- 1National Tuberculosis Reference Laboratory, Chinese Center for Disease Control and Prevention, Beijing, China
- 2Beijing Center for Disease Control and Prevention, Beijing Institute of Tuberculosis Control, Institute for Immunization and Prevention, Beijing Academy for Preventive Medicine, Beijing, China
- 3Laboratory of Respiratory Diseases, Beijing Key Laboratory of Pediatric Respiratory Infection Diseases, Beijing Children’s Hospital, Key Laboratory of Major Diseases in Children, Ministry of Education, National Clinical Research Center for Respiratory Diseases, National Center for Children’s Health, Beijing Pediatric Research Institute, Capital Medical University, Beijing, China
To analyze subtypes, microbiological characteristics and antimicrobial susceptibility of Mycobacterium kansasii in China, a total of 153 M. kansasii isolates, collected from national drug resistance surveillance, were genotyped with whole genome sequencing and explored the antimicrobial susceptibility with broth microdilution. All isolates were classified as M. kansasii type I based on Average Nucleotide Identity(ANI). The 153 M. kansasii representatives were differentiated into 3 clusters with 141 genotypes, including 17 isolates from a cluster and 136 isolates with unique patterns. The EXS-1, EXS-3 and EXS-5 regions were involved in all isolates. Rifabutin and clarithromycin were the most highly active against M. kansasii strains, with the susceptible rate of 100 and 99.35%, respectively. Followed by amikacin and linezolid, the resistance rate was 5.88 and 7.19%, respectively. The resistance rate to rifampin (RIF) was 22.22%. As for the antibiotics without the breakpoint values, all isolates had very low MIC50 (0.03 μg/mL) and MIC90 (≤0.06 μg/mL) values against bedaquiline, sutezolid, delamanid, and clofazimine. Except for ciprofloxacin and moxifloxacin, the resistance rate of other drugs in cluster 3 was higher than that in cluster 1 and cluster 2. In conclusion, M. kansasii type I was the predominant genotype in China, and rifabutin and clarithromycin presented strong activities. The new drugs, used for the treatment of multidrug - resistant tuberculosis, have the potential to be potent agents in the treatment of M. kansasii infection. The clustering might contribute to the high resistance rate of M. kansasii.
Introduction
Mycobacterium kansasii, a leading cause of pulmonary, extrapulmonary and disseminated diseases in immunocompromised individuals, stands out as one of the most frequently isolated non-tuberculous mycobacterial (NTM) species worldwide (Hoefsloot et al., 2013; Campo and Campo, 1997; Pintado et al., 1999). Initially, seven subtypes (I-VII) along with two intermediate (I/II) and atypical (IIb) types, were taxonomically reclassified as species-level members of the M. kansasii complex (MKC) (Taillard et al., 2003; Iwamoto and Saito, 2006; Tagini et al., 2019; Jagielski et al., 2020). Though multiple molecular techniques have been developed to genotype M. kansasii, the resolution was low when relying solely on certain gene sequences (Zhang et al., 2004). Therefore, we conducted whole genome sequence (WGS) to enable high-resolution subtyping analysis.
Due to the difference in the pathogenicity of M. kansasii subtypes, it is necessary to perform drug susceptibility testing (DRS) to predict the clinical outcome of patients infected with M. kansasii (Kim et al., 2021; Li et al., 2016). Daily therapy with isoniazid (INH), rifampin (RIF), and ethambutol (EMB) was recommended by the American Thoracic Society/Infectious Disease Society of America (ATS/IDSA) for M. kansasii (Griffith et al., 2007). Given that treatment failure is associated with RIF resistance, there is an urgent need to conduct DRS for other medications (Griffith et al., 2007). Besides, considering the natural resistance to most antibacterial drugs for NTM, novel and more effective antibiotics were urgently needed (Raju et al., 2016). Whereas data on DRS of M. kansasii was limited. Therefore, this study was designed to analyze subtypes, microbiological characteristics and antimicrobial susceptibility of M. kansasii isolates in China.
Materials and methods
M. kansasii isolates and genotyping
Sputum specimens were collected from suspected pulmonary tuberculosis patients with acid-fast bacilli-positive isolates, from 8 provinces and 3 municipalities included in the Chinese Drug Resistance Surveillance Program (DRS) running between 2016 and 2020. Patients were excluded if the samples were unqualified. Clinical samples treated with NALC-NaOH were subsequently cultured on differential medium containing paranitrobenzoic acid (PNB) and thiophen-2-carboxylic acid hydrazide (TCH) to distinguish NTM from Mycobacterium tuberculosis complex (MTBC). Isolates identified as NTM using biochemical method were further identified as MKC by sequencing the genes encoding 16S rRNA, hsp65, rpoB, and the 16S–23S rRNA internal transcribed spacer (ITS), and non-purified isolates were excluded from further analysis. The MKC strains were further re-identified by MALDI-TOF-MS, representing I-VII genotypes of Mycobacterium kansasii spectra reference database, followed by WGS, which resulted in the final identification of all isolates as M. kansasii.
Genome sequencing
All genomic DNA was extracted using the cetyl-trimethyl-ammonium bromide (CTAB) method, as previously described (Kigozi et al., 2018). Extracted genomic DNA was quantified using a Qubit 2.0 Fluorometer (Thermo Fisher, Singapore). Genomic DNA libraries were constructed using the Illumina Nextera kit according to the manufacturer’s protocol. Each library was sequenced using an Illumina NovaSeq 6,000 system (Illumina, Inc.) that generates 150-bp paired-reads from each end (read length of 2 × 150 bp) for each DNA fragment in a library. In order to secure exhaustive coverage of the target genome, each sample underwent sequencing to reach a depth of 150x and the genome coverage was requested to be above 90%. All whole-genome sequencing procedures were performed by Annoroad Gene Technology (Beijing, China).
The Raw Data were filtered to remove contaminants and low - quality reads before analysis. SPAdes (v3.15.5) and Unicycler (v0.5.0) were used for assembly in three steps: correction, assembly and mismatch correction. GapFiller (v1.11) optimized the assembly and filled gaps. Seqkit (v2.4.0) filtered out fragments < 200 bp for the final result. Prokka (v1.14.5) was used for annotation.
Identifying
Resulting genomic sequences were analyzed to identify distinct subspecies according to average nucleotide identity (ANI) values, which were calculated using pyani.1 The results were visualized as a heatmap using R package ComplexHeatmap (Gu et al., 2016). Isolates yielding genome sequences with ANI values of >95% were deemed members of the same species (Kim et al., 2014). The utilization of ANI values for species - level discrimination can significantly enhance the resolution of identification, enabling more precise differentiation at the subspecies level.
Phylogenetic tree construction
Core single nucleotide polymorphisms (SNPs) in genome sequences obtained for each isolate were detected by mapping reads to the M. kansasii reference strain (ATCC12478) sequence using Snippy v4.4.5, then core SNPs were concatenated and aligned using the snippy-multi script. A maximum likelihood tree was generated based on core-SNP alignment then the resulting tree was visualized using the Interactive Tree of Life (iTOL) online tool (Minh et al., 2020; Letunic and Bork, 2011).
Virulence identification
Contigs were screened to detect known virulence genes using ABRicate and the Virulence Factors Database (VFDB). Next, virulence factor analysis was performed on potential virulence gene sequences with sequence coverage rates of ≥85% and rates of sequence identity with known virulence genes of ≥85% (Guo et al., 2022). Heatmaps were generated using the heatmap function of the R package.
Antimycobacterial drug susceptibility testing
Antimicrobial susceptibility testing was performed on Sensititre SLOMYCO® plates (Thermo Fisher Scientific, United States) according to Clinical and Laboratory Standards Institute (CLSI) guidelines (Parrish, 2023). Minimal inhibitory concentrations (MICs) determined within the concentration range of 0.0625–128 mg/mL were performed for a total of 17 antimicrobial agents (Table 1). The MIC is defined as the lowest concentration of antibiotic that inhibits visible mycobacterial growth. In this work, MIC test results were interpreted based on CLSI-recommended breakpoints (Parrish, 2023). The abovementioned M. kansasii reference strain was tested along with each batch of isolates as a quality control measure. Drug concentrations that inhibited the growth of tested isolates by 50 and 90% were expressed as MIC50 and MIC90 values, respectively.
Statistical analysis
All statistical analyses were performed using SPSS software, version 24 (IBM, United States). Intergroup difference was deemed statistically significant for results with p < 0.05. Drug MIC distributions for the 153 isolates were analyzed using GraphPad Prism software (version 7.00, La Jolla, CA, United States).
Sequence information
The WGS data has been submitted to the National Center for Biotechnology Information (NCBI) with the Bio project number of PRJNA925290 or submission ID: SUB12542119.
Results
Identification and genotyping
All 153 isolates were ultimately classified as M. kansasii based on ANI values, which indicated that the genome sequence of each isolate shared >95% identity with that of the M. kansasii reference strain ATCC 12478 (Figure 1). The 153 M. kansasii representatives were differentiated into 3 clusters with 141 genotypes, including 17 isolates from a cluster (2–9 isolates per cluster) and 136 isolates with unique patterns (Figure 2). The clustering rate of M. kansasii was 11.1% (17/153).
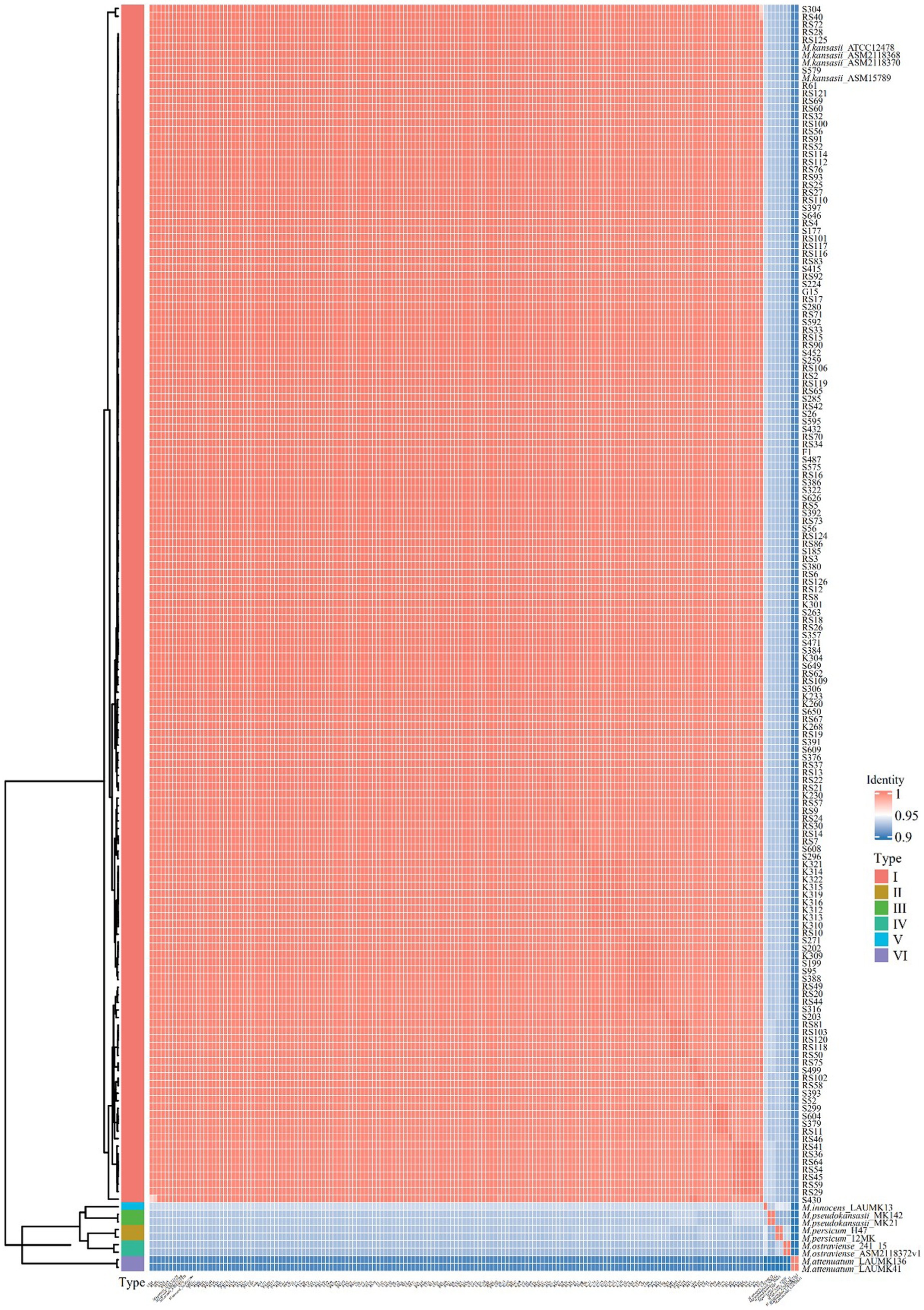
Figure 1. Pairwise comparisons of ANIs of M. kansasii subtypes I-VI. The heatmap displayed the Average Nucleotide Identity (ANI) values among 153 M. kansasii strains and the standard MKC strains. The color gradients in the heatmap represent different intensity levels of values. Red areas indicate higher ANI values, while blue areas signify lower ANI values. The bar chart on the left corresponds to different MKC species and conducts a clustering analysis of the samples.
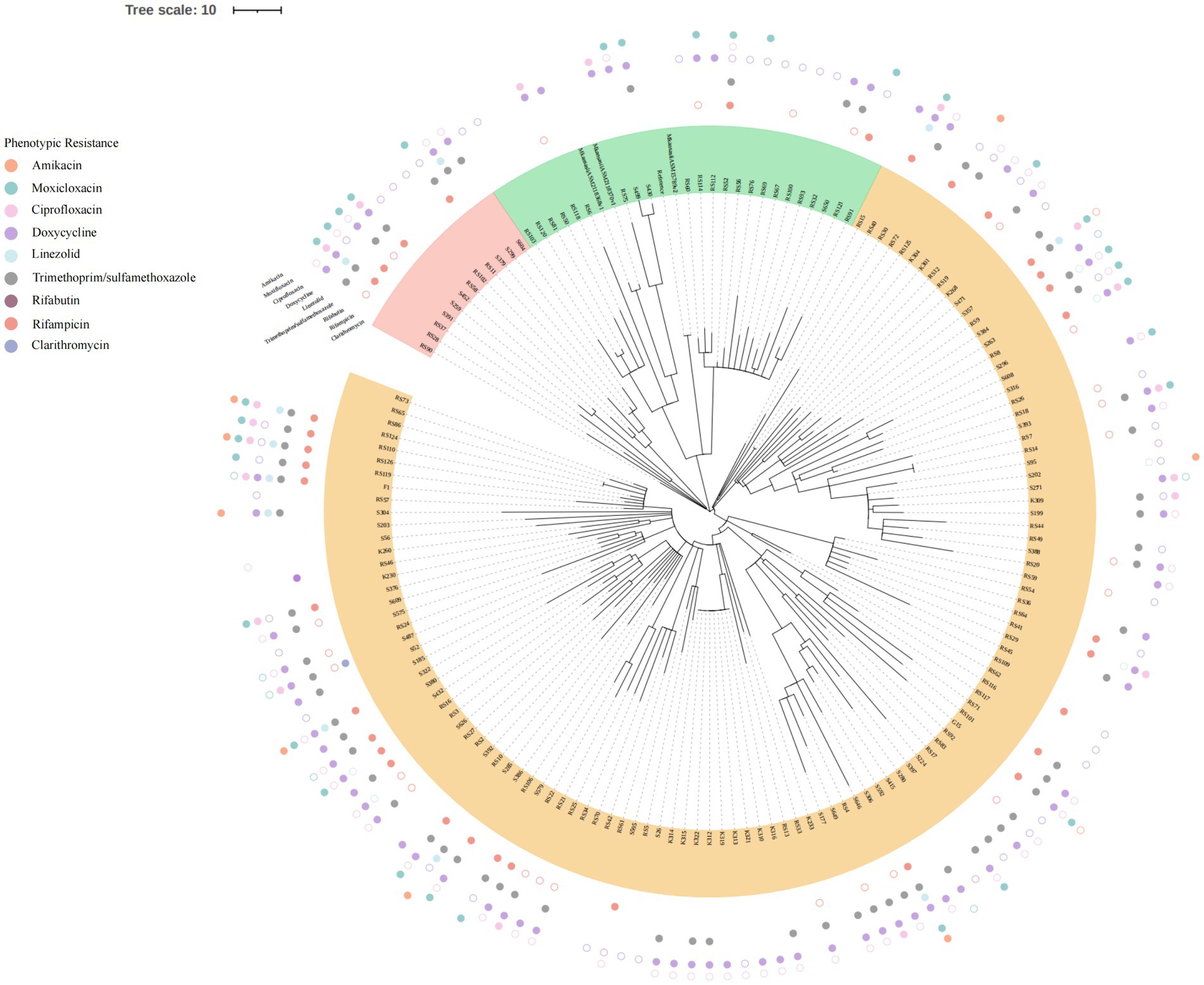
Figure 2. Phylogenetic tree based on core SNP. All 153MKC strains were divided into three clusters, with each cluster represented by a unique color. The outlying circles depicted the outcomes of the drug sensitivity tests. Distinct colors were employed to signify various drugs. Solid circles denoted drug resistance, while hollow circles represented intermedia.
Virulence factor-encoding gene
Referring to the filter threshold of the reference virulence gene, homologous genes with a coverage of 80% and a similarity of 80% were listed in Figure 3. The EXS-1, EXS-3 and EXS-5 regions were involved in all isolates. And fbpA, fbpB, fbpC, hbhA, ideR, mbtH, mgtC, and phoP gene were present in all M. kansasii subtype I strains.
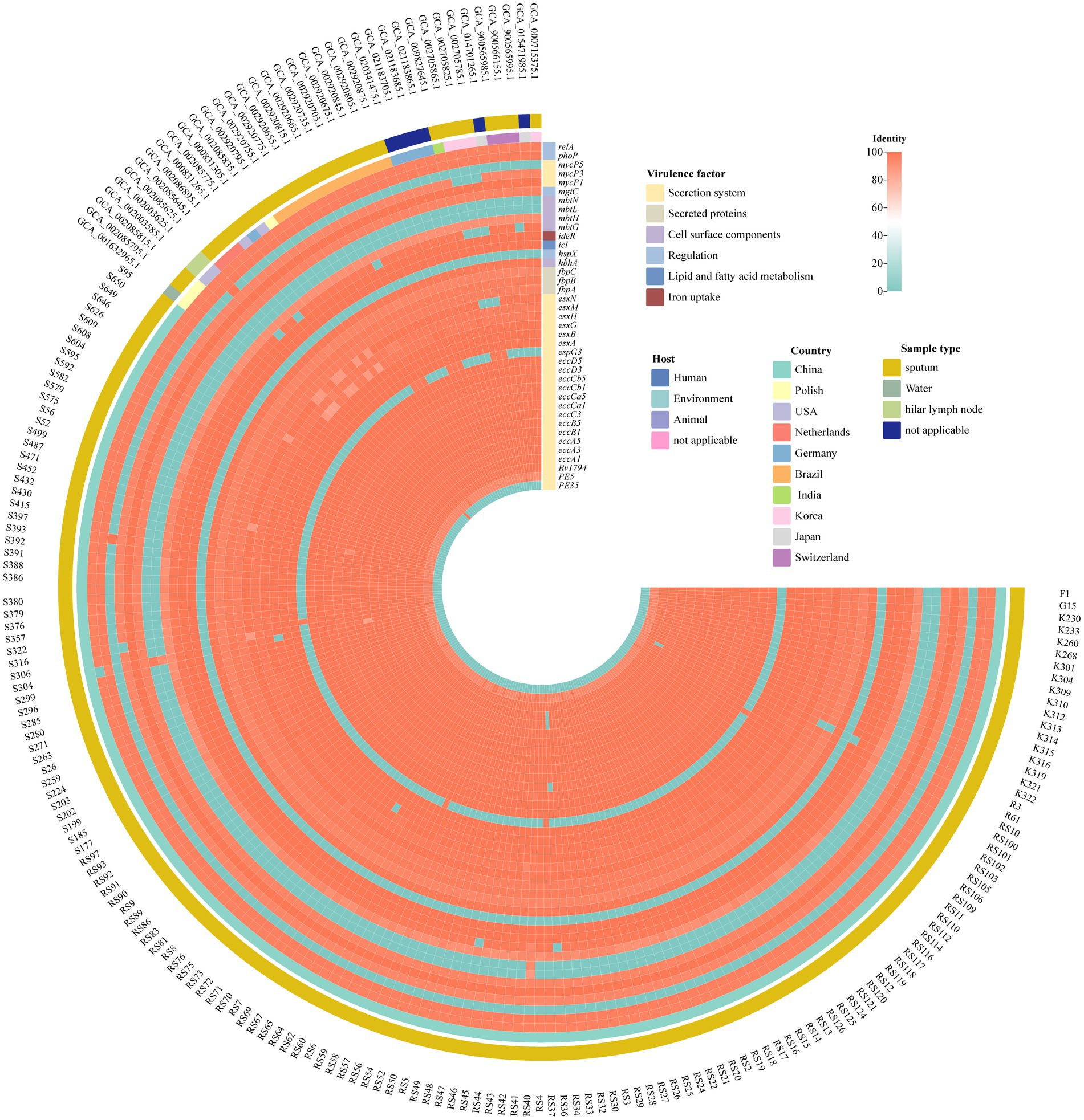
Figure 3. Virulence factors in M. kansasii from different sources. The heatmap displayed the identity of multiple virulence genes. The horizontal axis is labeled with samples while the vertical axis enumerates individual virulence genes. A color—coded gradient is employed to depict the degree of identity among the genes. A score of 100 is represented by red, signifying a high level of sequence similarity, conversely, a light blue color corresponds to an identity score of 0, indicating a lack of significant similarity.
Drug susceptibility testing
For the antimicrobial agents with the breakpoint values recommended by CLSI, rifabutin (RFB) and clarithromycin (CLA) were the most highly active against M. kansasii strains, with the susceptibility rate of 100% and 99.35%, respectively. Followed by amikacin (AMK) and linezolid (LZD), the resistance rate was 5.88% and 7.19%, respectively (Table 1). For the fluoroquinolones, the resistance rate to ciprofloxacin (CIP) and moxifloxacin (MXF) was 12.42% and 20.92%, respectively. Of the 22 isolates resistant to MXF, 10 isolates (45.45%) exhibited resistance to CIP (Supplementary Figure S1). The resistance rate to RIF was 22.22%. As for the antibiotics without breakpoint values, all isolates had very low MIC50 (0.03 μg/mL) and MIC90 (≤0.06 μg/mL) values against bedaquiline (BDQ), sutezolid (SZD), delamanid (DLM), and clofazimin (CFZ). Ethionamide (ETO) was also active against M. kansasii, with MIC50 and MIC90 values of 0.6 μg/mL and 2.5 μg/mL, respectively (Figure 4).
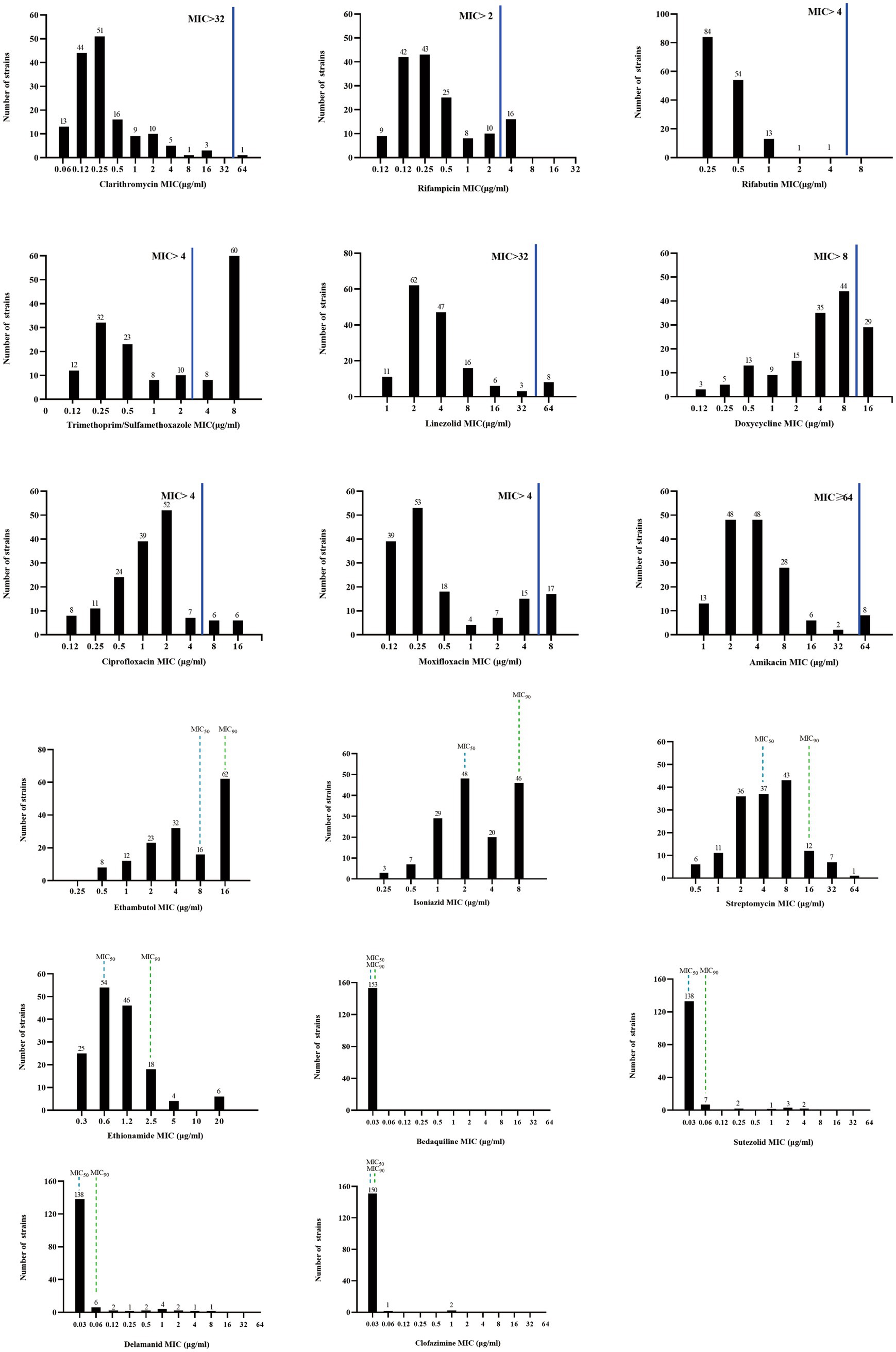
Figure 4. MIC distributions of M. kansasii clinical isolates. The solid blue line indicates the CLSI-recommended threshold for determining drug susceptibility, above which a resistant strain is considered. Drugs lacking critical values of MIC50 and MIC90 are denoted by dashed lines. MIC50: the minimum concentration of an antimicrobial agent required to inhibit the growth of 50% of the organisms. MIC90: the minimum concentration of an antimicrobial agent required to inhibit the growth of 90% of the organisms.
Association between drug resistance profiles and genotypes
Although no statistically significant associations between clusters and drug resistance profiles were observed, CLA, RIF, RFB, TMP/SMX, LZD, DOX, and AMK in cluster 3 were 1.18, 24.71, 1.18, 49.41, 8.24, 50.59, and 8.24% respectively, which exceeded corresponding rates observed for cluster 1 (0, 20, 0, 32.5, 7.5, 45, and 2.5%) and cluster 2 (0, 17.86, 0, 42.86, 0, 46.43, and 3.57%) isolates. Meanwhile, CIP and MXF resistance rates of cluster 3 (10.59 and 15.29%) isolates were lower than corresponding rates obtained for cluster 1 (12.50 and 30%) and cluster 2 (14.29 and 25%) isolates (Table 2).
Discussion
M. kansasii, the most pathogenic subspecies, is responsible for most NTM infections worldwide (Luo et al., 2021; Johnston et al., 2017). Previous studies in China have reported M. kansasii as predominant species, comprising 71.8% of MKC isolates, with 14.1% belonging to M. persicum (Li et al., 2016). In contrast, all MKC isolates analyzed in this study were classified as M. kansasii. The discrepancy may be attributed to the fact that M. persicum is an opportunistic pathogen, which primarily infects immunocompromised individuals, and thus was not detected in our cohort of immunocompetent patients (Taillard et al., 2003). Alternatively, M. kansasii isolates in this study may exhibit greater genetic homogeneity compared to those circulating in other regions of China (Mokrousov, 2017).
Intriguingly, the clustering rate observed in the current study (11%) was substantially lower than those reported in prior investigations (68–99.3%) (Taillard et al., 2003; Zhang et al., 2004; Bakula et al., 2018). This discrepancy may be attributed to methodological differences in typing methods used across studies. Earlier studies predominantly utilized variable number tandem repeat (VNTR)-based assays or pulsed-field gel electrophoresis (PFGE) for MKC typing (Taillard et al., 2003; Zhang et al., 2004; Bakula et al., 2018). However, compared to WGS, these conventional methods are more time-consuming, labor-intensive, resource-intensive, require a higher degree of expertise, and offer significantly lower discriminatory power, highlighting the superior of WGS as a high-throughput and high-resolution genotyping platform (Bakula et al., 2018).
The type-VII secretion system comprises five homologous secretion systems (ESX-1 to ESX-5), which are critical for the pathogenicity of both M. tuberculosis and M. kansasii (Guan et al., 2020). In this study, genes encoding the conserved components of the EXS-1, EXS-3, and EXS-5 system were detected in genomes of all 153 M. kansasii isolates. Additionally, esxG gene, which encodes an ESX-3 substrate known to enhance M. tuberculosis virulence by facilitating iron acquisition (Tufariello et al., 2016), was also universally present. Moreover, the fbpC gene, encoding an enzyme involved in transferring mycolic acid from trehalose monomycolate to the mycolyl-arabinogalactan complex (Favrot et al., 2013), was detected in 99.35% (152/153) of isolates. By contrast, results of another study conducted in China by Guo et al., revealed that the fbpC gene was absent in genomes of all M. kansasii isolates, while the esxG gene was detected in only 6.7% (4/60) of cases (Guo et al., 2022). We hypothesize that these discrepancies may reflect variations in the virulence profiles of M. kansasii strains circulating in different geographic areas.
Core treatment regimens for M. kansasii infections consist of RIF, INH, and EMB (Parrish, 2023). Nevertheless, the clinical utility of INH and EMB is limited, as their MIC values correlate poorly with treatment outcomes, rendering them unreliable prognostic indicators (Parrish, 2023). In this study, the RIF resistance rate was 22.22% (34/153), similar to that reported in Shanghai (20.0%) (Guo et al., 2022), but lower than that observed in Beijing, China (56.4%) (Li et al., 2016). Notably, our resistance rates were higher than those documented in Poland and Brazil, where all tested isolates remained fully susceptible to RIF (Bakuła et al., 2018; de Carvalho et al., 2019). These disparities suggest potential geographical variations in resistance patterns, possibly influenced by regional differences in antibiotic usage and susceptibility profiles (Bakuła et al., 2018). In cases of treatment failure associated with RIF resistance, DST for secondary antimicrobial agents should be conducted to identify effective alternatives and optimize therapeutic outcomes (Parrish, 2023). Consistent with previous findings, all isolates in this study remained susceptible to RFB, reinforcing its potential as an effective substitute for RIF in clinical practice (Guo et al., 2022).
The CLA susceptibility rate for our isolates was 99.35% (152/153), aligning with previous reports of <1% resistance (Guo et al., 2022; de Carvalho et al., 2019). However, another study reported a substantially higher CLA resistance rate (20.5%), possibly due to macrolide overuse driving resistance development (Li et al., 2016). Additionally, AMK and LZD exhibited excellent in vitro activities against M. kansasii, with sensitivity rates exceeding 90%, indicating them to be promising options for M. kansasii infections (Guo et al., 2022; de Carvalho et al., 2019). SZD, a newer oxazolidinone, also exhibited excellent in vitro activity against M. kansasii, consistent with previously reported (Yu et al., 2021; Zheng et al., 2023). Notably, BDQ, DLM, and CFZ, which are newer drugs used to treat multidrug-resistant tuberculosis cases, demonstrated consistent activity against M. kansasii in our study and previous research (Zheng et al., 2022), highlighting their potential as alternative therapies for M. kansasii infections.
This research has several limitations. First, as this retrospective study relied on tuberculosis DRS, the clinical information and treatment history were unavailable for patients diagnosed with M. kansasii pulmonary disease, who were discontinued from follow-up. Second, we did not collect MKC isolates from environmental sources to demonstrate the sources of clinical infections. Third, all M. kansasii isolates were obtained from patients in China and thus the conclusions drawn from an analysis of these isolates may not be generalizable to clinical isolates from other countries.
Conclusion
M. kansasii type I was the predominant genotype in China, and RFB and CLR presented strong activities against M. kansasii strains. Three clusters were separated based on core SNP throughout the genome. The new drugs BDQ, DLM, SZD and CFZ have the potential to be potent agents for the treatment of M. kansasii infection.
Data availability statement
The datasets presented in this study can be found in online repositories. The names of the repository/repositories and accession number(s) can be found in the article/Supplementary material.
Author contributions
YW: Conceptualization, Formal analysis, Methodology, Visualization, Writing – original draft. XO: Investigation, Methodology, Writing – review & editing. BZ: Data curation, Methodology, Supervision, Writing – review & editing. HX: Project administration, Resources, Writing – review & editing. YZhe: Data curation, Software, Writing – review & editing. YZho: Data curation, Resources, Writing – review & editing. RX: Data curation, Methodology, Writing – review & editing. YS: Conceptualization, Investigation, Writing – review & editing. SW: Funding acquisition, Methodology, Writing – review & editing. YZha: Funding acquisition, Resources, Writing – review & editing. HZ: Conceptualization, Formal analysis, Funding acquisition, Writing – review & editing.
Funding
The author(s) declare that financial support was received for the research and/or publication of this article. This work was supported by the National Key R&D Program of China (2022YFC2305204, 2023YFC2307301), Beijing Natural Science Foundation (7224328), China CDC-Tuberculosis Control and Prevention Project (2528), Funding for Reform and Development of Beijing Municipal Health Commission (EYGF-HX-05).
Conflict of interest
The authors declare that the research was conducted in the absence of any commercial or financial relationships that could be construed as a potential conflict of interest.
Generative AI statement
The authors declare that no Gen AI was used in the creation of this manuscript.
Publisher’s note
All claims expressed in this article are solely those of the authors and do not necessarily represent those of their affiliated organizations, or those of the publisher, the editors and the reviewers. Any product that may be evaluated in this article, or claim that may be made by its manufacturer, is not guaranteed or endorsed by the publisher.
Supplementary material
The Supplementary material for this article can be found online at: https://www.frontiersin.org/articles/10.3389/fmicb.2025.1573448/full#supplementary-material
Footnotes
References
Bakula, Z., Brzostek, A., Borowka, P., Zaczek, A., Szulc-Kielbik, I., Podpora, A., et al. (2018). Molecular typing of Mycobacterium kansasii using pulsed-field gel electrophoresis and a newly designed variable-number tandem repeat analysis. Sci. Rep. 8:4462. doi: 10.1038/s41598-018-21562-z
Bakuła, Z., Modrzejewska, M., Pennings, L., Proboszcz, M., Safianowska, A., Bielecki, J., et al. (2018). Drug susceptibility profiling and genetic determinants of drug resistance in Mycobacterium kansasii. Antimicrob. Agents Chemother. 62:e01788-17. doi: 10.1128/AAC.01788-17
Campo, R. E., and Campo, C. E. (1997). Mycobacterium kansasii disease in patients infected with human immunodeficiency virus. Clin. Infect. Dis. 24, 1233–1238. doi: 10.1086/513666
de Carvalho, L. D., de Queiroz Mello, F. C., Redner, P., Campos, C. E. D., de Souza Caldas, P. C., da Silva Lourenco, M. C., et al. (2019). Drug susceptibility profile of Mycobacterium kansasii clinical isolates from Brazil. J. Glob. Antimicrob. Resist. 19, 228–230. doi: 10.1016/j.jgar.2019.05.003
Favrot, L., Grzegorzewicz, A. E., Lajiness, D. H., Marvin, R. K., Boucau, J., Isailovic, D., et al. (2013). Mechanism of inhibition of Mycobacterium tuberculosis antigen 85 by ebselen. Nat. Commun. 4:2748. doi: 10.1038/ncomms3748
Griffith, D. E., Aksamit, T., Brown-Elliott, B. A., Catanzaro, A., Daley, C., Gordin, F., et al. (2007). An official ATS/IDSA statement: diagnosis, treatment, and prevention of nontuberculous mycobacterial diseases. Am. J. Respir. Crit. Care Med. 175, 367–416. doi: 10.1164/rccm.200604-571ST
Gu, Z., Eils, R., and Schlesner, M. (2016). Complex heatmaps reveal patterns and correlations in multidimensional genomic data. Bioinformatics 32, 2847–2849. doi: 10.1093/bioinformatics/btw313
Guan, Q., Ummels, R., Ben-Rached, F., Alzahid, Y., Amini, M. S., Adroub, S. A., et al. (2020). Comparative genomic and transcriptomic analyses of Mycobacterium kansasii subtypes provide new insights into their pathogenicity and taxonomy. Front. Cell. Infect. Microbiol. 10:122. doi: 10.3389/fcimb.2020.00122
Guo, Y., Cao, Y., Liu, H., Yang, J., Wang, W., Wang, B., et al. (2022). Clinical and microbiological characteristics of Mycobacterium kansasii pulmonary infections in China. Microbiol. Spectr. 10:e0147521. doi: 10.1128/spectrum.01475-21
Hoefsloot, W., van Ingen, J., Andrejak, C., Angeby, K., Bauriaud, R., Bemer, P., et al. (2013). The geographic diversity of nontuberculous mycobacteria isolated from pulmonary samples: an NTM-NET collaborative study. Eur. Respir. J. 42, 1604–1613. doi: 10.1183/09031936.00149212
Iwamoto, T., and Saito, H. (2006). Comparative study of two typing methods, hsp65 PRA and ITS sequencing, revealed a possible evolutionary link between Mycobacterium kansasii type I and II isolates. FEMS Microbiol. Lett. 254, 129–133. doi: 10.1111/j.1574-6968.2005.00013.x
Jagielski, T., Borówka, P., Bakuła, Z., Lach, J., Marciniak, B., and Brzostek, A. (2020). et al, Genomic insights into the Mycobacterium kansasii complex: an update. Front. Microbiol. 10:2918. doi: 10.3389/fmicb.2019.02918
Johnston, J. C., Chiang, L., and Elwood, K. (2017). Mycobacterium kansasii. Microbiol. Spectr. 5:5. doi: 10.1128/microbiolspec.TNMI7-0011-2016
Kigozi, E., Kasule, G. W., Musisi, K., Lukoye, D., Kyobe, S., Katabazi, F. A., et al. (2018). Prevalence and patterns of rifampicin and isoniazid resistance conferring mutations in Mycobacterium tuberculosis isolates from Uganda. PLoS One 13:e0198091. doi: 10.1371/journal.pone.0198091
Kim, Y. G., Lee, H. Y., Kwak, N., Park, J. H., Kim, T. S., Kim, M. J., et al. (2021). Determination of clinical characteristics of Mycobacterium kansasii-derived species by reanalysis of isolates formerly reported as M. kansasii. Ann. Lab. Med. 41, 463–468. doi: 10.3343/alm.2021.41.5.463
Kim, M., Oh, H. S., Park, S. C., and Chun, J. (2014). Towards a taxonomic coherence between average nucleotide identity and 16S rRNA gene sequence similarity for species demarcation of prokaryotes. Int. J. Syst. Evol. Micr. 64, 346–351. doi: 10.1099/ijs.0.059774-0
Letunic, I., and Bork, P. (2011). Interactive tree of life v2: online annotation and display of phylogenetic trees made easy. Nucleic Acids Res. 39, W475–W478. doi: 10.1093/nar/gkr201
Li, Y., Pang, Y., Tong, X., Zheng, H., Zhao, Y., and Wang, C. (2016). Mycobacterium kansasii subtype I is associated with clarithromycin resistance in China. Front. Microbiol. 7:2097. doi: 10.3389/fmicb.2016.02097
Luo, T., Xu, P., Zhang, Y., Porter, J. L., Ghanem, M., Liu, Q., et al. (2021). Population genomics provides insights into the evolution and adaptation to humans of the waterborne pathogen Mycobacterium kansasii. Nat. Commun. 12:2491. doi: 10.1038/s41467-021-22760-6
Minh, B. Q., Schmidt, H. A., Chernomor, O., Schrempf, D., Woodhams, M. D., von Haeseler, A., et al. (2020). IQ-TREE 2: new models and efficient methods for phylogenetic inference in the genomic era. Mol. Biol. Evol. 37, 1530–1534. doi: 10.1093/molbev/msaa015
Mokrousov, I. (2017). Revisiting the hunter Gaston discriminatory index: note of caution and courses of change. Tuberculosis 104, 20–23. doi: 10.1016/j.tube.2017.02.002
Parrish, N. M. (2023). Susceptibility testing of mycobacteria, Nocardia spp., and other aerobic actinomycetes. USA: Clinical and Laboratory Standards Institute.
Pintado, V., Gomez-Mampaso, E., Martin-Davila, P., Cobo, J., Navas, E., Quereda, C., et al. (1999). Mycobacterium kansasii infection in patients infected with the human immunodeficiency virus. Eur. J. Clin. Microbiol. Infect. Dis. 18, 582–586. doi: 10.1007/s100960050351
Raju, R. M., Raju, S. M., Zhao, Y., and Rubin, E. J. (2016). Leveraging advances in tuberculosis diagnosis and treatment to address nontuberculous mycobacterial disease. Emerg. Infect. Dis. 22, 365–369. doi: 10.3201/eid2203.151643
Tagini, F., Aeby, S., Bertelli, C., Droz, S., Casanova, C., Prod'hom, G., et al. (2019). Phylogenomics reveal that Mycobacterium kansasii subtypes are species-level lineages. Description of Mycobacterium pseudokansasii sp. nov., Mycobacterium innocens sp. nov. and Mycobacterium attenuatum sp. nov. Int. J. Syst. Evol. Microbiol. 69, 1696–1704. doi: 10.1099/ijsem.0.003378
Taillard, C., Greub, G., Weber, R., Pfyffer, G. E., Bodmer, T., Zimmerli, S., et al. (2003). Clinical implications of Mycobacterium kansasii species heterogeneity: Swiss National Survey. J. Clin. Microbiol. 41, 1240–1244. doi: 10.1128/JCM.41.3.1240-1244.2003
Tufariello, J. M., Chapman, J. R., Kerantzas, C. A., Wong, K. W., Vilcheze, C., Jones, C. M., et al. (2016). Separable roles for Mycobacterium tuberculosis ESX-3 effectors in iron acquisition and virulence. Proc. Natl. Acad. Sci. USA 113, E348–E357. doi: 10.1073/pnas.1523321113
Yu, X., Huo, F., Wang, F., Wen, S., Jiang, G., Xue, Y., et al. (2021). In vitro antimicrobial activity comparison of linezolid, Tedizolid, Sutezolid and Delpazolid against slowly growing mycobacteria isolated in Beijing, China. Infect. Drug. Resist. 14, 4689–4697. doi: 10.2147/IDR.S332835
Zhang, Y., Mann, L. B., Wilson, R. W., Brown-Elliott, B. A., Vincent, V., Iinuma, Y., et al. (2004). Molecular analysis of Mycobacterium kansasii isolates from the United States. J. Clin. Microbiol. 42, 119–125. doi: 10.1128/JCM.42.1.119-125.2004
Zheng, H., Wang, Y., He, W., Li, F., Xia, H., Xiao, J., et al. (2023). Letter to the editor: in vitro activity of Oxazolidinone against nontuberculous mycobacteria in China. Microb. Drug Resist. 29, 112–114. doi: 10.1089/mdr.2022.0216
Keywords: Mycobacterium kansasii , drug susceptibility testing, genotype, whole genome sequencing (WGS), phylogenetic analysis
Citation: Wang Y, Ou X, Zhao B, Xia H, Zheng Y, Zhou Y, Xing R, Song Y, Wang S, Zhao Y and Zheng H (2025) Genomic-based genotype and drug susceptibility profile of Mycobacterium kansasii in China. Front. Microbiol. 16:1573448. doi: 10.3389/fmicb.2025.1573448
Edited by:
Samira Tarashi, Pasteur Institute of Iran (PII), IranReviewed by:
Muzafar Ahmad Rather, University of Minnesota Twin Cities, United StatesAnwar Alam, Sharda University, India
Denis Lagutkin, Karolinska Institutet (KI), Sweden
Copyright © 2025 Wang, Ou, Zhao, Xia, Zheng, Zhou, Xing, Song, Wang, Zhao and Zheng. This is an open-access article distributed under the terms of the Creative Commons Attribution License (CC BY). The use, distribution or reproduction in other forums is permitted, provided the original author(s) and the copyright owner(s) are credited and that the original publication in this journal is cited, in accordance with accepted academic practice. No use, distribution or reproduction is permitted which does not comply with these terms.
*Correspondence: Yanlin Zhao, emhhb3lsQGNoaW5hY2RjLmNu; Huiwen Zheng, aGFuaHVpODIwQDEyNi5jb20=