- 1College of Veterinary Medicine, Northwest A&F University, Xianyang, China
- 2Key Laboratory of Animal Biotechnology, Ministry of Agriculture and Rural Affairs, Northwest A&F University, Xianyang, China
The gut bacterial community is essential for maintaining lifelong health and productivity in ruminants, but the relationship between the gut microbiota and the sustained productivity of ruminants remains inadequately understood. In this study, we selected long-lived dairy cows in mid-lactation (≥5 parities) with different levels of milk production (n = 10). Significant differences were observed in the rumen bacterial structures between the two groups of dairy cows, whereas no significant differences were detected in the rectum bacterial communities. Additionally, there were no significant differences in serum oxidative stress biomarkers, inflammatory markers, or immunological markers between the long-lived high-yield (LH) and long-lived low-yield (LL) dairy cows. Furthermore, the concentrations of propionate (Pr) in the rumen and butyrate (Bu) in the rectum were elevated in the high-yield group. Spearman correlation and microbial co-occurrence network analyses revealed that several rumen-enriched bacteria, such as Syntrophococcus, Lachnospira, Shuttleworthia, Erysipelotrichaceae_UCG-2, and Roseburiaare associated with rumen propionate (Pr) production. In the rectum, the reduced abundance of Christensenellaceae_R-7_group and Moryella favors butyrate production. Furthermore, Random Forest machine learning analysis demonstrated that six bacterial taxa in the rumen combined with one serum biomarker, as well as three bacterial taxa in the rectum combined with three serum biomarkers, can serve as potential biomarkers for distinguishing between LH and long- LL dairy cows, achieving prediction accuracies of 92 and 99%, respectively. The findings of this study indicate that rumen and rectum bacteria are associated with the milk production phenotypes of dairy cows with sustained productivity. The rumen microbes are closely linked to the long-term productive capacity of dairy cows and represent a key target for the development of gut microbiota-based interventions. The unique bacterial communities of the rumen and rectum of long-lived high-yielding dairy cows contribute to maintaining their productive capacity.
1 Introduction
Milk and dairy products are high-quality nutritional sources, providing energy, essential amino acids, fats, vitamins, and minerals, of the greatest benefit to people in developing countries in the fight against hunger and malnutrition (Headey, 2023). In 2023, the global dairy market was valued at $944.7 billion, and global dairy production (with milk accounting for approximately 81%) was projected to grow by 1.5% annually over the next decade. Therefore, improving dairy cow productivity and milk yield are key objectives for the future development of the global dairy industry (Organization for Economic Co-Operation and Development, Food and Agriculture Organization of the United Nations, 2023). The overall profitability of a dairy herd depends on the lifetime productivity of the cows, which is a trait that combines production level and longevity (Zhang H. et al., 2024). The profit per kilogram of fat and protein-corrected milk (FPCM) is positively correlated with productive lifespan. Extending the productive lifespan of dairy cows from 1 to 7 years reduces replacement costs from 38% to 9%, while increasing the lifetime production of milk, beef, and edible protein (Grandl et al., 2019). Additionally, extending the productive lifespan of dairy cows can decrease the need for replacement cows, thereby reducing associated feeding costs and greenhouse gas emissions, contributing to more sustainable milk production (Han et al., 2024). The average productive lifespan of dairy cows is typically 3 to 4 years, whereas their natural lifespan can reach approximately 20 years (De Vries, 2020; De Vries and Marcondes, 2020; Hu et al., 2021; Shrestha et al., 2025). The productive performance of older dairy cows declines with age, and high-yielding older cows are more susceptible to health issues, such as reproductive disorders, mastitis, and metabolic diseases, increasing the risk of involuntary culling, and reducing their productive lifespan and lifetime productivity (Pinedo et al., 2010; De Vries and Marcondes, 2020).
The rumen is the primary site for digestion and nutrient absorption in ruminants and the resident microbes are essential for optimal nutrition. Rumen microbial fermentation produces short-chain fatty acids (SCFAs), primarily acetate, propionate, and butyrate, which supply 70% of the daily energy requirements for ruminants (Yeoman and White, 2014). Acetate produced during rumen microbial fermentation participates in de novo fatty acid synthesis, along with medium-chain and long-chain fatty acids generated during feed degradation, jointly contributing to milk fat synthesis (Toral et al., 2018). Rumen microbes degrade fiber and starch in the feed to produce propionate, which serves as a precursor for glucose synthesis through hepatic gluconeogenesis, and the glucose can be subsequently used to produce lactose (Aschenbach et al., 2010). As the primary sugar component in milk, lactose concentration plays a crucial role in maintaining osmotic pressure within the mammary gland, driving the transfer of water from the bloodstream into milk, and sustaining milk production (Antanaitis et al., 2024). Butyrate produced during rumen fermentation serves as an important energy substrate for the rumen epithelial cells of dairy cows, stimulating their proliferation and reducing apoptosis. Additionally, butyrate supplementation enhances fatty acid synthesis and increases the concentrations of milk fat and milk protein (Xu et al., 2018; Kang et al., 2023; Francis et al., 2024). In addition, rumen microbes are a key source of high-quality protein for absorption in the small intestine and for milk protein synthesis (Larson, 1965). Studies on the differences in rumen microbial composition and functionality related to milk protein yield (MPY) have found that the microbial composition and activity of the rumen microbiota account for 18 and 22% of the variation in MPY, respectively (Xue et al., 2020). The relative abundances of members of the phyla Firmicutes and Bacteroidetes are significantly positively correlated with milk fat content (Jami et al., 2014; Si et al., 2023). Dysbiosis of the rumen microbiota promotes the development of mastitis induced by subclinical ketosis and subacute ruminal acidosis by allowing more LPS to enter the bloodstream and disrupting the blood-milk barrier (Hu et al., 2022; Zhao C. et al., 2023; Tang et al., 2024). In transition and ketotic cows, reduced populations of rumen bacteria such as Succinivibrio ruminantium and Megasphaera elsdenii may promote energy imbalance and the progression of ketosis (Wang et al., 2012).
In mammals, the hindgut microbiota plays a pivotal role in nutrient absorption, metabolism, and immune function. An increase in the abundance of Bifidobacteria in feces is associated with improvements in several dairy product characteristics, including milk yield, milk fat and protein yields (Brulin et al., 2024). Based on the relative abundance of microbial genera obtained by 16S rRNA gene sequencing, microbiability calculations can account for 43.1% of the variation in the postpartum serum oxidative stress index (Gu et al., 2023a). Alterations in the hindgut microbiota and their functions, particularly those related to secondary bile acid synthesis, inhibit monocyte function during excessive fat mobilization in transition cows, leading to immune suppression in the postpartum period (Gu et al., 2023b). Oxidative stress during this phase, coupled with immune suppression, increases the proportion of dairy cows with displaced abomasum and clinical ketosis by 3.6% (Ospina et al., 2010). Transplantation of fecal microbiota from cows with mastitis to mice has provided evidence that dysbiosis of the hindgut microbiota contributes to the development of mastitis and alters the mammary microbiota (Ma et al., 2018; Zhao et al., 2022). The rumen and hindgut microbiota are directly associated with dairy production traits, mammary health, and reproductive performance, significantly affecting the productive lifespan of cows. In a study of 2054 herds in the eastern United States, low production and mastitis each accounted for 12% of the reasons for cow culling, while reproductive disorders accounted for 18% (De Vries and Marcondes, 2020).
In this study, we hypothesized that cows with identical DMI and dietary compositions exhibit different metabolic phenotypes driven by the predominant bacteria of the rumen and rectum, thereby influencing their sustained productivity. To test this hypothesis, we selected high-yielding and low-yielding cows with extended productive lifespans. We determined the bacterial compositions of the rumen and rectum, predicted their functions, and measured rumen and rectum fermentation parameters, serum biomarkers, and milk production phenotypes for each group. The aim of the study was to identify the unique differences in the rumen and rectum microbiota of cows with long-term productive capacity and their potential impact on milk production phenotypes, and to provide new insights for selecting and breeding dairy cows to optimize the productive lifespan of dairy herds.
2 Materials and methods
2.1 Ethics approval statement
All experimental procedures involving animals were conducted in accordance with the guidelines recommended by the Administration of Affairs Concerning Experimental Animals (Ministry of Science and Technology, China, revised 2004). The experimental protocol was reviewed and approved by the Animal Research and Technology Centre of Northwest A&F University (Yangling, Shaanxi, China). The study adhered to ethical standards for the use of animals in research.
2.2 Animal, study design, and sample collection
This study involved a total of 1,529 healthy lactating Holstein cows from a commercial dairy farm in Ningxia, China. A subset of 219 multiparous mid-lactation Holstein cows with five or more parities, with a mean parity of 5.42 ± 0.77 (mean ± standard deviation) and mean [DIM of 148.19 ± 41.09 (mean ± standard deviation), was selected]. Within one week before the experiment began, based on previous research (Edmonson et al., 1989), experienced farm staff assessed each cow’s BCS using visual and tactile evaluations, scoring on a scale from 1 to 5 with 0.25 increments.
All cows were fed the same diet, consisting of a concentrate-to-forage ratio of 54:46 on a dry matter basis (Supplementary Table S1). The cows were fed and milked three times daily at 06:00, 14:00, and 22:00, with ad libitum access to feed and water, under the same management conditions.
The feed intake of each cow was recorded over seven consecutive days by manually measuring the initial feed weight and the remaining feed weight after each feeding period. MY was recorded continuously over 7 days, and on the seventh day, milk samples were collected in a 4:3:3 volume ratio corresponding to each milking time. The milk components, including MF, MP, ML, MSNF, MTS, MFP, MD, EC, and Ash, were analyzed using a spectrophotometer (Foss-4000; Foss Electric A/S, Hillerød, Denmark). Milk protein/fat content was multiplied by the MY recorded on the seventh day to calculate the MFY/MPY. The FE was calculated using 3.5% FCM and DMI. Cows were selected based on the absence of a history of reproductive disorders, no recorded diseases in the past 3 months, and a historical 305-day milk yield consistently exceeding 12,000 kg. Based on milk performances, 10 LH cows (parity = 5.80 ± 0.79, DIM = 128.90 ± 9.32, BCS = 3.33 ± 0.46; mean ± standard deviation) and 10 LL cows (parity = 6.1 ± 0.74, DIM = 132 ± 4.32, BCS = 3.28 ± 0.42; mean ± standard deviation) were selected for further analysis. The MY, MFY, and MPY values of LH cows were 55.32 ± 3.14 kg/day, 1.85 ± 0.11 kg/day, and 2.08 ± 0.21 kg/day (mean ± standard deviation), respectively, while those of LL cows were 38.86 ± 3.75 kg/day, 1.43 ± 0.21 kg/day, and 1.28 ± 0.18 kg/day (mean ± standard deviation) respectively. On the morning of the seventh day, before feeding, rumen contents were collected via oral tubing and filtered through four layers of sterile cheesecloth, and rectum contents were manually collected using sterile gloves, followed by 16S rRNA gene sequencing analysis, SCFAs analysis and rumen pH (RpH) measurement (Leici PHS-3C; Shanghai INESA Scientific Instrument Co., Ltd., Shanghai, China). Blood samples from all cows were collected from the tail vein in tubes without anticoagulants, and then centrifuged at 3,500 × g for 15 min at 4°C (Centrifuge 5810R, Eppendorf, Germany) to separate serum for biochemical parameter analysis.
2.3 Plasma parameter measurement
The concentrations of TP, ALB, GLB, ALT, AST, ALP, TBA, γ-GGT, BUN, CRE, LDH, GLU, TC, TG, HDL-C, LDL-C, IgG, IgA, SOD, GPx, T-AOC, T-GSH, and CAT in plasma were measured using an AutoAnalyzer KHB-1280 instrument (Shanhai Keshun Science and Technology Co., Ltd., Shanghai, China) with commercial kits (Beijing Jinhai Keyu Biotechnology Development Co., Ltd., Beijing, China). In addition, NEFA, BHB, HPT and SAA were analyzed using commercial enzyme-linked immunosorbent assay (ELISA) kits from Shanghai Keshun Bioengineering Institute (Shanghai, China). TNF-α, IL-1β, IL-6, IL-10, and MDA, #A003-1-2were analyzed with commercial ELISA kits from Nanjing Jiancheng Bioengineering Institute (Nanjing, China). PCwas measured using commercial ELISA kits from Beijing Solarbio Science & Technology Co., Ltd. (Beijing, China). All assays were conducted according to the manufacturer’s instructions. All samples were measured in triplicate, and the average value was calculated. All reagent kit catalogue numbers are listed in Supplementary Table S15.
2.4 Determination of SCFAs concentrations in ruminal fluid
Based on previous studies (Wang et al., 2023a; Wang et al., 2023b), SCFAs were determined using gas chromatography (Agilent 7820A, Santa Clara, CA, United States) with a capillary column (AE-FFAP, 30 m × 0.25 mm × 0.33 μm; ATECH Technologies Co., Lanzhou, China). In brief, rumen fluid samples were centrifuged at 12,000 × g for 10 min at 4°C. Three milliliters of the supernatant was mixed with 400 μL of 25% meta-phosphoric acid (w/v). For fecal samples, approximately 1.0 g of the sample was added to 3.0 mL distilled water and vortexed vigorously, then centrifuged at 12,000 × g for 15 min at 4°C. Two milliliters of the supernatant was then mixed with 400 μL of 25% meta-phosphoric acid (w/v). The mixture was placed at 4°C for 4 h and subsequently centrifuged at 16,000 × g for 10 min at 4°C. Then, 200 μL of caprylic acid (10 g/L) was added to 200 μL aliquots of the supernatant, and the mixture was filtered through a 0.45 μm filter. The injector and detector temperatures were set to 200°C and 250°C, respectively. The column temperature was increased from 45°C to 150°C at a rate of 20°C/min and held at 150°C for 5 min. SCFAs, including acetate (Ac), propionate (Pr), isobutyrate (iBu), butyrate (Bu), isovalerate (iVal), valerate (Val), 4-Methylvalerate (4MVal) and hexanoate (Hex), were quantitatively analyzed. In this method, the internal standard used was 2-ethylbutyrate, and the other standards included acetate, propionate, isobutyrate, butyrate, isovalerate, valerate, 4-Methylvalerate, and hexanoate. The concentration of each SCFA was quantified by constructing a standard curve using standard solutions with concentrations of 25, 50, 100, 200, 400, and 800 μM, and the total SCFA concentration was calculated by summing the concentrations of all individual SCFAs.
2.5 DNA extraction, 16S rRNA sequencing, and data processing for microbiota
Based on the instructions provided with the FastPure Stool DNA Isolation Kit (MJYH, Shanghai, China), total genomic DNA of the microbial community was extracted from rumen fluid and fecal samples. The integrity of the extracted genomic DNA was assessed using 1% agarose gel electrophoresis, and DNA concentration and purity were determined with a NanoDrop2000 (Thermo Scientific, United States).
Using the extracted DNA as the template, the V3–V4 hypervariable regions of the 16S rRNA gene were PCR-amplified with a forward primer 338F (5′-ACTCCTACGGGAGGCAGCAG-3′) carrying a barcode sequence and a reverse primer 806R (5′-GGACTACHVGGGTWTCTAAT-3′) (Liu et al., 2016). The PCR reaction mixture consisted of 4 μL of 5 × TransStart FastPfu buffer, 2 μL of 2.5 mM dNTPs, 0.8 μL of the forward primer (5 μM), 0.8 μL of the reverse primer (5 μM), 0.4 μL of TransStart FastPfu DNA polymerase, 10 ng of template DNA, and ddH₂O to a final volume of 20 μL. The amplification program was as follows: initial denaturation at 95°C for 3 min; 27 cycles of 95°C for 30 s (denaturation), 55°C for 30 s (annealing), and 72°C for 30 s (extension); followed by a final extension at 72°C for 10 min, with samples subsequently stored at 4°C (PCR instrument: ABI GeneAmp® 9700). The PCR products were recovered using 2% agarose gel electrophoresis and purified with the DNA Gel Recovery Purification Kit (PCR Clean-Up Kit, China Yuhua). Quantification of the purified products was performed using a Qubit 4.0 (Thermo Fisher Scientific, United States). Subsequently, the purified PCR products underwent library construction using the NEXTFLEX Rapid DNA-Seq Kit, which involved: (1) Adapter ligation. (2) Magnetic bead screening to remove adapter self-ligation fragments. (3) PCR amplification for library template enrichment. (4) Magnetic bead recovery of the PCR products to obtain the final library. Sequencing was conducted on the Illumina NextSeq2000 platform (Shanghai Meiji Biomedical Technology Co., Ltd.).
The paired-end raw sequencing reads were quality controlled using fastp (Chen et al., 2018) (https://github.com/OpenGene/fastp, version 0.19.6) and merged using FLASH (Magoč and Salzberg, 2011) (http://www.cbcb.umd.edu/software/flash, version 1.2.11) with the following steps: (1) Filter out bases at the read ends with quality values below 20 using a 50 bp sliding window; if the average quality in the window is below 20, trim the bases from the beginning of the window; remove reads shorter than 50 bp after quality control, and discard reads containing ambiguous “N” bases. (2) Merge paired-end reads into a single sequence based on the overlap between the paired-end reads, with a minimum overlap length of 10 bp. (3) Allow a maximum mismatch rate of 0.2 in the overlap region of the merged sequence and filter out sequences that do not meet this criterion. (4) Demultiplex the samples and adjust sequence orientations according to the barcode and primer sequences at both ends, allowing 0 mismatches for barcodes and up to 2 mismatches for primers. Using the default parameters, the quality-controlled and merged sequences were denoised using the DADA2 (Edgar, 2013) plugin within the Qiime2 pipeline (Barberán et al., 2012). The sequences resulting from DADA2 denoising are commonly referred to as ASVs (amplicon sequence variants). All sequences annotated as chloroplast or mitochondrial were removed from all samples. To minimize the impact of sequencing depth on subsequent alpha and beta diversity analyses, all samples were rarefied to 20,000 sequences, with the average Good’s coverage per sample remaining at 99.09%. Taxonomic classification of the ASVs was performed using a Naive Bayes classifier in QIIME2, based on the SILVA 16S rRNA gene database (v138) (Quast et al., 2013). Functional prediction of 16S data was conducted using PICRUSt2 (Segata et al., 2011) (version 2.2.0). Additionally, using BugBase to assess microbial community characteristics (Ward et al., 2017).
2.6 Biomarker selection for LH and LL cows using Random Forest
To differentiate between LH cows and LL cows, a random forest classification model was constructed using the RandomForest package in R. Bacterial relative abundance from the rumen and rectum, concentrations of SCFAs and serum biochemical parameters were included as independent variables in the model. The classification performance was validated using the area under the receiver operating characteristic (ROC) curve (area under the curve, AUC). Variable importance was assessed using the mean decrease accuracy (MDA) metric to identify biomarkers that significantly contributed to the classification.
2.7 Statistical analysis
Physiological parameters, serum parameters, rumen and rectum SCFA concentrations, and bacterial abundance and functional data were analyzed using SAS software (version 9.4, SAS Institute Inc., Cary, NC, United States). A mixed procedure was used as follows:
3 Results
3.1 Differences in microbial composition of the rumen and rectum between LH and LL groups
The richness of the rumen microbiota in LH cows was significantly lower than that in LL cows (p < 0.05), while diversity and evenness showed no significant differences (Figure 1A). The alpha diversity of the rectum microbiota also did not differ significantly between the two groups (Figure 1B). At the ASV level in the rumen, the Chao1, ACE, and Shannon indices were negatively correlated with MY, while the Simpson index showed a positive correlation with MY (p < 0.05). However, no significant correlations were observed between the alpha diversity of the rectum microbiota and production phenotypes at any taxonomic level (Supplementary Table S2). PCoA revealed that the rumen bacterial community compositions differed significantly between LH and LL cows (p < 0.05); however, no significant differences were observed in rectum microbiota community between the two groups (Figures 1C,D). At the phylum level, only Bacteroidota, Fibrobacterota, and Verrucomicrobiota exhibited significant differences in the rumen bacterial composition between LH and LL cows (p < 0.05), with LH cows exhibiting significantly lower levels than LL cows. However, there were no differences in rectum bacterial composition at the phylum level between the two groups of cows (Supplementary Figure S1A and Supplementary Tables S3, S4). At the genus level, there were 43 differentially abundant genera in the rumen bacteria between the LH and LL groups, and 14 differentially abundant genera in the rectum bacteria between the two groups (Supplementary Figure S1B and Supplementary Tables S3, S4). LEfSe analysis identified enriched bacterial genera (LDA >2), including unclassified_f__Lachnospiraceae, norank_f__norank_o__Clostridia_UCG-014, Shuttleworthia, Syntrophococcus, Eubacterium_ruminantium_group, and Olsenella in the rumen microbiota of the LH group (Figure 1E and Supplementary Figure S1A). Bacterial genera (LDA >2) such as Eubacterium_oxidoreducens_group, Breznakia, Parabacteroides, and norank_f__Flavobacteriaceae were enriched in the rectum samples from the LH group (Figure 1F and Supplementary Figure S2B). In the rumen, the most abundant genera included unclassified_f__Lachnospiraceae, norank_f__norank_o__Clostridia_UCG-014, Eubacterium_ruminantium_group, Shuttleworthia, Syntrophococcus, Olsenella, Lachnospira, norank_f__Selenomonadaceae, Dialister, Desulfovibrio, Erysipelotrichaceae_UCG-002, and Roseburia (relative abundance >0.1%; p < 0.05) showed a positive correlation with milk production phenotypes, including MY, ECM, 3.5% FCM, MFY, MPY, and FE, while Prevotellaceae_UCG-001, norank_f__F082, norank_f__Bacteroidales_RF16_group, norank_f__norank_o_Bacteroidales, norank_f__p-251-o5, UCG-001, Anaerovibrio, and g__CAG-352 (relative abundance >0.1%; p < 0.05) were negatively correlated with milk production phenotypes (Figure 1G). In the rectum, genera such as norank_f__Paludibacteraceae, Parabacteroides, Anaerovorax, Mailhella, and Erysipelotrichaceae_UCG-007 (relative abundance >0.01%; p < 0.05) showed a positive correlation with milk production phenotypes, while the Christensenellaceae_R-7_group, Lachnospiraceae_NK3A20_group, Moryella, norank_f__Bifidobacteriaceae, and norank_f__Clostridium_methylpentosum_group (relative abundance >0.01%; p < 0.05) were negatively correlated with milk production phenotypes (Figure 1H).
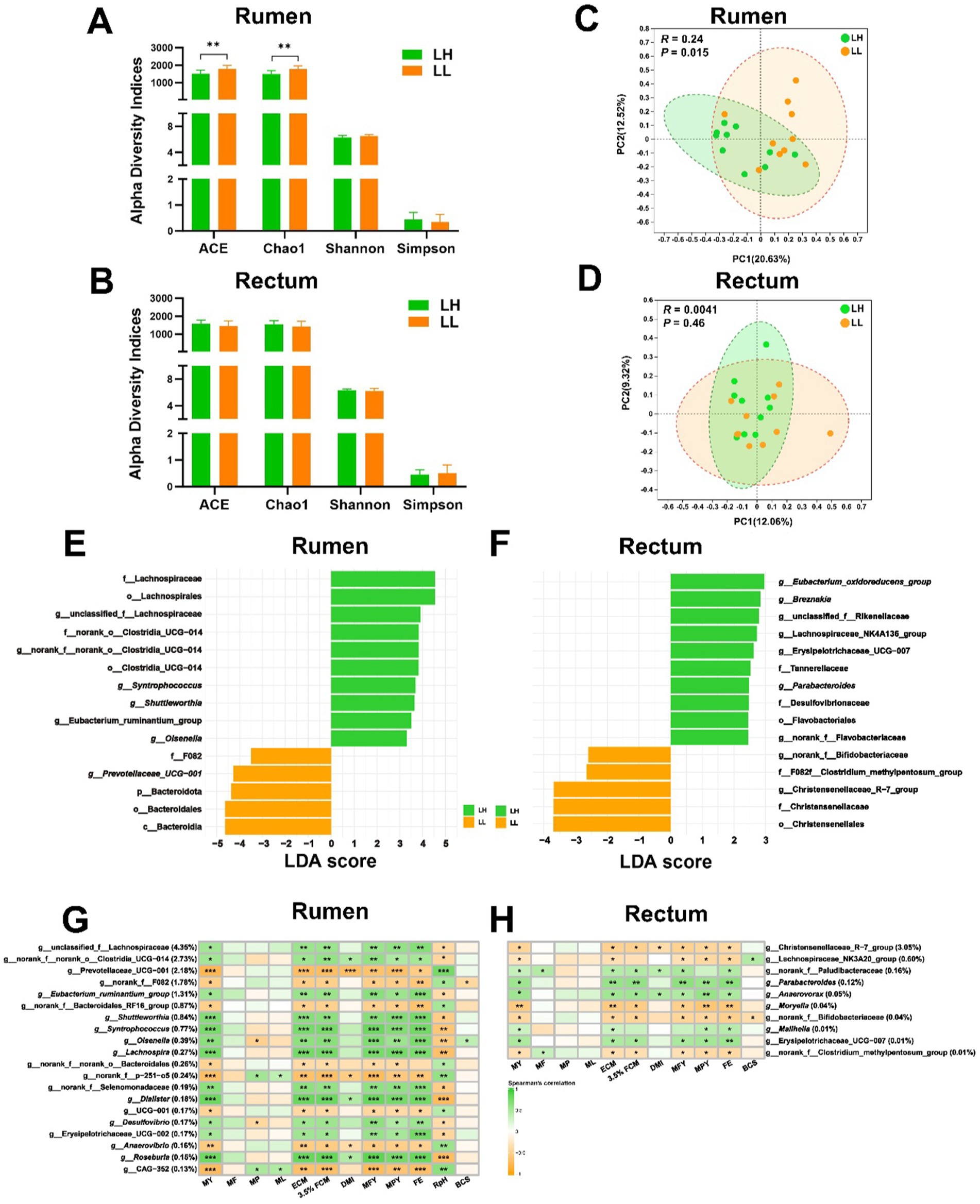
Figure 1. Comparison of microbiota diversity and structure in the rumen and rectum of LH and LL groups. (A,B) Alpha diversity indices of rumen and rectum microbiota. Error bars represent mean ± SEM. **p < 0.01. (C,D) The PCoA of rumen and rectum microbiota at the ASV level were based on the Bray–Curtis dissimilarity. Dissimilarity was analyzed using ANOSIM statistical tests with 999 permutations. (E,F) The LEfSe bar plots show differentially abundant bacterial taxa between LH and LL groups in rumen and rectum microbial community. The significance threshold was set at LDA >2 and p < 0.05. (G,H) Heatmaps show the association between rectum and rumen bacterial genera (average relative abundance > 0.1 and 0.01%) and the production and physiological parameters (Spearman’s correlation). *p < 0.05, **p < 0.01, and ***p < 0.001.
3.2 Distinct rumen and rectum bacterial phenotypes in LH vs. LL cows
BugBase predictive analysis of nine bacterial phenotypes in the rumen and rectum of both groups revealed that the pathogenic potential of rumen bacteria in LH cows was lower than in LL cows (p < 0.05), while no significant differences were observed in the rectum bacterial phenotypes (Figures 2A,B). These results indicate that the rumen bacterial community in LH cows exhibits lower pathogenic potential.
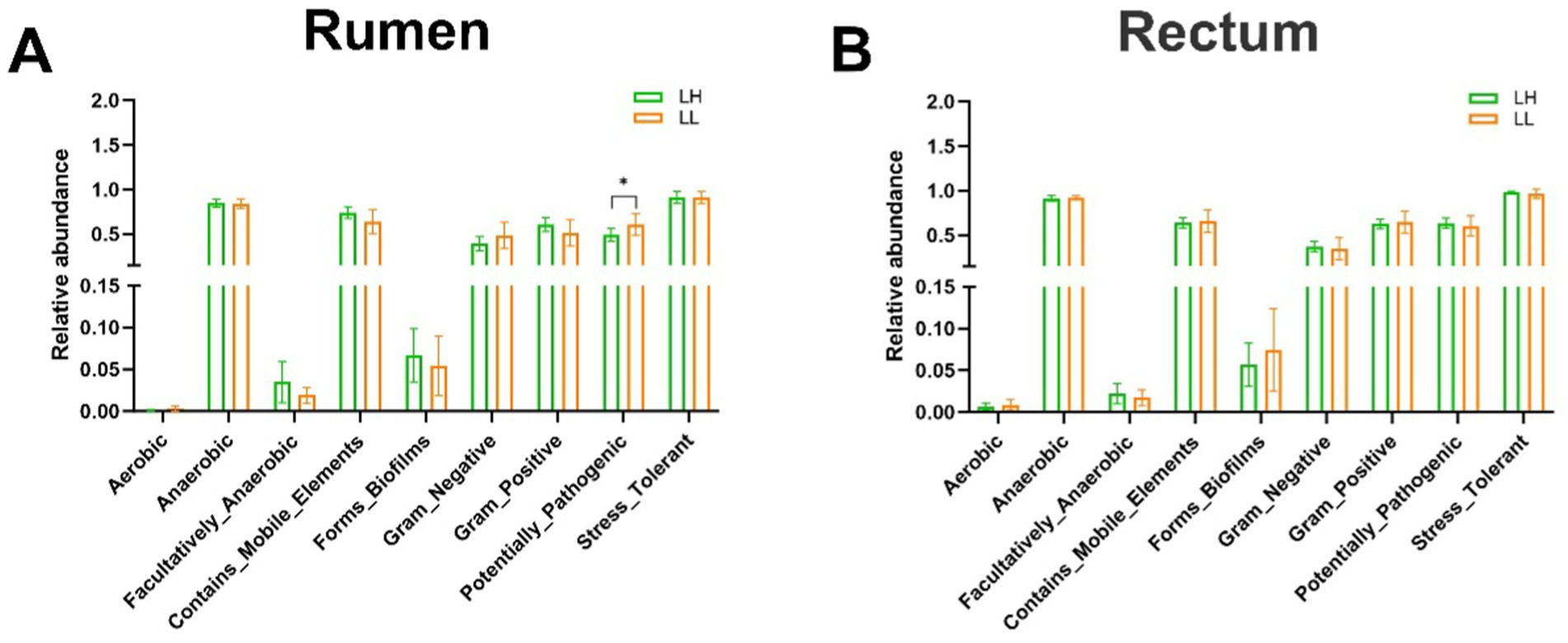
Figure 2. Differences in the BugBase-predicted microbial phenotypes between LH and LL groups. (A,B) Prediction of nine microbial phenotypes in the rumen and rectum of LH and LL groups using BugBase phenotype prediction. The bars represent mean ± SEM. *p < 0.05.
3.3 Different phenotypes and their association with bacteria
The milk composition and DMI showed no significant differences between LH and LL cows. However, LH cows exhibited significantly higher MY, MFY, MPY, ECM, 3.5% FCM, FE, 305-d MY, historical average 305-d MY, and lower RpH compared with LL cows (p < 0.05; Table 1). In the serum of LH cows, TC, HDL-C, and LDL-C were significantly higher, while GLB and TG were significantly lower than in LL cows (p < 0.05; Table 2). Compared to the LL group (p < 0.05), LH cows showed higher concentrations of Pr and lower concentrations of Hex in the rumen, along with higher Bu concentrations in the rectum; acetate and other SCFAs did not show significant differences between the two groups in rumen and rectum (Figures 3A,B and Supplementary Table S5). Results of the Mantel test based on the rumen bacteria matrix indicated that rumen Bu and Hex concentrations were negatively correlated with MY (p < 0.05), while rumen Pr, serum TC, HDL-C, and LDL-C concentrations were positively correlated with milk production phenotypess; rumen acetate and other SCFAs were not found to be associated with any milk production phenotypes (p < 0.05; Figure 3C). The Mantel test results based on the rectum bacteria matrix indicated a positive correlation between rectum Bu concentration and milk production phenotypes, a negative correlation between serum GLB concentration and milk production phenotypes, and positive correlations between HDL-C, LDL-C, and milk production phenotypes; rectum acetate and other SCFAs were also not found to be associated with any milk production phenotypes (p < 0.05; Figure 3D). In the rumen, the relative abundances of MY-associated bacteria unclassified_f__Lachnospiraceae, Eubacterium_ruminantium_group, Shuttleworthia Syntrophococcus, Lachnospira, Desulfovibrio, Erysipelotrichaceae_UCG-002, and Roseburia were positively correlated with Pr concentrations (p < 0.05; Figure 3E). In the rectum, the relative abundance of MY-associated bacteria of the Christensenellaceae_R-7_group and Moryella showed negative correlations with Bu concentration (p < 0.05; Figure 3F).
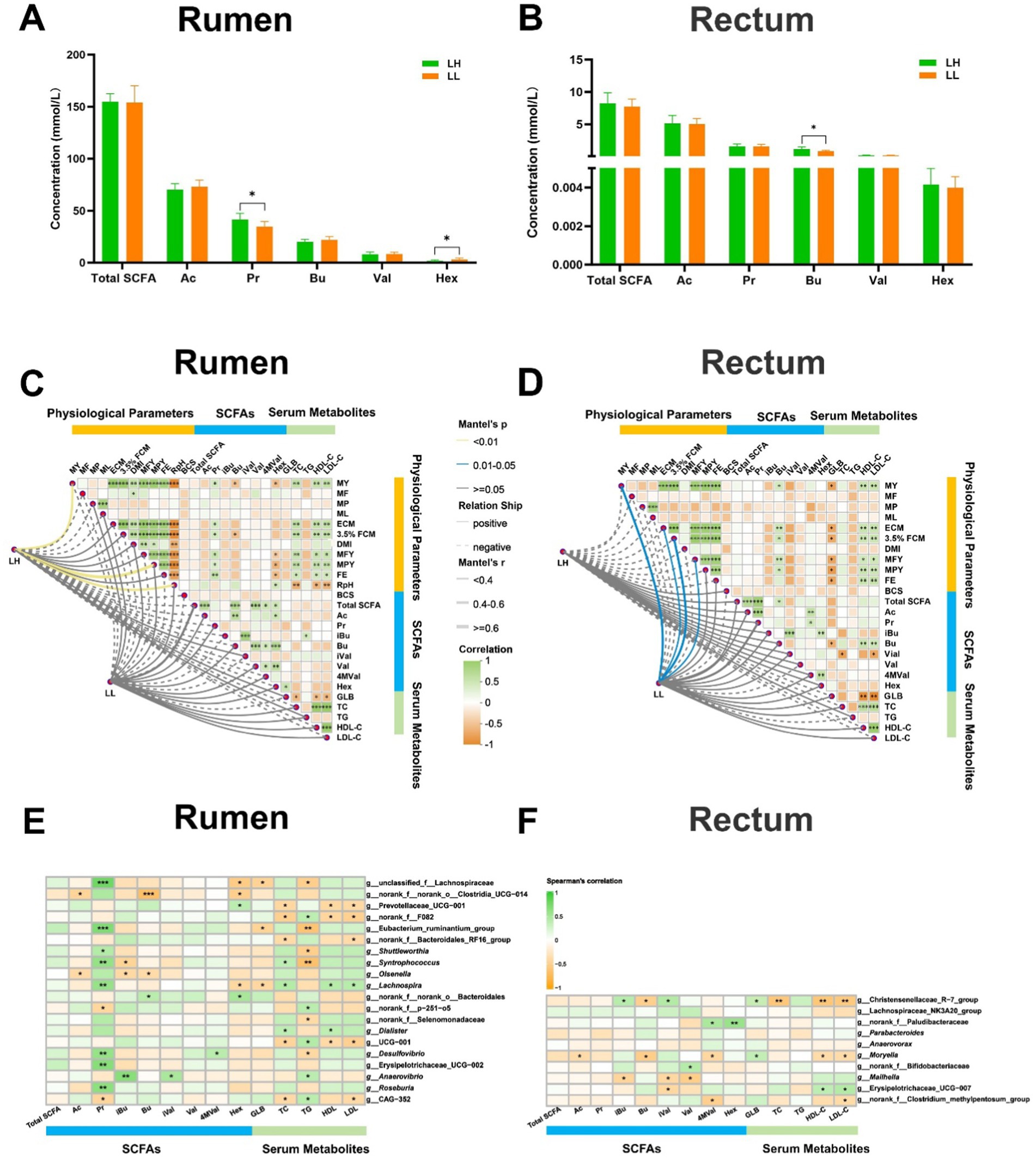
Figure 3. Correlation between rumen and rectum SCFAs, differential serum parameters and MY-related microbiota. (A,B) Concentrations of SCFAs in the rumen and rectum of the LH and LL groups. The bars represent mean ± SEM. *p < 0.05. (C,D) Correlation matrix among physiological parameters, SCFA concentrations in rumen and rectum, and serum metabolites in the LH and LL groups were calculated using Mantel’s test. The distance matrix for clinical factors was computed based on the Bray–Curtis algorithm, while Spearman’s correlation coefficients were used to evaluate associations. *p < 0.05, **p < 0.01, and ***p < 0.001. (E,F) Spearman’s rank correlation heatmaps between MY-related bacteria and SCFAs in rumen and rectum. The color gradient represents the values of correlation coefficients. *p < 0.05, **p < 0.01, and ***p < 0.001.
3.4 Microbial co-occurrence patterns and their association with phenotypes
We used microbial co-occurrence networks to identify key microbial populations in LH and LL cows. In the rumen bacteria of LH cows, Module 1 was centered on the Prevotellaceae_UCG-001, which was directly connected to 25 bacterial genera and had the highest closeness centrality, thereby establishing it as the key pathway node. Module 1 was negatively correlated with milk production phenotypes, as well as rumen Pr, serum TC, HDL-C, and LDL-C concentrations (p < 0.05). In Module 6, Syntrophococcus exhibited the highest betweenness centrality and was connected with unclassified_f__Lachnospiraceae, Roseburia, Eubacterium_ruminantium_group, and Erysipelotrichaceae_UCG-002, all of which were enriched in LH cows. Module 6 was positively correlated with MY, FE, rumen Pr, and serum TC, HDL-C, and LDL-C concentrations (p < 0.05). In Module 7, Lachnospira and Shuttleworthia showed the highest betweenness centrality and closeness centrality, connecting directly with 25 and 29 bacterial genera, respectively, thus making them the core nodes of this module (Figure 4A and Supplementary Table S6). This module, centered on Lachnospira and Shuttleworthia, was positively correlated with MY, rumen Ac, Pr and serum TC, HDL-C, and LDL-C concentrations (p < 0.05; Figure 4B). In contrast, none of the modules showed a correlation between the rumen bacteria of LL cows and MY. However, in Module 5, Anaerovibrio exhibited the highest betweenness centrality and closeness centrality, serving as the core node (Figure 4C and Supplementary Table S6). This module was negatively correlated with total SCFAs, Ac, and Pr concentrations in the rumen (p < 0.05; Figure 4D).
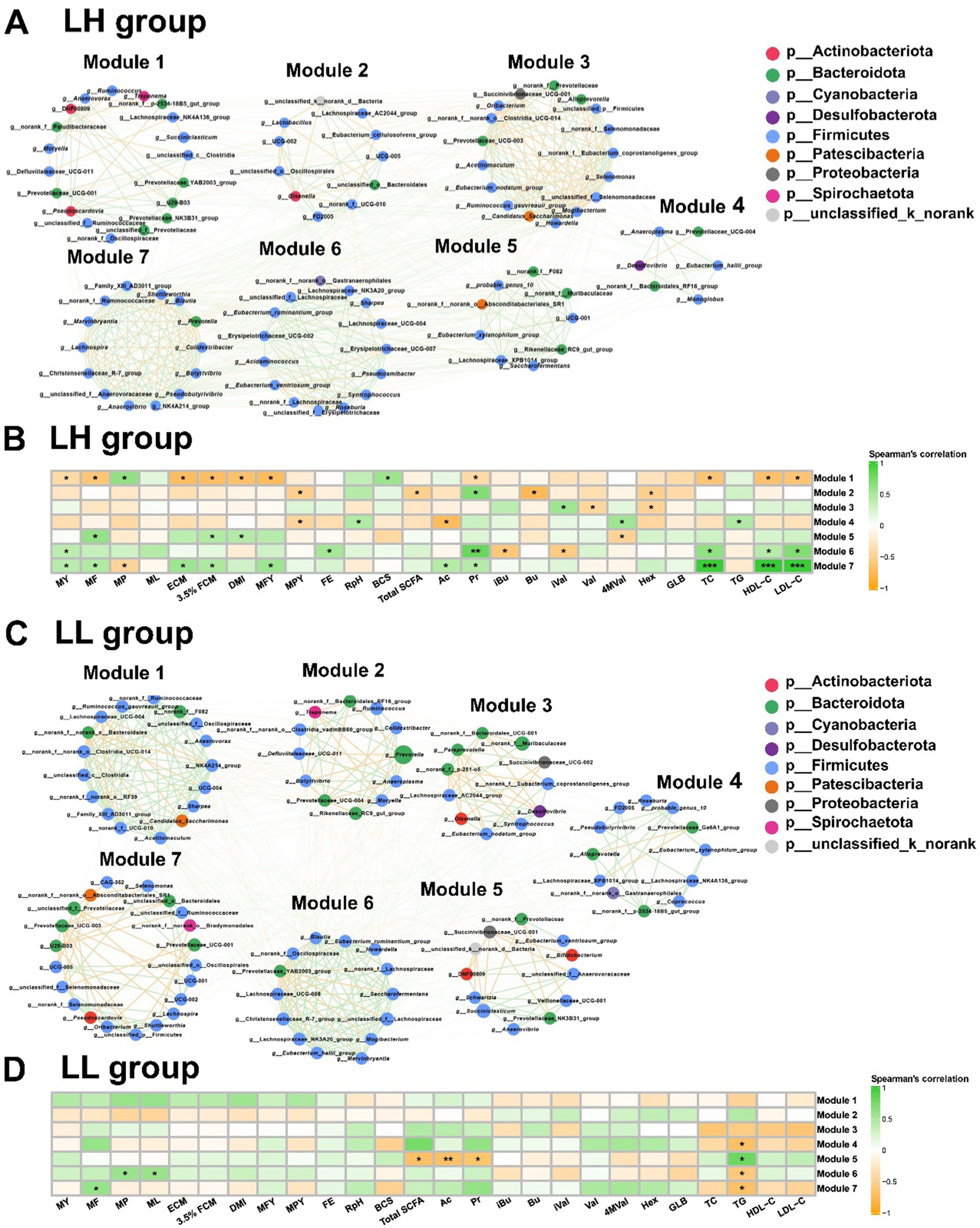
Figure 4. Co-occurrence networks and correlation analysis of rumen microbiota in LH and LL cows. (A,C) Co-occurrence network of rumen bacteria at the genus level, with bacterial genera assigned based on their network roles in LH and LL cows (n = 10 per group). Nodes represent bacterial genera, with node size indicating the relative abundance of each genus. The color of the edges between nodes indicates positive (green) or negative (yellow) correlations (Spearman’s |r| >0.60 and p < 0.05). The thickness of the edges represents the magnitude of Spearman’s |r|. (B,D) Correlation analysis on the rumen microbiota modules and phenotypes in LH and LL group. The color gradient represents the values of correlation coefficients (Spearman’s correlation). *p < 0.05, **p < 0.01, and ***p < 0.001.
For the rectum bacteria in LH cows, Module 1 was positively correlated with MY, rectum Bu, and Hex concentration (p < 0.05; (Supplementary Figures S4A,B). Although the Eubacterium_oxidoreducens_group, a core bacterium within this module, was not directly correlated with MY, it was enriched in LH cows, connected to 13 other genera, and had the highest betweenness centrality and closeness centrality, suggesting that it could have indirectly influenced MY by maintaining connectivity and functional cooperation within the module (Supplementary Figures S3A,B and Supplementary Table S7). In the rectum bacteria of LL cows, none of the modules showed any correlation with MY (Supplementary Figures S3C,D).
3.5 Differential functions of the rumen microbiome between LH and LL groups
Using PICRUSt2, the functional prediction of rumen microbial communities compared the KEGG pathways between the two groups of dairy cows. At KEGG pathway level 1, the rumen microbiota of dairy cows was functionally identified with Metabolism (78.22%), Genetic Information Processing (8.84%), Environmental Information Processing (4.23%), Cellular Processes (3.873%), Human Diseases (3.047%), and Organismal Systems (1.841%). No significant differences were observed between the two groups of cows at this level. At KEGG pathway levels 2 and 3, the global and overview maps and citrate cycle pathway in the rumen microbiota of LL cows were significantly higher than those of LH cows (Figure 5 and Supplementary Table S8). For the rectum microbiota of the two groups, no significant differences were detected at any KEGG pathway level (Supplementary Table S9 and Supplementary Figure S4).
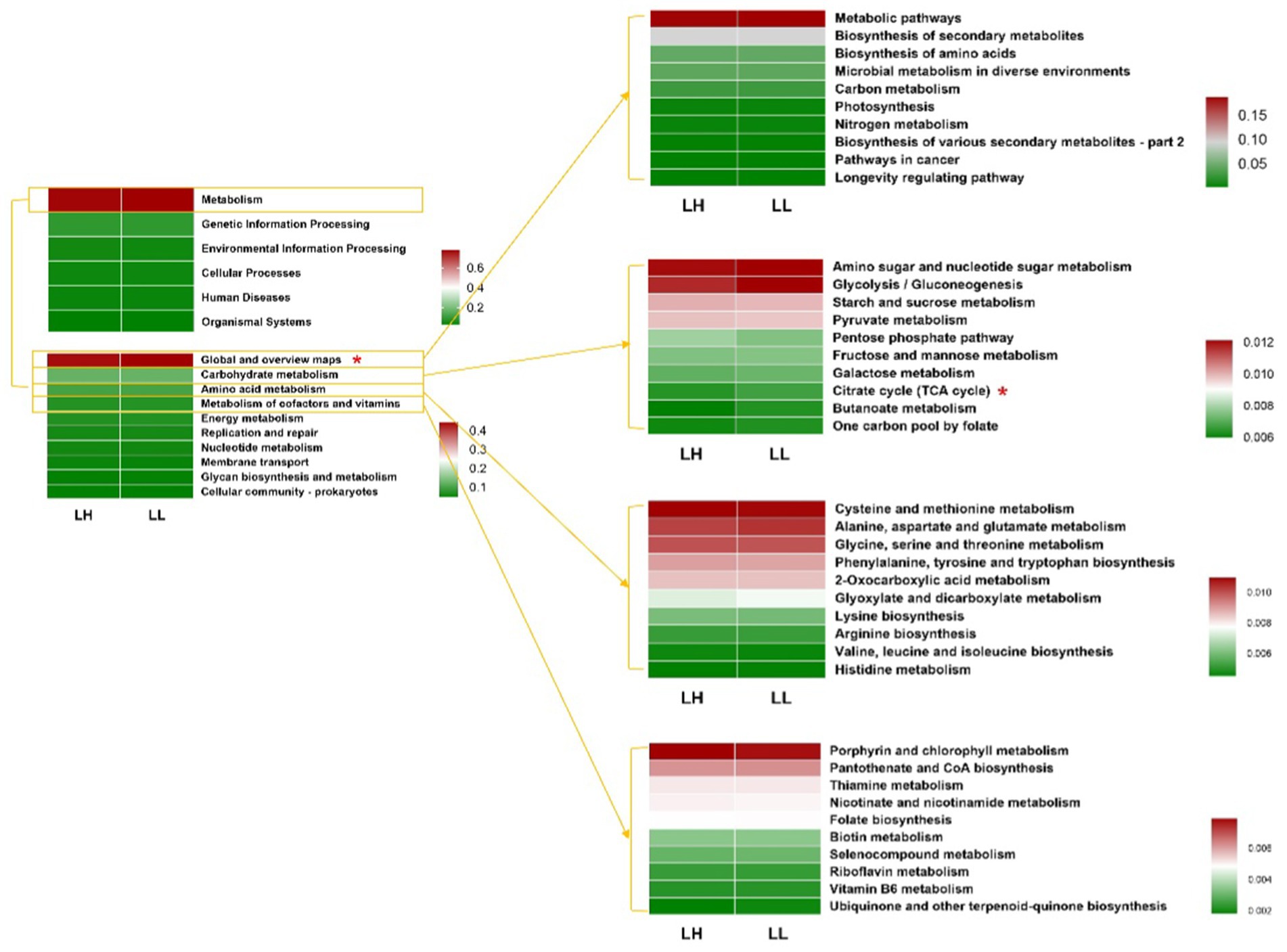
Figure 5. Functional predictions of rumen bacteria between LH and LL groups by PICRUSt2. Relative abundances of functional KEGG pathways at level 1, level 2, and level 3 in rumen bacteria (top 10 of global and overview map, carbohydrate metabolism, amino acid metabolism, and metabolism of cofactors and vitamins). KEGG pathways were compared using Student’s t-tests. *p < 0.05.
Additionally, seven KEGG enzymes related to carbohydrate and fatty acid metabolism were significantly enriched in the LH group (log2 fold change >1, LDA >2 and p < 0.05) (Figures 6A,B). Among the KEGG enzymes involved in carbohydrate metabolism, EC 3.2.1.10 (oligo-1,6-glucosidase; involved in the hydrolysis of α-1,6 glycosidic bonds in oligosaccharides derived from starch and glycogen), EC 3.2.1.11 (dextranase; involved in the hydrolysis of α-1,6 glycosidic bonds in dextran), EC 3.2.1.3 (glucoamylase; involved in the hydrolysis of terminal α-1,4 glycosidic bonds in starch and related polysaccharides), EC 3.2.1.135 (neopullulanase; involved in the hydrolysis of pullulan by cleaving α-1,4 glycosidic bonds) and EC 4.2.1.42 (galactarate dehydratase; involved in the dehydration of galactarate to 5-dehydro-4-deoxy-D-glucarate) were more abundant in the LH group. EC 3.1.2.21 [medium-chain acyl-(acyl-carrier-protein) hydrolase; involved in hydrolyzing medium-chain acyl groups from acyl carrier protein] and EC 3.1.2.20 (acyl-CoA hydrolase; involved in hydrolyzing acyl-CoA to produce free fatty acids and coenzyme A) in the fatty acid biosynthesis pathway were more abundant in the LH group (Figure 6C). However, two KEGG enzymes, EC 1.1.1.303 (diacetyl reductase; involved in reducing diacetyl to Acetoin) and EC 1.1.1.4, (R,R)-butanediol dehydrogenase [involved in the reversible conversion between (R,R)-2,3-butanediol and acetoin], which promote the conversion of propionate to (R,R)-butane-2,3-diol, were significantly reduced in the rectums of LL cows (log2 fold change >1, LDA >2 and p < 0.05) (Supplementary Figures S5A,B). The mixed model analysis confirmed these findings with consistent results (p < 0.05) (Supplementary Table S14).
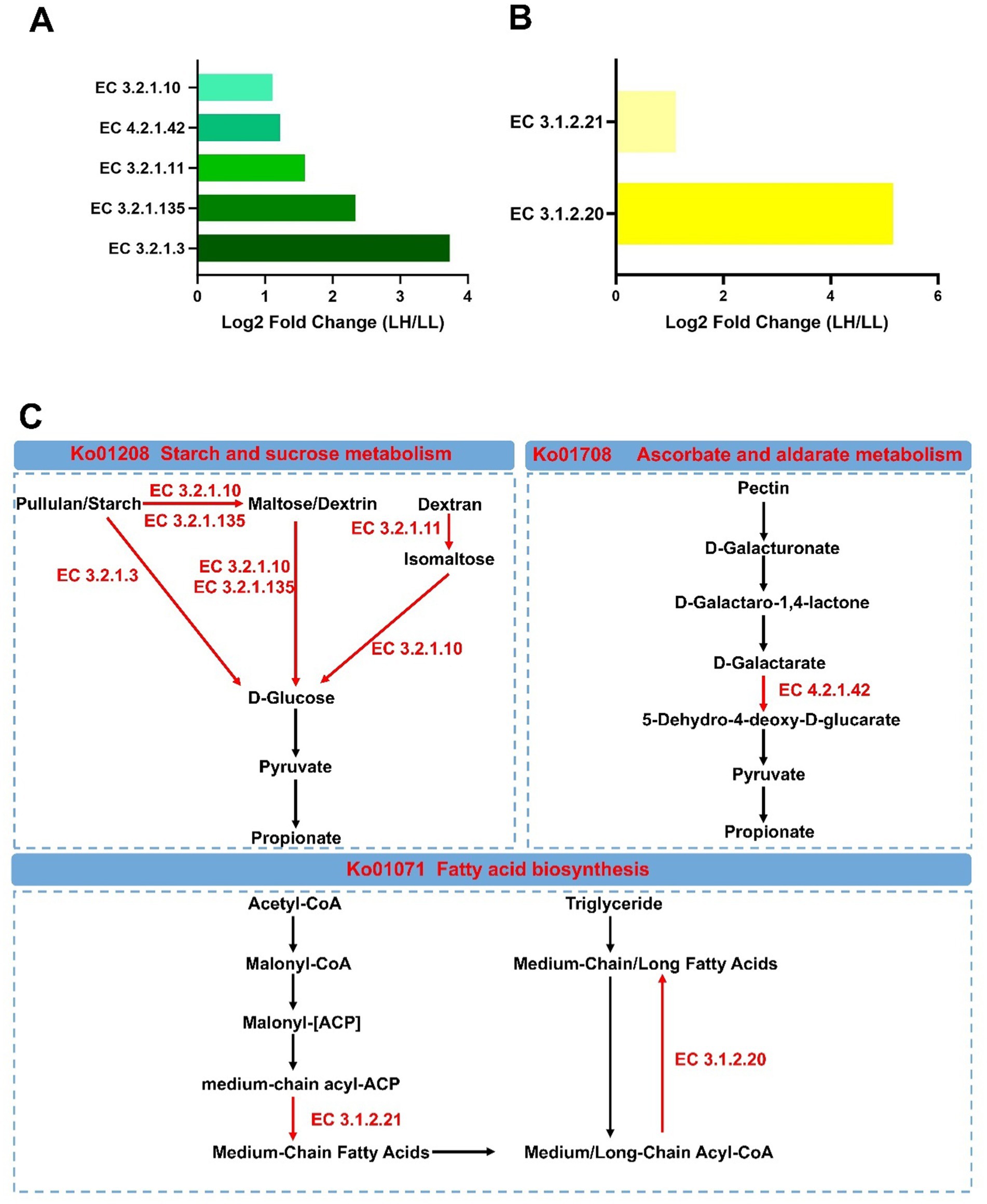
Figure 6. Differential rumen KEGG enzymes and metabolic pathways between LH and LL groups. (A,B) Significantly different rumen carbohydrate-related and lipid-related KEGG enzymes between LH and LL groups (LDA >2, p < 0.05). (C) Differential microbial metabolic pathways between LH and LL groups. Red names and arrows indicate KEGG orthology and KEGG enzymes enriched in LH cows. EC, Enzyme Commission.
3.6 Relationships between microorganisms, microbial functions and phenotypes
A multi-layered network based on Spearman rank correlations was constructed to determine the relationships among MY-associated rumen bacterial taxa, microbial KEGG enzymes predicted by PICRUSt2, rumen fermentation parameters, serum parameters and milk production phenotypes (Figures 7A,B and Supplementary Tables S10, S11). Based on the network’s betweenness centrality and closeness centrality, Roseburia and Erysipelotrichaceae_UCG-002 were identified as core bacterial taxa. Moreover, the relative abundance of three KEGG enzymes associated with carbohydrate metabolism: EC 3.2.1.135, EC 4.2.1.42, and EC 3.2.1.10 were positively correlated with rumen Pr concentrations (p < 0.05), which were positively linked to five milk production phenotypes (p < 0.05). In addition, the positive interaction network of bacteria associated with milk production was positively correlated with two lipid metabolism-related enzymes, EC 3.1.2.21 and EC 3.1.2.20 (p < 0.05). These two enzymes were also positively correlated with five milk production phenotypes (p < 0.05).
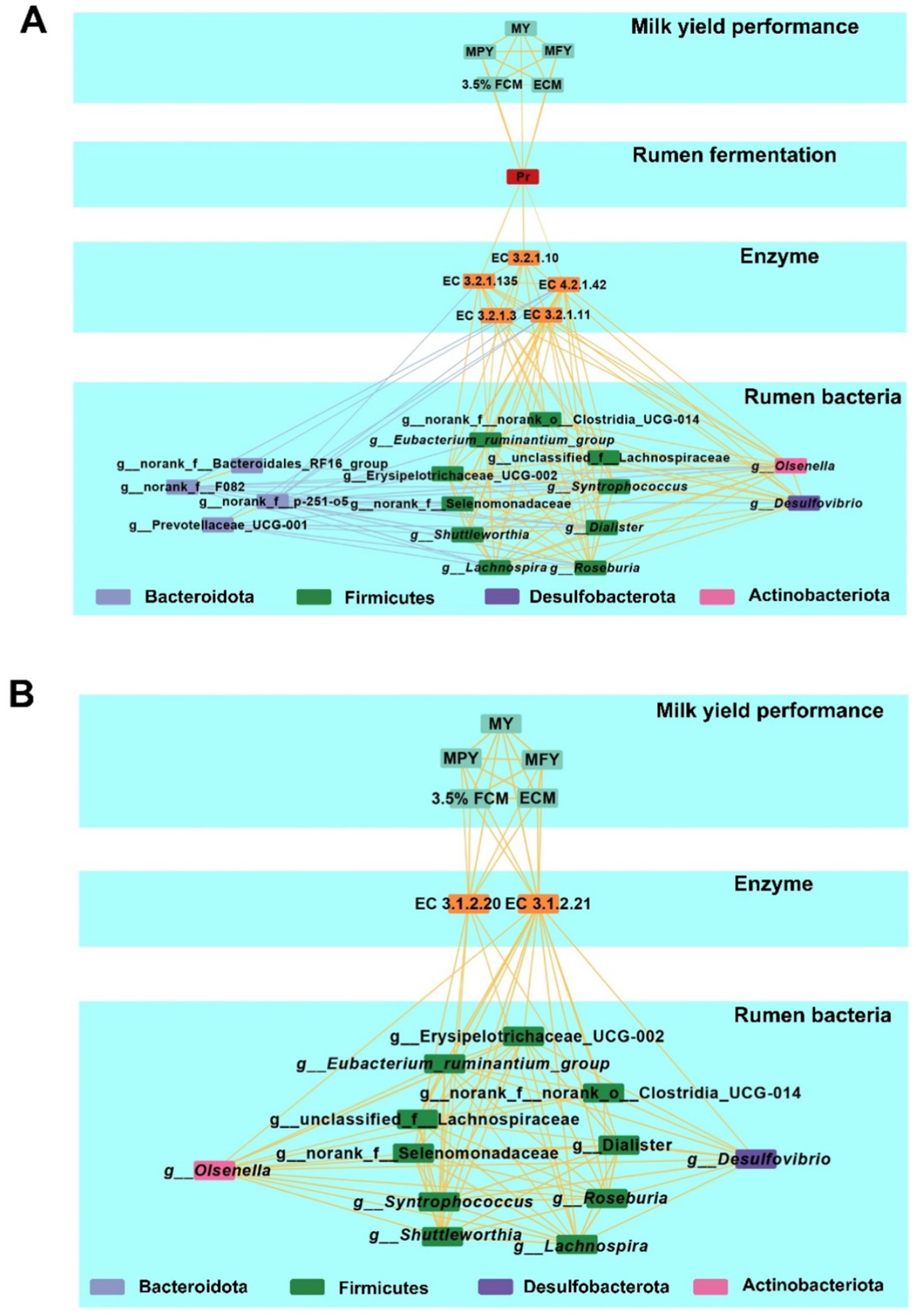
Figure 7. Multiplex networks showing the relationships between phenotypes. (A) Multiplex networks about the relationships between rumen bacteria, rumen carbohydrate-related KEGG enzymes, rumen fermentation parameter, and milk production phenotypes. (B) Multiplex networks showing the relationships between rumen bacteria, rumen lipid-related KEGG enzymes, and milk production phenotypes. Lines between two nodes represent the correlation, with a yellow line indicating a positive correlation and a blue line indicating a negative correlation; Line thickness represents the strength of the correlation (Spearman’s |r| >0.50 and p < 0.05).
Using a similar correlation-based network for MY-associated rectum bacteria, functional enzymes, fermentation parameters, serum parameters and milk production phenotypes (Supplementary Tables S12, 13 and Supplementary Figure S6), positive correlations were observed between the relative abundances of Moryella and the Christensenellaceae_R-7_group within the Firmicutes (p < 0.05). Moryella abundance was positively correlated with EC1.1.1.303 (p < 0.05), while Christensenellaceae_R-7_group abundance was positively correlated with EC1.1.1.4 (p < 0.05). Both EC1.1.1.303 and EC1.1.1.4 were negatively associated with rectum Bu concentrations, which were negatively correlated with serum GLB concentrations (p < 0.05). Notably, serum GLB concentrations were inversely associated with five milk production phenotypes (p < 0.05).
3.7 Classification of milk production in long-lived cows using rumen and rectum microbiota and phenotypic data in random-forest plots
Using rumen bacteria, rumen SCFAs, and serum parameters, a random forest model was applied to predict long-lived dairy cows with high production efficiency. The relative abundances of six bacterial taxa, Lachnospira, norank_f__Bacteroidales_RF16_group, Shuttleworthia, CAG-352, Lactobacillus, and Dialister, combined with rumem Hex concentration achieved the highest discrimination ability between LH and LL cows (AUC = 0.92, Figure 8A; Supplementary Figure S7A). Similarly, for rectum bacteria, rectum SCFAs, and serum parameters, the relative abundance of Parabacteroides, Breznakia, and unclassified_f__Eggerthellaceae, together with serum HDL-C, GLB, and rectum Bu concentrations, achieved near-perfect discriminatory power between LH and LL cows (AUC = 0.99, Figure 8B; Supplementary Figure S7B).
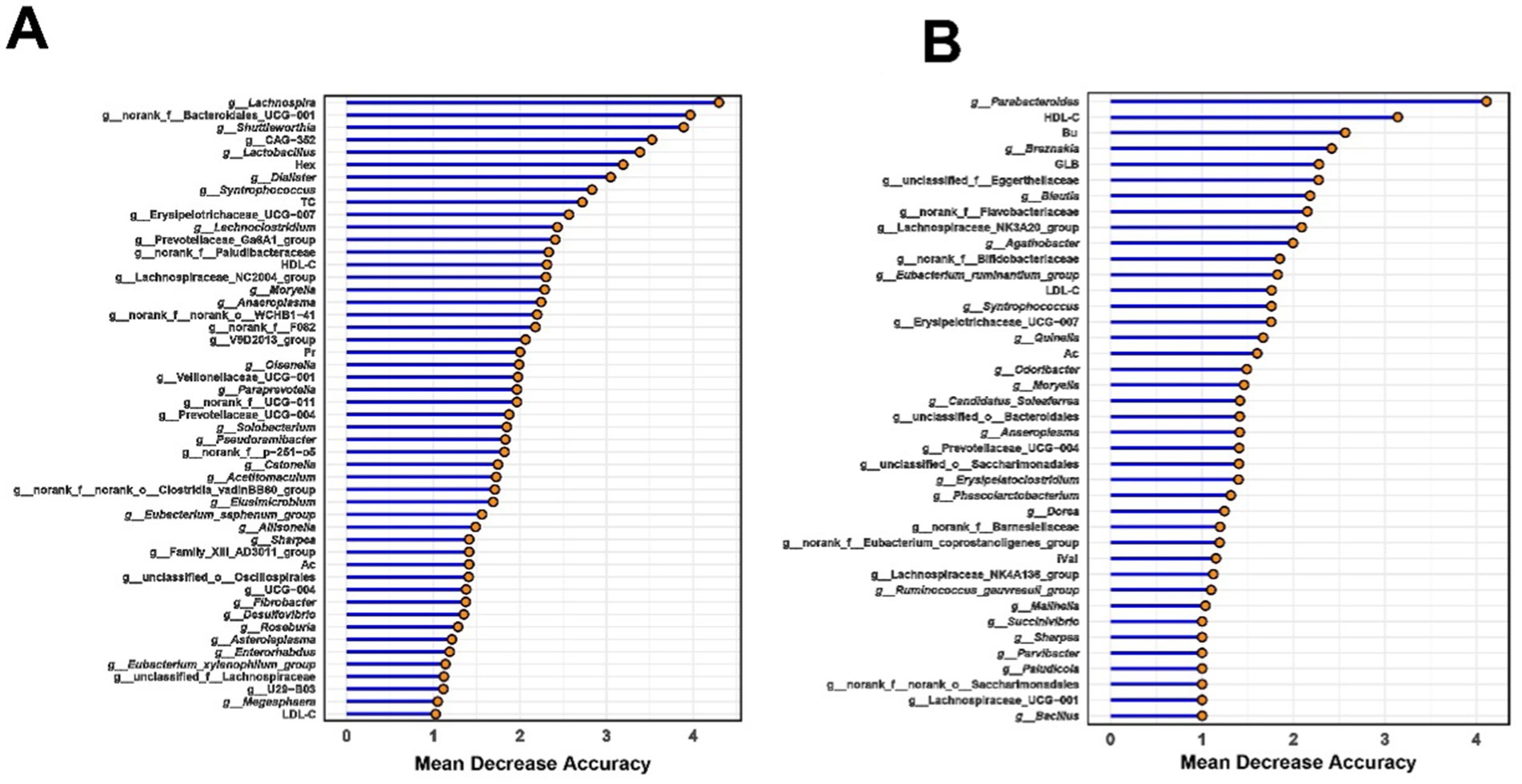
Figure 8. Random forest-based classification of milk yield in long-lived cows using (A) rumen and (B) rectum microbiota and phenotypic data. Important phenotypes were ranked by mean decrease in accuracy for classifying LH and LL groups. The classification model integrated SCFAs, serum parameters, and bacteria data to differentiate milk production categories using a random-forest algorithm.
4 Discussion
The gastrointestinal microbiota play crucial roles in maintaining milk production and health of dairy cows (Xue et al., 2018; Brulin et al., 2024) by directly influencing MY, productive lifespan, and lifetime productivity (Pinedo et al., 2010). Current research on dairy cow lifetime productivity primarily focuses on integrating pedigree with phenotypic and genomic data (Clasen et al., 2017; Zhang H. et al., 2024), which is useful, but the role of the gastrointestinal microbiota needs to receive similar attention. In this study, we compared the rumen and rectum bacterial communities and host phenotypes of high-yield cows and low-yield cows with long-term productive lifespans. The data show that rumen bacteria can modulate carbohydrate and lipid metabolism, thereby influencing milk production (Figures 5–7). Rectum bacteria can potentially affect milk production phenotypes by influencing rectum butyrate production (Supplementary Figures S4–S6).
The adaptation of dairy cows to high-energy total mixed rations (TMR) during peak lactation increases the Firmicutes: Bacteroidetes ratio, enhancing energy utilization efficiency. Within the Firmicutes phylum, bacteria such as the Lachnospiraceae, which ferment high energy substrates to produce acetate—a key precursor for milk fat synthesis—showed increased abundance with a positive correlation to milk fat yield (Jami et al., 2014; Wang et al., 2021). Prevotella, a member of the phylum Bacteroidetes, known for its ability to ferment carbohydrates and proteins, also dominated the microbial community (Betancur-Murillo et al., 2022). This enhanced the adaptation of rumen bacteria in high-yielding cows to lactation diets results in a more specialized and simplified microbial composition, reducing bacterial richness (Ogata et al., 2019a; Rivera-Chacon et al., 2024). Our findings also confirmed that long-lived high-yielding cows exhibit lower rumen bacterial richness (Figure 1A), reflecting their superior adaptation to lactation-specific diets. Moreover, no significant difference was observed in the rectum microbiome α-diversity in this study (Figure 1B), which may be due to the fact that the rectum microbiome is likely more influenced by long-term adaptation and is generally more stable compared to the rumen microbiome (Ogata et al., 2019b; Wang et al., 2023a).
Inflammatory levels in dairy cows increase with age, with older cows exhibiting significantly elevated levels of inflammatory cytokines such as TGF-β, TNF-α, and IL-10 (Zhang et al., 2019). In addition, high-yielding dairy cows experience greater metabolic stress, leading to increased concentrations of AST and ALB in the serum (Amin et al., 2022). In this study, no significant differences were observed between LH and LL cows in serum energy metabolism indicators, oxidative stress biomarkers, or inflammatory and immunological markers, and all measurements remained within normal ranges. This may be attributed to the good health status of cows with long-term productive lifespans, as cow health is an important determinant of productive longevity (Pinedo et al., 2010). Moreover, LH cows had significantly higher levels of TC, HDL-C, and LDL-C compared to LL cows, while TG and GLB levels were significantly lower in LH cows. Most lipids in milk exist in the form of milk fat globules, with cholesterol being a crucial component of these globules and also influencing their size (Maheshwari et al., 2024). LH cows exhibited higher MFY, and the demand for cholesterol in mammary epithelial cells was also higher; however, only 20% of the cholesterol in milk is synthesized in the mammary gland (Long et al., 1980). The majority of cholesterol in the mammary gland originates from liver-produced TC transported via the bloodstream (Viturro et al., 2009). LDL is endocytosed by LDL receptors such as CD36 on mammary epithelial cells, facilitating the transport of cholesterol (LDL-C) into mammary cells (Bionaz and Loor, 2008). HDL can collect excess cholesterol from peripheral tissues and transport it back to the liver or transfer it to LDL (Rader and Hovingh, 2014). HDL-C levels partially reflect HDL cholesterol transport function and are inversely associated with the incidence of cardiovascular diseases (Nazir et al., 2020). The increase in TC, HDL-C, and LDL-C in the serum of LH cows reflects their heightened demand for cholesterol and mobilization of other lipids. Triglycerides carried by VLDL are the primary source of fatty acids for mammary epithelial cells. TGs are hydrolyzed by lipoprotein lipase (LPL) on the surface of mammary endothelial cells into fatty acids for mammary utilization, with LPL activity and expression significantly increasing during lactation (Fielding and Frayn, 1998; Bionaz and Loor, 2008). The observed decrease in TG levels in this study may result from elevated LPL activity in LH cows. Elevated GLB levels are often associated with chronic inflammation (Sadat et al., 2023; Stanojević et al., 2023), indicating an increased risk of inflammation in LL cows (Zhang et al., 2019).
LH cows also have higher Pr concentrations in the rumen, which is positively correlated with higher milk production phenotypes. Previous research showed that rumen Pr concentration was positively correlated with MY (Xue et al., 2018). Heritable rumen microbes can reduce the A/P ratio to increase ECM production (Zhang C. et al., 2024). Consistent with previous studies, rumen-enriched core bacteria associated with milk production phenotypes, such as Syntrophococcus, Lachnospira, Shuttleworthia, Erysipelotrichaceae_UCG-2, and Roseburia, demonstrated significant positive correlation in this study with rumen Pr concentrations (Figure 1G).
Syntrophococcus and Lachnospira are primarily Ac-producing bacteria, while Erysipelotrichaceae_UCG-2 produces both lactate and Ac. Moreover, some Ac-producing bacteria, such as Syntrophococcus, can also produce CO₂ (Rode et al., 1981; Doré and Bryant, 1990). The production of lactate and CO₂ provides substrates for Pr-producing bacteria to synthesize propionate (Gonzalez-Garcia et al., 2017). Shuttleworthia and Roseburia produce butyrate and CO₂ (Zhao Y. et al., 2023; Zhang X. et al., 2024), thereby helping to maintain microbial homeostasis in the rumen environment and supporting propionate production (Ungerfeld, 2020). Butyrate serves as an energy source for rumen epithelial cells, promoting growth and development, and facilitating the absorption of SCFAs (Aschenbach et al., 2019). Previous studies suggested that the symbiotic relationships among these bacteria synergistically optimize energy flow in the rumen, promoting its development in calves and improving production performance in adult dairy cows (Hao et al., 2021; Kong et al., 2022). A multi-tiered network analysis has demonstrated that these bacteria can enhance the decomposition of polysaccharides such as pullulan, maltose/dextrin, and dextran by influencing five carbohydrate-related enzymes enriched in high-lactation dairy cows. This supports glycolysis and Pr production, thereby improving productivity (Figure 7A). Interestingly, it has also been found that these bacteria can influence two enzymes related to lipid metabolism, supporting a milk-producing phenotype (Figure 7B). These enzymes facilitate the production of medium-chain fatty acids (MCFAs) while suppressing the utilization of medium- and long-chain fatty acids (M/LCFAs) in the rumen. The inhibition of M/LCFA utilization in the rumen not only allows for a greater escape of M/LCFAs into the small intestine but also preserves functional fatty acids for milk fat synthesis. An increased flow of M/LCFAs to the small intestine for utilization can reduce the risk of excessive fat mobilization in high-producing dairy cows (De Beni Arrigoni et al., 2016; Bionaz et al., 2020). In the later stages of a cow’s productive lifespan, lipid metabolism regulated by the rumen microbiome appears to play a crucial role in sustaining production performance. Targeted modulation of lipid metabolism through microbiome interventions may represent a novel strategy for maintaining the productivity of dairy cows with extended productive lifespans.
Bu concentrations were elevated in the rectum contents of LH dairy cows (Figure 3B), while the Christensenellaceae_R-7_group and Moryella exhibited reduced abundance (Supplementary Table S4). These bacterial groups were negatively correlated with Bu level, and positively correlated with serum GLB (Figure 3D). Christensenellaceae R-7 group is known as a butyrate producer and is enriched in the gut of beef cattle suffering from ruminal acidosis. In high-LH dairy cows, the decreased abundance of the Christensenellaceae R-7 group appears to indicate a healthier hindgut microbial environment (Wu et al., 2024). Moryella has been demonstrated to coexist with pathogenic bacteria and is enriched in the rumens of cows with mastitis (Yuan et al., 2021; Png et al., 2022; Guo et al., 2024). A reduction in the abundance of the Christensenellaceae R-7 group and Moryella supports the maintenance of a balanced rectum microbiome, thereby favoring the production of SCFAs by hindgut microbes—especially butyrate, which plays a crucial role in maintaining intestinal barrier function and exerting anti-inflammatory effects. Our multi-layered network analysis revealed positive correlations between the relatively less abundant genera of the Christensenellaceae_R-7_group and Moryella, and two less abundant enzymes involved in the (R,R)-butane-2,3-diol synthesis pathway. These enzymes work together to facilitate the conversion of pyruvate to (R,R)-butanediol (García-Quintáns et al., 2008; Radoš et al., 2015). The decreased abundance of the two bacterial groups and enzymes may indirectly promote Bu synthesis by reducing the conversion of propionate to (R,R)-butane-2,3-diol. Increased Bu levels in the rectum enhance the expression of tight junction proteins and aid in the repair of the mucosal epithelium, which reduces both intestinal and systemic inflammation (Peng et al., 2009; Liu et al., 2024), may result in lower serum GLB concentrations in LH cows (Supplementary Figure S6). Additionally, enhanced hindgut health benefits the absorption of S/MCFAs (Jørgensen et al., 1998). Machine learning methods have been widely used to distinguish ruminants with different production performances (Xue et al., 2022; Wang et al., 2023a). In this study, it was found that combining rumen and rectal bacteria with phenotypic indicators can accurately predict cows with sustained productivity. Notably, the random forest machine learning algorithm revealed that the concentration of Hex (a rumen fermentation indicator), together with five rumen bacteria—Lachnospira, norank_f__Bacteroidales_RF16_group, Shuttleworthia, CAG-352, Lactobacillus, and Dialister—can serve as key microbial markers for distinguishing between LH and LL cows, achieving an accuracy of 92%. Similarly, rectum Parabacteroides, Breznakia, and unclassified_f__Eggerthellaceae, together with serum HDL-C, GLB, and rectum Bu, can also serve as key microbial markers for distinguishing between LH and LL cows, with an accuracy as high as 99% (Figure 8; Supplementary Figure S7). However, further expansion of the sample size and the integration of other omics data such as genomics and metabolomics are necessary for this conclusion to be applied in practice.
Although this study preliminarily investigated the effects of differential bacterial functions using PICRUSt2 predictions and correlation analysis, the inherent limitations of PICRUSt2 may result in discrepancies between predicted functions and actual biological processes (Sun et al., 2020). Therefore, future research should employ metagenomic sequencing to directly assess the presence and abundance of functional genes, providing comprehensive data for validation. Additionally, although all LH dairy cows in this study were managed under identical feeding and housing conditions to minimize non-genetic influences on the gut microbiome, host genetics play a crucial role in shaping and regulating the composition, function, and metabolites of the rumen microbiome through host-microbe interactions, which significantly affects lactation performance (Bai et al., 2024; Zhang C. et al., 2024). Future studies should explore how the host genotype modulates the composition of intestinal bacteria in cows and apply this knowledge to manipulating the gut microbiome by selective breeding of cows with long-term productive lifespans (see Figure 9).
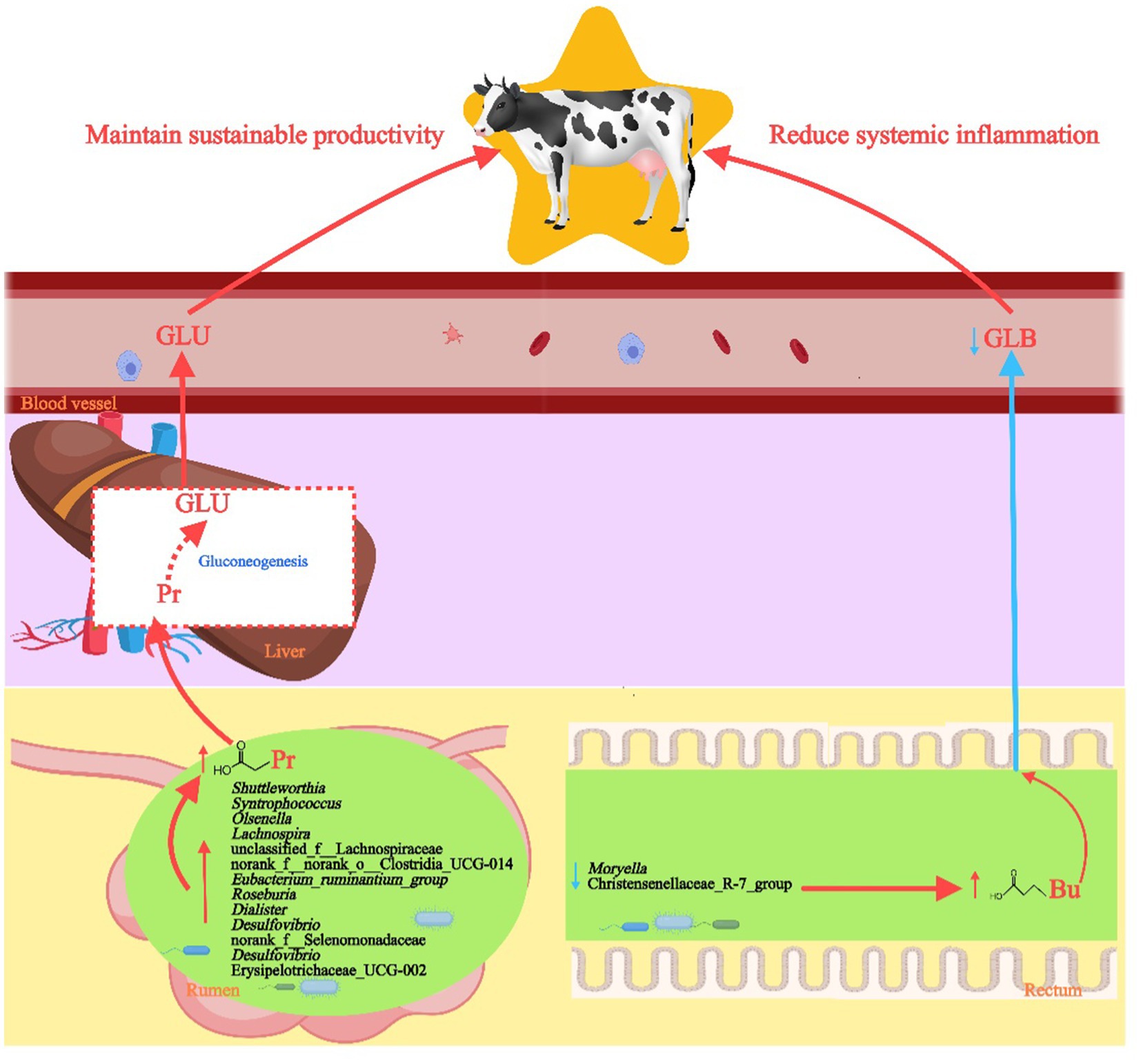
Figure 9. Diagram of the effects of rumen and rectum bacteria on the production performance of high-yielding and long-lived dairy cows. FFAs, free fatty acids; Gro, glycerol; M/LCFAs, medium and long-chain fatty acids; CM, chylomicron.
5 Conclusion
In summary, this study found that rumen and rectum bacteria potentially influence the production performance of dairy cows with long-term productive lifespans through different pathways (Figure 9). Specifically, LH cows exhibit increased abundance of rumen bacteria with carbohydrate metabolism capabilities that promote Pr production, such as Syntrophococcus, Lachnospira, Shuttleworthia, Erysipelotrichaceae_UCG-2, and Roseburia, along with decreased abundance of key rectum genera in the Christensenellaceae_R-7_group and Moryella, which favors the production of Bu. The rumen and rectum microbiomes, along with serum phenotypes, can also serve as effective biomarkers for selecting high-yielding dairy cows with extended productive lifespans. These findings enhance our understanding of how different microbiomes affect the sustained productivity of dairy cows over their lifetimes and open avenues for future research aimed at breeding cows with long-term productive lifespans and extending the productive lifespan of the entire dairy cow population through microbiome selection and interventions.
Data availability statement
Raw sequencing data have been deposited in the National Center for Biotechnology Information (NCBI) under BioProject accession number PRJNA1246358.
Ethics statement
The animal studies were approved by Animal Research and Technology Centre of Northwest A&F University. The studies were conducted in accordance with the local legislation and institutional requirements. Written informed consent was obtained from the owners for the participation of their animals in this study.
Author contributions
JY: Conceptualization, Data curation, Visualization, Writing – original draft. YL: Data curation, Methodology, Supervision, Writing – review & editing. MS: Data curation, Methodology, Supervision, Writing – review & editing. YZ: Data curation, Methodology, Supervision, Writing – review & editing. SG: Data curation, Methodology, Supervision, Writing – original draft. DZ: Data curation, Methodology, Supervision, Writing – review & editing. PL: Data curation, Methodology, Supervision, Writing – review & editing. AW: Data curation, Methodology, Supervision, Writing – review & editing. YJ: Conceptualization, Data curation, Formal analysis, Funding acquisition, Investigation, Methodology, Project administration, Resources, Software, Supervision, Validation, Visualization, Writing – original draft, Writing – review & editing.
Funding
The author(s) declare that financial support was received for the research and/or publication of this article. This study was supported by grants from the National Key R&D Program of China (No. 2023YFD1801100), Shaanxi Livestock and Poultry Breeding Double-Chain Fusion Key Project (Grant No. 2022GD-TSLD-46), and the Key R&D Program of Ningxia Hui Autonomous Region (Grant No. 2018BBF33001).
Acknowledgments
The authors would like to thank the reviewers for their valuable suggestions and insightful comments on this work.
Conflict of interest
The authors declare that the research was conducted in the absence of any commercial or financial relationships that could be construed as a potential conflict of interest.
Generative AI statement
The authors declare that no Gen AI was used in the creation of this manuscript.
Publisher’s note
All claims expressed in this article are solely those of the authors and do not necessarily represent those of their affiliated organizations, or those of the publisher, the editors and the reviewers. Any product that may be evaluated in this article, or claim that may be made by its manufacturer, is not guaranteed or endorsed by the publisher.
Supplementary material
The Supplementary material for this article can be found online at: https://www.frontiersin.org/articles/10.3389/fmicb.2025.1565034/full#supplementary-material
References
Amin, A. B., Zhang, L., Zhang, J., and Mao, S. (2022). Metagenomic and metabolomic insights into the mechanism underlying the disparity in milk yield of Holstein cows. Front. Microbiol. 13:844968. doi: 10.3389/fmicb.2022.844968
Antanaitis, R., Džermeikaitė, K., Krištolaitytė, J., Girdauskaitė, A., Arlauskaitė, S., Tolkačiovaitė, K., et al. (2024). The relation between milk lactose concentration and the rumination, feeding, and locomotion behavior of early-lactation dairy cows. Animals 14:836. doi: 10.3390/ani14060836
Aschenbach, J. R., Kristensen, N. B., Donkin, S. S., Hammon, H. M., and Penner, G. B. (2010). Gluconeogenesis in dairy cows: the secret of making sweet milk from sour dough. IUBMB Life 62, 869–877. doi: 10.1002/iub.400
Aschenbach, J. R., Zebeli, Q., Patra, A. K., Greco, G., Amasheh, S., and Penner, G. B. (2019). Symposium review: the importance of the ruminal epithelial barrier for a healthy and productive cow. J. Dairy Sci. 102, 1866–1882. doi: 10.3168/jds.2018-15243
Bai, H., Lai, Z., Zhang, J., Zheng, X., Zhang, J., Jin, W., et al. (2024). Host genetic regulation of specific functional groups in the rumen microbiome of dairy cows: implications for lactation trait. J. Adv. Res. doi: 10.1016/j.jare.2024.11.012
Barberán, A., Bates, S. T., Casamayor, E. O., and Fierer, N. (2012). Using network analysis to explore co-occurrence patterns in soil microbial communities. ISME J. 6, 343–351. doi: 10.1038/ismej.2011.119
Betancur-Murillo, C. L., Aguilar-Marín, S. B., and Jovel, J. (2022). Prevotella: a key player in ruminal metabolism. Microorganisms 11:1. doi: 10.3390/microorganisms11010001
Bionaz, M., and Loor, J. J. (2008). Gene networks driving bovine milk fat synthesis during the lactation cycle. BMC Genomics 9:366. doi: 10.1186/1471-2164-9-366
Bionaz, M., Vargas-Bello-Pérez, E., and Busato, S. (2020). Advances in fatty acids nutrition in dairy cows: from gut to cells and effects on performance. J. Anim. Sci. Biotechnol. 11:110. doi: 10.1186/s40104-020-00512-8
Brulin, L., Ducrocq, S., Even, G., Sanchez, M. P., Martel, S., Merlin, S., et al. (2024). Short communication: Bifidobacterium abundance in the faecal microbiota is strongly associated with milk traits in dairy cattle. Animal 18:101243. doi: 10.1016/j.animal.2024.101243
Chen, S., Zhou, Y., Chen, Y., and Gu, J. (2018). fastp: an ultra-fast all-in-one FASTQ preprocessor. Bioinformatics 34, i884–i890. doi: 10.1093/bioinformatics/bty560
Clasen, J. B., Norberg, E., Madsen, P., Pedersen, J., and Kargo, M. (2017). Estimation of genetic parameters and heterosis for longevity in crossbred Danish dairy cattle. J. Dairy Sci. 100, 6337–6342. doi: 10.3168/jds.2017-12627
De Beni Arrigoni, M., Martins, C. L., and Factori, M. A. (2016). “Lipid metabolism in the rumen” in Rumenology (Cham: Springer), 103–126.
De Vries, A. (2020). Symposium review: why revisit dairy cattle productive lifespan? J. Dairy Sci. 103, 3838–3845. doi: 10.3168/jds.2019-17361
De Vries, A., and Marcondes, M. I. (2020). Review: overview of factors affecting productive lifespan of dairy cows. Animal 14, s155–s164. doi: 10.1017/s1751731119003264
Doré, J., and Bryant, M. P. (1990). Metabolism of one-carbon compounds by the ruminal acetogen Syntrophococcus sucromutans. Appl. Environ. Microbiol. 56, 984–989. doi: 10.1128/aem.56.4.984-989.1990
Edgar, R. C. (2013). UPARSE: highly accurate OTU sequences from microbial amplicon reads. Nat. Methods 10, 996–998. doi: 10.1038/nmeth.2604
Edmonson, A. J., Lean, I. J., Weaver, L. D., Farver, T., and Webster, G. (1989). A body condition scoring chart for Holstein dairy cows. J. Dairy Sci. 72, 68–78. doi: 10.3168/jds.S0022-0302(89)79081-0
Fielding, B. A., and Frayn, K. N. (1998). Lipoprotein lipase and the disposition of dietary fatty acids. Br. J. Nutr. 80, 495–502. doi: 10.1017/s0007114598001585
Francis, F. L., Rusche, W. C., LaFleur, D., Hergenreder, J. E., and Smith, Z. K. (2024). Dose effects of encapsulated butyric acid and zinc on beef feedlot steer growth performance, dietary net energy utilization, rumen morphometrics, small intestine histology, and carcass characteristics. J. Anim. Sci. 102:skae240. doi: 10.1093/jas/skae240
García-Quintáns, N., Repizo, G., Martín, M., Magni, C., and López, P. (2008). Activation of the diacetyl/acetoin pathway in Lactococcus lactis subsp. lactis bv. diacetylactis CRL264 by acidic growth. Appl. Environ. Microbiol. 74, 1988–1996. doi: 10.1128/aem.01851-07
Gonzalez-Garcia, R. A., McCubbin, T., Navone, L., Stowers, C., Nielsen, L. K., and Marcellin, E. (2017). Microbial propionic acid production. Fermentation 3:21. doi: 10.3390/fermentation3020021
Grandl, F., Furger, M., Kreuzer, M., and Zehetmeier, M. (2019). Impact of longevity on greenhouse gas emissions and profitability of individual dairy cows analysed with different system boundaries. Animal 13, 198–208. doi: 10.1017/s175173111800112x
Gu, F., Zhu, S., Hou, J., Tang, Y., Liu, J. X., Xu, Q., et al. (2023a). The hindgut microbiome contributes to host oxidative stress in postpartum dairy cows by affecting glutathione synthesis process. Microbiome 11:87. doi: 10.1186/s40168-023-01535-9
Gu, F., Zhu, S., Tang, Y., Liu, X., Jia, M., Malmuthuge, N., et al. (2023b). Gut microbiome is linked to functions of peripheral immune cells in transition cows during excessive lipolysis. Microbiome 11:40. doi: 10.1186/s40168-023-01492-3
Guo, C., Liu, J., Wei, Y., Du, W., and Li, S. (2024). Comparison of the gastrointestinal bacterial microbiota between dairy cows with and without mastitis. Front. Microbiol. 15:1332497. doi: 10.3389/fmicb.2024.1332497
Han, R., Kok, A., Mourits, M., and Hogeveen, H. (2024). Effects of extending dairy cow longevity by adjusted reproduction management decisions on partial net return and greenhouse gas emissions: a dynamic stochastic herd simulation study. J. Dairy Sci. 107, 6902–6912. doi: 10.3168/jds.2023-24089
Hao, Y., Guo, C., Gong, Y., Sun, X., Wang, W., Wang, Y., et al. (2021). Rumen fermentation, digestive enzyme activity, and bacteria composition between pre-weaning and post-weaning dairy calves. Animals 11:2527. doi: 10.3390/ani11092527
Headey, D. (2023). Can dairy help solve the malnutrition crisis in developing countries? An economic analysis. Anim. Front. 13, 7–16. doi: 10.1093/af/vfac083
Hu, X., Li, S., Mu, R., Guo, J., Zhao, C., Cao, Y., et al. (2022). The rumen microbiota contributes to the development of mastitis in dairy cows. Microbiol. Spectr. 10:e0251221. doi: 10.1128/spectrum.02512-21
Hu, H., Mu, T., Ma, Y., Wang, X., and Ma, Y. (2021). Analysis of longevity traits in Holstein cattle: a review. Front. Genet. 12:695543. doi: 10.3389/fgene.2021.695543
Jami, E., White, B. A., and Mizrahi, I. (2014). Potential role of the bovine rumen microbiome in modulating milk composition and feed efficiency. PLoS One 9:e85423. doi: 10.1371/journal.pone.0085423
Jørgensen, J., Holtug, K., Jeppesen, P. B., and Mortensen, P. B. (1998). Human rectal absorption of short- and medium-chain C2–C10 fatty acids. Scand. J. Gastroenterol. 33, 590–594. doi: 10.1080/00365529850171846
Kang, X., Li, C., Liu, S., Baldwin, R. L. T., Liu, G. E., and Li, C. J. (2023). Genome-wide acetylation modification of H3K27ac in bovine rumen cell following butyrate exposure. Biomol. Ther. 13:1137. doi: 10.3390/biom13071137
Kong, F., Zhang, Y., Wang, S., Cao, Z., Liu, Y., Zhang, Z., et al. (2022). Acremonium terricola culture’s dose-response effects on lactational performance, antioxidant capacity, and ruminal characteristics in Holstein dairy cows. Antioxidants 11:175. doi: 10.3390/antiox11010175
Larson, B. L. (1965). Biosynthesis of the milk proteins. J. Dairy Sci. 48, 133–139. doi: 10.3168/jds.s0022-0302(65)88178-4
Liu, M., Ma, J., Xu, J., Huangfu, W., Zhang, Y., Ali, Q., et al. (2024). Fecal microbiota transplantation alleviates intestinal inflammatory diarrhea caused by oxidative stress and pyroptosis via reducing gut microbiota-derived lipopolysaccharides. Int. J. Biol. Macromol. 261:129696. doi: 10.1016/j.ijbiomac.2024.129696
Liu, C., Zhao, D., Ma, W., Guo, Y., Wang, A., Wang, Q., et al. (2016). Denitrifying sulfide removal process on high-salinity wastewaters in the presence of Halomonas sp. Appl. Microbiol. Biotechnol. 100, 1421–1426. doi: 10.1007/s00253-015-7039-6
Long, C. A., Patton, S., and McCarthy, R. D. (1980). Origins of the cholesterol in milk. Lipids 15, 853–857. doi: 10.1007/bf02534376
Ma, C., Sun, Z., Zeng, B., Huang, S., Zhao, J., Zhang, Y., et al. (2018). Cow-to-mouse fecal transplantations suggest intestinal microbiome as one cause of mastitis. Microbiome 6:200. doi: 10.1186/s40168-018-0578-1
Magoč, T., and Salzberg, S. L. (2011). FLASH: fast length adjustment of short reads to improve genome assemblies. Bioinformatics 27, 2957–2963. doi: 10.1093/bioinformatics/btr507
Maheshwari, A., Mantry, H., Bagga, N., Frydrysiak-Brzozowska, A., Badarch, J., and Rahman, M. M. (2024). Milk fat globules: 2024 updates. Newborn 3, 19–37. doi: 10.5005/jp-journals-11002-0085
Nazir, S., Jankowski, V., Bender, G., Zewinger, S., Rye, K. A., and van der Vorst, E. P. C. (2020). Interaction between high-density lipoproteins and inflammation: function matters more than concentration! Adv. Drug Deliv. Rev. 159, 94–119. doi: 10.1016/j.addr.2020.10.006
Ogata, T., Kim, Y. H., Masaki, T., Iwamoto, E., Ohtani, Y., Orihashi, T., et al. (2019a). Effects of an increased concentrate diet on rumen pH and the bacterial community in Japanese Black beef cattle at different fattening stages. J. Vet. Med. Sci. 81, 968–974. doi: 10.1292/jvms.19-0077
Ogata, T., Makino, H., Ishizuka, N., Iwamoto, E., Masaki, T., Ikuta, K., et al. (2019b). Long-term high-grain diet altered the ruminal pH, fermentation, and composition and functions of the rumen bacterial community, leading to enhanced lactic acid production in Japanese Black beef cattle during fattening. PLoS One 14:e0225448. doi: 10.1371/journal.pone.0225448
Organization for Economic Co-Operation and Development, Food and Agriculture Organization of the United Nations (2023). OECD-FAO agricultural outlook 2023–2032. Paris: OECD Publishing.
Ospina, P. A., Nydam, D. V., Stokol, T., and Overton, T. R. (2010). Association between the proportion of sampled transition cows with increased nonesterified fatty acids and beta-hydroxybutyrate and disease incidence, pregnancy rate, and milk production at the herd level. J. Dairy Sci. 93, 3595–3601. doi: 10.3168/jds.2010-3074
Peng, L., Li, Z. R., Green, R. S., Holzman, I. R., and Lin, J. (2009). Butyrate enhances the intestinal barrier by facilitating tight junction assembly via activation of AMP-activated protein kinase in Caco-2 cell monolayers. J. Nutr. 139, 1619–1625. doi: 10.3945/jn.109.104638
Pinedo, P. J., De Vries, A., and Webb, D. W. (2010). Dynamics of culling risk with disposal codes reported by dairy herd improvement dairy herds. J. Dairy Sci. 93, 2250–2261. doi: 10.3168/jds.2009-2572
Png, C. W., Lee, W. J. J., Chua, S. J., Zhu, F., Yeoh, K. G., and Zhang, Y. (2022). Mucosal microbiome associates with progression to gastric cancer. Theranostics 12, 48–58. doi: 10.7150/thno.65302
Quast, C., Pruesse, E., Yilmaz, P., Gerken, J., Schweer, T., Yarza, P., et al. (2013). The SILVA ribosomal RNA gene database project: improved data processing and web-based tools. Nucleic Acids Res. 41, D590–D596. doi: 10.1093/nar/gks1219
Rader, D. J., and Hovingh, G. K. (2014). HDL and cardiovascular disease. Lancet 384, 618–625. doi: 10.1016/s0140-6736(14)61217-4
Radoš, D., Carvalho, A. L., Wieschalka, S., Neves, A. R., Blombach, B., Eikmanns, B. J., et al. (2015). Engineering Corynebacterium glutamicum for the production of 2,3-butanediol. Microb. Cell Fact. 14:171. doi: 10.1186/s12934-015-0362-x
Rivera-Chacon, R., Pacífico, C., Ricci, S., Petri, R. M., Reisinger, N., Zebeli, Q., et al. (2024). Prolonged feeding of high-concentrate diet remodels the hindgut microbiome and modulates nutrient degradation in the rumen and the total gastrointestinal tract of cows. J. Dairy Sci. 107, 9235–9250. doi: 10.3168/jds.2024-24919
Rode, L. M., Genthner, B. R., and Bryant, M. P. (1981). Syntrophic association by cocultures of the methanol- and CO2-H2-utilizing species Eubacterium limosum and pectin-fermenting Lachnospira multiparus during growth in a pectin medium. Appl. Environ. Microbiol. 42, 20–22. doi: 10.1128/aem.42.1.20-22.1981
Sadat, A., Farag, A. M. M., Elhanafi, D., Awad, A., Elmahallawy, E. K., Alsowayeh, N., et al. (2023). Immunological and oxidative biomarkers in bovine serum from healthy, clinical, and sub-clinical mastitis caused by Escherichia coli and Staphylococcus aureus infection. Animals 13:892. doi: 10.3390/ani13050892
Segata, N., Izard, J., Waldron, L., Gevers, D., Miropolsky, L., Garrett, W. S., et al. (2011). Metagenomic biomarker discovery and explanation. Genome Biol. 12:R60. doi: 10.1186/gb-2011-12-6-r60
Shannon, P., Markiel, A., Ozier, O., Baliga, N. S., Wang, J. T., Ramage, D., et al. (2003). Cytoscape: a software environment for integrated models of biomolecular interaction networks. Genome Res. 13, 2498–2504. doi: 10.1101/gr.1239303
Shrestha, B., Paudyal, S., Kaniyamattam, K., and Grohn, Y. T. (2025). Organic dairy cattle longevity and economic implications: contemporary perspectives. J. Dairy Sci. 108, 3734–3745. doi: 10.3168/jds.2024-25767
Si, B., Liu, K., Huang, G., Chen, M., Yang, J., Wu, X., et al. (2023). Relationship between rumen bacterial community and milk fat in dairy cows. Front. Microbiol. 14:1247348. doi: 10.3389/fmicb.2023.1247348
Stanojević, J., Kreszinger, M., Radinović, M., Kladar, N., Tomanić, D., Ružić, Z., et al. (2023). Assessment of mastitis patterns in Serbian dairy cows: blood serum metabolic profile and milk composition parameters. Pathogens 12:1349. doi: 10.3390/pathogens12111349
Sun, S., Jones, R. B., and Fodor, A. A. (2020). Inference-based accuracy of metagenome prediction tools varies across sample types and functional categories. Microbiome 8:46. doi: 10.1186/s40168-020-00815-y
Tang, R., Yang, W., Song, J., Xiang, K., Li, S., Zhao, C., et al. (2024). The rumen microbiota contributed to the development of mastitis induced by subclinical ketosis. Microb. Pathog. 187:106509. doi: 10.1016/j.micpath.2023.106509
Toral, P. G., Monahan, F. J., Hervás, G., Frutos, P., and Moloney, A. P. (2018). Review: modulating ruminal lipid metabolism to improve the fatty acid composition of meat and milk. Challenges and opportunities. Animal 12, s272–s281. doi: 10.1017/s1751731118001994
Traag, V. A., Waltman, L., and van Eck, N. J. (2019). From Louvain to Leiden: guaranteeing well-connected communities. Sci. Rep. 9:5233. doi: 10.1038/s41598-019-41695-z
Ungerfeld, E. M. (2020). Metabolic hydrogen flows in rumen fermentation: principles and possibilities of interventions. Front. Microbiol. 11:589. doi: 10.3389/fmicb.2020.00589
Viturro, E., Koenning, M., Kroemer, A., Schlamberger, G., Wiedemann, S., Kaske, M., et al. (2009). Cholesterol synthesis in the lactating cow: induced expression of candidate genes. J. Steroid Biochem. Mol. Biol. 115, 62–67. doi: 10.1016/j.jsbmb.2009.02.011
Wang, D., Chen, L., Tang, G., Yu, J., Chen, J., Li, Z., et al. (2023a). Multi-omics revealed the long-term effect of ruminal keystone bacteria and the microbial metabolome on lactation performance in adult dairy goats. Microbiome 11:215. doi: 10.1186/s40168-023-01652-5
Wang, X., Li, X., Zhao, C., Hu, P., Chen, H., Liu, Z., et al. (2012). Correlation between composition of the bacterial community and concentration of volatile fatty acids in the rumen during the transition period and ketosis in dairy cows. Appl. Environ. Microbiol. 78, 2386–2392. doi: 10.1128/aem.07545-11
Wang, Y., Nan, X., Zhao, Y., Wang, Y., Jiang, L., and Xiong, B. (2021). Ruminal degradation of rumen-protected glucose influences the ruminal microbiota and metabolites in early-lactation dairy cows. Appl. Environ. Microbiol. 87:e01908. doi: 10.1128/aem.01908-20
Wang, D., Tang, G., Zhao, L., Wang, M., Chen, L., Zhao, C., et al. (2023b). Potential roles of the rectum keystone microbiota in modulating the microbial community and growth performance in goat model. J. Anim. Sci. Biotechnol. 14:55. doi: 10.1186/s40104-023-00850-3
Ward, T., Larson, J., Meulemans, J., Hillmann, B., Lynch, J., Sidiropoulos, D., et al. (2017). BugBase predicts organism-level microbiome phenotypes. bioRxiv. Available online at: https://doi.org/10.1101/133462. [Epub ahead of preprint].
Wu, F., Ji, P., Yang, H., Zhu, X., and Wu, X. (2024). Interpretation of the effects of rumen acidosis on the gut microbiota and serum metabolites in calves based on 16S rDNA sequencing and non-target metabolomics. Front. Cell. Infect. Microbiol. 14:1427763. doi: 10.3389/fcimb.2024.1427763
Xu, T., Ma, N., Wang, Y., Shi, X., Chang, G., Loor, J. J., et al. (2018). Sodium butyrate supplementation alleviates the adaptive response to inflammation and modulates fatty acid metabolism in lipopolysaccharide-stimulated bovine hepatocytes. J. Agric. Food Chem. 66, 6281–6290. doi: 10.1021/acs.jafc.8b01439
Xue, M., Sun, H., Wu, X., Guan, L. L., and Liu, J. (2018). Assessment of rumen microbiota from a large dairy cattle cohort reveals the pan and core bacteriomes contributing to varied phenotypes. Appl. Environ. Microbiol. 84:e00970. doi: 10.1128/aem.00970-18
Xue, M. Y., Sun, H. Z., Wu, X. H., Liu, J. X., and Guan, L. L. (2020). Multi-omics reveals that the rumen microbiome and its metabolome together with the host metabolome contribute to individualized dairy cow performance. Microbiome 8:64. doi: 10.1186/s40168-020-00819-8
Xue, M. Y., Xie, Y. Y., Zhong, Y., Ma, X. J., Sun, H. Z., and Liu, J. X. (2022). Integrated meta-omics reveals new ruminal microbial features associated with feed efficiency in dairy cattle. Microbiome 10:32. doi: 10.1186/s40168-022-01228-9
Yeoman, C. J., and White, B. A. (2014). Gastrointestinal tract microbiota and probiotics in production animals. Annu. Rev. Anim. Biosci. 2, 469–486. doi: 10.1146/annurev-animal-022513-114149
Yuan, D., Chen, W., Qin, J., Shen, D., Qiao, Y., and Kong, B. (2021). Associations between bacterial vaginosis, candida vaginitis, trichomonas vaginalis, and vaginal pathogenic community in Chinese women. Am. J. Transl. Res. 13, 7148–7155.
Zhang, C., Liu, H., Jiang, X., Zhang, Z., Hou, X., Wang, Y., et al. (2024). An integrated microbiome- and metabolome-genome-wide association study reveals the role of heritable ruminal microbial carbohydrate metabolism in lactation performance in Holstein dairy cows. Microbiome 12:232. doi: 10.1186/s40168-024-01937-3
Zhang, X., Lv, J., Hui, J., Wu, A., Zhao, L., Feng, L., et al. (2024). Dietary saccharin sodium supplementation improves the production performance of dairy goats without residue in milk in summer. Anim. Nutr. 18, 166–176. doi: 10.1016/j.aninu.2024.04.018
Zhang, G., Wang, Y., Luo, H., Qiu, W., Zhang, H., Hu, L., et al. (2019). The association between inflammaging and age-related changes in the ruminal and fecal microbiota among lactating Holstein cows. Front. Microbiol. 10:1803. doi: 10.3389/fmicb.2019.01803
Zhang, H., Wang, A., Xiao, W., Mi, S., Hu, L., Brito, L. F., et al. (2024). Genetic parameters and genome-wide association analyses for lifetime productivity in Chinese Holstein cattle. J. Dairy Sci. 107, 9638–9655. doi: 10.1016/j.jods.2024.10.001
Zhao, C., Bao, L., Qiu, M., Wu, K., Zhao, Y., Feng, L., et al. (2022). Commensal cow Roseburia reduces gut-dysbiosis-induced mastitis through inhibiting bacterial translocation by producing butyrate in mice. Cell Rep. 41:111681. doi: 10.1016/j.celrep.2022.111681
Zhao, C., Hu, X., Qiu, M., Bao, L., Wu, K., Meng, X., et al. (2023). Sialic acid exacerbates gut dysbiosis-associated mastitis through the microbiota-gut-mammary axis by fueling gut microbiota disruption. Microbiome 11:78. doi: 10.1186/s40168-023-01528-8
Zhao, Y., Yu, S., Li, L., Zhao, H., Li, Y., Jiang, L., et al. (2023). Feeding citrus flavonoid extracts decreases bacterial endotoxin and systemic inflammation and improves immunometabolic status by modulating hindgut microbiome and metabolome in lactating dairy cows. Anim Nutr 13, 386–400. doi: 10.1016/j.aninu.2023.03.007
Keywords: rumen bacteria, rectum bacteria, production performances, milk yield, productive lifespan
Citation: Yang J, Li Y, Sun M, Zhang Y, Guo S, Zhou D, Lin P, Wang A and Jin Y (2025) Associations of rumen and rectum bacteria with the sustained productive performance of dairy cows. Front. Microbiol. 16:1565034. doi: 10.3389/fmicb.2025.1565034
Edited by:
Alejandro Belanche, University of Zaragoza, SpainReviewed by:
Waroon Khota, Khon Kaen University, ThailandJulia María Alatorre Cruz, Meritorious Autonomous University of Puebla, Mexico
Copyright © 2025 Yang, Li, Sun, Zhang, Guo, Zhou, Lin, Wang and Jin. This is an open-access article distributed under the terms of the Creative Commons Attribution License (CC BY). The use, distribution or reproduction in other forums is permitted, provided the original author(s) and the copyright owner(s) are credited and that the original publication in this journal is cited, in accordance with accepted academic practice. No use, distribution or reproduction is permitted which does not comply with these terms.
*Correspondence: Yaping Jin, eWFwaW5namluQG53YWZ1LmVkdS5jbg==