A), 11 KEGG pathways showed significant differences. The NAA group exhibited upregulation in Nucleocytoplasmic transport, Arginine and proline metabolism, Pentose and glucuronate interconversions, and Galactose metabolism. Downregulated pathways included Terpenoid backbone biosynthesis, Aminoacyl-tRNA biosynthesis, Protein export, Pyrimidine metabolism, Carbon fixation pathways in prokaryotes, Zeatin biosynthesis, and Protein processing in the endoplasmic reticulum.
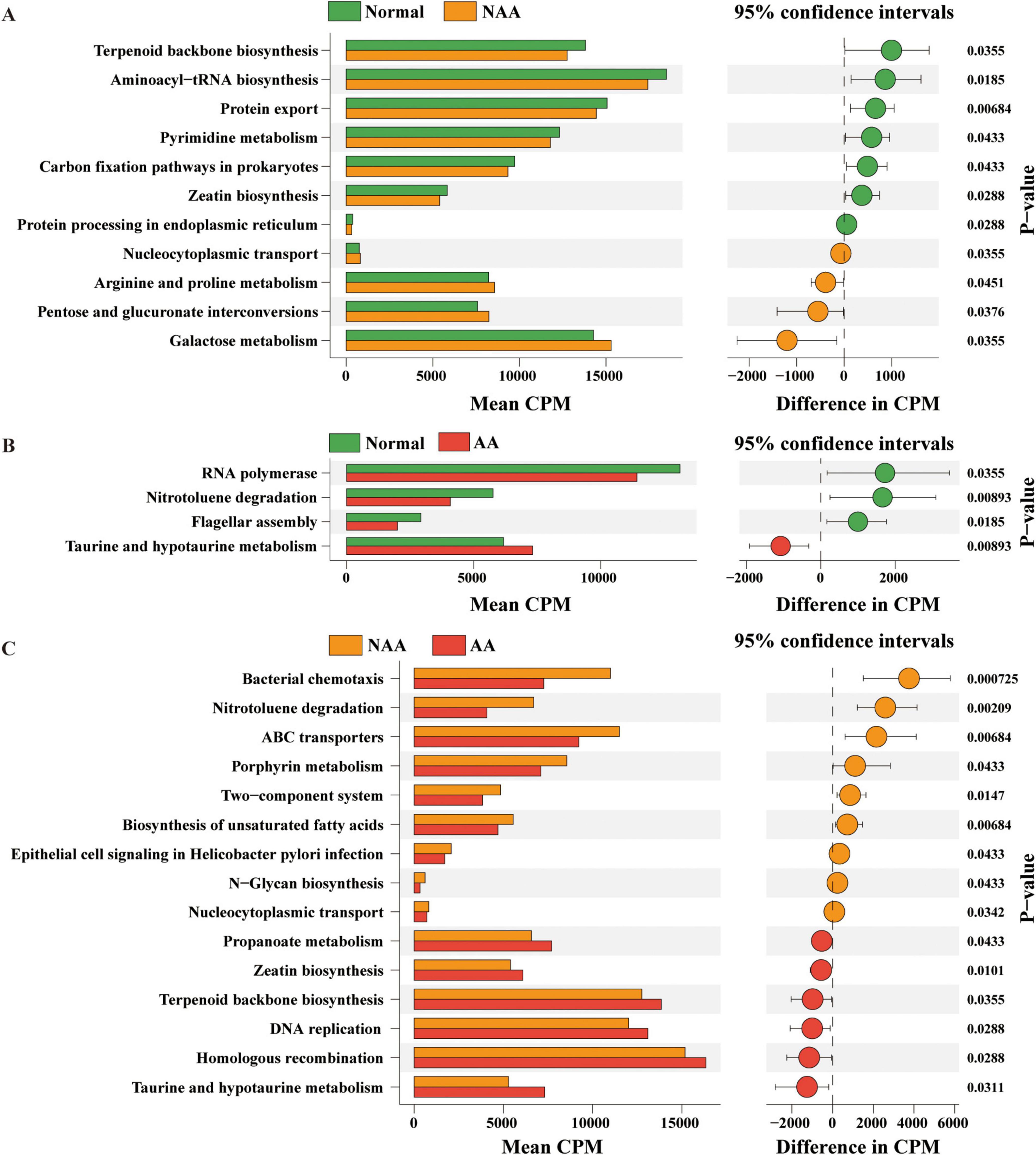
Figure 4. Kyoto Encyclopedia of Genes and Genomes (KEGG) functional pathways predicted in the gut microbiome of normal, NAA, and AA groups using Picrust2. (A) Linear discriminant analysis of predicted microbial metabolic functions between normal and NAA. (B) Linear discriminant analysis of predicted microbial metabolic functions between normal and AA. (C) Linear discriminant analysis of predicted microbial metabolic functions between NAA and AA. Selection of discriminative microbial pathways between groups were based on an LDA score cutoff of 3.0 and differences in the relative abundances of pathway (converted to log base 10) were statistically determined based on a Kruskal-Wallis and pairwise Wilcoxon tests. A p-value of <0.05 and a score ≥3.0 were considered significant in Kruskal-Wallis and pairwise Wilcoxon tests, respectively, at a significance level of 0.05; n = 10. Normal, normal group; NAA, non-advanced adenoma group; AA, advanced stage adenoma group.
In the normal vs. AA comparison (Figure 4B), three KEGG pathways showed significant differences. The AA group exhibited upregulation in Taurine and hypotaurine metabolism and downregulation in RNA polymerase, Nitrotoluene degradation, and Flagellar assembly.
In the NAA vs. AA comparison (Figure 4C), 15 KEGG pathways showed significant differences. The AA group exhibited upregulation in Propanoate metabolism, Zeatin biosynthesis, Terpenoid backbone biosynthesis, DNA replication, Homologous recombination, and Taurine and hypotaurine metabolism. Downregulated pathways included Bacterial chemotaxis, Nitrotoluene degradation, ABC transporters, Porphyrin metabolism, Two-component system, Biosynthesis of unsaturated fatty acids, Epithelial cell signaling in Helicobacter pylori infection, N-Glycan biosynthesis, and Nucleocytoplasmic transport.
3.4 Correlations between the microbial pathways and gut microbes
Figure 5 provides an analysis of the correlations between various bacterial taxa and functional metabolic pathways in the gut microbiota at different stages of CRA (normal, NAA, AA). The heatmap organizes these taxa into two phyla, Bacillota and Bacteroidota. Significant positive correlations are observed for taxa like Blautia, Blautia faecis, Anaerostipes, and Anaerostipes hadrus with metabolic pathways such as Taurine and hypotaurine metabolism, Propanoate metabolism, and Zeatin biosynthesis. On the other hand, taxa such as Faecalimonas, Faecalimonas umbilicata, Turicibacteraceae, Turicibacter, and Turicibacter bilis are negatively correlated with pathways including Aminoacyl-tRNA biosynthesis, Terpenoid backbone biosynthesis, and Flagellar assembly.
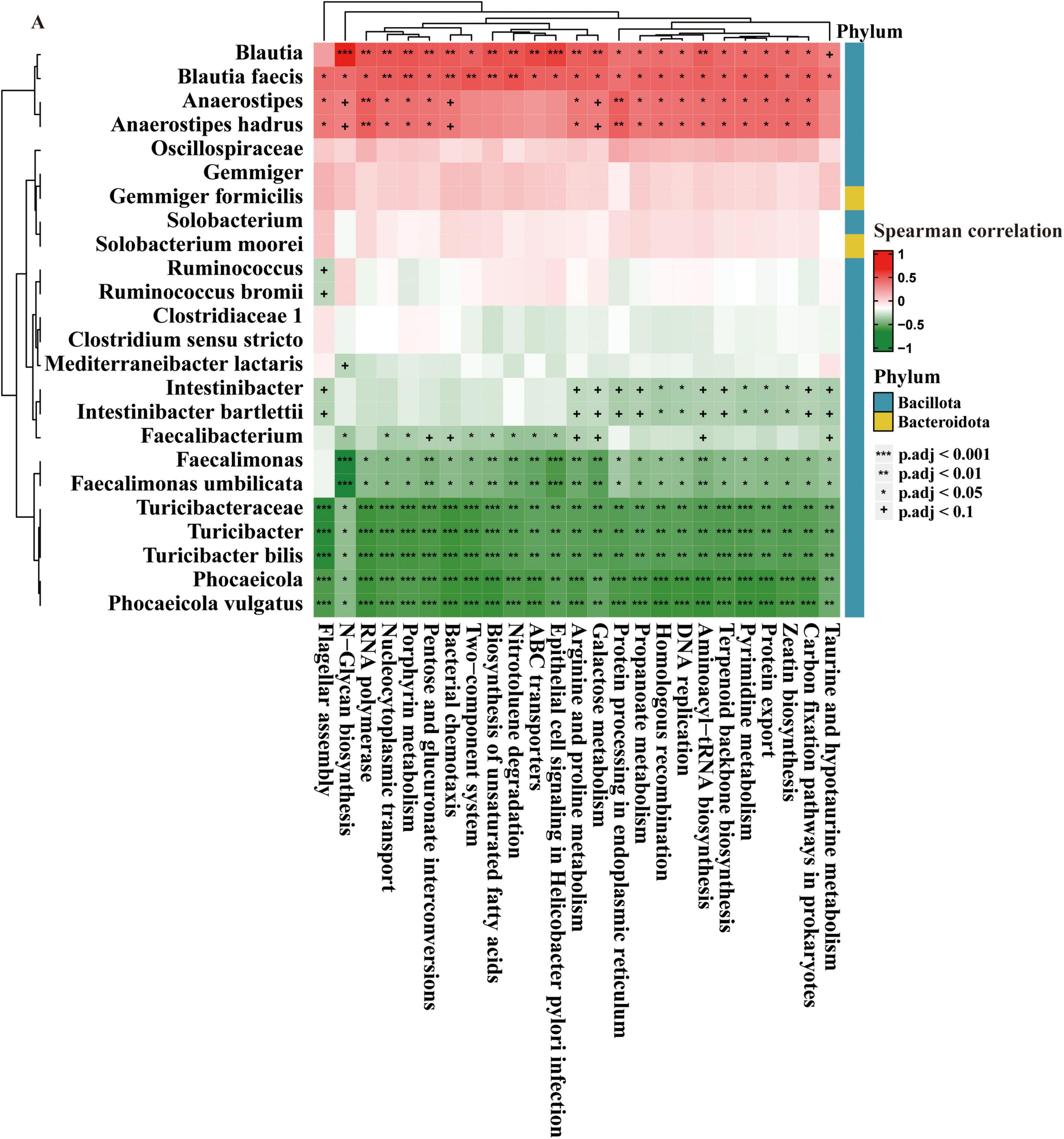
Figure 5. Correlation of gut microbes and microbial pathways. The data are presented as means ± SD, n = 10. +P < 0.1; *P < 0.05; **P < 0.01; ***P < 0.001.
3.5 PD-L1 expression and correlation of IL-6, IFN-γ, and gut microbes
Immunohistochemistry revealed increasing PD-L1 expression across the groups: normal (+), NAA (++), and AA (+++) (Figure 6A). Western blot analysis confirmed this trend, showing a progressive rise in PD-L1 levels from normal to AA (Figure 6B). Negative correlations were found between IFN-γ and Faecalimonas, Faecalimonas umbilicata, Solobacterium, and Solobacterium moorei (p < 0.1). Positive correlations were identified between IL-6 and Gemmiger, Gemmiger formicilis, Anaerostipes, and Anaerostipes hadrus (p < 0.05 for Gemmiger and Gemmiger formicilis, p < 0.1 for others) (Figure 6C).
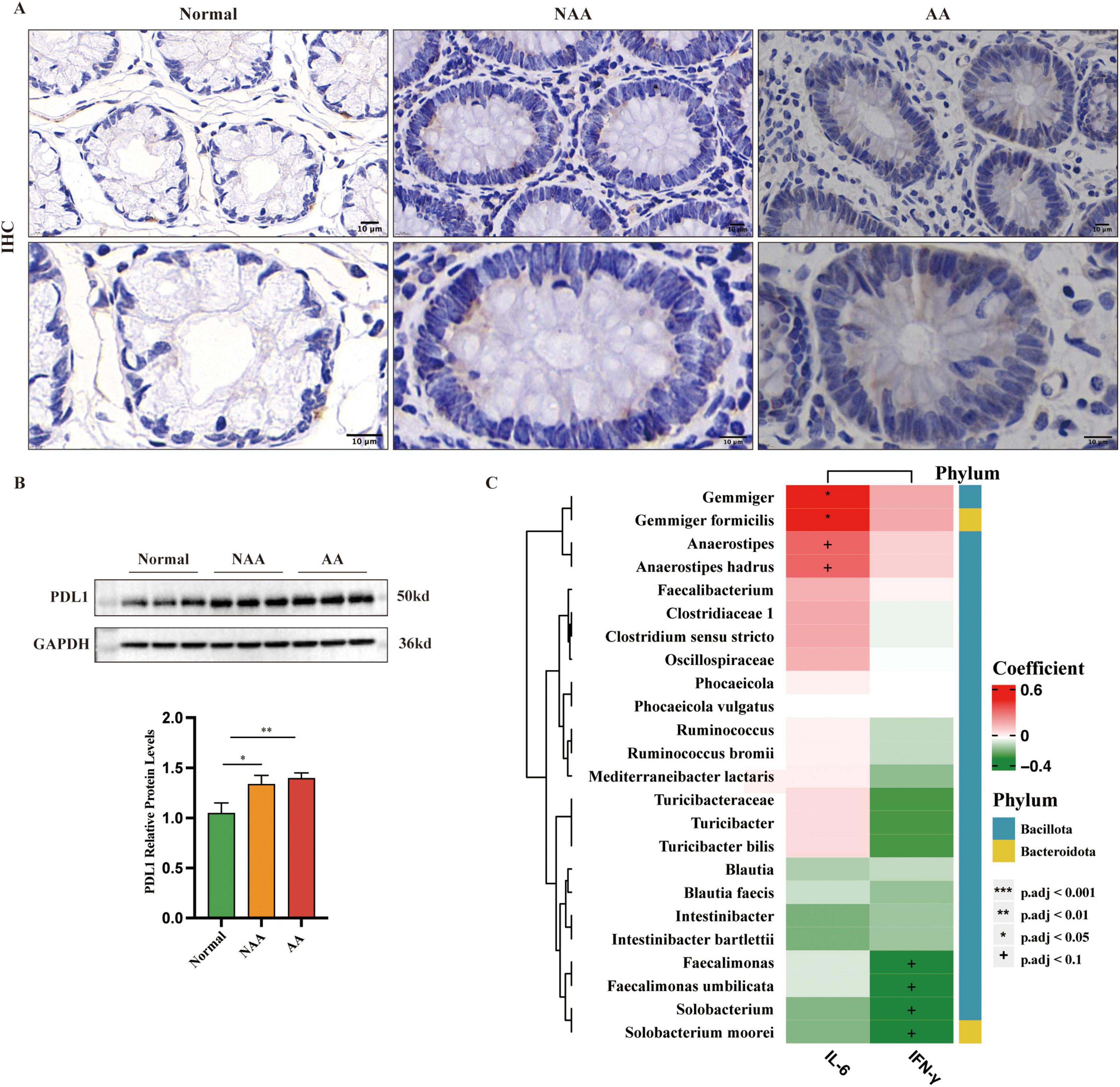
Figure 6. Expression of PDL1 as detected by immunohistochemistry (IHC) and western blot and correlations between the abundance of IL-6, IFN-γ and gut microbes. (A). IHC stained sections from each of the normal, NAA, and AA groups. Membranous or cytoplasmic staining in shades of brown or yellow-brown indicates positive PD-L1 expression (100×, 200× magnification; scale bar: 10 μm). (B) Protein expression of PDL1 as detected by western blot (n = 5). (C) Correlations between the abundance of IL-6, IFN-γ and gut microbes. Normal, normal group; NAA, non-advanced adenoma group; AA, advanced stage adenoma group. Data were analyzed using one-way ANOVA. The data are presented as means ± SD, n = 10. +P < 0.1; *P < 0.05; **P < 0.01; ***P < 0.001.
4 Discussion
This study adds to the growing body of evidence linking the gut microbiome to CRC, with a specific focus on the precancerous stage of adenomas. By examining tissue PD-L1 protein expression, serum levels of inflammatory cytokines (IL-6, IFN-γ), and fecal gut microbiota composition across various stages of CRA, including normal, NAA, and AA, our research aimed to identify biomarkers indicative of significant changes during adenoma progression. Our research aims to identify biomarkers that signify important changes during adenoma progression and provide scientific support for dietary interventions by elucidating the functional roles of microbial taxa involved.
The observed reduction in α-diversity within the fecal microbiome associated with adenomas aligns with findings from Peters et al. (2016) who reported similar trends. However, contrasting results from Feng et al. (2015) study, which showed higher diversity in pre-tumor and tumor groups, highlight the complex nature of microbiome alterations during adenoma development. This discrepancy suggests that the relationship between microbiota diversity and adenoma progression may be influenced by additional factors, such as genetic predispositions, environmental influences, and lifestyle choices, that warrant further investigation. Specifically, dietary habits are known to play a crucial role in shaping gut microbiota composition. For instance, a high-fiber diet may enhance the diversity of beneficial microbial populations (Yang et al., 2021), while a Western-style diet may lead to an overrepresentation of pro-inflammatory bacteria (Shin et al., 2021). These dietary factors likely contribute to the observed differences in microbiota diversity and may be a key factor in adenoma progression. Notably, participants with the highest dietary fiber intake, particularly from cereals and fruits, had a lower risk of colorectal adenoma and distal colon cancer (Kunzmann et al., 2015), underscoring its protective role in CRC prevention.
The gut microbiota in the normal group was characterized by the enrichment of Anaerostipes, Blautia, Gemmiger, and Ruminococcus. Among them, higher levels of Gemmiger are associated with Western dietary patterns, while its reduced abundance in CRC patients exhibiting symptoms like bloody stools suggests a potential influence of diet on its prevalence (Chénard et al., 2020; Hur et al., 2022). Similarly, the positive correlation between dietary fiber intake and the higher population of Ruminococcus highlights its role in the fermentation of complex carbohydrates, emphasizing the significant impact of diet on gut microbiota composition and colorectal health. Recent studies have further demonstrated that specific dietary fibers may particularly promote the growth of beneficial bacteria such as Ruminococcus, further reinforcing the critical role of diet in CRC prevention (Abell et al., 2008; Abell et al., 2011).
Anaerostipes and Blautia are recognized as key producers of short-chain fatty acids (SCFAs), including acetate and butyrate. Butyrate, as a primary energy source, plays a crucial role in promoting the proliferation and repair of intestinal epithelial cells and exhibits strong anti-inflammatory effects by suppressing the nuclear factor-κB (NF-κB) signaling pathway. These bacteria generate SCFAs through the metabolism of lactate and acetate, as well as the fermentation of sugars and dietary fibers, which are essential for maintaining intestinal barrier integrity and controlling inflammation (Katsaounou et al., 2023; Maki et al., 2022; Song et al., 2023). In addition, Anaerostipes and Blautia are positively correlated with several important metabolic pathways, such as taurine and hypotaurine metabolism, propanoate metabolism, and zeatin biosynthesis. Emerging evidence highlights the role of taurine metabolism in regulating bile acid composition and enhancing gut microbiota diversity, which are pivotal in preventing the progression of adenomas (Duszka, 2022; Xu et al., 2021).
The abundance of these bacteria in the normal group decreases significantly during the NAA stage, with Blautia initially showing a slight higher before a marked reduction in the AA stage. This pattern indicates a gradual loss of protective bacterial functions and metabolic activities as adenomas progress. Furthermore, recent studies suggest that the metabolic capacity of the gut microbiota may undergo compensatory enhancement during the early stages of adenoma but collapse in later stages due to the intensification of the inflammatory environment and metabolic dysregulation. These findings highlight the importance of early intervention, particularly through dietary modulation, to support the growth of Anaerostipes and Blautia. Such interventions may serve as potential strategies for preventing adenoma progression. These findings emphasize the importance of early intervention, particularly through dietary modulation, to promote the growth of Anaerostipes and Blautia. Such interventions could be effective strategies for preventing adenoma progression.
In contrast, the NAA and AA groups were characterized by an enrichment of Solobacterium. This bacterium was more prevalent in the NAA group compared to the normal group. Its elevation in adenomatous polyps and negative correlation with IFN-γ suggest that Solobacterium may promote polyp progression via the NF-κB signaling pathway, potentially contributing to chronic inflammation and compromised intestinal barriers (Yu et al., 2024). These findings align with recent studies indicating that Solobacterium induces pro-inflammatory responses, making it a promising target for microbiome-based therapeutic interventions aimed at preventing adenoma progression.
PD-L1, a critical factor in tumor immune escape by inhibiting T-cell function, was assessed across different stages of CRA development. While some studies report elevated PD-L1 in high-grade adenomas and early invasive cancers, others find lower levels in progressive adenomas compared to benign adenomas (Gatenbee et al., 2022; Miller et al., 2018). Our study confirmed that PD-L1 levels higher with adenoma progression, yet the expression dynamics from normal tissue to CRC remain unclear, necessitating further investigation. Additionally, we found negative associations between Faecalimonas, Faecalimonas umbilicata, Solobacterium, and Solobacterium moorei with IFN-γ, and positive associations of Gemmiger, Gemmiger formicilis, Anaerostipes, and Anaerostipes hadrus with IL-6. These mixed findings regarding PD-L1 suggest a complex regulatory environment within the tumor microenvironment that warrants further study. Understanding these dynamics could lead to more targeted immunotherapies for CRC, potentially improving patient outcomes.
The modulation of diet to encourage the growth of beneficial bacteria, such as Anaerostipes and Blautia, is a promising strategy for slowing the progression of CRA and enhancing colorectal health. However, the primary challenge in dietary therapy is determining how to implement effective dietary adjustments, particularly in the early stages. A crucial aspect of this is the development of personalized dietary interventions as part of individualized treatment. A core question in clinical practice is how to use diet to alter the composition of the gut microbiome. Certain dietary components—such as dietary fiber, antioxidants, and fatty acids—may affect different populations in varying ways. For example, dietary fiber promotes the growth of beneficial bacteria and strengthens intestinal barrier function, while antioxidant-rich foods can help reduce inflammation. Despite the evident potential of dietary interventions in preventing CRA and CRC, the clinical application of such therapies faces several challenges. In traditional healthcare systems, dietary therapy is often underappreciated, and there is a lack of comprehensive understanding among clinicians. Additionally, patient compliance presents a significant barrier to the widespread adoption of dietary interventions. Future research should focus on further clarifying the relationships between dietary components, gut microbiota, and metabolic pathways. It should also assess the impact of different dietary strategies on gut health and explore their practical applications in clinical treatment.
This study has several limitations that warrant consideration. First, the relatively small sample size and single-center design may introduce selection bias and restrict the generalizability of the findings. Second, the reliance on 16S rRNA sequencing, particularly the V3-V4 region, constrains the taxonomic resolution to the genus level for most taxa, with species-level identifications being inferred probabilistically rather than determined definitively. The KEGG functional pathway analysis in this study was conducted using PICRUSt2 predictions, which are computational inferences rather than direct measurements. To address these limitations, future studies should include larger, more diverse cohorts and utilize advanced sequencing technologies to improve taxonomic resolution. Additionally, further research should focus on elucidating the mechanistic pathways that link specific microbial taxa to adenoma progression, providing deeper insights into their functional roles.
5 Conclusion
The study emphasizes the critical role of the gut microbiota in colorectal adenoma progression, highlighting key microbial taxa and metabolic pathways as potential biomarkers for early detection and therapeutic intervention. Early dietary modulation to promote beneficial bacteria such as Anaerostipes and Blautia could serve as a promising strategy to mitigate adenoma progression and improve colorectal health.
Data availability statement
The original contributions presented in this study are included in this article/Supplementary material, further inquiries can be directed to the corresponding authors. Sequences analyzed in this study are available in GenBank with the accession number PRJNA1107827.
Ethics statement
The studies involving humans were approved by the Ethics Committees of the First Affiliated Hospital of Kunming Medical University. This study was approved by the Ethics Committees of the First Affiliated Hospital of Kunming Medical University (project license number: L-26/2022). The studies were conducted in accordance with the local legislation and institutional requirements. The participants provided their written informed consent to participate in this study.
Author contributions
XW: Writing – original draft, Writing – review and editing. HC: Writing – original draft, Writing – review and editing. MY: Conceptualization, Writing – original draft. MH: Conceptualization, Data curation, Investigation, Writing – original draft. DZ: Methodology, Writing – original draft. ML: Conceptualization, Writing – original draft. HW: Conceptualization, Investigation, Writing – original draft. QZ: Conceptualization, Writing – original draft. LL: Conceptualization, Writing – original draft. YL: Conceptualization, Writing – original draft. JY: Writing – original draft, Writing – review and editing. LM: Writing – original draft, Writing – review and editing.
Funding
The author(s) declare that financial support was received for the research and/or publication of this article. This work was supported by grants from the National Natural Science Foundation of China (Nos. 82160117 and 81760108), Scientific Research Fund Project of Yunnan Education Department (No. 2024Y223), Joint fund of Yunnan Provincial Science and Technology Department and Kunming Medical University (No. 202401AY070001-009), Kunming Medical University Graduate Student Innovation Fund (No. 2024S045), First-Class Discipline Team of Kunming Medical University (No. 2024XKTDYS02), and Doctoral Service Industry Research and Innovation Cultivation Project (No. FWCY-BSPY2024082).
Acknowledgments
We would like to extend our gratitude to BioRender for providing the tools that significantly aided in the creation of scientific illustrations for this publication.
Conflict of interest
The authors declare that the research was conducted in the absence of any commercial or financial relationships that could be construed as a potential conflict of interest.
Generative AI statement
The authors declare that no Generative AI was used in the creation of this manuscript.
Publisher’s note
All claims expressed in this article are solely those of the authors and do not necessarily represent those of their affiliated organizations, or those of the publisher, the editors and the reviewers. Any product that may be evaluated in this article, or claim that may be made by its manufacturer, is not guaranteed or endorsed by the publisher.
Supplementary material
The Supplementary Material for this article can be found online at: https://www.frontiersin.org/articles/10.3389/fmicb.2025.1556056/full#supplementary-material
References
Abell, G., Cooke, C., Bennett, C., Conlon, M., and McOrist, A. (2008). Phylotypes related to Ruminococcus bromii are abundant in the large bowel of humans and increase in response to a diet high in resistant starch. FEMS Microbiol. Ecol. 66, 505–515. doi: 10.1111/j.1574-6941.2008.00527.x
Adak, A., and Khan, M. (2019). An insight into gut microbiota and its functionalities. Cell. Mol. Life Sci. 76, 473–493. doi: 10.1007/s00018-018-2943-4
Anderson, M. J. (2001). A new method for non-parametric multivariate analysis of variance. Austral Ecol. 26, 32–46. doi: 10.1111/j.1442-9993.2001.01070.pp.x
Bolyen, E., Rideout, J., Dillon, M., Bokulich, N., Abnet, C., Al-Ghalith, G., et al. (2019). Reproducible, interactive, scalable and extensible microbiome data science using QIIME 2. Nat. Biotechnol. 37, 852–857. doi: 10.1038/s41587-019-0209-9
Callahan, B., McMurdie, P., Rosen, M., Han, A., Johnson, A., and Holmes, S. (2016). DADA2: High-resolution sample inference from Illumina amplicon data. Nat. Methods 13, 581–583. doi: 10.1038/nmeth.3869
Cao, Y., Liang, W., Fang, L., Liu, M., Zuo, J., Peng, Y., et al. (2022). PD-L1/PD-L1 signalling promotes colorectal cancer cell migration ability through RAS/MEK/ERK. Clin. Exp. Pharmacol. Physiol. 49, 1281–1293. doi: 10.1111/1440-1681.13717
Chen, J., Pitmon, E., and Wang, K. (2017). Microbiome, inflammation and colorectal cancer. Semin. Immunol. 32, 43–53. doi: 10.1016/j.smim.2017.09.006
Chénard, T., Malick, M., Dubé, J., and Massé, E. (2020). The influence of blood on the human gut microbiome. BMC Microbiol. 20:44. doi: 10.1186/s12866-020-01724-8
Conteduca, V., Sansonno, D., Russi, S., and Dammacco, F. (2013). Precancerous colorectal lesions (Review). Int. J. Oncol. 43, 973–984. doi: 10.3892/ijo.2013.2041
Douglas, G., Maffei, V., Zaneveld, J., Yurgel, S., Brown, J., Taylor, C., et al. (2020). PICRUSt2 for prediction of metagenome functions. Nat. Biotechnol. 38, 685–688. doi: 10.1038/s41587-020-0548-6
Duszka, K. (2022). Versatile triad alliance: Bile acid, taurine and microbiota. Cells 11:2337. doi: 10.3390/cells11152337
Feng, Q., Liang, S., Jia, H., Stadlmayr, A., Tang, L., Lan, Z., et al. (2015). Gut microbiome development along the colorectal adenoma-carcinoma sequence. Nat. Commun. 6:6528. doi: 10.1038/ncomms7528
Flemer, B., Lynch, D., Brown, J., Jeffery, I., Ryan, F., Claesson, M., et al. (2017). Tumour-associated and non-tumour-associated microbiota in colorectal cancer. Gut 66, 633–643. doi: 10.1136/gutjnl-2015-309595
Freitas, J., Gullo, I., Garcia, D., Miranda, S., Spaans, L., Pinho, L., et al. (2021). The adaptive immune landscape of the colorectal adenoma-carcinoma sequence. Int. J. Mol. Sci. 22:9791. doi: 10.3390/ijms22189791
Fu, X., Xu, Y., Han, X., Lin, X., Wang, J., Li, G., et al. (2025). Exploring the mechanism of canmei formula in preventing and treating recurrence of colorectal adenoma based on data mining and algorithm prediction. Biol. Proced. Online 27:4. doi: 10.1186/s12575-025-00266-5
Gatenbee, C., Baker, A., Schenck, R., Strobl, M., West, J., Neves, M., et al. (2022). Immunosuppressive niche engineering at the onset of human colorectal cancer. Nat. Commun. 13:1798. doi: 10.1038/s41467-022-29027-8
Hong, W., Dong, L., Stock, S., Basharat, Z., Zippi, M., and Zhou, M. (2018). Prevalence and characteristics of colonic adenoma in mainland China. Cancer Manag. Res. 10, 2743–2755. doi: 10.2147/CMAR.S166186
Huang, W., Berndt, S., Shiels, M., Katki, H., Chaturvedi, A., Wentzensen, N., et al. (2019). Circulating inflammation markers and colorectal adenoma risk. Carcinogenesis 40, 765–770. doi: 10.1093/carcin/bgz027
Hung, Y., Lyu, W., Tsai, M., Liu, C., Lai, L., Tsai, M., et al. (2022). To compare the performance of prokaryotic taxonomy classifiers using curated 16S full-length rRNA sequences. Comput. Biol. Med. 145:105416. doi: 10.1016/j.compbiomed.2022.105416
Hur, H., Wu, X., Yang, H., Kim, M., Lee, K., Hong, M., et al. (2022). Beneficial effects of a low-glycemic diet on serum metabolites and gut microbiota in obese women with prevotella and bacteriodes enterotypes: A randomized clinical trial. Front. Nutr. 9:861880. doi: 10.3389/fnut.2022.861880
Abell, G. C., Christophersen, C. T., McOrist, A. L., and Clarke, J. M. (2011). Dietary resistant and butyrylated starches have different effects on the faecal bacterial flora of azoxymethane-treated rats. Br. J. Nutr. 105, 1480–1485. doi: 10.1017/S0007114510005349
Katsaounou, K., Yiannakou, D., Nikolaou, E., Brown, C., Vogazianos, P., Aristodimou, A., et al. (2023). Fecal microbiota and associated volatile organic compounds distinguishing no-adenoma from high-risk colon adenoma adults. Metabolites 13:819. doi: 10.3390/metabo13070819
Kunzmann, A., Coleman, H., Huang, W., Kitahara, C., Cantwell, M., and Berndt, S. (2015). Dietary fiber intake and risk of colorectal cancer and incident and recurrent adenoma in the prostate, lung, colorectal, and ovarian cancer screening trial. Am. J. Clin. Nutr. 102, 881–890. doi: 10.3945/ajcn.115.113282
Langille, M., Zaneveld, J., Caporaso, J., McDonald, D., Knights, D., Reyes, J., et al. (2013). Predictive functional profiling of microbial communities using 16S rRNA marker gene sequences. Nat. Biotechnol. 31, 814–821. doi: 10.1038/nbt.2676
Liang, J., Li, T., Nakatsu, G., Chen, Y., Yau, T., Chu, E., et al. (2020). A novel faecal Lachnoclostridium marker for the non-invasive diagnosis of colorectal adenoma and cancer. Gut 69, 1248–1257. doi: 10.1136/gutjnl-2019-318532
Lim, S., Li, C., Xia, W., Cha, J., Chan, L., Wu, Y., et al. (2016). Deubiquitination and Stabilization of PD-L1 by CSN5. Cancer Cell. 30, 925–939. doi: 10.1016/j.ccell.2016.10.010
Maki, J., Lippolis, J., and Looft, T. (2022). Proteomic response of Turicibacter bilis MMM721 to chicken bile and its bile acids. BMC Res. Notes 15:236. doi: 10.1186/s13104-022-06127-8
Mang, T. (2019). [Colorectal cancer: Role of imaging in screening]. Radiologe 59, 23–34. doi: 10.1007/s00117-018-0479-0
Miller, T., Mccoy, M., Hemmings, C., Iacopetta, B., and Platell, C. (2018). Expression of PD-L1 and SOX2 during rectal tumourigenesis: Potential mechanisms for immune escape and tumour cell invasion. Oncol. Lett. 16, 5761–5768. doi: 10.3892/ol.2018.9337
Munteanu, C., and Schwartz, B. (2024). Interactions between dietary antioxidants, dietary fiber and the gut microbiome: Their putative role in inflammation and cancer. Int. J. Mol. Sci. 25:8250. doi: 10.3390/ijms25158250
Pan, J., Cen, L., Xu, L., Miao, M., Li, Y., Yu, C., et al. (2020). Prevalence and risk factors for colorectal polyps in a Chinese population: A retrospective study. Sci. Rep. 10:6974. doi: 10.1038/s41598-020-63827-6
Peters, B., Dominianni, C., Shapiro, J., Church, T., Wu, J., Miller, G., et al. (2016). The gut microbiota in conventional and serrated precursors of colorectal cancer. Microbiome 4:69. doi: 10.1186/s40168-016-0218-6
Segata, N., Izard, J., Waldron, L., Gevers, D., Miropolsky, L., Garrett, W., et al. (2011). Metagenomic biomarker discovery and explanation. Genome Biol. 12:R60. doi: 10.1186/gb-2011-12-6-r60
Sepich-Poore, G., Zitvogel, L., Straussman, R., Hasty, J., Wargo, J., and Knight, R. (2021). The microbiome and human cancer. Science 371:eabc4552. doi: 10.1126/science.abc4552
Shi, Y., Li, Z., Zheng, W., Liu, X., Sun, C., Laugsand, J., et al. (2015). Changes of immunocytic phenotypes and functions from human colorectal adenomatous stage to cancerous stage: Update. Immunobiology 220, 1186–1196. doi: 10.1016/j.imbio.2015.06.003
Shin, J., Ahn, Y., Chung, W., Lim, M., Hong, S., Kim, J., et al. (2021). Effect of saengshik supplementation on the gut microbial composition of healthy korean adults: A single-group pilot study. Front. Nutr. 8:743620. doi: 10.3389/fnut.2021.743620
Song, C., Kim, N., Nam, R., Choi, S., Jang, J., Kim, E., et al. (2023). The possible preventative role of lactate- and butyrate-producing bacteria in colorectal carcinogenesis. Gut Liver 18, 654–666. doi: 10.5009/gnl230385
Song, M., Wu, K., Meyerhardt, J., Ogino, S., Wang, M., Fuchs, C., et al. (2018). Fiber intake and survival after colorectal cancer diagnosis. JAMA Oncol. 4, 71–79. doi: 10.1001/jamaoncol.2017.3684
Sung, H., Ferlay, J., Siegel, R., Laversanne, M., Soerjomataram, I., Jemal, A., et al. (2021). Global cancer statistics 2020: Globocan estimates of incidence and mortality worldwide for 36 cancers in 185 countries. CA Cancer J. Clin. 71, 209–249. doi: 10.3322/caac.21660
Wu, M., Huang, Q., Xie, Y., Wu, X., Ma, H., Zhang, Y., et al. (2022). Improvement of the anticancer efficacy of PD-1/PD-L1 blockade via combination therapy and PD-L1 regulation. J. Hematol. Oncol. 15:24. doi: 10.1186/s13045-022-01242-2
Xu, J., Yu, X., Ye, H., Gao, S., Deng, N., Lu, Y., et al. (2021). Comparative metabolomics and proteomics reveal vibrio parahaemolyticus targets hypoxia-related signaling pathways of takifugu obscurus. Front. Immunol. 12:825358. doi: 10.3389/fimmu.2021.825358
Yang, W., Liu, Y., Yang, G., Meng, B., Yi, Z., Yang, G., et al. (2021). Moderate-intensity physical exercise affects the exercise performance and gut microbiota of mice. Front. Cell Infect. Microbiol. 11:712381. doi: 10.3389/fcimb.2021.712381
Yu, S., Wang, X., Li, Z., Jin, D., Yu, M., Li, J., et al. (2024). Solobacterium moorei promotes the progression of adenomatous polyps by causing inflammation and disrupting the intestinal barrier. J. Transl. Med. 22:169. doi: 10.1186/s12967-024-04977-3
Zheng, R., Sun, K., Zhang, S., Zeng, H., Zou, X., Chen, R., et al. (2019). [Report of cancer epidemiology in China, 2015]. Zhonghua Zhong Liu Za Zhi. 41, 19–28. doi: 10.3760/cma.j.issn.0253-3766.2019.01.005
Keywords: colorectal adenoma, colorectal cancer, microbiota, PD-L1, IL-6, IFN-γ
Citation: Wang X, Chen H, Yang M, Huang M, Zhang D, Li M, Wang H, Zhou Q, Lu L, Li Y, Yu J and Ma L (2025) Influence of gut microbiota and immune markers in different stages of colorectal adenomas. Front. Microbiol. 16:1556056. doi: 10.3389/fmicb.2025.1556056
Received: 06 January 2025; Accepted: 24 March 2025;
Published: 16 April 2025.
Edited by:
Yunkyoung Lee, Jeju National University, Republic of KoreaReviewed by:
Wanli Zhao, Chinese Academy of Sciences, ChinaJing Liu, University of Pennsylvania, United States
Yuting Ke, Massachusetts Institute of Technology, United States
Copyright © 2025 Wang, Chen, Yang, Huang, Zhang, Li, Wang, Zhou, Lu, Li, Yu and Ma. This is an open-access article distributed under the terms of the Creative Commons Attribution License (CC BY). The use, distribution or reproduction in other forums is permitted, provided the original author(s) and the copyright owner(s) are credited and that the original publication in this journal is cited, in accordance with accepted academic practice. No use, distribution or reproduction is permitted which does not comply with these terms.
*Correspondence: Lanqing Ma, bWFsYW5xaW5nQGttbXUuZWR1LmNu; Jiangkun Yu, eWprNTU1QHludS5lZHUuY24=
†These authors have contributed equally to this work